- Department of Civil Engineering, McMaster University, Hamilton, ON, Canada
The growing global population and climate change threaten the availability of many critical resources, and have been directly impacting the food and agriculture sector. Therefore, new cultivation technologies must be rapidly developed and implemented to secure the world's future food needs. Closed-loop greenhouse agriculture systems provide an opportunity to decrease resource reliance and increase crop yield. Greenhouses provide versatility in what can be grown and the resources required to function. Greenhouses can become highly efficient and resilient through the application of a closed-loop systems approach that prioritizes repurposing, reusing, and recirculating resources. Here, we employ a text mining approach to research the available research (meta-research) and publications within the area of closed-loop systems in greenhouses. This meta-research provides a clearer definition of the term “closed-loop system” within the context of greenhouses, as the term was previously vaguely defined. Using this meta-research approach, we identify six major existing research topic areas in closed-loop agriculture systems, which include: models and controls; food waste; nutrient systems; growing media; heating; and energy. Furthermore, we identify four areas that require further urgent work, which include the establishment of better connection between academic research to industry applications; clearer criteria surrounding growing media selection; critical operational requirements of a closed-loop system; and the functionality and synergy between the many modules that comprise a closed-loop greenhouse systems.
1. Introduction
As a result of the disparate distribution of the global food supply (Messner et al., 2021), food loss, and food waste (Kusumowardani et al., 2022), current food supply management and production practices are insufficient to support the projected global population of 9.9 billion by the year 2050 (The International Institute for Sustainable Development, 2020; Movilla-Pateiro et al., 2021). The increase in global population impacts every country, not only those experiencing growth. There will be increased demand for food production and supply in order to feed the world. Environmental factors, including climate change, water scarcity, and declining soil nutrition, pose additional challenges for producers (Goddek et al., 2015).
For centuries, agriculture predominantly consists of crop cultivation in outdoor settings. Food cultivation in indoor environment has been explored as a viable alternative since the 20th century. Greenhouses are the most common indoor system for agriculture is a greenhouse, which provides controlled weather conditions, modulates flow of mass and energy, and minimizes disease risks (Goto et al., 2013; Manonmani et al., 2018). More recently, closed-loop systems emerged as an area for innovation in agriculture. A closed-loop system is used for repurposing, reusing, and recirculating resources within a system. In the context of agriculture, the objective of a closed-loop system is to minimize the inputs needed throughout a growing cycle in all aspects, including water, nutrients, and soil, among others. Due to their ability to conserve resources, closed-loop systems within greenhouse infrastructure show promise in advancing the food and agriculture industry (Goumopoulos et al., 2014; Ruf́ı-Saĺıs et al., 2020). The implementation of closed-loop systems in various components of greenhouses contributes to sustainable production, sustainable agriculture, and food security, which are included as part of the United Nations Sustainable Development Goals (SDGs) (United Nations Department of Economic and Social Affairs, 2022).
There are various sustainability benefits of a closed-loop greenhouse. Agriculture practices can impact the environment and ecology as a result of their energy consumption, water use and disposal, and emissions (Wu et al., 2019). Current agriculture practices are contributing to the continual rise in water scarcity around the world, since 72% of the freshwater withdrawn globally is used for agriculture purposes (United Nations Water, 2021). This is impacting areas currently suffering from water stress and can cause water stress in new areas (Goddek et al., 2015). Closed-loop systems have the potential to alleviate water shortage concerns in arid and semi-arid climates through water recirculation (Li et al., 2020). Indeed, water conservation in closed-loop systems has been demonstrated in numerous experiments (Chrouta et al., 2019). The conservation of land resources is another beneficial feature of closed-loop greenhouse systems. The amount of land resources available for traditional agriculture is decreasing (Helmstedt et al., 2018) while existing agricultural methods negatively impact the land's soil quality (Wu et al., 2019). Farmland is decreasing as a result of the population sprawling and expanding urban areas (de Jesus Rubio et al., 2011). Research has shown that rooftop greenhouses with closed-loop systems are suitable for a variety of buildings and show promise in solving urban agriculture limitations and constraints (Ruf́ı-Saĺıs et al., 2020). Optimizing greenhouses within the framework of closed-loop approach can allow for increased flexibility in where food is grown and increased yields while decreasing costs (Su et al., 2017b).
Lastly, closed-loop greenhouse systems contribute to minimizing and eliminating waste, which reduces the environmental footprint of food production (Williams, 2021). Some studies have conducted life cycle assessments (LCA) on closed-loop greenhouse systems, which revealed the benefits and constraints of these systems. Long-term benefits of closed-loop greenhouse systems include improvement of local resource production (Williams, 2021). However, short-term negative impacts of closed-loop greenhouse systems include the use of fossil fuels and the associated greenhouse gas emissions when developing new infrastructure (Ruf́ı-Saĺıs et al., 2020). Greenhouses have a shorter lifespan compared to other infrastructure, which requires that their carbon footprint and resource demand during construction to be properly accounted for in an LCA (Torrellas et al., 2012). LCA of closed-loop greenhouses systems is an essential step in rationally designing these systems to offset negative impacts from the construction of greenhouse infrastructure.
In addition to application on Earth, closed-loop systems have been considered for space missions to the Moon and Mars. With extremely minimal resources available on space exploration missions, it is critical that life support systems reuse and repurpose as many inputs and outputs as possible. The Micro-Ecological Life Support System Alternative (MELiSSA) design began in 1989, and evolved over the years to house various compartments for the crew, food growth, and others, and connect them all together for a fully operational loop to share inputs and outputs (Poughon et al., 2009). Since this project, various other research agencies have begun their own development. Other projects such as the EDEN International Space Station project have been prototyped and are being tested (Maiwald et al., 2021). The development of closed-loop systems for greenhouses for space exploration shows that this is a promising method to grow a reliable food supply with minimal inputs.
The adoption of a closed-loop system, however, does not come without challenges. Various components of the overall greenhouse have differing limitations. For example, while the use of biochar in closed-loop agriculture systems reduces waste production and reduces water usage, its production creates higher air pollution (Li et al., 2020). Another example would be the implementation of food waste recovery in a closed-loop system (Dsouza et al., 2021). The implementation of such a system would allow for reduced system outputs and allow for the reuse of nutrients already within the system. However, the heterogeneity of food waste makes it challenging to predict the outcome of the composting process.
With the vast potential application of closed-loop systems in greenhouses, it is critical to clearly understand the areas that past research has explored and where knowledge gaps still remain. Smart literature review and meta-research have used topic modeling for many years. Topic modeling is a favorable approach to this form of research as it groups text by similarities and content, in order to prescribe the topics (Asmussen and Møller, 2020). This approach can highlight key topics of work that may not otherwise emerge from a qualitative review of the literature. Topic modeling is also replicable, allowing further analysis to be run in the future with additional papers to identify potential new emerging topics (Asmussen and Møller, 2020). The approach has been applied to various research areas including but not limited to, supply chain analytics for enterprise information systems (Asmussen and Møller, 2020), resilient cities' critical infrastructure interdependence (Haggag et al., 2019), and prevention of COVID-19 (Nothacker et al., 2022).
This study uses meta-research and text-mining techniques to identify key topic areas based on past research regarding closed-loop agriculture systems has explored (Blei et al., 2003). Quantifiable results were generated by leveraging targeted topic modeling strategies. This work aims to provide an unbiased review of existing research on closed-loop systems in greenhouse infrastructure and demonstrate that there is an opportunity for greater impact when closed-loop systems are applied to the entirety of a greenhouse rather than individual components.
2. Methodology
This section details the approach used to employ text analytics on the dataset of publications under review. Text analytics in the form of topic modeling was used to uncover significant topics systematically. Topic modeling reveals existing research areas and uncovers knowledge gaps. The approach is advantageous as it minimizes biases associated with topic selection. This is because the process is guided by the papers under revisions being grouped based on relatability which then form the overall research topics (Asmussen and Møller, 2020). The approach we follow for this review can be summarized into three stages. Stage 1 of this review involved using four keywords as a guide for a literature database search as seen in Figure 1. The selected keywords of relevance for the desired objective are agriculture, closed-loop, food and greenhouse. Stage 2 topics were uncovered through word significance and topic modeling. Following that, Stage 3 provided a quantitative analysis of the topics identified in Stage 2. Stage 3 leveraged the quantifications to then cluster various words presented in the abstracts being reviewed. These clusters were an indicator of words frequently being discussed together with respect to closed-loop systems and provided the basis for the topics used when discussing the state of current research. The review of available literature indicated that research is very niche and narrow.
