- International Livestock Research Institute (ILRI), Nairobi, Kenya
Feminisation of agriculture’ is generally utilized to indicate an expansion of women’s engagement in agricultural production, as labourers or decision-makers. Feminization of Agriculture is often reported as a global trend. While literature on feminization of agriculture (FoA) has seen a steady rise in the last decade, there is little consensus on defining feminization of agriculture and consistent approaches that provide comparative quantitative data on FoA are lacking. This compromises the ability to provide a comparative understanding of the extent of feminization across various regions. In this paper, we develop a methodological approach to assessing the extent of FoA and deploying it on DHS data from South and South-East Asia. Our data show that in Cambodia, India, Indonesia and Nepal the trend has been towards a Masculinization of Agriculture between 2005 and 2017, while no trend could be determined for the Philippines. We discuss the implications of our results while highlighting some of the limitations of our approach and suggesting possible next research steps.
1. Introduction
While literature on feminization of agriculture (FoA) has seen a steady rise in the last decade, there is little consensus on defining feminization of agriculture and a comparative understanding of the extent of feminization across various regions. ‘Feminisation of agriculture’ is generally utilized to indicate an expansion of women’s engagement (or increased visibility of their engagement) in agricultural production, as labourers or decision-makers. Research on FoA has focused on 1. characterizing the increasing role of women in the agricultural workforce and their capabilities to undertake such role (Tavenner et al., 2019; de Brauw et al., 2021); 2. the drivers behind these changes in women’s role and whether they had a say about their involvement (Doss et al., 2021); 3. whether the change in agricultural labour involvement was commensurate with changes in their decision-making about agriculture (Abdelali-Martini et al., 2010) and; 4. outcomes for women of such increased roles in agriculture (Slavchevska et al., 2016; Khatri-Chhetri and Chanana, 2017).
Doss et al. (2021) and Kawarazuka et al. (2022) discuss the complexity of phenomena that the term FoA may entail and the need to appreciate the gendered processes of rural transformation behind it. Understanding changes in the agricultural labour force, its characteristics, needs, constraints and opportunities, can help better target the development of institutional and technological innovations, and of policies so that gendered values and priorities in agriculture are considered. However, the diversity of definitions and methodologies adopted to study FoA may limit the potential to explore the phenomenon, its complexity, and its connotations in a national or regional context.
Farnworth et al. (2023) discuss how various FoA definitions and methodologies are shaping the evidence produced on FoA. Overall, two methodological shortcomings emerge from the literature on FoA. One is about the need for more comprehensive measures to capture the complex dynamics behind “feminization of agriculture” (Kawarazuka et al., 2022). The other is the need for more systematic approaches to assess FoA given that the diversity in the methodology, indicators, scope and study designs used to understand women’s involvement in agriculture makes cross-country comparisons difficult (Slavchevska et al., 2016). Such cross-country evidence would help understand, for example, how globalization is affecting national patterns of agricultural labour and develop regional and global level strategies to strengthen women’s opportunities for paid, dignified work in agricultural labour markets in various regions.
The limitations of disparate approaches to studying FoA is evident, for example, in cross-country comparisons of studies on FoA that focus on increased workload. These are undermined by the different definitions of “work” across national surveys conducted in different countries (Sen et al., 2019). Another challenge that impedes a broad cross-regional understanding of women’s involvement is the complexity and diversity of intra-household labour and decision-making arrangements in various cropping systems and regions (CIT).
Evidence on FoA from South-East Asia is relatively limited and mostly focused on local processes of change, with little evidence on regional trends. These studies mostly point to two aspects associated with FoA: women are increasingly taking on agricultural tasks that were traditionally performed by men as the men move to the cities to look for paid work; and women’s agricultural work is underpaid as compared to men’s.
de Brauw et al. (2021) and Salve Bacud et al. (2019) working in Bangladesh and Viet Nam, respectively, found increasing opportunities in non-agriculture work in urban areas leads to the outmigration of male household members from rural to urban areas - causing an increased agricultural labour burden among women. Women are taking up additional tasks such as, seedbed and land preparation, fertilizer application and pesticide spraying, which have been traditionally dominated by men. The authors also report that the wage gap between men and women continues to persist with men labourers earning more than their female counterparts – a gender-based disadvantage that is even more pronounced with FoA (de Brauw et al., 2021). Salve Bacud et al. (2019) add that the number of salaried women working on rice farms in Viet Nam is also decreasing as more women opt to work on family farms. Because this process of FoA is causing decreasing rice yields – which make farming less profitable – and is characterized by low female wages or unpaid work on family farms, the authors conclude that FoA is a curse to the women of the two countries rather than an empowering phenomenon.
In Nepal, Farnworth et al. (2019) argue that FoA can be empowering if women can receive support from the government, society, families and most importantly, their husbands. In their study, they found that despite wheat farming in Nepal getting feminized, women still do not have control over the farm assets and do not have access to financial services. Also, women are not viewed as farmers by extension officers and hence are not included in capacity development initiatives or in projects to develop agricultural innovations. Still, they are expected to adopt innovations such big machinery and hybrid crops developed for male users.1 Despite these challenges, Farnworth et al. (2019) point out the success of some women who operate outside the official agricultural organizations and have become leaders in wheat farming. This happened to women who were encouraged by their partners, in-laws, and extension workers to become innovative on their farms. Successful women innovators attract respect from their in-laws and husbands who in turn encourage the women to make more decisions.
