- 1Department of Agriculture Economics, Achraya N. G. Ranga Agricultural University, Bapatla, Andhra Pradesh, India
- 2Extension Education Institute, Professor Jayashankar Telangana State Agricultural University, Hyderabad, Telangana, India
- 3PME Cell, Indian Council of Agricultural Research–Central Tobacco Research Institute, Rajahmundry, Andhra Pradesh, India
- 4Division of Crop Production, Indian Council of Agricultural Research–Central Tobacco Research Institute, Rajahmundry, Andhra Pradesh, India
- 5Natural Resource Management, Indian Council of Agricultural Research–Central Coastal Agricultural Research Institute, Velha Goa, Goa, India
- 6Division of Agricultural Economics, Indian Council of Agricultural Research-Indian Agricultural Research Institute, New Delhi, India
- 7Department of Agronomy, All India Coordinated Research Project on Castor, University of Agricultural Sciences, Bengaluru, India
The phenomena of climate change pose multifaceted challenges to crop and livestock farming, with severe implications on smallholder farmers' income and livelihoods. Climate change has profound implications (economic, environmental, and social) predominantly on rainfed regions in developing countries like India, where agriculture constitutes the backbone of the economy. In this context, the current study analyzes how farmers perceive climate change in the rainfed ecosystem in India, farmers' adaptation strategies, and their major determinants in addressing climate change. Data were collected from 400 sample farmers in South India. Discriminant and multinomial logit models were employed to identify the adaptation strategies of the farmers. It was evident that the factors such as off-farm income, farm income, and farming experience significantly influenced the adaptation strategies for tackling climate change. Furthermore, access to climate change information and literacy level are vital determinants in different climate change adaptation strategies, including crop diversification, integrated farming system, contingency plans for farm operations, and adoption of soil and water conservation techniques. However, the study highlights the increasing role of institutions (government and private) in future to safeguard the interests of farmers by offering a wide range of policy, research, and technology interventions. In a nutshell, R&D focus on climate-resilient agriculture, application of ICTs in agro-advisory services, and creation off-farm employment opportunities for the farmers is crucial to sustaining their livelihoods as these serve as potential mitigation strategies to impart resilience to climate-sensitive sectors like agriculture in rainfed ecosystems in India or any other countries.
Introduction
Climate change jeopardizes long-term agricultural development, which is dependent on three layers of environmental, economic, and social effects that are all integrated. The significance of agriculture emanates from the fact that it is vital to the economic growth of developing countries, serving as the backbone of their economies by providing food, fiber, raw materials, and employment opportunities to the major chunk of the population (Ogen, 2007). Agriculture is the prime source of sustainable food and nutrition, which is extremely dependent on and influenced by weather and harsh climatic manifestations (Mjelde et al., 1989; Das, 2005; Motha and Murthy, 2007; Sivakumar, 2011; CIE, 2014). Climate change has harmed crop production and productivity in major agricultural regions around the world in recent decades (Almaraz et al., 2008; Reidsma et al., 2009). Moreover, the negative effects of climate change on agricultural production have resulted in high poverty rates (Mendelsohn et al., 2006) and global food insecurity (Das, 2005; Rosenzweig and Tubiello, 2007; Nelson et al., 2009; Misra, 2012; Connoly-Boutin and Smit, 2015). Conversely, a smallholder farmer possesses limited resources or capacity to adapt to climate change (Verchot et al., 2007). However, developing countries have a lower adaptive capacity and do not have the advanced technology to mitigate climate change (Lotze-Campen and Schellnhuber, 2009). Crop production fluctuations have an impact on food availability, prices, and farm revenues, all of which bewilder rural economic advancement. According to some estimates, annual agricultural revenue losses due to climate change in India are predicted to be in the range of 15–18%, rising to 20–25% for unirrigated areas (Government of India, 2017).
