- 1College of Humanities and Social Development, Northwest A&F University, Xianyang, Shaanxi, China
- 2School of Agriculture and Food Sciences, University of Queensland, Brisbane, QLD, Australia
- 3School of Management, Xi'an University of Science and Technology, Xi'an, China
The development of green technology (GT) may have a vital influence in decreasing carbon releases, and the linkage between the advancement of GT and CO2 releases in China's agricultural industry has not attracted enough attention. The main objectives of this study are to assess the influence of agricultural green technology advancement on efficiency enhancement, release control capabilities, agricultural energy structure, and agriculture industrial structure. This article decomposes the advancement of green technology (AGTP) in the agricultural industry in China into resource-saving green technology advancement (AEGTP) and emission reduction green technology advancement (ACGTP). At the same time, to evaluate the intermediary impact of green technology advancement, a two-step econometric model and an intermediary impact model were utilized to evaluate the panel data of 30 provinces in China from 1998 to 2018. The role of AGTP (including ACGTP and AEGTP) and CO2 release concentration has also been explored critically. The results show that (i) under the two-step measurement method, AGTP has substantial favorable impacts on agricultural energy efficiency (EF) and possesses a negative impact on agriculture industrial structure (PS) and agricultural energy structure (ES). Agricultural energy efficiency (EF) and agriculture industrial structure (PS) under AGTP will reduce CO2 release concentration, but the path of agricultural energy structure (ES) will increase CO2 release concentration. (ii) At the national level, AGTP has an immediate unfavorable influence on CO2 releases. After introducing the intermediary variables, the intermediary impact of AGTP on CO2 releases through agricultural energy efficiency (EF), agriculture industrial structure (PS), and agricultural energy structure (ES) is also significantly negative, and the direct impacts of each variable are higher than the intermediary impact. (iii) In terms of different zones, the direct impacts of AGTP are all significant. The order of significance of the direct impacts of different zones is west to central and central to eastern. The overall significance ranking of the mediating impact is ACGTP > AEGTP > AGTP, and the significance ranking of each index is ES > EF > PS. Finally, this article puts forward some policy recommendations to reduce CO2 releases.
1. Introduction
Greenhouse gas releases seriously threaten the global ecological environment and all countries' sustainable development goals, and are considered a major global crisis (Bennett, 2017; Kumar et al., 2019). Though China's CO2 release concentration reduced from 1.328 million tons in 2010 to 754,000 tons in 2017, the proportion is nearly double that of the worldwide average emissions (Liu et al., 2021; Tang et al., 2021). Since China retained the “Paris Agreement” in 2016 and committed to at least 60% lessening of CO2 releases by 2005 to 2030 (Gallagher et al., 2019), the notion of green technological development gained much more attention from the governmental agendas. In the general debate of the 75th United Nations General Assembly, China projected that CO2 releases should be under control before 2030, and will attempt to accomplish the goal of becoming a carbon-neutral nation by 2060 (Wei et al., 2020). As an essential part of CO2 releases, agriculture accounts for 17% of total CO2 releases (Hong et al., 2021). Therefore, in March 2021, China initiated the “14th Five-Year Plan (2021–2025),” which highlights environmental safety and a green transition and also highlights the importance of green production by availing responsible usage of natural resources (Wang et al., 2021) and focusing on reducing CO2 releases (Xu et al., 2020). It also proposed “high-efficiency, high-quality and multi-resistance technology,” “environmental-friendly and high-efficiency fertilizers,” and “agricultural pesticides, and 20 kinds of green agricultural technologies,” including biological preparation technology, energy-conservation, and low-consumption intelligent agricultural equipment technology (Xiong et al., 2016). It shows the determination of China to broaden the development of green agriculture, promote the advancement of GT, and reduce CO2 releases. Notably, the agricultural industry is a crucial sector that not only influences the local economy but also amends as a decisive factor in altering comprehensive food security and supply, which influences people's livelihoods and determines the development of any country's gross domestic product (GDP). Therefore, a comprehensive understanding of how and to what extent the development of green production technology reduces CO2 undoubtedly holds outstanding empirical significance.
In this case, there has been aroused a profound ground of potentiality of researching how the advancement of green technology applications to facilitate a well-optimized, innovative allocation of production factors that can ensure agricultural CO2 release reduction and eventually formulate green development of agriculture (Chen Z. et al., 2021). This is a prime motivation for the study to explore the impacts of mechanisms on green technology development toward agricultural carbon emission reduction. Tracing the advancement of green technology is a complex phenomenon that may depend on various internal and external factors (Norse, 2012). It mainly denotes two distinct varieties, resource-conservation green technology advancement and emissions reduction green technology advancement (Cui et al., 2021b), which need to be explored critically. Moreover, the advancement of green technology in China possesses profound regional individuality, and different economic and social structures may impact the advancement process (Xu et al., 2019). Thus, it should be explored in the prospects of heterogeneity.
This article contributes to the existing corpus in five distinct approaches. First, the green technological advancement (AGTP) of China's plantation industry is decomposed into resource-conservation green technology advancement (AEGTP) and emissions reduction green technology advancement (ACGTP), which will be one of the first attempts to the best of our knowledge. Second, this article empirically explores the effect of different forms of AGTP on CO2 and draws corresponding conclusions and findings. Third, it discusses the influence paths of different forms of AGTP on CO2 in the eastern, central, and western zones and gives precise conclusions and discoveries of direct and indirect impacts. Fourth, we split green technological advancement (AGTP) into emission-reducing green technological advancement (ACGTP) and resource-conservation green technological advancement (AEGTP) and analyze the effects of those on CO2 release concentration. Finally, the intermediary path impacts of green technology advancement (AGTP) in different zones are studied. To a certain extent, the assessment can be used as a base point for formulating corresponding green technology application plans and supervision structures in different parts of the world.
2. Literature review
Global population expansions, the current era of modern lifestyle, and updated consumption patterns continuously pose severe demands to existing worldwide production patterns. This “pioneer revolution” had hardly begun in 1850, yet had reached on peak within the twentieth century, which massively changed the way of producing food and fiber (Sarkar et al., 2021). The change was almost synchronous worldwide and must have led to the release of substantial quantities of CO2 into the atmosphere (DeAngelo et al., 2022). Moreover, the sector is liable for CO2 outputs from using natural organisms (mostly woods and natural forest ecologies) to land and as non-carbon emissions from crop and animal operations at the farm gate (Karimi Alavijeh et al., 2022), which is responsible for 24% of greenhouse gases emissions in the world (Yurtkuran, 2021). Existing literature (such as Tang and Wang, 2023; Zhao et al., 2023) indicates that, like most other major sectors, agriculture significantly contributes to massive carbon emissions. According to a study by Frank et al. (2018), ever-increasing carbon emissions from the agriculture sector cause serious environmental concerns, as it is the most significant reason for accelerating global warming and global climate change. Due to this, several initiatives emerged. Countries that have signed international climate accords (such as the Kyoto Protocol in 1997 and the Paris Agreement in 2015) have committed to keeping global warming below 2°C and working toward keeping it below 1.5°C (Sarkar et al., 2022).
Therefore, technological advancement has been established to be an impactive manifestation of reducing CO2 releases in the agriculture sector, and every nation on earth has made a plea to raise its degree of technological advancement to combat climate change, global warming, and uneven seasonal changes (Moore et al., 2017), primarily through green technology (Abou-Elela, 2019). “Green technology” is a term referring to a technology mechanism that has the potential to decrease consumption and contamination of natural resources while simultaneously improving the ecosystem (Du and Li, 2019). However, in the existing literature, the influence of green technology on reducing CO2 releases has been explored widely. Such as Du et al. (2019) explored 71 countries' data from 1996 to 2012 and found that green technology and energy consumption significantly influenced CO2 release. In a study of time-series data from Singapore, Meirun et al. (2021) highlighted an optimistic connection between green technological interventions and carbon release in both the long and short run. “Energy Development Action Plan 2014–2020 of China” also pointed out that promoting innovative technological advancements can considerably decrease energy-based CO2 releases.
Moreover, various researchers have found that technological advancement can impassively reduce CO2 concentration (Tian et al., 2019), and some researchers found that the notion can foster low emissions-based development (such as Mulugetta and Urban, 2010; Kang et al., 2018; Wang and Feng, 2020). In a study of APEC economies, Wu et al. (2016) evaluated the effect of GTA on CO2 releases and highlighted its prominent effects on lowering CO2 releases. Similarly, based on China's core environmental data, Zhao et al. (2017) outlined that the direct impact of energy-conservation technological advancement is more significant than that of emission reduction technology. Similarly, Yan et al. (2020) found that green technology, which favors energy conservation, release reduction, and cleaner production, is the prime influencer for facilitating the notion of low-carbonization growth of the industrial structure. Song et al. (2022) evaluated the resource-based enterprises of China and found that the advancement of green technology has a positive influence on the development of green industrial productivity and the expansion of a low-carbon base economic transition, and the impact is significantly higher than the impact of GT efficiency.
In agriculture, mainstream exploration primarily emphasizes the research on the influence of agricultural technology advancement on carbon release reduction. Then, Paroussos et al. (2020) explored Italian green growth and identified that renewable power innovation reduces the total factor CO2 performance index. As an improvement or innovation of agricultural technology (Chen Z. et al., 2021), the advancement of green agricultural technology can favor the development of green technologies for energy conservation and cleaner production and increase the marginal output of green agricultural products (Zhang and Chen, 2021). Ge et al. (2022) found technological advancement through research on pig breeding. They also revealed that impactive and environmentally friendly waste management could significantly cut carbon release growth. Hu (2018) identified that agricultural technological advancement and technological efficiency negatively impact CO2 release concentration, and technological efficiency mainly significantly reduces releases through scale efficiency. In a study of agricultural CO2 concentration in China, Zhong et al. (2022) depicted that the development and structural changes in agricultural activities have increased the total amount of agricultural carbon releases, but it is also conducive to the reduction of agricultural CO2 release concentration.
Researchers and policymakers from a variety of fields are looking for methods and techniques in order to mitigate the ever-increasing effects on the environment and guarantee the protection of the natural world. In recent years, green technologies have arisen as an innovative form of interdisciplinary progress that may be used to combat the serious environmental sustainability concerns that are now being faced by reducing harmful emissions, improving farm-level energy efficiencies, and reducing other detrimental environmental impacts. Such technologies are environmentally friendly and resource-efficient and positively impact public wellbeing.
Technological advancement affects energy efficiency by conserving relatively cheap production factors and reducing energy usage per unit of output, thereby helping to reduce CO2 release concentration (Yang et al., 2021). Though technological advancement is not always neutral, to fully grasp the benefit of certain production factors and individuals in the economy, technological advancement will reduce CO2 release concentration through different paths (Chen et al., 2020; Hasanov et al., 2021). However, the biased theoretical analysis of technological advancement believes that factor substitution substantially controls energy competence (Liao and Ren, 2020). Interestingly, technological advancement tends to save relatively expensive production factors such as the cost of new energy technologies and promote the efficient use of agricultural resources.