From the above, we see that Vietnam, Bangladesh and Nepal, countries within the south and southeast Asia region, are experiencing similar impacts of FoA although the processes of change behind it may differ. While evidence on local FoA processes is important to appreciate the complexity and diversity of rural changes associated with FoA and gender equality, analyzing such data within a regional context may be needed for developing effective policy interventions. Such evidence can help governments formulate policies with a regional relevance (e.g., cross-border migration, labour, trade or agricultural policies) that intentionally support the empowerment of women in agriculture and progress towards both agricultural development and gender equality.
In this study, we focus on nationally representative data in South and South-East Asia to show the FoA trends in the region. To this aim, we have undertaken an explorative analysis and mapping of countries that are reported to be experiencing FoA in these regions using a common definition, methodology and the same indicators. We adopt a definition of FoA focused on increased women’s labour involvement in agriculture vis-à-vis men’s involvement over time (we call the opposite trend ‘Masculinization of Agriculture’ (MoA)). We assess the intra-household allocation of labour in agricultural households using indicators from the Demographic and Health Surveys (DHS Program (RRID:SCR_000905)), nationally representative surveys designed to collect data on monitoring and impact evaluation indicators important for individual countries and cross-country comparisons (Basu and Koolwal, 2005; Kazembe, 2020). DHS surveys are implemented using standard definitions, codes and variables which enable an easy cross-country comparison.
This paper, therefore, provides a methodology to assess national trends in FoA or MoA that allows regional comparisons. We provide data to show the trends towards FoA or MoA in 6 countries of South and South-East Asia and identify some factors which might be contributing to these trends. This paper is organized as follows: in the next section we describe in detail the methodology that we adopted. We then show the results of our analysis of women’s and men’s involvement in agriculture in South and South-East Asia, identify some potential drivers of involvement and determine feminization or masculinization trends. In the final section, we discuss these results as well as the limitations of our approach in relation to the existing literature on the topic.
2. Methodology
Our approach to determining whether a country is characterized by Feminization or Masculinization of Agriculture is based on large national surveys which were repeated over several years. The resulting datasets indicate whether the interviewed adult woman in the household and her partner are engaged in agricultural or non-agricultural activities. The change in the proportion of women in agriculture over the years is compared to the change in the proportion of men. A higher increase in the proportion of women in agriculture over the years compared to a change in the men’s proportion would be associated with FoA. The opposite trend would characterize a MoA.
In order to determine trends in occupation, we used data collected within the framework of Demographic and Health Surveys (DHS) Program, implemented repeatedly with the support of USAID by over 90 countries. Currently over 400 such nationally representative surveys have been conducted. This study is based on DHS data from the following five South and South-East Asia countries, for which data from at least two survey rounds were available: Bangladesh, India, Nepal, Cambodia, Indonesia and the Philippines. The women’s survey data, contained in the main individual file and downloaded on 18th August 2021, had been obtained by interviewing women of ages 15 to 49 years. A report is published by each country for each survey round, outlining the specifics of the sampling and data collection approaches. These reports can be obtained from the DHS Program website.2 The occupation response was standardized under recode 5, therefore, eligible surveys for our analysis were under recode 5 to 7. To target only rural households for our analysis, we filtered the data to include only responses from women living in rural areas. Finally, we excluded single-headed households by considering only responses from married women to be able to compare the changes in the agricultural engagement of women and men (see Table 1 for the number of records per country and survey round, and the discussion section).
We then selected variables that explained women’s and their partner’s occupations, the women’s sample weights, and drivers that have been reported to influence choices in occupation for both women and their partners. The selected drivers are shown in Table 2. From the occupation variables we created dummy variables (woman_occupation and partner_ occupation) to simplify the women and partner occupation variables with the following coding:- Not working for those who reported “Did not work” - Non-agricultural worker for those who reported being in “Professional/technical/managerial,” “Clerical,” “Sales,” “Household and domestic,” “Services,” “Skilled manual,” and “Unskilled manual” – Agricultural worker for those who reported being in “Agricultural – self-employed” and “Agricultural – employee.” Those whose occupations were classified as “Do not know,” “Missing” and “Not applicable” were replaced by NAs. Using the women’s sample weights we computed weighted proportions of each new classification dropping the NAs. And then compared the proportions by country and survey rounds. We also compared the involvement of men and women in agriculture over the years.
However, the survey data used in this study only included information on the time spent on agricultural activities by the woman and not the partner. Therefore, we could only determine gendered involvement by ‘who is involved in agricultural activities’, and not by ‘to what extent is this person involved in agricultural activities’. Hence, our analysis focuses only on households in which only the woman or only the man is involved in agriculture. The change in their share of all rural dual-headed households is interpreted as the indicator of changes in women’s and men’s involvement in agriculture. Households in which both women and men are involved in agriculture are not considered because their individual contributions to agricultural activities could not be quantified.
