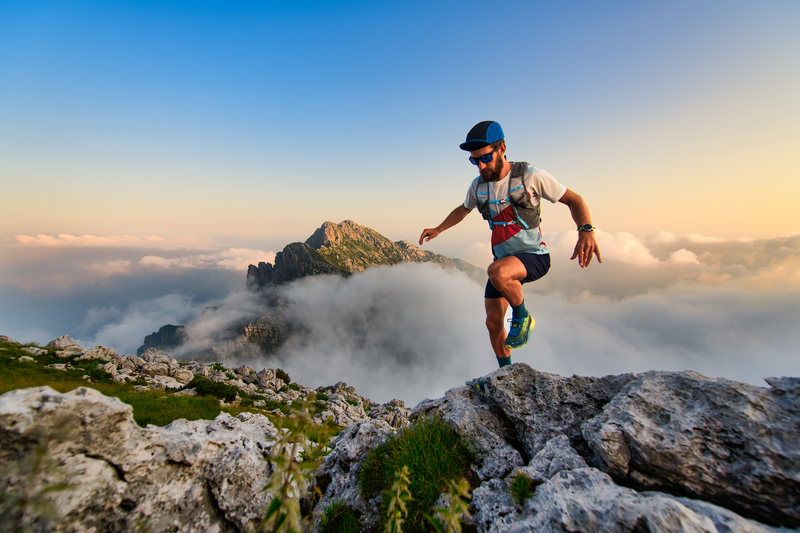
94% of researchers rate our articles as excellent or good
Learn more about the work of our research integrity team to safeguard the quality of each article we publish.
Find out more
ORIGINAL RESEARCH article
Front. Sustain. Food Syst. , 24 July 2023
Sec. Land, Livelihoods and Food Security
Volume 7 - 2023 | https://doi.org/10.3389/fsufs.2023.1091346
Introduction: Insight into the resilience of local food systems—variability driven by climate, conflict, and food price shocks—is critical for the treatment and prevention of child acute malnutrition.
Methods: We use a combination of latent class mixed modeling and time-to-event analysis to develop and test a measure of resilience that is outcome-based, sensitive to specific shocks and stressors, and captures the enduring effects of how frequently and severely children face the risk of acute malnutrition.
Results: Harnessing a high-resolution longitudinal dataset with anthropometric information on 5,597 Kenyan households for the 2016–20 period, we identify resilience trajectories for 141 wards across Kenya. These trajectories—characterized by variation in the duration and severity of episodes of acute malnutrition—are associated with differential risk: (1) some 57% of wards exhibit an increasing trajectory—high household risk despite growing resilience; (2) 39% exhibit chronic characteristics—showing no real signs of recovery after an episode of crisis; (3) 3% exhibit robust characteristics—low variability with low-levels of individual household risk; whereas (4) 1% show a steady decrease in resilience—associated with high levels household risk.
Discussion: Our findings highlight the importance of measuring resilience at the ward-level in order to better understand variation in the nutritional status of rural households.
Child acute malnutrition is characterized by rapid and substantial weight loss in otherwise healthy children, most often in a matter of days or weeks (FAO et al., 2022). As opposed to stunting—indicative of a long-term nutritional deficit (WHO, 2009, 2021)—the prevalence of acute malnutrition is widely recognized as an early warning indicator for an impending nutrition crisis (FewsNet, 2018; IPC, 2022). In the drylands of Africa, child acute malnutrition is endemic. Each year, protracted regional nutrition crises account for over half of all global humanitarian assistance (Young, 2020). Extreme weather brought about by climate change and ecosystem fragility interact with novel forms of sub-national conflict to produce new configurations of risk (WFP, 2020; Anderson et al., 2021; Brown et al., 2021; Grace et al., 2022). Humanitarian actors consequently seek to alter the nature of their interventions, increasingly adopting a more proactive, as a complement to what has typically been a more reactive, stance. Building resilience in local food systems—fostering the capacity of households and communities to provide food security in times of crisis (Hamm and Bellows, 2003; Tendall et al., 2015; Mazzocchi et al., 2022; Zurek et al., 2022)—features prominently in this regard.
Resilience, defined as “the ability of people to mitigate, withstand, and ‘bounce back' from shocks and stresses” (Maxwell et al., 2017, p. 1) is directly undermined by child acute malnutrition, given the immediate pathological consequences as well as the strains placed on local food systems (Béné, 2020; Klassen and Murphy, 2020; Fanzo et al., 2021; Niles et al., 2021; Reisch, 2021; von Braun et al., 2021; Westerveld et al., 2021; Borman et al., 2022). Granular information on the diverse pathways through which malnutrition affects the ability of households and communities to cope and recover from crises—a measure of robust system behavior—is therefore key. Despite conceptual advances (Fraccascia et al., 2018; Lamothe et al., 2019; Alinovi et al., 2022) and general agreement that resilience measures should be (i) outcome-based, (ii) analyzed with regard to the experience of specific shocks and associated background stressors, (iii) emphasize long-lasting effects on the outcome variable, in contrast to similar concepts such as vulnerability, and (iv) subjected to tests of validity and reliability to assess predictive power, research on resilience and adaptation in the context of food security has fallen short in these regards (Constas et al., 2013; d'Errico et al., 2018; von Braun et al., 2021).
Case studies on household coping behavior are difficult to validate (Corbett, 1988; Watts and Bohle, 1993). Index-based measures of resilience (Browne et al., 2014; Boukary et al., 2016; FAO, 2016; Berg and Emran, 2020) represent a step forward in this regard, yet they do not account for sequential behavior over time (see Egamberdiev et al., 2023 for an exception). In a similar vein, scholarship that examines household behavior in the aftermath of natural disasters, specifically droughts or floods (Park et al., 2011; Alexander et al., 2013; Desai et al., 2015), tends to rely on cross-sectional surveys. These event-centered studies are unable to capture the possible “legacy effects” of previous episodes of crises and thus neglect the socio-environmental dynamics associated with repeated periods of stress and associated responses. By design, such approaches are limited in their ability to integrate notions of time and duration—the sequential analysis of protracted crises in relatively short-periods of time, or the notion of repeated household behaviors that form typical response trajectories (Lampousi et al., 2021; Ratz et al., 2022).
