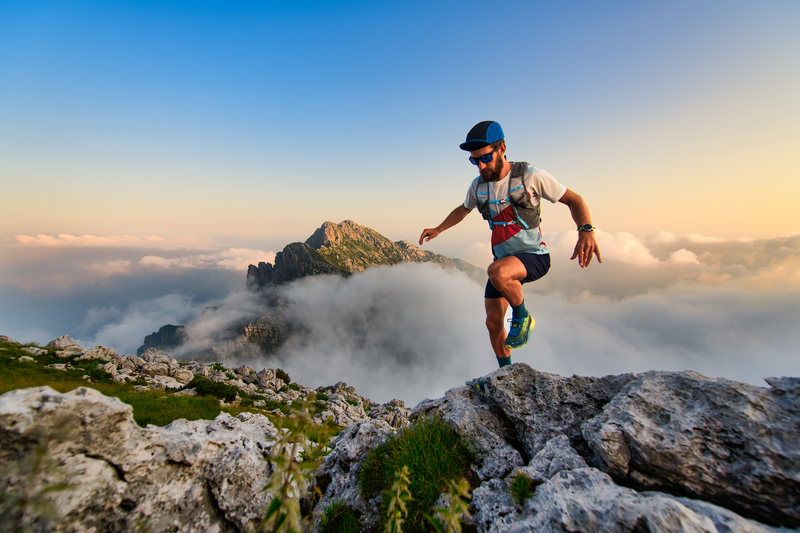
95% of researchers rate our articles as excellent or good
Learn more about the work of our research integrity team to safeguard the quality of each article we publish.
Find out more
REVIEW article
Front. Sustain. Food Syst. , 05 July 2023
Sec. Sustainable Food Processing
Volume 7 - 2023 | https://doi.org/10.3389/fsufs.2023.1040567
This article is part of the Research Topic Fermented-Based Foods As Sustainable Alternative Sources To Meet Future Demand For Protein View all 8 articles
In the era of Omics, metabolomics and metaproteomics have emerged as powerful tools for the deep analysis of the fermentation of various foods. These two are relatively new in the field of science. However, they are already proven to be crucial for a more proper understanding of microbes, their interactions with hosts and surrounding environments, metabolic activity, and intracellular biochemical reactions. Metabolomics is a quantitative and qualitative determination study of metabolites of participated microbes in the process of fermentation that helps to analyze several biochemical pathways concerning chemical changes that occur during different stages of fermentation. Hence, it provides a deep insight into the microscopic world as well as helps to significantly enhance the available nutraceuticals and probiotic characterizations in fermented foods. Evaluating the possible pathways of microbes in generating particular compounds of interest is proven to be beneficial for increasing the bioavailability of nutraceuticals and nutrients. It can be utilized to reshape the general process of fermentation in a more improved and optimized way for better yielding of nutritive components while decreasing the antinutrient components in the specific product. Metabolomics studies can be used to modify strains based on specific needs, for example, different stress-tolerant microbial strains for the optimal production of desired metabolites in stressed conditions, modulation in metabolic pathways to naturally increase the bioavailability of beneficial compounds, etc. These “Omics” methods are paving the way for a more thorough understanding of microbial life, metabolism systems, and genetic characteristics regarding protein synthesis and are undoubtedly becoming a potent weapon or a universal key in modulating fermented food products in a more advanced and sustainable approach possible. This present review aims to constitute a relevant approach for understanding the metabolic processes that can occur or are currently occurring in a given system and for implementing novel strategies focused on solving production problems or improving sustainability.
The term “Omics” is derived from the Greek word “Ome” which means “whole” or “complete.” Metabolomics is the science of critical analysis of different metabolites present in biological samples (Manchester and Anand, 2017). However, metabolomics is primarily used for the identification of low molecular weight metabolites, but it is quite successively utilized for the identification of overall metabolite flora. There are several aspects where metabolomics can be helpful due to its state-of-the-art technologies and advanced scientific methodologies. Metabolomics is an innovative scientific approach important for the identification of several different microbial metabolites in a fermentation environment. Metabolomics takes into account two different approaches, (i) Targeted and (ii) Untargeted metabolomics (Figure 1). Targeted metabolomics is applied for the quantification of metabolites of interest. Whereas, untargeted metabolomics approaches are applied for the identification and quantification of overall metabolite profiles (Pereira Braga and Adamec, 2019). Metabolomics studies can be used for the isolation and identification of bioactive compounds, which are of great interest as they are the potential compounds to be used in drug design. These new approaches help to identify several metabolites in fermented foods. The data generated from these give an insight into what can happen at the time of fermentation. Moreover, specific strains can be used to obtain desired compound profiles as these approaches have already analyzed the strain-specific metabolite formation data. State-of-the-art analytical methodologies, including untargeted metabolomics based on high-resolution mass spectrometry, are required for the profiling of these compounds in complex matrices, including plant food materials and biofluids (Koistinen et al., 2018).
Metabolomics and lipidomics have been used to investigate intracellular and extracellular metabolites as well as for the non-targeted profiling and fingerprinting of metabolites. The beneficial effects of consuming probiotics on metabolic profiles have been observed through metabolomics and lipidomics analysis (Chung et al., 2018).
Fermented foods represent a significant category of foods consumed worldwide for their unique flavors and nutritional qualities (Garcia-Gutierrez and Sayavedra, 2022). Fermentation is a unique biochemical process that relies on microbial activities. It modifies different sensorial qualities of fermented foods. Genetic characteristics of diverse microorganisms are responsible for the complete formation of metabolites in fermented foods. Enzymes excreted or produced by the microbes break down the complex compounds into simpler metabolites. Ethnic fermented foods can be defined as fermented foods, which are generally produced and consumed by ethnic communities around the world using locally available raw materials and native knowledge of them. However, in the present time of globalization, ethnic fermented foods are no longer limited to the regional level. Thus, the term now shall be used in broader means. Ethnic fermented foods are getting the acceptance of people from various parts of society. In this study, some of the ethnic foods and traditional foods are discussed under broad categories (such as wine, cheese, yogurt, and soy-based fermented foods). Yogurt and cheese are one of the most consumed dairy-based fermented foods. Lactic acid bacteria (LAB) are the pivotal group of responsible microbes, which are crucial for most dairy-based fermentations. Lipolytic, proteolytic, esterolytic, and amylolytic activity of microbes (Lactic acid bacteria, Pseudomonas, Bacillus, Achromobacter, etc.) seem to be the major contributors behind the metabolic changes in fermented foods (Medina et al., 2004; García-Cano et al., 2019). Wine's characteristic aroma and flavors are nothing but the outcome of interactions between microbes and abundant compounds present in grape juices (Carpena et al., 2020). Metabolomics studies are not only helpful in identifying metabolites and associated metabolic pathways but also beneficial for the possible detection of microbial toxins, pesticides, plastic fragments, and other types of compounds. Fermented foods are not only a great system to study microbial life but also there are several biochemical reactions, which primarily produce metabolite bouquet of the particular system. It is a wonder how microbes are being utilized for human welfare activities. In the production of fermented foods, various microbes have played significant roles ranging from the production of different enzymes to exerting probiotic activities. Therefore, metabolomics study with other Omics techniques has been efficiently utilized to monitor various alterations in the fermented foods depending on the specific modifications of starter culture, microbiota in the fermentation system, substrate, temperature, and other parameters. This approach has several advantages over other conventional processes. Identification of metabolic pathways, metabolite concentration and identification, key variables affecting metabolite formation, and biomarker selection are typically some of the major advantages. Authenticity is an important parameter that helps to discriminate fake products from original ones. There are various products, which are having high market value due to their history, place of origin, or distinct production style. However, mischievous practices persist wherein fake products are being sold under the guise of authentic ones. In some complex scenarios, metabolomic studies are used to scientifically prove the authenticity of valuable fermented foods. Wine is the best example where metabolomic study plays a crucial role in testing authenticity. Thus, metabolomics can be helpful to prove the authenticity of certain important products in such scenarios. Not only that, it is becoming a prominent tool for deep analysis of metabolites, metabolic pathways, and interspecies interactions.
It is the era of artificial intelligence, machine learning, and deep learning. Therefore, it is pivotal to look beyond conventional procedures. These new fields can undoubtedly add depth to the critical understanding and development of metabolomics. Despite being a new aspect of comprehensive analysis, the field of metabolomics is gathering much interest in the critical area of fermented foods, naturally known for their various beneficial attributes. They contain several bioactive compounds, which are generally the outcome of cellular metabolism. However, the understandings of metabolic pathways are not well-constructed. The applications of the metabolomics approach in ethnic fermented foods are of great interest in today's era.
Metabolomics is a combined tool of different analytical procedures for the evaluation of metabolic characteristics through the evaluation of metabolites. It is a great scientific approach for suggesting possible hypotheses related to metabolite generation as well as evaluating suggested hypotheses. Toxicology, pharmacology, inborn metabolic errors, and nutrition are some major fields where metabolomics is first applied (Spratlin et al., 2009). Metabolomics applies to different fields such as human disease diagnosis, biomarker determination, toxicological studies, drug discovery, disease pathogenesis, food safety, food nutrition, microbial biotechnology, enzyme discovery, and biochemical analysis (Gomez-Casati et al., 2013). It is more sensitive as it accounts for the whole evaluation of the metabolome over a certain period. Because the metabolome represents the overall metabolites of a particular bio-sample, it is easy to determine changes over time in metabolite profiles for correctly addressing the underlying activities.
The mass spectrometry-based metabolomics approach is one of the most advanced and highly sensitive techniques available. Along with the sensitivity, these methods are equally having complexity as they give numerous spectral data, which need to be analyzed further. Thus, statistical methods are employed, which help cluster the most relevant data. Nuclear Magnetic Resonance (NMR) spectroscopy is a highly advanced technique, which can determine molecular structure, phase change, conformational distinctions, solubility, and other chemical properties (Singh and Singh, 2022). NMR spectroscopy relies on the magnetic properties of atomic nuclei for the detection of the compound in which it resides. It is a non-destructive, highly sensitive analytical technique that can detect a significant amount of compounds present in the sample (Mohamed et al., 2020). There are mostly two types of NMR, 1D and 2D NMR, but several other types of NMR are also available. It is the most widely used analytical technique with high precision until mass spectrometry became available. There are numerous types of research regarding metabolomics study in foods (for example, milk, citrus fruits, and fermented foods) where NMR as an analytical technique is used (Sundekilde et al., 2013; Cicero et al., 2015; García-García et al., 2018). Mass spectrometry is a crucial analytical tool besides NMR and it is becoming more advance with the incorporation of several different cutting-edge types of machinery. It has found its way into different fields of science due to its unique specificity, high sensitivity, low instrumental errors, and capability of identifying numerous compounds at a time (Zhang and Gqamana, 2020). Although there are certainly other techniques such as Ultra High-Performance Liquid Chromatography (UHPLC) and infrared (IR) spectroscopy, mass spectroscopy-based approach has a significant edge over these types of methods, because with the help of a mass spectrophotometer coupled with gas chromatography, liquid chromatography, and capillary electrophoresis, a large number of metabolites can be identified in a single turn. While, for the analysis of volatile organic compounds in food samples, the GC/MS-based analytical technique is the most widely used (Diez-Simon et al., 2019; Putri et al., 2022). It relies on the principle of ionization and fragmentation. In the case of identification by MS, the sample compounds are ionized through different ionization processes such as electrospray ionization (ESI), atmospheric pressure chemical ionization (APCI), atmospheric pressure photoionization (APPI), and fast atom bombardment (FAB) (Zhou et al., 2012). The unique fragmentation patterns are responsible for the identification of particular compounds. For the selection of mass and time-of-flight (TOF), Quadrupole and Orbitrap are two such advanced techniques implied in the process (Cotter et al., 2020). Sometimes in the graphical plot, a metabolite can be seen to have different peaks with distinct m/z values due to the presence of different isotopes, adducts, etc. (Zhou et al., 2012). These analytical tools are now widely used in metabolomic analysis. Fourier Transform Infrared spectroscopy and Electronic Nose are some other techniques used in the metabolomics approach. In Figure 2, an overview of the different steps in the metabolomics study is given.
There are some databases, which provide significant data regarding metabolites of various matrixes. Some of the available databases for accurately identifying isolated compounds are given in Table 1.
Table 1. Some of the available metabolomics databases available for the identification of isolated metabolites.
With the improvements in separation techniques, it is now possible to identify every trace element and compound in food samples. The art of making wine is too old but to date, the different steps in wine making are poorly understood on a molecular level. It is based on alcoholic fermentation, which generally comprises simple biochemical reactions such as converting sugar compounds to ethyl alcohol and carbon dioxide by the enzymatic actions of yeasts. The production of wine includes several other reactions along with different pathways, which result in the formation of several other metabolites in wine. Therefore, a metabolomics study will be helpful to evaluate not only the metabolite profiles of wine but also to analyze toxic substances present in the same. In the beginning, basic NMR spectroscopic technology is applied primarily to identify the presence of alcohol, but later on, other less abundant compounds are also determined with the help of modified NMR techniques (Anders et al., 1976; Amargianitaki and Spyros, 2017). Not only that, there are other types of non-invasive, non-destructive, high throughput identification techniques such as High-Performance Liquid Chromatography-Mass Spectrometry (HPLC-MS), Ultra-Performance Liquid Chromatography–Quadrupole Time-of-Flight Mass Spectrometry (UPLC–QTOF MS), Gas Chromatography-Mass Spectroscopy, and Fourier Transform-Infrared Spectroscopy. They are now being widely used for the qualitative evaluation of different metabolic compounds. The fermentation process of wine consists of several distinct phases. Interestingly, each of them contributes various metabolites in the process.