We use Web of Science (https://www.webofscience.com/) to identify relevant publications in closed-loop agriculture systems (Figure 2). Specifically, our query leveraged the four keywords in seven search combinations and is limited to publications between 1990 and February 4, 2022. The word “closed-loop” was a required word in each search in the following manner: (1) closed-loop and agriculture, (2) closed-loop and food, (3) closed-loop and greenhouse, (4) closed-loop and agriculture and food, (5) closed-loop and agriculture and greenhouse, (6) closed-loop and food and greenhouse, and (7) closed-loop and agriculture and greenhouse and food. Search combinations were applied to both title search and abstract search. The search yielded 2,877 publications, which were then reviewed manually to assess their relevancy. After the filtering and evaluation were completed, there was a selection of 287 publications of relevance considered for this study.
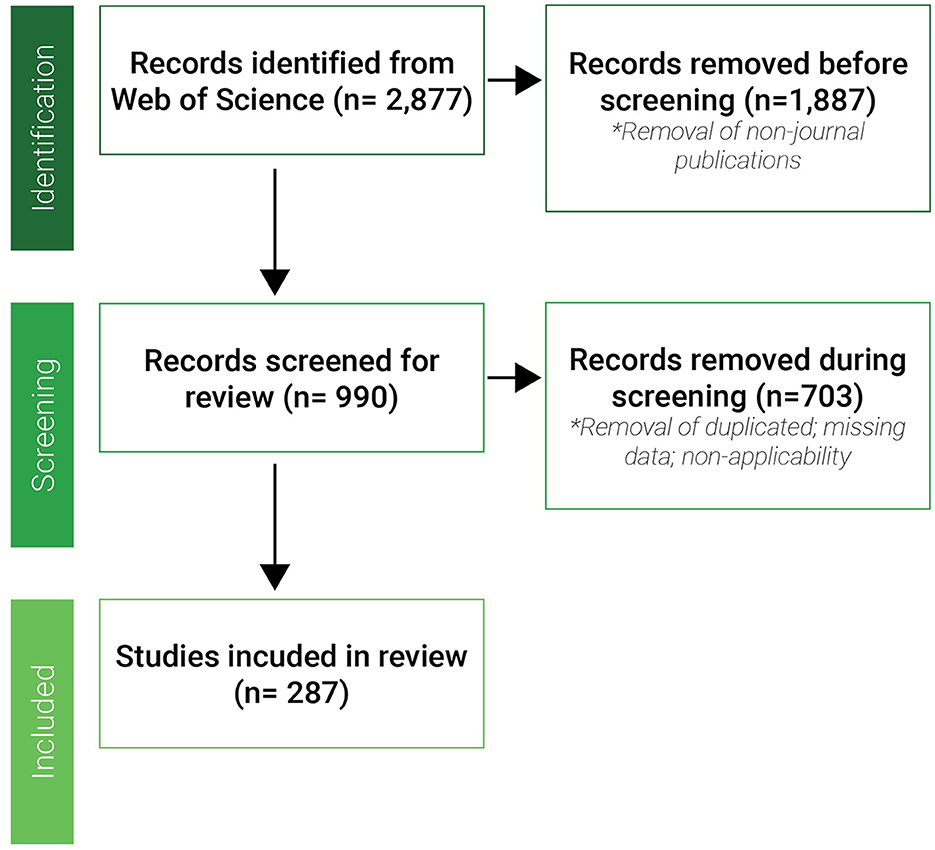
Figure 2. The Preferred Reporting Items for Systematic reviews and Meta-Analyses (PRISMA) (Page et al., 2021) as applied in this systematic review paper, indicating the number of studies included in the review.
In order to extract topics of significance from the publications, pre-processing involving steps to clean the text within the dataset was first applied, followed by word importance techniques. The outlined steps of (1) pre-processing, (2) word importance, and (3) Latent Dirichlet Allocation (LDA) were applied to the complete dataset of publications, including the titles and abstracts. Pre-processing was completed within R software (RStudio Team, 2019; R Core Team, 2020). A text transformation package, tm_map function available in R, was applied to the dataset (RDocumentation, 2019). This allows for the determination of the frequency of words identified within each individual abstract under revision and the frequency of words collectively (Haggag et al., 2019; Feinerer, 2020). LDA was then applied allowing for the assessment of discrete data through generative probabilistic modeling (Blei et al., 2003). The application of LDA indicated key topics for exploration.
2.1. Text analysis procedure
2.1.1. Pre-processing
We subject the abstracts to four data pre-processing steps (Miner et al., 2012): (1) transformation, which converts all text to lowercase format, (2) tokenization, which converts larger strings of words such as paragraphs and sentences into individual words and characters, (3) treatment, which removes common words such as “the”, “there”, “a”, etc., and (4) stemming, which removes affixes.
In order to gain a clearer understanding of significant words within the dataset, the bag of words technique was used to gain a holistic understanding of word importance within the dataset (Feinerer, 2020). The bag of words technique counts the number of occurrences of each word, and the higher occurrence words show higher significance within the dataset. Additionally, we combine common synonyms to yield a word cloud of the significant words within the dataset (Figure 3). Upon inspecting the word clouds, the following key terms were found to dominate the research field: system, control, water, food, closed-loop, and product.
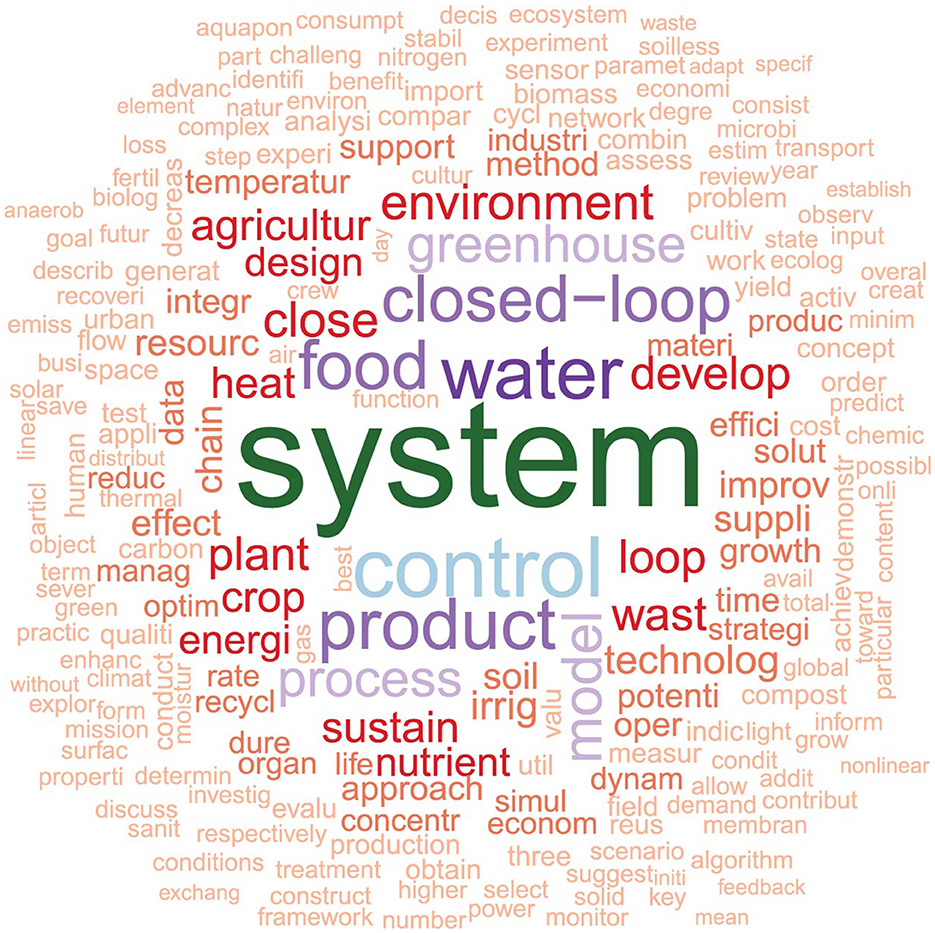
Figure 3. Illustrated word cloud following the application of all pre-processing steps. Words that occur at a higher rate in the dataset appear in larger fonts within the word cloud, while those that are not as common within the abstracts are smaller in size.
