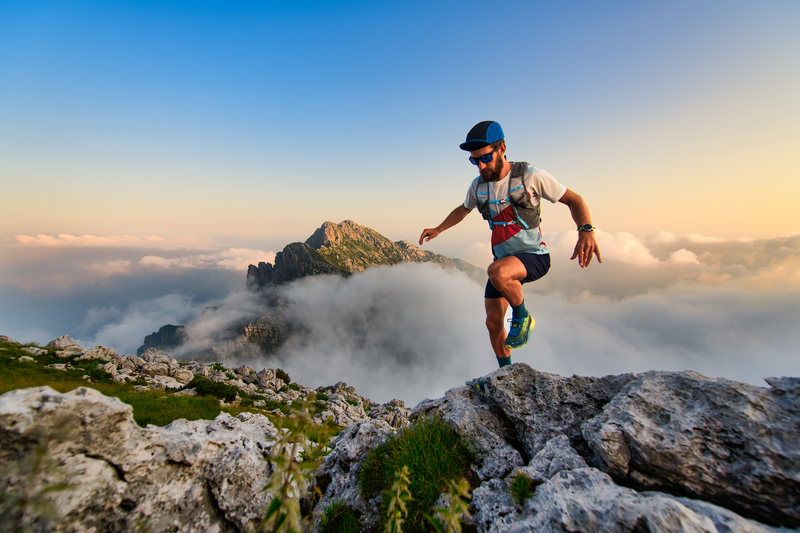
95% of researchers rate our articles as excellent or good
Learn more about the work of our research integrity team to safeguard the quality of each article we publish.
Find out more
ORIGINAL RESEARCH article
Front. Sustain. Food Syst. , 30 March 2023
Sec. Land, Livelihoods and Food Security
Volume 7 - 2023 | https://doi.org/10.3389/fsufs.2023.1106766
This article is part of the Research Topic Enhancing Food Production System Resilience for Food Security Facing a Changing Environment View all 15 articles
Introduction: To date, African swine fever (ASF) is the greatest challenge to sustainable development in the pig farming industry in Sichuan and elsewhere. Biosecurity measures adopted by farmers are an important way to prevent ASF. As a way to advocate the adoption of biosecurity measures by farmers, government regulations (GRs) can guide and promote farmers' biosecurity awareness and adoption of related measures and thereby support the sustainable development of pig farming.
Method: In this study, a theoretical framework for systematic analysis is established, and survey data of 351 pig farmers are used to estimate the effects of GRs on the farmers' adoption of biosecurity measures.
Conclusions: The main conclusions are as follows. (1) The different types of GRs all pass the significance test and promote farmers' adoption of biosecurity measures, indicating that the Chinese government's biosecurity policies at this stage are effective. (2) According to a mediation effect model, GRs can promote farmers' adoption of biosecurity measures by increasing the level of biosecurity awareness among farmers. (3) From the results of a heterogeneity test, the effects of GRs on the adoption of biosecurity measures among farmers in the low rearing income level, short rearing time and low rearing scale groups are much greater than those in the high rearing income level, long rearing time and high rearing scale groups.
Policy recommendations: Based on the above conclusions, the results of this study suggest that the government should continue to strengthen GRs, especially guided GRs, such as biosecurity measure training. Moreover, for farmers with different backgrounds, the government should adopt distinct strategies to improve the effectiveness of GRs.
African swine fever (ASF) is an animal disease with high infectivity and mortality (Mason-D'Croz et al., 2020). As an exotic disease, ASF has been highly disastrous to the Chinese livestock industry (Zhao, 2019; Yao et al., 2022). According to the National Bureau of Statistics of China (NBS, 2022), in 2019, pig production in the country declined severely to 310.41 million heads, representing a 27.5% decline from 2018 (NBS, 2022). Within a short period, ASF spread to all regions of mainland China, and most farmers were forced to stop production or reduce the scale of pig farming (Xu et al., 2022; Yao et al., 2022). The shortcomings of biosecurity prevention and control in the pig farming industry have been revealed. Biosecurity measures are essential for maintaining healthy farms (Horrillo et al., 2022). Therefore, it is important to promote farmers' adoption of biosecurity measures to sustainably develop the pig farming industry (Xu et al., 2022).
Government regulations (GRs) are the main measures to regulate farmer behaviors (Zhao et al., 2018). Since the emergence of ASF in northeastern China at the end of 2018, the Chinese government has issued several successive notices to slow the destructiveness of the disease. These policies include the Notice of the General Office of the State Council on Doing a Good Job in the Prevention and Control of ASF and Other Animal Diseases and the Notice of the Ministry of Agriculture, and Rural Affairs on Printing and Distributing Eight Bans on the Prevention and Control of ASF. However, because of the high transmission and mortality and the weak biosecurity control abilities of farmers, biosecurity control has not been visibly effective. From 2019 to 2021, the Chinese government issued policies to improve farmer biosecurity prevention and control capabilities, including the Opinions on Strengthening the Prevention and Control of ASF, ASF Emergency Implementation Plan (2019 Edition), ASF Emergency Implementation Plan (2020 Edition), and Technical Guidelines for Normalized Prevention and Control of ASF (Trial Version). In 2021, ASF was successfully controlled in China, and the yield of pigs in that year reached 670 million heads, returning to the level of a normal year (2017). The Chinese government has made great contributions to the prevention of ASF. Therefore, a full understanding of the role of Chinese government regulations in farmers' adoption of biosecurity measures has tremendous implications for other countries experiencing ASF.
ASF is devastating to the pig industry; improving the biosecurity behaviors of farmers has become a popular topic. Xie (2022) conducted a study on whether policy insurance is conducive to farmers recovering lost pig production based on 360 questionnaires in Jiangsu Province, China; the researchers found that compensation encourages farmers to adopt biosecurity measures to a certain extent. However, Beach et al. (2007) believes that there is a certain substitution relationship between government compensation and farmers' biosecurity input, leading to the deviation of farmers' behaviors from policy goals and creating adverse incentives. The reason for this phenomenon may be that farmers' biosecurity behavior or incentive policies distort market signals, reducing decision-making motivation due to information asymmetry (Bicknell et al., 1999). In addition, some scholars have discussed the factors that affect the biosecurity behaviors of pig farmers. Sahlström et al. (2014) found that farmers' sex, age, education level, and risk preference have positive effects on biosecurity behaviors. Other scholars have found that the scale of rearing, the standardization of pig farm facilities, and the content of training play important roles in the prevention of disease and the control of farmers' biosecurity (Zhang and Wu, 2012; Wu et al., 2015; Hennessy and Wolf, 2018). Although there are some studies on the biosecurity behaviors of farmers in the literature, some inadequacies remain. Firstly, there are many types of GRs, and the effects of GRs on the behaviors of pig farmers are not fully explained from a single perspective; thus, deeper discussion is necessary. Secondly, the literature rarely discusses the influence mechanism of GRs on the adoption of biosecurity measures by pig farmers, while improving the effectiveness of GRs is an important way to ensure the sustainable development of the pig farming industry.