We address the need for a multi-dimensional, dynamic measure of resilience in this paper. Prior work operationalizes resilience as a household-level characteristic, capturing the ability of individual households to cope with shocks and stressors. The approach we develop integrates measurements at different units of analysis (Brown et al., 2020; Bakhtsiyarava and Grace, 2021; Grace et al., 2022; Wang and Do, 2023), tracing how household behavior shapes resilience at the ward-level while accounting for feedback loops and nonlinear scaling processes (Béné et al., 2015; Béné, 2020; Bhavnani et al., 2020, 2023). In a given ward, we measure how many children across households fall above/below the malnutrition threshold and to what extent. It follows that a ward is more (less) resilient, the fewer (larger) the number of children that fall below the threshold over time. Our outcome-based measure effectively captures the ratio of the “area” above and below the MUAC line—the greater the area above the line relative to the area below the line, the more resilient the ward as a whole. Integrated over time, this effectively captures a ward's resilience “trajectory”. Using latent class mixed modeling (LCMM), an unsupervised statistical learning method, we identify typical resilience trajectories for 141 Kenyan wards using longitudinal, anthropometric data on children from 5,597 Kenyan households for the 2016–20 period.
The paper is organized as follows. Section 2 describes materials and methods. Section 3, presents resilience trajectories identified by the LCMM and their association with differential household acute malnutrition risk. Section 4 discusses the implications of our findings for better food policies and interventions.
A recurring issue in the famine early warning community concerns the lack of high quality, disaggregated data to track changes in nutrition status over time (Cafiero et al., 2022; Deconinck et al., 2022). Data are most commonly collected as repeat cross-sections; when longitudinal data are available they are usually at a resolution that is insufficiently granular to track changes in household nutrition status (Brown et al., 2020). In the Kenyan context, child anthropometric information is commonly based on one or more waves of the Kenya Demographic Health Surveys (KDHS) that have been conducted in 2003, 2008/9, 2014, and 2022 (DHS, 2022), and to a lesser extent the SMART nutrition surveys (SMART, 2021). While the KDHS lacks temporal granularity, SMART surveys are conducted more frequently, albeit in a small number of counties. By comparison, household nutrition surveys conducted by the Kenyan National Drought Management Authority (NDMA) provide longitudinal coverage at monthly intervals. We use NDMA data on the nutrition status of households, sampled repeatedly over time, in combination with open source data on stressors. Supplementary Section 1 provides an overview of the data sources and aggregation rules.
The NDMA is a governmental drought early warning mechanism, tasked with nutrition information collection and monitoring in the most drought-prone regions in Kenya: the drylands. Figure 1 illustrates the 141 wards (ADM3)—so-called “sentinel sites”—that are spread across the arid (red) and semi-arid (pink) regions (NDMA, 2023). In each ward, the NDMA tracks monthly anthropometric information for children from 30 households (drawn from one of two villages) over a period of 12 months. After 12 months, the NDMA moves to another village within the same ward and re-samples children/households there. As livelihood strategies are homogeneous across villages, the purposive sample of 30 households is considered representative of a ward. Based on 49 consecutive survey rounds for the period between January 2016 and April 2020, the dataset features N = 54,839 observations from 5,597 individual households. The inclusion criteria for households are: (i) complete information on the outcome and identifier variables; and (ii) participation in a minimum of three consecutive survey rounds. On average, households are tracked over a period of 6 months (SD = 3.4). We use the Mid-Upper Arm Circumference (MUAC) as a basis to estimate outcome-based resilience, providing a more timely and accurate description of an unfolding humanitarian emergency relative to index-based resilience conceptualizations.
Household nutrition survey data is complemented with equally granular data on climate, conflict, and food price stressors to account for changes in contextual factors across Kenyan wards. For reasons of market price data availability, the unit of analysis for all exogenous shocks is county (ADM1)-month.
To measure the impact of climate change and extreme weather on resilience to acute malnutrition, we rely on the seasonal normalized difference vegetation index (NDVI) (Didan et al., 2015). The NDVI, a measure of “greenness”, is used as a proxy for the amount of vegetation produced, with greener wards being more food abundant. Therefore, the higher the value of vegetation, the more productive local agricultural and pastoral production. We first calculate monthly deviation rates from a 3-year long term average (LTA) and categorize each county-month as stressed or not. Next, we define the intensity of climate stress as the mean deviation from the LTA, and z-standardize such that values closer to 1 represent higher climate stressor intensity.
To account for the intensity of conflict, we draw on the Uppsala Conflict Data Program's Georeferenced Events Dataset (Sundberg and Melander, 2013; Hegre et al., 2020). The basic unit of analysis of UCDP-GED is the “event”—an individual incident of lethal violence committed by an organized actor against another organized actor, or against civilians occurring at a given time and place (Högbladh, 2020). This definition of conflict is restricted to events which result in a fatality as reported by the media. Smaller conflicts, episodes of cattle rustling between neighboring communities or natural resource conflicts, are effectively excluded. The intensity of conflict is defined as the mean number of fatalities in a county-month, and z-standardized such that values closer to 1 indicate more fatalities and higher conflict stressor intensity. Supplementary Section 1.2 uses a different dataset on conflict events, namely from the Armed Conflict Location & Event Data Project (Raleigh et al., 2010), to underpin the sensitivity of the conflict stresssor intensity measurement.
We use data on food prices in local markets from NDMA's “County Early Warning Bulletins” (NDMA, 2023) to account for the intensity of economic shocks. As a complement to nutrition surveys, the NDMA conducts “key informant assessments (KIA)” with local experts to assess the average monthly retail prices of key staple commodities. We analyze changes in the cost of maize—the staple food for all Kenyans for which data is readily available. We first calculate monthly deviation rates from a 3-year average and categorize each county-month as stressed or not. We then define the intensity of food price stress as the mean price increase above the LTA, and z-standardize such that values closer to 0 represent the lowest and values closer to 1 the highest prices.
