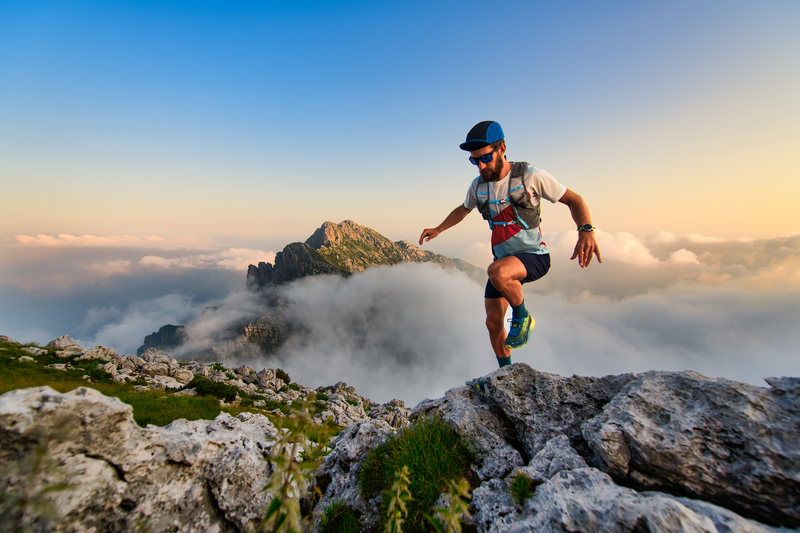
94% of researchers rate our articles as excellent or good
Learn more about the work of our research integrity team to safeguard the quality of each article we publish.
Find out more
ORIGINAL RESEARCH article
Front. Sustain. Food Syst. , 13 June 2023
Sec. Land, Livelihoods and Food Security
Volume 7 - 2023 | https://doi.org/10.3389/fsufs.2023.1098642
This article is part of the Research Topic Sustainable Intensification of Smallholder Farming Systems in Sub-Saharan Africa and South Asia View all 16 articles
Introduction: Efficiency in food production is crucial for sustainable agriculture in developing countries. This paper contributes to the existing literature by presenting an innovative approach to modeling productive efficiency in beef cattle production. Treating farm performance across regions as unobserved heterogeneity, we determine technical efficiency of beef cattle production in Botswana. We aim to shed light on the factors influencing efficiency in this sector.
Methods: The study utilized block-level data from various annual agricultural surveys (2006–2014) covering 26 agricultural districts and six agro-ecological regions in Botswana. We employed a latent class stochastic frontier model complemented with the stochastic meta-frontier analysis.
Results: Results show that the best performing farming systems in terms of efficiency are districts with well-developed infrastructure and better access to output and input markets. In contrast, the farming systems that perform poorly consist of agricultural districts without access to livestock advisory centers, with higher average temperatures and foot and mouth disease, limiting access to export markets. The mean technical efficiency scores for beef production for agricultural districts in class one and two were 62 and 59%, respectively, implying high potential to improve beef production using the same level of agricultural inputs through efficiency-enhancing investments.
Discussion: Based on our results, it is crucial for agricultural policies to prioritize regionally specific investments that address the needs of the under-performing districts. By targeting the lagging districts, policymakers can help beef producers improve their input efficiency and bridge the technological gaps to the meta-frontier. This can be achieved through investments in infrastructure, access to livestock advisory services, and disease control measures. Such efforts will not only enhance the efficiency of beef production but also contribute to the overall sustainability of the agricultural sector in Botswana.
With the goal of improving the productive efficiency of beef cattle production in developing countries and advancing the way efficiency can be modeled in empirical research, we present a latent class (finite mixture) stochastic meta-frontier analysis of beef cattle production in Botswana. Our objective is to examine technological heterogeneity and technical efficiency differences amongst beef cattle producing districts over time in the context of a developing country. Botswana is one of the few sub-Saharan African (SSA) countries that export beef to high-value markets such as the European Union. Livestock production plays a vital role in the rural economy and development of the country as a source of food, income, employment, and investment opportunities for most rural dwellers (van Engelen et al., 2013; Statistics Botswana, 2015). In 2019, the livestock sector contributed about 45% of the value-added in the agricultural sector while agriculture contributed 2.1% of the economy’s value added (Statistics Botswana, 2022). In addition, beef is the only contributor to foreign exchange earnings from the livestock sector. Therefore, understanding Botswana’s technical efficiency of the beef cattle production systems and its drivers could be vital to the future orientation of Botswana’s and similar countries’ livestock industries, rural economies, and associated policy targeting.
Considering the above, this paper makes the following contribution to the literature. We exploit the observed heterogeneity of beef farms to identify technologies endogenously, measure their efficiency, and determine their sources and drivers. We adopt a latent class model (LCM) that combines a stochastic frontier approach (SFA) with a latent class structure. Unlike the approach used by previous researchers on a similar subject (e.g., Bahta et al., 2015; Temoso et al., 2015b), whereby a precise prior classification of farms is not made, farms are clustered according to differences in production technology. In fact, previous studies used farm management data bases which may not capture well the observable characteristics of farm (Besstremyannaya, 2011). Thus, our motivation to using a latent class stochastic frontier analysis stems from the fact that a LCM simultaneously identifies potentially unobservable technological differences and measures technical efficiency thereby providing management aspects that distinguish the most efficient location from the least efficient location.
While the use of the latent class stochastic frontier model (LCSFM) has proven useful in studying selected agricultural sectors, e.g., dairy farms (Alvarez and del Corral, 2010; Alvarez et al., 2012; Orea et al., 2015); and crops (Baráth and Fertő, 2015), its empirical application to the beef cattle sector is, to date, limited to a few studies (Martinez-Cillero et al., 2019; Dakpo et al., 2021). Martinez-Cillero et al. (2019) used the approach to evaluate the technical efficiency and technological heterogeneity of Irish beef farms, whilst Dakpo et al. (2021) measured the productivity of intensive and extensive grazing livestock farming systems in France. All these studies have concluded that if unobserved technology heterogeneity is not accounted for to model technical efficiency, results may be biased and the policy implications from those studies would be misleading. We build on this literature by applying the LCSFM methods to the beef sector in Botswana to provide an empirical evidence basis to inform policy design for Botswana and other developing countries whose beef sectors are their economic and social development mainstay.
The livestock sector in developing countries is an excellent example where efficiency of livestock production is under-researched despite being an important agricultural sector that contributes considerably to their economies. Apart from being under-researched, a few studies that estimate the technical efficiency of livestock production systems generally assume farms operate under a homogeneous technology. However, as shown by previous studies (e.g., Alvarez and del Corral, 2010; Alvarez et al., 2012; Baráth and Fertő, 2015; Orea et al., 2015; Martinez-Cillero et al., 2019; Dakpo et al., 2021), assuming homogenous technology may lead to unreliable technical efficiency, productivity estimates, and policy recommendations.
Moreover, technical efficiency analysis of beef cattle production matters because agricultural policy in most countries like Botswana favors the livestock sector, especially beef, at the expense of crop production (Bahta and Malope, 2014; Temoso et al., 2015a). Botswana’s previous government initiatives include the Livestock Management and Infrastructure Development (LIMID) program, which promotes food security through improved productivity of cattle, small stock, and poultry [Ministry of Agriculture (MoA), 2010]; and the International Livestock Research Institute’s (ILRI) project.1 The ILRI project identified factors affecting the productivity of smallholder livestock farms and assessed their competitiveness and conditions for market participation and value addition (Bahta et al., 2013). International support to Botswana’s livestock sector includes international development agencies (e.g., The World Bank, 2016) and international development researchers (e.g., ILRI, the Australian Centre for International Agricultural Research). However, despite receiving key public policy support, most of the funds and human resources allocated to the livestock sector are directed mainly to monitoring disease outbreaks, conducting vaccination campaigns, and implementing the traceability system (LITS)2 (Bahta and Malope, 2014; Bahta et al., 2015). The remaining funds are spent on management advances that might boost productivity, such as improving input quality and allocation, technology adoption, training, and enhancing production efficiency and market access (Sigwele and Orlowski, 2015; Temoso et al., 2018).