The aroma profile of the wine largely depends on a metabolic compound which is a crucial section of interest. The utilization of different available chemical constituents by the participating microbes in generating aroma-producing compounds is subjected to extensive research in recent times. For example, a group of researchers has determined around 58 aroma compounds from the wine sample, which they created from juice manipulation (Pinu et al., 2014). Some of the notable compounds from their findings are esters (ethyl isobutyrate, ethyl hexanoate, ethyl butanoate, etc.), acetate esters (isoamyl acetate, β-phenylethyl acetate, etc.), fatty acids (isobutyric acid, isovaleric acid, etc.), alcohol (isoamyl alcohol, hexanol, etc.), norisoprenoids (β-ionone, β-damascenone, etc.), and terpenes. They have successfully demonstrated that aroma compounds can be modulated through pre-fermentative adjustments as the yeast utilized different substrates to form aroma compounds through various metabolic pathways. This study is an approach to the new aspects of flavor and aroma enhancement by understanding metabolomics. Esters are an important group of compounds that primarily contribute to the aroma profile of wine (Mina, 2017). Therefore, evaluating changes in the concentration of esters can provide insight into the aroma profile of the wine.
Ly et al. (2018) reported the microbiota associated with five dried alcoholic starters of Cambodia and the effect of a traditional fermentation process using a metagenomics sequencing analysis and HS-SPME-GCMS for the characterization of the aromatic and alcoholic compounds at the end of fermentation. The amylolytic and alcoholic starters used for the fermentation and production of alcoholic beverages from various sugary and starchy materials consist of microbial consortia of yeasts, molds, and LAB, which play a vital role in flavor, aroma development, final product development, and biopreservation (Sha et al., 2017, 2018, 2019).
The strain-specific wine fermentation process is another aspect of metabolomics' application as each strain has a few distinct gene sequences, which encode for some other characteristic proteins and possess some distinguishable metabolic pathways. Saccharomyces cerevisiae, primary participating yeast, has been well-studied for its metabolic activities. Metagenomics along with metabolomics can be used to understand the correlation between genomic characteristics and metabolite production and how gene modifications can significantly have affected the overall metabolic bouquet. Mendes et al. (2017) have successfully investigated the correlation of different S. cerevisiae strains collected from the various fermented product, their genetic characteristics, and their corresponding metabolite expression with an emphasis on aroma compound profile. Their work not only displays how the different gene expressions resulted in the production of different aroma compounds but also ensures that to obtain a specific metabolite profile, genetic manipulation can play a significant role in future. However, the genomics data accessible in public databases should be considered with caution because of inaccurate gene annotations due to automated annotation pipelines, thereby possibly overruling original, high-quality, well-curated gene annotations that are reported by Weckx et al. (2018). Several kinds of research have confirmed that ethyl acetate gave a fruity and floral aroma to wine. Authors have interpreted that the utilization of the Z23 strain of S. cerevisiae in wine fermentation can significantly increase the production of ethyl acetate hence elevating the fruity and floral aroma profile.
The glycolytic pathway in S. cerevisiae is the primary process of alcohol production. Not only ethyl alcohol but various other types of alcohols, for example, isoamylalcohol, phenylethanol, isobutanol, n-propanol, 2-phenyl ethanol, and methionol are also produced by S. cerevisiae in various kinds of wine (Okunowo and Osuntoki, 2007; Velić et al., 2018). Not only the yeast strain but also the grape varieties, and other parameters are undoubtedly responsible for the overall compound profile. The terroir effect is another important aspect where metabolomic study can be applied. Wine sensory attributes are directly associated with the terroir effects. Terroir can be defined as the group of parameters (cultivar, ecological system of cultivated place such as climate, topography, soil, and geography) that directly interact with the plant genetics, resulting in different compound profiles in the grape-derived wine products (van Leeuwen, 2010). Sherman et al. (2020) suggested that a non-volatile metabolome study can be more useful for accurately assessing the quality of wines from diverse origins than volatile compounds. Partial least squares regression (PLSR) study revealed that a non-volatile metabolome analysis is better than a volatile metabolome to analyze the quality of a wine because non-volatile compounds have more influence on the wine quality than the rest. Pinot noir wine's quality depends directly on the dipeptides and unsaturated fatty acids concentration but is inversely related to N-(3-13 methylbutyl) acetamide and xanthine concentration.
Biogenic amines are produced from amino acids through decarboxylation or by amination and transamination of aldehydes and ketones. Biogenic amines such as histamine, tyramine, and phenylethylamine are mildly toxic beyond the recommended level (Wöber and Wöber-Bingöl, 2010). Also, they are associated more frequently with migraine and headaches (Wöber and Wöber-Bingöl, 2010). Most wine varieties contain a minimal amount of biogenic amines. That may not be harmful, but sometimes opportunistic microbes (including spoilage or pathogenic microbes) may produce more bioactive amines in the sample through decarboxylase activity (Costantini et al., 2019). Hence, metabolomics studies can help to identify and quantify the number of bioactive amines in wine samples (Costantini et al., 2019).
Very few works have been done on untargeted metabolomics studies of wine. Rocchetti et al. (2018) have demonstrated the applicability of an untargeted metabolomics study for the evaluation of phenolic compounds in the Chardonnay wines, originating from different geographic regions (three samples from different parts of Italy, and the rest are from Israel, France, and Australia). In Supplementary Table S1, wine varieties, the analytical methods, and the metabolite identified have been outlined. Phenolic compounds are of significant interest since the introduction of analytical biochemistry because these compounds are exclusively responsible for the characteristic flavors of wine and additionally show various beneficial health-promoting attributes such as anti-hypertensive, anti-diabetic, and anti-tumor activities (Visioli et al., 2020). Their investigations show the phenolic compounds present in the diverse samples of Chardonnay wines primarily comprise hydroxycinnamic acids, coumaric acid, and caffeic acid. Campesteryl ferulate is the major hydroxycinnamic acid (polyphenolic compound) in all the samples. As per the researchers, 112 different phenolic compounds have been isolated, and among them, 29 are lignan compounds. Kaempferol 3-O-sophoroside, Quercetin 3-O-galactoside 7-O-rhamnoside, and Quercetin 3-O-rutinoside are some flavonols they have quantified. They have concluded that to identify and quantify abundant molecules in the wine samples, UHPLC-ESI and QTOF-MS are proven pivotal techniques. Another targeted metabolomic approach has been studied for a better understanding of characteristic wine color forming anthocyanin derivatives over the prolonged aging of wine, to estimate the stages of wine aging, and to determine the authenticity of vintage wine (Zhang et al., 2021). Anthocyanin, composed of an anthocyanidin backbone with sugar and acyl conjugates, is a group of polyphenolic compounds that lies in the flavonoid class. They are primarily water-soluble and display enormous anti-oxidative properties (Stommel et al., 2009; Tena et al., 2020). The color of the wine is a characteristic organoleptic property, which can be attributed based on the result of different pigments, categorically anthocyanin derivatives. Pyranoanthocyanins are the anthocyanin derivatives that are most stable in the wine aging process, and significantly pure anthocyanins actively react with yeast metabolites (acetone, pyruvic acid, acetoacetic acid, acetaldehyde, etc.) during fermentation resulting in the formation of some pivotal pyroanthocyanins, such as vitisins, methylpyranoanthocyanins (Marquez et al., 2013; Ruta and Farcasanu, 2019). The complex formation of significant pyranoanthocyanins is directly related to yeast strain specificity and their corresponding metabolite bouquet, for example, an investigation showcases how the Schizosaccharomyces pombe produced more pyruvic acid compared to the S. cerevisiae and Saccharomyces uvarum consequently resulted in more Vitisin-A production (Morata et al., 2012). According to the researchers, pinotins, a specific type of pyranoanthocyanin, are generally associated with tawny tonality as a critical function of wine aging based on the ultimate result of PLSR methods. NMR studies of different wines have presented close insight into diverse compound flora (Morata et al., 2012).
Cheese is one of the best-fermented foods consumed worldwide for its superb palatability. They are of several types depending on the geographical location, milk sources, microflora, ripening process, etc. Lactic acid metabolism, proteolysis, and lipolysis are the primary sources of volatile acid compounds in cheese (Zhang et al., 2021). Fermentation of cheese involves different microbes, and each microbe possesses some characteristic metabolic pathways. Microflora contributes differently to the metabolite profiles of various kinds of cheese; unique textures and flavors are the outcomes of the interactions between available substrates and participating microbes.
Cheesomics is the pathway to understanding cheese flavor and quality by using Next Generation Sequencing (NGS) and bioinformatics tools (Afshari et al., 2020). Through whole-metagenome shotgun sequencing, it was possible to investigate the metabolic potential of the resident microorganisms and show how variations in the microbial populations influence crucial aspects of cheese ripening, especially flavor development (Afshari et al., 2020).
Lipolysis is one of the vital biochemical processes that minutely contribute to the overall cheese aroma profile. It is a chemical process in which triacylglycerols (TAGs) are hydrolyzed into free-fatty acids and glycerol (van der Spek et al., 2012). Studies have shown that free-fatty acids are capable of stimulating taste buds (Laugerette et al., 2005). Lactic acid bacteria and Propionibacteria are the primary microbes participating in the cheese ripening process. These bacterias possess intense lipolytic activity, which interacts with the milk fat and converts them into free-fatty acids. Metabolomic studies are not only helpful in the identification of different FFAs present in the cheese sample but also helpful in monitoring the FFAs concentration concerning the ripening time. Brevibacterium linens show a significant amount of lipolytic activity in the Limburger cheese ripening process (Thierry et al., 2017).
A study revealed that cheese fermented with Lactobacillus casei-VC199 produces more free-fatty acid than Enterococcus lactis-BT161 (Battelli et al., 2019). Lipolytic activity widely varies among different strains of microbes. For example, a comparative evaluation of three Penicillium roqueforti strains, namely PS1 (PRB 6 HYP 5 D, Danis-co Deutschland GmbH, Niebull, Germany), PS2 (PR4, Chr. Hansen, Hørsholm, Denmark), and PS3 (PV LYO 10 D, Danisco Deutschland GmbH, Niebull, Germany) in the production of blue type cheese, reveals that PS3 strain produces significantly more FFAs than the others. That means the third strain possesses more lipolytic activity than the rest two. Authors assumed that α-hydroxybutyric acid concentration in cheese samples might be the outcome of lower dehydrogenase enzyme activity of lactic acid bacteria (Salvatore et al., 2015). Other than this, the authors isolate several other compounds such as lactic acid, acetic acid, valine, alanine, glycine, oxalic acid, leucine, proline, succinic acid, aspartic acid, myo-inositol, and pantothenic acid. LAB are responsible for the production of diacetyl, acetoin, acetic acid, and 2,3-butanediol through lactose metabolism, which are responsible for LAB-based flavors modulation in cheese (Bintsis, 2018). Diacetyl is an organic compound related to an intense buttery flavor (Rincon-Delgadillo et al., 2012). In Supplementary Table S2, different cheese varieties and their respective metabolite flora with respect to the microorganisms involved are given.
Lactose is predominantly found in milk samples that are further metabolized into different flavor-giving compounds by the lactic acid bacteria, for example, diacetyl, acetoin, and many more (Blaya et al., 2018). Cheese volatilome is an interesting aspect of metabolomic study because mostly all the unique flavor and aroma-giving compounds are volatile compounds produced from microbial metabolisms. Metabolomic approaches are generally used to evaluate FFAs, organic acids, and volatile compounds; these are also responsible for cheese's characteristic flavor and aroma. Volatile esters are one of the primary odorants in different cheeses (Singh et al., 2003). Hence, for a long time, the metabolomic approaches are of great interest to evaluate volatile metabolite profile and their correlation with microbial species. Some researchers identify numerous volatile organic compounds, for example, 1-hexanol, 2-methyl butanal, 2-butanone, 2-hexanone, octanal, and 2-octenal in Taga cheese during the ripening process (Criste et al., 2020). The esterase activity of lactic acid bacteria may be linked to the availability of esters in the cheese (Bertuzzi et al., 2018).
A 1H NMR-based investigation of alpine Asiago PDO cheese is helpful to discriminate Asiago cheese samples based on the cows' feeding system. Asiago cheese has Protected Designation of Origin (PDO) status by the EU; hence, its authentication is undoubtedly an important aspect (Council of the European Union, 2006). 2,3-Butanediol, choline, lysine, and tyrosine are among the crucial metabolites identified in these cheese samples, and their concentration over time helps to discriminate cheese samples (Segato et al., 2019).
Yogurt is an ancient ethnic dairy product having various beneficial effects on humans. They are of several types based on their production procedure, starter cultures or natural microflora, source of milk, geological factors, and ripening time. Generally, yogurts are rich in many nutraceuticals, for example, Gamma-aminobutyric acid (GABA), α-hydroxyisocaproic acid, and myo-inositol (Chhetri, 2019; Murgia et al., 2019; Grewal, 2020).
Probiotics are highly beneficial microbes present in large amounts in fermented foods and beverages. Probiotics with prebiotics give more productive results in the host by improving the survival of beneficial live microbes or by activating the metabolism of health-promoting bacteria in the gastrointestinal tract (Gibson and Roberfroid, 1995). Ingestion of probiotic bacteria has the potential to stabilize the immunological barrier in the gut mucosa by reducing the generation of local pro-inflammatory cytokines. Preliminary reports have shown clinical benefits in reversing some of the immunological disturbances characteristic of Crohn's disease (Wu et al., 2019).
Fermented dairy products having consortia of lactic acid bacteria such as Enterococcus durans and E. lactis have shown probiotic potential with health attributes (Ghatani and Tamang, 2017; Ghatani et al., 2022). Strain specificity is an important aspect of the metabolomic approach because each strain contributes differently to the metabolite profile of yogurt. Hence, they release different nutraceuticals in the yogurt. Probiotic strains help modify yogurt with the metabolites of interest possessing great beneficial activities. GABA (C4H9NO2) is a naturally occurring inhibitory neurotransmitter in the brain (Manchia et al., 2019). A 1H NMR-based metabolomic study showed that the production of GABA in the yogurt sample can be enhanced by the addition of simple sugars (Glucose, sucrose, and fructose) along with using Lactobacillus plantarum Taj-Apis362 strain with high glutamate decarboxylase activity (Hussin et al., 2021). In Figure 3, an illustration of their work has been given where the addition of glucose-fructose and sucrose (2% w/v) in addition to L. plantarum Taj-Apis362 strain with high glutamate decarboxylase activity enhances the production of GABA (97.65 mg/100 g compared to 25.10 mg/100 g) and increases the production of probiotic microbes. Except for this, histidine, alanine, glutamate, proline, cystine, lactic acid, and N-Acetylglucosamine were found in the particular yogurt. Another research evaluated the influence of specific probiotic strains in addition to the co-culture/co-fermentative traditional microbes on yogurt's metabolite profiles. In this study, set yogurts fermented with Lactobacillus rhamnosus GG and Bifidobacterium animalis subsp. lactis BB12 separately from the co-fermentative traditional starter cultures were evaluated for their metabolic differences based on their ripening time and refrigerated storage period (Settachaimongkon et al., 2014). They found that there are no such influences of probiotic strains on the acidity and key-aroma volatile metabolites, but the yogurt fermented with L. rhamnosus GG strain subsequently influences the non-volatile compounds during storage time. In addition to this, there are significant other volatile and non-volatile compounds found in yogurt samples, such as acetaldehyde, diacetyl, acetoin, 2,3-pentanedione, acetone, 2- butanone, acetic acid, acetate, ethanol, alanine, phenylalanine, tyrosine, and valine. Their finding suggests that there are no significant changes in different metabolite profiles, which can contribute to the yogurt's aroma and texture.