We calculate ward resilience r using the integral of incidence of child acute malnutrition, operationalized as MUAC measurements m, in a given ward i (at time t) around the threshold w over time1:
Integrals Equations 2 and 3 capture the total weight of MUAC measurements m (in ward i, at time t) above and below the acute malnutrition threshold, respectively. They vary with the number of children in a sample of households in unit i at time t above or below the normative threshold w (risk of acute malnutrition, w = 135 mm), as well as with how far above/below these observations are, thereby permitting the identification of vulnerable areas. With the aggregation from child-level MUAC observations to household-level nutrition outcomes, we effectively account for one of the key shortcomings in the data: the inability to discern, with absolute certainty, whether exactly the same children have been observed repeatedly for a given household. We note that ward resilience r is highly suited to data-poor settings where time-intensive, multi-dimensional surveys are less frequent relative to MUAC assessments, and where the nutrition situation may change abruptly, and may be calculated for other specifications and threshold values, depending on the research question.
Our measure r effectively captures how many children fall above/below the malnutrition threshold and by what extent, for households in a given ward. The underlying rationale is that the greater the number of children with MUAC values above/further vs. below/closer to the normative threshold w, the more resilient the ward. Take two ostensibly similar wards with 90% of children above and 10% below the threshold. In the first, the 10% are much further below the threshold whereas the 90% are barely above the threshold. In the second, the 10% are just below and the 90% well-above. It follows that the local food system in the first ward is less resilient relative to the second, given that a smaller shock/stressor will likely shift the distribution of children affected by acute malnutrition significantly. Whereas, household resilience typically connects household characteristics—access to basic services, assets, adaptive capacity, and social safety nets (FAO, 2016)—to household-level nutritional outcomes such as food expenditure and dietary diversity, our measure captures the ability of local food systems, in this case wards, to respond to shocks—a complement to household level measures.
We use a latent class mixed model (LCMM) for the classification of ward-level nutrition dynamics into distinct resilience trajectories (Proust-Lima et al., 2014, 2016, 2022; Sène et al., 2014; Andrinopoulou et al., 2021). Latent class models have successfully been applied in the fields of criminology (Francis and Liu, 2015; Stone et al., 2023) and psychology (Simon et al., 2022), and more recently in human epidemiology (Lennon et al., 2018). We follow the eight-step framework proposed by Lennon et al. (2018) and the Guidelines for Reporting on Latent Trajectory Studies (“GRoLTS-Checklist”) (Van De Schoot et al., 2017) (see Supplementary Table S2) for model development and implement the analysis in R, using the LCMM package (Proust-Lima and Liquet, 2011, 2016) (see Supplementary Section 2.1). We compare model fit based on the Bayesian Information Criterion (BIC)—selecting the model with the lowest BIC (BIC = 13, 969) and at least 1% of observations in the resulting classes. In particular, we select the LCMM with a quadratic splines link-function, using ward resilience to acute malnutrition as the outcome variable, while controlling for three external shocks: climate, conflict, and economic stressor intensity. Mixed effects are used to account for the likely correlation of repeated measurements within the same unit. For each ward, we include a random intercept based on the assumption that that effects of confounding variables are broadly comparable across latent classes. With model convergence, each ward is assigned to a class with the highest posterior probability.
In a second step, we use the survival (Therneau and Lumley, 2015) and survminer (Kassambara et al., 2017) R-packages to specify Cox regression models to assess the adjusted influence of class membership on household risk of acute malnutrition in a given ward, depending on the latent class assigned. To evaluate simultaneously the effect of ward resilience and several household characteristics on the risk of falling below a normative nutrition threshold h(t), we assume:
with h0 as baseline hazard, i.e., the intercept of the linear regression model, at time t, and the hazard ratio exp(bi), whereby coefficients bi measure the impact of the resilience trajectory and our set of household control variables (xi). xi includes characteristics that other studies have found to be important predictors of the nutrition status, including the highest level of education (Fratkin, 2019) and gender of the household head (Rao et al., 2019), the predominant livelihood (Brown et al., 2020), as well as the main sources of income and water (Sick, 2014; Doss and Meinzen-Dick, 2015; McCarthy and Kilic, 2017; Takeshima et al., 2019).
Ward resilience is defined as the degree of variability in nutrition outcomes over time—the ability to withstand repeated crises of varying intensity. Robust wards are characterized by low variability, indicating above-average resilience at all times, independent of the severity of a crisis. The increasing and chronic trajectories are characterized by larger variation in resilience relative to the robust group, and tend oscillate around the threshold for nutritional sufficiency, although in opposite directions. Of note, the chronic group shows no real sign of recovery after an episode of crisis; the worsening of the nutrition situation continues, independent of its magnitude. In contrast, the increasing type shows continuous improvement in their resilience trajectory with decreasing severity of episodes of crises, albeit with lower baseline resilience relative to the robust trajectory, indicating successful adaptation in this specific sub-group of wards. The decreasing resilience trajectory is characterized by the largest variability in resilience over time, showing continuous worsening of the situation after repeated episodes of crisis.
Figure 2 depicts the nutrition situation in Kenyan wards for the period between January 2016 and April 2020. Ward resilience trajectories assigned by the LCMM (in pink on the left) and average resilience (in green on the right), are presented relative to the mean proportion of acutely malnourished children [“Global acute malnutrition (GAM)” prevalence, with blue dots]. Figure 3 depicts resilience to acute malnutrition at monthly intervals (in gray), with the mean resilience trajectory for each of the four resilience trajectories highlighted, along with the intensity of climate, conflict and market stressors across Kenya at the bottom.2
Figure 2. Resilience trajectories per ward assigned using a latent mixed class mixed model (left) vs. average resilience for the period between 2016 and 2020 (right), with smaller values indicating lower resilience. Blue dots display the mean proportion of malnourished children [“Global acute malnutrition (GAM)” prevalence] relative to the population size per ward for the same period.
Figure 3. Observed trajectories of ward resilience to acute malnutrition at monthly intervals. Mean trajectories derived from a four-class latent class mixed model are smoothed using a generalized additive model and are depicted including the 0.95 confidence interval (top), Kenya stressor intensity (bottom).