We therefore created new binary variables woman_agri (1 – woman only in agriculture; 0 – both in agriculture or woman in non-agriculture) and man_agri (1 – man only in agriculture; 0 – both in agriculture or man in non-agriculture) to identify households in which only the woman and only the man are in agriculture, respectively. Subsequently we computed proportions amongst rural married households for these two variables, weighted within countries to compensate for sampling imbalances, and compared across countries and survey rounds as initial descriptive indications of prevailing trends.
To investigate whether there were any recurring associations between household and individual characteristics and gendered engagement in agriculture within the overall dataset that could hint at possible ‘drivers’ behind these phenomena, we developed a logistical regression model for these two dependent variables (woman_agri and man_agri). A logistical model is appropriate for binary dependent variables and multi-level model considers a nested data structure; within the DHS data survey rounds are nested within countries. The household and individual characteristics, included in the model as fixed variables, were “wealth index,” “woman education,” “partner education,” and “partners living together”; shown in Table 2 below. The categorical wealth index, provided with the survey data, was transformed at the household level by subtracting its values from the overall mean wealth index of the five countries considered in this study. We considered the woman’s and partner’s education levels as numeric variables with higher values signifying higher education levels. We converted the variable “currently residing with husband/ partner” into a binary variable with Yes for “living with her” and No for “staying elsewhere.” Missing values were dropped in the regressions.
To determine if agriculture in a country is feminizing or masculinizing, the main objective of this paper, we computed the difference in the proportions of woman_agri and man_agri between the first and the last survey round for each country. The changes in man_agri proportions obtained were then subtracted from the changes in woman_agri proportions by country. Where the results were positive, i.e., the change in woman_agri households was greater than the change in man_agri households, the country was classified as experiencing feminization of agriculture; if the results were negative the country’s agriculture was masculinizing.
Subsequently, we verified the observed trends through two null multi-level logistic regressions, with the woman_agri and the man_agri variables as dependent variables. The models included each country x survey round combination as a random effect, but no variables representing individual or household characteristics. These regressions considered all survey rounds rather than only the first and last rounds, as in the previous analysis investigating trends. We first determined the probabilities of achieving a 1 for the two variables (being a “woman only in agriculture” or being a “man only in agriculture” household), calculated from the regression intercepts [exp (intercept)]/[1 + exp (intercept)]. Then we compared how the probabilities changed by country and survey round. If the difference in the probabilities of woman_agri being 1 and that of man_agri being 1 increased in successive years, then a country would be confirmed as feminizing.
Finally, we examined how much country and survey round influenced the probabilities of achieving a 1 value for the two variables (woman_agri and man_agri) by computing the Variance Partition Coefficient (VPC). VPC represents the proportion of the total observed individual (household in our case) variation in the outcome that is attributable to between-cluster (country and survey round) variation. We used R to carry out the analysis in this study.
3. Results
We included two datasets for India, and three each for Nepal, Cambodia, Indonesia, and the Philippines. Table 1 below shows a summary of the downloaded data for each country and year. The proportion of married women in the rural areas included in this study ranged from 94% in Indonesia (2007) to 50% in Nepal (2017).
3.1. Identifying the occupation of respondents
3.1.1. Women’s occupation
To gain an initial insight into the work activities of women and men, we calculated the proportion of those involved in agriculture, those involved in non-agriculture economic activities and of those not working, across the selected countries and survey rounds (Figures 1, 2).
All countries experienced a shift in women’s economic activities which varied from country to country (see Figure 1 below). In the last round of surveys, the proportions of women engaged in agriculture were lower than those in the first round of surveys across all countries. Women who reported not being involved in any economic activity (shown as “not working” in Figure 1) were increasing in Cambodia, India, and Nepal. The trend is not clear in Indonesia and the Philippines, although the proportions of those not working remained high in these countries. The proportion of women engaged in non-agricultural economic activities increased in the Philippines between 2008 and 2017; it increased in Indonesia and Nepal between the first and second survey round and decreased in the third survey round; it decreased in Cambodia during the second survey; it remained stable in India.
3.1.2. Partner occupation
Similarly, we calculated the proportion of the respondents’ partners involved in agriculture, those involved in non-agriculture economic activities and those not working, across the countries and over the survey rounds. Overall, the partners of surveyed women were found to be involved in both agriculture and non-agriculture economic activities across all countries and over the years. In Cambodia, Nepal, and the Philippines the proportion of partners in non-agricultural activities increased between the first and the last rounds of surveys, while the proportion of those in agriculture decreased. The opposite is true for India and Indonesia. The proportion of partners not working remained very low compared to women. These are shown in Figure 2 below.
Figure 3 focuses only on agriculture, and on comparing the proportion of women and their spouses involved in agriculture, whether alone or together with their partner, in each country and survey round.