In the agriculture sector, the notion of carbon emission reduction is fostered through organic fertilizers, biological control, physical control, and other green prevention and control technologies. Then, it increases the proportion of biomass fuels, wind, and solar power by changing the input ratio of production factors in agricultural production supplies (Yue et al., 2021). Aldieri et al. (2021) utilized the technological advancement framework to assess the influence of techno-ecological advancement on CO2 release reduction and found that it significantly reduced the cost of carbon release reduction through the learning impact and increased the social benefits of carbon release reduction. The last is the path of the agricultural industry structure. Technological advancement is biased to alter the industrial structure to increase the competence of agro-production and change the marginal output of factors (Frank et al., 2018), breaking the equilibrium state of the original factor allocation among industries, and promoting the complementarity or substitution between factors. This, in turn, affects the industrial structure (Shen et al., 2018). Technological advancement tends to change the supply of production resources in society through innovation or alternative resource elements (Frank et al., 2019) and speed up the development of specific industries. However, when input and output structures are highly interrelated, the coordination of industrial development is also high, which leads to the rational allocation and proficient use of production factors (Grzelak et al., 2019; Han et al., 2021).
However, the study mentioned earlier has explored the impact of green technological advancement on carbon release or concentration and the path of action from different perspectives. Moreover, those sets of research still have several limitations. For example, based on heterogeneity, limited articles have examined the influence of technological advancement on CO2 release concentration. Carbon release concentration is different, as the impact of different green technology advances. Therefore, various technological attributes demand a much more comprehensive analysis and comprise the following research questions. (i) What is the path of green technological advancement on carbon release concentration? (ii) Especially from a perspective of heterogeneity analysis, what are the different pathways of various green technological advancements on carbon release concentration? (iii) What are the strategies to promote green technological advancement in different zones to achieve low-carbon releases? (iv) What are the spatial effects of various GT on carbon release concentrations? The study intends to answer the aforementioned questions. The central motivation of this article is to make in-depth assessments of the emission reduction effect of GT and provide comprehensive recommendations for the government.
3. Material and methods
In the article, we have focused on the planting industry and measured how the advancement of green agricultural technology reduces agricultural carbon emissions. Regarding the research of Mohammadi et al. (2013), Rebolledo-Leiva et al. (2017), and Khoshroo et al. (2018), the study used Data Envelopment Analysis (DEA) to craft its findings. The prime reason for choosing DEA is that it is a benchmarking tool that efficiently evaluates the performance of any specific phenomenon by using the input to outputs. More specifically, we have crafted our findings from two perspectives: the first is based on resource-saving aspects, which primarily involves minimizing the use of input materials related to the production process, which involves explicitly hidden carbon sources, such as chemical fertilizers, pesticides, and agricultural films. The second is the prospect of emission reduction from direct fossil fuel and other energy usages to agricultural production. As mentioned earlier, the resource-saving green technology advancement and emission reduction green technology advancement may provide an intermediary impact on agricultural carbon emission reduction performance. Therefore, by following the study of Balcombe et al. (2008), Naseem and Guang Ji (2021), and Hong et al. (2022), we have used the two-step econometric and intermediary impact model to measure the two prospects' combined impact.
3.1. Model construction
3.1.1. Green technology advancement model and its decomposition model
Currently, there are many measuring methods for technological advancement and CO2 releases. However, a non-parametric assessment technique such as Data Envelopment Analysis (DEA) is commonly manifested (Feng et al., 2015; Syp et al., 2015), and it does not involve predetermined production processes and capabilities to effectively assess linkages that have been concealed or overlooked in ecological processes (Nabavi-Pelesaraei et al., 2014). Moreover, it can objectively analyze the poor efficiency of non-impactful judgment mechanisms (Khoshnevisan et al., 2013). Interestingly, Total Factor Productivity (TFP) is often utilized as a metric for productivity. Conversely, it does not include the energy-conservation technologies that might significantly minimize production element usage and pollution (Mohammadi et al., 2013). Therefore, to solve the issue, Lovell (2003) segmented the Malmquist index into technical (TECH) and efficiency (EFFCH) adjustments and subsequently dissected the TECH indicator into the MATECH, OBTECH, and IBTECH metrics. This technique conceptually analyzes the scope of biased technological development based on the rotation and radial deviation of productivity frontiers. Moreover, it is limited to assessing just two input parameters, while AGTP is characterized by various inputs and outcomes (Song et al., 2017). Thus, we adjust the constraints of AGTP and use an advanced slacks-based measure (SBM) model to quantify and decompose AGTP as recommended by van der Werf (2008), Song and Wang (2018), and Yang et al. (2020). The methods include both energy-conservation and emission-controlling technological advancements (Yue et al., 2021), and the specific equations are as follows.
where x, e, y, and b are input variables, energy input, anticipated return, and undesirable output, respectively. While s signifies the slack factor and ρB is the conservational effectiveness. Seemingly, the Malmquist index of AGTP is interpreted as follows.
Here, x and y denote input and output variables, s and t highlight two different times, and ρX rectifies the competence measured according to the variable-return-to-scale framework. However, the AGTP is calculated by:
3.1.2. Two-step econometric model
Concerning the framework used by Sjöholm and Lundin (2013, p.49–75) and Yang et al. (2020), this research employs a two-step econometric framework to measure the impact of AGTP on EF, PS, and ES (to save space, both AcGTP and AeGTP are included in AGTP). The assessment follows the subsequent model to explore the impacts of AGTP on EF, PS, and ES. The formula is as follows.
Among them, AG stands for AGTP, AcGTP, and AeGTP; EF stands for agricultural energy efficiency; PS stands for agriculture industrial structure; and ES stands for agricultural energy structure, where i and t represent province and year, respectively, and ε is a random disturbance term. In addition, we also use the five control variables denoted by X, namely, imperative environmental policy, incentive environmental policy, voluntary environmental policy, incremental agricultural productivity, and per capita agricultural production. Whereas, to measure the influence of AGTP and EF, PS, and ES on carbon release concentration, we set the following model AGTP and the influence of EF, PS, and ES on carbon release concentration, and the formula is as follows.
Among them, AG stands for AGTP, AcGTP, and AeGTP; EF stands for agricultural energy efficiency; PS stands for agriculture industrial structure; and ES stands for agricultural energy structure. Seemingly, i and t represent province and year, respectively; and ε is a random disturbance term. In addition, we also use the five control variables denoted by X, namely, imperative environmental policy, incentive environmental policy, voluntary environmental policy, incremental agricultural production value, and per capita agricultural production value.
3.1.3. Mediating impact model
To assess the intermediary influences of agricultural energy efficiency (EF), agriculture industrial structure (PS), and agricultural energy structure (ES) in AGTP and carbon release concentration, respectively, the current research endorses the criterion proposed by Baron and Kenny (1986). First, according to the AGTP analysis and introduction of the mechanism of carbon release concentration and its multiple intermediary models, this article sets the following basic measurement models to test the impact of AGTP, AcGTP, and AeGTP on carbon release concentration.
Among them, CO2 is the explained variable; AG is the prime explanatory variable, representing AGTP, AeGTP, and AcGTP, respectively; and X is the control variable, which contains several variables that are independent of the influence of AG on CO2. Seemingly, μ_i represents the unobservable individual fixed impacts of variables; b_i is the unobservable fixed impacts of time variables; and ε_it is the random disturbance term. According to existing research (Ismael et al., 2018; Xu et al., 2019), AGT may influence CO2 release concentration through agricultural energy efficiency (EF), agriculture industrial structure (PS), and agricultural energy structure (ES). Similarly, to test the intermediary impacts of agricultural energy efficiency (EF), agricultural industry structure (PS), and agricultural energy structure (ES) in the process of AGT's impact on carbon release concentration, this article utilizes a multiple mediator impact framework. The framework is set as follows.
Among them, agricultural energy efficiency (EF), agriculture industrial structure (PS), and agricultural energy structure (ES) are the intermediary variables. Equations (12–16) list the AGTP's influence on the CO2 regression equation through the intermediary variables of agricultural energy efficiency (EF), agriculture industrial structure (PS), and agricultural energy structure (ES). Seemingly, X∧′, X∧”, and X∧′” are control variables, which contain several variables that are independent of the impact of AGTP on CO2 release concentration; μ_i∧′, μ_i∧”', and μ_i∧′” represent unobservable variables and individual fixed impacts; b_i∧′, b_i∧”, and b_i∧′” are unobservable fixed impacts of time variables. According to the multiple mediation impact model, the mediation impacts of specific paths are β11∅2, γ11∅3, and σ11∅4; the overall mediation impact is β11∅13+γ11∅13+σ11∅14; and the direct impact is ϕ_1. Combining formulas (12–16), the multiple mediation impacts are judged by sequentially testing the regression coefficients.
3.2. The explained variable
3.2.1. Carbon release concentration (CEI)
The CO2 releases and the gross production value of the planting industry measured by the carbon measurement model are used to measure the CO2 release concentration in each province (Pang et al., 2020).
3.2.2. Green technological advancement (AGTP)
As per the conceptual viewpoint, the Green Technological Advancement of China has been rectified by environmentally friendly green technological advancement, and it has been decomposed into emission-reducing green technological advancement and resource-conservation green technology advancement.
3.3. Intermediary variables
3.3.1. Agricultural energy efficiency
Improving energy efficiency in agricultural production is a crucial tactic to facilitate green and low-carbon-based agricultural development at present and in the future. The level of national energy usage in agriculture is predominantly significant. The higher agricultural energy competence denotes the control of the CO2 release concentration. Therefore, it is imperative to expand the competence of agrarian energy use. This article selects the proportion of the added value of agricultural, forestry, livestock, and fisheries to the total agricultural energy consumption to measure. The larger ratio usually lowers agricultural energy competence and vice-versa (Zhao et al., 2020).
3.3.2. Agriculture industrial structure (PS)
Agriculture industrial structure denotes the modification and amendment of the modernization of agrarian structure. The adjustment of industrial structure may improve agricultural performance, cause excessive input of production factors, and is not favorable for improving agricultural productivity (Li et al., 2021). Moreover, industry restructuring will also affect the advancement and application of green technology to a certain extent, thereby affecting CO2 releases (Feng et al., 2015). The selection of agriculture industrial structure indicators must consider the dynamic process that reflects the evolution of agricultural structure. Therefore, this article introduces the Moore value of the structural change coefficient and uses various agricultural sectors as a dynamic whole to measure the level of change (Moore, 2015). The formula for calculating the Moore value (for more details, read Schaller, 1997) is as follows.
Here, M_t denotes the ratio of Moore structure change; W_(i,t−1) represents the proportion of the ith industry in period t-1, and W_(i,t) signifies the proportion of the ith industry in period t. The Moore value of each period represents the relative change in the level of the PS in the current period. Let us define the angle of change between industrial vectors in different periods as θ_t, where θ_t=arccosM_t. The θ value in each period represents the relative change degree of the agriculture industrial structure in the current period (Carrillo, 2021).
3.3.3. Agricultural energy structure
The agricultural energy structure reflects the usage of various types of energy in the processes of agrarian intervention, and the influence of different types of energy consumption on carbon release concentration varies. This article selects the proportion of total energy consumption in the current year to measure ES. The larger the ratio, the higher the proportion of agricultural energy utilization, and vice versa, the ratio of agricultural energy-conservation (Xu et al., 2021).