Figure 3. Graphical illustration of the research work of Hussin et al. (2021).
A metabolomic approach is used for the evaluation and differentiation of metabolite profiles of two kinds of yogurt, i.e., one fermented with Lactobacillus bulgaricus LB4 and Streptococcus thermophilus ST447 and the other one co-fermented with yogurt starters and Lacticaseibacillus paracasei IMC502 (Gu et al., 2020). It has given a deep insight into their possible bio-chemical pathways and the influence of different strains on the overall metabolic fingerprints during various times (Gu et al., 2020). The concentration of other acids such as butanoic acid and acetic acid was found to effectively increase during storage. Significantly, nonanoic, n-decanoic, and heptanoic acids are only present during the time of storage, while they are not found after the fermentation process. They identified around 44 volatiles and 196 non-volatile compounds from the yogurts. Using multivariate statistical analysis, they identified 94 differential non-volatile metabolites among the yogurts. Acetoin, acetone, 2-nonanone, 1- hexanol, hexanal, 2-ethylhexanol, 1,4-butanediol, benzaldehyde, 2-heptanone, hexanoic acid ethyl ester, and p-xylene are some of the key volatile compounds detected during various ripening period and storage time. Lacticaseibacillus paracasei IMC502 increases the production of acetone and butanoic acid (Gu et al., 2020).
Metabolomic assessment is a helpful method for the identification of differential metabolites present in yogurts produced from different milk sources. A comparative GC-MS-based untargeted metabolomic analysis of metabolite profiles of two yogurts prepared from cow and goat milk, respectively, showed significant differences in metabolite profiles at various times (Sharma et al., 2021). They identified 163 metabolites in the yogurt samples. Analysis of yogurt samples on day 14 showed a relatively high number (21) of differential metabolites, while analytical results of days 0 and 28 show 13 and 9 displaying significant differential metabolites, respectively, with the help of the Partial least squares-discriminant analysis method. Dodecanoic acid, Pimelic acid, N-Butyryl-L-homoserine lactone, α-hydroxybutyric acid, glyceryl-3-phosphorylcholine, suberic acid, adipic acid, glutaric acid, and other metabolites had been detected in yogurts samples. Sebacic acid and glucopyranosiduronic acids are 2.8- and 2.5-fold greater in goat milk-based yogurt than the cow-based on the 14th day. Authors reported that a greater concentration of di-peptides, free amino acids, medium chain fatty acids, linoleic acid, and linolenic acid are in goat milk-based yogurt. Another study on two different yogurts produced from goat and sheep milk further enhances the understanding of species specificity in metabolite production and possible biosynthesis pathways (Murgia et al., 2019). Their finding suggests that goat milk-based yogurt are significantly higher in the concentration of GABA, glutamic acid, pyroglutamic acid, β-phenyllactic acid, α-hydroxyisocaproic acid, methionine, aspartic acid, isoleucine, valine, phenylalanine, threonine, serine, methionine, lysine, glutamine/glutamic acid, proline, and uracil than the sheep milk-based yogurt (Murgia et al., 2019). While sheep milk-based yogurt had a high concentration of N-acetylglucosamine, N-acetylgalactosamine, α-hydroxyisobutyric acid, myo-inositol, xylose, ribonic acid, and glucitol than goat milk-based yogurt. In addition to this, their findings showed that there are 15 metabolites solely found in the yogurt sample but not in the respective milk and vice versa, and 9 compounds are only found in milk. Goat milk compared with sheep milk undergoes intense metabolites changes after inoculums. Overall, the findings of both kinds of research are similar, which means goat milk has a significant amount of metabolites compared to the other two yogurts. In addition, some of the identified metabolites possess beneficial activity for consumers. Therefore, the metabolomics approach to the various yogurts prepared from different milk sources can give a deep insight into the possible biochemistry of microbes and their produced metabolites through the effective utilization of different available milk components. Statistical analytical techniques such as principal component analysis (PCA), orthogonal partial least square discriminant analysis (OPLS-DA), and Partial least squares-discriminant analysis are proven effective in metabolomic studies for the detection of metabolites concerning different parameters.
Soybean-based fermented foods are one of the primary contributors to the global fermented food bouquets. Most soybean-based fermented foods typically belong to Southeast Asian countries, but it is interesting to know that each country has its own version of soybean-based fermented foods. Their production involves different processes, microbial cultures, climatic conditions, and other parameters. Doenjang (Korea), Cheonggukjang (Korea), Japanese natto, Gochujang (Korea), Tempeh (Indonesia), Sieng (Cambodia, Laos), Pepok (Myanmar), Thua nao (Thailand), Douchi (China), Sufu (China), Kinema (Nepal, Darjeeling hills, Sikkim, and South Bhutan), Hawaijar (Manipur), Tungrymbai (Meghalaya), Bekang (Mizoram), Aakhone (Nagaland), and Peruyaan (Arunachal Pradesh) are few of the fermented soy-based products found worldwide (Shin and Jeong, 2015; Tamang, 2015; Jang et al., 2021). Cheonggukjang is a fermented Korean dish that is prepared using the fermentation of boiled soybean rice straw (rice straws are kept over boiled rice and allowed to ferment) (Kim et al., 2021). While, Meju is a raw material used for preparing various Korean fermented soy-based products (doenjang, ganjang, etc.).
Most importantly, principal soy-based fermented foods contain a significant amount of protein along with other bioactive compounds (Jang et al., 2021). Different soy-based fermented foods have various biologically functional attributes such as anti-diabetic effects, antioxidant effects, anticancer effects, and anti-inflammatory and anti-hyperlipidemic effects (Chen et al., 2005; Kim et al., 2008; Seo et al., 2009; Wardhani et al., 2013; Yoon and Park, 2014). Doenjang is a fermented soy-based paste from Korea. It is prepared from meju (a dry soybean brick) in brine using naturally occurring microbes. Various metabolomics studies give an insight into the metabolite profiles of Doenjang. HS-SPME/GC-TOF-MS-based metabolomic study shows that Doenjang contained a higher amount of carboxylic acids, aldehydes, esters, furans, sulfur-containing compounds, and methoxyphenol than cheonggukjang and meju (Lee et al., 2021). While flavor compounds, including maltol, branched-chain carboxylic acids, and methoxyphenol (from lignin and pyrazine) are relatively higher in cheonggukjang than meju and doenjang (Lee et al., 2021). 7,4-dihydroxyflavone, malonyldaidzin, malonyglycitin, malonygenistin, acetylglycitin, acetylgenistin, daidzin, glycitin, daidzein, glycitein, genistein, 2,3-dihydro-2,5-dihydroxy-6- methyl-4H-pyran-4-one (DDMP)-conjugated hydroxyisoflavone, soyasapogenol, soyasaponins, non-DDMP-conjugated soyasaponins, histamine, tryptamine, and phenylethylamine are some primary metabolites found in doenjang (Lee et al., 2014, 2016b; Song et al., 2021). Among these compounds found, daidzein shows significant activity against colon cancer and the hepatitis C virus (He et al., 2021; Salama and Allam, 2021). Biogenic amines such as histamine, tryptamine, and phenylethylamine are produced significantly in doenjang and ganjang. Ganjang is a Korean traditional fermented soy sauce. The micro-flora is responsible for the production of isoflavone and soya saponin aglycones as they are most capable of producing the β-glucosidase enzyme that reacts with the glucoside form of these compounds to break down the sugar moiety associated with it (Malashree et al., 2012). Some of the primary genera of microbes found in doenjang are Meyerozyma, Hyphopichia, Debaryomyces, Aspergillus, Wickerhamomyces, Trichosporon, Tetragenococcus, Staphylococcus, Meyerozyma, Millerozyma, Tetragenococcus (Jung et al., 2016; Song et al., 2021). Some researchers stated that the availability of primary and secondary metabolites through the breakdown of peptides, amino acids, and sugar conjugates is much higher in long-fermented soy-based foods than the short-fermented soy-based food (Jayachandran and Xu, 2019). Serine, threonine, alanine, glycine, isoleucine, phenylalanine, leucine, methionine, valine, and proline are highly abundant in Doenjang and Natto. Soyasapogenol C is highly rich in Doenjang due to its long fermentation time as saponin hydrolase from Aspergillus oryzae hydrolyzes Soyasapogenols (Kwon et al., 2019). Dehydrosoyasaponin I, Soyasaponin II, Soyasaponin III, Soyasaponin IV, and Soyasaponin V are commonly found as secondary metabolites among Natto, Cheonggukjang, Doenjang, Miso, Doubanjiang, and Tianmianjiang (Kwon et al., 2019). An untargeted metabolomics study helps to evaluate the changes in metabolite profiles that occur due to the manufacturing process compared to the traditional process of Kinema production, fermented soy product from North East India. Researchers have been able to identify 55 differential metabolites among traditional and modernized Doenjang (Lee et al., 2022). Among them, Arginine, Choline, Citric acid, Cytidine, Glucaric acid, and Hypoxanthine were highly abundant in modernized doenjang, while 2-Amino-isobutyric acid, 2-Phenyl-ethylamine, Citrulline, GABA, N5-Ethyl-glutamine, Putrescine, Stachydrine, Tryptamine, and Tyramine are high in traditional doenjang. They further elaborated on the possible relationship between microbes and metabolite variations. Aspergillus oryzae, Penicillium miczynskii, and S. cerevisiae are positively correlated with the increased compound concentration in modernized Doenjang, which are mentioned earlier. Citrulline is positively correlated with Penicillium spp. They have also reported that Stachydrine, a potent bioactive compound is positively correlated with Fusarium spp., soil-derived yeast. It is an interesting research work on the utilization of untargeted metabolomics for the comprehensive evaluation of alterations in metabolite profiles of two distinct types of Doenjang.
Recent advances in metabolomics help to identify some crucial compounds such as chrysin, swainsonine, and 3-hydroxy-L-kynurenine, benzimidazole with potent bioactivities, which possess strong anticancer activity (Kharnaior and Tamang, 2022). A total of 361 metabolites have been isolated from the Kinema sample through LC-MS analysis. They have proved that Bacillus spp. is the major contributor to the formation of many metabolites. They have successfully established relations between microbes and metabolites through integrated Omics techniques.
Douchi is a fermented soy-based food product, widely consumed in Southeast Asian regions. Untargeted metabolomics analysis of Mucor racemosus Douchi gives an insight into the complex metabolite bouquet. Microorganisms produce a phytase enzyme, which hydrolyzes phytic acid to inositol and phosphate (Quan et al., 2003). Myo-inositol, putrescine, 2-butyne-1,4-diol, N(α)-dimethyl-l-histidine, and isopropyl-β-thiogalactopyranoside are the metabolites, which are increasing over time in Douchi fermentation (Li et al., 2019). PCA and Orthogonal partial least squares-discriminant analysis help discriminate the metabolite concentration in douchi over various stages of fermentation. LABs produce protease enzyme, which actively hydrolyzes soy proteins into soy amines and amino acids.
Sufu is a traditional fermented soy-based food from China. The metabolomic analysis is also helpful to distinguish these two types of Sufu, i.e., red Sufu and white Sufu, based on color and flavor in the market (Han et al., 2004; Xie et al., 2018). Metabolomic study shows that there is a significant difference in the levels of phenylalanine, isoleucine, tyrosine, methionine, valine, threonine, alanine, serine, and histidine (Tan et al., 2020). Overall concentrations of free amino acid contents are also higher in White Sufu than the Red Sufu (Han et al., 2004; Huang et al., 2018; Tan et al., 2020). Bacillus lichenformis surges lactic acid formation in Sufu and consecutively results in increased sourness (Liu et al., 2021). The Umami taste of Sufu depends on the indole and 2-pentylfuran compound that is correlated with Weissella and Lactococcus (Huang et al., 2018). Comamonas testosteroni found in Sufu produces aspartate-β-decarboxylase, which can effectively convert aspartate to alanine (Tan et al., 2020). Hypoxanthine, xanthine, betaine, choline, GABA, and uracil are some nitrogen compounds found in Sufu samples by 1H NMR and HS-SPME-GC-MS (Huang et al., 2018). The concentrations of Choline and uracil increase significantly over the fermentation period and the amount of GABA surges after 60 days of ripening. Huang et al. (2018) concluded that flavor production in Sufu mostly correlated with Lactococcus, Pseudomonas, and Acinetobacter and also state that around 72 volatile compounds (15 alcohols, seven phenols, six acids, 17 esters, 17 aldo-ketones, and 10 heterocyclic compounds) have been identified. For the identification of harmful metabolites, metabolomic analysis can be a helpful tool. Analysis of fermented foods in different periods can detect any contamination through the identification of toxins and taking appropriate safety measures. In addition to this cross-species, interactions and symbiotic relationships between species are now well understood with the help of different Omic techniques. Tempe is another type of fermented soy-based food subjected to metabolomic analysis, while PCA and OPLS-based statistical methods are implied. A GC/MS-based analysis identifies 81 metabolites in Tempe. Daidzein, genistein, putrescine, 2-aminoadipic acid, 3-hydroxybutyric acid, gluconic acid, n-acetyl glucosamine, aspartic acid, proline, saccharopine, lanthionine, and lysine are some common metabolites in Tempe (Kadar et al., 2018; Dahlan et al., 2022). Metabolomics analysis showed a significant difference in the metabolite profile of controlled Tempe and Tempe soaked in vinegar (Dahlan et al., 2022). Tempe soaked in vinegar significantly increased N-α-acetylornithine, daidzein, and genistein levels (Dahlan et al., 2022). Vinegar significantly affected the production of the overall metabolites in Tempe, which primarily contributed to the differential sensory attributes, resulting in different metabolite profiles. Based on the finding of Kadar et al. (2020), daidzein, glyceric acid, 4-aminobutyric acid, fumaric acid, glutamic acid, and tryptophan are characteristic compounds crucial for the distinction of Tempe produced from different soybeans (Kadar et al., 2020). Phytic acids, found in soy-based fermented foods are having some enzyme inhibitory activity and additionally affect the bioavailability of minerals in the body (Samtiya et al., 2020). To quantify the amount of phytic acid present in the sample, the metabolomics approach can be the best tool to consider.