Local differences in children between 6 and 58 months are evident. Less than 2% of severely undernourished children are located in semi-arid and coastal regions such as Lamu. Yet, only some 100 km away in wards like Liboi—located in Garissa county and bordering Somalia—children are five times more likely to experience episodes of undernutrition. More generally, GAM prevalence lies above 15% in many arid regions to the North, in many cases for multiple years—referred to as “persistent GAM” (Young and Marshak, 2018). Our findings suggest that regions with lower GAM prevalence tend to display higher resilience; and that regions with higher GAM prevalence tend to be less resilient, albeit with significant variation across wards. Samburu and Wajir counties, arid regions characterized by high GAM prevalence, display particularly high variability in terms of resilience.
Figure 4 illustrates the resilience trajectories of Kenyan wards, per assigned latent class. While the majority, some 57% of wards, are assigned to the increasing type (Class B), 39% exhibit chronic resilience trajectories (Class A, where resilience is usually below average at baseline). Robust resilience over time is visible in 3% of Kenyan wards (Class C), and 1% shows a decreasing resilience trajectory (Class D).3 These patterns would not be evident from an analysis of acute malnutrition prevalence, which does not consider the full trajectory of severity and duration of acute malnutrition across a ward-year. They would also be unobservable at less granular levels of temporal and spatial aggregation. How then does ward resilience relate to individual household risk?
Figure 4. Resilience trajectories per assigned latent class for Kenyan wards, with 0.95 confidence intervals.
We assess household-level risk of acute malnutrition—the likelihood for at least one child between 6 and 59 months in a given household to be at risk of acute malnutrition (MUAC < 135 mm) (WHO, 2020) at a given point in time—depending on the assigned ward resilience trajectory. The unadjusted Cox regression curves diverge early and the log-rank test is significant (p < 0.001), indicating that there exist significant differences between latent classes.
Figure 5 presents the results of the adjusted cox regression analysis, controlling for a number of fixed household characteristics. The effect of ward resilience trajectories on household acute malnutrition risk, measured as the time to fall below the normative nutritional threshold (MUAC < 135 mm), remains significant (p < 0.05). Taking the chronic trajectory as a reference, the multivariate cox regression reveals that households in wards with a robust trajectory have a significantly lower risk of becoming acutely malnourished [hazard ratio (HR) 0.42 (95% CI 0.33—0.54)], while the decreasing trajectory is significantly more at risk of acute malnutrition [hazard ratio (HR) 3.05 (95% CI 1.14–8.17)]. Of note is the small, albeit significant effect of the increasing trajectory [hazard ratio (HR) 1.08 (95% CI 1.00–1.17)] relative to the chronic trajectory.
Figure 5. Multivariate Cox regression results for household risk. Probabilities represent the risk of a household falling under the nutritional threshold at time t, as a function of the ward resilience trajectory. We control for a set of fixed household characteristics. N indicates the number of observations at the household/month level and *, ** and *** indicate significance levels of covariates at 10, 5 and 1% levels respectively.
In addition to ward resilience, household risk depends on a number of individual household characteristics. Pastoralist livelihoods are associated with a significant increase and educated household heads with a significant decrease in household risk, whereas unsafe water sources and male household heads do not appear to have a significant influence on the time it takes for a household to fall below a nutrition threshold.
In children between 6 and 59 months, episodes of acute malnutrition are associated with an increase in the risk of mortality, ceteris paribus (WHO, 2020). Where prevalence rates regularly exceed the emergency threshold of >15%, acute malnutrition impedes the overall wellbeing of children (Hoddinott et al., 2008; Bhutta et al., 2017; Brown et al., 2020; Medialdea et al., 2021; Mullen, 2021; Raḿırez-Luzuriaga et al., 2021), households and communities (von Braun and Birner, 2017; Béné, 2020). Yet, risk factors are typically measured at a single point in time, the implicit assumption being that a single measure suffices over the short- to medium-term for a given spatial unit. This approach oversimplifies the extent to which local regions, wards in this case, vary with respect to their resilience to acute malnutrition.
In an effort to address this shortcoming, we develop a new measure of ward resilience. Our measure complements existing measures of household resilience, given that it builds on the same conceptual foundation but captures the resilience of local food systems as an outcome of longitudinal malnutrition prevalence. Using a latent-class approach, we identify four distinct “resilience signatures” for Kenyan wards: robust, chronic, increasing, and decreasing—associated with differential risks of becoming acutely malnourished. Notably, whereas some 39% of wards exhibit chronic characteristics, showing no real sign of recovery after an episode of crisis, 57% exhibit increasing resilience trajectories, with robust and decreasing resilience, respectively, visible in 3 and 1% of Kenyan wards.
As the results from our multivariate Cox regression demonstrate, these ward-level resilience trajectories effectively predict individual household malnutrition risk: relative to households in the chronic category, households in wards with robust (decreasing) resilience are significantly less (more) at risk. More generally, we find that wards in arid counties—Samburu, Wajir and Garissa in particular—are characterized by significantly lower resilience relative to wards in semi-arid counties, such as Narok or Makueni, albeit with large differences between wards within the same county. We also observe significantly lower resilience in wards that share a border with Somalia, as is the case in Jarajila and Liboi. The same hold for wards where religious extremism, violence against refugees, and gun proliferation is endemic, as in Mandera county (Sahgal et al., 2019; Schetter et al., 2022).
It follows that acute malnutrition trajectories can effectively be conceptualized in terms of dynamic latent classes, modeling the dependency between longitudinal markers, event time and covariates. Cross-sectional studies and definition-driven conceptualizations of resilience, such as the FAO Resilience Index Measurement and Analysis (RIMA) (FAO, 2016; Egamberdiev et al., 2023) and the “PEOPLES” tools (Cimellaro et al., 2016) effectively overlook or conflate these distinct trajectories. Chronic acute malnutrition, for example, may be conflated with decreasing resilience depending on when it is assessed. Only by charting trajectories of resilience can more specific risks and interventions be identified and implemented.