To date, ASF is still the biggest challenge for the sustainable development of the pig farming industry. Many countries have invested large amounts of money and specialized technical staff to address ASF. Therefore, it is valuable to study whether GRs effectively promote farmers' adoption of biosecurity measures. The main objective of this study is to analyze the mechanisms and interventions by which GRs influence farmers' adoption of biosecurity measures to improve the sustainable development of the pig farming industry. The specific goals include (1) to theoretically reveal the influence mechanism of GRs on farmers' adoption of biosecurity measures; (2) to empirically analyze the effects of GRs (including different types of GRs) on the adoption of biosecurity measures by pig farmers by using survey data; (3) to test the mediating effect of biosecurity awareness of pig farmers; and (4) to analyze the role of GRs in the adoption of biosecurity measures by pig farmers in different farming contexts. There are three marginal contributions. Firstly, we use microsurvey data to analyze the impacts of GRs on farmers' adoption of biosecurity measures, providing insights for government decision-making. Secondly, we use a mediation effects model to analyze the transmission mechanisms by which GRs influence farmers' adoption of biosecurity measures, contributing to an in-depth understanding of methods to promote farmers' adoption of biosecurity measures. Third, grouping regressions are used to test the heterogeneous effects of GRs on biosecurity measures adopted by farmers with different backgrounds, which can help government policy become more targeted and effective.
As economic agents, farmers' biosecurity behaviors have great externalities in their biosecurity behaviors (Brennan and Christley, 2013). Ellis-Iversen et al. (2010) and Brennan and Christley (2013) argued that in the processes of farming production and disease prevention and control, some farmers have refused to implement biosecurity measures; thus, they sell infected livestock to reduce costs and obtain high returns, harming the interests of other farmers and generating large negative externalities (Ellis-Iversen et al., 2010; Annes and Bessiere, 2018). In practical production, pig farmers are limited rational agents (Liu, 2021). If the cost of adopting biosecurity measures greatly exceeds the sales revenue of farmers, it is difficult for pig farmers to choose to focus on biosecurity issues. Therefore, the resulting negative externalities require government intervention (Zhou et al., 2022). To maximize utility in the decision-making process, the sum of utilities obtained by farmers adopting biosecurity measures, without considering external factors, is expressed as follows (1):
where ΔU1 is the total utility obtained by the adoption of biosecurity measures by a farmer without considering external factors; UT is the utility gained from the production improvement (T) due to the adoption of biosecurity measures (UT ≥ 0 as an increasing function of T); UPE is the utility of the positive externality (E) gained by farmers adopting biosecurity measures (UPE ≥ 0 and is an increasing function of E); UC1 is the reduced utility due to the cost of adopting biosecurity measures (C1, 0 ≤ C1 ≤ BDG, where BDG is a budget constraint for farmers; ΔU1 ≥ 0 is an increasing function of C1). When U1 > 0, the farmer adopts biosecurity measures; otherwise, these measures are not adopted.
By considering external institutional factors, the utility of farmers' adoption of biosecurity measures includes the government benefits received. Then, the utility obtained by the farmer is expressed as follows (2):
where ΔU2 is the total utility obtained by farmers adopting biosecurity measures considering external institutional factors; US is the utility of government support (S) received by farmers for adopting biosecurity measures (US ≥ 0, which is an increasing function of S); UC2 is the reduced utility (UC2 ≥ 0, which is an increasing function of C2) of government penalties (C2, 0 ≤ C2 ≤ BDG) for biosecurity risks caused by farmers who do not adopt biosecurity measures, such as the unreasonable disposal of breeding waste and the sale of dead pigs; and UL is the utility loss caused by the biosecurity risk.
GRs include three dimensions: guided government regulations (GGRs), incentive government regulations (IGRs), and constraint government regulations (CGRs) (Ajzen, 1991; Zhu et al., 2021). In the context of farmers' biosecurity measures, GGRs describe regulations by which the government conducts various activities, such as publicity, promotion, and training, related to disease prevention and control of pig production to enhance farmers' biosecurity awareness. This approach enables farmers to realize the economic benefits created by adopting biosecurity measures (UT and UPE increase while UL decreases), and reduce pig farmers' information collection and technical learning costs (UC1 decreases), thereby increasing the adoption of biosecurity measures. IGRs include regulations in which the government reduces the cost of safe production (Cb) for farmers by providing subsidies to ensure that they receive minimum compensation and to promote their expected benefits. Under these measures, the actual expenditure of farmers adopting biosecurity measures is Cr (Cr = C1-Cb), and the cost–utility of adopting biosecurity measures for farmers decreases to UCr. Therefore, the utility obtained by the farmer is . Because C1 > Cr, UC1 > UCr, and , thus, . CGRs encompass regulations in which the government restricts the production measures available to farmers by promulgating relevant laws and policies and increasing the degree of punishment for illegal activities. Pig farmers that do not adopt biosecurity measures are punished (C2) for unreasonable biosecurity risks caused by abusing veterinary drugs and randomly disposing of infected or dead pigs, increasing UC2. Therefore, for government constraints, the cost for farmers likely increases. To reduce the cost of violations, farmers must comply with the relevant GRs. Therefore, we propose the following hypothesis (H1):
H1: GRs are conducive to promoting farmers' adoption of biosecurity measures, and IGRs, CGRs and GGRs have the same promoting effect.