2.1.2. Latent Dirichlet Allocation
We apply topic modeling in the form of Latent Dirichlet Allocation (LDA) to identify key topics within the dataset. LDA is a probability-based modeling algorithm specifically designed for text-based datasets (Blei et al., 2003). The Griffiths' measure was selected as the LDA model. The Griffiths' measure (Griffiths and Steyvers, 2004) provides an indication of the optimal number of topics. The model aims to maximize the outcomes by indicating a measurement close to the upper limit of 1.0, based on the input parameters and constraints. These parameters and constraints set lower and upper bounds that guide the number of topics the model assesses. The model leverages Gibbs sampling as well as the Monte Carlo Markov Chain method. The posterior distribution is used to assess the given data based on previous distributions of topics and the function of likelihood.
To determine the key number of topics, instructions were first provided to analyze only abstracts within the dataset and an upper limit of topics (k). A maximum number of topics (k) was set at 20, which was selected based on the limited number of publications within the dataset for assessment. Following the application of the constraints and parameters, Griffiths' measure was generated. Subsequently, a random assignment will indicate the topic that applies to each word. A gamma distribution is then generated, indicating the probabilistic distribution of documents per topic. It then concludes with a beta distribution which indicates the probabilistic keywords that are present in each topic and includes a metric indicating how strongly present the word is within the topic.
Griffiths' measure was used to assess the distribution of topic likelihood, as shown in Figure 4. The upper limit of topics (k) was set at 20, and the lower limit of topics (k) was set at 2. The purpose of Griffiths' measure is to find the optimal number of topics which is shown by indicating the maximized value. When generating Griffiths' measure, topic maximization was indicated at 17 topics. As there are 287 publications under consideration for this study, proceeding with 17 topics would be far too many. Many of the topics would overlap and result in a less meaningful and targeted review. With this consideration, this study will select the number of topics that are captured at 75% when reviewing Griffiths' measure. The selected 75% capture rate results in 7 topics emerging from the dataset. This lower value in topics should yield well-rounded topics encompassing various aspects of a closed-loop system with minimal overlap. This results in a better review of the literature available.
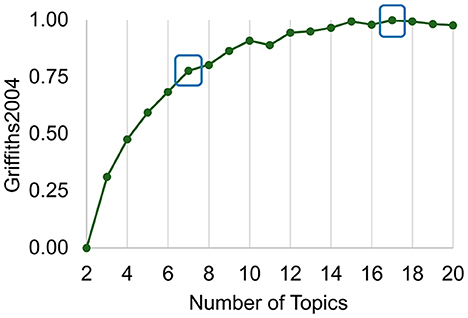
Figure 4. Latent Dirichlet Allocation of Griffiths measure. Griffiths measure also referred to as Griffiths and Steyvers (2004) along the y-axis vs. the number of topics along the x-axis.
2.2. Topic analysis
The application of Latent Dirichlet Allocation in earlier steps generated a Beta for each of the seven topics, which is the probability of words being present in the same document within each topic. While seven topics have been selected at this stage, as indicated in Figure 5, it can be seen that some topics have a significantly lower likelihood of words being present, which is indicated by the beta value. This simply indicates that they are not as likely to be present within the dataset, but they contribute to one of the topics that emerged. It can also be seen that while some topics have a distinct leading word or term with a high likelihood, there are others, such as topic six, where various words have a high probability of appearing together.
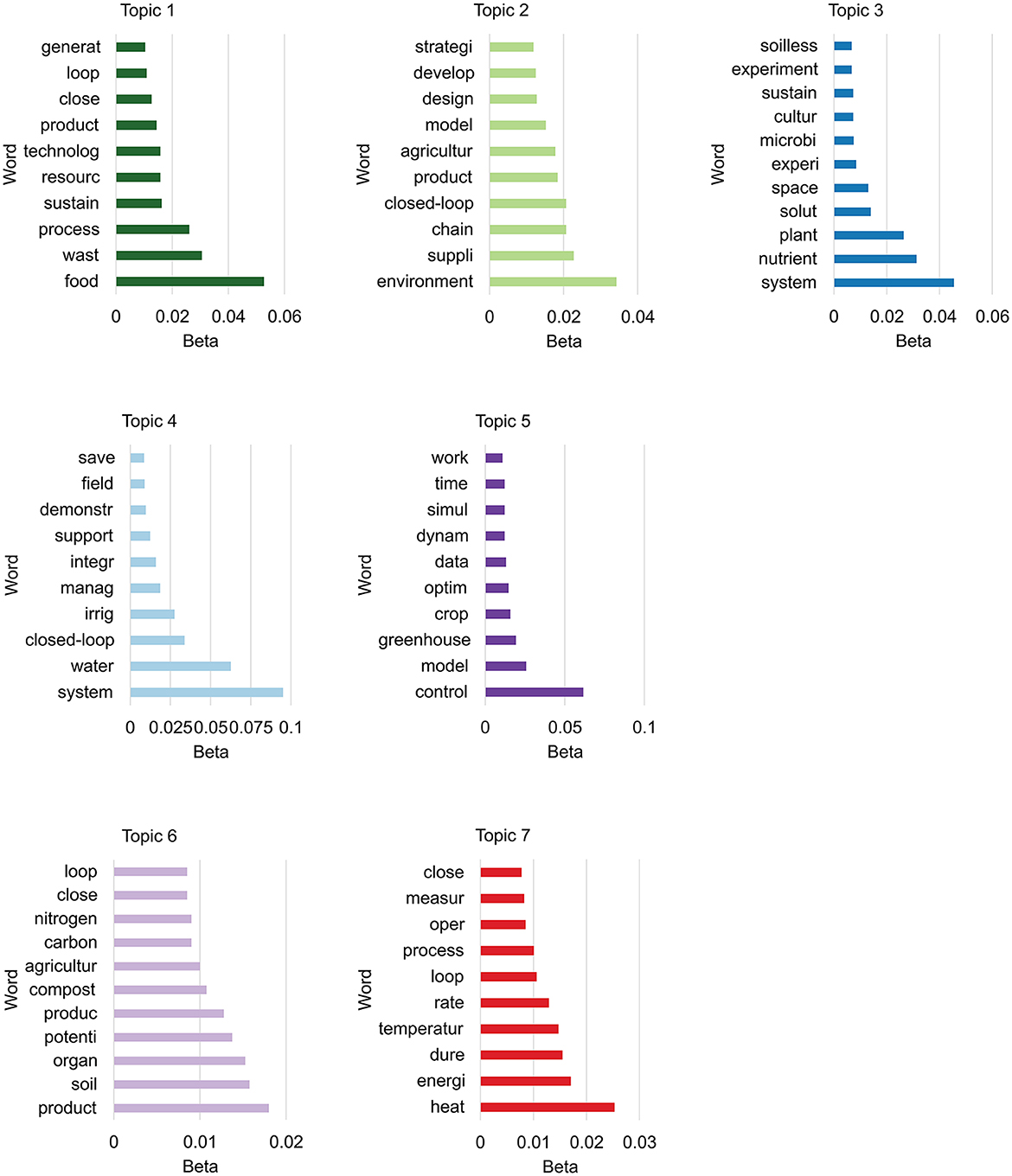
Figure 5. Beta distributions of each of the topics generated by the text-mining approach. Graphical representation of the frequency of occurrence of various words within a topic. Seven topics are uncovered, with the likelihood of a term appearing within each topic indicated along the x-axis.
