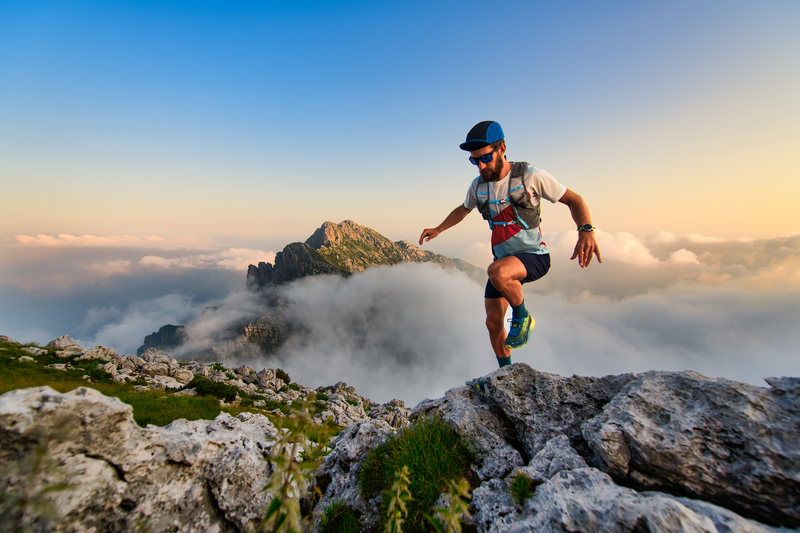
95% of researchers rate our articles as excellent or good
Learn more about the work of our research integrity team to safeguard the quality of each article we publish.
Find out more
ORIGINAL RESEARCH article
Front. Sustain. Food Syst. , 14 September 2023
Sec. Land, Livelihoods and Food Security
Volume 7 - 2023 | https://doi.org/10.3389/fsufs.2023.1081127
This article is part of the Research Topic Sustainable Intensification of Smallholder Farming Systems in Sub-Saharan Africa and South Asia View all 16 articles
Efficient resource utilization in small-scale farms is crucial to achieving farm sustainability through endogenous mechanisms. However, the precise mechanisms to integrate farm resources to achieve farm sustainability are not very clear yet. By capturing the interaction among farm resources as a network phenomenon, we aimed to identify the discrete resource interactions (RIs) associated with higher farm sustainability in different farm types of Indian Sundarbans. First, we assessed the sustainability of 140 integrated farms using a synthesized assessment framework. Then, we considered four network motifs, namely linkage (a one-way link between two resources), reciprocal linkage (a two-way link between two resources), triad (three resources having closed interconnectedness), and the presence of a farm resource at the core of a network. Using RI network data of 140 farms and employing a graph theoretic approach we identified discrete network motifs (i.e., resource interaction) associated with highly sustainable farms in different farm types. We found a predominance of rice, vegetables and pond-based integration and identified 32 linkages, 11 reciprocal linkages, 21 triads, and three resources at the network core that occurred and co-occurred on highly sustainable farms, and thus critical to achieving farm sustainability. Further, multivariate analyses established that the properties of RI networks could explain farm sustainability significantly. We anticipate that sustainability in small-scale farms can be achieved by strategically designing new RIs on the farm. However, there may be limitations to such achievement depending on the nature of RI and the type of farm.
Nearly 2.5 billion small-scale farms operate on 60 per cent of the world's arable lands, and their sustainability is critically important to meet the growing demand for food in the coming decades (Cui et al., 2018; Guiomar et al., 2021). These farms, across the globe, are typically characterized by resource-poor conditions, vulnerability to biotic and abiotic stresses, climatic variability, and structural constraints (Altieri et al., 2012) and needs strategic intensifications to improve their food-income-energy-natural resource nexus (Gathala et al., 2020, 2021). Achieving sustainability in this large number of small farms will not only play an essential role in ensuring global food and nutritional security (Cui et al., 2018; Brunori et al., 2020; Giller et al., 2021) but also present a means to secure inclusive economic growth, reduce the vulnerability of rural communities, and rapidly reduce poverty (Apata et al., 2020; Gomez y Paloma et al., 2020). Since small-scale farms often cannot access the same external resources as well-off farms, the existing resources on small farms must be used strategically to move toward sustainability, irrespective of external interventions. Thus, it is crucial to examine how decisions to use a set of available farm resources affect its sustainability.
The speed of exit from smallholder systems remains slow in many developing countries, and increasing population pressure on these systems may lead to resource degradation and reduced efficiency in the long run (Hazell and Rahman, 2014). Both the ideas of “sustainable intensification” (SI) (Rockström et al., 2017; Cassman and Grassini, 2020) and “ecological intensification” (EI) (Tittonell, 2014a; MacLaren et al., 2022) evoke that productivity and sustainability can be synergistic, rather than merely needing to strike a balance between the two (Pretty et al., 2018). For the past few decades, examples of sustainable forms of intensification have shown promise in both developed and developing countries (Pretty and Bharucha, 2014; Jat et al., 2020) and emerged as alternative ways of farming (Wezel et al., 2014). However, the mechanisms of SI have been summarized only recently (Pretty et al., 2018; Cassman and Grassini, 2020; Kuyah et al., 2021), and understanding the pattern to combine existing farm resources (referred to as resource interaction in this article) as a means of SI remains an outstanding issue.
Sustainable forms of intensification may be achieved by either increasing efficiency, substituting new technologies and practices, or redesigning the way a system functions (Hill, 1985). It is argued that the conscious designing of farming systems can be a strategy for smallholder farmers to achieve sustainable livelihoods (Tittonell, 2014b; Goswami et al., 2016; Andrieu et al., 2019). We posit that farm families can negotiate multiple factors, internal and external to the farming systems, and consciously design and readjust the farm resources to achieve short- and long-term sustainability. Attributing such resource interactions (RI) with farm sustainability can enhance our ability to design farming systems for strategic gains in smallholder sustainability. By RI we refer to human-managed material and energy flow and space sharing between different farm components such as land, water bodies, livestock, and vegetation. Farm components are physical entities that host the resources and their interactions.
The existing systems analytic approaches in agriculture have advanced our understanding of how farming systems function (Roling, 1991; Collinson, 2000; Hall et al., 2003; Temel et al., 2003; Spielman et al., 2011; Holzworth et al., 2015; Schut et al., 2015; Basso et al., 2016; Jones et al., 2017), but linking discrete SI with multiple outcomes in farming systems is extremely limited (Musumba et al., 2017; Li et al., 2020). By capturing the interaction among farm resources as a network phenomenon, we aimed to identify the discrete RIs associated with higher farm sustainability in different types of 140 small-scale farms in the Indian Sundarbans. Also, we linked farm sustainability with the network properties of farm RI in different farm types. The analyses may open up the study of agricultural systems from a network perspective and encourage researchers to find discrete RI “motifs” for an informed design of farming systems, something analogical to the genetic code of sustainable agricultural systems.