According to the theory of planned behaviors (Ajzen, 1991), the decision-making of the agent is a dynamic process in which the agent forms their awareness by collecting and screening information, guiding their behavioral responses (Lin et al., 2018). Biosecurity awareness refers to a subject's understanding of specific information, government policies, and safety values; this aspect has an important impact on the overall prevention and control of animal diseases (Breen et al., 2013). In this paper, farmers' awareness of biosecurity behaviors refers to their understanding of biosecurity policies, information, and values. As the main body of biosecurity behaviors, the farmers' biosecurity awareness level is the basis for promoting the adoption of biosecurity measures (Xu et al., 2022). Farmers' biosecurity awareness reflects whether they understand methods for maintaining the biosecurity situation of the farm and whether they have a sense of responsibility for this action. The expansion of farmers' awareness of biosecurity may help guide the emergence of biosecurity behaviors among farmers (Qiao and Zhang, 2019). Based on the above analysis, we propose the following hypothesis (H2):
H2: Biosecurity awareness can encourage farmers to adopt biosecurity measures.
GRs may affect farmers' adoption of biosecurity measures by increasing their biosecurity awareness. Due to the limitations of age and education experience, it is difficult for most farmers in China to learn biosecurity knowledge and skills without assistance (Yu and Yu, 2019). To improve farmers' productivity and revive the industry, the government has formulated biosecurity prevention and control policies, conducted awareness training, and monitored and disciplined farmers (Toma et al., 2013). These actions can guide farmers to understand pig farming and biosecurity policies, regulate their production behaviors, and reduce biosecurity risks (David et al., 2021). This information leads to the following hypothesis (H3):
H3: GRs promote the adoption of biosecurity measures by enhancing farmers' biosecurity awareness.
Sichuan Province is a major pig production area in China and has been ranked among the top regions for pig production for many years (Wang et al., 2015). Before the outbreak of ASF, the pig rearing volume in Sichuan Province reached 43.77 million heads in 2017, accounting for ~10% of the national total (NBS, 2022). After the spread of ASF in Sichuan Province, government departments provided substantial support to resume pig rearing, enabling the province to recover pig production capacity. According to the NBS, in 2020, the number of pigs reared in Sichuan Province reached 56.14 million heads, accounting for 10.65% of the total number in the country and ranking first in China. Moreover, Sichuan Province's economic development conditions and rearing environment exhibit obvious stepped characteristics. For example, the levels of economic development and rearing technology are relatively high in the central plain and relatively low in the hilly and plateau areas. These factors have a certain influence on the implementation of government policies and the biosecurity behaviors of farmers, which better represent the production recovery of farmers at different levels. Therefore, we take Sichuan Province as the field investigation area in this paper.
The research method involved one-on-one household interviews in Sichuan Province from June to September 2021. The sample selection of farmers was based on the principle of combining purposive, stratified and random sampling. The specific sampling investigation process was as follows. Firstly, according to regional characteristics, Sichuan Province was divided into five regions: the Chengdu plain economic zone, southern Sichuan economic zone, northeast Sichuan economic zone, Panxi economic zone, and northwest Sichuan ecological economic zone. We chose the Chengdu plain economic zone, southern Sichuan economic zone and northeast Sichuan economic zone for investigation. These three regions have a greater concentration of pig farms and have the largest number of pigs (Wang et al., 2015). Then, 1 large pig rearing county was randomly selected from each region. Secondly, to maximize the validity and representativeness of the sample, we interviewed regional agricultural departments and technical extension officers to gain a more detailed understanding of the development of the pig farming industry in the study area (Xu et al., 2022). According to expert suggestions, we selected the three towns with the largest rearing volume and randomly selected 40–50 pig farmers. We completed a total of 370 questionnaires through a survey. After screening and eliminating important data that were missing and inconsistent from questionnaires, a total of 351 valid questionnaires were obtained, for an effective rate of 94.86%. A map of the sample locations is shown in Figure 1.
Table 1 shows the basic information of the surveyed farmers. Among the sample, in terms of individual basic characteristics, 269 male farmers were interviewed, accounting for 76.64% of the total sample. Most farmers were between 40 and 59 years old; the education level of farmers was mainly junior high school and below in the total sample, accounting for 83.19%. Concerning production and operation, 67.24% of the pig farmers had been raising pigs for more than 10 years. Most farmers had raised fewer than 100 pigs (61.82%), and their rearing income exceeded 50% (70.37%) of the total household income. This finding was consistent with the fact that pig farming in rural areas in China is dominated by males and middle-aged and elderly people with a low overall education level on small- and medium-sized farms. In addition, only 11.97% of the interviewed farmers who participated in the production organization were among the 73.22% of farmers who purchased rearing insurance.
In this study, we selected the farmers' adoption of biosecurity measures (FABM) as the explained variables and expressed them in terms of the number of biosecurity measures adopted by farmers. According to Center People's Government of the People's Republic of China (2010) issued by the Ministry of Agriculture Rural Affairs of the People's Republic of China (2010) and the research of Zhou et al. (2020) and Bai et al. (2012), we selected 15 core biosecurity measures: site selection and layout of pig farms, foreign personnel and vehicle management, entry and exit management of carrying items, introduction and isolation management, all-in and all-out farming, optimized management of rearing environment, clean and dirty management, food and drug storage management, rational use of veterinary drugs, regular epidemic prevention management of inputs (feed, drinking water, and drug), anti-mosquito, rat and bird facilities, internal disinfection management of pig farms, farm management record file, separate rearing of sick pigs in farms, and regular epidemic prevention and management of pigs. We categorized the number of biosecurity measures adopted by farmers into five categories, with farmers adopting 1–3 biosecurity measures being assigned a value of 1, farmers adopting 4–6 biosecurity measures being assigned a value of 2, farmers adopting 7–9 biosecurity measures being assigned a value of 3, farmers adopting 10–12 biosecurity measures being assigned a value of 4, and farmers adopting 13–15 biosecurity measures being assigned a value of 5.
The core explanatory variables of this paper are GRs, including (1) GGRs, which include the number of biosecurity trainings organized by the government as a proxy variable; (2) IGRs, which include the amount of government subsidy funds as a proxy variable; and (3) CGRs, which include the intensity of government supervision on farms as a proxy variable.
The mediation variable in this paper is biosecurity awareness, including (1) policy awareness (PA), using farmers' understanding of biosecurity Technical Guidelines for the MOA (2020) as a proxy indicator; (2) value awareness (VA), using farmers' value awareness of biosecurity behaviors to prevent and control epidemic diseases as a proxy indicator; and (3) content awareness (CA), using farmers' understanding of the content and requirements of farm biosecurity management as a proxy indicator.