Within each topic, there are words with a higher beta value; those are treated as major words that shape the topic's contents. They are then complemented by minor words based on the beta values, these words allow the topics to be tailored. Within topic 1, the words food and waste have a high probability of appearing in the same piece of literature. In topic 2, the word environment appears along words such as supplies, chain and closed-loop. Topic 3 keywords indicate an emphasis on nutrient systems, while topic 4 words indicate a focus on water systems. In topic 5, where the primary word is control, the words model, greenhouse and crop are often referred to. Topic 6 words appear as product and soil. Lastly, topic 7 focuses on heat and considers energy.
While each of these topics identifies different aspects of a closed-loop system, topic 2 will be removed from the literature review assessment. This decision has been made since the concept of the environment, closed-loop systems and supplies are represented in the literature regarding topics 1, 3, 4, 5, 6, and 7. A clearer indication of research can be provided regarding each individual topic that details its contributions and impacts on the keywords presented in topic 2. The environment is considered one of the driving factors for the utilization and implementation of closed-loop systems. Accordingly, six topics will be formed, each reviewing and assessing various aspects of closed-loop: models and controls, food waste, nutrient systems, water systems, growing media, and heat and energy.
3. Results
3.1. Models and controls
As the growth in population and climate change continue to pose challenges regarding food security, greenhouses are emerging as a favorable solution. Greenhouses provide an opportunity to grow food in various climates and geographic locations. Food accessibility is a major driving factor in the desired application of controlled environment agriculture in urban cities (Dsouza et al., 2021). Traditional greenhouse systems are challenging to control as a result of their physical and biological subsystems (Manonmani et al., 2018). The physical subsystem encompasses components of such as the greenhouse infrastructure, while the biological system encompasses factors such as the plant being grown. As there are multiple subsystems, the application of a closed-loop system is favorable to enhance the interaction and influences between the subsystems. Water systems, nutrient systems, food waste, growing media and heating and energy are some of the many components of a closed-loop greenhouse system. To best understand and optimize their functionality, models and controls are implemented.
When considering the climate and environment of a closed-loop greenhouse system, there are various components to address. When looking at energy utilization and heating, these components contribute to the biological and physiological subsystems (Manonmani et al., 2018). As such, a study was conducted to understand if neural networks could be used to provide a reliable manner in which growing conditions would be maintained. Neural networks provide an opportunity to test performance and alter parameters, such as the algorithms being used, to best optimize the system. Climate conditions such as temperature and humidity within greenhouse systems impact growing conditions and can be user-controlled (del Sagrado et al., 2016). In order to determine the best manner in which these can be controlled and optimized, studies have leveraged Bayesian networks, fuzzy adaptive controls, and parameter self-tuning proportional–integral–derivative (PID) controls, in addition to other neural network approaches.
To optimize the climate conditions, the Bayesian network can be applied to gain probabilistic outcomes of the controls and environmental conditions (del Sagrado et al., 2016). Studies have indicated that the Bayesian network provides a good level of approximation for the controls, which can then be altered by the grower. Parameter self-tuning PID controls were explored as a means of retaining aspects of system modularity (Su et al., 2020). A simulation has indicated that climate controls within greenhouses can benefit from the utilization of parameter self-tuning PID control. Various system components are distributed amongst PID controllers, which allows for modularity to occur for optimization of specific PID controllers while retaining an overall closed-loop function. Another simulation which utilized fuzzy adaptive controls aimed to determine manners in which energy within the system would be conserved by reallocation (Su et al., 2017a). Heating, fogging, and CO2 injection are the measured control laws. The simulation uses a fuzzy logic system to gauge the difference between the controller and actuator outputs. This is done as a means of calculating the actuator saturation. Based on the values captured, energy can then be allocated to other areas of the greenhouse. This provides an opportunity to resource conservation and reuse.
Lighting, water and nutrients are additional influencers of growing conditions within greenhouses. Various experiments and simulations utilizing neural networks, wireless sensor networks, Irrigation control systems, and electrical conductivity sensors have aimed to understand optimal conditions and manners in which conditions can be manipulated based on data from the system. Various control systems are implemented in order to conserve resources. A supplemental lighting management model has been developed, allowing specific plants in a greenhouse to receive additional wavelengths (Mohagheghi and Moallem, 2021). The model utilizing neural networks conserves energy by only supplying plants identified as having a deficiency based on growth indicators. This experiment was conducted in a proof-of-concept greenhouse in order to measure the success of the experimental results and was used to confirm previous studies conducted in this area. Lighting in a system can influence temperature. As a result, it influences what might be implemented for irrigation systems.
Irrigation systems can be controlled using data from a crop water stress index, an evapotranspiration model to assess the volume of water during irrigation, and infrared thermometry for the temperature of the plant's canopy (Prenger et al., 2005). Prenger et al. (2005) conducted a comparative study comparing a closed-loop and open-loop irrigation system approach and found that there was less than a 2 L/m2 per event error based on the amount of water introduced into the system when applying a closed-loop irrigation system, which improves the system's performance. The application of wireless sensor networks is an alternative approach that can be considered for irrigation systems (Yin et al., 2015). When introduced into greenhouse systems, wireless sensor networks provide automatic monitoring and controls and have been applied for drip irrigation pipes and ventilation equipment. Communication nodes allow for a network to be established and actions to be carried out based on decisions made by a dynamic simulation model. Based on simulation findings, this approach was implemented in a greenhouse and found to have a high correlation efficiency when comparing the model prediction and the observed values. Nutrients are often introduced and maintained through the water in a greenhouse system. As such, nutrient monitoring techniques have been studied for both soil and soilless culture systems. A greenhouse experiment assessed soilless culture systems in various system designs, including a closed-loop system applying electrical conductivity sensors (Ahn et al., 2021). Results indicated that succeeding nutrient ratios could be controlled by utilizing a computed nutrient ratio specifically within a closed-loop system setup. This provides an advantage in better assessing the needs of the plant being grown, and determining its future needs prior to any deficiencies possibly presenting themselves.
The simulations and experiments demonstrate the opportunity available to address various components of closed-loop systems in order to optimize outcomes and efficiencies. Growing conditions need to be maintained and monitored for optimal plant and crop production, while minimizing resource needs (Su et al., 2017b).
3.2. Food waste
Research regarding food waste within the food and agriculture sector indicated several dominant practices. The practices of anaerobic digestion, biochar, and bio-gas production are discussed as emerging solutions (Andriamanohiarisoamanana et al., 2020). By applying appropriate practices, such as a circular economy approach to organic food waste, there is an opportunity to generate revenue, reduce waste management issues, and bolster the economy (Rashid and Shahzad, 2021). However, the level of understanding regarding the advantages of adopting closed-loop technologies for organic waste varies by country and poses a challenge (van der Velden et al., 2022).
The practices proposed for application are inclusive of biochar production. Biochar can be produced from various organic materials. Green waste from tomatoes, an organic material, was repurposed into a substrate that can effectively be applied in various growing conditions (Dunlop et al., 2015). Another studied aspect of biochar is its benefits, allowing for efficient water-reutilization (Payne et al., 2018).
The practice of anaerobic digestion presents advantages for energy recovery and pollution management (Andriamanohiarisoamanana et al., 2020). Anaerobic digestion allows organic waste to be converted into biogas and digestate. Digestate can be used as fertilizer, while biogas, can contribute to renewable energy sources. There is, however, limited research on the impacts of biogas (Lin et al., 2021). With food waste being a growing global concern (Allegue et al., 2020), finding ways in which it can be repurposed can benefit the cultivation of foods.
3.3. Nutrient systems
Various aspects of a closed-loop system can be considered when researching nutrients. The vast majority of research that has been completed on closed-loop nutrient systems has been applied within hydroponics. Many studies have been conducted in order to assess the benefits and advantages of hydroponics for the circulation of nutrients within a system (Ruf́ı-Saĺıs et al., 2020).