Some fermented foods are not widely consumed around the globe and are thus subjected to less metabolomics analysis. For example, in China, Crab paste is consumed, which is a fermented food. Traditionally, fermented crab paste is produced from fresh swimming crab, Portunus trituberculatu. As the production does not involve any sterilization step, there may be a possibility of contamination by harmful microbes. A 1H NMR-multivariate statistical analysis-based metabolomics approach helps to understand different aspects of the fermentation of Crab paste (Chen et al., 2016). Their findings suggest that lactate formation is inhibited while the concentration of two organic acids (acetate and formate) is increased. It implies that the LABs are not well-grown in the paste, thus the production of lactate halted while the other two compound-forming microbes grow. Along with that, researchers have evaluated concentration changes of metabolites and the formation of new metabolites through the various metabolic and enzymatic activities of abundant microbes in the crab paste. During fermentation, enzymatic degradation of Trimethylamine-N-oxide (TMAO) into trimethylamine (TMA) occurs, which is an essential change. The concentration of glutamate, sucrose, formate, acetate, TMA, and hypoxanthine gradually increases over time in the fermentation system. They have suggested that trimethylamine (TMA) concentration can be an index parameter for the freshness of crab paste (Chen et al., 2016). Fermented fish sauce is emerging as a popular side dish in Southeast Asian countries such as Vietnam, Indonesia, South Korea, China, and Cambodia (Muzaddadi et al., 2016). Chinese fish sauce, namely, fermented Mandarin fish (Siniperca chuatsi), was studied by Wang et al. (2022) through UHPLC-QE-MS/MS and their finding suggests that L-palmitoylcarnitine, heptadecanoyl carnitine, acetic acid, butyric acid, glutamine, valyl-valine, leucyl-arginine, lysyl-leucine, threoninyl-leucine, lysyl-valine, valyl-serine, adipic acid, 2-hydroxymyristic acid, and valeric acid varied heavily during the fermentation period. They have also suggested 28 key metabolic pathways and among these, most of them are regulating amino acids in the fermentation process (Chen et al., 2016). The microbial metabolic pathway regulates glutamic acid, responsible for the freshness flavor of mandarin fish. There is a major interest in location-based differentiation in metabolite profiles of fermented fish pastes. Ting is sorghum-based African fermented food mainly consumed during festivities, which has its characteristic bitter taste (Sekwati-Monang, 2011). During its fermentation cyclic compounds, a sugar derivative and fatty acid methyl ester were reduced over the fermentation period and overall concentrations of fatty acids increased because of the lipolytic activity of Lactobacillus fermentum FUA 3165 and L. fermentum FUA 3321 (Adebo et al., 2019). It was concluded that inherent metabolic compositions of raw sorghum have a deep influence on the changes in fermented Ting. Trans-13-octadecenoic acid, 9,12-octadecadienoic acid, ethanone, 1-(2-hydroxyl-5-methylphenyl), and many more are some of the metabolites identified in Ting (Adebo et al., 2019). It is suggested that Ting fermented with L. fermentum FUA 3321 are more preferable as it produces relevant metabolites during fermentation. Table 2 includes some other fermented foods and beverages, and their metabolite profiles, along with the microorganisms involved. Salchichón is a meat-based dry fermented sausage usually consumed in Spain (Martín et al., 2021). The metabolomics approach identifies different metabolites in the Salchichón sample such as amino acids, peptides and analogs, carbohydrates, organic acids and derivatives, nucleosides, nucleotides and analogs, fatty acids, acetone, phosphocholine, and inosine 5'-phosphate (García-García et al., 2018).
Kombucha is a traditional fermented tea made from sweetened (5–8%) black or green tea (Gaggı̀a et al., 2018). It is mostly produced through the interactive actions of acetic acid bacteria and osmophilic yeasts (Gaggı̀a et al., 2018). Thus, it contains a diverse range of metabolites, some of which have different bioactivities. Metabolomic analysis of kombucha identifies isovaleric, succinic, malic, citric acid, propionic acid, 2,3 butanediol, glycerol, 4-hydroxyphenylacetic acid, cholate, and many more (Villarreal Soto et al., 2020). They identified that Actinobacteria and Proteobacteria were dominant in kombucha samples.
In the era of scientific advancement, genetic engineering is easy to handle. One of the greatest discoveries of recent times in the field of life sciences is the CRISPR system, which is a great platform to alter genetic constituents in a particular microbe. As it is already known that metabolomics gives the essential data regarding the metabolite profiles, it will be a breakthrough if the CRISPR system has been applied for the genetic engineering of suitable microbes for the enhancement of particular characteristics through alterations in the production of compounds. Although some research has been conducted in this field, there are still many unexplored aspects that require further investigation.
In Figure 4, an approach for the production of fermented food products with added nutritional qualities and desired characteristics is illustrated to show how metabolomics tools can be used for modifications of different fermented products. The metabolomics approach helps to find out changes in nutritional quality through the identification of different metabolites. They found a decrease in carbohydrate metabolites after fermentation, which implied that the glycolysis process has become more dominant (Mok et al., 2019). Soymilk normally consists of different important classes of compounds. When soymilk is fermented with Bacillus subtilis BSNK-5, it produces several different metabolites including differential metabolites. Metabolomics study proves that the relative abundance of metabolites and beneficial metabolites is higher in unfermented soymilk than the fermented soymilk (Gao et al., 2020). According to the authors, flavor-related substances (acetate, isovalerate, and 2-methylbutyrate), nutrient-related substances (12 free amino acids), and functional substances (taurine, GABA, and genistein) are primarily increased through fermentation. These are a few examples of metabolomics in the development of desired characteristics in fermented food whether it is ethnic or general fermented foods. Like this, other ethnic fermented food can be taken into consideration for the improvement of desired characteristics through the help of metabolomics.
Figure 4. Pictorial representation of a possible procedure for metabolomics-based value-added fermented food product development.
Gluten-free Hemp-enriched sourdough bread is a perfect example of how metabolomics can be helpful in value-added product development. There is a considerable increase in different nutritional qualities along with the addition of volatile organic compounds in Hemp seed flour, when fermented with lactic acid bacteria (L. plantarum 98a, Lb. sanfrancisciensis Bb12, and S. cerevisiae LBS) (Nissen et al., 2020). SPME-GC-MS-based metabolomics approach is used to analyze metabolites in the Gluten-Free Hemp-Enriched Sourdough Bread which shows a significant increase in different metabolites such as 2-butanone-3-hydroxy, acetic acid, ethanol, and 1,4-butanediol. The metabolomic study has given a possible way for future advancement such as the enhancement of brown milk's sensory characteristics through fermentation with S. thermophilus (Li et al., 2020). Interesting work has been done on the usage of fermented date fruits for the production of Dodol, an Asian confectionary. This work shows how fermented dates fruits (Khastawi) can be used for the production of bakery products and confectionaries (Muhialdin et al., 2021). In the case of Dodol, the addition of fermented date not only extends the shelf life of products but also increases nutritional qualities and bioactive compounds. Similarly, soy products comprise different odorants and many of these are not preferable to many consumers. Researchers tried to solve the issue through the help of fermentation by GC-MS (Nedele et al., 2022). Fermentation of soy drink and soy protein isolate with Lycoperdon pyriforme reduces different odorants primarily belonging to aldehyde at significant amounts. The microbes reduce aldehydes, primarily responsible for off-odor, and two pathways are postulated regarding the reduction of aldehydes, i.e., one is the reduction of a saturated aldehyde into alcohols and another is di-unsaturated aldehydes, which form non-volatile compounds thus conferring no odorant role (Nedele et al., 2022). Therefore, metabolomics can be a valuable approach for the development of value-added ethnic fermented foods and beverages.
Metabolomics study is a helpful tool for quality testing and authenticity checking. Some researchers have successfully established the potential usability of GC-FID-MS-based metabolomics for quality analysis of plum brandy (Ivanović et al., 2021). GC-FID-MS is capable of identifying discriminatory metabolites in plum brand samples of different origins. They have suggested that 1, 1-Diethoxyhexane and 1, 1-diethoxybutane are discriminatory metabolites for cultivar PoŽegača (PZ) and Crvena ranka (CR) (P. domestica L.) cultivar and cultivar PoŽegača (PZ), respectively. These are autochthonous plum cultivars from Serbia. Factoextra and Ropls are two R packages, which are used for identifying markers for authenticity testing in the selected Greek wines (Tzachristas et al., 2021). There are 17 metabolic compounds namely, Catechin, L-Proline, Coutaric acid, and Quercetin 3-glucuronide, which were found to be responsible for discrimination between wine samples (produced from Agiorgitiko and Xinomavro grape varieties). Therefore, these methodologies along with advanced statistical and computational approaches can be successively used for fermented foods and beverages authentication.
Untargeted metabolomics is helpful for the identification of anti-nutrients in fermented or pre-fermented foods. Levilactobacillus brevis used for the fermentation of leaves of cassava significantly decreases Cyanide and phytic acid (common anti-nutrient) levels (Okoth et al., 2022). Rice bran fermentation with Bifidobacterium longum, Limosilactobacillus fermentum, L. paracasei, L. rhamnosus, Escherichia coli, and Saccharomyces boulardii significantly reduces the anti-nutrient component Phytase (Seyoum et al., 2022). LABs are more capable of reducing anti-nutrients than random spontaneous fermentation (Ogodo et al., 2019). In addition to reducing anti-nutritional factor phytate, fermentation can also reduce α-Galactosides, tannins, and raffinose, which can be measured and investigated further through the help of metabolomics (Diouf et al., 2019). Metabolomics studies based on NMR or mass spectrometry can be utilized to monitor changes in anti-nutrient concentration in tempeh with respect to various fermentable microbes. Co-fermentation of Tempeh with Rhizopus oligosporus RT-3 and Actinomucor elegans DCY-1 successively degrades the anti-nutritional factors. Their study elucidates that carbohydrate-based anti-nutritional factors such as raffinose and stachyose are reduced by the enzymatic activity of the microbes involved (Huang et al., 2019). β-Conglycinin and glycinin are known protein-based allergens that are reduced by fermentation with R. oligosporus and A. elegans.
Metabolites are of several types depending on their chemical classification and most of them do not necessarily possess bioactivities. The metabolomic study will not only be helpful for the characterization of compounds but will be beneficial for further modification. 1H NMR metabolomics study helps to identify bioactive metabolites in fermented date fruits. Propylene glycol, lactic acid, acetic acid, acetoin, and γ-Aminobutyric acid are some of the bioactive compounds identified in fermented dates (Muhialdin et al., 2021). Homovanillin, 6, 7-Benzocoumarin, Gentisyl alcohol, and 2, 5-Dihydroxyanisol were higher in unhopped beer when fermented with probiotic strains L. paracasei Lpc-37 and S. cerevisiae CNCM I-3856 (Loh et al., 2021). They have elaborated on the formation of bioactive compounds such as indole and indoleacrylic acid. A recent trend in the food industry is to develop value-added and probiotic foods. So, a recent approach has been made to produce new fermented coffee brews. Researchers analyzed fermented coffee brew for potential bioactive compounds by an untargeted metabolomics approach. Lacticaseibacillus rhamnosus GG and S. boulardii CNCM-I745 are used for the co-fermentation of coffee brews. They have identified different postbiotics through a metabolomics approach. Saccharomyces boulardii CNCM-I745 produces postbiotic metabolites such as 2-isopropylmalic acid and hydroxydodecanoic acid while L. rhamnosus GG produces indole-3-lactate, p-hydroxyphenyllactate, 3-phenyllactate, and hydroxydodecanoic acid. These compounds are having several different bioactivities (Chan et al., 2021). They have also established the potentiality of LC-QTOF-based untargeted metabolomics to analyze bioactive metabolites in the fermented coffee brew.
The metabolomics approach along with metagenomics gives vital information regarding the microbe-metabolite relationship. The metabolomics approach helps to look deep into the synergistic effects of LAB and yeast in an unhopped wine fermentation system (Loh et al., 2021). Synergistic relationships among them lead to the formation of (S)-(–)-2-hydroxyisocaproic acid, phenyllactic acid, hydroxyphenyllactic acid, and indolelactic acid. Water kefir grains consist of different microbes that are often used as a natural starter culture. As it has a large diversity of microbes, distinct metabolites are formed into the fermented product through different metabolic pathways and enzymatic degradations (Azi et al., 2021). Fermentation with water kefir grains' microbiota increased the availability of N-(1- deoxy-1-fructosyl) leucine, N-acetyl-L-phenylalanine, and acetyl-DL-leucine while reducing the concentration of gamma-glutamyltyrosine, gamma-glutamylphenylalanine, and Lalloisoleucine. The author suggested that the increased amount of peptides is due to the proteolytic activity of microbial enzymes. Saccharomyces cerevisiae, Pichia membranifaciens, Dekkera bruxellensis, Mortierella longigemmata, Candida kruisii, and Saitozyma podzolica are most abundant in the particular (Azi et al., 2021). There are numerous other examples, which are already mentioned earlier in this article. Thus, metabolomics is no doubt a capable tool for the deep understanding of metabolite profiles.