Based on Li et al. (2014) and Wang et al. (2016), we select the factors affecting farmers' adoption of biosecurity measures from two aspects: the basic characteristics of farmers and the characteristics of production and operation. Among these aspects, the basic characteristics of farmers include sex, age, education experiences (EE), and the labor force of the farming family (LF). The characteristics of production and operation include rearing time (RT), rearing scale (RS), rearing income as a proportion of the total household income (RIS), whether the farm is a standardized farm (SF), whether the farmers have joined a cooperative organization (CO), and whether they buy rearing insurance (RI). Table 2 shows the descriptive statistical characteristics of the variables.
The number of biosecurity measures adopted by farmers is an ordinal variable, an O-logistic model used to analyze the impact of GRs on farmers' adoption of biosecurity measures. The specific equation is as follows.
where is the explained variable, which represents the number of biosecurity measures adopted by farmers; GRsi represents the GRs, when i = 1,2,3, GRs refer to IGRs, GGRs, and CGRs, respectively; xi are control variables, which include the farmer's age, sex, education experience, etc.; εI is the random error term; β1 and βi are the coefficients. cannot be directly observed, so it needs to be represented by the variable yi that can be directly observed. Let λ1 < λ2 < … < λn−1 represent the threshold. In the case of n=5, there are 4 thresholds, and the corresponding relationship between yi and is shown in formula (4).
To understand the internal transmission mechanism of the mediation effect model, we used the KHB model (Karlson and Holm, 2011; Xu et al., 2022) to decompose the total effect into direct effect and indirect effect test. The calculation process is as follows.
Suppose the linear regression model is as follows (5):
where Y is the explained variable; X is the core explanatory variable; M is the mediating variable; C is the control variable; and X affects Y through M. In this model, β1 is the direct effect of X on Y.
To further calculate the total effect of X on Y:
The indirect effect of the core explanatory variable X on the explained variable Y by affecting the mediating variable M can be expressed as:
In this paper, if X affects Y* through M, then:
The direct effect can be expressed as b1 = β1/σ1; total effect: b2 = β2/σ2. σ1 and σ2 are scale parameters, which are the residual standard errors of Equations (5) and (6). At this point, the indirect effect of the logistic model can be expressed as (10):
The indirect effect is determined by the two scale parameters σ1 and σ2, which can be calculated by measuring the residual of the linear regression of the mediating variables on the explanatory variables.
where a and b are linear regression coefficients, and R is the residual. Substituting R into model (6), we can obtain Equation (12):
, is the residual standard deviation of the above formula, and . Then (13) and (14) can be calculated as follows:
In the same way, the proportion of each coefficient can be calculated as:
To verify the mediation effect of the above model, it is only necessary to test the hypothesis H0:b1 = b2, that is, to test whether β1 and β2 are equal.
where b is the coefficient of X to M in Equation (11), for (16) not to be 0, the direct effect of M on Y must not be 0, that is , and X is related to M, that is, b ≠ 0.
The Sobel test can be used to obtain the following test formula for the indirect effect:
where a′ represents the vector, ∑ is the covariance matrix variance of γ2 and b.
To ensure the accuracy of model parameter estimation, we conduct a multicollinearity test on each variable before regression. The results show that the tolerance is much larger than 0.1, and the maximum value of VIF is 1.81; this value is much smaller than 10, indicating that there is no multicollinearity among the variables.
We use an O-logistic model to analyze the influences of GRs and biosecurity awareness levels on farmers' adoption of biosecurity measures. To further prove the robustness of the results, we use an ordered-probit (O-probit) model for analysis. The benchmark regression results (Table 3) show that the significance and direction of each variable are consistent with the regression results of the O-logistic model, indicating that the regression results are relatively robust.
According to the results in Table 3, all three types of GRs passed the significance test, indicating that the top-down governance method significantly affected farmers' biosecurity behaviors. Specifically, GGRs, IGRs, and IGRs all passed the significance test (P ≤ 10%), and the coefficients were positive. This finding indicated that GGRs, IGRs, and IGRs played significant roles in promoting the adoption of farmers' biosecurity measures.
All three types of biosecurity awareness passed the significance test, indicating that biosecurity awareness significantly impacted farmers' adoption of biosecurity measures. Specifically, both biosecurity policy awareness and content awareness were significant at the 1% level, and the coefficients were positive. Value awareness was significant at the 1% level, but value awareness had an inhibitory effect on farmers' adoption of biosecurity measures.
Sex was significant at the 5% level, and the coefficient was positive, indicating that sex played a significant role in promoting the adoption of biosecurity measures by farmers. Family labor was significant at the 10% level, and the coefficient was positive, indicating that the more laborers in the family were engaged in pig rearing, the more inclined the farmers were to adopt biosecurity measures. The rearing scale and standardized farms were significant at the 1% level, respectively, and both promoted the adoption of biosecurity measures. Rearing insurance was significant at the 5% level, and the coefficient was positive, indicating that farmers who purchased rearing insurance had higher biosecurity awareness levels and were more inclined to adopt biosecurity measures.
The estimation results of the benchmark model show that GRs and biosecurity awareness significantly impact farmers' adoption of biosecurity measures. On this basis, we discuss whether there is a mediating role in the influences of GRs on farmers' adoption of biosecurity measures, the relationship between the direct and indirect impact paths, and the contribution of each mediation variable to farmers' adoption of biosecurity measures. According to the results of the mediation effect test in Table 4, the overall fitting effect of the model is acceptable.
Column (1) in Table 4 shows the results of the O-logistic model without mediating variables, and columns (2)–(4) show the results of the regression tests with mediating variables. According to the test results of the mediation effect model, GRs positively affect policy, content, and value awareness levels, and all of these effects pass the significance test. Relative to column (1), there is no significant shift in the coefficients of GRs in column (5). Policy awareness, content awareness, and value awareness all pass the significance test, proving that GRs have a mediating effect on farmers' adoption of biosecurity measures. Thus, hypotheses 1, 2, and 3 are further verified.