Enumerators collected the field data from farms in selected areas of Indian Sundarbans, which are constituted of 19 community development blocks (CDB) (Supplementary Figure S1). We randomly selected one CDB each from 13 CDBs of South 24 Parganas district and six CDBs of North 24 Parganas District in the West Bengal State of India. From each CDB, we selected one Gram Panchayat (GP) (village self-governing body) randomly. Fifty-two farms from the selected GP of South 24 Parganas and 88 farms from the selected GP of North 24 Parganas representing nearly 15% of the farm families in those GPs—were randomly selected from a list of farms prepared in consultation with the local stakeholders of agricultural development. We selected farms below the size of two ha to maintain a normative classification of the agricultural census. But, because of the extremely small size of farms, all farms (except for two) were less than one hectare in size.
There are six agro-climatic zones—based on climate, soil, and physiography—in the state of West Bengal in India, and the Sundarbans region comes under the coastal saline zone (Gajbhiye and Mandal, 2000). The Indian part of Sundarbans consists of 4,200 km2 of reserved forest and 5,400 km2 of non-forest areas, and it is intersected by large numbers of rivers, rivulets, and tidal waterways. Fifty-four islands are inhabited by over 4.4 million people (World Bank, 2014). River embankments guard the boundaries of islands in the upstream areas, but tidal saline water often intrudes into the embankments and floods farmlands. Soil salinity increases in dry months to render the soil uncultivable. The region is vulnerable to cyclonic storms and prolonged inundation. Rice is the main crop grown over different land terrains and seasons. Spring paddy, sesame, and green gram in the early wet season; jute and aman rice in the wet season; maize, different oilseeds and pulses; and vegetables in the winter season are the important crops. Apart from agriculture, a large portion of the population depends on non-timber forest products and migrates to urban centers of India and abroad. Population density has risen rapidly over the past decade and is well above the national and state average. Sustainable use of natural resources is critical to managing livelihoods in the region. Nearly half of the region's population live below the poverty line, and limited employment opportunity creates conflict between livelihoods and environmental sustainability. Nearly all farmers are living marginally, having less than one hectare of land, and sustaining households using small parcels of land within the context of socioeconomic; environmental vulnerability is a great challenge to support smallholders in Sundarbans.
We collected field data through face-to-face interviews with a pre-tested standardized interview schedule (RKMVERI/NDP/EC/RG/Fulb/2018-19) and farm-level measurements. The interview schedule consisted of respondents' background information, livelihood assets, household income and expenditure, details on farming practices, and resource interaction on the farm. The data collection instrument had all the sustainability assessment indicators. The draft instrument was piloted on non-sampled integrated farms in the study areas and modified based on piloting experience. The enumerators stayed in the villages before collecting data, and the actual field data were collected on 140 farms from March-June 2018. Observations and measurements were made on the farms during the interview itself. Summarily, we collected field data to (a) delineate farm types and characterize them, (b) assess the farm's sustainability, and (c) assess farm resource interaction.
Since the smallholder system is heterogeneous in terms of evolution, resource endowments, and vulnerabilities, the smallholder farmers are expected to employ different resource intensification strategies and might pursue different trajectories of sustainability outcomes (Tittonell, 2014b). Different types of farms employ different mechanisms of RI to achieve sustainable livelihoods. Hence, typology delineation for small farms is a pragmatic step to simplify the diverse farming systems before studying the interrelationship of RI and farm sustainability. Based on representative literature (Netting, 1993), we selected 18 potential indicators (Supplementary Table S1) to define the smallholder system in the study region. To reduce the multicollinearity of the data, we used principal component analysis (PCA) to extract six principal components (PCs). These PC scores were then used in hierarchical cluster analysis and K-means cluster analysis to arrive at five distinct farm types (Supplementary Tables S2–S4). Once we classified the farms based on these six PCs, we characterized them in terms of a set of background variables (Supplementary Table S5). We tested whether the five farm types differed in terms of this set of background variables. Significant variables in the one-way ANOVA and Chi-square tests suggested the efficacy of our classification. For quantitative variables, we used post-hoc tests after the one-way ANOVA to identify the distinctly high or low farm types for the individual variables (Supplementary Table S6). Mostly, these distinct high and low values of indicators were used to characterize different farm types. When the mean value of a variable for a farm type was highest and significantly higher than the other farm types in the post-hoc test, we called it “high”. Similarly, the statistically significant lowest value of a variable was called “low”. Anything in between was called “moderate”. A qualitative description of the delineated farm types is given in Supplementary Table S7.
To assess smallholder farms' sustainability, we scouted a suite of indicators following relevant assessment frameworks of local and global importance (Scoones, 1998; Rao and Rogers, 2006; FAO, 2014; Goswami et al., 2017). Our framework is grounded on the sustainable livelihoods framework and guided the selection of indicators (Supplementary Figure S2), the coverage of social, economic, and ecological dimensions of sustainability, access and availability of related data sources, cost of measurement, time involved, and understanding of the indicator by the respondents (Dasgupta et al., 2017). Thus, we identified 39 indicators covering social (16), economic (12), and ecological (11) dimensions of sustainability (Supplementary Table S8). We incorporated these 39 indicators in a data collection instrument and pre-tested them on non-sampled integrated farms before the final data collection on 140 integrated farms. These indicators were standardized using max-min standardization, winsorized (to manage outliers), weighted, and aggregated to develop a composite sustainability index (SI) (OECD, 2008; Goswami et al., 2017). We employed principal component analysis on the dataset and used factor loadings of the principal components for weighing individual indicators. Finally, we aggregated the weighed indicators linearly to develop a composite sustainability index. The index value ranged from 0 to 100, “0” being the lowest and “100” being the highest possible value (Supplementary Section S3; see Supplementary Table S9 for comparison of sustainability indicators across farm types).
Farm families of the study locations identified 10 types of distinct resources/farm components, namely Rice field (R), Vegetable plots (V), Cattle (C), Poultry (PL), Pond (PN), Homestead (H), Tree (T), Kitchen (K), Common property resources (CP), and Fallow land (F) before the actual data collection. We considered the presence of a resource interaction (RI) on the farm when a perceived flow of energy or matter or sharing of space between any two of these 10 resources existed. We ascertained such existence in consultation with the respondents coupled with farm visits and measurements. We recorded the RI in a 10x10 binary matrix for all 140 farms. Thus, there were 140 RI networks and 140 binary matrices. In graph-theoretic parlance, a node in the RI network represents a resource/farm component, and a directed tie represents the interaction between two nodes. Based on the matrices, we generated two types of network information for individual farms: First, we identified the linkage, reciprocal linkage, triads, and presence of a resource/farm component at the core of the RI network (Borgatti and Everett, 2000) to understand the structural composition of the farm resource interaction. Second, we computed different network properties of individual farms, namely density, component ratio, connectedness, fragmentation, compactness, dependency ratio, Weiner index, closure, transitivity, clustering coefficient, and arc reciprocity, to understand the nature of resource interaction in the farms (Supplementary Table S10). We used UCINET for Windows software (Borgatti et al., 2002) for matrix manipulation and analyses of the farm RI's structural composition, i.e., the ties, reciprocal ties, triads, and presence at the core. We used the same software for the computation of the network properties of RI. The structural compositions were then related to farm sustainability and farm types in a graph-theoretic layout using NetDraw software (Borgatti, 2002). NetDraw was also used for the visualization of the co-occurrence of RI.