Agriculture is the socioeconomic foundation for achieving food security, which is based on the elimination of extreme poverty and hunger (Von Braun et al., 2005). In rural and marginal areas, agriculture is critical to community livelihoods. In this context, agricultural policies and government interventions in rural communities are essential tools for poverty reduction as part of an inclusive approach to economic and social development (Croppenstedt et al., 2018). Nevertheless, climate change, according to the IPCC (2013), affects people's livelihoods, agriculture, freshwater supplies, and other natural resources, which are vital to human survival. Climate change affects crop output, particularly among vulnerable people in rural areas, such as smallholder farmers who rely on rainfed agriculture for a living (Turpie and Visser, 2013). Given the emerging importance of climate change, various studies have been conducted to establish the effects of climate change on farm productivity and describe farmers' climate change adaptation strategies (CCAS) in a particular region. Shrestha et al. (2012) examined the effects of climate change on winter and summer paddy yields in the central area as well as numerous CCAS in Vietnam. Changing planting dates, supplemental irrigation, correct nutrient management, and switching to new rice varieties are among the probable adaptive options for rice cultivation in the region (Shrestha and Bui, 2015). However, some farmers resorted to adaptive practices in the region in response to climate change, for instance, altering transplanting dates and introducing supplementary irrigation.
Climate-resilient agriculture (CRA) is being encouraged to enable climate change adaptation and mitigation. Environmental changes, including climate change, land-use change, and natural resource degradation, have aggravated the vulnerability of agricultural production across the countries in the world. Among these, climate change has emerged as the biggest developmental challenge, especially for developing countries like India, by disrupting the normal socioeconomic settings, particularly of poor people (Narain et al., 2009). Its adverse effects are much more severe on the agricultural sector in affecting both food and nutrition security and sustainable development. Therefore, it is imperative on the part of farmers to face climate change in agriculture by following various adaptation strategies that demand collaborative efforts from different stakeholders. Of course, the major driving force for taking up climatic adaptation strategies comes from farmers' perceptions to tackle the climate change phenomenon.
The review of the literature identifies adaptation to climate change as an established strategy that is thoroughly tangled with developmental activities (Agrawala and Lemos, 2015; Anik et al., 2021; Mushore et al., 2021). India experienced a series of droughts (Figure 1), and the one in 1987 was one of the worst, with an overall rainfall deficiency of 19%, which affected 60% of the normal cropped area and a population of 285 million. This was repeated in 2002 when the overall rainfall deficiency for the country as a whole was again 19%. Over 300 million people spread over 18 states are affected by drought along with around 150 million cattle. Food grain production registered an unprecedented steep fall of 29 million tons. Subsequently, the drought in 2018 is considered the second most severe one, affecting ~42% of the land area and 500 million people (almost 40% of the country's population). With the advent of climate change since the 1990s (Narain et al., 2009), failed monsoon is the primary reason for frequent droughts in India. Since it is not possible to avoid the adverse impacts of climate change (Figure 2), it is vital to promote adaptation strategies among the farmers to mitigate it in their farm fields. Before this, it is essential to analyze their perceptions about CCAS and determinants of the same for their effective implementation. The past studies, conducted in South Africa (Tshikororo et al., 2021), Ghana (Mwinkom et al., 2021), Ethiopia (Belay et al., 2017), Uganda (Nabikilo et al., 2012), and Fiji (Asafu-Adjaye, 2008), highlighted that farmers changed their cultivation practices as adaptation strategies in various ways, including change in crop calendar, crop varieties, farm machinery for cultivation practices, crop diversification, integrating crops with livestock (farming systems approach), and soil and water conservation practices. Even strategies such as the System of Rice Intensification (SRI)—an innovative method of rice cultivation and microirrigation, were adopted by the farmers to combat water scarcity conditions. They also implemented strategies for coping with declining soil productivity by increasing organic manure application, compost making and application, crop rotation, and crop residue retention (Belay et al., 2017; Tshikororo et al., 2021). In India, the government started promoting the formation of Farmer Producer Organizations when a single farmer could not afford adaptation strategies (Singh et al., 2019). However, the study conducted by Niles et al. (2016) revealed an interesting finding that the farmers' attitudes and perceptions toward climate change do not correlate with their actual adoption. Climate change jeopardizes long-term agricultural development, which is dependent on three layers of environmental, economic, and social effects that are all merging. Climate change not only is an environmental issue but also has significant economic and social implications, particularly for emerging countries that are particularly sensitive, offering significant challenges to their agricultural development and wellbeing (Tesfahunegn et al., 2016).