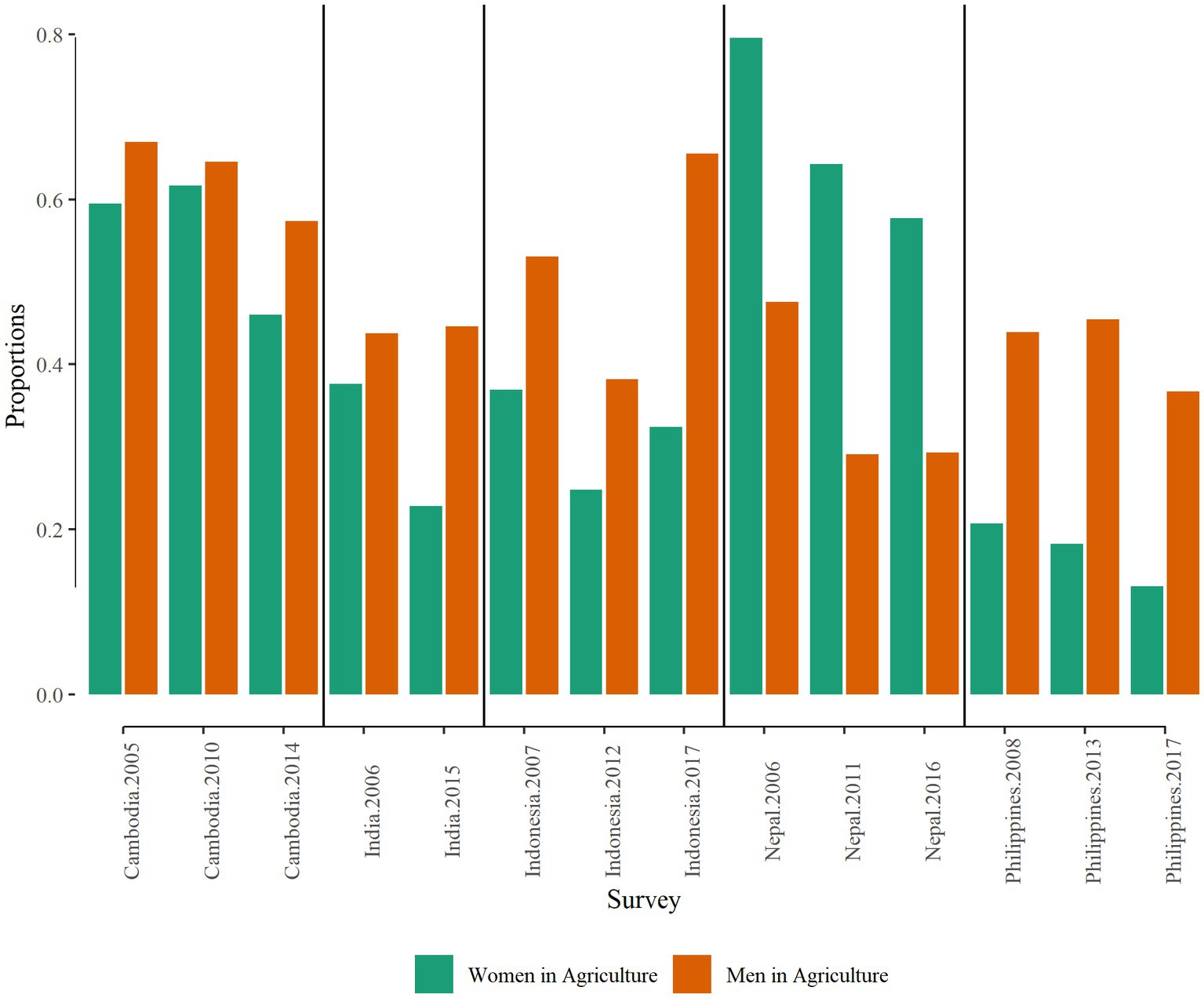
Figure 3. Overview of the total proportion of women and men in agriculture by country and survey round.
3.1.3. Woman-and man-only agriculture
We then focused on households in which one adult only was engaged in agriculture in order to be able to assign agricultural activities to either the woman or the man. In the available data, it is not possible to determine the contribution of women and men in households in which both genders are active in agriculture (see Methodology). The proportions of households with women only in agriculture (woman_agri) and of those with men only in agriculture (man_agri) in each country and each survey year are presented in Figure 4.
Nepal has the highest proportion of households where women only are in agriculture compared to households where men only are in agriculture over the survey years, even though, the proportion of households where men only are in agriculture is slowly rising. The proportion of households with women only in agriculture is on the decline in India, Indonesia, and the Philippines, while Cambodia is characterized by a rise followed by a decline. Apart from Nepal and India, there is no other country that is showing a clear trend in the proportion of households with men only in agriculture (see Figure 4). Such changes in the proportion of households with women only or men only are in agriculture can be due to for instance married men getting out of agriculture completely and married women taking over all agricultural activities.
3.2. Drivers
We then looked at household characteristics (couples living together and household wealth index) and individual characteristics (woman and partner education) that could be contributing to a household deciding on who is to remain in agriculture using a multilevel logistic regression. Table 3 under model 2 shows the regression coefficients.