We examined the relationship between RI and farm sustainability following two approaches. First, we developed a two-mode network (network involving both the resources and individual farms) of 140 RI networks and visualized it in the graph-theoretic layout. This helped to identify the RI motifs associated with highly sustainable farms. Then, to study the co-occurrence of RI motifs on individual farms we converted the two-way networks into one-way networks (considering RI motifs as the nodes) and visualized them in a metric multi-dimensional layout. The proximity of RI motifs denoted their higher tie strength and thus a higher probability of co-occurrence. The RI motifs that were associated with highly sustainable farms and co-occurred on the same integrated farms were identified as the critically important motifs for achieving farm sustainability.
Second, we used two extracted principal components from 11 network properties of 140 farms to explain their sustainability score (Supplementary Tables S11, S12). Using SPSS Modeler 18.1 (IBM_Corp, 2016), we employed 12 models together, and the output is based on the three best-performing models in terms of their correlation value and relative error (Supplementary Table S13). The relationship was also examined separately for all five farm types to examine whether it held across farm types.
We identified five farm types using a sequence of principal component analysis (PCA) and hierarchical cluster analysis (CA) to reduce the heterogeneity of smallholder systems and created socio-ecological boundaries within which resource management could be understood better (Kansiime et al., 2018) (Supplementary Information details the typology delineation and characterization in Supplementary Tables S1–S6, Supplementary Sections S1, S2). Farm type-1 (22 no., 15.71% of farms) were resource-rich extended families who employed low-to-moderate input intensity and demonstrated moderate-to-high system yield and system profitability in their farms. Farm type-2 (28 no., 20% of farms) were resource-poor extended families, who achieved high system yield by employing high input intensity and family labor. Farm type-3 (33 no., 23.57% of farms) were resource-poor feminized subsistence farms that diversified with livestock and non-farm incomes, used low input intensity in farming, and received moderate system yield and low system profitability. Farm type-4 (35 no., 25% of farms) was predominated by resource-poor and marginalized tribal nuclear families, who survived on off-farm wages, used low input intensity on their farms, and achieved moderate system yield and low system profitability. Farm type-5 (22 no., 15.71% of farms) was resource-poor, profit-oriented nuclear families, which diversified with livestock, earned little or no off-farm income and employed moderate management intensity to maintain moderate system yield and profitability. A summarized qualitative description of farm types is given as Supplementary Information (Supplementary Figure S3; Row 1, Supplementary Table S7).
We assessed the sustainability of 140 farms by a composite index built on 39 indicators covering social, economic, and ecological dimensions of sustainability (Supplementary Table S8). The mean sustainability score for FT-1 was highest, followed by FT-2, FT-5, FT-3, and FT-4 (Figure 1A). The resource-rich large families (FT-1) fared better than other farms in terms of multifunctionality, balanced soil reaction, contact with extension functionaries, lower cost of production, availability of cereals, and adoption of sustainable agricultural practices (Figure 1B). FT-2 closely followed FT-1 in all the above indicators and came next to highest in terms of women's engagement as family labor, family dependency ratio, women's access to farm resources, use of family labor in farming, lesser soil salinity, tree species diversity, per-capita income, the proportion of irrigated land, system profitability, use of organic manure, and rice equivalent yield. FT-5 followed FT-1 or FT-2 in terms of several indicators and emerged next to the highest in livestock index and distance to the road. FT-3 fared best in terms of Nitrogen, Phosphorus, and Potassium (NPK) use, pesticide use, distance to market, access to financial institutions, use of indigenous knowledge and per-capita food availability, apart from being second best in terms of livestock index, training, availability of cereals, and soil fertility. FT-4 fared well in terms of income diversity and soil fertility; they emerged second best in terms of distance to market, NPK use, pesticide use, and use of indigenous knowledge. An explanatory note on the rationale of abovesaid characterization (Supplementary Section S2, Supplementary material) and a summarized description (Row 2, Supplementary Table S7) are given as Supplementary material. An explanatory scheme shows sustainability regimes of different farm types concerning their assets and capabilities for a better comprehension of the readers (Supplementary Figure S4).
Figure 1. (A) Sustainability score of farms under five farm types. Sustainability is assessed by a composite sustainability index developed from 39 indicators (Supplementary Table S3). The indicators are normalized, winsored, weighted and linearly aggregated to develop the composite index. (B) Performance of different farm types in terms of 39 sustainability indicators. Indicator scores are standardized weighted values. Circles are scaled by indicator scores. Encircled dots have higher variability (>100% CV) within a farm type, and the deeper colors represent high variability. Boxes at the extreme right show indicator-level variation (average CV% of 39 indicators), deeper colors being more variable. Larger dots, which are not encircled, are considered more stable and used to characterize farm types.
We examined the abundance of four types of RI motifs, namely linkage, reciprocal linkage, triad, and presence (of a resource) at the core (see definitions in Supplementary Table S10) in five identified farm types (Figure 2) to characterize them in terms of these interactions. The heatmap cells represent the proportion of farms under individual farm types having a specific RI motif. Both FT-1 and FT-2 are characterized by R- (Rice->Cattle), H-(Homestead->Tree), and V (Vegetables->Poultry) based linkages, R-based reciprocal linkages (Rice <->Cattle), R- and V-based triads (ΔRice <->Cattle <->Homestead), and presence of R and V (R, V, and C for FT-2) at the core of RI network. Although the abundance of RI is similar in FT-1 and FT-2, the difference primarily exists in the magnitude of their abundance. Also, in FT-2, the importance of C and the use of CP and F are unique. Unlike FT-1 and FT-2, FT-3 is characterized by linkages involving diverse resources such as R, H, V, PL, PN, and C; predominantly H, PL, PN, and V-based reciprocal linkages; R, V, PN, PL, and H-based triads; and the presence of H and V at the core of the RI networks. FT-4 shows fewer linkages, reciprocal linkages, and triads, and features the presence of H at the core of the networks. FT-5 is characterized by a smaller number of diverse linkages involving R, H, and C, very few reciprocal linkages and triads, and the presence of R, V, and C at the core of the RI networks. Overall, FT-1 and FT-2 employed similar resource integration strategies centering around R, H, and V, with FT-2 integrating C and V slightly more than FT-1. FT-3 used diverse resources for interaction—centering around H—that could spatially accommodate V, PN, PL, C, and K due to their physical proximity. For FT-4, the paucity of resources and dependence on off-farm income might have resulted in a lack of resource interaction on their farms. FT-5, on the other hand, showed a diverse but non-predominant nature of resource interactions centering on rice fields (R), homesteads (H), and cattle (C) (see Row 3, Supplementary Table S7 for a typology-wise detailed description).
Figure 2. Abundance of different types of resource interaction motifs across farm types—(A) Tie/linkage; (B) Reciprocal tie/linkage; (C) Triad; and (D) Presence in the core. Abundance indicates the percentage of farms having a type of resource interaction within a given farm type.