The applications of metabolomics study are extensive and can be observed across various fields. One such application can be the use of untargeted metabolomics in determining the bioaccessibility of metabolites from fermented foods which has been already discussed earlier. The oats-based fermented beverage is subjected to untargeted metabolomics (Bocchi et al., 2021). Avenic acid B, Avenic acid A, L-Serine, L-Methionine, Nonanal, gamma-muurolene, 2-Oxopentanedioic acid, Isoorientin, 10-Hydroxymyristic acid methyl ester, beta-cyclocitral, 1, 26-Hexacosanediol diferulate, and Avenalumin III are some of the compounds which are upregulated in the fermented beverage. OPLS-DA-based statistical approach discriminates the differential metabolites. The digestion of metabolites in the gut increases the release of phenolic compounds; therefore, the fermentation of oat increases the bioaccessibility of phenolic compounds. Moreover, fermentation does not change the oligosaccharide profiles that are beneficial for probiotic strains in the gut environment and potentially act as a prebiotic. Hence, metabolomics can be used to investigate the bioavailability of different metabolites, and based on the data, significant alteration can be done in the fermentation procedure.
Tran et al. (2022) showed the interactions between yeasts (B. bruxellensis and Hanseniaspora valbyensis) and acetic acid bacteria (Acetobacter indonesiensis), and they concluded that acetic acid bacteria and yeasts exhibit a mutualistic relationship by, respectively, consuming hydroxystearate from yeasts and dehydroquinate from A. indonesiensis. They are also able to highlight mutualistic relationships among them. Like this, there are several possibilities for understanding possible relationships between different microbes in a fermentation system, which can help increase the product's desired characteristics along with value addition. Thus, it helps to analyze interactions between various microbes in a fermentation system.
Fermented oat beverages produced various unique compounds when subjected to digestion. Another research has established a predict-verify strategy (Xiang et al., 2020). The strategy was used to analyze different characteristic alterations during the fermentation of turmeric with Monascus purpureus and Eurotium cristatum (Xiang et al., 2020). Researchers tried to evaluate and compare the bioavailability of curcuminoids in fermented turmeric samples. Predictive analytical software is used for the screening of possible metabolites. They have successfully identified approximately 115 curcuminoids in fermented and crude turmeric samples, among which 31 metabolites are uniquely present in fermented turmeric. They have suggested that the breakdown of larger curcuminoids into smaller curcuminoids may increase the bioavailability of these compounds, as these compounds are more water-soluble. Researchers have successfully demonstrated that the newly developed predict-verify strategy based on targeted metabolomics is highly reliable and can be further used in the targeted analysis for studying different predictable metabolites in various fermented foods (Xiang et al., 2020).
To convert waste materials into commercially important products, metabolomics is a helpful analytical process. An example of it is the valorization of CTC tea waste through kombucha production and the analysis of metabolomes through GC-MS-based metabolomics (Majumder et al., 2022). Desulphosinigrin, 1,3-propanediol, and 2-hydroxy-gamma-butyrolactone are some of the bioactive compounds found in waste tea kombucha. In addition to these, there are various other applications of metabolomics.
Artificial intelligence (AI) is a growing tool for its immense advantages over conventional methods. AI has tremendous capabilities such as data visualization, data interpretation, system automation, deep searching, and data predictions. Data obtained from metabolomics studies can be further utilized for the establishment of different relations between microbes (their genomic data, biochemical pathways, and metabolic pathways) and metabolites with the help of AI. It can pre-assume the concentration of the possible metabolites based on different metabolomics data. It can be valuable for the identification of probable microbes involved. Sufficient data from metabolomics and metagenomics analysis can be synchronized to further elaborate the relationship between them in fermented foods and beverages. Figure 5 graphically illustrates the uses of AI in fermented food metabolomics. Machine learning is a system, which can be employed for the best possible isolation techniques along with complex statistical operations.
For the extraction of metabolites through solvent-based methods, if the various literature data is already present, AI and machine learning systems can suggest the best possible protocol to look into depending on different parameters. Machine learning tools are tremendous in terms of their usability. It can undoubtedly help organize different metabolite databases so that when necessary, they can be employed for diverse purposes, such as in comparison of the identical type of fermented food (having differences in various processes or parameters), in defining the relationship between microbes and substrates, in optimization of data from varied databases for value-added product development, and many more. AI and machine learning will be proven as a breakthrough in combination with metabolomics. Machine learning is a helpful tool for the validation of experimental procedures also. MelonPan is an example of computational method-based software for predicting metabolite composition. MSHub + EI-GNPS, AlpsNMR, CSMDB. SUMMER JS-MS 2.0, and rawR built on various programming languages such as R, C++, Java, JavaScript, and HTML are analytical tools for LC-MS-based metabolomics data. Metpropagate is another example of an analytical tool for untargeted LC-MS purely based on some of the most crucial programming languages such as R and Python (Graham Linck et al., 2020). There are some Comprehensive R Archive Network (CRAN) packages such as Lilikoi, Omu, Wilson, and MetaClean, which are helpful for metabolomics research (Misra, 2021). Metagenomics reveals entire microbial communities of fermented foods and alcoholic beverages by using PCR-DGGE and the Next Generation Sequencing approach (Sha et al., 2016, 2017, 2018, 2019).
Omu is an interesting R package, which can analyze large metabolomics datasets rapidly and can also perform various statistical analyses. MetaClean is a CRAN package helpful for eliminating false peaks in LC-MS spectral datasets and automated spectral data corrections (Chetnik et al., 2020).
Machine learning algorithms such as Random Forest (RF) and Support Vector Machine (SVM) can predict the wine aging stage through chromatic features and anthocyanin derivatives, which can be further utilized for future usage (Zhang et al., 2021). Another example of the usage of machine learning is the application of a Back propagation artificial neural network (BP-ANN) in the identification of fermentation stages of golden Pomfret (Trachinotus ovatus) (Chen et al., 2021). The authors also concluded that the program is successfully used for evaluating the fermentation stage, which can be helpful for the development of a better strategy for fermentation. For untargeted metabolomics, the spectral data are large and overlapping, which must be optimized for the best results. A deep neural network-based tool is a pivotal approach for spectral-peaks filtering.
The data from metabolomics and metagenomics are massive in number and highly complex. AI and machine learning programs are the primary tools, which are essential for data interpretation and reduction of data errors in metabolomics datasets. It is necessary to build separate databases to generate data from metabolomics studies for better understanding and organization for future purposes. Python, C++, Structured Query Language, and many more are helpful languages for creating a database. Machine learning algorithms, when employed with the database system will be an advancement in this field. Statistical analysis when aligned with AI and machine learning can give significant depth into the insight of microbial lives. Kyoto Encyclopedia of Genes and Genomes (KEGG), Human Metabolome Database (HMDB), Yeast Metabolome Database (YMDB), and METLIN tandem mass spectrometry (MS/MS) are some of the primary databases extensively used, but there is a need of more emphasis on pure sub-database building and inter-linking among them.
Databases play a vital role in biological and food sciences. These archives serve to protect the biological data, whether it may be genetic materials, proteins, whole genomes, etc., (Figure 2). Databases can also be referred to as conservation centers where information is properly stored and easily shared among researchers. Zhulin (2015) reviewed different databases important to microbiologists. Microbionet is a database developed as an approach to rapidly explore and analyze data from food microbial communities. The database provides an interactive web-based visualization that displays a visual exploration of the relationships between Operational Taxonomic Units (OTUs) and samples, and the identification of core- and sample-specific bacterial communities (Parente et al., 2016).
Fermentation has long been used to preserve and enhance the shelf life, flavor, texture, and functional properties of foods. Fermentation has been used to increase the bioavailability of vitamins and minerals as well as to modify its flavor, improve its stability, and even create new food products. Metabolomics is creating promising new avenues in the field of fermentation. The fermentation process can be modified through a comprehensive understanding of metabolomics. There are several ample scopes for the potential application of metabolomics study in fermentation. While metabolomics provides an outlook into the diverse flora of metabolites, transcriptomics and metagenomics studies similarly unveil the potential gene sequence responsible for the production of particular metabolites. Hence, by seamlessly combining the results, genome editing can be done to better optimize the product with suitable characteristics. Metabolomics, a new aspect of biological sciences has a long way to go. Undoubtedly, it is proven the best approach to rely on in many scenarios, such as to identify metabolic pathways, biochemical interactions, and discriminatory metabolite signatures or to determine the safety aspects of fermented foods. The combined approach of metabolomics, metagenomics, and metaproteomics gives a clear overall view of possible events in the fermentation process. Certainly, statistical tools are helpful in clustering and filtering the raw data available from LC-MS/GC-MS-based studies regarding metabolites. Hence, PCA and OPLS-DA are well-established statistical methods for metabolomics analysis. There is a need for a deep understanding of the relationship between the microorganisms involved and their corresponding metabolites. Although several kinds of research have been carried out in this regard, only a few have critically addressed the general relationship between metabolites produced and the microorganism involved. Metabolomics will be the prime field in the upcoming years in generating crucial data related to microbial life through accessing the metabolite profiles. There are numerous fermented foods around the world. An integrated metabolomics approach will certainly work as a wonder for a proper understanding of the overall metabolomics of these foods. There are a vast number of fermented foods and beverages in the world, so it will be beneficial to integrate relevant “Omics” techniques for deep evaluation. Hence, metabolomics study not only helps to accurately evaluate metabolic fingerprinting but also is equally helpful in ensuring the optimum safety aspects of fermented foods. “Metabolomics” as a new pioneer in the field has several possibilities. It helps to dive deep into complex matrices of fermentation. Metabolomics with AI and machine learning will alter the present perspective of how we analyze and understand fermentation dramatically. Metatranscriptomics allows identifications and quantifications of the transcripts expressed by the microbiome. It represents an “Omics” discipline with immense potential because it enables researchers to precisely determine the profile and changes in gene expression typically occurring in a microbial community in response to environmental (experimental) variations.
KG was involved in developing the hypothesis, drafting manuscript, and finalizing manuscript. SSa was involved writing and drafting the manuscript. SSh was involved in developing hypothesis and finalizing manuscript. All authors contributed to the article and approved the submitted version.
All authors very much grateful to the Department of Food Technology, University of North Bengal for providing the facilities and opportunity to complete this review work.
The authors declare that the research was conducted in the absence of any commercial or financial relationships that could be construed as a potential conflict of interest.
All claims expressed in this article are solely those of the authors and do not necessarily represent those of their affiliated organizations, or those of the publisher, the editors and the reviewers. Any product that may be evaluated in this article, or claim that may be made by its manufacturer, is not guaranteed or endorsed by the publisher.
The Supplementary Material for this article can be found online at: https://www.frontiersin.org/articles/10.3389/fsufs.2023.1040567/full#supplementary-material
Adebo, O. A., Kayitesi, E., Tugizimana, F., and Njobeh, P. B. (2019). Differential metabolic signatures in naturally and lactic acid bacteria (LAB) fermented ting (a Southern African food) with different tannin content, as revealed by gas chromatography mass spectrometry (GC–MS)-based metabolomics. Food Res. Int. 121, 326–335. doi: 10.1016/j.foodres.2019.03.050
Afshari, R., Pillidge, C. J., Read, E., Rochfort, S., Dias, D. A., Osborn, A. M., et al. (2020). New insights into cheddar cheese microbiota-metabolome relationships revealed by integrative analysis of multi-omics data. Sci. Rep. 10, 3164. doi: 10.1038/s41598-020-59617-9
Amargianitaki, M., and Spyros, A. (2017). NMR-based metabolomics in wine quality control and authentication. Chem Biol. Technol. Agric. 4, 9. doi: 10.1186/s40538-017-0092-x
Anders, U., Tittgemeier, F., and Hailer, G. (1976). H-NMR determination of ethanol in wine, wine-like beverages and spirits. Z. Lebensm. Unters. Forch. 162, 21–24. doi: 10.1007/BF01104356
Azi, F., Tu, C., Meng, L., Zhiyu, L., Cherinet, M. T., Ahmadullah, Z., et al. (2021). Metabolite dynamics and phytochemistry of a soy whey-based beverage bio-transformed by water kefir consortium. Food Chem. 342, 128225. doi: 10.1016/j.foodchem.2020.128225
Battelli, G., Scano, P., Albano, C., Cagliani, L. R., Brasca, M., Consonni, R., et al. (2019). Modifications of the volatile and nonvolatile metabolome of goat cheese due to adjunct of non-starter lactic acid bacteria. LWT 116, 108576. doi: 10.1016/j.lwt.2019.108576
Bertuzzi, A. S., McSweeney, P. L. H., Rea, M. C., and Kilcawley, K. N. (2018). Detection of volatile compounds of cheese and their contribution to the flavor profile of surface-ripened cheese. Compr. Rev. Food Sci. Food Saf. 17, 371–390. doi: 10.1111/1541-4337.12332
Bintsis, T. (2018). Lactic acid bacteria as starter cultures: an update in their metabolism and genetics. AIMS Microbiol. 4, 665–684. doi: 10.3934/microbiol.2018.4.665
Blaya, J., Barzideh, Z., and LaPointe, G. (2018). Symposium review: interaction of starter cultures and nonstarter lactic acid bacteria in the cheese environment1. J. Dairy Sci. 101, 3611–3629. doi: 10.3168/jds.2017-13345
Bocchi, S. Rocchetti G., Elli, M, Lucini, L, Lim, C-Y, and Morelli, L. (2021). The combined effect of fermentation of lactic acid bacteria and in vitro digestion on metabolomic and oligosaccharide profile of oat beverage. Food Res. Int. 142, 110216. doi: 10.1016/j.foodres.2021.110216
Carpena, M., Fraga-Corral, M., Otero, P., Nogueira, R. A., Garcia-Oliveira, P., Prieto, M. A., et al. (2020). Secondary aroma: influence of wine microorganisms in their aroma profile. Foods 10, 51. doi: 10.3390/foods10010051
Chan, M. Z. A., Lau, H., Lim, S. Y., Li, S. F. Y., and Liu, S.-Q. (2021). Untargeted LC-QTOF-MS/MS based metabolomics approach for revealing bioactive components in probiotic fermented coffee brews. Food Res. Int. 149, 110656. doi: 10.1016/j.foodres.2021.110656
Chen, D., Ye, Y., Chen, J., and Yan, X. (2016). Evolution of metabolomics profile of crab paste during fermentation. Food Chem. 192, 886–892. doi: 10.1016/j.foodchem.2015.07.098
Chen, Q., Wang, Y., Wu, Y., Li, C., Li, L., Yang, X., et al. (2021). Investigation of fermentation-induced changes in the volatile compounds of Trachinotus ovatus (meixiangyu) based on molecular sensory and interpretable machine-learning techniques: comparison of different fermentation stages. Food Res. Int. 150, 110739. doi: 10.1016/j.foodres.2021.110739
Chen, Y. C., Sugiyama, Y., Abe, N., Kuruto-Niwa, R., Nozawa, R., Hirota, A., et al. (2005). DPPH radical-scavenging compounds from dou-chi, a soybean fermented food. Biosci. Biotechnol. Biochem. 69, 999–1006. doi: 10.1271/bbb.69.999
Chetnik, K., Petrick, L., and Pandey, G. (2020). MetaClean: a machine learning-based classifier for reduced false positive peak detection in untargeted LC–MS metabolomics data. Metabolomics 16, 117. doi: 10.1007/s11306-020-01738-3
Chhetri, D. R. (2019). Myo-inositol and its derivatives: their emerging role in the treatment of human diseases. Front. Pharmacol. 10, 1172. doi: 10.3389/fphar.2019.01172
Chung, H. J., Sim, J. H., Min, T. S., and Choi, H. K. (2018). Metabolomics and lipidomics approaches in the science of probiotics: a review. J. Med. Food 21, 1086–1095. doi: 10.1089/jmf.2017.4175
Cicero, N., Corsaro, C., Salvo, A., Vasi, S., Giofré, S. V., Ferrantelli, V., et al. (2015). The metabolic profile of lemon juice by proton HR-MAS NMR: the case of the PGI Interdonato Lemon of Messina. Nat. Prod. Res. 29, 1894–1902. doi: 10.1080/14786419.2015.1012166
Costantini, A., Vaudano, E., Pulcini, L., Carafa, T., and Garcia-Moruno, E. (2019). An overview on biogenic amines in wine. Beverages 5, 19. doi: 10.3390/beverages5010019
Cotter, D. R., Sabherwal, S., and Schubert, K. O. (2020). “Chapter 25 - Proteomics for diagnostic and therapeutic blood biomarker discovery in schizophrenia and other psychotic disorders,” in Personalized Psychiatry, ed B. T. Baune (San Diego, CA: Academic Press), 307–317. doi: 10.1016/B978-0-12-813176-3.00025-0
Council of the European Union (2006). Council Regulation (EC) No 510/2006 of 20 March 2006 on the protection of geographical indications and designations of origin for agricultural products and foodstuffs. Off. J. Eur. Union 50, 12–25.