The KHB model is further applied to decompose the effects of GRs on farmers' adoption of biosecurity measures. According to Table 5, the total effect of the model is 1.656, of which the direct effect is 1.153, the indirect effect is 0.502 and the effect is significant at the 1% level. In addition, Table 5 shows that the indirect effect accounts for 30.31% of the total effect, indicating that GRs greatly impact farmers' adoption of biosecurity measures by enhancing biosecurity awareness levels. The total, direct, and indirect effects were positive in terms of the coefficient symbol, indicating that GRs may directly promote the adoption of biosecurity measures by farmers and produce indirect positive effects through mediation variables.
According to the results in Table 6, the indirect effects of GRs through policy, value, and content awareness are 0.465, −0.037, and 0.192, respectively. Policy awareness has the largest indirect effect on farmers' adoption of biosecurity measures, accounting for 74.97% of the indirect effect and 44.24% of the total effect. The indirect effect of value awareness is minimal, and its contribution is negative, accounting for −5.98% of the indirect effect and −3.53% of the total effect; these findings are consistent with the previous results.
Table 6. Decomposition of the indirect effects of GRs on the farmers' adoption of biosecurity measures.
To examine the stability and reliability of the research methodology and the explanatory power of the indicators, and to prevent the estimation bias caused by certain uncontrollable factors from making the research conclusions unconvincing, based on the use of the O-probit model (Table 3), this paper further tests the robustness by replacing the core explanatory variables. The variable “government supervision intensity” is replaced with “government supervision scope on farm safety”, and GR is re-measured by the entropy method. The results of the robustness test (Table 7) indicate that the core explanatory variables and mediating variables pass the significance test, and the direction of the coefficients is mostly consistent with the results in Table 4, further suggesting that the results of this study are robust.
We study the mechanisms by which GRs influence farmers' adoption of biosecurity measures; however, the rearing income level, rearing time, and rearing scale of different farmers influence GRs and farmers' adoption of biosecurity measures. Therefore, we divide the rearing income level, rearing time and rearing scale into groups to further explore the impacts of GRs on farmers' adoption of biosecurity measures. We use the mean values of the rearing income level, rearing time, and rearing scale as the grouping criteria; additionally, we divide the farmers into low-mean groups (low rearing income level, low rearing scale and short rearing time) and high-mean groups (high rearing income level, high rearing scale and long rearing time). Next, we take farmers' adoption of biosecurity measures as the explained variables and GRs, IGRs, CGRs, and GGRs as the explanatory variables and use the O-logistic model to examine the contributions of different rearing income levels, rearing times, and rearing scale groups to GRs and farmers' adoption of biosecurity measures. In this paper, the rearing income as a proportion of the total household income is used to express the rearing income level of farmers.
From Table 8, the effects of GRs on farmers' adoption of biosecurity measures in the low-mean groups exceed those in the high-mean groups, indicating that GRs are more effective in the low-mean groups. Table 9 shows the effects of IGRs, CGRs, and GGRs on farmers' adoption of biosecurity measures in different groups. Notably, the results shown in Table 9 are generally consistent with those in Table 8. The coefficients of various GRs in the low-mean groups are higher than those in the high-mean groups, which further shows that the roles of GRs in the low-mean groups are more significant.
Table 9. Effects of IGRs, CGRs, and GGRs on farmers' adoption of biosecurity measures in different groups.
ASF has brought great harm to the pig farming industry in the world, so it is of great significance to find effective solutions for the pig farming industry and residents' diets. Effectively preventing the spread of animal diseases requires government support and farmers' adoption of biosecurity measures. However, the effectiveness of GRs largely depends on farmers' acceptance of different GRs and their biosecurity awareness (Xu et al., 2023). Therefore, it is necessary to examine the mechanism of different types of GRs on biosecurity measures adopted by farmers with different backgrounds to develop more perfect biosecurity prevention and control policies. This study focused on the impact of GRs, farmers' biosecurity awareness, farmers' basic characteristics and production operation characteristics on farmers' adoption of biosecurity measures. To examine these contents, a total of 351 valid samples from pig farmers were collected and were analyzed using O-probit, mediation effect model and heterogeneity test method.
Through empirical analysis, we demonstrate that current Chinese GRs can effectively promote the adoption of biosecurity measures by pig farmers. This result shows that the recent epidemic prevention policy formulated by the Chinese government to address ASF was more effective than other measures, encouraging farmers to adopt biosecurity measures to prevent the spread of immune diseases, and protect the health of pigs. However, different types of GRs have distinctly varying roles. As shown in Table 3, GGR has the largest coefficient (0.629), while IGR has the smallest coefficient (0.265). This means that GGR plays a stronger role than IGR. According to the survey study, most of the government subsidies received by pig farmers were <1,000 yuan (Table 2), and the cost of pig mortality far exceeded this value, which might be the reason for the low role of IGR. This shows that when large-scale animal diseases occur, relying on only less subsidies is not the best way, and technical training by the government may be more conducive to the farmers' adoption of biosecurity measures.
Farmers' personal characteristics and production operation characteristics also have an impact on the adoption of biosecurity measures by farmers. We find that farmers with larger size, more labor, and more standardization are willing to adopt more biosecurity measures. As stated by Ghimire and Huang (2016), larger farmers are more inclined to adopt advanced technologies to expand their returns and protect their assets. Compared with women, male interviewees express a willingness to adopt more biosecurity measures, and the reason for this phenomenon could be that female farmers tend to be more conservative in their decision-making regarding biosecurity measure adoption (Liu et al., 2019). Farmers with rearing insurance are more likely to adopt biosecurity measures. Rearing insurance, as a vital risk management tool, improves the risk tolerance of farmers by dispersing risks (Ghosh et al., 2022). This condition allows farmers to try new technologies without worrying about the adverse consequences of these technologies, thus improving the allocation efficiency of resources (Wu and Li, 2023).
Farmers' biosecurity awareness has a significance impact on farmers' adoption of biosecurity measures. However, although pig farmers are familiar with government policies and biosecurity measures, the policy values do not seem to be effective in promoting farmers' adoption of biosecurity measures. These results are similar to those of Lanyon et al. (2015) and Frössling and Nöremark (2016); that is, although farmers seemed to believe that implementing biosecurity measures was beneficial (Sayers et al., 2013), they did not widely implement such measures. There could be several reasons for this phenomenon. The interviewed farmers were small-scale free-range farmers, and there was a difference in the biosecurity awareness levels of traditional backyard farmers and standardized large-scale farmers, and the awareness levels of the investigated farmers could deviate from the actual behaviors (Li et al., 2014). Even if the farmers recognized that biosecurity behaviors can effectively prevent and control diseases, they may not have been able to adopt biosecurity measures for production due to practical factors such as the cost of adopting measures and human resource constraints (Liu et al., 2017). Some farmers even reduced production costs by decreasing the production scale and decreasing biosecurity measures (Tian and Stephan, 2020).