We examined the relationship of RI with farm sustainability by examining the proximity of discrete RI (squares) with the sustainable farms (larger circles, area scaled by farm sustainability score) in a graph theoretic layout of the two-mode RI-farm network (see Supplementary Figures S5a–S5d; an explanatory description is given in Section S4) and identified the discrete RI associated with highly sustainable farms (Row 4, Supplementary Table S7). Scrutiny of the farms in the ninth decile of the sustainability score provided a hint of how the highest sustainability was achieved, irrespective of farm types, by using specific RI motifs and not others (Figures 3A–D; Row 1, Table 1). Scrutiny of Row 1 in Table 1 and Row 4 in Supplementary Table S7 (and Figures 3A–D and Supplementary Figures S5a–S5d) shows a high commonality of RI associated with sustainable farms. This indicates that higher sustainability was achieved by a small number of unique RI motifs in individual farm types. Summarily, we found that farms achieved higher sustainability by having R-, V, C-, PN-, PL-, H-, and K-based linkages; R- and PN-based reciprocal linkages; R-, V-, PN-, K-, and H-based triads; and presence of R, V, C, K, PN, and PL at the core.
Figure 3. Relationship between the occurrence of different types of resource interaction (RI) motifs (boxes) with farm sustainability among the farms of ninth decile (of farm sustainability score). Four types of RI motifs are—(A) Tie/linkage; (B) Reciprocal ties/linkage; (C) Triads; and (D) Presence in the Core. The closeness of boxes and circles in the graph-theoretic layout denotes higher tie strength between farms and RI. Color scheme of nodes: FT 1—Red; FT 2—Green; FT 5—Gray. No farms from FT-4 and FT-5 featured in the figures. Nodes are moved minimally to make the labels legible.
Table 1. Occurrence and cooccurrence of resource integrations patterns in the highly sustainable farms.
We also studied the co-occurrence of RI motifs, meaning the simultaneous occurrence of RI motifs on the same farm. We performed the co-occurrence study for two reasons—first, the co-occurrence of resource interaction on a farm is expected to be more stable because of its possible interdependence tested by the farmers. Hence, studying them gives us an idea of what RIs go together and stabilize in smallholder systems of a region as a response to the internal and external stimulus of change to the farming systems. Second, in the absence of panel data on farm RI or empirical study on the evolution of regional farming systems, the study of the co-occurrence of RI motifs could suggest the possible evolutionary stage of RI in smallholder systems. Despite the limitation of snapshot data, we can still examine how these co-occurrence patterns of RI gravitated toward highly-sustainable farms. We segregated the co-occurrence of RIs for farms of the ninth decile of sustainability (Figures 4A–D; Row 2, Table 1). These farms, irrespective of their farm types, featured 35 co-occurring linkages, 11 co-occurring reciprocal linkages, 28 co-occurring triads, and the presence of R, V, and PN at the core in terms of their high tie strengths (Row 2, Table 1). This means that these RIs are more closely linked and likely to co-occur on the same farm to achieve high farm sustainability. Summarily, we found that farms achieved higher sustainability by having R-, V-, C-, PN-, H-, PL- and K-based linkages; R- and PN-based reciprocal linkages; R-, V-, PN-, K-, and H-based triads; and presence of R, C, and K at the core. The co-occurrence network for all 140 farms is given as supplementary information (Supplementary Figures S6a–S6d). Notably, a high proportion of co-occurrences (recorded on 140 farms) are retained by the highly-sustainable farms of the highest decile.
Figure 4. Co-occurrence pattern of different types of resource interactions (RI) motifs for the farms of the ninth decile of sustainability score. Co-occurrence patterns for the RI motifs are—(A) Tie/linkage; (B) Reciprocal ties/linkage; (C) Triads; and (D) Presence in the Core. The proximity of RIs indicates the similarity of tie strength in a metric multi-dimensional layout of valued data. Nodes are moved minimally to make the labels legible.
Finally, we identified 32 linkages, 11 reciprocal linkages, 21 triads, and three core elements (Row 3, Table 1) that occurred and co-occurred in the highly sustainable farms (of the highest decile), and thus most critical to contribute to farm sustainability. Concrete examples of these critical RI motifs are given in Table 2.
We also explained the sustainability of the sampled farms by the properties of the individual farm's RI networks. We computed 11 network properties (Supplementary Table S10) for all the farms' RI networks and reduced their multicollinearity to two principal components (PC)—“connectedness” (PC1) and “reciprocity and transitivity” (PC2), and used them as the predictors of farm sustainability score. After examining 12 models, we found a model explaining 88.7% of the variance in farm sustainability scores (Figure 5A). We also examined how the model performs within different farm types (Figure 5B) and found the linear correlation for the five farm types to be 0.809, 0.715, 0.638, 0.727, and 0.86. These findings imply that resource interaction within a farm when expressed in terms of its network properties, can explain the farm sustainability measured by 39 multi-dimensional indicators, and this holds significantly true for all farm types.
Figure 5. Relationship between the observed and predicted value of sustainability score (A) for all farms taken together and (B) for five farm types separately. Predictor variables are two principal components extracted from 11 network properties of farm resource interaction. The output is based on the three best-performing models in terms of their correlation value and relative error.
We also examined the nature of this relationship at different values of PC1 and PC2 separately (Figures 6A, B). We found that farm sustainability increased monotonically with increased PC1 and found two areas of stacked points (farms) (Figure 6A), roughly the boundary of PC1 for one or more type/s of farms. While the first stack is the boundary of PC1 for FT-3 and FT-4, the second stack is the boundary for all other farm types. This indicated that RI, as a result of increased connectedness, could not be advanced by those groups of farms beyond a specific limit, although differential sustainability was achieved by farms of the same type with that same level of connectedness. These boundaries suggest a resource-limiting condition for a large number of resource-poor farms. We also found that PC2 enhanced farm sustainability up to a point and declined after that (Figure 6B). Here, also we find two stacks of farms—first, the highly-sustainable farms of FT-1, FT-2, and a few farms from FT-5; second, the poorly-sustainable farms of FT-3 and FT-4. We anticipate that highly-sustainable farms do not need to engage in many reciprocal and transitive RI on their farms since they could achieve substantial sustainability with one-way connectedness and do not need to move further toward the right of the x-axis. On the other hand, the second stack of farms has limited resources, which are located close to each other and easily linked resources (enhancing their reciprocity and transitivity). However, this could lift only a smaller proportion of farms above-median sustainability (Figure 6B).
Figure 6. Relationship between the principal components (PC) of network properties of resource interaction and farm sustainability for different farm types. Two PCs, namely PC1 (Connectedness) and PC2 (Reciprocity and Transitivity) are extracted from 11 network properties, and the sustainability score is measured by a composite index. (A) PC1 shows a linear, and (B) PC2 shows a non-linear relationship with farm sustainability score. Lines flanking the trendline are confidence intervals, and the horizontal line is the median sustainability score.