Criste, A., Copolovici, L., Copolovici, D., Kovacs, M., Madden, R. H., Corcionivoschi, N., et al. (2020). Determination of changes in the microbial and chemical composition of Ṭaga cheese during maturation. PLoS ONE, 15, e0242824–e0242824. doi: 10.1371/journal.pone.0242824
Dahlan, H. A., Nambu, Y., Putri, S. P., and Fukusaki, E. (2022). Effects of soaking tempe in vinegar on metabolome and sensory profiles. Metabolites 12, 30. doi: 10.3390/metabo12010030
Diez-Simon, C., Mumm, R., and Hall, R. D. (2019). Mass spectrometry-based metabolomics of volatiles as a new tool for understanding aroma and flavour chemistry in processed food products. Metabolomics 15, 41. doi: 10.1007/s11306-019-1493-6
Diouf, A., Sarr, F., Sene, B., Ndiaye, C., Fall, S., Ayessou, N., et al. (2019). Pathways for reducing anti-nutritional factors: prospects for Vigna unguiculata. J. Nutr. Health Food Sci. 7, 1–10. doi: 10.15226/jnhfs.2019.001157
Gaggìa, F., Gaggìa, F., Baffoni, L., Galiano, M., Nielsen, D. S., Jakobsen, R. R., et al. (2018). Kombucha beverage from green, black and rooibos teas: a comparative study looking at microbiology, chemistry and antioxidant activity. Nutrients 11, 1. doi: 10.3390/nu11010001
Gallo, K., Kemmler, E., Goeda, A., Becker, F., Dunkel, M., Preissner, R., et al. (2023). SuperNatural 3.0—a database of natural products and natural product-based derivatives. Nucleic Acids Res. 51, D654–D659. doi: 10.1093/nar/gkac1008
Gao, Y., Xu, B., Fan, H., Zhang, M. R., Zhang, L. J., Lu, C., et al. (2020). 1H NMR-based chemometric metabolomics characterization of soymilk fermented by Bacillus subtilis BSNK-5. Food Res. Int. 138, 109686. doi: 10.1016/j.foodres.2020.109686
García-Cano, I., Rocha-Mendoza, D., Ortega-Anaya, J., Wang, K., Kosmerl, E., Jiménez-Flores, R., et al. (2019). Lactic acid bacteria isolated from dairy products as potential producers of lipolytic, proteolytic and antibacterial proteins. Appl. Microbiol. Biotechnol. 103, 5243–5257. doi: 10.1007/s00253-019-09844-6
García-García, A. B., Lamichhane, S., Castejón, D., Cambero, M. I., and Bertram, H. C. (2018). 1H. HR-MAS NMR-based metabolomics analysis for dry-fermented sausage characterization. Food Chem. 240, 514–523. doi: 10.1016/j.foodchem.2017.07.150
Garcia-Gutierrez, E., and Sayavedra, L. (2022). “3.07-Diet, microbiota and the gut-brain axis,” in Reference Module in Food Science, ed Glibetic, M. (Oxford: Elsevier), 69–83. doi: 10.1016/B978-0-12-819265-8.00089-9
Ghatani, K., and Tamang, B. (2017). Assessment of probiotic characteristics of lactic acid bacteria isolated from fermented yak milk products of Sikkim, India: Chhurpi, Shyow, and Khachu. Food Biotechnol. 3, 210–232. doi: 10.1080/08905436.2017.1335212
Ghatani, K., Thapa, S., Sha, S. P., Sarkar, S., and Modak, D. (2022). Revealing probiotic potential of Enterococcus strains isolated from traditionally fermented chhurpi and healthy human gut. Front. Microbiol. 13, 909987. doi: 10.3389/fmicb.2022.909987
Gibson, G. R., and Roberfroid, M. B. (1995). Dietary modulation of the human colonic microbiota: introducing the concept of prebiotics. J. Nutr.125, 1401–1412. doi: 10.1093/jn/125.6.1401
Go, E. P. (2010). Database resources in metabolomics: an overview. J. Neuroimmune Pharmacol. 5, 18–30. doi: 10.1007/s11481-009-9157-3
Gomez-Casati, D. F., Zanor, M. I., and Busi, M. V. (2013). Metabolomics in plants and humans: applications in the prevention and diagnosis of diseases. BioMed Res. Int. 2013, 792527. doi: 10.1155/2013/792527
Graham Linck, E. J., Richmond, P. A., Tarailo-Graovac, M., Engelke, U., Kluijtmans, L. A. J., Coene, K. L. M., et al. (2020). metPropagate: network-guided propagation of metabolomic information for prioritization of metabolic disease genes. Npj Genom. Med. 5, 25. doi: 10.1038/s41525-020-0132-5
Grewal, J. (2020). Gamma-aminobutyric acid (GABA): a versatile bioactive compound. Eur. J. Mol. Clin. Med. 7, 3068–3075.
Gu, Y., Li, X., Xiao, R., Dudu, O. E., Yang, L., Ma, Y., et al. (2020). Impact of Lactobacillus paracasei IMC502 in coculture with traditional starters on volatile and non-volatile metabolite profiles in yogurt. Process Biochem. 99, 61–69. doi: 10.1016/j.procbio.2020.07.003
Han, B.-Z., Rombouts, F. M., and Nout, M. J. R. (2004). Amino acid profiles of sufu, a Chinese fermented soybean food. J. Food Composit. Anal. 17, 689–698. doi: 10.1016/j.jfca.2003.09.012
He, Y., Huang, M., Tang, C., Yue, Y., Liu, X., Zheng, Z., et al. (2021). Dietary daidzein inhibits hepatitis C virus replication by decreasing microRNA-122 levels. Virus Res. 298, 198404. doi: 10.1016/j.virusres.2021.198404
Huang, L., Wang, C., Zhang, Y., Chen, X., Huang, Z., Xing, G., et al. (2019). Degradation of anti-nutritional factors and reduction of immunoreactivity of tempeh by co-fermentation with Rhizopus oligosporus RT-3 and Actinomucor elegans DCY-1. Int. J. Food Sci. Technol. 54, 1836–1848. doi: 10.1111/ijfs.14085
Huang, X., Yu, S., Han, B., and Chen, J. (2018). Bacterial community succession and metabolite changes during sufu fermentation. LWT 97, 537–545. doi: 10.1016/j.lwt.2018.07.041
Hussin, F. S., Chay, S. Y., Hussin, A. S. M., Ibadullah, W. Z. W., Muhialdin, B. J., Ghani, M. S. A., et al. (2021). GABA enhancement by simple carbohydrates in yoghurt fermented using novel, self-cloned Lactobacillus plantarum Taj-Apis362 and metabolomics profiling. Sci. Rep. 11, 9417. doi: 10.1038/s41598-021-88436-9
Ivanović, S., Simić, K., Tešević, V., Vujisić, L., Ljekočević, M., Godevac, D., et al. (2021). GC-FID-MS based metabolomics to access plum brandy quality. Molecules 26, 1391. doi: 10.3390/molecules26051391
Jang, C. H., Oh, J., Lim, J. S., Kim, H. J., and Kim, J. S. (2021). Fermented soy products: beneficial potential in neurodegenerative diseases. Foods 10, 636. doi: 10.3390/foods10030636
Jayachandran, M., and Xu, B. (2019). An insight into the health benefits of fermented soy products. Food Chem. 271, 362–371. doi: 10.1016/j.foodchem.2018.07.158
Jung, W. Y., Jung, J. Y., Lee, H. J., and Jeon, C. O. (2016). functional characterization of bacterial communities responsible for fermentation of doenjang: a traditional korean fermented soybean paste. Front. Microbiol. 7, 827. doi: 10.3389/fmicb.2016.00827
Kadar, A. D., Aditiawati, P., Astawan, M., Putri, S. P., and Fukusaki, E. (2018). Gas chromatography coupled with mass spectrometry-based metabolomics for the classification of tempe from different regions and production processes in Indonesia. J. Biosci. Bioeng. 126, 411–416. doi: 10.1016/j.jbiosc.2018.03.020
Kadar, A. D., Astawan, M., Putri, S. P., and Fukusaki, E. (2020). Metabolomics-based study of the effect of raw materials to the end product of tempe-an indonesian fermented soybean. Metabolites 10, 367. doi: 10.3390/metabo10090367
Kale, N. S., Haug, K., Conesa, P., Jayseelan, K., Moreno, P., Rocca-Serra, P., et al. (2016). MetaboLights: an open-access database repository for metabolomics data. Curr. Protoc. Bioinformatics 53, 14.13.1–14.13.18. doi: 10.1002/0471250953.bi1413s53
Karp, P. D., Riley, M., Paley, S. M., and Pellegrini-Toole, A. (2002). The MetaCyc database. Nucleic Acids Res. 30, 59–61. doi: 10.1093/nar/30.1.59
Kharnaior, P., and Tamang, J. P. (2022). Metagenomic-metabolomic mining of kinema, a naturally fermented soybean food of the Eastern Himalayas. Front. Microbiol. 13, 868383. doi: 10.3389/fmicb.2022.868383
Kim, D. J., Jeong, Y. J., Kwon, J. H., Moon, K. D., Kim, H. J., Jeon, S. M., et al. (2008). Beneficial effect of chungkukjang on regulating blood glucose and pancreatic β-cell functions in C75BL/KsJ-db/db Mice. J. Med. Food 11, 215–223. doi: 10.1089/jmf.2007.560
Kim, I.-S., Hwang, C.-W., Yang, W.-S., and Kim, C.-H. (2021). Current perspectives on the physiological activities of fermented soybean-derived Cheonggukjang. Int. J. Mol. Sci. 22, 5746. doi: 10.3390/ijms22115746
Koistinen, V., Bento da Silva, A., Abrankó, L., Low, D., Garcia Villalba, R., TomásBarberán, F., et al. (2018). Interlaboratory coverage test on plant food bioactive compounds and their metabolites by mass spectrometry-based untargeted metabolomics. Metabolites 8, 46. doi: 10.3390/metabo8030046
Kopka, J., Schauer, N., Krueger, S., Birkemeyer, C., Usadel, B., Bergmüller, E., et al. (2005).R01EQENTQi5EQg==: the Golm metabolome database. Bioinformatics 21, 1635–1638. doi: 10.1093/bioinformatics/bti236
Kwon, Y. S., Lee, S., Lee, S. H., Kim, H. J., and Lee, C. H. (2019). Comparative evaluation of six traditional fermented soybean products in east asia: a metabolomics approach. Metabolites 9, 183. doi: 10.3390/metabo9090183
Laugerette, F., Passilly-Degrace, P., Patris, B., Niot, I., Febbraio, M., Montmayeur, J.-P., et al. (2005). CD36. involvement in orosensory detection of dietary lipids, spontaneous fat preference, and digestive secretions. J. Clin. Invest. 115, 3177–3184. doi: 10.1172/JCI25299
Lee, D. E., Lee, S., Jang, E. S., Shin, H. W., Moon, B. S., Lee, C. H., et al. (2016a). Metabolomic profiles of Aspergillus oryzae and Bacillus amyloliquefaciens during rice koji fermentation. Molecules 21, 773. doi: 10.3390/molecules21060773
Lee, J.-E., Park, S. R., and Lim, S. (2016b). Mass spectrometry-based metabolomic study of traditional Doenjang effects against hepatic fibrosis. Appl. Biol. Chem. 59, 775–780. doi: 10.1007/s13765-016-0225-0
Lee, J.-E., Yun, J.-H., Lee, E., and Hong, S. P. (2022). Untargeted metabolomics reveals doenjang metabolites affected by manufacturing process and microorganisms. Food Res. Int. 157, 111422. doi: 10.1016/j.foodres.2022.111422
Lee, S. H., Lee, S., Lee, S. H., Kim, H. J., Singh, D., Lee, C. H., et al. (2021). Integrated metabolomics and volatolomics for comparative evaluation of fermented soy products. Foods 10, 2516. doi: 10.3390/foods10112516
Lee, S. Y., Lee, S., Lee, S., Oh, J. Y., Jeon, E. J., Ryu, H. S., et al. (2014). Primary and secondary metabolite profiling of doenjang, a fermented soybean paste during industrial processing. Food Chem. 165, 157–166. doi: 10.1016/j.foodchem.2014.05.089