3.4. Control variables
The control factors set in this article comprise command-based environmental regulations (mlgov), incentive-based environmental regulations (jlgov), voluntary environmental regulations (zygov), incremental agricultural production (IAP), and total agricultural production per capita (PGDP). The command-based environmental regulation (mlgov) reflects “regulatory guidelines.” This means the government created guidelines for dealing with actual or projected ecological issues and is essential for enacting agrarian carbon release reduction strategies. This article uses the quantitative indicators of the ecological parameter strategies adopted by the provinces in the year (Guo and Yuan, 2020). In the case of incentive ecological regulation (jlgov), this article uses the CO2 release trading market as the basis of the incentive environmental regulation, drawing lessons from the research of Lin Zhang et al. (2013). Voluntary environmental regulation (zygov) denotes the costs of resolving real and possible ecological issues to inspire, control, and direct carbon release reduction policies (Gonzalez-Martinez et al., 2021). We utilize the level of investment accomplished in contamination mechanism developments to GDP to measure it (Zhang et al., 2020). The increment of Agricultural Product Value (IAP) reflects the proportion of agrarian economic expansion, and the higher the value of the increment, the higher the impact on CO2 releases. This article utilizes the incremental value of the planting industry's total output to characterize the per capita agricultural product (PGDP). The per capita agricultural product reflects the proportion of agrarian economic expansion, and the more advanced the proportion of economic expansion, the higher the impact on carbon releases. This article uses the planting sector's total output level to characterize the per capita agricultural production value.
3.5. Data sources
This article takes planting as the research object and selects 30 provinces in China (excluding Hong Kong, Macao, Taiwan, and Tibet) as the research area, and the period is set from 1998 to 2018 (due to the complete availability of the set of information). Aligned with the existing literature (such as Lin and Xu, 2018; Chen Y. et al., 2021; Zhou et al., 2022), the input elements used in the data include synthetic fertilizers, insecticides, agricultural film, agricultural fuel usage, used machinery, crop planting area, and labor supply. The output data include the planting industry's total productivity and expected output, and the CO2 release concentration has been set as undesired output. All data are collected from the “China Rural Statistical Yearbook,” “China Agricultural Statistical Yearbook,” and “China Fishery Statistical Yearbook.” The datasets were collected in April 2022. Table 1 presents the details of each variable.
4. Results
4.1. The impact of green technology advancement on intermediary variables
Interestingly, this article makes a preliminary judgment on the relationship between mediation variables and carbon release concentration indicators to verify the mediation impact. This article uses STATA 15 statistical software and selects a fixed-impact model to investigate the correlation between green technological advancement and the intermediary factors by employing Hausman's test. The estimated outcomes of the framework are shown in Table 2. Columns (1–3) of Table 2 consider the influence of AGTP on EF. The calculation results show that for each increase of AGTP, AcGTP, and AeGTP by 1 unit, EF will increase by 0.87, 1.45, and 1.09 units, respectively. This means that AGTP, AcGTP, and AeGTP can impactive and improve agricultural energy efficiency (EF) levels. This is consistent with the research of Chen and Liu (2021). Technological advancement increases energy competence by aggregating the marginal factor efficiency or changing the input and competence of factors in different proportions. The first strategy involves increasing the productive capacity of elements while maintaining a similar percentage, whereas the second strategy involves adjusting the inflow and utilizing the components' effectiveness in varying quantities.
In columns (4–6), we consider the influence of GTA on the agricultural industry structure (PS), and at the same time, AGTP, AcGTP, and AeGTP have a substantial undesirable influence on the agrarian industry structure (PS). This means that AGTP, AcGTP, and AeGTP will moderate the rationality of the agrarian industrial structure (PS). In columns (7–9), we include the influence of GTA on the ES. The possible explanation is that technological advancement usually means mutual substitution between old and new technologies, and different technologies may be used in different industries (production content). Under the condition of limited production factors, green technologies are substituted. The process will cause different industries (production content) to use relatively scarce green production factors competitively. However, the scarcity of green production factors does not mean the rationality of the industrial structure, such as the “high carbon-low efficiency” characteristics of the planting industry itself. Compared with other “low carbon-high-efficiency” agricultural industries, there is no green development advantage (Yun, 2016), and the reduction in the planting proportion does not mean that the industrial structure is reasonable.
It can be seen that AGTP, AcGTP, and AeGTP all have a substantial adverse influence on ES, but the coefficient is small. When ACGTP is increased by 1 unit, ES will decrease by 0.01, 0.02, and 0.02 units. This means that AGTP, AcGTP, and AeGTP are not favorable to the rationality of the agricultural energy structure (ES). This is inconsistent with expectations. The possible explanation is that AGTP, AcGTP, and AeGTP can better force farmers or agricultural enterprises to make technological innovations to compensate for high costs and adjust the agricultural industry structure, thereby improving the eminence of economic development and minimizing the issues of resource and environmental protection. However, technological improvements through AGTP, AcGTP, and AeGTP have increased the input demand for production factors, so its impact on the agricultural energy structure is substantially adverse. The projected outcomes of the control variables are presented in Table 2. The control variables substantially influence the agricultural industry structure (PS). However, the values of Adgov and Zygov are significant for agricultural energy efficiency (EF). Except for PGDP, all control variables are more significant to the intermediary variable agricultural energy structure (ES).
4.2. The influence of AGTA and intermediary variables on carbon release concentration
At the same time, after introducing intermediary variables, this article examines the influence of GTA and intermediary variables on carbon release concentration. The outcomes are revealed in Table 3. Among them, columns (1–3), respectively, show the estimated impact of AGTP and intermediary variables [agricultural energy efficiency (EF), agriculture industrial structure (PS), and agricultural energy structure (ES)] on carbon release concentration results. As a result of the combined impact of AGTP and intermediary variables, AGTP negatively affects the carbon release concentration, which is in line with basic expectations. When the EF increases by 1 unit, the carbon release concentration decrease by 110 units, and when the agriculture industrial structure (PS) escalates by 1 unit, the carbon release concentration increases by 1,086 units, and the agricultural energy structure (ES) increases by 1 unit. Moreover, the carbon release concentration increased by 12,742 units. This means that under AGTP, agricultural energy efficiency (EF) and agriculture industrial structure (PS) will reduce CO2 release concentration, but agricultural energy structure (ES) will increase CO2 release concentration. The possible explanation is similar to the results in Table 2. Seemingly, AGTP can better force farmers or agricultural enterprises to adopt technology to compensate for rising costs and adjust the agricultural industry structure to solve the problems of resource scarcity and environmental protection (Sá et al., 2017). At the same time, AGTP will further increase the input of production factors, so its impact on the agricultural energy structure is significantly negative, increasing the carbon release concentration. Columns (4–6), respectively, show the estimated results of the combined impacts of AcGTP and the intermediary variable agricultural energy efficiency (EF), agriculture industrial structure (PS), and agricultural energy structure (ES) on carbon release concentration. Columns (7–9), respectively, show the estimated results of the impacts of AeGTP and the intermediary variables [agricultural energy efficiency (EF), agriculture industrial structure (PS), and agricultural energy structure (ES)] on CO2 release concentration under the combined action of AeGTP. The significant impacts of these two parts are the same as those in columns (1–3). The above are all preliminary observations, and a more accurate intermediary relationship between the variables can only be obtained after regression analysis and testing. In addition, the estimated outcomes of the control factors (Table 3) are consistent with Table 2, and this article will not elaborate.
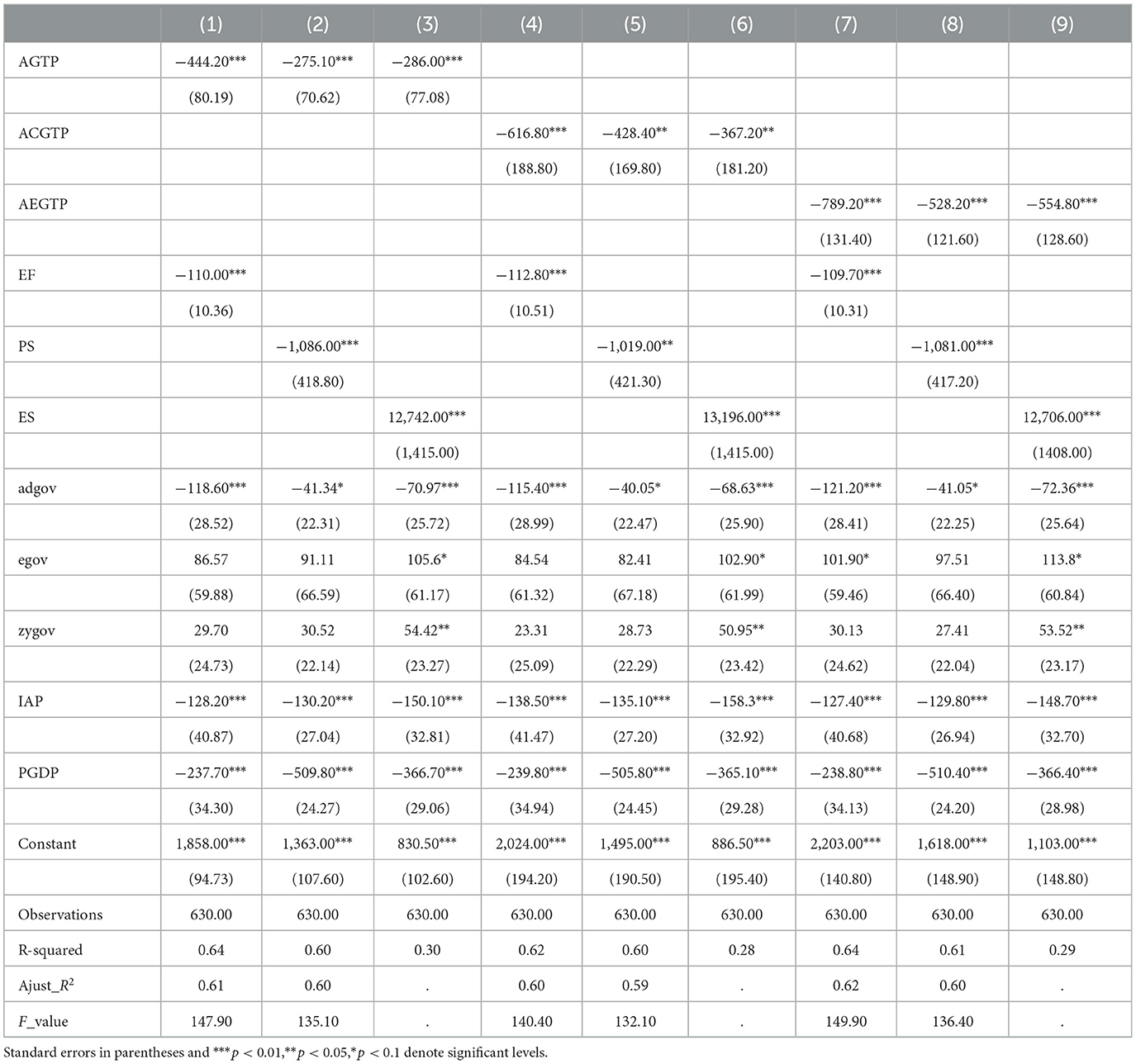
Table 3. Empirical outcomes of the influence of GTA and intermediary variables on carbon release concentration.