Composting is an approach by which nutrient deficiencies or lack of nutrient accessibility can be addressed (Dsouza et al., 2021). A conceptual study based on a closed-loop environment addressed various technologies that could possibly supplement multiple aspects of the system currently posing a challenge. The ability to incorporate composting within a closed-loop system decreases biowaste and repurposes materials that can still have a positive impact. Leaves, an organic material within greenhouse systems, also impact and provides indicators in order to optimize weather regulation, determine mineral nutrient requirements, and impact the health of flora and fauna (Krupanidhi et al., 2017). Utilizing organic materials within a system can provide insights into the health and needs of the system and can often be used as an input to bolster any deficiencies.
In the early 2000s, the nutrient film technique gained popularity. This technique provides a shallow channel of water where the roots of a plant are in contact with the water, allowing for nutrients to be carried to the plants. A study was conducted to modify the concentration of nutrients present in irrigation water depending on the environmental surroundings (Zolnier et al., 2004). While the experiment had challenges with results early in the plant's growth phase and at various times in the day, it put into practice a closed-loop hydroponic system that aimed to model evapotranspiration, which influences nutrient concentration.
Lighting within a greenhouse system can lend itself to nutrient quality and availability in a system. Studies have assessed light sources and their impacts on the availability of a specific ion. As an example, a study measuring iron, which interacts and was thought to be impacted by light, determined that iron is an ion that is not inherently affected (Lykas et al., 2001). It additionally noted that there were benefits to agitating the water within a closed hydroponic system as it helped maintain the ion concentration for a more extended period.
Overall, there are various benefits when considering the nutrient systems within closed-loop greenhouse systems, which can be seen in the plants, the environment, and resource dependency. Between 35% and 54% of the nutrients within a closed-loop system were saved through recirculation (Ruf́ı-Saĺıs et al., 2020), indicating that efficient application of nutrient systems can enhance sustainability and create a feasible method for food growth.
3.4. Water systems
The agriculture sector is a heavy contributor to water usage. Determining efficient utilization strategies is significant for a sustainable closed-loop agriculture system. Literature in this area looks at both how certain aspects of water systems can be altered to adopt new practices and how technology can guide the optimization of the systems.
Transpiration is one of the primary utilizers of water within a closed-loop system (Goddek and Körner, 2019). In order to understand methods through which water can be conserved, a study conducted on a recirculated aquaponic system explored multi-loop systems. The goal of the study was to determine if a recirculating aquaculture system could feasibly be decoupled from a hydroponic unit and turned into a multi-loop aquaponics system. An aquaponic sizing simulator was then created, and simulations were run with details regarding plant growth and hydroponic system needs, amongst others.
Hydroponic research indicates water circulation allows for the conservation of resources while allowing nutrients to be reintroduced (Ruf́ı-Saĺıs et al., 2020). A study from the Universitat Autonoma de Barcelona indicates that experimental conditions allowed for 40% conservation in irrigation water daily (Ruf́ı-Saĺıs et al., 2020). The study has proved that when recirculating water in urban agriculture practices, there could be between 35 and 54% retention in nutrients. While this experimental system had advantages, there are shortcomings in that additional infrastructure is required.
Another method that can be applied to water systems discussed in previous topics is biochar. Biochar has gained traction as it repurposes organic waste. It has the potential to be used in various components of a closed-loop system. Within agriculture, biochar could be applied to treat point-source and diffuse-source wastewater in a system (Li et al., 2020). The application could decrease dependency on water while providing economic benefits as well.
Irrigation is another aspect of food cultivation that is a heavy utilizer of water. When considering irrigation as an aspect of a water system, research was conducted to determine how the wastefulness of water can be mitigated (Bwambale et al., 2022). Technological advancements have allowed irrigation systems to leverage various concepts and techniques such as neural network classifications (Anguraj et al., 2021) and the internet of things (Maja and Robbins, 2018). This allows for smart irrigation systems to surface and provides an opportunity for precision and accuracy (Castro et al., 2016), which in return reduces wastefulness.
3.5. Growing media
Various systems utilize different growing media; this section will review both soil and soilless cultivation. As more strain is placed on the environment, there are advantages of integrating a soilless culture system into a closed-loop greenhouse (Gruda, 2019). While peat and rockwool are two materials available as alternatives to soil, there are additional solutions and alternatives. As an example, by repurposing raw materials and organic materials within the system, growing medium can be developed. As discussed within the topic of food waste, biochar, made of organic materials such as green waste, would possess the ability to act as a substrate for cultivation under the appropriate conditions (Dunlop et al., 2015). Converting organic material waste from the plants already within the system could also benefit nutrient availability. It is additionally possible to leverage urine, another form of waste. Human urine that is rich in nitrogen and phosphate can potentially support cultivation in soilless systems (El-Nakhel et al., 2021). New techniques, such as the utilization of human urine, as well as traditional techniques have been shown to positively support the growth of lettuce.
Soil moisture dynamics are impacted by water systems and the climate within the greenhouse. In order to gain a deeper understanding of soil moisture dynamics, and its impact on plant growth, a study utilized AQUACROP simulations (Adeyemi et al., 2018). This simulation applied Dynamic Neural Network models to predict the volumetric soil moisture. Models were generated at various locations throughout the greenhouse, to understand how a dry surface layer of soil interacts with the overall soil composition, fluid transport and temperature. Various layers of soil were observed as they influence the overall composition of the soil and influence the growth of the plant. Through the collection of several data points, it was found that heat, moisture migration and temperature strongly influence moisture transport in unsaturated soil. It was also observed that between 20 and 46% of water could be conserved with its application. As soil is influenced by other aspects of a system, such as water and nutrients, additional findings from the literature review are included amongst other topics that have been identified, including nutrient systems and water systems.
3.6. Heating and energy
There are various aspects of a greenhouse system to consider when reviewing heating and energy within a system design. Climate controls, ventilation, and lights are all internal contributors to heating and energy. Additionally, there are external conditions outside of a greenhouse that can impact and influence a closed-loop greenhouse. The objective of optimizing greenhouses is to have more favorable growing conditions.
Heating systems can be woven into a system at various locations and levels. A study conducted on a greenhouse located near Firat University in Turkey applied a ground-source heat pump heating system (Benli, 2011). Benli found that utilizing this system is advantageous in energy savings but noted the higher initial cost of such investment. Another heating system that has demonstrated efficiency is the earth-to-air heat exchanger (Hepbasli, 2013). The heating system has been assessed to have around 72% energy efficiency. The challenge with an earth-to-air heat exchanger is that there is a significant amount of infrastructure changes that need to occur. This is because it relies on transporting heat generated from power plants to greenhouse infrastructure through various pipes and subsystems. While the energy efficiency at a reference environment temperature of 0°C is around 19%, it drastically decreased to between 1.01 to 1.24% at 18°C.
Another system that has been studied within greenhouse environments is horizontal heat exchangers (Awani et al., 2017). The system's objective is to heat the glass substrate of the greenhouse using a water-air heat pump in conjunction with a ground heat exchanger. The horizontal heat exchangers leverage renewable energy in the surrounding area, in this instance, solar energy. Assessing a geothermal heat pump system, the study collected data for the operation of both a single heat pump unit and two heat pump units (Kang et al., 2014). The study concluded that if a greenhouse relies on multiple heat pumps, all pumps must function, or there will be a significant decrease in performance. This is because the number of pumps is influenced by other aspects within the system, such as water pumps.
While some of the discussed heating systems studies examine the energy requirements or efficiency, additional practices and strategies for energy should be considered. Lighting is commonly found in greenhouses and consumes energy. Roughly 30% energy savings can be obtained if there is consideration toward using sunlight integrated with artificial lights (Jiang et al., 2020). To understand how the sun could be used to save energy, a Smith predictor was used in order to achieve the balance between the various spectrums of light in unison with the sun. Since the sun can be interrupted by clouds, a study applied cloud data to determine when a system should use reserved energy resources and when it is most efficient to do so (Li et al., 2018). By integrating weather information into the system controls for an intelligent solar greenhouse, the system can use data to drive its decision-making regarding climate control in the greenhouse. This can ensure that the climate is regulated to ensure optimal growing conditions and that there is no waste of energy. It is also important to note that environmental conditions changes within a greenhouse that occur for short intervals of time are unlikely to impact the plants which are being grown (Su et al., 2017a).