Assessments of malnutrition stand to benefit from the local-level approach to resilience measurement developed in this paper. First, analyzing trajectories of prevalence, in conjunction with cross-sectional snapshots, provides for a more nuanced understanding of risk, and consequently, the timing and scope of appropriate intervention. Second, the identification of at-risk sub-national units, such as wards, constitutes an improvement over approaches that are less granular—identifying country-wide or ADM1-level risk factors. And third, the identification of resilience trajectories based on latent class modeling is “data-light”, insofar as it requires only a minimal information set that may be gleaned from existing initiatives.
All data used in this study are available via the Harvard Dataverse, a FAIR compliant data repository using the following link: https://doi.org/10.7910/DVN/GGW9F7.
The studies involving human participants were reviewed and approved by the Ethics Review Committee of the Graduate Institute of International and Development Studies (IHEID) and Amref Health Africa. Written informed consent for participation was not required for this study in accordance with the national legislation and the institutional requirements.
RB served as the project's PI. NS and MR organized the dataset. NS performed the joint latent class and time to event analysis. RB, NS, and KD wrote the original and revised the final manuscript. All authors contributed to the design of the study and conceptualization of the resilience measure.
This paper is a product of the Modeling Early Risk Indicators to Anticipate Malnutrition (MERIAM) project, funded by the UK Foreign, Commonwealth & Development Office (Strengthening Data for Nutrition Program, Contract # 7442). The work was supported by subcontracts from Action Against Hunger to the Geneva Graduate Institute.
The authors declare that the research was conducted in the absence of any commercial or financial relationships that could be construed as a potential conflict of interest.
All claims expressed in this article are solely those of the authors and do not necessarily represent those of their affiliated organizations, or those of the publisher, the editors and the reviewers. Any product that may be evaluated in this article, or claim that may be made by its manufacturer, is not guaranteed or endorsed by the publisher.
The views expressed in this paper are those of the authors.
The Supplementary Material for this article can be found online at: https://www.frontiersin.org/articles/10.3389/fsufs.2023.1091346/full#supplementary-material
1. ^We define households as malnourished if the MUAC of at least one child between six and 59 months and per self-identified household is smaller than 135 mm, corresponding to the cut-off value for risk of acute malnutrition in the WHO child growth standard (WHO, 2009, 2021). To assess the sensitivity of our measures, Supplementary Figure S1 depicts the relationship between malnutrition prevalence and our resilience index at the ward-year level, using different normative MUAC thresholds to classify children as acutely malnourished.
2. ^Note that categories reflect the five phases on the corresponding “International Phase Classification” (IPC) scale (IPC, 2022), with GAM >15% corresponding to Phase 4 (“Critical”).
3. ^Figure 4 suggests that some wards categorized into the increasing trajectory (Class B) would also fit our definition of the robust trajectory (Class C). This underpins the heuristic nature of our classification, i.e., classes were first assigned based on the highest posterior probabilities and our interpretation followed, and/or classification error.
Alexander, B., Cox, L. J., and Mochizuki, J. (2013). Integrated risk identification, analysis, and assessment: a dynamic household economy analysis methodology and example. Int. J. Disast. Risk Sci. 4, 77–88. doi: 10.1007/s13753-013-0009-7
Alinovi, L., Mane, E., and Romano, D. (2022). Towards the Measurement of Household Resilience to Food Insecurity: Applying? a Model to Palestinian Household Data. Deriving Food Security Information From National Household Budget Surveys. Rome: Food and Agricultural Organization of the United Nations, 137.
Anderson, W., Taylor, C., McDermid, S., Ilboudo-Nébié, E., Seager, R., Schlenker, W., et al. (2021). Violent conflict exacerbated drought-related food insecurity between 2009 and 2019 in sub-Saharan Africa. Nat. Food 2, 603–615. doi: 10.1038/s43016-021-00327-4
Andrinopoulou, E.-R., Harhay, M. O., Ratcliffe, S. J., and Rizopoulos, D. (2021). Reflection on modern methods: dynamic prediction using joint models of longitudinal and time-to-event data. Int. J. Epidemiol. 50, 1731–1743. doi: 10.1093/ije/dyab047
Bakhtsiyarava, M., and Grace, K. (2021). Agricultural production diversity and child nutrition in Ethiopia. Food Sec. 13, 1407–1422. doi: 10.1007/s12571-021-01173-9
Béné, C. (2020). Resilience of local food systems and links to food security-a review of some important concepts in the context of covid-19 and other shocks. Food Sec. 12, 805–822. doi: 10.1007/s12571-020-01076-1
Béné, C., Headey, D., Haddad, L., and von Grebmer, K. (2015). Is resilience a useful concept in the context of food security and nutrition programmes? Some conceptual and practical considerations. Food Sec. 8, 123–138. doi: 10.1007/s12571-015-0526-x
Berg, C., and Emran, M. S. (2020). Microfinance and vulnerability to seasonal famine in a rural economy: evidence from monga in Bangladesh. BE J. Econ. Anal. Plicy 20, 20202001. doi: 10.1515/bejeap-2018-0359
Bhavnani, R., Donnay, K., and Reul, M. (2020). Evidence-Driven Computational Modeling. The SAGE Handbook of Research Methods in Political Science and International Relations. London: SAGE Publications, 60.