4.3. Analysis of the intermediary impact at the national level
This article first examines the impact of AGTP on carbon from the national level. Formulas (11–16), respectively, correspond to the regression outcomes of the framework in Table 4. In Model (1), the coefficient of AGTP is −518.3, with a 1% level of significance, representing that AGTP has a direct and substantial negative impact on CO2 releases and AGTP can reduce carbon releases. Among them, the mediation and direct impact need to be analyzed according to the calculation method given previously and collectively with the outcomes in Table 4. First, let us analyze the mediating impact of a specific path. This specifies that AGTP negatively impacts carbon release concentration through the agricultural energy efficiency (EF) approach. The intermediary impact (−54.88) of AGTP through the EF is the product of the AGTP coefficient (0.87) in column (2) and the agricultural energy efficiency (EF) coefficient (−62.86) in the Model (5) and with the impact at the 5% level of significance; that is, for every 1% increase in AGTP, 54.88% points of CO2 release reduction will be achieved through this approach.
It illustrates that AGTP significantly reduces the carbon release concentration through the intermediary impact of the agricultural industry structure (PS). The mediating impact of AGTP through the agriculture industrial structure (PS) is −22.12 (−0.01 × 1,400), and it is significant at the 5% level. The mediating impact of AGTP through the agricultural energy structure (ES) is −182.40 (−0.01 × 13,818), which is significant at the 1% level. This specifies that AGTP reduces CO2 release concentration by optimizing the agricultural energy structure (ES). Every 1% growth in AGTP will decrease the CO2 release concentration by 182.40 units. In addition, the mediating impact produced a higher value than the mediating impact of the combined impact of agricultural energy efficiency (EF) and agriculture industrial structure (PS).
Second, from the overall mediation impact analysis, the total mediation impact is 259.21 (54.88 + 22.12 + 182.40) by adding the aforementioned specific mediation impacts, and the overall mediation impact is statistically significant. It shows that green technological advancement decreases the overall carbon concentration through agricultural energy efficiency (EF), agriculture industrial structure (PS), and agricultural energy structure (ES). Third, let us analyze the contrast mediation impact. The previous analysis depicts that the intermediary impacts of AGTP on carbon emissions control through the three paths of agricultural energy efficiency (EF), agriculture industrial structure (PS), and agricultural energy structure (ES) are 54.88, 22.12, and 182.40, respectively, which account for respective proportions in the overall intermediary impact. They are 21.16, 8.52, and 70.32%, whereas the mediating influence of agricultural energy structure (ES) is more robust than that of agricultural energy efficiency (EF) and agriculture industrial structure (PS). In Model (4), the coefficient of AGTP is −298.40, which reflects the direct impact of AGTP on CO2, indicating that every 1% point increase in AGTP will directly reduce the CO2 release concentration by 298.40 units. This shows that in addition to the intermediary impact produced by the three paths of agricultural energy efficiency (EF), agriculture industrial structure (PS), and agricultural energy structure (ES), AGTP will also have a direct influence on carbon concentration. For the national level of CO2 release concentration, the direct impact of AGTP is higher than the mediating impact. After excluding the intermediary impacts as mentioned earlier, the immediate release reduction impact of AGTP itself on CO2 concentration is still very obvious. In addition, the direct impact can also be estimated by the coefficient of AGTP (-518.30) in Model 1 of Table 4, and the total influence of AGTP on CO2 concentration minus the value of the just obtained overall mediating impact (259.40), the result is 258.90. In Model (5), this value is not much different from −298.40, and the selection error of the disturbance term and the control variable mainly cause the difference.
Similarly, this article also measures the mediation path impact of AcGTP and AeGTP on carbon concentration. Both AcGTP and AeGTP can reduce CO2 concentration. Table 5 presents that the impacts of AcGTP and AeGTP on CO2 concentration through agricultural energy efficiency (EF), agriculture industrial structure (PS), and agricultural energy structure (ES) are consistent with the results of AGTP and are more significant than AGTP. From the overall mediating impact, the overall mediating impacts of AcGTP and AeGTP are −470.99 and −413.11, respectively, which are more significant than AGTP. Regarding direct impacts, the direct impacts of AcGTP and AeGTP are −329.30 and −570.70, respectively, which are more significant than AGTP. The significance levels of AcGTP and AeGTP are higher than AGTP because AGTP, as a general technological advancement, has too many types of technologies, while AcGTP and AeGTP, release-reduction and resource-conservation technological advancements, influence agricultural energy competence. The impacts of (EF), agricultural industry structure (PS), and agricultural energy structure (ES) are easier to distinguish and more direct, thus, the impact on CO2 concentration is relatively higher.
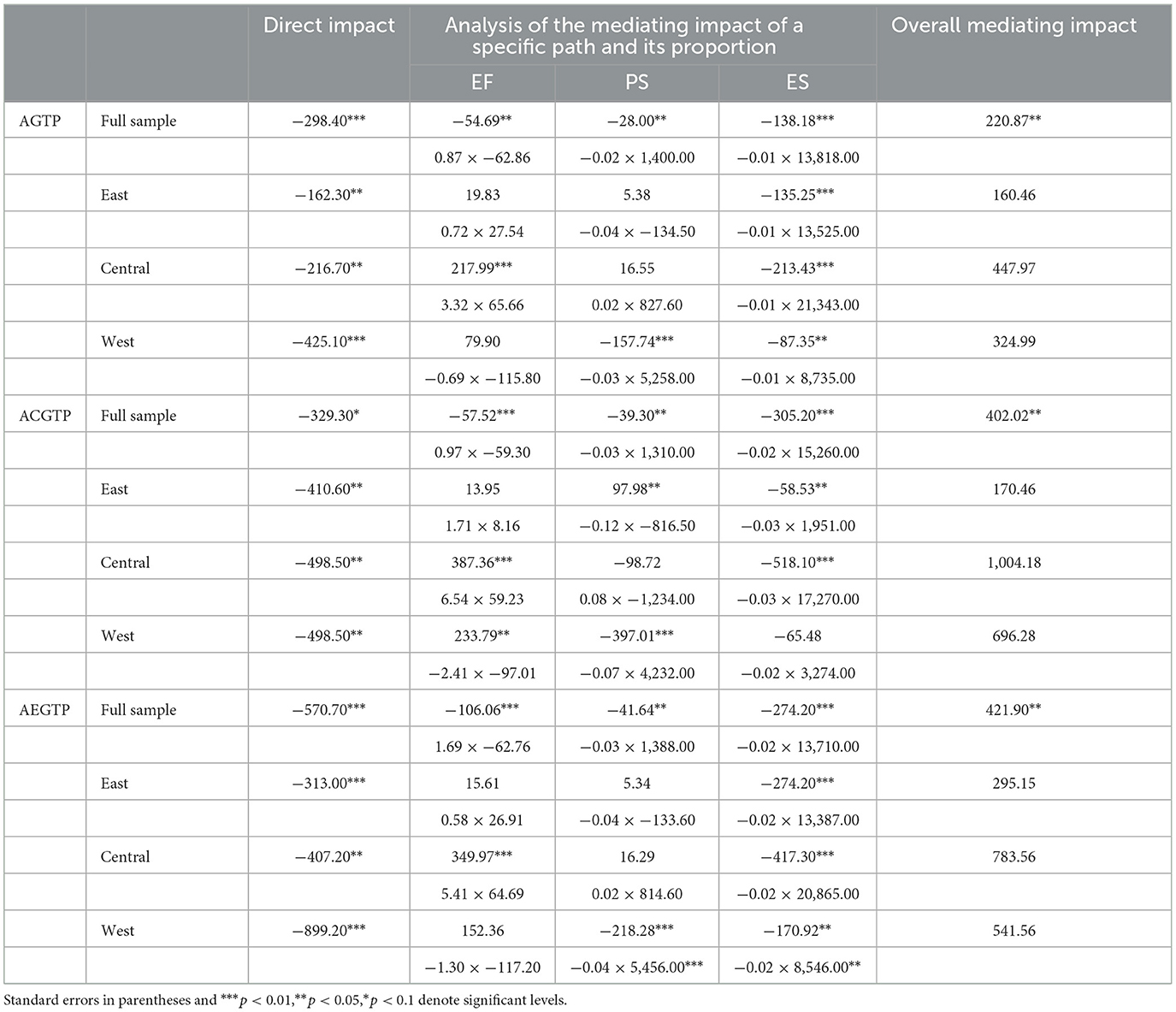
Table 5. Analysis of the mediating impact of AGTP, AcGTP, and AeGTP on carbon in the whole sample and sub-zones.
4.4. Analysis of mediating impact by area
The existing literature found that green technology advancement has a significant influence on carbon concentration through the three paths of agricultural energy efficiency (EF), agriculture industrial structure (PS), and agricultural energy structure (ES) at the national level. After excluding the above three intermediary impacts, the direct promotion of CO2 release decrease by green technological advancement is still very obvious. Since AGTP, AcGTP, AeGTP, agricultural energy efficiency (EF), agricultural industry structure (PS), and agricultural energy structure (ES) are diverse in different zones, it is essential to examine whether the mediating influence of GTA on CO2 release concentration is significant heterogeneity. According to economic growth, this article divides China into the middle east and the west. Moreover, it verifies that the AGTP, AcGTP, and AeGTP in the east, middle, and west have passed agricultural energy efficiency (EF), agriculture industrial structure (PS), and agricultural energy structure (ES). Table 5 presents the mediating impact of agricultural energy structure (ES) on the influence of carbon concentration.
4.5. Analysis of the intermediary impact in the eastern area
The mediating impact of AGTP in the eastern area through agricultural energy efficiency (EF) is 19.69 (0.71 × 27.54), but it is not statistically significant; its mediating impact through the agriculture industrial structure (PS) is 5.62 (−0.04 × −134.50), it is still not statistically significant; and its mediating impact through the agricultural energy structure (ES) is −178.53 (−0.01 × 13,525), with 1% level of significance. The mediating impact of AcGTP through agricultural energy efficiency (EF) in the eastern area is 13.96 (1.71 × 8.16), which is not statistically significant. The mediating impact of AcGTP through the agriculture industrial structure (PS) is 95.53 (−0.12 × −816.50), with a 5% level of significance, which is inconsistent with the outcomes at the national level. The influence of the agriculture industrial structure (PS) in the eastern area on CO2 release concentration is different from that of the national PS. The influence of the PS in the eastern area on CO2 release concentration is considered undesirable, but the national agricultural industry. The influence of agriculture industrial structure (PS) on CO2 release concentration is considerably positive; that is, the AcGTP in the eastern area cannot impactive endorse optimizing and upgrading the agricultural and industrial configuration, which is not conducive to CO2 release reduction. The probable cause of that the eastern area has a relatively developed economy and an advanced agricultural system, but it is highly dependent on AcGTP for agricultural production energy. However, as agriculture is a particular sector, the reduction in the proportion of high-energy planting does not mean the advancement of the PS, thus, changes in the agriculture industrial structure do not mean release reduction. Another explanation is that the planting industry's own “high carbon2-low efficiency” characteristics do not have the advantage of carbon release reduction compared with other agricultural industries with “low carbon-high efficiency” characteristics (Piwowar, 2019).