In addition, government regulations are more effective for farmers with short farming time, low farming income and low farming scale. These results are similar to those of Simon-Grifé et al. (2013); that is, farmers with a larger rearing scale, higher rearing income, and longer rearing time have higher management and biosecurity awareness levels, making it difficult for the government to form an obvious incentive and play a guiding role for these farmers. In contrast, smaller-scale farmers generally lack the application of biosecurity measures and awareness (Costard et al., 2009). With the help of the government, the biosecurity behaviors of small-scale farmers will effectively improve. Moreover, we see that GGRs have the greatest impact on farmers' adoption of biosecurity measures, while IGRs have the smallest impact. This result suggests that farmers are willing to receive government training, not just sanctions and subsidies (Moya et al., 2021). Therefore, the government needs to understand the characteristics of local farmers when formulating relevant policies and it should not concentrate all subsidies on large-scale farms, especially in developing countries where there are more small-scale farmers.
Although our study provides help in promoting farmers' adoption of biosecurity measures, there are some limitations. With the dual limitations of ASF and COVID-19, it is difficult for us to conduct continuous tracking surveys to form panel data. Panel data can better identify changes in farmers' adoption behaviors toward biosecurity measures under different levels of risk. In addition, another limitation of this paper is the lack of discussion of biosecurity measure adoption behaviors in large-scale farming enterprises. The huge risk posed by ASF led to many small-scale farmers withdrawing from the farming industry, thus rapidly increasing the number of large-scale farming enterprises. These farming enterprises have more capital and advanced technology and are able to provide technical and financial assistance to small-scale farmers. Therefore, future scholars should adopt panel data to analyze the factors influencing farmers' adoption of biosecurity measures. It can also focus on whether large-scale pig farming enterprises can generate technical and economic spillover effects for surrounding small-scale farmers. We believe that more in-depth studies can provide better empirical evidence for the sustainable development of pig farming.
We use survey data from 351 pig farmers to investigate the transmission mechanisms and heterogeneity characteristics of the effects of GRs on farmers' adoption of biosecurity measures. The main conclusions are as follows. (1) According to the benchmark regression results, we demonstrate that GRs positively impact farmers' adoption of biosecurity measures. Furthermore, the three types of GRs—IGRs, GGRs, and CGRs—all promote the adoption of biosecurity measures by pig farmers; this finding indicates that the Chinese government's biosecurity measures at this stage are effective. (2) Through the mediation effect test, GRs can promote the adoption of biosecurity measures by enhancing farmer biosecurity awareness. Among these roles, the indirect effects of policy awareness and content awareness are more significant, while value awareness does not contribute significantly. (3) From the results of the heterogeneity test, we find that the effects of GRs on farmer biosecurity behaviors in the low-mean groups (low rearing income level, low rearing scale, and short rearing time) are considerably greater than those in the high-mean groups (high rearing income, high rearing scale, and long rearing time).
According to the research conclusions, to further strengthen the biosecurity prevention and control capabilities of farmers and to promote sustainable development in the pig farming industry, we propose the following policy recommendations. (1) The government should continue to strengthen GRs, especially GGRs, because they have the greatest positive impact on farmers' adoption of biosecurity measures. For example, the government can establish a biosecurity prevention and control information platform to share measures and technologies. (2) The government should reasonably guide farmers' awareness levels of policies and biosecurity behaviors and actively promote farmers' adoption of biosecurity measures. To mitigate farmers' fear of ASF, good publicity by government department staff and veterinarians is needed. (3) The government should strengthen the guidance and incentives for small-scale farmers to maximize the efficiency of government biosecurity measures.
The raw data supporting the conclusions of this article will be made available by the authors, without undue reservation.
Ethical review and approval was not required for the study on human participants in accordance with the local legislation and institutional requirements. Written informed consent from the participants was not required to participate in this study in accordance with the national legislation and the institutional requirements.
JL and MY: conceptualization and software. MY and KZ: methodology, formal analysis, and data curation. MY and HW: validation. KZ and HW: resources. JL: writing—original draft preparation. JL and KZ: writing—review and editing and supervision. MY: visualization. JL and HW: funding acquisition. All authors contributed to the article and approved the submitted version.
This research was funded by National Natural Science Foundation for Youth Foundation, grant number 72103152.
Thanks to the government staffs who helped the researchers and to the farmers who accepted the questionnaire.
The authors declare that the research was conducted in the absence of any commercial or financial relationships that could be construed as a potential conflict of interest.
All claims expressed in this article are solely those of the authors and do not necessarily represent those of their affiliated organizations, or those of the publisher, the editors and the reviewers. Any product that may be evaluated in this article, or claim that may be made by its manufacturer, is not guaranteed or endorsed by the publisher.
African swine fever, ASF; Government regulations, GRs; guided government regulations, GGRs; incentive government regulations, IGRs; constraint government regulations, CGRs; policy awareness, PA; value awareness, VA; content awareness, CA; education level, EL; the labor force of the farming family, LF; rearing time, RT; rearing scale, RS; the rearing income as a proportion of the total household income, RIS; standardized farm, SF; cooperative organization, CO; rearing insurance, RI; farmers' adoption of biosecurity measures, FABM; National Bureau of Statistics of China, NBS.
Ajzen, I. (1991). The theory of planned behavior. Organ. Behav. Hum. Decis. Process. 50, 179–211. doi: 10.1016/0749-5978(91)90020-T
Annes, A., and Bessiere, J. (2018). Staging agriculture during on-farm markets: how does french farmers' rationality influence their representation of rurality? J. Rural Stud. 63, 34–45. doi: 10.1016/j.jrurstud.2018.07.015
Bai, B., Wu, Z., Yan, R., Zhao, X., and Chen, T. (2012). Establishment of swine fever risk assessment model for large-scale pig farms. Adv. Anim. Med. 33, 105–109.