The study results suggested a suite of rice, vegetables and pond-based integration strategies for the integrated farms in Indian Sundarbans. The region is marked by serious biophysical stresses such as salt-water intrusion, prolonged inundation, and seasonal salinity, coupled with extreme climatic events. Moreover, the farm size is extremely small (mostly below one ha) and optimal resource use is key to livelihood sustenance and resilience against vulnerabilities. Resource integration is a survival strategy for the farmers and there are popular instances of resource integration models in the region (Basu et al., 2008; Mandal et al., 2019). While the focus on such models is overwhelming at the policy level, there could be more parsimonious and context-specific interventions in these farming systems. The structural bases (RI motifs) of such resource interactions are scattered across millions of farms in coastal India and they are not necessarily assorted in models, which are demonstrated by research institutions. We tried to decipher the discrete RI motifs that evolved over the years and hold key to the farm sustainability in these fragile socioecological systems.
It is often debated that farm sustainability depends on farm size, although there exist diverse alternative arguments and perspectives (Woodhouse, 2010; Dasgupta et al., 2021), and for our data set, no such association was found between the two. Moreover, an increase in the size of individually-owned farmland is unlikely to occur in most developing nations (Hazell and Rahman, 2014), and it is pragmatic to find ways of achieving sustainability in these smaller parcels of land by using endogenous resources innovatively and efficiently. Parallel to this, evidence suggests a limit to agricultural intensification in densely-populated areas (Jayne et al., 2014; Peng et al., 2022). We try to understand what could happen to farm sustainability had we not intervened externally and let them operate with their existing resources, practices, and technologies. We wanted to know what resource interactions were working well out there and for whom? We argue that farm types in the study regions have distinct characteristics, which have evolved over time, and farm resources and their interactions are among the underlying bases and mechanisms of such evolution (Chopin et al., 2015; Thomson et al., 2019). We tell a part of the story of farm sustainability in this article since farms do not sustain by resource interaction only. There is a limit beyond which resource interaction may not work and need external intervention to move to a different sustainability regime (Tittonell, 2014a). We show what “rules of the game” (pattern of RI motifs) operated in small farms, for whom, and how they could have translated into farm sustainability.
Farming systems undergo endogenous intensification due to land constraints (Boserup, 1965), often resulting in the present context of sustainable intensification. Most farms in FT-1 have not felt the pressure of unsustainability, probably due to the larger land size owned by joint extended families and substantial off-farm income that could support the family across seasons. On the other extreme, FT-4 had little resources and access to formal institutions to get themselves extricated from unsustainability and a poverty trap. While FT-2 intensified their smaller lands and earned off-farm incomes to sustain their farm and livelihoods, FT-3 resorted to migration and received off-farm income in the form of remittance. FT-5 diversified and commercialized farming with little dependence on off-farm income. Hence, the Boserupean explanation of agricultural intensification as a response to increased pressure on resources holds partially true for FT-2 and FT-5.
On the other hand, FT-3 demonstrates something close to a Malthusian explanation where farming is abandoned partially for earning off-farm incomes. Interestingly, apart from FT-5, all other FTs depended on off-farm income to sustain their farms and livelihoods. This trend of simultaneous intensification and resource integration in farms and migration is reported in the context of Africa and Asia (Demont et al., 2007; Hua et al., 2019). Similarly, the overarching importance of off-farm income (Supplementary Figure S4) suggests a need to examine further the role of off-farm income in shaping resource-use patterns and farm sustainability. Although there are dissimilarities between different farm types in terms of their characteristics (assets, practices, and outcomes) and abundance of RI motifs (Figure 2), we find only a few unique co-occurrences of RI motifs on the same farm. This suggests that a group of farms can potentially be put on a different sustainability regime (that is, a farm type) just by introducing a minimal number of, or combinations of, RI motifs in farming systems.
We find that particular occurrences (Figures 3A–D) and co-occurrence (Figures 4A–D) of RIs are associated with highly-sustainable farms. This commonality of occurrence and co-occurrence of RI motifs on sustainable farms gives us reasonable confidence to conclude that these motifs are associated with higher farm sustainability. Our analyses have identified 32 discrete linkages, 11 reciprocal linkages, 21 triads, and three core components associated with higher sustainability of small-scale farms since they were associated with highly-sustainable farms and at the same time co-occurred on the same farm. This conclusion is of crucial significance since we not only claim a relationship between RI and farm sustainability but find discrete RI motifs associated with farm sustainability. This is a direct entry point into the design of sustainable farming systems that avoids promoting farming system models that are either too burdensome to accept as such or need significant logistic support and social mobilization to succeed in the long run.
Our study results suggest the importance of understanding the network phenomenon of resource interaction in small farms to achieve farm sustainability through informed technological intervention. We could also understand how different farm types engaged different RIs to achieve sustainability, indicating a typology-based intervention strategy. The analysis also reveals the importance of rice-based integrations in farm sustainability of the study areas, followed by vegetables and pond-based integrations with typology-specific variations. Such findings seem realistic because of the large number of waterbodies in the study region and traditional rice-growing lowlands across the region.
In addition to finding that discrete resource interactions are associated with farm sustainability, we also found that the network properties of RIs can explain farm sustainability (Figures 5A, B) without having to include any socioeconomic and biophysical variables in the model. This observation suggests the existence of a network phenomenon of RI on small-scale farms that link to farm sustainability. The linear relationship between “connectedness” (PC1) in RIs and farm sustainability also suggests that higher resource integration can lead to higher farm sustainability. On the other hand, a non-linear relationship between “transitivity and reciprocity” (PC2) and farm sustainability—where sustainability first increases and then declines—suggests a limit to which sustainability could be increased by enhancing reciprocal resource integration or extending the resource integration indefinitely. Considering the types of farms, it emerges that more sustainable farms (mostly FT-1 and FT-2) and less sustainable farms (FT-3 and FT-4) stack at a point of the x-axis as if a sieve is stopping the farms from moving further ahead (Figures 6A, B). This suggests the limit of “connectedness” (or singular integration) and “transitivity and reciprocity” (or reciprocal and extended integration) with available farm resources.
Both sustainable and less-sustainable farms enhance sustainability by increasing RIs, but the less-sustainable farms may not increase the extent of RI due to resource constraints (Figure 6A) and increase reciprocal and extended integration to enhance sustainability (Figure 6B). Already sustainable farms, most likely, do not move ahead with enhanced reciprocation and extension of RIs, perhaps because they achieve sustainability well before the saturation in the integration using all possible resources. Alternatively, there are likely some costs to resource integration, perhaps in terms of labor and/or management complexity, that lead farms to stop once they attain a certain sustainability threshold. Less-sustainable farms continue to enhance reciprocal and extended linkages (instead of one-way linkages) but cannot enhance sustainability beyond a point. Literature also suggests a limit to which such intensification takes place in densely populated areas (Willy et al., 2019; Jain et al., 2020). This is very important to one of our fundamental rationales of the study—to optimize existing resources to achieve farm sustainability. We understand that RI can contribute to higher sustainability for resource-rich farms (with more lands/trees/livestock) and put them on a higher regime of farm sustainability; however, the smaller farms will need external support and innovation to get out of the unsustainability trap (Supplementary Figure S4). Also, sustainable farms have demonstrated a limit to sustainability which can be extended by introducing innovations and policy regulations that operate outside the scope of the farm resource use (such as marketing support, and tax reforms). Further, despite the limit to achieving sustainability with enhanced resource integration, there remains considerable variation in the magnitude of sustainability within the same farm type, suggesting farm-specific refinements within the broader scope of typology-specific interventions. On the other hand, the alternative agricultural movements, despite their huge potential, ask for investment in social capital and institution building (Rosset et al., 2011; Dorin, 2022). We argue that our approach is considered a value-added tool to those long-term efforts for transforming agricultural value chains.