Holding all other variables constant, an increase in wealth index reduces the likelihood of both the woman only and the man only in agriculture. This means that the richer the household the more likely it is that the couple are either both engaged in agriculture or not at all. However, the effect is weaker for man only in agriculture compared to woman only in agriculture. Woman’s education, partner education and partners living together have opposite effects in the two models. Higher woman’s education reduces the likelihood of a household with only the woman in agriculture by 25% while it increases the likelihood of a household with only the man in agriculture by 9%. On the other hand, higher man education level increases the likelihood of a household with only the woman in agriculture by 22% but reduces the likelihood of getting a household with only the man in agriculture by 16%. Living together reduced the likelihood of a household with only the woman in agriculture by 70% but increased the likelihood of a household with only the man in agriculture by 184%. This means that in households where partners live together, more women will drop out of agriculture compared to households where men have out migrated. Also, in rural households where the partners live together, more men are likely to be involved in agricultural activities compared to households where men have out migrated.
3.3. Feminization or masculinization of agriculture
In this study we define feminization or masculinization of agriculture as the difference in trends of woman-only and man-only agriculture. Figure 5 shows these trends, considering the first and the last survey rounds for each country, and the differences between these trends (change in woman only in agriculture minus change in man only in agriculture). These results indicate that all countries are experiencing masculinization of agriculture, with Indonesia having the highest difference in its trends followed by India, then Cambodia and Nepal. In the Philippines masculinization of agriculture appears to be occurring the least. In no country can we determine a greater increase in woman-only-agriculture than in man-only-agriculture, which would indicate feminization.
These results contrast with the common perception of women assuming a greater role in agriculture in the study region. Therefore, to further verify our results, we investigated the association of gendered agriculture with time and country variables through a multilevel logistic regression for both woman only in agriculture and man only in agriculture situations, with only the random effects of country and survey round as independent variables. The overall probability of a household being a “woman only in agriculture” household is lower (10.0%) was lower than that of being a “man only in agriculture in agriculture” (17.1%). However, both probabilities were lower than the probability of not having either only man or woman in agriculture (i.e., having both woman and man working in agriculture or both in non-agriculture). The probabilities varied considerably across country and survey rounds as shown in the caterpillar plot in Figure 6. This shows how the likelihoods (representing the intercepts of the regressions) of either having a woman only or man only in agriculture varied across countries and survey rounds. Positive values indicate that the probability is higher than the overall estimated value and vice versa. Figure 6 shows the variation of the probabilities in the null model for households where women only were in agriculture as hollow circles and where men only are in agriculture as filled circles. It shows a projection of the representativeness of survey data in the wider population (while Figure 4 presents the actual survey data). The likelihood of households where only the woman is in agriculture decreased with consecutive survey rounds in India, Indonesia and Cambodia, while the change was insignificant in the Philippines and Nepal. Nepal also had the highest probability of having a household where only the woman is in agriculture compared to the other countries. In comparison, the likelihood that men only are in agriculture reduced and then increased with more recent surveys for Nepal, Cambodia, and Indonesia, while it increased for India over the two survey rounds conducted there. The man-only likelihoods in this model did not differ statistically across the available survey rounds for the Philippines. The results of these multilevel logistic regressions confirm the observed trends shown in Figure 5 – which are based on weighted proportions.
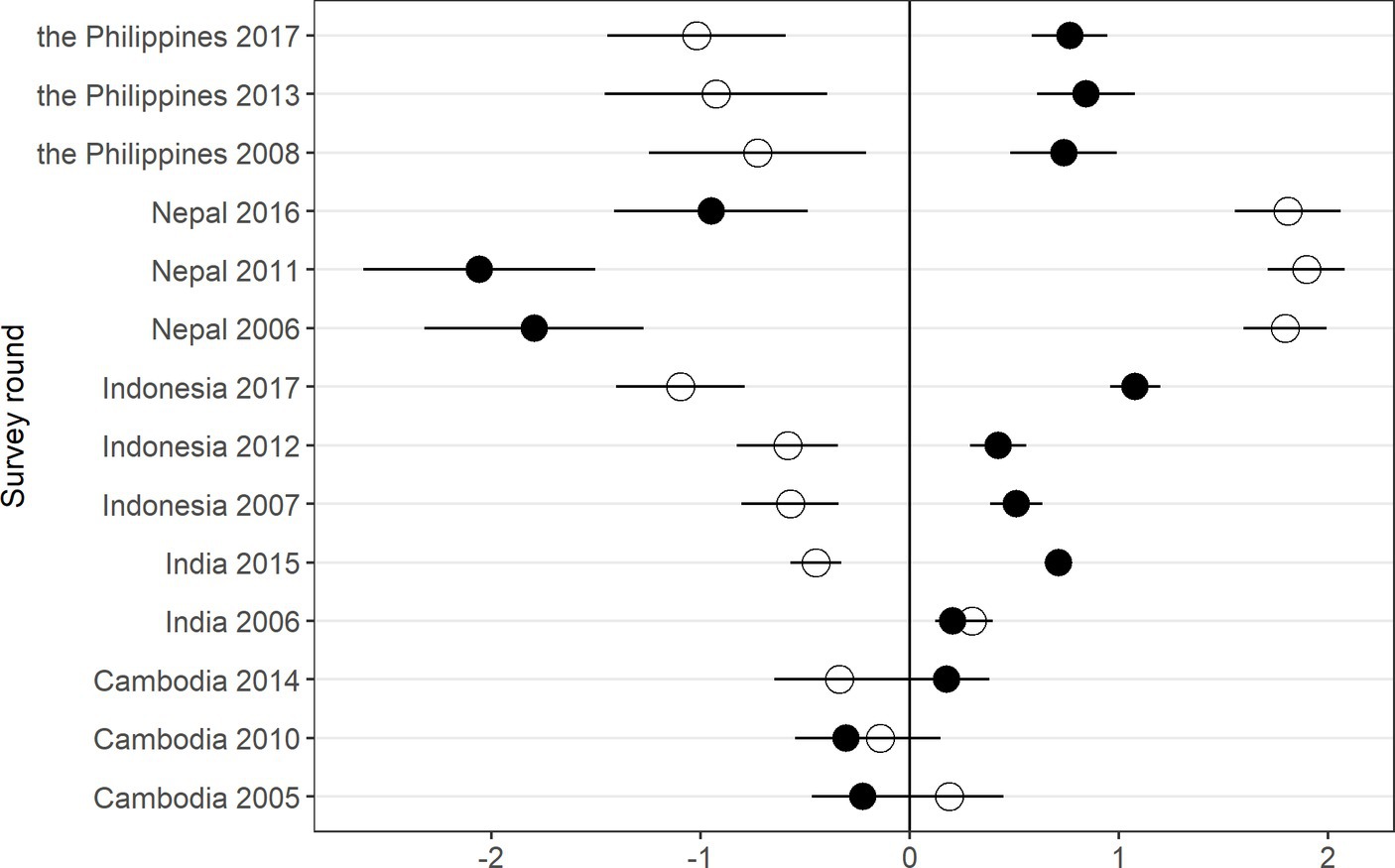
Figure 6. Random effects of the null model where women only are in agriculture are shown by hollow circles and men only in agriculture are shown by filled circles by country and survey round.