4. Research gaps and opportunities
Through this bird's-eye view of previous research pertaining to closed-loop systems, it can be seen that there has been a steady increase in research (Figure 6). However, there was a limited number of publications that lent themselves to this literature review. Due to the nature of a closed-loop system and the agriculture sector, many topics that the LDA models indicated crossed paths. Implementing a change in one component of a closed-loop system often affects and influences several others. When considering the number of publications contributing to each of these topics, a gamma score was calculated, indicating the per-document-per-topic probability. As seen in Figure 7, the gamma scores among topics 1, 2, and 5 were relatively similar, and topics 3, 4, and 6 were in the range of one another. Topic seven is an outlier with a lower gamma score than all others.
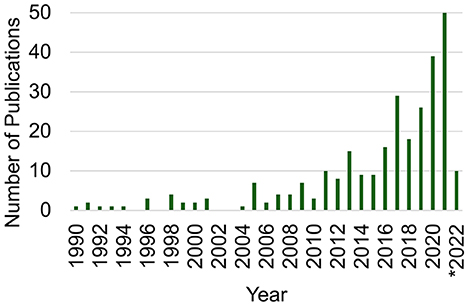
Figure 6. The number of publications on closed-loop agricultural systems increased steadily from 1990 to 2022. *The article pull occurred on February 2, 2022.
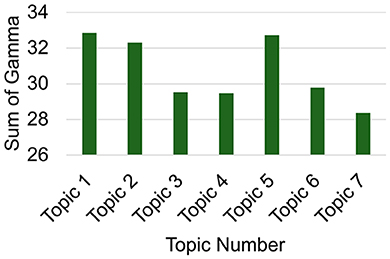
Figure 7. Per-document-per-topic summation. An indication of the number of articles contributing to each proposed topic.
Following the review of literature applicable to each of the topics pursued, several areas could benefit from additional research: connecting research and industry application, energy dependency and other critical operational requirements, criteria to select the appropriate soil or soilless growing environment, and the integration and resilience of a closed-loop system composed of many individual modules as seen in Figure 8.
The first research gap, connecting research and industry application, is very important within the food and agriculture sector. The research presented in this paper is based on modeling various concepts and frameworks, in addition to some experiments. There was limited information available on whether there was success in implementing any of the frameworks discussed throughout the literature. With the various concerns brought forward in the pieces of literature in regards to climate change, resource depletion, and human adaptation to technological advancements, further work needs to be done to understand the feasibility of applying these frameworks to real-life practice.
The second research gap is energy dependency and other critical operational requirements. While various papers addressed components within the topic of energy, there was a lack of evidence to suggest what the dependency of a functioning closed-loop system within a greenhouse would be. The usage of solar panels has been studied in addition to ways that systems can be automated to make resource allocation decisions. However, given the fact that each aspect of a closed-loop system influences and impacts one another, it's important to understand which aspects will require energy utilization and how it's possible to ensure accessibility of the resource, given that within a closed-loop system, the resource should be available, reusable and repurposable.
The third research gap is criteria to select the appropriate soil or soilless growing environment. Research is readily available to understand nutrient levels within soils and how to understand moisture within soil and fluid flow. Additionally, it is possible to review the soilless cultivation opportunities, and one can understand the demands of this type of cultivation. However, the gap indicated here refers to research being conducted concerning how three aspects of the system would interact while ensuring that the plants being grown are effectively being taken care of. Given a soil-based growing environment, as it is influenced by heat, moisture, and temperature, among others, it is feasible to conduct additional research in this space. This will allow for a better understanding of what types of growing media will optimize the closed-loop greenhouse system, which in turn would yield optimal production rates.
The fourth and final research gap refers to the integration and resilience of a closed-loop system composed of many individual modules. Given the discussion throughout the paper and how each component influences one another, it would be valuable to have further research on understanding how a fully closed-loop greenhouse system can be built. As discussed in the topic of heat and energy, having one pump stop running amongst a group of four pumps may not on its own critically impact the greenhouse. If, however, we're creating a closed-loop system where these pumps are dependent on various energy sources, and those energy sources are dependent on other resource availability and the repurposing of existing resources, then it is critical that there is experimental-based research that assesses these various aspects. The application of Internet-of-Things (Maraveas et al., 2022) and artificial intelligence (Maraveas, 2023) can potentially allow for the optimization and increased communication between various components of a closed-loop greenhouse. In addition, there are benefits concerning data collection in the system, allowing the closed-loop system to be more robust and resilient to factors such as climate change. This fourth research gap truly does present itself as the most challenging research gap as it requires infrastructure accessibility and likely numerous experimental and optimization methods; however, a thorough understanding will allow for the development of a method through which food production can be optimized and implemented in the real world. The more rapidly solutions can be applied into practice, the greater the opportunity to overcome some of the challenges that humanity is currently facing regarding food production and climate change.
5. Conclusion
Implementing dependable and sustainable food systems is more critical than ever before. The United Nations SDGs prompt society to mitigate and adapt in order to preserve the world as a collective. Utilizing a text-mining approach allowed for a data-driven approach to examining existing research. The method provided a qualitative and quantitative lens on an arguably important area of study. Topic modeling allowed for a categorical review of 287 research publications, which revealed six unique topics. Each topic focused on a component of closed-loop systems in greenhouses: models and controls, food waste, nutrient systems, water systems, growing media, and heat and energy. Various techniques have been assessed through simulations and experiments to understand the viability, feasibility, and sustainability of the six topics. The studies yielded some best practices dependent on the component and prescribed closed-loop technique. For example, the use of biochar can reduce the amount of organic waste present in the system. A second example is that nutrient ratios can be effectively used to determine a system's needs in advance, which could be a best practice for resource conservation. However, research regarding closed-loop systems in greenhouse infrastructure still keeps a narrow focus. As a result of the narrow research on only some components and not all, areas requiring more investigation and study have been summarized. While discussion and impact are broader, the application of closed-loop is limited to one to three components of a greenhouse system rather than the larger infrastructure as a whole. Furthermore, there remains a disconnect between academic research and industry implementation and application. The area of energy dependence and critical operational requirements also demand more research. Climate and resource availability has been indicated to vary between different growing media, and its strong connection with various system components lends itself to additional research. Lastly, and most challenging, is the need for detailed research that evaluates each component of a greenhouse utilizing a closed-loop system as one. The intent of this study is to highlight exciting developments occurring within this area of research while calling attention to aspects where there is an opportunity for additional research. As research on closed-loop greenhouse progresses, it will supply relief to the current food and agriculture production system.
Data availability statement
The raw data supporting the conclusions of this article will be made available by the authors, without undue reservation.
Author contributions
WE-D and BZ designed the research. MR performed research and analyzed data. MR and MH wrote the text-mining code. MR, WE-D, and BZ wrote the paper. All authors contributed to the article and approved the submitted version.
Funding
This research was supported by the Natural Sciences and Engineering Research Council of Canada (NSERC) Discovery Grants (Grant Nos. RGPIN-2019-07162 and RGPIN-2021-03983).
Acknowledgments
The authors are grateful for the support of the INTERFACE Institute of McMaster University.
Conflict of interest
The authors declare that the research was conducted in the absence of any commercial or financial relationships that could be construed as a potential conflict of interest.
Publisher's note
All claims expressed in this article are solely those of the authors and do not necessarily represent those of their affiliated organizations, or those of the publisher, the editors and the reviewers. Any product that may be evaluated in this article, or claim that may be made by its manufacturer, is not guaranteed or endorsed by the publisher.