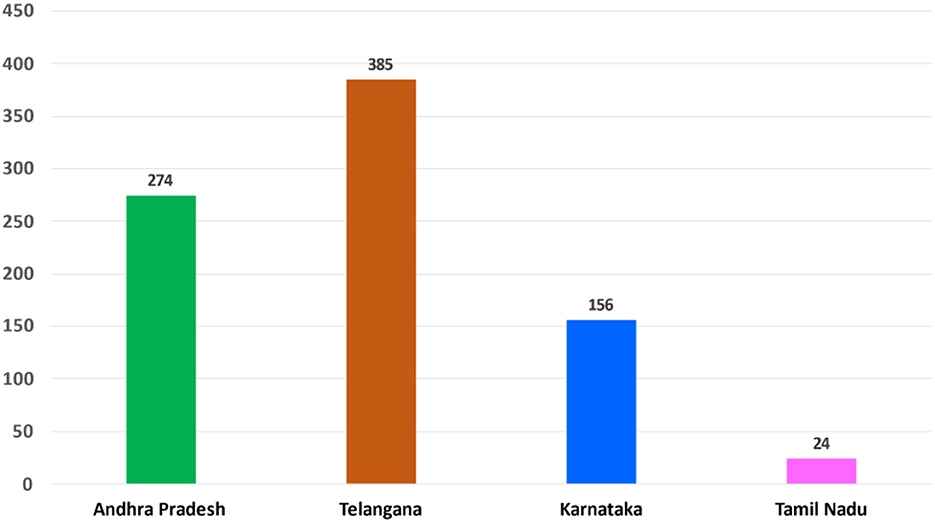
Figure 1. Number of Mandals declared drought in the southern states of India, 2019 (Source: Statistical Abstracts of selected States, 2020).
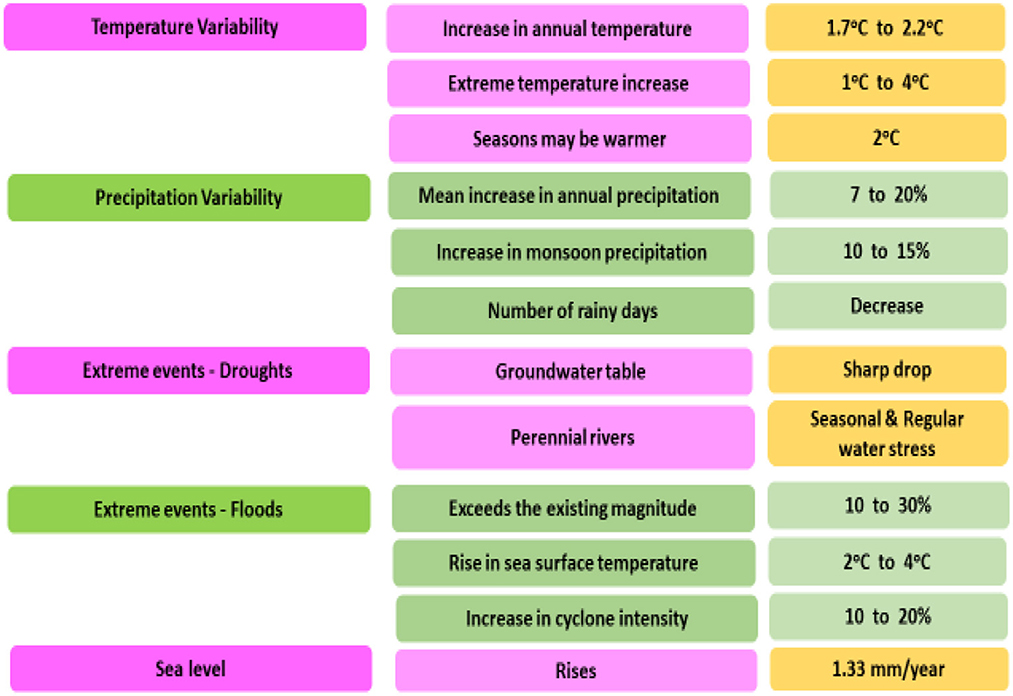
Figure 2. Climate change projections for India by 2030 (compiled by Narain et al., 2009).