The Variance Partition Coefficient (VPC) of the logistic regression models shows the interclass variation (i.e., between country x survey round classes). Accordingly, in the model with fixed household and individual characteristics (determining drivers) these variables explain 18.3 and 18.9% of the variation in the probabilities of a household with the woman only in agriculture and man only in agriculture, respectively. The variation explained by interclass variation increased to 25 and 22.1% for woman only in agriculture and man only in agriculture, respectively, in the null multilevel logistic regression (investigating country and survey round variation without household and individual characteristics). The VPC values range from 0, where there is no within class homogeneity, to 100%, where all households within country X survey round are homogenous. Thus, the VPC results highlight the considerable differences between countries and survey rounds within the studied region in regard to household labour allocation patterns.
4. Discussion
This study demonstrates that changes are occurring in labour allocations at national levels in South and South-East Asia. We see a general decline in the proportion of households where only the women are in agriculture. However, the trend in households where men only are in agriculture was not linear as it was characterized by an increase and decrease in the weighted sample proportions. The non-linear trend was also confirmed by the result of the man only in agriculture null multilevel logistic regression shown in Figure 6. The changes in labour allocation are also captured in the results of VPC which are significantly above zero. This means that labour allocation on either agricultural or non-agricultural activity depends on political boundaries and changes with time as captured by country and survey rounds, respectively.
But based on the definition of femininization of agriculture we adopted, i.e., the increase of women’s involvement in agricultural activities vis-à-vis men’s over the years, we see a general trend in South and South-East Asia towards masculinization of agriculture. This is because women’s involvement in agriculture continues to decline steadily (excluding Nepal and the Philippines) as opposed to men’s involvement which is characterized with alternating periods of increase and decrease over the years. Such changes in men’s and women’s involvement in agriculture over the years also show that MoA or FoA may not represent long-term and steady trends but, rather, frequent changes in agricultural labour allocation (affected by changing circumstances – see section on drivers).
This finding is contrary to the general notion that feminization of agriculture is the predominant global trend. This study shows that within the study region, masculinization of agriculture is occurring at a national level and across the region. This trend is present also in countries where agriculture is mostly in the hands of women, like in Nepal. Even though agriculture in Nepal appears to be highly feminized, there is an increase in households where men choose to engage in agricultural activities while women opt for other non-agricultural activities or decide not to be involved in any economic activity, thus driving masculinization of agriculture in the country. This is in line with the work of Kawarazuka et al. (2022), challenging the myth that feminization of agriculture is the predominant global trend.
However, two important factors need to be taken into account when interpreting our results. One is about the methodology that we adopted. The study focused only on married women living in rural areas (see Methodology). Therefore, it ignored single-headed households and agricultural activities carried out within urban areas. Of all the women surveyed, the study dropped more than half who did not meet the selection criteria. The results, therefore, might not be a true representation of the population. Also, they may indicate that, if FoA is indeed occurring in some of these countries, it would mostly involve an increase in the number of single women in agriculture. A possible way to address this issue is to broaden the definition of feminization, considering the overall involvement of women and men in agriculture by also taking into account the move out of agriculture of single-headed households. Also, the choice of dependent variables, due to the absence of work-load data for all adults, may hide changes in relative involvement in households with both men and women involved in agriculture, which might be very different to the changes in single-involvement households.