However, the advancement of release reduction technology has increased the proportion of the plantation industry, and CO2 sequestration will reduce the CO2 releases of the plantation industry. Conversely, it will also increase the input of production factors, which is even more detrimental to carbon release reduction. The mediating impact of AcGTP in the eastern area through the agricultural energy structure (ES) is −48.97 (−0.03 × 1,951), which is significant at 5%. The intermediary effect of AeGTP through agricultural energy efficiency (EF) and agriculture industrial structure (PS) in the eastern area is not statistically significant; the intermediary impact through agricultural energy structure (ES) is −261.04 (−0.02 × 13,387), which is at 1% significance level. Overall, AGTP, AcGTP, and AeGTP in the eastern area have no substantial mediating influence on the impact of CO2 release concentration. It is apparent that the agricultural energy structure (ES) in the east has significantly minimized the CO2 release concentration, but the agricultural energy efficiency (EF) and the agriculture industrial structure (PS) are not entirely significant. For the above results, the probable explanation is that the eastern area is a pioneer area of reform and opening up, actively participating in and integrating into the global competition, and market-based resource allocation has a decisive role. Relying on market competition, mechanisms can stimulate enterprise technological innovation and promote agricultural energy structure optimization and upgrade. At the same time, many provinces in the eastern area have already actively explored the path to the sustainable development of ecological safeguard and economic development. Agricultural energy competence is relatively high, and the impact of green technology advancement on agricultural energy efficiency is relatively small. The market dominates the agricultural industry structure in the eastern area, thus, the advancement of green technology has little impact on the agricultural industry structure (PS). These may be the reasons for the insignificant mediation impact.
4.6. Analysis of the intermediary impact in the central area
The mediating impact of AGTP in the central area through agricultural energy efficiency (EF) is 217.99 (3.32 × 65.66), which is statistically significant at 1%. This shows that AGTP in the central area improves CO2 release concentration through agricultural energy efficiency (EF). This is inconsistent with expectations. From the variable point of view, 3.32 and 65.66 are both positive and significant, which is inconsistent with the substantial adverse influence of agricultural energy efficiency on CO2 release concentration at the national level. Thus, the AGTP in the central area reduces agricultural energy efficiency (EF). This fosters growth in the concentration of agricultural carbon releases. The mediating impact of the AGTP in the central area through the agricultural industry structure (PS) is 16.30 (−0.02 × 827.60), which is not statistically significant, while the mediating impact of the AGTP through the agricultural energy structure (ES) is −262.51 (−0.01 × 21,343), with a 1% level of significance. The mediating impact of AcGTP in the central area through agricultural energy efficiency (EF) is 387.30 (6.54 × 59.23), with a 5% significance level. The mediating impact through the agriculture industrial structure (PS) is −97.36 (0.08 × −1,234), which is not statistically significant, and the mediating impact through the agricultural energy structure (ES) is −433.47 (−0.03 × 17,270), with 1% level of significance.
The mediating impact of AeGTP through agricultural energy efficiency (EF) in the central area is 349.77 (5.41 × 64.69), and the mediating impact through the agriculture industrial structure (PS) is not statistically significant; the mediating impact through agricultural energy structure (ES) is −394.35 (−0.02 × 20,865), with 1% level of significance. Overall, AGTP, AcGTP, and AeGTP in the central area had no substantial mediating impact on CO2 release concentration during the research period. The significant results show that the impacts of AcGTP, AeGTP, and AGTP in the central area on CO2 release concentration through EF are all significantly positive. A possible explanation is that AGTP has the same rebound impact as an industry (Brookes, 1990). The probable explanation is that the process of agricultural expansion in the central provinces inevitably caused the development issue of “high input, high pollution, high consumption, non-circulation, and low efficiency” (Gao et al., 2014). The development of advanced foreign technology reduces energy depletion and decreases pollutant discharges, but the high cost will lead to the reduction of subsequent profits, which hinders the improvement of energy competence (Song et al., 2018). Seemingly, the concentration of CO2 released has not decreased but increased. Another possible explanation is that the expansion of AGT has gradually enhanced the use competence of energy production factors, but the improvement in efficiency may also increase resource usage, and therefore its impact on carbon release reduction is not apparent. Seemingly, after technological improvement, the input of a specific high-carbon element in agricultural production factors will be increased, while the input of other low-carbon elements will remain unchanged, increasing carbon release concentration (He et al., 2021).
4.7. Analysis of the intermediary impact in the western area
The mediating impact of AGTP in the western area through agricultural energy efficiency (EF) is 79.67 (−0.69 × −115.80), but it is not statistically significant; the mediating impact through the agriculture industrial structure (PS) is −136.18 (−0.03 × 5,258), with 1% level of significance. The mediating impact through the agricultural energy structure (ES) is −100.45 (−0.01 × 8,735), which is significant at the 5% level. The mediating impact of AcGTP in the western area through agricultural energy efficiency (EF) is 233.30 (−2.41 × −97.01), with a 5% significance level. The probable cause is that, like the central area, advanced production technology fosters energy efficiencies and decreases pollutant discharges, thereby improving the extensive development mode to a certain extent. Because of the excessively high technical input cost, it will lead to later profits.
The analysis of the intermediary impact mentioned earlier shows that only the agricultural industry structure (PS) and the agricultural energy structure (ES) have a significant intermediary impact between green technological advancement and CO2 release concentration in the western area. The mediating impact of AcGTP in the western area through the agriculture industrial structure (PS) is −307.67 (−0.07 × 4,232), which is significant at 1%; the mediating impact of the AcGTP through the agricultural energy structure (ES) is −71.05 (−0.02 × 3,274) but not statistically significant. The mediating impact of AeGTP through agricultural energy efficiency (EF) in the western area is 151.89 (−1.30 × −117.20), which is not statistically significant. After the green technological progress of agriculture has increased the input of non-clean elements in the planting industry, farmers and enterprises have made technological innovations to make up for the high cost and adjust the agricultural industry structure. However, technological progress and structural adjustment will also promote rapid economic growth, thus generating new demand for energy and partially offsetting the saved energy (Yue et al., 2021), which is insignificant. The mediating impact through the agriculture industrial structure (PS) is −241.16 (−0.04 × 5,456), with a 5% significance level. Seemingly, the mediating impact through the agricultural energy structure (ES) is −133.32 (−0.02 × 8,546), which is significant at the 5% level. The mediating impact of agricultural energy efficiency (EF) in the western area between AcGTP and carbon release concentration is insignificant. The possible reason is that the agricultural energy competence in the west has always been high, and AcGTP has little impact on agricultural energy competence, thus, the mediation impact is insignificant.
4.8. Regional horizontal comparison
To sum up, there is apparent heterogeneity of intermediary impacts across the country and sub-zones. Regarding direct impacts, the direct impacts of sub-zones are all significant, with west>central>eastern. The possible reason is that the western area has unique advantages in natural resources, coupled with abundant light resources and biological resources, and relatively more ecological functional zones. The advantages of farmland green and environmentally green products are more significant than those in the central and eastern zones. Consequently, the release reduction impact of its green technological advancement is higher than in central and eastern zones. The direct impacts of the three indicators of AeGTP, AcGTP, and AGTP as AeGTP > AcGTP > AGTP prove that the impact of resource-conservation technological advancement is more evident than that of release-reducing technological advancement. It is crucial to develop resource-conservation technological advancements.
In terms of the overall mediation impact, only the national mediation impact is significant, and the mediation impact in different zones is not completely significant. From the overall mediating impact of AeGTP, AcGTP, and AGTP, AcGTP > AeGTP > AGTP, the secondary influence of release reduction technology on CO2 release concentration is the most important. From the viewpoint of intermediary factors, the significance of the intermediary variables is ES > EF > PS. Compared with agricultural energy efficiency (EF) and agriculture industrial structure (PS), agricultural energy structure (ES) has a significant mediating impact. For this result, the probable explanation is the substitution of agrarian production factors. Under limited economic production factors, the substitution mentioned earlier will cause different production units to use scarce production factors competitively, thus triggering changes in the production structure (Malerba, 2007). Agricultural energy efficiency (EF) mainly aims to increase the adoption rate of production factors through marginal impacts, but the production factors that it changes are limited, so its carbon release reduction impact is not strong.
5. Discussion
Based on China planting industry data, the study evaluated the impact of green agricultural technology on carbon emission reduction within the prospects of resource-saving and emission reduction green technology. We also have explored the mediation impact by comprising national, regional, and horizontal perspectives of the emission reduction impact of agricultural GT. The study found that the impact of green technology progress on agricultural efficiency (EF) is significantly positive, and the impact on agricultural industrial and energy structures is significantly negative. The possible reason for this is that the energy efficiency of China's agricultural sector is low (Zhao et al., 2018), and the potential for energy conservation and emission reduction is relatively large (Ji and Hoti, 2022). Thus, the government should increase investment in environmentally biased technological innovation, especially in technologies that reduce release and energy-conservation bias. They should distinguish the differences in CO2 release reduction of different technologies under different paths, emphasize the development of AcGTP's carbon release reduction advancement, and solve the CO2 release problem from the perspective of energy conservation. Moreover, the layout of the agricultural industry structure needs to be adjusted effectively. The degree of agglomeration of the agricultural industry is not enough to form a specific comparative advantage (He et al., 2022). Therefore, it needs to be expanded appropriately to form a professional market grid prospect by integrating planting, production, processing, and sales in a proper organizational structure.
As per the path analysis of the study, the path of agricultural energy efficiency and industrial structure reduces carbon emissions, but the path of agricultural energy structure increases carbon emissions. Currently, China is facing contradictions between agricultural development and environmental protection (Xu and Lin, 2017), and the traditional carbon-based agricultural development model is no longer suitable for current agricultural production. The unreasonable consumption structure of China's agriculture, dominated by chemical fertilizers and pesticides, has been maintained for a long time (Sarkar et al., 2022). However, the continuously increasing energy input to improve the economic benefits of agricultural products in the traditional production process not only has a significant impact on the ecological environment but also leads to agricultural carbon emission pollution becoming more and more serious (Balsalobre-Lorente et al., 2019). Therefore, adjusting the unreasonable agricultural energy structure has become one of the significant problems to be solved urgently in China's agricultural low-carbon emission reduction. The optimization and improvement in the agricultural energy structure (ES) should be strengthened and actively promote the role of green technological advancement to enhance the agricultural energy framework and decrease unreasonable input of energy elements in agricultural production.