Bhavnani, R., Schlager, N., Donnay, K., Reul, M., Schenker, L., Stauffer, M., et al. (2023). Household behavior and vulnerability to acute malnutrition in Kenya. Human. Soc. Sci. Commun. 10, 63. doi: 10.1057/s41599-023-01547-8
Bhutta, Z. A., Berkley, J. A., Bandsma, R. H., Kerac, M., Trehan, I., and Briend, A. (2017). Severe childhood malnutrition. Nat. Rev. Dis. Prim. 3, 1–18. doi: 10.1038/nrdp.2017.67
Borman, G. D., de Boef, W. S., Dirks, F., Gonzalez, Y. S., Subedi, A., Thijssen, M. H., et al. (2022). Putting food systems thinking into practice: integrating agricultural sectors into a multi-level analytical framework. Global Food Sec. 32, 100591. doi: 10.1016/j.gfs.2021.100591
Boukary, A. G., Diaw, A., and Wünscher, T. (2016). Factors affecting rural households' resilience to food insecurity in Niger. Sustainability 8, 181. doi: 10.3390/su8030181
Brown, M. E., Backer, D., Billing, T., White, P., Grace, K., Doocy, S., et al. (2020). Empirical studies of factors associated with child malnutrition: highlighting the evidence about climate and conflict shocks. Food Sec. 12, 1241–1252. doi: 10.1007/s12571-020-01041-y
Brown, M. E., Grace, K., Billing, T., and Backer, D. (2021). Considering climate and conflict conditions together to improve interventions that prevent child acute malnutrition. Lancet Planet. Health 5, e654–e658. doi: 10.1016/S2542-5196(21)00197-2
Browne, M., Ortmann, G., and Hendriks, S. L. (2014). Developing a resilience indicator for food security monitoring and evaluation: index construction and household classification for six African countries. Agrekon 53, 31–56. doi: 10.1080/03031853.2014.929011
Cafiero, C., Fonseca, M. B., Chelle, M., Egamberdieva, D., Kanter, R., Kimani, S., et al. (2022). Data Collection and Analysis Tools for Food Security and Nutrition: Towards Enhancing Effective, Inclusive, Evidence-Informed, Decision Making. A Report by the High Level Panel of Experts on Food Security and Nutrition of the Committee on World Food security. Rome: High-Level Panel of Experts on Food Security and Nutrition (HLPE-FSN).
Cimellaro, G. P., Renschler, C., Reinhorn, A. M., and Arendt, L. (2016). PEOPLES: a framework for evaluating resilience. J. Struct. Eng. 142, 04016063. doi: 10.1061/(ASCE)ST.1943-541X.0001514
Constas, M., Frankenberger, T., and Hoddinott, J. (2013). Resilience Measurement Principles: Toward an Agenda for Measurement Design. Food Security Information Network, Resilience Measurement Technical Working Group, Technical Series 1. Rome: Food Security Information Network (FSIN).
Corbett, J. (1988). Famine and household coping strategies. World Dev. 16, 1099–1112. doi: 10.1016/0305-750X(88)90112-X
Deconinck, K., Giner, C., Jackson, L. A., and Toyama, L. (2022). Making better policies for food systems will require reducing evidence gaps. Global Food Sec. 33, 100621. doi: 10.1016/j.gfs.2022.100621
d'Errico, M., Romano, D., and Pietrelli, R. (2018). Household resilience to food insecurity: evidence from Tanzania and Uganda. Food Sec. 10, 1033–1054. doi: 10.1007/s12571-018-0820-5
Desai, B., Maskrey, A., Peduzzi, P., De Bono, A., and Herold, C. (2015). Making Development Sustainable: The Future of Disaster Risk Management, Global Assessment Report on Disaster Risk Reduction. Geneva: United Nations Office for Disaster Risk Reduction (UNISDR).
DHS (2022). Kenya: Standard dhs, 2022. Available online at: https://dhsprogram.com/methodology/survey/survey-display-566.cfm (accessed June 11, 2023).
Didan, K., Munoz, A. B., Solano, R., and Huete, A. (2015). Modis Vegetation Index User's Guide (mod13 Series). Tucson, AZ: University of Arizona, Vegetation Index and Phenology Lab.
Doss, C. R., and Meinzen-Dick, R. (2015). Collective action within the household: insights from natural resource management. World Dev. 74, 171–183. doi: 10.1016/j.worlddev.2015.05.001
Egamberdiev, B., Bobojonov, I., Kuhn, L., and Glauben, T. (2023). Household resilience capacity and food security: evidence from Kyrgyzstan. Food Sec. 1–22. doi: 10.1007/s12571-023-01369-1
Fanzo, J., Haddad, L., Schneider, K. R., Béné, C., Covic, N. M., Guarin, A., et al. (2021). Rigorous monitoring is necessary to guide food system transformation in the countdown to the 2030 global goals. Food Policy 104, 102163. doi: 10.1016/j.foodpol.2021.102163
FAO (2016). RIMA-II: Resilience Index Measurement and Analysis - II: Analysing Resilience for Better Targeting and Action. Rome.
FAO IFAD, UNICEF, WFP, and WHO. (2022). The State of Food Security and Nutrition in the World 2022. Rome.
FewsNet (2018). Integrating Acute Malnutrition and Mortality Into Scenario Development. Washington, DC: Technical report, Famine Early Warning Systems Network.
Fraccascia, L., Giannoccaro, I., and Albino, V. (2018). Resilience of complex systems: state of the art and directions for future research. Complexity. 2018, 44. doi: 10.1155/2018/3421529
Francis, B., and Liu, J. (2015). Modelling escalation in crime seriousness: a latent variable approach. Metron 73, 277–297. doi: 10.1007/s40300-015-0073-4
Fratkin, E. (2019). Surviving Drought And Development: Ariaal Pastoralists of Northern Kenya. Thames: Routledge.
Grace, K., Verdin, A., Brown, M., Bakhtsiyarava, M., Backer, D., and Billing, T. (2022). Conflict and climate factors and the risk of child acute malnutrition among children aged 24-59 months: a comparative analysis of Kenya, Nigeria, and Uganda. Spat. Demogr. 10, 329–358. doi: 10.1007/s40980-021-00102-w
Hamm, M. W., and Bellows, A. C. (2003). Community food security: background and future directions. J. Nutr. Educ. Behav. 35, 37–43. doi: 10.1016/S1499-4046(06)60325-4
Hegre, H., Croicu, M., Eck, K., and Högbladh, S. (2020). Introducing the ucdp candidate events dataset. Res. Polit. 7, 2053168020935257. doi: 10.1177/2053168020935257
Hoddinott, J., Maluccio, J. A., Behrman, J. R., Flores, R., and Martorell, R. (2008). Effect of a nutrition intervention during early childhood on economic productivity in guatemalan adults. Lancet 371, 411–416. doi: 10.1016/S0140-6736(08)60205-6
Högbladh, S. (2020). UCDP GED Codebook Version 20.1. Uppsala: Department of Peace and Conflict Research, Uppsala University.
Kassambara, A., Kosinski, M., Biecek, P., and Fabian, S. (2017). Package ‘survminer'. Available online at: https://cran.r-project.org/package=survminer (accessed June 11, 2023).