Li, D., Zheng, Y., Kwok, L., Zhang, W., and Sun, T. (2020). Metabolic footprinting revealed key biochemical changes in a brown fermented milk product using Streptococcus thermophilus. J. Dairy Sci. 103, 2128–2138. doi: 10.3168/jds.2019-16881
Li, P., Tang, H., Shi, C., Xie, Y., Zhou, H., Xia, B., et al. (2019). Untargeted metabolomics analysis of Mucor racemosus Douchi fermentation process by gas chromatography with time-of-flight mass spectrometry. Food Sci. Nutr. 7, 1865–1874. doi: 10.1002/fsn3.1042
Liu, J., Chen, J., Li, S., Tian, W., Wu, H., Han, B., et al. (2021). Comparison of volatile and non-volatile metabolites in sufu produced with bacillus licheniformis by rapid fermentation. Int. J. Food Prop. 24, 553–563. doi: 10.1080/10942912.2021.1901733
Loh, L. X., Ng, D. H. J., Toh, M., Lu, Y., and Liu, S. Q. (2021). Targeted and nontargeted metabolomics of amino acids and bioactive metabolites in probiotic-fermented unhopped beers using liquid chromatography high-resolution mass spectrometry. J. Agric. Food Chem. 69, 14024–14036. doi: 10.1021/acs.jafc.1c03992
Ly, S., Mith, H., Tarayre, C., Taminiau, B., Daube, G., Fauconnier, M.-L. Delvigne F., et al. (2018). Impact of microbial composition of cambodian traditional dried starters (Dombea) on flavor compounds of rice wine: combining amplicon sequencing with HP-SPME-GCMS. Front. Microbiol. 9, 894. doi: 10.3389/fmicb.2018.00894
Majumder, S., Ghosh, A., Chakraborty, S., Saha, S., and Bhattacharya, M. (2021). Metabolomics affirms traditional alcoholic beverage raksi as a remedy for high-altitude sickness. J. Ethn. Foods 8, 17. doi: 10.1186/s42779-021-00094-4
Majumder, S., Ghosh, A., Saha, S., and Acharyya, S. (2022). Valorization of CTC tea waste through kombucha production and insight into GC-MS based metabolomics. Canrea J. Food Technol. Nutr. Culin. J. 5, 38–56. doi: 10.20956/canrea.v5i1.594
Malashree, L. Mudgil, P., Dagar, S. S., Kumar, S., and Puniya, A. K. (2012). β-glucosidase activity of lactobacilli for biotransformation of soy isoflavones. Food Biotechnol. 26, 154–163. doi: 10.1080/08905436.2012.670832
Manchester, M., and Anand, A. (2017). “Chapter two - Metabolomics: strategies to define the role of metabolism in virus infection and pathogenesis,” in Advances in Virus Research, eds M. Kielian, T. C. Mettenleiter, and M. J. Roossinck (Cambridge, MA: Academic Press), 57–81. doi: 10.1016/bs.aivir.2017.02.001
Manchia, M., Comai, S., Pinna, M., Pinna, F., Fanos, V., Denovan-Wright, E., et al. (2019). “Chapter Four - Biomarkers in aggression,” in Advances in Clinical Chemistry, ed G. S. Makowski (Amsterdam: Elsevier), 169–237. doi: 10.1016/bs.acc.2019.07.004
Marquez, A., Serratosa, M., and Merida, J. (2013). Pyranoanthocyanin derived pigments in wine: structure and formation during winemaking. J. Chem. 2013, 713028. doi: 10.1155/2013/713028
Martín, I., Rodríguez, A., Sánchez-Montero, L., Padilla, P., and Córdoba, J. J. (2021). Effect of the dry-cured fermented sausage “salchichón” processing with a selected lactobacillus sakei in listeria monocytogenes and microbial population. Foods. 10, 856. doi: 10.3390/foods10040856
Martins, D., Jesus, N., Figueiredo, F., Arunachalam, K., and Caraballo Rodríguez, A. (2020). Global Natural Products Social Molecular Networking (GNPS): Fundamentals and Applications. 1st edition. Editora CRV.
Medina, R. B., Katz, M. B., González, S., and Oliver, G. (2004). Determination of esterolytic and lipolytic activities of lactic acid bacteria. Methods Mol. Biol. 268, 465–470. doi: 10.1385/1-59259-766-1:465
Mendes, I., Sanchez, I., Franco-Duarte, R., Camarasa, C., Schuller, D., Dequin, S., et al. (2017). Integrating transcriptomics and metabolomics for the analysis of the aroma profiles of Saccharomyces cerevisiae strains from diverse origins. BMC Genomics 18, 455. doi: 10.1186/s12864-017-3816-1
Mina, M. (2017). “Contribution of yeast in wine aroma and flavour,” in Yeast - Industrial Applications, eds A. Morata, and I. Loira (Rijeka: IntechOpen), 5. doi: 10.5772/intechopen.70656
Misra, B. B. (2021). New software tools, databases, and resources in metabolomics: updates from 2020. Metabolomics 17, 49. doi: 10.1007/s11306-021-01796-1
Mohamed, M. A., Hir, Z. A. M., Mokhtar, W. N. A. W., and Osman, N. S. (2020). “Features of metal oxide colloidal nanocrystal characterization,” in Colloidal Metal Oxide Nanoparticles Synthesis, Characterization and Applications, eds S. Thomas, A. Tresa Sunny, P. B. Velayudhan (Amsterdam: Elsevier), 83–122. doi: 10.1016/B978-0-12-813357-6.00008-5
Mok, W. K., Tan, Y. X., Lee, J., Kim, J., and Chen, W. N. (2019). A metabolomic approach to understand the solid-state fermentation of okara using Bacillus subtilis WX-17 for enhanced nutritional profile. AMB Express 9, 60. doi: 10.1186/s13568-019-0786-5
Morata, A., Benito, S., Loira, I., Palomero, F., González, M. C., Suárez-Lepe, J. A., et al. (2012). Formation of pyranoanthocyanins by Schizosaccharomyces pombe during the fermentation of red must. Int. J. Food Microbiol. 159, 47–53. doi: 10.1016/j.ijfoodmicro.2012.08.007
Muhialdin, B. J., Marzlan, A. A., Kadum, H., Arulrajah, B., Mohamad Asri, N., Fathallah, S., et al. (2021). Metabolomics profiling and antimicrobial activity of fermented date fruit (Khastawi) used as functional ingredients for making Asian confectionary (Dodol). Biotechnol. Biotechnol. Equip. 35, 478–486. doi: 10.1080/13102818.2021.1892526
Murgia, A., Scano, P., Cacciabue, R., Dessì, D., and Caboni, P. (2019). GC-MS metabolomics comparison of yoghurts from sheep's and goats' milk. Int. Dairy J. 96, 44–49. doi: 10.1016/j.idairyj.2019.03.012
Muzaddadi, A. U., Devatkal, S., and Oberoi, H. S. (2016). “Seafood enzymes and their application in food processing,” in Agro-Industrial Wastes as Feedstock for Enzyme Production, eds G. S. Dhillon and S. B. Kaur (San Diego, CA: Academic Press), 201–232. doi: 10.1016/B978-0-12-802392-1.00009-5
Naveja, J. J., Rico-Hidalgo, M. P., and Medina-Franco, J. L. (2018). ‘Analysis of a large food chemical database: chemical space, diversity, and complexity [version 2; peer review: 3 approved]. F1000Res. 7, Chem Inf Sci-993. doi: 10.12688/f1000research.15440.2
Nedele, A.-K., Schiebelbein, R., Bär, A., Kaup, A., and Zhang, Y. (2022). Reduction of aldehydes with green odor in soy products during fermentation with Lycoperdon pyriforme and analysis of their degradation products. Food Res. Int. 152, 110909. doi: 10.1016/j.foodres.2021.110909
Nissen, L., Bordoni, A., and Gianotti, A. (2020). Shift of volatile organic compounds (VOCs) in gluten-free hemp-enriched sourdough bread: a metabolomic approach. Nutrients 12, 1050. doi: 10.3390/nu12041050
Ogodo, A. C., Agwaranze, D. I., Aliba, N. V., Kalu, A. C., and Nwaneri, C. B. (2019). Fermentation by lactic acid bacteria consortium and its effect on anti-nutritional factors in maize flour. J. Biol. Sci. 19, 17–23. doi: 10.3923/jbs.2019.17.23
Okoth, R. A., Matofari, J. W., and Nduko, J. M. (2022). Effectiveness of Levilactobacillus brevis fermentation on antinutrients and protein quality of leaves of selected cassava varieties. Appl. Food Res. 2, 100134. doi: 10.1016/j.afres.2022.100134
Okunowo, W., and Osuntoki, A (2007). Quantitation of alcohols in orange wine fermented by four strains of yeast. Afr. J. Biochem. Res. 1, 95–100.
Parente, E., Cocolin, L., De Filippis, F., Zotta, T., Ferrocino, I., O'Sullivan, D., et al. (2016). FoodMicrobionet: a database for the visualisation and exploration of food bacterial communities based on network analysis. Int. J. Food Microbiol. 219, 28–37. doi: 10.1016/j.ijfoodmicro.2015.12.001
Park, M. K., and Kim, Y.-S. (2020). Comparative metabolic expressions of fermented soybeans according to different microbial starters. Food Chem. 305, 125461. doi: 10.1016/j.foodchem.2019.125461
Peng, J., Ma, L., Kwok, L. Y., Zhang, W., and Sun, T. (2022). Untargeted metabolic footprinting reveals key differences between fermented brown milk and fermented milk metabolomes. J. Dairy Sci. 105, 2771–2790. doi: 10.3168/jds.2021-20844
Pereira Braga, C., and Adamec, J. (2019). “Metabolome analysis,” in Encyclopedia of Bioinformatics and Computational Biology, eds S. Ranganathan, M. Gribskov, K. Nakai, and C. Schönbach (Oxford: Academic Press), 463–475. doi: 10.1016/B978-0-12-809633-8.20134-9
Pinu, F. R., Edwards, P. J. B., Jouanneau, S., Kilmartin, P. A., Gardner, R. C., Villas-Boas, S. G., et al. (2014). Sauvignon blanc metabolomics: grape juice metabolites affecting the development of varietal thiols and other aroma compounds in wines. Metabolomics 10, 556–573. doi: 10.1007/s11306-013-0615-9
Putri, S. P., Ikram, M., Sato, A., Dahlan, H. A., Rahmawati, D., Ohto, Y., et al. (2022). Application of gas chromatography-mass spectrometry-based metabolomics in food science and technology. J. Biosci. Bioeng. 133, 425–435. doi: 10.1016/j.jbiosc.2022.01.011
Quan, C. S., Fan, S. D., and Ohta, Y. (2003). Immobilization of Candida krusei cells producing phytase in alginate gel beads: an application of the preparation of myo-inositol phosphates. Appl. Microbiol. Biotechnol. 62, 41–47. doi: 10.1007/s00253-003-1247-1
Rincon-Delgadillo, M. I., Lopez-Hernandez, A., Wijaya, I., and Rankin, S. A. (2012). Diacetyl levels and volatile profiles of commercial starter distillates and selected dairy foods. J. Dairy Sci. 95, 1128–1139. doi: 10.3168/jds.2011-4834
Rocchetti, G., Gatti, M., Bavaresco, L., and Lucini, L. (2018). Untargeted metabolomics to investigate the phenolic composition of Chardonnay wines from different origins. J. Food Composit. Anal. 71, 87–93. doi: 10.1016/j.jfca.2018.05.010
Ruta, L. L., and Farcasanu, I. C. (2019). Anthocyanins and anthocyanin-derived products in yeast-fermented beverages. Antioxidants 8, 182. doi: 10.3390/antiox8060182
Salama, A. A. A., and Allam, R. M. (2021). Promising targets of chrysin and daidzein in colorectal cancer: amphiregulin, CXCL1, and MMP-9. Eur. J. Pharmacol. 892, 173763. doi: 10.1016/j.ejphar.2020.173763
Salvatore, E., Addis, M., Pes, M., Fiori, M., and Pirisi, A. (2015). Evaluation of lipolysis and volatile compounds produced by three Penicillium roqueforti commercial cultures in a blue-type cheese made from ovine milk. Ital. J. Food Sci. 27, 437–442.