The excessive dependence on fossil fuels should be lowered, and an increase in the use of biomass energy and biogas should be endorsed within core agricultural activities. The government should reasonably lower the total amount of agricultural energy consumption of agricultural production, gradually change the structure of agricultural energy utilization, and promote high-efficiency, energy-saving, and green emission reduction technologies in agriculture. The proper use of chemical fertilizers and pesticides has to be confirmed to reduce environmental pollution. The present findings add to the existing corpus of knowledge and facilitate quantitative measures of how green technology affects the decrease of carbon emissions. Moreover, the results align with prior studies (Ismael et al., 2018; Liu and Yang, 2021). The study also articulated that the impacts of AcGTP, AeGTP, and AGTP in the central area on carbon release concentration through EF are all significantly positive. The direct effect of the agricultural GT progress within China's western region is greater than that of the central region and more significant than that of the eastern region. This outcome is parallel to the study of Xu et al. (2019). For this reason, the Chinese government vigorously develops and promotes modern industries that can be facilitated resource efficiencies and environmental safety, strengthen ecological restoration, and gradually become a regionally crucial ecological area. The state restricts Western China as a development zone for its weak resource endowment capacity (Chen Y. et al., 2021).
Previous research (such as Tian et al., 2014; Han et al., 2018; Cui et al., 2022) identified that the level of agricultural carbon emissions in the eastern region is lower than the national average and reached the highest point of total agricultural carbon emissions in 2010, and has continued to decline since then, with the level of carbon emission reduction at a top level. The current study also found similar findings. The main reason is that the eastern region has superior natural resources, promising economic development, and the best technology, capital, and infrastructure, which provide favorable support for solving the problems of high agricultural investment (Cui et al., 2021a). Thus, the space for carbon emission reduction in eastern China is lower than that of the middle western region. Therefore, the progress of green technology in Western China is better, which also shows that China has achieved remarkable results in green and low-carbon construction in the west. The government should implement regionally differentiated agricultural energy conservation and emission reduction strategies: Improve agricultural energy efficiency (EF), emphasize the demonstration and leading role of advantageous zones, and realize cooperation and cross-regional synergy improvement of agricultural energy efficiency between each zone. Within the area, identify target zones with the same production conditions and similar industrial characteristics, especially contiguous zones where large-scale operations are operable. Lastly, use a point-to-face approach to promote the experience that can be used for reference.
The intermediary influence of AcGTP in the eastern area through the agriculture industrial structure (PS) is significantly positive, and the direction of agricultural development should be continuously adjusted to reduce the degree of dependence on agricultural production energy. Thus, the government should aim at the heterogeneity of agricultural energy efficiency (EF) in Western China and design improvement strategies based on its characteristics. In the eastern area, the government should promote agricultural technology innovation, encourage the development of high-value-added agricultural practices such as organic agriculture, urban agriculture, and facility agriculture, and continue to explore the introduction of modern industrial development concepts. The government should endorse innovation-driven development by significantly emphasizing the central and western zones. Similarly, according to the current policy scenario of each region and combining the specific characteristics of different zones, agricultural carbon release reduction policies should be modified.
6. Conclusion
China is acknowledged as the nation with the highest CO2 emissions due to its massive population and rapid economic growth. However, as socioeconomic progress accelerates, more fossil fuel is used by advanced technologies, which impacts agricultural economic expansion both explicitly and implicitly. Based on the bottlenecks of carbon emissions, the Chinese government has set a target date of 2060 for reaching carbon neutrality. Therefore, based on the empirical data from the planting industry in China, the study aims to explore the impacts of agricultural green technology advancement on agricultural carbon emission reduction. In the study, we used data envelopment analysis to outline the core framework of the study. In addition, we have critically revealed the intermediary impact of green technology advancement by utilizing the intermediary impact model. First of all, the agricultural green technology advancement has been outlined as dual approaches of resource-saving and emission reduction green technological advancement and thus used a two-way econometric model to outline the impacts of both approaches. Then, the structure of the whole sector has been divided into industry and energy structures. After that, the whole sample of China's planting industry was divided into three distinct regions (east, central, and west) to evaluate the impact of regions.
Empirical findings indicate that energy and resource-saving technology positively impact reducing carbon emissions. At the same time, the impact on the agricultural industry structure and agricultural energy structure is significantly negative, and the path of carbon reduction intensity is energy and resource efficiency, followed by agricultural industry structure and agricultural energy structure. The study also indicated that carbon emission is substantially increased in the region where the agricultural industry structure is well-developed. In terms of region, the western area has a more significant direct impact than either the central or eastern regions. These findings contribute to a better understanding of the association between green technology advancement and carbon emission reduction. However, the model and setup used in the study can be extended to explore relatively larger regions. More specifically, a multination perspective should provide more robust outcomes. This article is mainly from the macro point of view to consider the mechanism of analysis, but in fact, the impact of green technology progress on carbon emissions is a more micro-level behavioral change. Because of the limitations of the data, micro-mechanisms have not been studied in this article. In the future, it is worth further exploring the mechanism of technological progress on carbon emissions under the micro-mechanism and exploring farmers' preferences under spatial heterogeneity. The study utilized data envelopment analysis. However, DEA does not allow project comparisons using multiple measures. Thus, future studies should implement more structured tools, such as the slacks-based model (SBM) and the structural equation model (SEM), for managing multiple measures.
Data availability statement
The raw data supporting the conclusions of this article will be made available by the authors, without undue reservation.
Author contributions
SX and AS: conceptualization, methodology, data curation, and writing—original draft preparation. SX and DY: software and formal analysis. ZH, AS, and TF: validation. TF: investigation and visualization. AS: resources and project administration. AS and ZH: writing—review and editing. ZH: supervision. SX: funding acquisition. All authors have read and agreed to the published version of the manuscript.
Acknowledgments
We are thankful to the reviewers for making our study more presentable and maintaining higher quality.
Conflict of interest
The authors declare that the research was conducted in the absence of any commercial or financial relationships that could be construed as a potential conflict of interest.
Publisher's note
All claims expressed in this article are solely those of the authors and do not necessarily represent those of their affiliated organizations, or those of the publisher, the editors and the reviewers. Any product that may be evaluated in this article, or claim that may be made by its manufacturer, is not guaranteed or endorsed by the publisher.
References
Abou-Elela, S. I. (2019). “Constructed wetlands: the green technology for municipal wastewater treatment and reuse in agriculture,” in Unconventional Water Resources and Agriculture in Egypt The Handbook of Environmental Chemistry, ed A. M. Negm (Cham: Springer International Publishing), 189–239. doi: 10.1007/698_2017_69
Aldieri, L., Brahmi, M., Chen, X., and Vinci, C. P. (2021). Knowledge spillovers and technical efficiency for cleaner production: an economic analysis from agriculture innovation. J. Clean. Prod. 320, 128830. doi: 10.1016/j.jclepro.2021.128830
Balcombe, K., Fraser, I., Latruffe, L., Rahman, M., and Smith, L. (2008). An application of the DEA double bootstrap to examine sources of efficiency in Bangladesh rice farming. Appl. Econ. 40, 1919–1925. doi: 10.1080/00036840600905282
Balsalobre-Lorente, D., Driha, O. M., Bekun, F. V., and Osundina, O. A. (2019). Do agricultural activities induce carbon emissions? The BRICS experience. Environ. Sci. Pollut. Res. 26, 25218–25234. doi: 10.1007/s11356-019-05737-3
Baron, R. M., and Kenny, D. A. (1986). The moderator-mediator variable distinction in social psychological research: conceptual, strategic, and statistical considerations. J. Pers. Soc. Psychol. 51, 1173–1182. doi: 10.1037/0022-3514.51.6.1173
Bennett, E. M. (2017). Changing the agriculture and environment conversation. Nat. Ecol. Evol. 1, 1–2. doi: 10.1038/s41559-016-0018
Brookes, L. (1990). The greenhouse effect: the fallacies in the energy efficiency solution. Energy Policy 18, 199–201. doi: 10.1016/0301-4215(90)90145-T
Carrillo, M. J. (2021). Migrant Flows: hydraulic infrastructure, agricultural industrialization, and environmental change in Western Mexico, 1940–64. Environ. Hist. 26, 231–254. doi: 10.1093/envhis/emaa081
Chen, J., Gao, M., Mangla, S. K., Song, M., and Wen, J. (2020). Effects of technological changes on China's carbon emissions. Technol. Forecast. Soc. Change 153, 119938. doi: 10.1016/j.techfore.2020.119938
Chen, Y., and Liu, Y. (2021). How biased technological progress sustainably improve the energy efficiency: an empirical research of manufacturing industry in China. Energy 230, 120823. doi: 10.1016/j.energy.2021.120823
Chen, Y., Miao, J., and Zhu, Z. (2021). Measuring green total factor productivity of China's agricultural sector: a three-stage SBM-DEA model with non-point source pollution and CO2 emissions. J. Clean. Prod. 318, 128543. doi: 10.1016/j.jclepro.2021.128543