A second factor that may affect our results are local gender norms. These may strongly affect women’s own acknowledgment and ability to declare their role in agriculture when asked in a survey. Women may emphasize their alignment with local gender norms (e.g., the woman looks after the children and household chores and does not manage the farm) particularly in cases when men live elsewhere, to compensate for such “outlier behavior and guarantee social approval. Galiè and Farnworth (2019) refer to this phenomenon as “gender norms façade”: women and men may publicly declare to abide by gender norms particularly in situations where daily reality pushes them to break gender stereotypical behaviors. However, it is less clear whether the effect of these norms would change over the survey rounds. Follow-up qualitative analysis could help establish such gender norms and their dynamics.
The findings also show that the number of women not working is generally high: highest in India and lowest in Cambodia. This number is generally increasing across the years with stable trends in the Philippines and Indonesia. The number of women involved in economic non-agricultural activities varied considerably across the years. It increased overall in the Philippines only. Nevertheless, the results show an overall trend out of agriculture, especially for women, which is in line with the expectations of emerging economies.
Although our analysis does not engage with exploring the complex dynamics of rural transformation as recommended by Kawarazuka et al. (2022) we identified three main drivers of rural transformation impacting FoA or MoA: education, wealth and living together. The higher education levels both women and men attained, the less likely they were to be involved in agricultural activities. This implies that with higher education both men and women are likely to look for non-agricultural economic activity or to stop working. Hence, higher education is a major cause of moving out of agriculture for both women and men. Higher education of either spouse corresponds to a slight increase in the probability of the other spouse working in agriculture. In the case of men’s education there was a strong positive effect on women’s involvement in agriculture, while women’s education only slightly increased the probability of only male involvement in agriculture. An analysis of the wealth index showed that the richer the household the more likely it was that both household spouses were engaged in agriculture (reducing migration) or not at all (moving out of agriculture).
Future research could help identify some of the mechanisms behind these associations, for example, whether local gender and social norms dictate that an educated woman should marry an educated man (and consequently an educated woman means an educated husband and result in neither working in agriculture) but not the other way round (and consequently an educated man may be married to an uneducated woman who is likely to work in agriculture). Also, more research can shed light on the social dynamics affecting the correlation between wealth and agricultural work: one could hypothesize that poorer households may need to work in agriculture while richer households may choose to do so (or not) when larger assets are available and may make farming more lucrative.
Living together was used as a proxy for rural out migration in this study. Without out migration of the partner, the woman is less likely to be involved in agricultural activities while the likelihood of the man getting involved is higher. This seems to indicate that men tend to take on agricultural work, rather than the women, when living together in rural areas. When the husband lives elsewhere, however, the woman engages more in agricultural activities. Our results therefore, confirm that rural out migration of the partner is associated with a greater involvement of women in agriculture in line with Doss et al. (2021) pointing to rural out migration as a key driver of feminization of agriculture. However, out migration was only prominent in few of the studied countries, especially in Nepal and to some extent in Cambodia. This relates to a more general point that while regional assessments are important, the considerable differences between countries, as also indicated by VPC results quantifying variation contributions, suggest that a more detailed analysis of individual countries might yield specific new insights.
5. Conclusion
In this paper, we engage with the concept of Feminization of Agriculture which is often reported as a global trend. We address the lack of consistent approaches that provide comparative quantitative data on FoA by developing a methodological approach and deploying it on data from South and South-East Asia. We identified 5 relevant countries and determined FoA and MoA by comparing DHS data on the occupation of the household adult woman and man (Woman occupation and Husband/partner occupation) and their variation across two rounds of DHS data.
Our data show that in Cambodia, India, Indonesia and Nepal the trend has been towards a Masculinization of Agriculture between 2005 and 2017, while no trend could be determined for the Philippines. Women’s engagement in agriculture has generally decreased. The number of women not working is generally high; highest in India and lowest in Cambodia; it is generally increasing across the years with stable trends in the Philippines and Indonesia. The main drivers determining involvement in agriculture appear to be education, wealth and living together. The more educated the household members the more likely they would not be involved in agriculture. Household wealth was associated with less engagement in agriculture, especially for women. Living together entailed that more women drop out of agriculture and more men work in agriculture as compared to households where men have out migrated. We discuss the limitations of our methodology and suggest possible ways forward in such analysis. We recommend that further studies on trends towards feminisation or masculinization of agriculture could be improved with data on work-load contributions in households where both women and men are involved in agriculture and by also including single-headed households.
Data availability statement
Publicly available datasets were analyzed in this study. This data can be found at: https://dhsprogram.com.
Ethics statement
Ethical review and approval was not required for the study on human participants in accordance with the local legislation and institutional requirements. Written informed consent from the participants was not required to participate in this study in accordance with the national legislation and the institutional requirements.
Author contributions
SO and AG contributed to the conception, design of the study, and the writing of the first draft. SO performed the statistical analysis. NT supervised the statistical analysis. All authors contributed to the article and approved the submitted version.
Funding
This work has been carried out under the CGIAR GENDER Platform and the Sustainable Animal Productivity for Livelihoods, Nutrition and Gender Inclusion (SAPLING) Initiative, which are grateful for the support of CGIAR Trust Fund Contributors.