Farm sustainability is often framed along a dichotomy of external input-driven farming and internally-sustained agroecological farming. While helpful in comparing key differences, this dichotomy ignores the critical observation that smallholder farms are typically distributed between these two extremes and can change their nature over time. Moreover, wholesale adoption of more integrated and “agroecologically” informed farming models is often dependent on region-specific details and exceptional social learning for mass popularization. The present study demonstrates a very different approach, more endogenous to farms themselves, by linking farm sustainability with the network properties of a farm's resource-use patterns. It also identifies discrete resource-interaction strategies that help farmers to achieve long-term sustainability. This work points to a more incremental path toward sustainability, in contrast to a wholesale transformation, which can be challenging for smallholder farms that may be risk-averse due to their already marginal socioeconomic status. Thus, the results support the argument that the promotion of discrete resource interactions can move farms along the sustainability gradient more parsimoniously (Goswami et al., 2016).
We acknowledge that sustainability in small-scale farms cannot be improved indefinitely by creating new RIs on the farms, and there is a limit to such achievement for different farm types. We understand that feasible actions on a farm are often guided by a set of biophysical and socio-political realities that fundamentally guide and constrain on-farm decision-making. Ultimately, farmers' capacity to achieve critical RIs must be understood within the broader framework of culture, power, and access to resources. Our analysis does not intend to side-step those broader issues, which fundamentally affect the success of agricultural development efforts worldwide, enriching our understanding of the crucial elements of farm sustainability.
This novel approach opens up the possibility to theorize farm sustainability as an outcome of the network phenomenon of resource utilization. It explains the structural bases of sustainability (the RI motifs) that could help design farming systems to achieve a set of desired outcomes. This is analogical to deciphering the “genetic code” of farming systems that holds the key to their sustainability, and this can open up a fundamentally different way to study farm sustainability.
In this study, the identified RI motifs (32 linkages, 11 reciprocal linkages, 21 triads, and three resources at the core) should receive attention from agricultural research to develop appropriate technology and extension for the participatory design of the farming system to achieve different pathways to farm sustainability in coastal India. For example, in the study areas, pond-based interventions hold key to the farm sustainability when a farm owns (and is ready to spare) enough land to dig/expand the pond area. Moreover, such action might involve substantial investment, which could be channelized through public-funded land development schemes. The smaller motifs (e.g., triads) of resource interaction can act as potential entry points for on-farm experimentation involving existing or new agro-technologies. For smaller landowners, resource recycling plans may be developed in a participatory manner and supported by external inputs and collectivization mechanisms. A large proportion of farm income is earned through non-farm income but rarely invested back in farming. It is important that circular investment be ensured by external or remittance-supported means to sustain productive farming. This may be in the form of small livestock or excavation of water harvesting structures, thus extending the limit of small farms' ability to enhance sustainability through resource recycling. The analytical approach may also be extended to study differential structures of RI networks in different agroecological regions and open up a new stream of functional research in farming systems research and development.
The raw data supporting the conclusions of this article will be made available by the authors, without undue reservation.
The studies involving humans were approved by Ethical Committee at the IRDM Faculty Centre, RKMVERI. The studies were conducted in accordance with the local legislation and institutional requirements. The participants provided their written informed consent to participate in this study.
RG and SN conceptualized and designed the study. SP, PD, and RG collected and supervised field data collection. RG analyzed data in discussion with SN. RG, SN, SB, and BM participated in writing, editing, and commenting on the manuscript. All authors contributed to the article and approved the submitted version.
RG was awarded the Fulbright-Nehru Fellowship, provided by the United States-India Educational Foundation which allowed him to conduct this research.
The authors declare that the research was conducted in the absence of any commercial or financial relationships that could be construed as a potential conflict of interest.
All claims expressed in this article are solely those of the authors and do not necessarily represent those of their affiliated organizations, or those of the publisher, the editors and the reviewers. Any product that may be evaluated in this article, or claim that may be made by its manufacturer, is not guaranteed or endorsed by the publisher.
The Supplementary Material for this article can be found online at: https://www.frontiersin.org/articles/10.3389/fsufs.2023.1081127/full#supplementary-material
Altieri, M. A., Funes-Monzote, F. R., and Petersen, P. (2012). Agroecologically efficient agricultural systems for smallholder farmers: contributions to food sovereignty. Agronomy Sustain. Dev. 32, 1–13. doi: 10.1007/s13593-011-0065-6
Andrieu, N., Howland, F., Acosta-Alba, I., Le Coq, J. F., Osorio-Garcia, A. M., Martinez-Baron, D., et al. (2019). Co-designing climate-smart farming systems with local stakeholders: a methodological framework for achieving large-scale change. Front. Sustain. Food Syst. 3, 37. doi: 10.3389/fsufs.2019.00037
Apata, T. G., N'Guessan, Y. G., Ayantoye, K., Borokini, A., Okanlawon, M., Bamigboye, O., et al. (2020). Doggedness of small farms and productivity among smallholder farmers in Nigeria: Empirical linkage and policy implications for poverty reduction. Bus. Strat. Dev. 3, 128–142. doi: 10.1002/bsd2.83
Basso, B., Liu, L., and Ritchie, J. T. (2016). A comprehensive review of the CERES-wheat,-maize and-rice models' performances. Adv. Agron. 136, 27–132. doi: 10.1016/bs.agron.2015.11.004
Basu, D., Banerjee, S., and Goswami, R. (2008). Vegetable cultivation on ail (bund): a successful farmer-led technology transfer. Int. Sympos. Socio-Econ. Impact Modern Veg. Prod. Technol. Tropical Asia 809, 155–160. doi: 10.17660/ActaHortic.2009.809.13
Borgatti, S, P., Everett, M, G., and Freeman, L, C. (2002). UCINET 6 for Windows: Software for Social Network Analysis. Harvard, MA: Analytic Technologies.
Borgatti, S. P., and Everett, M. G. (2000). Models of core/periphery structures. Soc. Net. 21 375-395. doi: 10.1016/S0378-8733(99)00019-2
Boserup, E. (1965). The Conditions of Agricultural Growth: The Economics of Agrarian Change Under Population Pressure. Ithaca: Aldine Publishing Company; Costa Rica: USA and CATIE. Turrialba.
Brunori, G., Avermaete, T., Bartolini, F., Brzezina, N., Grando, S., Marsden, T., et al. (2020). “Small farming and food and nutrition security,” in Innovation for Sustainability (Research in Rural Sociology and Development, Vol. 25), eds. Brunori, G., and Grando, S,. Bingley: Emerald Publishing Limited, 19–38.