Notwithstanding these efforts, scientific evidence suggests that the productive performance of beef cattle farms in developing countries (e.g., Botswana, Ghana, Kenya, and Zambia) is declining, with negative consequences on income and gross domestic products (Temoso et al., 2015b, 2018; Manyeki, 2020; Ankrah and Jiang, 2021; Odubote, 2022). For example, in Botswana, over the previous years, both off-take and birth rates remained below 10% except in 2013 and 2014, when the birth rate rose to 12.9 and 12.4%, respectively (Statistics Botswana, 2019). These findings suggest that there is potential to improve the performance of beef cattle in the traditional sector by scaling up both birth rates and off-take rates and reducing mortality rates. Beef productivity has been affected by various factors: recurring droughts; endemic animal diseases; biological inefficiencies (low birth rates and high mortality rates); inefficient operation of farms; slow adoption of improved breeding; and ineffective feeding approaches in the Botswana environment (van Engelen et al., 2013; Bahta and Baker, 2015; Temoso et al., 2015b; Statistics Botswana, 2019).
Scaling-up beef farmers’ performance may require identifying the unobserved technological differences between regions to fully effective policy targeting. Therefore, as part of our contribution to the literature, we build on the LCSFM framework by estimating a stochastic meta-frontier (SMF) production function that encompasses all class frontiers (covering regions) to account for potential inter-class variation in technology gaps in beef production. Instead of applying the LCSFM in conjunction with a deterministic meta-frontier as in Mekonnen et al. (2015), we adopt Huang et al.’s (2014) approach in the second stage whereby technology gap ratios are specified as a function of exogenous variables to account for group-specific environmental heterogeneity. While Mekonnen et al. (2015)’s study is close to ours, despite their novelty, Mekonnen et al.’s semi-parametric approach did not report any statistical properties of their meta-frontier estimates. A SMF approach mitigates this (Huang et al., 2014; Le et al., 2018). In particular, the SMF approach has an advantage over the deterministic meta-frontier approach in that its estimation uses a maximum likelihood function rather than mathematical programming and therefore is more suitable to separate potential random shocks from the technology gaps. Moreover, Mekonnen et al.’s (2015) study focuses on innovation systems in Africa while ours focuses on beef production systems in Botswana.
Several researchers have proposed methods that deal with technological heterogeneity (Battese et al., 2004; Greene, 2005; Kumbhakar et al., 2009). In the case of observed heterogeneity, the most commonly used approach has two stages. The first stage involves splitting the sample into groups based on prior information about farms and specific exogenous characteristics. In the second stage, different production functions are estimated for each group (e.g., see, Battese et al., 2004; Newman and Matthews, 2006). One such approach is the SFA-based meta-frontier, which has been applied in the dairy industry (Moreira and Bravo-Ureta, 2010) and in a few cases to beef production (e.g., Otieno et al., 2014; Bahta et al., 2015; Temoso et al., 2015b, 2016). Otieno et al. (2014) classified beef cattle farms in Kenya into three production systems (nomadic pastoralism, agro-pastoralism, and ranches). Bahta et al. (2015) delineated three farm types while Temoso et al. (2016) and De Ridder and Wagenaar (1984) considered two farm types of production systems. Further, Temoso et al. (2015b) defined different production technologies based on their location in agro-ecological regions. A typology based on communal versus freehold livestock farms was used in Barnes et al. (2008) and Mahabile et al. (2002).
While it is essential that these studies have examined the performance of Botswana’s livestock given the growing policy support, using partial measures of productivity (Abel, 1997; Behnke, 1985) has the limitation that input–output relationships are not attributed to either technologies or management. Analyses using more complete estimates of productivity that assume homogeneous production systems amongst farms have the limitation that productivity changes are associated with technology (Mahabile et al., 2002; Barnes et al., 2008). We are aware of only three studies (i.e., Bahta, 2014; Temoso et al., 2015b, 2016) that have measured the productivity of Botswana’s livestock production systems while accounting for technological differences amongst systems. Bahta et al. (2015) applied a deterministic meta-frontier approach to cross-sectional data from three major livestock producing districts (i.e., South East, Chobe, and Central). Three types of beef production systems were recognized, namely cattle only, cattle and crops, and mixed farms. Results featured a tendency for efficiency to be positively related to production system diversity. Farm system makeup is clearly associated with technology. Temoso et al. (2015b) adopted a similar approach to panel data from 26 agricultural districts representing all six agro-ecological regions in Botswana. Their study found that prevailing environmental conditions and economic development in a given region influence productivity and the production technologies employed. Temoso et al. (2016) measured the productivity gap between traditional and commercial beef production systems and found significantly different productivity and deployment of production technologies between the two systems.
Similarly, Melo-Becerra and Orozco-Gallo (2017) assessed the efficiency of crop and livestock production systems in Colombia. Considering the different production systems with regard to climate, geography, and soil types, Melo-Becerra and Orozco-Gallo (2017) built on previous research by using stochastic meta-frontier techniques. They found that farmers in some production systems were benefiting from better production conditions due to the available natural resources, climate, and more favorable socio-economic conditions.
The methods used by the papers discussed above have received some criticism. First, if the prior classification is not precise, the first stage is likely to generate errors, which will deliver biased and inefficient estimates of the technological parameters in the second stage (Kumbhakar et al., 2009). Another practical limitation of the two-stage approach is that researchers usually must consider a specific exogenous characteristic to divide the sample and estimate the separate frontiers. This is likely to lead to an incomplete division of the sample because firms included in separate groups may share some features (Alvarez and del Corral, 2010; Martinez-Cillero et al., 2019). Also, this essentially mirrors criticism of the use of technological indicators, as outlined above, as such groupings may be both arbitrary and incomplete.
A recommended approach to account for potential unobserved heterogeneity is one that searches for a finite number of structures (or “classes”) within the data (Alvarez et al., 2012). The latent class stochastic frontier model (LCSFM), sometimes referred to as a mixture of models, offers these facilities. In LCSFM, each firm or geographic location (district, country, region, etc.) can be assigned to a group using the estimated probabilities of possessing certain characteristics (separating variables) that are proxies for different technologies or production systems (Sauer and Paul, 2013). The LCSFM is suited to our empirical setting because livestock production in Botswana operates within a complex system (Bahta and Malope, 2014; Temoso et al., 2016), and the variation amongst farms may reflect a range of features such as breeds and genetics, soils and vegetation, land tenure systems, feeding systems, and disparities in the rate of uptake of new technologies.
Moreover, LCSFM is applicable where the researcher does not know ex-ante which farms belong to which particular production technology, nor the number of different technologies that exist in the sample (Mekonnen et al., 2015; Martinez-Cillero et al., 2019). Using panel data, this study extends the productivity analysis literature by combining the LCSFM with a stochastic meta-frontier analysis (in lieu of the deterministic meta-frontier). This is based on our conjecture that a stochastic meta-frontier envelops all the unobserved class frontiers thereby allowing us to account for potential inter-class variation in technology gaps, on an equally important sector for the global south – livestock production.
We utilized block-level data from various annual agricultural surveys (2006–2014) covering 26 agricultural districts and six agro-ecological regions. A block is a smallest geographical area unit defined by Statistics Botswana and form the building blocks for larger regions. The annual agricultural surveys are collected through a stratified sampling framework. According to Statistics Botswana (2018, p. 15), independent sample sizes were estimated for each individual block and added to give the total sample size at the agricultural district level, agricultural region level, and national level. In this study, 259 blocks within the traditional/communal beef cattle sector over a nine-year period (i.e., a total sample size of 2050) were used for analysis. This is a larger sample size than previous livestock studies in Botswana that have used aggregated data at district and regional levels (e.g., Temoso et al., 2016).
We focus on the traditional beef cattle production system because the majority (78%) of farmers and cattle population (78%) in Botswana fall under this system, and it contributes the largest share of output to the livestock sector (Statistics Botswana, 2018). In addition, the recent agricultural surveys (since 2012) focus on collecting data for the traditional sector while the commercial sector coverage has been low and as such the recent commercial farms data cannot be used to produce meaningful results to guide policy and decision making (Statistics Botswana, 2019).
Table 1 provides the definition, units, and summary measures of the production function variables, at the primary sample unit (PSU) level, that are used to explain technical inefficiency. For estimation, the dependent variable is beef output expressed in monetary value. Due to measurement difficulties, this study follows the revenue approach recently applied in the literature (Hong et al., 2019; Nguyen et al., 2021; Temoso et al., 2023) and defines output as:
where is the annual value of beef cattle output of the ith farm in the jth production system (measured in Botswana Pula3); R denotes any of the three forms of cattle output considered, i.e., current stock, sales or uses for other purposes in the past 12-month period; y is the number of beef cattle equivalents; p is the current price of existing stock or average price for cattle sold/used during the past 12 months; and t is the average maturity period for beef cattle in Botswana which, based on expert consultation, is assumed to be 4 years. Similarly, to ensure that the study captures the approximate share of feeds from different sources, the quantities of purchased and non-purchased (on-farm) feeds were first adjusted in accordance with the average annual number of dry and wet months,4 respectively, in the country.