Conflict of interest
The authors declare that the research was conducted in the absence of any commercial or financial relationships that could be construed as a potential conflict of interest.
Publisher’s note
All claims expressed in this article are solely those of the authors and do not necessarily represent those of their affiliated organizations, or those of the publisher, the editors and the reviewers. Any product that may be evaluated in this article, or claim that may be made by its manufacturer, is not guaranteed or endorsed by the publisher.
Footnotes
1. ^Kawarazuka et al. (2022) show how gender norms affect the way agricultural innovations designed for men tend to be capital intensive (men are assumed to be ‘the farmers’ and ‘entrepreneurs’) Women farmers, on the other hand, are more likely to engage in low-input innovations which might include experimenting with a new crop in a small piece of land, and introducing low-investment livestock such as chicken, geese rabbit and pig.
References
Abdelali-Martini, M., Goldey, P., Jones, G. E., and Bailey, E. (2010). Towards a feminization of agricultural labour in Northwest Syria. J. Peasant Stud. 30, 71–94. doi: 10.1080/03066150412331311139
Basu, A., and Koolwal, G. (2005). Two concepts of female empowerment: Some leads from DHS data on womens status and reproductive health. Undefined.
de Brauw, A., Kramer, B., and Murphy, M. (2021). Migration, labor and women’s empowerment: evidence from an agricultural value chain in Bangladesh. World Dev. 142:105445. doi: 10.1016/J.WORLDDEV.2021.105445
Doss, C. R., Qaisrani, A., Kosec, K., Slavchevska, V., Galiè, A., and Kawarazuka, N. (2021). From the “Feminization of Agriculture” to Gender Equality. International Food Policy Research Institute. Washington, DC
Farnworth, C. R., Lecoutere, E., Galiè, A., Van Campenhout, B. C., Elias, M., Ihalainen, M., et al. (2023). ‘Methodologies for researching feminization of agriculture: what do they tell us?’. Progress in Development Studies. doi: 10.1177/14649934231173821
Farnworth, C. R., Jafry, T., Lama, K., Nepali, S. C., and Badstue, L. B. (2019). From working in the wheat field to managing wheat: women innovators in Nepal. Eur. J. Dev. Res. 31, 293–313. doi: 10.1057/S41287-018-0153-4/FIGURES/1
Galiè, A., and Farnworth, C. R. (2019). Power through: a new concept in the empowerment discourse. Glob. Food Sec. 21, 13–17. doi: 10.1016/J.GFS.2019.07.001
Kawarazuka, N., Doss, C. R., Farnworth, C. R., and Pyburn, R. (2022). Myths about the feminization of agriculture: implications for global food security. Glob. Food Sec. 33:100611. doi: 10.1016/J.GFS.2022.100611
Kazembe, L. N. (2020). Women empowerment in Namibia: measurement, determinants and geographical disparities. World Dev. Perspect. 19:100211. doi: 10.1016/J.WDP.2020.100211
Khatri-Chhetri, A., and Chanana, N. (2017). “Women’s groups reaping the benefits of solar energy for irrigation in Nepal’s Climate-Smart Villages,” CCAFS South Asia special edition newsletter on ‘Gender and Social Inclusion in Climate-Smart Agriculture’ on the occasion of International Day of Rural Women, ed. CCAFS Climate-Smart Agriculture Learning Platform, South Asia.
Salve Bacud, E., Puskur, R., Nhat, T., Duyen, L., Sander, O., and Luis, J. (2019). Rural outmigration – feminization – agricultural production nexus: case of Vietnam. Crit. Asian Stud. 10, 442–466. doi: 10.1080/21632324.2019.1679962
Sen, S., Mondal, S., Raj, D. A., Chatterjee, S., and Jain, S. (2019). Rural women at work or out of work: A gendered analysis of rural employment in eastern Gangetic Plains.
Slavchevska, V., Kaaria, S., and Taivalmaa, S.-L. (2016). Feminization of Agriculture in the Context of Rural Transformations. World Bank Publication. Pennsylvania
Keywords: feminization, masculinization, agriculture, South and South East Asia, DHS, labour allocation
Citation: Oloo SO, Galiè A and Teufel N (2023) Is agricultural labour feminizing in South and South East Asia: analysis of Demography and Health Services data on women and work. Front. Sustain. Food Syst. 7:1114503. doi: 10.3389/fsufs.2023.1114503
Edited by:
Wei Song, Chinese Academy of Sciences (CAS), ChinaReviewed by:
A. Amarender Reddy, National Institute of Agricultural Extension Management (MANAGE), IndiaNirmala Bandumula, Indian Institute of Rice Research (ICAR), India
Copyright © 2023 Oloo, Galiè and Teufel. This is an open-access article distributed under the terms of the Creative Commons Attribution License (CC BY). The use, distribution or reproduction in other forums is permitted, provided the original author(s) and the copyright owner(s) are credited and that the original publication in this journal is cited, in accordance with accepted academic practice. No use, distribution or reproduction is permitted which does not comply with these terms.
*Correspondence: Stephen Owambo Oloo, cy5vbG9vQGNnaWFyLm9yZw==