Klassen, S., and Murphy, S. (2020). Equity as both a means and an end: lessons for resilient food systems from covid-19. World Dev. 136, 105104. doi: 10.1016/j.worlddev.2020.105104
Lamothe, K. A., Somers, K. M., and Jackson, D. A. (2019). Linking the ball-and-cup analogy and ordination trajectories to describe ecosystem stability, resistance, and resilience. Ecosphere 10, e02629. doi: 10.1002/ecs2.2629
Lampousi, A.-M., Möoller, J., Liang, Y., Berglind, D., and Forsell, Y. (2021). Latent class growth modelling for the evaluation of intervention outcomes: example from a physical activity intervention. J. Behav. Med. 44, 622–629. doi: 10.1007/s10865-021-00216-y
Lennon, H., Kelly, S., Sperrin, M., Buchan, I., Cross, A. J., Leitzmann, M., et al. (2018). Framework to construct and interpret latent class trajectory modelling. BMJ Open 8, e020683. doi: 10.1136/bmjopen-2017-020683
Maxwell, D., Stites, E., Robillard, S., and Wagner, M. (2017). Conflict and Resilience: A Synthesis of Feinstein International Center Work on Building Resilience and Protecting Livelihoods in Conflict-Related Crises. Boston, MA: Feinstein International Center, Tufts University.
Mazzocchi, G., Dezio, C., and Marino, D. (2022). “The impacts of resilience practices on local food systems: evidences from italian case studies,” in [ECO] Systems of Resilience Practices: Contributions for Sustainability and Climate Change Adaptation (Amsterdam: Elsevier), 121–136.
McCarthy, N., and Kilic, T. (2017). Stronger Together: Intra-Household Cooperation and Household Welfare in Malawi. Policy Research Working Papers. Washington, DC: The World Bank.
Medialdea, L., Bogin, B., Thiam, M., Vargas, A., Marrodán, M. D., and Dossou, N. I. (2021). Severe acute malnutrition morphological patterns in children under five. Sci. Rep. 11, 1–13. doi: 10.1038/s41598-021-82727-x
Mullen, A. (2021). Chronic challenge of undernutrition in the under-fives. Nat. Food 2, 222–222. doi: 10.1038/s43016-021-00274-0
NDMA (2023). County Early Warning Bulletins. Available online at: https://www.ndma.go.ke/index.php/resource-center/category/64-county-early-warning-bulletins (accessed June 11, 2023).
Niles, M. T., Rudnick, J., Lubell, M., and Cramer, L. (2021). Household and community social capital links to smallholder food security. Front. Sustain. Food Syst. 5, 44. doi: 10.3389/fsufs.2021.583353
Park, J., Cho, J., and Rose, A. (2011). Modeling a major source of economic resilience to disasters: recapturing lost production. Nat Hazards 58, 163–182. doi: 10.1007/s11069-010-9656-9
Proust-Lima, C., Dartigues, J.-F., and Jacqmin-Gadda, H. (2016). Joint modeling of repeated multivariate cognitive measures and competing risks of dementia and death: a latent process and latent class approach. Stat. Med. 35, 382–398. doi: 10.1002/sim.6731
Proust-Lima, C., and Liquet, B. (2011). “Lcmm: an r package for estimation of latent class mixed models and joint latent class models,” in The R User Conference, useR! 2011 August 16-18 2011 University of Warwick (Coventry: Citeseer), 66.
Proust-Lima, C., and Liquet, B. (2016). Package ‘lcmm'. Available online at: https://cran.r-project.org/package=lcmm (accessed June 11, 2023).
Proust-Lima, C., Saulnier, T., Philipps, V., Traon, A. P.-L., Péran, P., Rascol, O., et al. (2022). Describing complex disease progression using joint latent class models for multivariate longitudinal markers and clinical endpoints. arXiv. doi: 10.48550/arXiv.2202.05124
Proust-Lima, C., Séne, M., Taylor, J. M., and Jacqmin-Gadda, H. (2014). Joint latent class models for longitudinal and time-to-event data: a review. Stat. Methods Med. Res. 23, 74–90. doi: 10.1177/0962280212445839
Raleigh, C., Linke, A., Hegre, H., and Karlsen, J. (2010). Introducing acled: an armed conflict location and event dataset: special data feature. J. Peace Res. 47, 651–660. doi: 10.1177/0022343310378914
Ramírez-Luzuriaga, M. J., Hoddinott, J., Martorell, R., Patel, S. A., Ramírez-Zea, M., Waford, R., et al. (2021). Linear growth trajectories in early childhood and adult cognitive and socioemotional functioning in a guatemalan cohort. J. Nutr. 151, 206–213. doi: 10.1093/jn/nxaa337
Rao, N., Mishra, A., Prakash, A., Singh, C., Qaisrani, A., Poonacha, P., et al. (2019). A qualitative comparative analysis of women's agency and adaptive capacity in climate change hotspots in Asia and Africa. Nat. Clim. Chang. 9, 964–971. doi: 10.1038/s41558-019-0638-y
Ratz, T., Pischke, C. R., Voelcker-Rehage, C., and Lippke, S. (2022). Distinct physical activity and sedentary behavior trajectories in older adults during participation in a physical activity intervention: a latent class growth analysis. Eur. Rev. Aging Phys. Act. 19, 1–11. doi: 10.1186/s11556-021-00281-x
Reisch, L. A. (2021). Shaping healthy and sustainable food systems with behavioural food policy. Eur. Rev. Agric. Econ. 48, 665–693. doi: 10.1093/erae/jbab024
Sahgal, G., Kimaiyo, T., Mohamed, A. H., Rotich, S., Karienye, D., and Warfa, A. O. (2019). Clan Conflict and Violent Extremism in the North-Eastern Counties of Kenya. Nairobi: Royal United Services Institute for Defence and Security Studies.
Schetter, C., Mkutu, K., and Müller-Koné, M. (2022). Frontier ngos: conservancies, control, and violence in northern Kenya. World Dev. 151, 105735. doi: 10.1016/j.worlddev.2021.105735
Sène, M., Bellera, C. A., and Proust-Lima, C. (2014). Shared random-effect models for the joint analysis of longitudinal and time-to-event data: application to the prediction of prostate cancer recurrence. J. Soc. Fran. Stat. 155, 134–155. Available online at: http://www.numdam.org/item/JSFS_2014__155_1_134_0/ (accessed July 6, 2023).