Table 1. Descriptive statistics of weighted production function variables and inefficiency effects at the block level.
Average feed prices were computed using the survey’s price information collected for purchased feed with further validation by animal nutrition experts in the Department of Agricultural Research (DAR). Both purchased and non-purchased (the value of the latter is zero since no own grown feed is recorded in the database) feeds were then converted to improved feed equivalents by multiplying the respective feed quantities by the ratio of their prices (or shadow prices) to the average per-unit price of improved fodder. Thus, following Otieno et al. (2011) and Bahta et al. (2015), the total annual improved feed equivalent was computed as:
where; and S denote, respectively, the ratio of prices of purchased and non-purchased feed to that of improved fodder; and represent the average quantities of purchased and non-purchased feeds, respectively, in kilograms per month; d is the approximate number of dry months (when purchased feeds are mainly used), while w is the length of the wet season (when farmers mostly use on-farm or non-purchased feeds) in a particular area. The other input variables included in the first stage of the model are land, labor, herd size, and average annual precipitation. Except for precipitation, the sample weight is used to obtain a weighted value for the other variables in the production function.
As shown in Table 1, the average total value of beef cattle output is BWP 177,960. On the input side, the average land size – measured in terms of arable land in hectares – is 1.6 ha. Access to grazing land varies from communal lands where most smallholders graze their cattle freely to fenced lands, with an average land size per PSU of about 25 ha. The average total cost of labor per PSU is BWP 3,750. Agricultural labor measures the total labor cost, including permanent and temporary labor costs. Another key input variable is herd size, which reflects the stock size and is measured in beef cattle equivalents (Otieno et al., 2012; Bahta et al., 2015). We also included farm household veterinary costs. The average veterinary cost per PSU is BWP 10,226. The variable annual rainfall or precipitation is included to account for geographic variation and averages 427 mm.
The proportion of exotic breeds5 may indicate adoption rates of advanced breeds and their impact on productivity (Temoso et al., 2016). The effect on productivity of the exotic breeds (both the Zebu/indicus and taurus) could especially be on crossbreeding and improving the Tswana breed in terms of weight of an animal which is one of the most important criteria in beef production. Gross off-take rates6 refer to the ratio of livestock sold to the total number purchased and home slaughtered, whereby low off-take rates are associated with poor management and lack of marketing facilities (Temoso et al., 2016). The mortality rate is the ratio of the total number of deaths to the total number of livestock during the survey year. It is expected that farms with lower mortality rates are using better technologies and managing their livestock farms well and are likely to attain better productivity (Temoso et al., 2016).
The last rows in Table 1 contain descriptive statistics for the industry-specific environmental variables considered in this study. As mentioned before, industry-specific environmental variables go into the inefficiency term of the SMF model. Here, we consider access to livestock advisory centers (LAC) and mean temperatures recorded in all blocks within agricultural districts in Botswana during the period of analysis. The LACs are centers that provide livestock services required to help control and prevent livestock diseases. Access to LACs is important in Botswana as it provides animal health services through the sale of drugs, vaccines, and animal equipment as well as offering advisory services to farmers about animal health (Malope et al., 2016). However, access to LACs in Botswana is heterogeneous as some districts have more than one LAC center while others do not and this affects the livestock industry. Temoso et al. (2023) found that LACs affect Botswana’s livestock’s total factor productivity and its growth suggesting that access to LACs is appropriate to be considered an industry-specific environmental variable. In this study, access to LACs has the value of zero for blocks whose farmers had zero access to LACs and equals 1 for blocks whose farmers had access to at least one LAC. As for mean temperatures, Neibergs et al. (2018) suggest that temperature affects the growth of forage and timing of forage availability for grazing, which ultimately impacts stocking rates, turn-out dates for beef cattle. Thus, temperature may have important implications on the beef cattle production’s meta-frontier, especially that it may not be homogeneous across all blocks within districts in Botswana. Because of the broadness of access to LACs and mean yearly temperatures, we consider these variables as industry-specific environmental variables in stage two of our SMF estimations.
Following Orea et al. (2015) and Mekonnen et al. (2015), we specify a production function from the LCSFM as follows:
where i represents blocks, t indicates time, and j = 1,…, J stands for class: we assume that the blocks within agricultural districts being analyzed operate an unknown finite number of different technologies which underlie the sample data. The dependent variable, is a measure of a block’s output, represent a vector of input variables, is a noise term that follows a normal distribution with zero mean and class-specific constant variance, and is a class-specific one-sided error that captures the blocks’ inefficiency (geometrically, the distance between the observation and the production frontier) and is assumed to follow a one-sided distribution (half-normal in this study). The two error components are assumed to be independent of each other.
The associated likelihood function conditional on class j for a block i at time t is given by:
where , , and and represent the standard normal density and cumulative distribution functions (Greene, 2005). Then, the overall contribution of the block i to the conditional likelihood is:
The unconditional likelihood for block i is obtained as a weighted sum of its likelihood function across j class, where the weights (Pij) are the probabilities of class membership, or:
Then, the logarithm of the overall likelihood function can be obtained as the sum of the individual likelihood functions , where represent the frontier specific parameters to be estimated:
The prior class probabilities are parameterized as a multinomial logit model, to ensure that such that:
where represents the vector of block-specific but time-invariant variables that separate the blocks within the agricultural districts into different classes, and s the vector associated with parameters to be estimated (Mekonnen et al., 2015).
Maximizing the overall likelihood function specified in Equation 7 provides asymptotically efficient estimates of all parameters. It should be noted that unlike the two-stage procedures discussed above, LCSFM allows for all the observations in the sample to be used to estimate the underlying technology for each class (Martinez-Cillero et al., 2019). Each block within an agricultural district belongs to one and only one class, which implies that the probabilities of class membership in LCSFM merely reflect the uncertainty that researchers have about the true parameter (Orea et al., 2015). The estimated parameters in Equation 7 can be used to compute the posterior probabilities of class membership using the following expression:
The probability in Equation 9 is then used to allocate each block to the class with its highest posterior probability. Alvarez et al. (2006) noted that Equation 9 is time-invariant, implying that each block within the agricultural district is modelled in the same group over time is the posterior probability for a given block i to belong to technology class J. It depends on prior parameters of class membership and the estimated parameters of the production function (θ, λ, σ). Following Mekonnen et al. (2015), we apply Schwarz Bayesian Information Criterion (SBIC) and Akaike Information Criterion (AIC) to our data in order to determine the number of classes. BIC and AIC can be computed as follows:
where log LF(j) represents the log-likelihood function of the model with j classes, N is the number of observations, and K represents the number of parameters to be estimated. After the j production frontiers have been defined, the technical efficiency of a block i in the tth period for class-j production frontier can be estimated using the following equation:
One of the contributions of this study is, for the first time, to determine and compare the technical efficiency of beef cattle production across all the blocks and agricultural districts in Botswana. However, the efficiency score estimates across classes from Equation 12 are not directly comparable due to their either constituting different frontiers or different weights within frontiers. Following Mekonnen et al. (2015), this can be resolved by estimating a meta-frontier that incorporates all the class frontiers and facilitates efficiency comparison across all the blocks in all technology classes. A meta-frontier production function uses either panel or cross-sectional data to measure efficiency and production technological gaps (Battese and Rao, 2002; Battese et al., 2004), and the estimates of meta-frontiers are commonly used to compare relative efficiency scores of different classes or groups (Temoso et al., 2016).
Since its introduction by Ruttan (1971), several developments have led to two main ways in which a meta-frontier production function is estimated: a deterministic meta-frontier (O’Donnell et al., 2008) and a stochastic meta-frontier frontier (Huang et al., 2014). O’Donnell et al. (2008) deterministic meta-frontier is estimated by mathematical programming techniques which do not account for idiosyncratic shocks, and thus results are prone to random noise (Huang et al., 2014; Chang et al., 2015). To address this, Huang et al. (2014) proposed a stochastic meta-frontier (SMF) model that, rather than using mathematical programming techniques, uses econometrics to estimate meta-frontier parameters and account for random noise in the second stage. Because of this advantage, it is no surprise that several studies (e.g., Huang et al., 2015; Li et al., 2017; Melo-Becerra and Orozco-Gallo, 2017; Ng’ombe, 2017; Alem et al., 2019; Obianefo et al., 2021 among others) have used a SMF model. A SMF approach requires specifying technology gap ratios (TGRs) as a function of exogenous environmental variables and has desirable statistical properties for inference (Huang et al., 2014). Thus, in the second stage, this study builds on previous latent class frontier research (e.g., Mekonnen et al., 2015; Orea et al., 2015) by estimating a SMF to incorporate all the class frontiers to facilitate efficiency comparison of all the blocks within the agricultural districts in all technology classes in Botswana.