Chen, Z., Sarkar, A., Rahman, A., Li, X., and Xia, X. (2021). Exploring the drivers of green agricultural development (GAD) in China: a spatial association network structure approaches. Land Use Policy 112, 105827. doi: 10.1016/j.landusepol.2021.105827
Cui, Y., Khan, S. U., Deng, Y., and Zhao, M. (2021a). Regional difference decomposition and its spatiotemporal dynamic evolution of Chinese agricultural carbon emission: considering carbon sink effect. Environ. Sci. Pollut. Res. 28, 38909–38928. doi: 10.1007/s11356-021-13442-3
Cui, Y., Khan, S. U., Deng, Y., Zhao, M., and Hou, M. (2021b). Environmental improvement value of agricultural carbon reduction and its spatiotemporal dynamic evolution: evidence from China. Sci. Total Environ. 754, 142170. doi: 10.1016/j.scitotenv.2020.142170
Cui, Y., Khan, S. U., Sauer, J., and Zhao, M. (2022). Exploring the spatiotemporal heterogeneity and influencing factors of agricultural carbon footprint and carbon footprint intensity: embodying carbon sink effect. Sci. Total Environ. 846, 157507. doi: 10.1016/j.scitotenv.2022.157507
DeAngelo, J., Saenz, B. T., Arzeno-Soltero, I. B., Frieder, C. A., Long, M. C., Hamman, J., et al. (2022). Economic and biophysical limits to seaweed farming for climate change mitigation. Nat. Plants 9, 45–57. doi: 10.1038/s41477-022-01305-9
Du, K., and Li, J. (2019). Towards a green world: how do green technology innovations affect total-factor carbon productivity. Energy Policy 131, 240–250. doi: 10.1016/j.enpol.2019.04.033
Du, K., Li, P., and Yan, Z. (2019). Do green technology innovations contribute to carbon dioxide emission reduction? Empirical evidence from patent data. Technol. Forecast. Soc. Change 146, 297–303. doi: 10.1016/j.techfore.2019.06.010
Feng, C., Chu, F., Ding, J., Bi, G., and Liang, L. (2015). Carbon emissions abatement (CEA) allocation and compensation schemes based on DEA. Omega 53, 78–89. doi: 10.1016/j.omega.2014.12.005
Frank, S., Beach, R., Havlík, P., Valin, H., Herrero, M., Mosnier, A., et al. (2018). Structural change as a key component for agricultural non-CO2 mitigation efforts. Nat. Commun. 9, 1060. doi: 10.1038/s41467-018-03489-1
Frank, S., Havlík, P., Stehfest, E., van Meijl, H., Witzke, P., Pérez-Domínguez, I., et al. (2019). Agricultural non-CO2 emission reduction potential in the context of the 1.5°C target. Nat. Clim. Change 9, 66–72. doi: 10.1038/s41558-018-0358-8
Gallagher, K. S., Zhang, F., Orvis, R., Rissman, J., and Liu, Q. (2019). Assessing the policy gaps for achieving China's climate targets in the Paris agreement. Nat. Commun. 10, 1256. doi: 10.1038/s41467-019-09159-0
Gao, Y., Zheng, J., and Bu, M. (2014). Rural-urban income gap and agricultural growth in China: An empirical study on the provincial panel data, 1978–2010. China Agric. Econ. Rev. 6, 92–107. doi: 10.1108/CAER-02-2012-0016
Ge, X., Sarkar, A., Ruishi, S., Rahman, M. A., Azim, J. A., Zhang, S., et al. (2022). Determinants of sick and dead pig waste recycling—a case study of Hebei, Shandong, and Henan Provinces in China. Animals 12, 775. doi: 10.3390/ani12060775
Gonzalez-Martinez, A. R., Jongeneel, R., Kros, H., Lesschen, J. P., de Vries, M., Reijs, J., et al. (2021). Aligning agricultural production and environmental regulation: an integrated assessment of the Netherlands. Land Use Policy 105, 105388. doi: 10.1016/j.landusepol.2021.105388
Grzelak, A., Guth, M., Matuszczak, A., Czyzewski, B., and Brelik, A. (2019). Approaching the environmental sustainable value in agriculture: how factor endowments foster the eco-efficiency. J. Clean. Prod. 241, 118304. doi: 10.1016/j.jclepro.2019.118304
Guo, R., and Yuan, Y. (2020). Different types of environmental regulations and heterogeneous influence on energy efficiency in the industrial sector: evidence from Chinese provincial data. Energy Policy 145, 111747. doi: 10.1016/j.enpol.2020.111747
Han, H., Zhong, Z., Guo, Y., Xi, F., and Liu, S. (2018). Coupling and decoupling effects of agricultural carbon emissions in China and their driving factors. Environ. Sci. Pollut. Res. 25, 25280–25293. doi: 10.1007/s11356-018-2589-7
Han, J., Qu, J., Maraseni, T. N., Xu, L., Zeng, J., and Li, H. (2021). A critical assessment of provincial-level variation in agricultural GHG emissions in China. J. Environ. Manage. 296, 113190. doi: 10.1016/j.jenvman.2021.113190
Hasanov, F. J., Khan, Z., Hussain, M., and Tufail, M. (2021). Theoretical framework for the carbon emissions effects of technological progress and renewable energy consumption. Sustain. Dev. 29, 810–822. doi: 10.1002/sd.2175
He, P., Zhang, J., and Li, W. (2021). The role of agricultural green production technologies in improving low-carbon efficiency in China: necessary but not effective. J. Environ. Manage. 293, 112837. doi: 10.1016/j.jenvman.2021.112837
He, Y., Wang, H., Chen, R., Hou, S., and Xu, D. (2022). The forms, channels and conditions of regional agricultural carbon emission reduction interaction: a provincial perspective in China. Int. J. Environ. Res. Public Health 19, 10905. doi: 10.3390/ijerph191710905
Hong, C., Burney, J. A., Pongratz, J., Nabel, J. E. M. S., Mueller, N. D., Jackson, R. B., et al. (2021). Global and regional drivers of land-use emissions in 1961–2017. Nature 589, 554–561. doi: 10.1038/s41586-020-03138-y
Hong, M., Tian, M., and Wang, J. (2022). Digital inclusive finance, agricultural industrial structure optimization and agricultural green total factor productivity. Sustainability 14, 11450. doi: 10.3390/su141811450
Hu, Z. (2018). A research on the affecting factors of farmers' comprehensive utilization of straw in china. IOP Conf. Ser. Earth Environ. Sci. 170, 022134. doi: 10.1088/1755-1315/170/2/022134
Ismael, M., Srouji, F., and Boutabba, M. A. (2018). Agricultural technologies and carbon emissions: evidence from Jordanian economy. Environ. Sci. Pollut. Res. 25, 10867–10877. doi: 10.1007/s11356-018-1327-5
Ji, H., and Hoti, A. (2022). Green economy based perspective of low-carbon agriculture growth for total factor energy efficiency improvement. Int. J. Syst. Assur. Eng. Manag. 13, 353–363. doi: 10.1007/s13198-021-01421-3
Kang, Z.-Y., Li, K., and Qu, J. (2018). The path of technological progress for China's low-carbon development: evidence from three urban agglomerations. J. Clean. Prod. 178, 644–654. doi: 10.1016/j.jclepro.2018.01.027
Karimi Alavijeh, N., Salehnia, N., Salehnia, N., and Koengkan, M. (2022). The effects of agricultural development on CO2 emissions: empirical evidence from the most populous developing countries. Environ. Dev. Sustain. doi: 10.1007/s10668-022-02567-1
Khoshnevisan, B., Rafiee, S., Omid, M., and Mousazadeh, H. (2013). Applying data envelopment analysis approach to improve energy efficiency and reduce GHG (greenhouse gas) emission of wheat production. Energy 58, 588–593. doi: 10.1016/j.energy.2013.06.030
Khoshroo, A., Izadikhah, M., and Emrouznejad, A. (2018). Improving energy efficiency considering reduction of CO2 emission of turnip production: a novel data envelopment analysis model with undesirable output approach. J. Clean. Prod. 187, 605–615. doi: 10.1016/j.jclepro.2018.03.232
Kumar, V., Kumar, P., and Singh, J. (2019). An introduction to contaminants in agriculture and environment. Contam. Agric. Environ. Health Risks Remediat. 1, 1–8. doi: 10.26832/AESA-2019-CAE-0159-01
Li, X., Liu, Y., and Zhao, R. (2021). A study on the influence of agricultural insurance on the upgrading and optimization of agricultural industrial structure in China-an empirical analysis based on GMM model of panel system. E3S Web Conf. 275, 01050. doi: 10.1051/e3sconf/202127501050
Liao, M., and Ren, Y. (2020). The ‘double-edged effect' of progress in energy-biased technology on energy efficiency: a comparison between the manufacturing sector of China and Japan. J. Environ. Manage. 270, 110794. doi: 10.1016/j.jenvman.2020.110794
Lin Zhang, Y., Yan Fang, F., Wei Ming, S., Li Hong, X., Shen Qiang, W., Xiang Fu, S., et al. (2013). Review of the advances and development trends in agricultural non-point source pollution control in China. Zhongguo Shengtai Nongye Xuebao 21, 96–101.
Lin, B., and Xu, B. (2018). Factors affecting CO2 emissions in China's agriculture sector: a quantile regression. Renew. Sustain. Energy Rev. 94, 15–27. doi: 10.1016/j.rser.2018.05.065
Liu, B., Gu, W., Yang, Y., Lu, B., Wang, F., Zhang, B., et al. (2021). Promoting potato as staple food can reduce the carbon–land–water impacts of crops in China. Nat. Food 2, 570–577. doi: 10.1038/s43016-021-00337-2
Liu, M., and Yang, L. (2021). Spatial pattern of China's agricultural carbon emission performance. Ecol. Indic. 133, 108345. doi: 10.1016/j.ecolind.2021.108345
Lovell, C. A. K. (2003). The decomposition of malmquist productivity indexes. J. Prod. Anal. 20, 437–458. doi: 10.1023/A:1027312102834
Malerba, F. (2007). “Innovation and the evolution of industries,” in Innovation, Industrial Dynamics and Structural Transformation: Schumpeterian Legacies, eds U. Cantner, and F. Malerba (Berlin: Springer), 7–27. doi: 10.1007/978-3-540-49465-2_2
Meirun, T., Mihardjo, L. W., Haseeb, M., Khan, S. A. R., and Jermsittiparsert, K. (2021). The dynamics effect of green technology innovation on economic growth and CO2 emission in Singapore: new evidence from bootstrap ARDL approach. Environ. Sci. Pollut. Res. 28, 4184–4194. doi: 10.1007/s11356-020-10760-w
Mohammadi, A., Rafiee, S., Jafari, A., Dalgaard, T., Knudsen, M. T., Keyhani, A., et al. (2013). Potential greenhouse gas emission reductions in soybean farming: a combined use of life cycle assessment and data envelopment analysis. J. Clean. Prod. 54, 89–100. doi: 10.1016/j.jclepro.2013.05.019
Moore, F. C., Baldos, U., Hertel, T., and Diaz, D. (2017). New science of climate change impacts on agriculture implies higher social cost of carbon. Nat. Commun. 8, 1607. doi: 10.1038/s41467-017-01792-x
Moore, J. W. (2015). Cheap food and bad climate: from surplus value to negative value in the capitalist world-ecology. Crit. Hist. Stud. 2, 1–43. doi: 10.1086/681007
Mulugetta, Y., and Urban, F. (2010). Deliberating on low carbon development. Energy Policy 38, 7546–7549. doi: 10.1016/j.enpol.2010.05.049
Nabavi-Pelesaraei, A., Abdi, R., Rafiee, S., and Mobtaker, H. G. (2014). Optimization of energy required and greenhouse gas emissions analysis for orange producers using data envelopment analysis approach. J. Clean. Prod. 65, 311–317. doi: 10.1016/j.jclepro.2013.08.019
Naseem, S., and Guang Ji, T. (2021). A system-GMM approach to examine the renewable energy consumption, agriculture and economic growth's impact on CO2 emission in the SAARC region. GeoJournal 86, 2021–2033. doi: 10.1007/s10708-019-10136-9
Norse, D. (2012). Low carbon agriculture: objectives and policy pathways. Environ. Dev. 1, 25–39. doi: 10.1016/j.envdev.2011.12.004
Pang, J., Li, H., Lu, C., Lu, C., and Chen, X. (2020). Regional differences and dynamic evolution of carbon emission intensity of agriculture production in China. Int. J. Environ. Res. Public Health 17, 7541. doi: 10.3390/ijerph17207541