Simon, L., Rab, S. L., Goldstein, P., Magal, N., and Admon, R. (2022). Multi-trajectory analysis uncovers latent associations between psychological and physiological acute stress response patterns. Psychoneuroendocrinology 145, 105925. doi: 10.1016/j.psyneuen.2022.105925
SMART (2021). Standardized Monitoring and Assessment of Relief and Transitions: Methodology. Available online at: https://smartmethodology.org/about-smart/ (accessed February 10, 2023).
Stone, A. G., Lloyd, C. D., Spivak, B. L., Papalia, N. L., and Serin, R. C. (2023). Trajectories of change in acute dynamic risk ratings and associated risk for recidivism in paroled New Zealanders: a joint latent class modelling approach. J. Quant. Criminol. 1–41. doi: 10.1007/s10940-022-09566-5
Sundberg, R., and Melander, E. (2013). Introducing the UCDP georeferenced event dataset. J. Peace Res. 50, 523–532. doi: 10.1177/0022343313484347
Takeshima, H., Akramov, K., Park, A., Ilyasov, J., and Ergasheva, T. (2019). Agriculture-nutrition linkages, cooking-time, intra-household equality among women and children: evidence from Tajikistan. Intl. Food Policy Res. Inst. 34, 940–977. doi: 10.2499/p15738coll2.133485
Tendall, D. M., Joerin, J., Kopainsky, B., Edwards, P., Shreck, A., Le, Q. B., et al. (2015). Food system resilience: defining the concept. Global Food Sec. 6, 17–23. doi: 10.1016/j.gfs.2015.08.001
Therneau, T. M., and Lumley, T. (2015). Package ‘survival'. Available online at: https://cran.r-project.org/package=survival (accessed June 11, 2023).
Van De Schoot, R., Sijbrandij, M., Winter, S. D., Depaoli, S., and Vermunt, J. K. (2017). The grolts-checklist: guidelines for reporting on latent trajectory studies. Struct. Eq. Model. 24, 451–467. doi: 10.1080/10705511.2016.1247646
von Braun, J., Afsana, K., Fresco, L. O., Hassan, M., and Torero, M. (2021). Food system concepts and definitions for science and political action. Nat. Food 2, 748–750. doi: 10.1038/s43016-021-00361-2
von Braun, J., and Birner, R. (2017). Designing global governance for agricultural development and food and nutrition security. Rev. Dev. Econ. 21, 265–284. doi: 10.1111/rode.12261
Wang, M., and Do, M. H. (2023). Reported shocks, households' resilience and local food commercialization in thailand. J. Econ. Dev. 25, 153–170. doi: 10.1108/JED-10-2022-0204
Watts, M. J., and Bohle, H. G. (1993). The space of vulnerability: the causal structure of hunger and famine. Prog. Hum. Geogr. 17, 43–67. doi: 10.1177/030913259301700103
Westerveld, J. J., van den Homberg, M. J., Nobre, G. G., van den Berg, D. L., Teklesadik, A. D., and Stuit, S. M. (2021). Forecasting transitions in the state of food security with machine learning using transferable features. Sci. Total Environ. 786, 147366. doi: 10.1016/j.scitotenv.2021.147366
WFP (2020). Impact of COVID-19 Outbreak on Livelihoods, Food Security and Nutrition in East Africa. Available online at: https://docs.wfp.org/api/documents/WFP-0000114546/download/?_ga=2.11384764.989337293.1591211403-1414798030.1591211403 (accessed June 11, 2023).
WHO (2009). Who Child Growth Standards and the Identification of Severe Acute Malnutrition in Infants and Children: Joint Statement by the World Health Organization and the United Nations Children's Fund. Technical Report. Geneva: World Health Organization.
WHO (2020). Global Action Plan on Child Wasting: A Framework for Action to Accelerate Progress in Preventing and Managing Child Wasting and the Achievement of the Sustainable Development Goals. Technical Report. Geneva: World Health Organization.
WHO (2021). Levels and Trends in Child Malnutrition. Technical Report. Geneva: World Health Organization.
Young, H. (2020). Nutrition in Africa's Drylands: A Conceptual Framework for Addressing Acute Malnutrition. Medford, MA: Feinstein International Center, Tufts University.
Young, H., and Marshak, A. (2018). A Discussion Paper on the Scope of the Problem, Its Drivers, and Strategies for Moving Forward for Policy, Practice, and Research. Medford, MA: Feinstein International Center, Tufts University.
Keywords: acute malnutrition, climate, conflict, Kenya, latent class modeling, resilience
Citation: Bhavnani R, Schlager N, Reul M and Donnay K (2023) Trajectories of resilience to acute malnutrition in the Kenyan drylands. Front. Sustain. Food Syst. 7:1091346. doi: 10.3389/fsufs.2023.1091346
Received: 06 November 2022; Accepted: 28 June 2023;
Published: 24 July 2023.
Edited by:
Davide Marino, University of Molise, ItalyReviewed by:
Giampiero Mazzocchi, Council for Agricultural and Economics Research (CREA), ItalyCopyright © 2023 Bhavnani, Schlager, Reul and Donnay. This is an open-access article distributed under the terms of the Creative Commons Attribution License (CC BY). The use, distribution or reproduction in other forums is permitted, provided the original author(s) and the copyright owner(s) are credited and that the original publication in this journal is cited, in accordance with accepted academic practice. No use, distribution or reproduction is permitted which does not comply with these terms.
*Correspondence: Karsten Donnay, ZG9ubmF5QGlwei51emguY2g=
Disclaimer: All claims expressed in this article are solely those of the authors and do not necessarily represent those of their affiliated organizations, or those of the publisher, the editors and the reviewers. Any product that may be evaluated in this article or claim that may be made by its manufacturer is not guaranteed or endorsed by the publisher.
Research integrity at Frontiers
Learn more about the work of our research integrity team to safeguard the quality of each article we publish.