Following Huang et al. (2014), a meta-frontier production function that would underlie all latent class frontiers in the tth period is where j denotes classes. By definition, is the meta-frontier that envelopes individual class frontiers: . Their relationship is
where which means and that the ratio of the jth class’s production frontier to the meta-frontier is the technology gap ratio (TGR) expressed in Equation 14:
Based on Equation 14, a TGR value that equals one implies that the most advanced technology to produce outputs was employed. A TGR value of less than one implies that an economic unit of interest failed to adopt the most advanced technology, perhaps due to economic and/or environmental conditions (Huang et al., 2014; Ng’ombe, 2017). Therefore, Huang et al. (2014) consider that the technology gap component of is group-, block-, and time-specific and that it would depend on the adoption of the meta-frontier production technology available. Given any input level , a meta-frontier and a block’s observed output can be decomposed into three components as
The three components are, respectively, the ith block’s , technical efficiency, and random noise . While it is well known that and lie between 0 and 1, the meta-frontier does not necessarily envelope all economic agents’ observed outputs due to random noise. It is the unrestricted fraction in Equation 15 that differentiates modeling a meta-frontier by stochastic frontier analysis (SFA) from using data envelopment analysis (DEA). To account for random noise, Equation 15 can be rewritten as
where is a block’s technical efficiency with respect to the meta-frontier production technology, instead of jth latent class’s production technology. In terms of estimation, mathematical programming techniques would minimize the sum of squared deviations between and and the standard errors associated with meta-frontier parameter estimates would be obtained by bootstrapping and or simulation methods (Ng’ombe, 2017). But under Huang et al.’s (2014) approach, for estimation purposes, Equation 13 is re-specified as
The class-specific frontier is not observable, but its estimates are from the first step. In this study, it is from LCSFM. Since the fitted values of (i.e., and true frontier values are different, Equation 17 becomes
where is the statistical noise to characterize the deviation of from . This can be shown as
Equation 18 looks like the common stochastic frontier regression model and is therefore referred to as the SMF model. Following Huang et al. (2014), since can be obtained by maximum likelihood estimation, its estimates are consistent and asymptotically normally distributed. The error is assumed to be distributed as while is assumed to be distributed as , where are now group-specific environmental variables. Huang et al.’s (2014) method allow for the estimated latent-specific frontier to be greater than or equal to the meta-frontier due to the error in Equation 18. But by construction, the meta-frontier is always higher than the true latent class-specific frontier, i.e., (Huang et al., 2014). The estimated TGR becomes
where which is the estimated residual of Equation 16. In sum, we use the LCM frontier analysis in the first step and SMF approach in the second step because the latter allows the presence of leading to the estimated TGR in Equation 20 not being influenced by random shocks, unlike the deterministic programming method (Huang et al., 2014).
In the efficiency literature, there are generally two functional forms used to specify the production function: the Cobb Douglas function and translog function. In this study, the translog function is assumed for the production function, which has sufficient parameters to provide a second-order approximation (Coelli et al., 2005). Unlike the Cobb Douglas production function, the translog production function does not impose prior restrictions on the production technology and can handle a large number of inputs and treat them interactively. However, the flexibility of translog function comes at a cost – there are more parameters to estimate, and this may give rise to econometric difficulties such as multicollinearity (Coelli et al., 2005) as well as failure to satisfy its basic theoretical restrictions (i.e., positivity, linear homogeneity, curvature, and monotonicity). However, the translog functional form remains the most widely used form in literature (Serletis and Feng, 2015). Our specification of the translog production function is:
where the β’s and δ’s are parameters to be estimated and k is the kth group or block. Whilst subscript i denotes the blocks within the agricultural district, t is the linear trend that accounts for neutral technical change, j denotes the different classes to be estimated, and whilst y and x are the logarithms of beef output and a vector of inputs, respectively. For consistency, we also assumed the translog functional form for the SMF model. As with the distribution of the inefficiency term, for consistency, a half-normal distribution is also assumed in the second step.
Estimation proceeded as follows. In the first stage, we estimated the LCSFM from which class j’s fitted value of output for the ith block in period t were pooled. Here technical efficiency scores associated with each class were estimated. In the second stage, we used the pooled fitted values from stage one for each class to estimate Equation 16. As mentioned before, we used access to LACs and the mean temperature per year in degrees Celsius that Botswana recorded during the period of analysis. Notice that following Wang and Schmidt (2002), Ng’ombe and Kalinda (2015), and Yu and Jaenicke (2020), the frontier and inefficiency part of the model in both first and second stage were estimated simultaneously to detour from inconsistency that comes with the two-stage approach whereby the frontier and inefficiency functions are estimated separately.
In this study, we determine the number of classes into which the blocks within the agricultural districts could be classified, using the Akaike Information Criteria (AIC) and Bayesian Information Criteria (BIC) (Orea and Kumbhakar, 2004; Alvarez and del Corral, 2010). The AIC was relatively the lowest for LCSFM with two classes, thus implying that it is the preferred model over the LCSFM with three classes. Table 2 presents the maximum likelihood estimates for the parameters in the stochastic production frontier. All estimated first-order parameters in the LCSFM fall between zero and one in both classes and the pooled frontier, thus satisfying a monotonicity condition that all marginal products are positive and diminishing at the mean inputs (with the exception of veterinary expenditure in Class 2).
The elasticity of output with respect to labor and arable land are both positive and statistically significant for both classes and larger than for any other input. A possible explanation for this could be that farmers who have more arable land are more likely to have more crop residues that they can use to supplement their animals and thus reduce feed costs (Bahta and Baker, 2015). Veterinary expenditure was significant for Class 2 and the pooled model but not for Class 1. Across classes (and the pooled model), the livestock feed variable was not significant. In general, our analysis shows that variables related to labor and inputs show more significance in the Class 2 model. Arable land and labor are more significant in the Class 1 model. This suggests a more intensive oriented production system in Class 1 than Class 2. The pooled model demonstrates a broader pattern of significance, but the two class models depart from it substantially, which suggests that the underlying technologies are different both from the pooled model and from each other.
Determinants of technical inefficiency are presented in Table 3: a negative coefficient indicates that the variable has a positive effect on TE. The table shows that all candidate determinants of technical efficiency are negative and statistically significant for the pooled model (except artificial insemination and mortality rate variables). In Class 1, all variables (except transport facility and crop income variables) were significant. However, in Class 2, a smaller set of variables [gender, exotic breed, cattle population (herd size), crop income, and gross off-take rates] were significant.
The coefficients for education and training are negative and significant for the pooled model and Class 1, from which we infer that blocks/agricultural districts with relatively more farmers with some formal schooling or having received agricultural training tend to be technically efficient. This may be due to farmers with more education responding more readily by adopting new technologies (Temoso et al., 2023). These results are consistent with Bahta and Malope (2014), who found a positive relationship between education and productive efficiency amongst the smallholder beef farmers in Botswana but contradicts Otieno et al. (2014), who found that smallholder farmers with formal education and higher income in Kenya, were relatively less efficient. The coefficient of gender is negative and statistically significant. Male farmers may generally be more efficient than female farmers in beef production possibly due to more access to resources, greater exposure to, and experience of livestock management in Botswana.
In both classes, we found a negative and significant coefficient of the use of exotic breeds, which indicates that the higher the proportion of exotic breeds, the more efficient the agricultural district. These results show that having fewer indigenous cattle breeds and more crossbreeds are likely to lead to higher beef production efficiency. Crossbreeds between exotic and indigenous cattle have the potential to improve productivity and their suitability to the adverse production environments compared to the indigenous breeds. Wollny (2003) points out that controlled cattle breeding could increase efficiency through the improvement of genetic quality, enhancing the adaptation of cattle to environmental conditions, and ensuring an optimum stocking rate to feed supply within and between years.
Herd size (cattle population) has a negative and significant effect, which implies farmers owning larger herds are more technically efficient than those owning small herds. The direction of the effects is consistent with a priori expectations. The results suggest that there is scope to increase productive efficiency by increasing herd size. However, such a strategy would need to be done with consideration of other factors such as the availability of suitable grazing and water, and local environmental conditions, the management in place in communal grazing areas.
The coefficient of crop income is negative and statistically significant for the pooled sample and Class 1, which implies earning income from crop production improves the technical efficiency of farmers. As observed by Bahta and Malope (2014, p. 415), “the results suggest that income from crop farming is being reinvested into livestock farming, and/or that there are other synergies between the two farm activities including the use of crop residues as feed resources.”