Cassman, K. G., and Grassini, P. (2020). A global perspective on sustainable intensification research. Nat. Sustain. 3, 262–268. doi: 10.1038/s41893-020-0507-8
Chopin, P., Blazy, J. M., and Doré, T. (2015). A new method to assess farming system evolution at the landscape scale. Agronomy Sustain. Dev. 35, 325–337. doi: 10.1007/s13593-014-0250-5
Cui, Z., Zhang, H., Chen, X., Zhang, C., Ma, W., Huang, C., et al. (2018). Pursuing sustainable productivity with millions of smallholder farmers. Nature 555, 363. doi: 10.1038/nature25785
Dasgupta, P., Goswami, R., Ali, M. N., Chakraborty, S., and Saha, S. (2017). Identifyin g sustainability assessment indicators for assessing the sustainability of smallholde r integrated farms in coastal West Bengal, India. Asian J. Agricult. Exten. Econ. Sociol. 1–14. doi: 10.9734/AJAEES/2017/35516
Dasgupta, P., Goswami, R., Chakraborty, S., and Saha, S. (2021). Current Research in Environmental Sustainability. Curr. Res. Environ. Sustain. 3, 100089. doi: 10.1016/j.crsust.2021.100089
Demont, M., Jouve, P., Stessens, J., and Tollens, E. (2007). Boserup versus Malthus revisited: Evolution of farming systems in northern Côte d'Ivoire. Agricult. Syst. 93, 215–228. doi: 10.1016/j.agsy.2006.05.006
Dorin, B. (2022). Theory, practice and challenges of agroecology in India. International J. Agricult. Sustain. 20, 153–167. doi: 10.1080/14735903.2021.1920760
FAO. (2014). Sustainability Assessment of Food and Agricultural Systems Guidelines, Version 3.0. Rome: FAO. Available online at: https://www.fao.org/3/i3957e/i3957e.pdf
Gajbhiye, K. S., and Mandal, C. (2000). Agroecological Zones, Their Soil Resource and Cropping Systems. Status of Farm Mechanization in India, Cropping Systems, Status of Farm Mechanization in India. p. 1–32. Available online at: http://el.doccentre.info/eldoc1/k30_/01jan00sfm1.pdf (accessed April 12, 2019).
Gathala, M. K., Laing, A. M., Tiwari, T. P., Timsina, J., Islam, M. S., Chowdhury, A. K., et al. (2020). Enabling smallholder farmers to sustainably improve their food, energy and water nexus while achieving environmental and economic benefits. Renew. Sustain. Energy Rev. 120, 109645. doi: 10.1016/j.rser.2019.109645
Gathala, M. K., Laing, A. M., Tiwari, T. P., Timsina, J., Rola-Rubzen, F., Islam, S., et al. (2021). Improving smallholder farmers' gross margins and labor-use efficiency across a range of cropping systems in the Eastern Gangetic Plains. World Dev. 138, 105266. doi: 10.1016/j.worlddev.2020.105266
Giller, K. E., Delaune, T., Silva, J. V., Descheemaeker, K., van de Ven, G., Schut, A. G., et al. (2021). The future of farming: who will produce our food? Food Security, 13, 1073–1099. doi: 10.1007/s12571-021-01184-6
Gomez y Paloma, S., Riesgo, L., and Louhichi, K. (2020). The Role of Smallholder Farms in Food and Nutrition Security. Berlin: Springer Nature. p. 251.
Goswami, R., Dasgupta, P., Saha, S., Venkatapuram, P., and Nandi, S. (2016). Resource integration in smallholder farms for sustainable livelihoods in developing countries. Cogent Food Agricult. 2, 1272151. doi: 10.1080/23311932.2016.1272151
Goswami, R., Saha, S., and Dasgupta, P. (2017). Sustainability assessment of smallholder farms in developing countries. Agroecol. Sustain. Food Syst. 41, 546–569. doi: 10.1080/21683565.2017.1290730
Guiomar, N., Godinho, S., Rivera, M., Pinto-Correia, T., Machado, R., Czekaj, M., et al. (2021). Assessing food availability: a novel approach for the quantitative estimation of the contribution of small farms in regional food systems in Europe. Global Food Secu. 30, 100555. doi: 10.1016/j.gfs.2021.100555
Hall, A., Sulaiman, V. R., Clark, N., and Yoganand, B. (2003). From measuring impact to learning institutional lessons: an innovation systems perspective on improving the management of international agricultural research. Agricult. Syst. 78, 213–241. doi: 10.1016/S0308-521X(03)00127-6
Hazell, P. B., and Rahman, A. (2014). New Directions for Smallholder Agriculture. Oxford: OUP Oxford.
Holzworth, D. P., Snow, V., Janssen, S., Athanasiadis, I. N., Donatelli, M., Hoogenboom, G., et al. (2015). Agricultural production systems modelling and software: current status and future prospects. Environ. Model. Softw.72, 276–286. doi: 10.1016/j.envsoft.2014.12.013
Hua, X., Kono, Y., Zhang, L., Xu, E., and Luo, R. (2019). How transnational labor migration affects upland land use practices in the receiving country: findings from the China-Myanmar borderland. Land Use Pol. 84, 163–176. doi: 10.1016/j.landusepol.2019.03.012
Jain, M., Solomon, D., Capnerhurst, H., Arnold, A., Elliott, A., Kinzer, A. T., et al. (2020). How much can sustainable intensification increase yields across South Asia? A systematic review of the evidence. Environ. Res. Lett. 15, 083004. doi: 10.1088/1748-9326/ab8b10
Jat, M. L., Chakraborty, D., Ladha, J. K., Rana, D. S., Gathala, M. K., McDonald, A., et al. (2020). Conservation agriculture for sustainable intensification in South Asia. Nat. Sustain. 3, 336–343. doi: 10.1038/s41893-020-0500-2
Jayne, T. S., Chamberlin, J., and Headey, D. D. (2014). Land pressures, the evolution of farming systems, and development strategies in Africa: a synthesis. Food Pol. 48, 1–17. doi: 10.1016/j.foodpol.2014.05.014
Jones, J. W., Antle, J. M., Basso, B., Boote, K. J., Conant, R. T., Foster, I., et al. (2017). Brief history of agricultural systems modeling. Agricult. Syst. 155, 240–254. doi: 10.1016/j.agsy.2016.05.014
Kansiime, M. K., van Asten, P., and Sneyers, K. (2018). Farm diversity and resource use efficiency: targeting agricultural policy interventions in East Africa farming systems. NJAS-Wageningen J. Life Sci. 85, 32–41. doi: 10.1016/j.njas.2017.12.001
Kuyah, S., Sileshi, G. W., Nkurunziza, L., Chirinda, N., Ndayisaba, P. C., Dimobe, K., et al. (2021). Innovative agronomic practices for sustainable intensification in sub-Saharan Africa. a review. Agronomy Sustain. Dev. 41, 1–21. doi: 10.1007/s13593-021-00673-4
Li, L., Li, X., Chong, C., Wang, C. H., and Wang, X. (2020). A decision support framework for the design and operation of sustainable urban farming systems. J. Clean. Prod. 268, 121928. doi: 10.1016/j.jclepro.2020.121928
MacLaren, C., Mead, A., van Balen, D., Claessens, L., Etana, A., de Haan, J., et al. (2022). Long-term evidence for ecological intensification as a pathway to sustainable agriculture. Nat. Sustain. 5, 770–779. doi: 10.1038/s41893-022-00911-x
Mandal, U. K., Burman, D., Bhardwaj, A. K., Nayak, D. B., Samui, A., Mullick, S., et al. (2019). Waterlogging and coastal salinity management through land shaping and cropping intensification in climatically vulnerable Indian Sundarbans. Agricult. Water Manag. 216, 12–26. doi: 10.1016/j.agwat.2019.01.012
Musumba, M., Grabowski, P., Palm, C., and Snapp, S. (2017). Sustainable Intensification Assessment Methods Manual (Working Draft). Available online at: https://cgspace.cgiar.org/bitstream/handle/10568/90517/ftf_manual_oct2017.pdf?sequence=1 (accessed February 7, 2019).