References
Adeyemi, O., Grove, I., Peets, S., Domun, Y., and Norton, T. (2018). Dynamic neural network modelling of soil moisture content for predictive irrigation scheduling. Sensors 18, 3408. doi: 10.3390/s18103408
Ahn, T. I., Shin, J. H., and Son, J. E. (2021). Theoretical and experimental analyses of nutrient control in electrical conductivity-based nutrient recycling soilless culture system. Front. Plant Sci. 12, 841. doi: 10.3389/fpls.2021.656403
Allegue, L. D., Puyol, D., and Melero, J. A. (2020). Food waste valorization by purple phototrophic bacteria and anaerobic digestion after thermal hydrolysis. Biomass Bioener. 142, 105803. doi: 10.1016/j.biombioe.2020.105803
Andriamanohiarisoamanana, F. J., Yasui, S., Yamashiro, T., Ramanoelina, V., Ihara, I., and Umetsu, K. (2020). Anaerobic co-digestion: a sustainable approach to food processing organic waste management. J. Mater. Cycles Waste Manage. 22, 1501–1508. doi: 10.1007/s10163-020-01040-3
Anguraj, D. K., Mandhala, V. N., Bhattacharyya, D., and hoon Kim, T. (2021). Hybrid neural network classification for irrigation control in wsn based precision agriculture. J. Ambient Intell. Human. Comput. 15, 1–12. doi: 10.1007/s12652-020-02704-6
Asmussen, C. B., and Møller, C. (2020). Enabling supply chain analytics for enterprise information systems: a topic modelling literature review and future research agenda. Enterprise Inf. Syst. 14, 563–610. doi: 10.1080/17517575.2020.1734240
Awani, S., Koôli, S., Chargui, R., and Guizani, A. A. (2017). Numerical and experimental study of a closed loop for ground heat exchanger coupled with heat pump system and a solar collector for heating a glass greenhouse in north of tunisia. Int. J. Refriger. 76, 328–341. doi: 10.1016/j.ijrefrig.2017.01.030
Benli, H. (2011). Energetic performance analysis of a ground-source heat pump system with latent heat storage for a greenhouse heating. Energy Conver. Manage. 52, 581–589. doi: 10.1016/j.enconman.2010.07.033
Blei, D. M., Ng, A. Y., and Jordan, M. I. (2003). Latent dirichlet allocation. J. Mach. Learn. Res. 3, 993–1022.
Bwambale, E., Abagale, F. K., and Anornu, G. K. (2022). Smart irrigation monitoring and control strategies for improving water use efficiency in precision agriculture: A review. Agric. Water Manage. 260, 107324. doi: 10.1016/j.agwat.2021.107324
Castro, N. D., Chamorro, L. E., and Viteri, C. A. (2016). A wireless sensor network for drip irrigation control and automation. Rev. Cien. Agrícolas 33, 106–116. doi: 10.22267/rcia.163302.57
Chrouta, J., Chakchouk, W., Zaafouri, A., and Jemli, M. (2019). Modeling and control of an irrigation station process using heterogeneous cuckoo search algorithm and fuzzy logic controller. IEEE Trans. Ind. Appl. 55, 976–990. doi: 10.1109/TIA.2018.2871392
de Jesus Rubio, J., Gutierrez, G., Pacheco, J., and Perez, H. (2011). Comparison of three proposed controls to accelerate the growth of the crop. Int. J. Innov. Comput. Inf. Control 7, 4097–4114.
del Sagrado, J., Sánchez-Molina, J. A., Díaz, F. R., and Berenguel, M. (2016). Bayesian networks for greenhouse temperature control. J. Appl. Logic. 17, 25–35. doi: 10.1016/j.jal.2015.09.006
Dsouza, A., Price, G. W., Dixon, M., and Graham, T. (2021). A conceptual framework for incorporation of composting in closed-loop urban controlled environment agriculture. Sustainability 13, 2471. doi: 10.3390/su13052471
Dunlop, S. J., Arbestain, M. C., Bishop, P. A., and Wargent, J. J. (2015). Closing the loop: Use of biochar produced from tomato crop green waste as a substrate for soilless, hydroponic tomato production. HortScience 50, 1572–1581. doi: 10.21273/HORTSCI.50.10.1572
El-Nakhel, C., Geelen, D., Paepe, J. D., Clauwaert, P., Pascale, S. D., and Rouphael, Y. (2021). An appraisal of urine derivatives integrated in the nitrogen and phosphorus inputs of a lettuce soilless cultivation system. Sustainability 13, 4218. doi: 10.3390/su13084218
Goddek, S., Delaide, B., Mankasingh, U., Ragnarsdottir, K. V., Jijakli, H., and Thorarinsdottir, R. (2015). Challenges of sustainable and commercial aquaponics. Sustainability 7, 4199–4224. doi: 10.3390/su7044199
Goddek, S., and Körner, O. (2019). A fully integrated simulation model of multi-loop aquaponics: a case study for system sizing in different environments. Agric. Syst. 171, 143–154. doi: 10.1016/j.agsy.2019.01.010
Goto, E., Kurata, K., Hayashi, M., and Sase, S. (2013). Plant Production in Closed Ecosystems. Tokyo: Springer Science &Business Media.
Goumopoulos, C., O'Flynn, B., and Kameas, A. (2014). Automated zone-specific irrigation with wireless sensor/actuator network and adaptable decision support. Comput. Electr. Agric. 105, 20–33. doi: 10.1016/j.compag.2014.03.012
Griffiths, T. L., and Steyvers, M. (2004). Finding scientific topics. Proc. Nat. Acad. Sci. 101, 5228–5235. doi: 10.1073/pnas.0307752101
Gruda, N. S. (2019). Increasing sustainability of growing media constituents and stand-alone substrates in soilless culture systems. Agronomy 9, 298. doi: 10.3390/agronomy9060298
Haggag, M., Ezzeldin, M., El-Dakhakhni, W., and Hassini, E. (2019). Resilient cities critical infrastructure interdependence: a meta-research. Sustain. Resil. Infrastr. 7, 291–312. doi: 10.1080/23789689.2020.1795571
Helmstedt, K. J., Stokes-Draut, J., Larsen, A. E., and Potts, M. D. (2018). Innovating at the food, water, and energy interface. J. Environ. Manage. 209, 17–22. doi: 10.1016/j.jenvman.2017.12.026
Hepbasli, A. (2013). Low exergy modelling and performance analysis of greenhouses coupled to closed earth-to-air heat exchangers (eahes). Energy Build. 64, 224–230. doi: 10.1016/j.enbuild.2013.05.012
Jiang, J., Mohagheghi, A., and Moallem, M. (2020). Energy-efficient supplemental led lighting control for a proof-of-concept greenhouse system. IEEE Trans. Ind. Electr. 67, 3033–3042. doi: 10.1109/TIE.2019.2912762
Kang, Y. K., Ryou, Y. S., Jang, J. K., and Kim, Y. H. (2014). Analysis on heating effects of the vertical type geothermal heat pump system. J. Biosyst. Eng. 39, 69–75. doi: 10.5307/JBE.2014.39.2.069
Krupanidhi, S., Sai, N. M., Leung, H., and Kineman, J. J. (2017). The leaf as a sustainable and renewable system. Syst. Res. Behav. Sci. 34, 564–576. doi: 10.1002/sres.2487
Kusumowardani, N., Tjahjono, B., Lazell, J., Bek, D., Theodorakopoulos, N., Andrikopoulos, P., et al. (2022). A circular capability framework to address food waste and losses in the agri-food supply chain: The antecedents, principles and outcomes of circular economy. J. Bus. Res. 142, 17–31. doi: 10.1016/j.jbusres.2021.12.020
Li, L., Li, J., Wang, H., Georgieva, T., Ferentinos, K. P., Arvanitis, K. G., et al. (2018). Sustainable energy management of solar greenhouses using open weather data on macqu platform. Int. J. Agric. Biol. Eng. 11, 74–82. doi: 10.25165/j.ijabe.20181101.2713
Li, S., Chan, C. Y., Sharbatmaleki, M., Trejo, H., and Delagah, S. (2020). Engineered biochar production and its potential benefits in a closed-loop water-reuse agriculture system. Water 12, 2847. doi: 10.3390/w12102847
Lin, H., Borrion, A., da Fonseca-Zang, W. A., Zang, J. W., Leandro, W. M., and Campos, L. C. (2021). Life cycle assessment of a biogas system for cassava processing in brazil to close the loop in the water-waste-energy-food nexus. J. Clean. Prod. 299, 126861. doi: 10.1016/j.jclepro.2021.126861
Lykas, C., Giaglaras, P., and Kittas, C. (2001). Availability of iron in hydroponic nutrient solutions for rose crops. J. Hortic. Sci. Biotechnol. 76, 350–352. doi: 10.1080/14620316.2001.11511376
Maiwald, V., Vrakking, V., Zabel, P., Schubert, D., Waclavicek, R., Dorn, M., et al. (2021). From ice to space: a greenhouse design for moon or mars based on a prototype deployed in antarctica. CEAS Space J. 13, 17–37. doi: 10.1007/s12567-020-00318-4
Maja, J. M. J., and Robbins, J. (2018). Controlling irrigation in a container nursery using iot. AIMS Agric. Food 3, 205–215. doi: 10.3934/agrfood.2018.3.205
Manonmani, A., Thyagarajan, T., Elango, M., and Sutha, S. (2018). Modelling and control of greenhouse system using neural networks. Transac. Inst. Measur. Control 40, 918–929. doi: 10.1177/0142331216670235
Maraveas, C. (2023). Incorporating artificial intelligence technology in smart greenhouses: Current state of the art. Appl. Sci. 13, 14. doi: 10.3390/app13010014
Maraveas, C., Piromalis, D., Arvanitis, K., Bartzanas, T., and Loukatos, D. (2022). Applications of IoT for optimized greenhouse environment and resources management. Comput. Electr. Agric. 198, 106993. doi: 10.1016/j.compag.2022.106993
Messner, R., Johnson, H., and Richards, C. (2021). From surplus-to-waste: A study of systemic overproduction, surplus and food waste in horticultural supply chains. J. Clean. Prod. 278, 123952. doi: 10.1016/j.jclepro.2020.123952
Miner, G., Elder IV, J., Fast, A., Hill, T., Nisbet, R., and Delen, D. (2012). Practical Text Mining and Statistical Analysis for Non-Structured Text Data Applications. Oxford: Academic Press.