The coefficient for the availability of transport is negative and significant only for the pooled model, which implies that having access to a transport facility has the potential to improve production efficiency. These results imply that those farmers with access to transport facilities can conveniently access distant markets in search of better sale prices for their output and also access key inputs such as veterinary medicine and supplementary feeds. This effect appears to be generally applicable to all cattle farms but is not reflected as specific to either of the two classes.
The coefficient of gross off-take rate (the ratio of livestock sold to the total number purchased and home slaughtered) is negative, indicating efficiency gains from an increase in gross off-take rates. Temoso et al. (2016) found similar results for traditional farmers and argued that they are due to farmers’ selling their animals to meet their immediate cash needs and during drought seasons as a drought risk management strategy.
Moreover, Table 4 presents the parameter estimates of the SMF model. All of the coefficients of the individual inputs are positive and significantly different from zero and imply that, on average, an extra unit of each of these inputs positively affects the technology gap ratios (TGRs) of beef production in Botswana. When they enter the production function as squared values of their original form, most of the inputs (except) feed have negative and statistically significant coefficients. However, when used interactively, some of the inputs affect TGRs of beef production positively or negatively to highlight heterogeneous effects of inputs on how far districts in each class may be off the country’s stochastic meta-frontier.
With regard to industry-specific environmental variables, average temperature and access to LACs are significantly different from zero at 1 and 5% significance levels, respectively. The negative coefficient on access to LACs implies that districts whose beef cattle farmers have access to LACs operate closer to the meta-frontier production function than those that do not have access to LACs. This is plausible because, through LACs, such services as the purchase of drugs, vaccines, animal equipment, and animal health advice are available to an agricultural district (Malope et al., 2016) and enabling such areas to operate near the frontier. On the other hand, we find that an increase in average temperature would result in a district operating far from its beef cattle production frontier. In sum, findings from industry-specific environmental variables imply that higher temperatures would result in the district’s technology at beef cattle production being inferior. However, districts whose farmers have access to LACs operate with superior technology to those without.
Generally, the agricultural blocks in latent Class 1 are more technically efficient than those in latent Class 2. The mean technical efficiency scores for beef production between 2006 and 2014 for the blocks in Class 1 and Class 2 are 0.616 and 0.591, respectively. While the difference in mean technical efficiency scores is small, it is significantly different from zero at a 1% significance level as indicated by the t statistics in Table 5. These results imply that the blocks in Class 1 may be more homogenous (Mekonnen et al., 2015) and on the same path toward their frontier further than those in Class 2. In addition, these results imply that there is high potential in both blocks in Class 1 and Class 2 to increase beef production output by 38.4 and 40.9%, respectively, using the same amount of inputs. With regard to MTE, its mean values for districts in Classes 1 and 2 are, respectively, 0.563 and 0.528 and the mean difference between them is significantly different from zero. These mean scores imply that, on average, districts in Class 1 are significantly closer to the industry’s beef production potential than their counterparts in Class 2. That is, beef producers in Class 2 would have to increase their production levels to close the gap with the industry’s potential and their counterparts in Class 1.
Our results are not directly comparable to previous estimates from Bahta et al. (2015) and Temoso et al. (2016), which may have been overestimated if technology heterogeneity is present in the sample but not accounted for in the estimation process. Based on the highest posterior probability, the model classified 125 blocks as Class 1 and the remaining 136 blocks as Class 2. It is noted that some agricultural districts have blocks that belong to either Class 1 or 2. Thus, a 50% frequency percentage was used as a cut point to classify the agricultural districts into either Class 1 or 2.7 The minimum posterior probability of belonging into either class is 0, whereas the maximum is 1 and 0.99 for classes 1 and 2, respectively. For the agricultural districts which belong to Class 1, the average posterior probability of belonging to class one is 99.6%, whereas, for those that are categorized as Class 2, it is about 96%.
Figure 1 provides the average Beef Technical Efficiency (TE) Scores of Agricultural Districts in Botswana, 2006 to 2014 (the average technical efficiency scores of beef production for Classes 1 and 2 agricultural districts are also presented in Appendix 1.1). In Class 1, the top five performing agricultural districts are, respectively, Gantsi, Hukuntsi, Letlhakane, Tutume, and Selebi Phikwe districts, while the three lowest performers are Ngamiland west, Kweneng South, and Barolong, respectively.
Figure 1. Average Beef Technical Efficiency (TE) Scores of Agricultural Districts in Botswana, 2006–2014.
These results possibly reflect livestock production specialization of those agricultural districts, where cattle production is the most dominant agricultural activity, followed by goats and sheep farming (Statistics Botswana, 2015). Moreover, these are the districts with a relatively large number of commercial farmers (e.g., Sandveld Ranches), so the results may indicate technology spillovers (i.e., adoption of better breeds and livestock management, etc.) from commercial farmers in those regions. For Class 2, the highest performers are Kweneng West and Bobonong, while the lowest performers are Kweneng South and Ngwaketse South (Figure 1 and Appendix 1.1).
Figure 2 presents the technology gap ratio scores (TGR) and meta-technical efficiency (MTE) scores, which are estimated with respect to the stochastic meta-frontier (SMF) that encompasses all the class stochastic frontiers, thus allowing direct comparison of the efficiency of a given agricultural district to any other agricultural district in Botswana. The TGR measures the technological gap faced by an agricultural district in each class when their performance is compared against any agricultural district in the sample. A higher (lower) TGR implies a smaller (larger) technology gap between the class frontier and the meta-frontier. A value of 1 (100%) is equivalent to a point where the class frontier coincides with the meta-frontier.
According to Figure 2 and Appendix 1.2, on average, Class 1 agricultural districts have superior beef production technology to Class 2 agricultural districts. Amongst Class 1 agricultural districts, Kweneng South, Selebi Phikwe, Borolong, Sorrow, Letlhakane, Serowe, Tutume, Mahalapye West, Plapye, and Tutume have the highest beef farming technology (TGR of more than 0.9), whilst Gantsi and Hukuntsi have the least beef farming technology (TGR of 0.87). In Class 2, Mahalapye East, Kgatleng, Chobe, and Ngwaketse West have the highest beef production technology (TGR more than 0.9), whilst Tati and Tonota have the least (TGR of 0.81–0.84). Figure 2 and Appendix 1.3 also shows that the technical efficiency score relative to the meta-frontier (available beef production technology) for Class 1 is, on average, higher than for Class 2 (Appendix 1.4). Overall, the top-performing agricultural districts in Botswana are Letlhakane, Gantsi, and Hukuntsi. Whilst, Barolong, with higher TGR, recorded relatively lower meta technical efficiency. Although Barolong district was traditionally a mixed farming area, in recent times, arable agriculture has become the mainstay of the area’s economy. This shift of farmers from beef cattle farming to arable agriculture may explain the lower performance of this district as compared to the other livestock specialization districts such as Gantsi and Hukuntsi.
The previous section reveals the existence of clear significant differences in beef production technology between Class 1 and Class 2 agricultural districts. Table 5 below further illustrates this difference, with Class 1 districts significantly outperforming Class 2 districts in all efficiency estimates.
A major reason for the difference in beef production technology is resource endowment. Most parts of Botswana are disadvantaged by unfavorable environmental conditions, and the performance of different sectors within agriculture is closely related to these conditions (Burgess, 2006). For example, a descriptive analysis showed that the average temperature in Class 1 is 21.5 degrees Celsius while it is 22.3 degrees Celsius in Class 2, whose difference is statistically significant. Most importantly, and not coincidentally, our SMF model results indicated that higher temperature affects productive efficiency of the district, and the higher temperatures recorded in Class 2 adds to a long list of plausible reasons for inferior productive efficiency in Class 2. Class 1 districts are mainly composed of livestock specialized districts (e.g., Gantsi, Hukuntsi, Tsabong, Mahalapye West, and Letlhakane), whilst Class 2 is composed of agricultural districts suitable for crop production (e.g., Chobe, Mahalapye East, and Tati) (Burgess, 2006; van Engelen et al., 2013). In addition, the least performing agricultural Class 2 districts are located in wildlife areas, foot-and mouth disease (FMD)-endemic areas, and FMD-intensive surveillance zones.