Netting, R. M. (1993). Smallholders, Householders: Farm Families and the Ecology of Intensive, Sustainable Agriculture. Stanford, CA, Stanford University Press.
OECD (2008). Handbook on Constructing Composite Indicators: Methodology and User Guide. Paris: OECD publishing.
Peng, W., Robinson, B. E., Zheng, H., Li, C., Wang, F., and Li, R. (2022). The limits of livelihood diversification and sustainable household well-being, evidence from China. Environ. Dev. 43, 100736. doi: 10.1016/j.envdev.2022.100736
Pretty, J., Benton, T. G., Bharucha, Z. P., Dicks, L. V., Flora, C. B., Godfray, H. C. J., et al. (2018). Global assessment of agricultural system redesign for sustainable intensification. Nat. Sustain. 1, 441. doi: 10.1038/s41893-018-0114-0
Pretty, J., and Bharucha, Z. P. (2014). Sustainable intensification in agricultural systems. Annals Botany 114, 1571–1596. doi: 10.1093/aob/mcu205
Rao, N. H., and Rogers, P. P. (2006). Assessment of agricultural sustainability. Current Sci. 91, 439–448. Available online at: https://www.currentscience.ac.in/Volumes/91/04/0439.pdf
Rockström, J., Williams, J., Daily, G., Noble, A., Matthews, N., Gordon, L., et al. (2017). Sustainable intensification of agriculture for human prosperity and global sustainability. Ambio 46, 4–17. doi: 10.1007/s13280-016-0793-6
Roling, N., and Engel, P. (1991). “The development of the concept of the Agricultural Knowledge and Information System (AKIS): implications for extension,” in Agricultural Extension, eds. Rivera, W. M., and Gustafson, D. J. Amsterdam: Elsevier Science Publishers.
Rosset, P. M., Machín Sosa, B., Roque Jaime, A. M., and Ávila Lozano, D. R. (2011). The Campesino-to-Campesino agroecology movement of ANAP in Cuba: social process methodology in the construction of sustainable peasant agriculture and food sovereignty. J. Peasant Stud. 38, 161–191. doi: 10.1080/03066150.2010.538584
Schut, M., Klerkx, L., Rodenburg, J., Kayeke, J., Hinnou, L. C., Raboanarielina, C. M., et al. (2015). RAAIS: Rapid Appraisal of Agricultural Innovation Systems (Part I). a diagnostic tool for integrated analysis of complex problems and innovation capacity. Agricult. Syst. 132, 1–11. doi: 10.1016/j.agsy.2014.08.009
Scoones, I. (1998). Sustainable Rural Livelihoods: A Framework for Analysis. IDS Working Paper 72. Brighton: IDS.
Spielman, D. J., Davis, K., Negash, M., and Ayele, G. (2011). Rural innovation systems and networks: findings from a study of Ethiopian smallholders. Agricult. Hum. Values 28, 195–212. doi: 10.1007/s10460-010-9273-y
Temel, T., Janssen, W., and Karimov, F. (2003). Systems analysis by graph theoretical techniques: assessment of the agricultural innovation system of Azerbaijan. Agricult. Syst. 77, 91–116. doi: 10.1016/S0308-521X(02)00087-2
Thomson, A. M., Ellis, E. C., Grau, H. R., Kuemmerle, T., Meyfroidt, P., Ramankutty, N., et al. (2019). Sustainable intensification in land systems: trade-offs, scales, and contexts. Current Opin. Environ. Sustain. 38, 37–43. doi: 10.1016/j.cosust.2019.04.011
Tittonell, P. (2014a). Ecological intensification of agriculture—sustainable by nature. Current Opin. Environ. Sustain. 8, 53–61. doi: 10.1016/j.cosust.2014.08.006
Tittonell, P. (2014b). Livelihood strategies, resilience and transformability in African agroecosystems. Agricult. Syst. 126, 3–14. doi: 10.1016/j.agsy.2013.10.010
Wezel, A., Casagrande, M., Celette, F., Vian, J. F., Ferrer, A., and Peigné, J. (2014). Agroecological practices for sustainable agriculture. A review. Agronomy Sustain. Dev. 34, 1–20. doi: 10.1007/s13593-013-0180-7
Willy, D. K., Muyanga, M., and Jayne, T. (2019). Can economic and environmental benefits associated with agricultural intensification be sustained at high population densities? A farm level empirical analysis. Land Use Pol. 81, 100–110. doi: 10.1016/j.landusepol.2018.10.046
Woodhouse, P. (2010). Beyond industrial agriculture? Some questions about farm size, productivity and sustainability. J. Agrarian Change 10, 437–453. doi: 10.1111/j.1471-0366.2010.00278.x
Keywords: resource integration, network analysis, sustainability, farm typology, small-scale farm
Citation: Goswami R, Brodt S, Patra S, Dasgupta P, Mukherjee B and Nandi S (2023) Resource interaction in smallholder farms is linked to farm sustainability: evidence from Indian Sundarbans. Front. Sustain. Food Syst. 7:1081127. doi: 10.3389/fsufs.2023.1081127
Received: 26 October 2022; Accepted: 29 August 2023;
Published: 14 September 2023.
Edited by:
Subhash Babu, Indian Agricultural Research Institute (ICAR), IndiaReviewed by:
Sanjit Maiti, National Dairy Research Institute (ICAR), IndiaCopyright © 2023 Goswami, Brodt, Patra, Dasgupta, Mukherjee and Nandi. This is an open-access article distributed under the terms of the Creative Commons Attribution License (CC BY). The use, distribution or reproduction in other forums is permitted, provided the original author(s) and the copyright owner(s) are credited and that the original publication in this journal is cited, in accordance with accepted academic practice. No use, distribution or reproduction is permitted which does not comply with these terms.
*Correspondence: Rupak Goswami, cnVwYWsuZ29zd2FtaUBnbS5ya212dS5hYy5pbg==
Disclaimer: All claims expressed in this article are solely those of the authors and do not necessarily represent those of their affiliated organizations, or those of the publisher, the editors and the reviewers. Any product that may be evaluated in this article or claim that may be made by its manufacturer is not guaranteed or endorsed by the publisher.
Research integrity at Frontiers
Learn more about the work of our research integrity team to safeguard the quality of each article we publish.