Mohagheghi, A., and Moallem, M. (2021). Intelligent spectrum controlled supplemental lighting for daylight harvesting. IEEE Trans. Ind. Inform. 17, 3263–3272. doi: 10.1109/TII.2020.3007614
Movilla-Pateiro, L., Mahou-Lago, X. M., Doval, M. I., and Simal-Gandara, J. (2021). Toward a sustainable metric and indicators for the goal of sustainability in agricultural and food production. Crit. Rev. Food Sci. Nutr. 61, 1108–1129. doi: 10.1080/10408398.2020.1754161
Nothacker, J., Stadelmaier, J., Siemens, W., Meerpohl, J. J., and Schmucker, C. (2022). Characteristics of registered and published systematic reviews focusing on the prevention of covid-19: a meta-research study. BMJ Open 12, e060255. doi: 10.1136/bmjopen-2021-060255
Page, M. J., McKenzie, J. E., Bossuyt, P. M., Boutron, I., Hoffmann, T. C., Mulrow, C. D., et al. (2021). The prisma 2020 statement: an updated guideline for reporting systematic reviews. BMJ 372, 105906. doi: 10.1136/bmj.n71
Payne, J. L., Bhakta, N. N., Lyons, S., Mohamed, R. A. M., Carroll, K. C., and Brewer, C. E. (2018). Potential of pyrolysis of spacecraft solid waste for water recovery and plant-growth media production. J. Anal. Appl. Pyrolysis. 135, 184–188. doi: 10.1016/j.jaap.2018.09.004
Poughon, L., Farges, B., Dussap, C. G., Godia, F., and Lasseur, C. (2009). Simulation of the melissa closed loop system as a tool to define its integration strategy. Adv. Space Res. 44, 1392–1403. doi: 10.1016/j.asr.2009.07.021
Prenger, J. J., Ling, P. P., Hansen, R. C., and Keener, H. M. (2005). Plant response-based irrigation control system in a greenhouse: system evaluation. Trans. ASAE. 48, 1175–1183. doi: 10.13031/2013.18500
R Core Team (2020). R: A Language and Environment for Statistical Computing. Vienna, Austria: R Foundation for Statistical Computing.
Rashid, M. I., and Shahzad, K. (2021). Food waste recycling for compost production and its economic and environmental assessment as circular economy indicators of solid waste management. J. Clean. Prod. 317, 128467. doi: 10.1016/j.jclepro.2021.128467
Rufí-Salís, M., Petit-Boix, A., Villalba, G., Sanjuan-Delmás, D., Parada, F., Ercilla-Montserrat, M., et al. (2020). Recirculating water and nutrients in urban agriculture: An opportunity towards environmental sustainability and water use efficiency? J. Clean. Prod. 261, 121213. doi: 10.1016/j.jclepro.2020.121213
Su, Y., Xu, L., and Goodman, E. D. (2017a). Greenhouse climate fuzzy adaptive control considering energy saving. Int. J. Control Autom. Syst. 15, 1936–1948. doi: 10.1007/s12555-016-0220-6
Su, Y., Xu, L., and Goodman, E. D. (2017b). Nearly dynamic programming nn-approximation-based optimal control for greenhouse climate: A simulation study. Opt. Control Applic. Methods 39, 638–662. doi: 10.1002/oca.2370
Su, Y., Yu, Q., and Zeng, L. (2020). Parameter self-tuning pid control for greenhouse climate control problem. IEEE Access 8, 186157–186171. doi: 10.1109/ACCESS.2020.3030416
The International Institute for Sustainable Development (2020). World population to reach 9.9 billion by 2050.
Torrellas, M., Antón, A., López, J. C., Baeza, E. J., Parra, J. P., Mu noz, P., et al. (2012). Lca of a tomato crop in a multi-tunnel greenhouse in almeria. Int. J. Life Cycle Assess. 17, 863–875. doi: 10.1007/s11367-012-0409-8
United Nations Department of Economic and Social Affairs (2022). The sustainable development goals report 2022. Technical report, United Nations.
United Nations Water (2021). Summary progress update 2021 - sdg 6 - water and sanitation for all. Technical report, United Nations, Geneva, Switzerland.
van der Velden, R., da Fonseca-Zang, W., Zang, J., Clyde-Smith, D., Leandro, W. M., Parikh, P., et al. (2022). Closed-loop organic waste management systems for family farmers in brazil. Environ. Technol. 43, 2252–2269. doi: 10.1080/09593330.2021.1871660
Williams, J. (2021). Circular cities: What are the benefits of circular development? Sustainability 13, 5725. doi: 10.3390/su13105725
Wu, F., Ghamkhar, R., Ashton, W., and Hicks, A. L. (2019). Sustainable seafood and vegetable production: aquaponics as a potential opportunity in urban areas. Integr. Environ. Assess. Manage. 15, 832–843. doi: 10.1002/ieam.4187
Yin, J., Yang, Y., Cao, H., and Zhang, Z. (2015). Greenhouse environmental monitoring and closed-loop control with crop growth model based on wireless sensors network. Trans. Inst. Measur. Control 37, 50–62. doi: 10.1177/0142331214531006
Keywords: closed-loop, agriculture, greenhouse, food, systems, topic modeling, meta-research
Citation: Ragany M, Haggag M, El-Dakhakhni W and Zhao B (2023) Closed-loop agriculture systems meta-research using text mining. Front. Sustain. Food Syst. 7:1074419. doi: 10.3389/fsufs.2023.1074419
Received: 19 October 2022; Accepted: 15 March 2023;
Published: 31 March 2023.
Edited by:
Eduardo Aguilera, Centro de Estudios e Investigación para la Gestión de Riesgos Agrarios y Medioambientales (CEIGRAM), SpainReviewed by:
Emmanuel Amagu Echiegu, University of Nigeria, Nsukka, NigeriaChrysanthos Maraveas, Agricultural University of Athens, Greece
Copyright © 2023 Ragany, Haggag, El-Dakhakhni and Zhao. This is an open-access article distributed under the terms of the Creative Commons Attribution License (CC BY). The use, distribution or reproduction in other forums is permitted, provided the original author(s) and the copyright owner(s) are credited and that the original publication in this journal is cited, in accordance with accepted academic practice. No use, distribution or reproduction is permitted which does not comply with these terms.
*Correspondence: Benzhong Zhao, cm9iaW56aGFvJiN4MDAwNDA7bWNtYXN0ZXIuY2E=