Another reason for the difference in productivity is that the more productive agricultural districts tend to have large commercial farms that employ improved livestock farming practices. In addition, smallholder farmers operating in the vicinity of these large commercial farms benefit from technology spillovers from these commercial farms. These findings are consistent with evidence from Southern Africa (e.g., Thirtle et al., 1993; Temoso et al., 2016). Moreover, a simple descriptive analysis indicates that at least 91% of farmers located in Class 1 have access to livestock advisory centers (LACs), while only 72% have access to LACs. We found access to LACs to have the potential to improve beef cattle production efficiency is a plausible justification for this difference in efficiency statistics between the two classes.
Other more productive agricultural districts such as Selebi Phikwe, Palapye, and Tutume are located in the Eastern part of the country where Government of Botswana agricultural policies and investment in agricultural infrastructure have produced better road networks, access to markets and information, and proximity to extension services and the main export abattoirs in Francistown and Lobatse (Temoso et al., 2015a,b).
The implication of these results is that the best performing agricultural districts have either benefited from enabling government agricultural policies (e.g., access to LACs) or have the resources to develop, adapt and implement good livestock production practices. By virtue of their existence in FMD zones and unfavorable mean temperatures, the least performing agricultural districts may be deprived of enabling government livestock development policies. This situation can be reversed with the adoption of a commodity-based trade approach that allows greater integration of FMD-free with endemic zones (Rich and Perry, 2011; Naziri et al., 2015; Rich and Bennett, 2019).
These differences suggest the presence of clearly differentiated technologies among smallholder beef producers in Botswana and hence where policies to improve productivity could be focused. This study shows that providing livestock farmers with relevant livestock extension and better access to markets would facilitate better use of available technology by the majority of farmers, who currently produce sub-optimally. Possible essential interventions would include improving farmer access to LACs to help or speed up the provision of appropriate knowledge on animal husbandry, such as cattle feeding methods, disease monitoring, and breeding (Bahta et al., 2015). Chronic under-investment in the livestock sector is a significant constraint to the livestock sector. Therefore, it is crucial for the livestock stakeholders in Botswana, particularly the department of animal production, to make a case for sustainable livestock investments. The country needs to improve the evidence base by adopting livestock master plans, which provide crucial evidence that livestock ministers often lack regarding returns on investment (Bahta et al., 2020). Such evidence is essential to get financial resources for livestock development from ministries of finance, donors, as well as public and private investors.
This study has employed a latent class stochastic frontier model (LCSFM) complemented with the stochastic meta-frontier (SMF) to identify different technology models for Botswana’s beef production and then assessed production efficiency within those models. This advances on previous studies of efficiency, particularly for agriculture and developing countries, in that the technologies were not imposed a priori but rather emerged from the analysis.
We conclude that there are differences in technologies applied in Botswana’s beef industry that led to differences in inefficiencies across agricultural districts. To our knowledge, we provide the first quantitative measures of these effects (TGR measures and average technical efficiency scores) applied to Botswana and to extensive livestock production systems. We find that the majority of Botswana’s agricultural districts that perform better at beef cattle production are those located in areas where well-developed infrastructure and access to both output and input markets exist. Although unsurprising, this result’s strong confirmation lends support to its advocacy on other subjects. The centrality of infrastructure and market access to production efficiency, valid across all technologies, supports calls for improvements in all production areas.
The demonstrated use of differentiated beef production technologies by agricultural districts lends support to the importance of correctly accounting for heterogeneity in order to make appropriate policy recommendations regarding beef production and performance. The results of the study indicate that amongst factors of production, the beef output is positively related to the availability of labor, the size of arable land areas, feed availability and herd size. These result advocates for the implementation of policies that promote ownership of arable land in which farmers can plant fodder and or other crops, from which they could use residues to feed their livestock.
Agricultural districts that were found to perform poorly in terms of efficiency are mostly those without access to LACs, higher mean temperatures as well as where there is an occurrence of foot and mouth disease, which limits access to the Botswana Meat Commission (BMC) abattoirs. These agricultural districts also have large herds of wildlife, which leads to conflicts between wildlife and livestock keepers as the wildlife kills their livestock, and, in some cases, there are carriers of the FMD virus. The implication of this is that better ways of minimizing conflicts between wildlife and livestock should be found in order to improve beef production efficiency.
The use of improved breeds (exotic and crossbreeds) was found to positively influence efficiency, and this result is valid across the technologies identified. Hence uptake and controlled cattle breeding practices should continue to be encouraged for all production systems, as it has the potential to improve efficiency through the improvement of genetic quality and to enhance adaptation of cattle to environmental conditions. Similarly, the study has also shown that beef production efficiency is positively associated with levels of formal education across all production systems, and hence policies that address education and training of smallholder farmers should be pursued.
We find that animal mortality is associated with reduced beef production efficiency. Offtake, although a contributor to efficiency, is also likely to indicate forced management procedures, perhaps as an alternative to mortality where sales are infeasible or carried out too late. These results also apply across the technology spectrum and advocate for improvement in animal husbandry, alongside better trading conditions and raised marketing skill levels with a focus on closing the technology investments in livestock development between the two classes.
Amongst substantial variation within the classes, the mean technical efficiency scores for Classes 1 and 2 are 62 and 59%, respectively. We infer that there is scope for improvement in production without using additional inputs. This study has provided insights into possible mechanisms for improving beef cattle production by identifying variation in efficiency statistics between districts while unearthing reasons for possible discrepancies between the localities and contexts. Several topics for future research are apparent, including the study of the detail of spillovers between production systems and the enhanced definition of outputs to include environmental products and contributions to sustainability.
The original contributions presented in the study are included in the article/Supplementary material, further inquiries can be directed to the corresponding author.
SB contributed to conceptualization, investigation, data curation, resources, methodology, writing, editing, and review. OT contributed to conceptualization, investigation, methodology, editing, review, and supervision. JNN contributed to investigation, methodology, writing, editing, and review. KR, DB, SK, and PM contributed to conceptualization, investigation, methodology, writing, editing, and review. All authors read and approved the final manuscript.
This research was conducted as part of the CGIAR Initiative Sustainable Animal Productivity for Livelihoods, Nutrition and Gender Inclusion (SAPLING) and is supported by contributors to the CGIAR Trust Fund.
We gratefully acknowledge the assistance and collaboration of the Botswana Ministry of Agriculture, particularly the Agricultural Statistics Division and Land Utilization Division, and the cooperation of the Botswana Institute of Development and Policy Analysis (BIDPA). Our thanks also go to Stephen Oloo (ILRI), who helped with mapping. The opinions expressed here belong to the authors and do not necessarily reflect those of the SAPLING or CGIAR. The content of this manuscript has been presented, in part or analysis with fewer degrees of freedom or data aggregated at the district level, [at the International Association of Agricultural Economists (IAAE) triennial conference held on July 28- August 2, 2018, Vancouver, British Columbia], [Bahta, S., Temoso, O., Mekonnen, D., Malope, P., and Staal, S. (2018). Technical efficiency of beef production in agricultural districts of Botswana: A Latent Class Stochastic Frontier Model Approach].
The authors declare that the research was conducted in the absence of any commercial or financial relationships that could be construed as a potential conflict of interest.
All claims expressed in this article are solely those of the authors and do not necessarily represent those of their affiliated organizations, or those of the publisher, the editors and the reviewers. Any product that may be evaluated in this article, or claim that may be made by its manufacturer, is not guaranteed or endorsed by the publisher.
The Supplementary material for this article can be found online at: https://www.frontiersin.org/articles/10.3389/fsufs.2023.1098642/full#supplementary-material
1. ^Competitive smallholder livestock in Botswana-http://surl.li/ajclx.
2. ^The LITS and disease control programs are both for export markets ‘access, particularly the European Union (EU) (Bahta et al., 2015).
3. ^One Botswana Pula is on average 0.083 USD (Yahoo Finance 2022).
4. ^Botswana is an arid country and according to expert information the length of the wet season when farmers mostly use on-farm or non-purchased feeds do not exceed 5 months. Consequently, the study uses 5 wet and 7 dry months, respectively.
5. ^The data from statistics Botswana aggregates all improved breeds as exotic breeds, hence not possible to evaluate the variation in terms of performance among different exotic breeds.
6. ^Gross off-take is calculated following Negassa and Jabbar (2008) as Gross Commercial offtake rate = Sales 0.5 (Opening stock+Ending stock)*100.
7. ^Hukuntsi and Ngwaketse North agricultural districts are exceptions since an equal number of blocks belong to Class 1 and 2, a score of exactly 50/50 or a cut point of 50%. A decision to delineate these agricultural districts to either class 1 or 2 was made by comparing the average technical efficiency scores.
Abel, N. (1997). Mis-measurement of the productivity and sustainability of African communal rangelands: a case study and some principles from Botswana. Ecol. Econ. 23, 113–133.