Beach, R. H., Poulos, C., and Pattanayak, S. K. (2007). Agricultural household response to avian influenza prevention and control policies. J. Agri. Appl. Econom. 39, 301–311. doi: 10.1017/S1074070800023002
Bicknell, K. B., Wilen, J. E., and Howitt, R. E. (1999). Public policy and private incentives for livestock disease control. Aust. J Agric. Resour. Econ. 43, 501–521. doi: 10.1111/1467-8489.00092
Breen, R., Karlson, K. B., and Holm, A. (2013). Total, direct, and indirect effects in logit and probit models. Sociol. Methods Res. 42, 164–191. doi: 10.1177/0049124113494572
Brennan, M. L., and Christley, R. M. (2013). Cattle producers' perceptions of biosecurity. BMC Vet. Res. 9, 71. doi: 10.1186/1746-6148-9-71
Center People's Government of the People's Republic of China. (2010). The Measures for the Review of Animal Epidemic Prevention Conditions. Available online at: http://www.gov.cn/zwgk/2010-01/28/content_1521669.htm (accessed September 2, 2021).
Costard, S., Porphyre, V., Messad, S., Rakotondrahanta, S., Vidon, H., Roger, F., et al. (2009). Multivariate analysis of management and biosecurity practices in smallholder pig farms in Madagascar. Prev. Vet. Med. 92, 199–209. doi: 10.1016/j.prevetmed.2009.08.010
David, J.-C., Buchet, A., Sialelli, J.-N., and Delouvée, S. (2021). The use of antibiotics in veterinary medicine: representations of antibiotics and biosecurity by pig farmers. Prat. Psychol. 27, 159–170. doi: 10.1016/j.prps.2020.06.003
Ellis-Iversen, J., Cook, A. J. C., Watson, E., Nielen, M., Larkin, L., Wooldridge, M., et al. (2010). Perceptions, circumstances and motivators that influence implementation of zoonotic control programs on cattle farms. Prev. Vet. Med. 93, 276–285. doi: 10.1016/j.prevetmed.2009.11.005
Frössling, J., and Nöremark, M. (2016). Differing perceptions – Swedish farmers' views of infectious disease control. Vet. Med. Sci. 2, 54–68. doi: 10.1002/vms3.20
Ghimire, R., and Huang, W. C. (2016). Adoption pattern and welfare impact of agricultural technology: empirical evidence from rice farmers in nepal. J. South Asian Dev. 11, 113–137. doi: 10.1177/0973174116629254
Ghosh, R. K., Patil, V., and Tank, N. (2022). Participation dynamics in multiple-peril agricultural insurance: insights from India. Int. J. Disast. Risk Reduct. 70,102781. doi: 10.1016/j.ijdrr.2021.102781
Hennessy, D. A., and Wolf, C. A. (2018). Asymmetric information, externalities and incentives in animal disease prevention and control. J. Agric. Econ. 69, 226–242. doi: 10.1111/1477-9552.12113
Horrillo, A., Obregón, P., Escribano, M., and Gaspar, P. (2022). A biosecurity survey on Iberian pig farms in Spain: farmers' attitudes towards the implementation of measures and investment. Res. Vet. Sci. 145, 82–90. doi: 10.1016/j.rvsc.2022.02.017
Karlson, K. B., and Holm, A. (2011). Decomposing primary and secondary effects: a new decomposition method. Res. Soc. Stratif. Mobil. 29, 221–237. doi: 10.1016/j.rssm.2010.12.005
Lanyon, S. R., Anderson, M. L., and Reichel, M. P. (2015). Survey of farmer knowledge and attitudes to endemic disease management in South Australia, with a focus on bovine viral diarrhoea (bovine pestivirus). Aust. Vet. J. 93,157–163. doi: 10.1111/avj.12316
Li, Y., Che, H., and Wang, W. (2014). Research on the effect and influencing factors of public policy on harmless disposal subsidies–Based on an empirical analysis of 773 samples from 14 counties (districts) in Shanghai and Zhejiang provinces (cities). J. Xiangtan Univ. 38, 42–47.
Lin, L., Liu, Z., Du, Y., Su, S., and Zheng, Y. (2018). Psychological cognition of pollution prevention and control behavior of large-scale pig farmers and the effect of environmental regulation. Chin. J. Ecol. Agric. 26, 156–166.
Liu, H. (2021). Finding the way out to African swine fever: analysis of Chinese government's subsidy programs to farms and consumers. Comp. Ind. Eng. 160, 107543. doi: 10.1016/j.cie.2021.107543
Liu, M., Lu, Q., and Zhang, S. (2017). A study on farmers' satisfaction with highly pathogenic avian influenza compensation policy: based on the data of Shapo district in Zhongwei, Ningxia. J. Hunan Agric. Univ. 18, 77–85.
Liu, Y., Ruiz-Menjivar, J., Zhang, L., Zhang, J., and Swisher, M. E. (2019). Technical training and rice farmers' adoption of low-carbon management practices: the case of soil testing and formulated fertilization technologies in Hubei, China. J. Clean. Prod. 226, 454–462. doi: 10.1016/j.jclepro.2019.04.026
Mason-D'Croz, D., Bogard, J. R., Herrero, M., Robinson, S., Sulser, T. B., Wiebe, K., et al. (2020). Modelling the global economic consequences of a major African swine fever outbreak in China. Nat. Food. 1, 221–228. doi: 10.1038/s43016-020-0057-2
Ministry of Agriculture and Rural Affairs of the People's Republic of China (2010). Available online at: http://www.moa.gov.cn/was5/web/search?searchword=%E9%9D%9E%E6%B4%B2%E7%8C%AA%E7%98%9F&channelid=233424&prepage=10&orderby=-DOCRELTIME (accessed March 8, 2022).
MOA. (2020). Normalization of ASF Prevention and Control (Trial). Available online at: https://www.moa.gov.cn/govpublic/xmsyj/202008/t20200810_6350130.htm (accessed August 1, 2021).
Moya, S., Chan, K. W. R., Hinchliffe, S., Buller, H., Espluga, J., Benavides, B., et al. (2021). Influence on the implementation of biosecurity measures in dairy cattle farms: communication between veterinarians and dairy farmers. Prev. Vet. Med. 190, 105329. doi: 10.1016/j.prevetmed.2021.105329
NBS (2022). National Bureau of Statistics. Available online at: https://data.stats.gov.cn/easyquery.htm?cn=E0103 (accessed March 8, 2022).