Samtiya, M., Aluko, R. E., and Dhewa, T. (2020). Plant food anti-nutritional factors and their reduction strategies: an overview. Food Prod. Process. Nutr. 2, 6. doi: 10.1186/s43014-020-0020-5
Segato, S., Caligiani, A., Contiero, B., Galaverna, G., Bisutti, V., Cozzi, G., et al. (2019). 1H NMR metabolic profile to discriminate pasture based alpine asiago PDO cheeses. Animals 9, 722. doi: 10.3390/ani9100722
Sekwati-Monang, B. (2011). Microbiological and chemical characterisation of ting, a sorghum-based sourdough product from Botswana. Int. J. Food Microbiol. 150, 115–121. doi: 10.1016/j.ijfoodmicro.2011.07.021
Seo, H. R., Kim, J. Y., Kim, J. H., and Park, K. Y. (2009). Identification of Bacillus cereus in a chungkukjang that showed high anticancer effects against AGS human gastric adenocarcinoma cells. J. Med. Food 12, 1274–1280. doi: 10.1089/jmf.2009.0081
Settachaimongkon, S., Nout, M. J. R., Antunes Fernandes, E. C., van Hooijdonk, T. C. M., Zwietering, M. H., Smid, E. J., et al. (2014). The impact of selected strains of probiotic bacteria on metabolite formation in set yoghurt. Int. Dairy J. 38, 1–10. doi: 10.1016/j.idairyj.2014.04.002
Seyoum, Y., Humblot, C., Baxter, B. A., Nealon, N. J., Weber, A. M., and Ryan, E. P. (2022). Metabolomics of rice bran differentially impacted by fermentation with six probiotics demonstrates key nutrient changes for enhancing gut health. Front. Nutr. 8, 795334. doi: 10.3389/fnut.2021.795334
Sha, S. P., Anupama, A., Pradhan, P., Prasad, G. S., and Tamang, J. P. (2016). Identification of yeasts by polymerase-chain-reaction-mediated denaturing gradient gel electrophoresis in marcha, an ethnic amylolytic starter of India. J. Ethnic Foods 3, 292–296. doi: 10.1016/j.jef.2016.11.009
Sha, S. P., Jani, K., Sharma, A., Anupma, A., Pradhan, P., Shouche, Y., et al. (2017). Analysis of bacterial and fungal communities in Marcha and Thiat, traditionally prepared amylolytic starters of India. Sci. Rep. 7, 10967. doi: 10.1038/s41598-017-11609-y
Sha, S. P., Suryavanshi, M. V., Jani, K., Sharma, A., Shouche, Y. S., Tamang, J. P., et al. (2018). Diversity of yeasts and molds by culture-dependent and culture-independent methods for mycobiome surveillance of traditionally prepared dried starters for the production of Indian alcoholic beverages. Front. Microbiol. 9, 2237. doi: 10.3389/fmicb.2018.02237
Sha, S. P., Suryavanshi, M. V., and Tamang, J. P. (2019). Mycobiome diversity in traditionally prepared starters for alcoholic beverages in India by high-throughput sequencing method. Front. Microbiol. 10, 348. doi: 10.3389/fmicb.2019.00348
Sharma, H., El Rassi, G. D., Lathrop, A., Dobreva, D. B., Belem, T. S., Ramanathan, R., et al. (2021). Comparative analysis of metabolites in cow and goat milk yoghurt using GC–MS based untargeted metabolomics. Int. Dairy J. 117, 105016. doi: 10.1016/j.idairyj.2021.105016
Sherman, E., Coe, M., Grose, C., Martin, D., and Greenwood, D. R. (2020). Metabolomics approach to assess the relative contributions of the volatile and non-volatile composition to expert quality ratings of pinot noir wine quality. J. Agric. Food Chem. 68, 13380–13396. doi: 10.1021/acs.jafc.0c04095
Shin, D., and Jeong, D. (2015). Korean traditional fermented soybean products: Jang. J. Ethn. Foods 2, 2–7. doi: 10.1016/j.jef.2015.02.002
Singh, M. K., and Singh, A. (2022). “Nuclear magnetic resonance spectroscopy,” in The Textile Institute Book Series, eds M. K. Singh, an A. B. Singh (Sawston: Woodhead Publishing), 321–339. doi: 10.1016/B978-0-12-823986-5.00011-7
Singh, T. K., Drake, M. A., and Cadwallader, K. R. (2003). Flavor of cheddar cheese: a chemical and sensory perspective. Compr. Rev. Food Sci. Food Saf. 2, 166–189. doi: 10.1111/j.1541-4337.2003.tb00021.x
Song, D. H., Chun, B. H., Lee, S., Son, S. Y., Reddy, C. K., Mun, H. I., et al. (2021). Comprehensive metabolite profiling and microbial communities of Doenjang (Fermented Soy Paste) and Ganjang (Fermented Soy Sauce): a comparative study. Foods 10, 641. doi: 10.3390/foods10030641
Soung, S.-H., Lee, S., Lee, S.-H., Kim, H.-J., Lee, N.-R., Lee, C.-H., et al. (2021). Metabolomic-based comparison of traditional and industrial doenjang samples with antioxidative activities. Foods 10, 1377. doi: 10.3390/foods10061377
Spratlin, J. L., Serkova, N. J., and Eckhardt, S. G. (2009). Clinical applications of metabolomics in oncology: a review. Clin. Cancer Res. 15, 431–440. doi: 10.1158/1078-0432.CCR-08-1059
Stommel, J. R., Lightbourn, G. J., Winkel, B. S., and Griesbach, R. J. (2009). Transcription factor families regulate the anthocyanin biosynthetic pathway in Capsicum annuum. J. Am. Soc. Hortic. Sci. 134, 244–251. doi: 10.21273/JASHS.134.2.244
Sud, M., Fahy, E., Cotter, D., Azam, K., Vadivelu, I., Burant, C., et al. (2016). Metabolomics Workbench: an international repository for metabolomics data and metadata, metabolite standards, protocols, tutorials and training, and analysis tools. Nucleic Acids Res. 44, D463–D470. doi: 10.1093/nar/gkv1042
Sundekilde, U. K., Larsen, L. B., and Bertram, H. C. (2013). NMR-based milk metabolomics. Metabolites 3, 204–222. doi: 10.3390/metabo3020204
Tamang, J. P. (2015). Naturally fermented ethnic soybean foods of India. J. Ethn. Foods 2, 8–17. doi: 10.1016/j.jef.2015.02.003
Tan, G., Hu, M., Li, X., Pan, Z., Li, M., Li, L., et al. (2020). High-throughput sequencing and metabolomics reveal differences in bacterial diversity and metabolites between red and white sufu. Front. Microbiol. 11, 758. doi: 10.3389/fmicb.2020.00758
Tena, N., Martín, J., and Asuero, A. G. (2020). State of the art of anthocyanins: antioxidant activity, sources, bioavailability, and therapeutic effect in human health. Antioxidants 9, 451. doi: 10.3390/antiox9050451
Thierry, A., Collins, Y. F., Abeijón Mukdsi, M. C., McSweeney, P. L. H., Wilkinson, M. G., Spinnler, H. E., et al. (2017). “Lipolysis and metabolism of fatty acids in cheese,” in Cheese, 4th edn. eds. P. L. H. McSweeney, P. F. Fox, P. D. Cotter, and D. W. Everett (San Diego, CA: Academic Press), 423–444. doi: 10.1016/B978-0-12-417012-4.00017-X
Tlais, A. Z. A., Lemos, W. J. F. J., Filannino, P., and Campanaro, S. (2022). How microbiome composition correlates with biochemical changes during sauerkraut fermentation: a focus on neglected bacterial players and functionalities. Microbiol. Spectr. 10, e0016822. doi: 10.1128/spectrum.00168-22
Tran, T., Roullier-Gall, C., Verdier, F., Martin, A., Schmitt-Kopplin, P., Alexandre, H., et al. (2022). Microbial interactions in kombucha through the lens of metabolomics. Metabolites 12, 235. doi: 10.3390/metabo12030235
Tzachristas, A., Dasenaki, M. E., Aalizadeh, R., Thomaidis, N. S., and Proestos, C. (2021). Development of a wine metabolomics approach for the authenticity assessment of selected greek red wines. Molecules 26, 2837. doi: 10.3390/molecules26102837
van der Spek, R., Kreier, F., Fliers, E., and Kalsbeek, A. (2012). “Circadian rhythms in white adipose tissue,” in The Neurobiology of Circadian Timing, ed A. Kalsbeek (Amsterdam: Elsevier), 183–201. doi: 10.1016/B978-0-444-59427-3.00011-3
van Leeuwen, C. (2010). “Terroir: the effect of the physical environment on vine growth, grape ripening and wine sensory attributes,” in Woodhead Publishing Series in Food Science, Technology and Nutrition, ed A. G. Reynolds (Sawston, CA: Woodhead Publishing), 273–315. doi: 10.1533/9781845699284.3.273
Velić, D., Klarić, A. D., Velić, N., Klarić, I., Tominac, V. P., Mornar, A., et al. (2018). “Chemical constituents of fruit wines as descriptors of their nutritional, sensorial and health-related properties,” in Descriptive Food Science, eds A. V. Díaz, and R. M. García-Gimeno (Rijeka: IntechOpen), p. 4. doi: 10.5772/intechopen.78796
Villarreal Soto, S., Bouajila, J., Pace, M., Leech, J., Cotter, P. D., Souchard, J.-P., et al. (2020). Metabolome-microbiome signatures in the fermented beverage, Kombucha. Int. J. Food Microbiol. 333, 108778. doi: 10.1016/j.ijfoodmicro.2020.108778
Visioli, F., Panaite, S.-A., and Tomé-Carneiro, J. (2020). Wine's phenolic compounds and health: a pythagorean view. Molecules 25, 4105. doi: 10.3390/molecules25184105
Wang, Y., Nie, S., Li, C., Xiang, H., Zhao, Y., Chen, S., et al. (2022). Application of untargeted metabolomics to reveal the taste-related metabolite profiles during mandarin fish (Siniperca chuatsi) fermentation. Foods 11, 944. doi: 10.3390/foods11070944
Wardhani, D. H., Fuciños, P., Vázquez, J. A., and Pandiella, S. S. (2013). Inhibition kinetics of lipid oxidation of model foods by using antioxidant extract of fermented soybeans. Food Chem. 139, 837–844. doi: 10.1016/j.foodchem.2013.01.049
Weckx, S., Van Kerrebroeck, S., and De Vuyst, L. (2018). Omics approaches to understand sourdough fermentation processes. Int. J. Food Microbiol. 302, 90–102. doi: 10.1016/j.ijfoodmicro.2018.05.029
Wöber, C., and Wöber-Bingöl, Ç. (2010). “Triggers of migraine and tension-type headache,” in Headache, eds M. J. Aminoff, F. Boller, and D. F. Swaab (Amsterdam: Elsevier), 161–172. doi: 10.1016/S0072-9752(10)97012-7
Wu, Y., Wan, J., Choe, U., Pham, Q., Schoene, N. W., He, Q., et al. (2019). Interactions between food and gut microbiota: impact on human health. Annu. Rev. Food Sci. Technol. 10, 389–408. doi: 10.1146/annurev-food-032818-121303
Xia, Y., Yu, J., Miao, W., and Shuang, Q. (2020). A UPLC-Q-TOF-MS-based metabolomics approach for the evaluation of fermented mare's milk to koumiss. Food Chem. 320, 126619. doi: 10.1016/j.foodchem.2020.126619
Xiang, X., Song, C., Shi, Q., Tian, J., Chen, C., Huang, J., et al. (2020). A novel predict-verify strategy for targeted metabolomics: comparison of the curcuminoids between crude and fermented turmeric. Food Chem. 331, 127281. doi: 10.1016/j.foodchem.2020.127281
Xie, C., Zeng, H., Wang, C., Xu, Z., and Qin, L. (2018). Volatile flavour components, microbiota and their correlations in different sufu, a Chinese fermented soybean food. J. Appl. Microbiol. 125, 1761–1773. doi: 10.1111/jam.14078
Yang, X., Hu, W., Xiu, Z., Jiang, A., Yang, X., Saren, G., et al. (2020). Microbial community dynamics and metabolome changes during spontaneous fermentation of northeast sauerkraut from different households. Front. Microbiol. 11, 1878. doi: 10.3389/fmicb.2020.01878
Yoon, G.-A., and Park, S. (2014). Antioxidant action of soy isoflavones on oxidative stress and antioxidant enzyme activities in exercised rats. Nutr. Res. Pract. 8, 618–624. doi: 10.4162/nrp.2014.8.6.618
Zhang, L., Huang, C., Johansen, P. G., Petersen, M. A., Poojary, M. M., Lund, M. N., et al. (2021). The utilisation of amino acids by Debaryomyces hansenii and Yamadazyma triangularis associated with cheese. Int. Dairy J. 121, 105135. doi: 10.1016/j.idairyj.2021.105135
Zhang, Y. V., and Gqamana, P. P. (2020). “Clinical mass spectrometry and its applications in traumatic brain injuries,” in Biomarkers for Traumatic Brain Injury, eds A. H. B. Wu, and W. F. Peacock (Cambridge, MA: Academic Press), 265–293. doi: 10.1016/B978-0-12-816346-7.00019-1
Zhou, B., Xiao, J. F., Tuli, L., and Ressom, H. W. (2012). LC-MS-based metabolomics. Mol. Biosyst. 8, 470–481. doi: 10.1039/C1MB05350G
Zhou, Y. Ma, X., Wu, J., Razak, MA., Yuan, L., and Gao, R. (2023). NMR-based metabolic analysis of Bacillus velezensis DZ11 applied to low-salt fermented coarse fish involved in the formation of flavor precursors. Food Sci. Technol. 43. doi: 10.1590/fst.117022
Keywords: metabolomics, fermented foods, metabolites, biochemical pathways, machine learning, artificial intelligence, omics
Citation: Sarkar S, Sha SP and Ghatani K (2023) Metabolomics of ethnic fermented foods and beverages: understanding new aspects through Omic techniques. Front. Sustain. Food Syst. 7:1040567. doi: 10.3389/fsufs.2023.1040567
Received: 09 September 2022; Accepted: 05 June 2023;
Published: 05 July 2023.
Edited by:
José Antonio Teixeira, University of Minho, PortugalReviewed by:
Pranita Jaiswal, Indian Council of Agricultural Research (ICAR), IndiaCopyright © 2023 Sarkar, Sha and Ghatani. This is an open-access article distributed under the terms of the Creative Commons Attribution License (CC BY). The use, distribution or reproduction in other forums is permitted, provided the original author(s) and the copyright owner(s) are credited and that the original publication in this journal is cited, in accordance with accepted academic practice. No use, distribution or reproduction is permitted which does not comply with these terms.
*Correspondence: Kriti Ghatani, Z2hhdGFuaWtAbmJ1LmFjLmlu
Disclaimer: All claims expressed in this article are solely those of the authors and do not necessarily represent those of their affiliated organizations, or those of the publisher, the editors and the reviewers. Any product that may be evaluated in this article or claim that may be made by its manufacturer is not guaranteed or endorsed by the publisher.
Research integrity at Frontiers
Learn more about the work of our research integrity team to safeguard the quality of each article we publish.