Alem, H., Lien, G., Hardaker, J. B., and Guttormsen, A. (2019). Regional differences in technical efficiency and technological gap of Norwegian dairy farms: a stochastic meta-frontier model. Appl. Econ. 51, 409–421. doi: 10.1080/00036846.2018.1502867
Alvarez, A., and del Corral, J. (2010). Identifying different technologies using a latent class model: extensive versus intensive dairy farms. Eur. Rev. Agric. Econ. 37, 231–250. doi: 10.1093/erae/jbq015
Alvarez, A., Amsler, C., Orea, L., and Schmidt, P. (2006). Interpreting and testing the scaling property in models where inefficiency depends on firm characteristics. J. Product. Anal. 25, 201–212.
Alvarez, A., del Corral, J., and Tauer, L. W. (2012). Modeling unobserved heterogeneity in New York dairy farms: one-stage versus two-stage models. Agric. Resour. Econ. Rev. 41, 275–285. doi: 10.1017/S1068280500001258
Ankrah, T. M., and Jiang, Y. (2021). The impact of climate change coping and adaptation strategies on livestock farmers’ technical efficiency: the case of rural Ghana. Environ. Sci. Pollut. Res. 28, 14386–14400. doi: 10.1007/s11356-020-11525-1
Bahta, S. (2014). Technical efficiency in beef cattle production in Botswana: a stochastic meta-frontier approach. Paper Presented at the Tropentag, Prague, Czech Republic.
Bahta, S., and Baker, D. (2015). Determinants of profit efficiency among smallholder beef producers in Botswana. Int. Food Agribusin. Manag. Rev. 18:107. doi: 10.22004/ag.econ.208497
Bahta, S., Baker, D., Malope, P., and Katijuongua, H. (2015). A metafronteir analysis of determinants of technical efficiency in beef farm types: an application to Botswana. Paper presented at the 2015 conference, August 9–14, 2015, Milan, Italy.
Bahta, S., Baker, D., Podisi, B., and Marobela, O. (2013). Competitive smallholder livestock in Botswana: results of a livestock value chain survey in the Central District of Botswana. Retrieved from Gaborone, Botswana.
Bahta, S., and Malope, P. (2014). Measurement of competitiveness in smallholder livestock systems and emerging policy advocacy: an application to Botswana. Food Policy 49, 408–417. doi: 10.1016/j.foodpol.2014.10.006
Bahta, S., Rich, K.M., and Baltenweck, I. (2020). Why a livestock master plan? ILRI brief. Nairobi, Keya: ILRI.
Baráth, L., and Fertő, I. (2015). Heterogeneous technology, the scale of land use, and technical efficiency: the case of Hungarian crop farms. Land Use Policy 42, 141–150. doi: 10.1016/j.landusepol.2014.07.015
Barnes, J. I., Cannon, J., and Macgregor, J. (2008). Livestock production economics on communal land in Botswana: effects of tenure, scale and subsidies. Dev. South. Afr. 25, 327–345. doi: 10.1080/03768350802212121
Battese, G. E., and Rao, D. S. P. (2002). Technology gap, efficiency, and a Stochastic metafrontier function. Int. J. Bus Econ. 1, 87–93.
Battese, G., Rao, D. P., and O’Donnell, C. J. (2004). A meta-frontier production function for estimation of technical efficiencies and technology gaps for firms operating under different technologies. J. Prod. Anal. 21, 91–103. doi: 10.1023/B:PROD.0000012454.06094.29
Behnke, R. H. (1985). Measuring the benefits of subsistence versus commercial livestock production in Africa. Agric. Syst. 16, 109–135. doi: 10.1016/0308-521X(85)90003-4
Besstremyannaya, G. (2011). Managerial performance and cost efficiency of Japanese local public hospitals: a latent class stochastic frontier model. Health Econ. 20, 19–34. doi: 10.1002/hec.1769
Burgess, J. (2006). BOTSWANA: Country Pasture/Forage Resource Profiles. Accessed June 2, 2022 from http://www.fao.org/ag/agp/agpc/doc/countprof/PDF%20files/Botswana.pdf.
Chang, B. G., Huang, T. H., and Kuo, C. Y. (2015). A comparison of the technical efficiency of accounting firms among the US, China, and Taiwan under the framework of a stochastic metafrontier production function. J. Prod. Anal. 44, 337–349. doi: 10.1007/s11123-014-0397-8
Coelli, T. J., Rao, D. S. P., O’Donnell, C. J., and Battese, G. E. (2005). An introduction to efficiency and productivity analysis. New York: Springer Science and Business Media.
Dakpo, K. H., Latruffe, L., Desjeux, Y., and Jeanneaux, P. (2021). Latent class modelling for a robust assessment of productivity: application to French grazing livestock farms. J. Agric. Econ. 72, 760–781. doi: 10.1111/1477-9552.12422
De Ridder, N., and Wagenaar, K. (1984). A comparison between the productivity of traditional livestock systems and ranching in eastern Botswana: publisher not identified. ILCA Newsletter. Vol 3, 5–7.
Greene, W. (2005). Reconsidering heterogeneity in panel data estimators of the stochastic frontier model. J. Econ. 126, 269–303. doi: 10.1016/j.jeconom.2004.05.003
Hong, Y., Heerink, N., Zhao, M., and van der Werf, W. (2019). Intercropping contributes to a higher technical efficiency in smallholder farming: evidence from a case study in Gaotai County, China. Agric. Syst. 173, 317–324. doi: 10.1016/j.agsy.2019.03.007
Huang, T. H., Chiang, D. L., and Tsai, C. M. (2015). Applying the new metafrontier directional distance function to compare banking efficiencies in central and eastern European countries. Econ. Model. 44, 188–199. doi: 10.1016/j.econmod.2014.10.029
Huang, C. J., Huang, T. H., and Liu, N. H. (2014). A new approach to estimating the metafrontier production function based on a stochastic frontier framework. J. Prod. Anal. 42, 241–254. doi: 10.1007/s11123-014-0402-2
Kumbhakar, S. C., Tsionas, E. G., and Sipiläinen, T. (2009). Joint estimation of technology choice and technical efficiency: an application to organic and conventional dairy farming. J. Prod. Anal. 31, 151–161. doi: 10.1007/s11123-008-0081-y
Le, V., Vu, X. B. B., and Nghiem, S. (2018). Technical efficiency of small and medium manufacturing firms in Vietnam: A stochastic meta-frontier analysis. Econ. Anal. Policy 59, 84–91.
Li, H. Z., Kopsakangas-Savolainen, M., Xiao, X. Z., and Lau, S. Y. (2017). Have regulatory reforms improved the efficiency levels of the Japanese electricity distribution sector? A cost metafrontier-based analysis. Energy Policy 108, 606–616. doi: 10.1016/j.enpol.2017.06.032
Mahabile, M., Lyne, M., and Panin, A. (2002). Factors affecting the productivity of communal and private livestock farmers in southern Botswana: a descriptive analysis of sample survey results. Agrekon 41, 326–338. doi: 10.1080/03031853.2002.9523601
Malope, P., Mmopelwa, D., and Bahta, S. (2016). Factors influencing the choice of a service provider between the public and private sector in the delivery of animal health services in Botswana. Unpublished Working Paper.
Manyeki, J. K. (2020). Microeconometric models of the livestock sector: a farm-level analysis of Southern rangelands of Kenya (Doctoral dissertation, szte). Szeged, Hungary: University of Szeged.
Martinez-Cillero, M., Thorne, F., Wallace, M., and Breen, J. (2019). Technology heterogeneity and policy change in farm-level efficiency analysis: an application to the Irish beef sector. Eur. Rev. Agric. Econ. 46, 193–214. doi: 10.1093/erae/jby028
Mekonnen, D. K., Spielman, D. J., Fonsah, E. G., and Dorfman, J. H. (2015). Innovation systems and technical efficiency in developing-country agriculture. Agric. Econ. 46, 689–702. doi: 10.1111/agec.12164
Melo-Becerra, L. A., and Orozco-Gallo, A. J. (2017). Technical efficiency for Colombian small crop and livestock farmers: a stochastic metafrontier approach for different production systems. J. Prod. Anal. 47, 1–16. doi: 10.1007/s11123-016-0487-x
Ministry of Agriculture (MoA). (2010). Livestock management and infrastructure development (LIMID) phase II programme. Gaborone: Ministry of Agriculture, Botswana.