Qiao, J., and Zhang, X. (2019). The impact of government intervention and moral responsibility on the performance of aquaculture waste management: From the perspective of farm households. J. China Agric. Univ. 24, 248–259.
Sahlström, L., Virtanen, T., Kyyrö, J., and Lyytikäinen, T. (2014). Biosecurity on finnish cattle, pig and sheep farms – results from a questionnaire. Prev. Vet. Med. 117, 59–67. doi: 10.1016/j.prevetmed.2014.07.004
Sayers, R. G., Sayers, G. P., Mee, J. F., et al. (2013). Implementing biosecurity measures on dairy farms in Ireland. Vet. J. 197, 259–267. doi: 10.1016/j.tvjl.2012.11.017
Simon-Grifé, M., Martín-Valls, G. E., Vilar, M. J., et al. (2013). Biosecurity practices in Spanish pig herds: perceptions of farmers and veterinarians of the most important biosecurity measures. Prev. Vet. Med. 110, 223–231. doi: 10.1016/j.prevetmed.2012.11.028
Tian, X., and Stephan, C. T. (2020). Economic consequences of African swine fever. Nat. Food 1, 196–197. doi: 10.1038/s43016-020-0061-6
Toma, L., Stott, A. W., Heffernan, C., Ringrose, S., and Gunn, G. J. (2013). Determinants of biosecurity behaviour of British cattle and sheep farmers—A behavioural economics analysis. Prev. Vet. Med. 108, 321–333. doi: 10.1016/j.prevetmed.2012.11.009
Wang, J., Liu, Z., and Pu, X. (2016). Analysis of the impact of policy cognition on the risk of improper handling of sick and dead pigs by pig farmers. China Rural Econ. 377, 84–95.
Wang, Y., Liu, G., Li, H., Chen, R., and Liu, S. (2015). Analysis of the influencing factors of farmers continuing to engage in live pig free-range breeding——Based on a survey of 25 counties (Cities, Districts) in Sichuan Province. China Rural Rev. 5, 85–96.
Wu, H., and Li, J. (2023). Risk preference, interlinked credit and insurance contract and agricultural innovative technology adoption. J. Innovat. Knowl. 8, 100282. doi: 10.1016/j.jik.2022.100282
Wu, L., Xu, G., and Yang, L. (2015). Research on the moderate pig breeding scale under the internalization of environmental pollution control costs. China Popul. Resour. Environ. 25, 113–119.
Xie, Z. (2022). The impact of policy insurance on the production recovery of farmers in Jiangsu Province under the background of African swine fever. Jiangsu Agric. Sci. 50, 229–233.
Xu, G., Sarkar, A., Qian, L., Shuxia, Z., Rahman, A., and Yongfeng, T. (2022). The impact of the epidemic experience on the recovery of production of pig farmers after the outbreak-evidence from the impact of African Swine Fever (ASF) in Chinese Pig Farming. Prev. Vet. Med. 15:105568. doi: 10.1016/j.prevetmed.2022.105568
Xu, T., Chen, H., Ji, Y., Qiao, D., and Wang, F. (2023). Understanding the differences in cultivated land protection behaviors between smallholders and professional farmers in Hainan Province, China. Front. Sustain. Food Syst 7, 1081671. doi: 10.3389/fsufs.2023.1081671
Yao, H., Zang, C., Zuo, X., Xian, Y., Lu, Y., Huang, Y., et al. (2022). Tradeoff analysis of the pork supply and food security under the influence of African Swine Fever and the COVID-19 Outbreak in China. Geogr. Sustainabil. 3, 32–43. doi: 10.1016/j.geosus.2022.01.005
Yu, T., and Yu, F. (2019). Analysis of the influence of the cognition of resource utilization of livestock and poultry breeding waste on the willingness of farmers to participate in the context of environmental regulation policy. China Rural Econ. 8, 91–108.
Zhang, Y., and Wu, X. (2012). Food safety and its control and pig farmers' micro-behavior: questionnaire survey based on pig farmers selling sick and dead pigs and epidemic reports. China Rural Econ. 7, 72–83.
Zhao, J. (2019). China needs long-term solutions for African Swine Fever. Sci. Bull. 3, 1469–1471. doi: 10.1016/j.scib.2019.08.015
Zhao, L., Wang, C., Gu, H., and Yue, C. (2018). Market incentive, government regulation and the behavior of pesticide application of vegetable farmers in China. Food Control 85, 308–317. doi: 10.1016/j.foodcont.2017.09.016
Zhou, K., Zheng, X., Long, Y., Wu, J., and Li, J. (2022). Environmental regulation, rural residents' health investment, and agricultural eco-efficiency: an empirical analysis based on 31 Chinese Provinces. IJERPH 19, 3125. doi: 10.3390/ijerph19053125
Keywords: government regulation, pig farmers, mediation effect model, biosecurity awareness, biosecurity measures
Citation: Li J, Yuan M, Wang H and Zhou K (2023) Government regulations, biosecurity awareness, and farmers' adoption of biosecurity measures: Evidence from pig farmers in Sichuan Province, China. Front. Sustain. Food Syst. 7:1106766. doi: 10.3389/fsufs.2023.1106766
Received: 24 November 2022; Accepted: 09 March 2023;
Published: 30 March 2023.
Edited by:
Liming Ye, Ghent University, BelgiumReviewed by:
Ian Jenson, University of Tasmania, AustraliaCopyright © 2023 Li, Yuan, Wang and Zhou. This is an open-access article distributed under the terms of the Creative Commons Attribution License (CC BY). The use, distribution or reproduction in other forums is permitted, provided the original author(s) and the copyright owner(s) are credited and that the original publication in this journal is cited, in accordance with accepted academic practice. No use, distribution or reproduction is permitted which does not comply with these terms.
*Correspondence: Kun Zhou, emhvdWt1bkBzdHUuc2ljYXUuZWR1LmNu
†These authors have contributed equally to this work and share first authorship
Disclaimer: All claims expressed in this article are solely those of the authors and do not necessarily represent those of their affiliated organizations, or those of the publisher, the editors and the reviewers. Any product that may be evaluated in this article or claim that may be made by its manufacturer is not guaranteed or endorsed by the publisher.
Research integrity at Frontiers
Learn more about the work of our research integrity team to safeguard the quality of each article we publish.