Paroussos, L., Fragkiadakis, K., and Fragkos, P. (2020). Macro-economic analysis of green growth policies: the role of finance and technical progress in Italian green growth. Clim. Change 160, 591–608. doi: 10.1007/s10584-019-02543-1
Piwowar, A. (2019). Low-carbon agriculture in Poland: theoretical and practical challenges. Polish J. Environ. Stud. 28, 2785–2792. doi: 10.15244/pjoes/92211
Rebolledo-Leiva, R., Angulo-Meza, L., Iriarte, A., and González-Araya, M. C. (2017). Joint carbon footprint assessment and data envelopment analysis for the reduction of greenhouse gas emissions in agriculture production. Sci. Total Environ. 593–594, 36–46. doi: 10.1016/j.scitotenv,.2017.03.147
Sá, J. C., Lal, R., Cerri, C. C., Lorenz, K., Hungria, M., and de Faccio Carvalho, P. C. (2017). Low-carbon agriculture in South America to mitigate global climate change and advance food security. Environ. Int. 98, 102–112. doi: 10.1016/j.envint.2016.10.020
Sarkar, A., Azim, J. A., Asif, A. A., Qian, L., and Peau, A. K. (2021). Structural equation modeling for indicators of sustainable agriculture: prospective of a developing country's agriculture. Land Use Policy 109, 105638. doi: 10.1016/j.landusepol.2021.105638
Sarkar, A., Wang, H., Rahman, A., Qian, L., and Memon, W. H. (2022). Evaluating the roles of the farmer's cooperative for fostering environmentally friendly production technologies-a case of kiwi-fruit farmers in Meixian, China. J. Environ. Manage. 301, 113858. doi: 10.1016/j.jenvman.2021.113858
Schaller, R. R. (1997). Moore's law: past, present and future. IEEE Spect. 34, 52–59. doi: 10.1109/6.591665
Shen, Z., Baležentis, T., Chen, X., and Valdmanis, V. (2018). Green growth and structural change in Chinese agricultural sector during 1997–2014. China Econ. Rev. 51, 83–96. doi: 10.1016/j.chieco.2018.04.014
Sjöholm, F., and Lundin, N. (2013). Foreign firms and indigenous technology development in the people's republic of China. Asian Dev. Rev. 30, 49–75. doi: 10.1162/ADEV_a_00015
Song, M., Chen, Y., and An, Q. (2018). Spatial econometric analysis of factors influencing regional energy efficiency in China. Environ. Sci. Pollut. Res. 25, 13745–13759. doi: 10.1007/s11356-018-1574-5
Song, M., Peng, L., Shang, Y., and Zhao, X. (2022). Green technology progress and total factor productivity of resource-based enterprises: a perspective of technical compensation of environmental regulation. Technol. Forecast. Soc. Change 174, 121276. doi: 10.1016/j.techfore.2021.121276
Song, M., and Wang, S. (2018). Measuring environment-biased technological progress considering energy saving and emission reduction. Proc. Saf. Environ. Prot. 116, 745–753. doi: 10.1016/j.psep.2017.08.042
Song, M., Zheng, W., and Wang, S. (2017). Measuring green technology progress in large-scale thermoelectric enterprises based on Malmquist–Luenberger life cycle assessment. Resourc. Conserv. Recycl. 122, 261–269. doi: 10.1016/j.resconrec.2017.03.001
Syp, A., Faber, A., Borzecka-Walker, M., and Osuch, D. (2015). Assessment of greenhouse gas emissions in winter wheat farms using data envelopment analysis approach. Pol. J. Environ. Stud. 24, 2197–2203. doi: 10.15244/pjoes/39682
Tang, K., Liu, Y., Zhou, D., and Qiu, Y. (2021). Urban carbon emission intensity under emission trading system in a developing economy: evidence from 273 Chinese cities. Environ. Sci. Pollut. Res. 28, 5168–5179. doi: 10.1007/s11356-020-10785-1
Tang, K., and Wang, D. (2023). “Cost-Effectiveness of agricultural carbon reduction in China,” in Carbon-Neutral Pathways for China: Economic Issues, ed K. Tang (Singapore: Springer Nature), 81–94. doi: 10.1007/978-981-19-5562-4_6
Tian, X., Bai, F., Jia, J., Liu, Y., and Shi, F. (2019). Realizing low-carbon development in a developing and industrializing region: impacts of industrial structure change on CO2 emissions in southwest China. J. Environ. Manage. 233, 728–738. doi: 10.1016/j.jenvman.2018.11.078
Tian, Y., Zhang, J., and He, Y. (2014). Research on spatial-temporal characteristics and driving factor of agricultural carbon emissions in China. J. Integr. Agric. 13, 1393–1403. doi: 10.1016/S2095-3119(13)60624-3
van der Werf, E. (2008). Production functions for climate policy modeling: an empirical analysis. Energy Econ. 30, 2964–2979. doi: 10.1016/j.eneco.2008.05.008
Wang, G., Yang, D., and Xiong, C. (2021). Dynamic evaluation and spatial distribution characteristics of agricultural green development level in restricted development areas: a case study of Yili River Valley, China. Pol. J. Environ. Stud. 30, 4255–4266. doi: 10.15244/pjoes/131815
Wang, M., and Feng, C. (2020). The impacts of technological gap and scale economy on the low-carbon development of China's industries: An extended decomposition analysis. Technol. Forecast. Soc. Change 157, 120050. doi: 10.1016/j.techfore.2020.120050
Wei, Y.-M., Han, R., Wang, C., Yu, B., Liang, Q.-M., Yuan, X.-C., et al. (2020). Self-preservation strategy for approaching global warming targets in the post-Paris agreement era. Nat. Commun. 11, 1624. doi: 10.1038/s41467-020-15453-z
Wu, J., Chu, J.-F., and Liang, L. (2016). Target setting and allocation of carbon emissions abatement based on DEA and closest target: an application to 20 APEC economies. Nat. Haz. 84, 279–296. doi: 10.1007/s11069-015-1973-6
Xiong, C., Yang, D., Xia, F., and Huo, J. (2016). Changes in agricultural carbon emissions and factors that influence agricultural carbon emissions based on different stages in Xinjiang, China. Sci. Rep. 6, 36912. doi: 10.1038/srep36912
Xu, B., and Lin, B. (2017). Factors affecting CO2 emissions in China's agriculture sector: evidence from geographically weighted regression model. Energy Policy 104, 404–414. doi: 10.1016/j.enpol.2017.02.011
Xu, X., Huang, X., Huang, J., Gao, X., and Chen, L. (2019). Spatial-temporal characteristics of agriculture green total factor productivity in China, 1998–2016: based on more sophisticated calculations of carbon emissions. Int. J. Environ. Res. Public Health 16, 3932. doi: 10.3390/ijerph16203932
Xu, X., Yang, H., and Yang, H. (2021). The threshold effect of agricultural energy consumption on agricultural carbon emissions: a comparison between relative poverty regions and other regions. Environ. Sci. Pollut. Res. 28, 55592–55602. doi: 10.1007/s11356-021-14831-4
Xu, Z., Chen, X., Liu, J., Zhang, Y., Chau, S., Bhattarai, N., et al. (2020). Impacts of irrigated agriculture on food–energy–water–CO2 nexus across metacoupled systems. Nat. Commun. 11, 5837. doi: 10.1038/s41467-020-19520-3
Yan, Z., Zou, B., Du, K., and Li, K. (2020). Do renewable energy technology innovations promote China's green productivity growth? Fresh evidence from partially linear functional-coefficient models. Energy Econ. 90, 104842. doi: 10.1016/j.eneco.2020.104842
Yang, G., Zha, D., Wang, X., and Chen, Q. (2020). Exploring the nonlinear association between environmental regulation and carbon intensity in China: the mediating effect of green technology. Ecol. Indic. 114, 106309. doi: 10.1016/j.ecolind.2020.106309
Yang, X., Jia, Z., Yang, Z., and Yuan, X. (2021). The effects of technological factors on carbon emissions from various sectors in China—a spatial perspective. J. Clean. Prod. 301, 126949. doi: 10.1016/j.jclepro.2021.126949
Yue, D., Sarkar, A., Yu, C., Qian, L., and Minjuan, Z. (2021). The evolution of factors influencing green technological progress in terms of carbon reduction: a spatial-temporal tactic within agriculture industries of China. Front. Energy Res. 9, 783. doi: 10.3389/fenrg.2021.661719
Yun, W. (2016). “Brief review of low-carbon agricultural economy,” in Ecological Economics and Harmonious Society Research Series on the Chinese Dream and China's Development Path, eds F. Qu, R. Sun, Z. Guo, and F. Yu (Singapore: Springer), 97–109. doi: 10.1007/978-981-10-0461-2_9
Yurtkuran, S. (2021). The effect of agriculture, renewable energy production, and globalization on CO2 emissions in Turkey: a bootstrap ARDL approach. Renew. Energy 171, 1236–1245. doi: 10.1016/j.renene.2021.03.009
Zhang, X., and Chen, H. (2021). Green agricultural development based on information communication technology and the panel space measurement model. Sustainability 13, 1147. doi: 10.3390/su13031147
Zhang, Y., Wang, W., Liang, L., Wang, D., Cui, X., and Wei, W. (2020). Spatial-temporal pattern evolution and driving factors of China's energy efficiency under low-carbon economy. Sci. Total Environ. 739, 140197. doi: 10.1016/j.scitotenv.2020.140197
Zhao, L., Lv, Y., Wang, C., Xue, J., Yang, Y., and Li, D. (2023). Embodied greenhouse gas emissions in the international agricultural trade. Sustain. Prod. Consump. 35, 250–259. doi: 10.1016/j.spc.2022.11.001
Zhao, L., Zha, Y., Wei, K., and Liang, L. (2017). A target-based method for energy saving and carbon emissions reduction in China based on environmental data envelopment analysis. Ann. Oper. Res. 255, 277–300. doi: 10.1007/s10479-016-2163-y
Zhao, R., Liu, Y., Tian, M., Ding, M., Cao, L., Zhang, Z., et al. (2018). Impacts of water and land resources exploitation on agricultural carbon emissions: the water-land-energy-carbon nexus. Land Use Policy 72, 480–492. doi: 10.1016/j.landusepol.2017.12.029
Zhao, Y., Wang, Q., Jiang, S., Zhai, J., Wang, J., He, G., et al. (2020). Irrigation water and energy saving in well irrigation district from a water-energy nexus perspective. J. Clean. Prod. 267, 122058. doi: 10.1016/j.jclepro.2020.122058
Zhong, R., He, Q., and Qi, Y. (2022). Digital economy, agricultural technological progress, and agricultural carbon intensity: evidence from China. Int. J. Environ. Res. Public Health 19, 6488. doi: 10.3390/ijerph19116488
Keywords: green, technological advancement, energy efficiency, agriculture, industrial structure, energy structure, slacks-based measure (SBM), CO2
Citation: Xinxing S, Sarkar A, Yue D, Hongbin Z and Fangyuan T (2023) The influences of the advancement of green technology on agricultural CO2 release reduction: A case of Chinese agricultural industry. Front. Sustain. Food Syst. 7:1096381. doi: 10.3389/fsufs.2023.1096381
Received: 30 November 2022; Accepted: 06 February 2023;
Published: 02 March 2023.
Edited by:
Eduardo Aguilera, Centro de Estudios e Investigación para la Gestión de Riesgos Agrarios y Medioambientales (CEIGRAM), SpainReviewed by:
László Vasa, Széchenyi István University, HungaryZhang Yucui, Chinese Academy of Sciences (CAS), China
Copyright © 2023 Xinxing, Sarkar, Yue, Hongbin and Fangyuan. This is an open-access article distributed under the terms of the Creative Commons Attribution License (CC BY). The use, distribution or reproduction in other forums is permitted, provided the original author(s) and the copyright owner(s) are credited and that the original publication in this journal is cited, in accordance with accepted academic practice. No use, distribution or reproduction is permitted which does not comply with these terms.
*Correspondence: Zhu Hongbin, zhhbin02@nwsuaf.edu.cn