Moreira, V. H., and Bravo-Ureta, B. E. (2010). Technical efficiency and meta technology ratios for dairy farms in three southern cone countries: a stochastic meta-frontier model. J. Prod. Anal. 33, 33–45. doi: 10.1007/s11123-009-0144-8
Naziri, D., Rich, K. M., and Bennett, B. (2015). Would a commodity-based trade approach improve market access for Africa? A case study of the potential of beef exports from communal areas of Namibia. Dev. Policy Rev. 33, 195–219. doi: 10.1111/dpr.12098
Negassa, A., and Jabbar, M. (2008). Livestock ownership, commercial off-take rates and their determinants in Ethiopia. Research Report 9. ILRI (International Livestock Research Institute), Nairobi, Kenya. pp. 52.
Neibergs, J. S., Hudson, T. D., Kruger, C. E., and Hamel-Rieken, K. (2018). Estimating climate change effects on grazing management and beef cattle production in the Pacific Northwest. Clim. Chang. 146, 5–17. doi: 10.1007/s10584-017-2014-0
Newman, C., and Matthews, A. (2006). The productivity performance of Irish dairy farms 1984–2000: a multiple output distance function approach. J. Prod. Anal. 26, 191–205. doi: 10.1007/s11123-006-0013-7
Ng’ombe, J. N. (2017). Technical efficiency of smallholder maize production in Zambia: a stochastic meta-frontier approach. Agrekon 56, 347–365. doi: 10.1080/03031853.2017.1409127
Ng’ombe, J., and Kalinda, T. (2015). A stochastic frontier analysis of technical efficiency of maize production under minimum tillage in Zambia. Sustain. Agric. Res. 4:31. doi: 10.5539/sar.v4n2p31
Nguyen, T. T., Tran, V. T., Nguyen, T. T., and Grote, U. (2021). Farming efficiency, cropland rental market and income effect: evidence from panel data for rural Central Vietnam. Eur. Rev. Agric. Econ. 48, 207–248. doi: 10.1093/erae/jbaa013
Obianefo, C. A., Ng’ombe, J. N., Mzyece, A., Masasi, B., Obiekwe, N. J., and Anumudu, O. O. (2021). Technical efficiency and technological gaps of rice production in Anambra State, Nigeria. Agriculture 11:1240. doi: 10.3390/agriculture11121240
O’Donnell, C. J., Rao, D. P., and Battese, G. E. (2008). Metafrontier frameworks for the study of firm-level efficiencies and technology ratios. Empirical economics 34, 231–255.
Odubote, I. K. (2022). Characterization of production systems and management practices of the cattle population in Zambia. Trop. Anim. Health Prod. 54, 1–11. doi: 10.1007/s11250-022-03213-8
Orea, L., and Kumbhakar, S. C. (2004). Efficiency measurement using a latent class stochastic frontier model. Empir. Econ. 29, 169–183. doi: 10.1007/s00181-003-0184-2
Orea, L., Perez, J. A., and Roibas, D. (2015). Evaluating the double effect of land fragmentation on technology choice and dairy farm productivity: a latent class model approach. Land Use Policy 45, 189–198. doi: 10.1016/j.landusepol.2015.01.016
Otieno, D. J., Hubbard, L., and Ruto, E. (2011). “Technical efficiency and technology gaps in beef cattle production systems in Kenya: A stochastic metafrontier analysis” in 85th annual Conference of the Agricultural Economics Society (AES) 18 – 20 April 2011 University of Warwick, UK
Otieno, D. J., Hubbard, L. J., and Ruto, E. (2012). Determinants of technical efficiency in beef cattle production in Kenya (No. 1007-2016-79780).
Otieno, D. J., Hubbard, L., and Ruto, E. (2014). Assessment of technical efficiency and its determinants in beef cattle production in Kenya. J. Dev. Agric. Econ. 6, 267–278. doi: 10.5897/JDAE2013.0525
Rich, K. M., and Bennett, B. (2019). Using preferential trade access to promote global development goals: the case of beef and market access to Norway from Namibia and Botswana. Agrekon 58, 485–502.
Rich, K. M., and Perry, B. D. (2011). The economic and poverty impacts of animal diseases in developing countries: new roles, new demands for economics and epidemiology. Prev. Vet. Med. 101, 133–147. doi: 10.1016/j.prevetmed.2010.08.002
Ruttan, V. W. (1971). Technology and the environment. Am. J. Agric. Econ. 53, 707–717. doi: 10.2307/1238069
Sauer, J., and Paul, C. J. M. (2013). The empirical identification of heterogeneous technologies and technical change. Appl. Econ. 45, 1461–1479. doi: 10.1080/00036846.2011.617704
Serletis, A., and Feng, G. (2015). Imposing theoretical regularity on flexible functional forms. Econ. Rev. 34, 198–227. doi: 10.1080/07474938.2014.945385
Sigwele, K. H., and Orlowski, D. W. (2015). Botswana - Agriculture public expenditure review (2000–2013). Washington, D.C: World Bank Group.
Statistics Botswana (2022). Gross domestic product: first quarter of 2022. Available at: http://www.statsbots.org.bw.
Temoso, O., Hadley, D., and Villano, R. (2015a). Performance measurement of extensive beef cattle farms in Botswana. Agrekon 54, 87–112. doi: 10.1080/03031853.2015.1116399
Temoso, O., Hadley, D., and Villano, R. (2016). Evaluating the productivity gap between commercial and traditional beef production systems in Botswana. Agric. Syst. 149, 30–39. doi: 10.1016/j.agsy.2016.07.014
Temoso, O., Hadley, D., and Villano, R. (2018). Sources of efficiency, productivity and output growth in Botswana agriculture. Rev. Dev. Econ. 22, 1105–1124. doi: 10.1111/rode.12376
Temoso, O., Ng’ombe, J. N., Bahta, S., and Hadley, D. (2023). Total factor productivity growth in livestock production in Botswana: what is the role of scale and mix efficiency change in beef production? Agrekon 62, 5–18. doi: 10.1080/03031853.2022.2156899
Temoso, O., Villano, R., and Hadley, D. (2015b). Agricultural productivity, efficiency and growth in a semi-arid country: case study of Botswana. Afr. J. Agric. Resour. Econ. 10, 192–206. doi: 10.22004/ag.econ.211667
The World Bank (2016). World development indicators. Available at: http://data.worldbank.org/data-catalog/world-development-indicators (Accessed May 19, 2017).
Thirtle, C., Atkins, J., Bottomley, P., Gonese, N., Govereh, J., and Khatri, Y. (1993). Agricultural productivity in Zimbabwe, 1970-90. Econ. J. 103, 474–480. doi: 10.2307/2234787
van Engelen, A., Malope, P., Keyser, J., and Neven, D. (2013). Botswana agrifood value chain project: beef value chain study. Rome: Food and Agriculture Organisation (FAO).
Wang, H. J., and Schmidt, P. (2002). One-step and two-step estimation of the effects of exogenous variables on technical efficiency levels. J. Prod. Anal. 18, 129–144. doi: 10.1023/A:1016565719882
Wollny, C. B. (2003). The need to conserve farm animal genetic resources in Africa: should policy makers be concerned? Ecol. Econ. 45, 341–351.
Keywords: latent class stochastic frontiers, stochastic meta-frontier analysis, technical efficiency, Botswana, beef cattle production
Citation: Bahta S, Temoso O, Ng'ombe JN, Rich KM, Baker D, Kaitibie S and Malope P (2023) Productive efficiency of beef cattle production in Botswana: a latent class stochastic meta-frontier analysis. Front. Sustain. Food Syst. 7:1098642. doi: 10.3389/fsufs.2023.1098642
Received: 15 November 2022; Accepted: 18 May 2023;
Published: 13 June 2023.
Edited by:
Patrick Meyfroidt, Universitécatholique de Louvain, BelgiumReviewed by:
Gideon Danso-Abbeam, University for Development Studies, GhanaCopyright © 2023 Bahta, Temoso, Ng'ombe, Rich, Baker, Kaitibie and Malope. This is an open-access article distributed under the terms of the Creative Commons Attribution License (CC BY). The use, distribution or reproduction in other forums is permitted, provided the original author(s) and the copyright owner(s) are credited and that the original publication in this journal is cited, in accordance with accepted academic practice. No use, distribution or reproduction is permitted which does not comply with these terms.
*Correspondence: Sirak Bahta, cy5iYWh0YUBjZ2lhci5vcmc=
Disclaimer: All claims expressed in this article are solely those of the authors and do not necessarily represent those of their affiliated organizations, or those of the publisher, the editors and the reviewers. Any product that may be evaluated in this article or claim that may be made by its manufacturer is not guaranteed or endorsed by the publisher.
Research integrity at Frontiers
Learn more about the work of our research integrity team to safeguard the quality of each article we publish.