From the growing body of literature, it is evident that the agriculture sector is heavily impacted by changing climatic circumstances (Lobell et al., 2011; Auffhammer and Schlenker, 2014; Campbell et al., 2016; Khanal and Mishra, 2017), the severity of which is expected to worsen shortly (Lobell et al., 2011; Auffhammer and Schlenker, 2014; Campbell et al., 2016; Khanal and Mishra, 2017). Furthermore, crop productivity levels are harmed by weather incongruities and sudden onset of extremes (dry spells, droughts, and floods) (IPCC, 2012, 2014) due to pest and disease outbreaks (Easterling et al., 2007; Gornall et al., 2010), changes in soil fertility (Tang et al., 2008; St Clair and Lynch, 2010), moisture content, and most importantly, water quality and resources (Food and Agriculture Organization, 2011; Misra, 2014; Malek et al., 2018). In Italy, for example, farmer innovation has been found to have a favorable impact on the adoption of water-saving devices (Pino et al., 2017). This reflects process innovation at the farm level, which is the act of adopting new farm methods and putting new information into action (Barzola Iza and Dentoni, 2020). Therefore, it is equally important to analyze the determinants of different climatic adaptation strategies being followed by the farmers in addition to their perceptions to tackle the climate change phenomenon.
Factors influencing farmers' climate change adaptation
Climate change adaptations are greatly influenced by socioeconomic and environmental indicators. The earlier studies identified the unpredictability of weather, high farm input cost, and lack of access to timely weather information and water resources as the major constraints of farmers' adaptation to climate change. Ndamani and Watanabe (2016) analyzed socioeconomic factors that influence farmers' adaptation to climate change in agriculture and opined that education, household size, annual household income, access to information, credit, and membership in farmer-based organizations as the most important factors that influence farmers' adaptation to climate change. Similarly, Belay et al. (2017) analyzed the smallholder farmers' CCAS and the determinants of their adaptation decisions in the Central Rift Valley of Ethiopia. The findings of the study revealed that the farmers' capacity to choose effective adaptation options was influenced by household demography, as well as positively by farm size, income, access to markets, access to climate information, extension, and livestock production. Zizinga et al. (2017) analyzed the household determinants that contribute to CCAS in the Mount Rwenzori area of southwestern Uganda. The study concluded the use of different crop varieties; tree planting, soil and water conservation, early and late planting, and furrow irrigation are the major adaptation practices. The findings of the discrete choice model indicated that the age of the household head, experience in farming, household size, climate change shocks, land size, use of agricultural inputs, landscape position (location), and crop yield varied significantly (p > 0.05) and influenced farmers' choice of CCAS. Mwinkom et al. (2021) investigated the factors influencing adaptation strategies to climate change in the Black Volta Basin of Ghana. The multivariate probit model revealed that gender, age, household size, farmer-based organization membership, farm income, years of education, districts of the location of respondents, farm size, and climate change awareness are the major factors that influenced households' adaptation to the changing climate. Tshikororo et al. (2021) analyzed the farmers' socioeconomic characteristics in tackling climate change in Limpopo Province, South Africa. The study concluded that formal education, agricultural education, age group, farming experience, and off-farm occupation significantly contributed to farmers' perception regarding tackling climate change.
In summary, inadequate information on adaptation methods and financial constraints are the major barriers to adaptation. Considering the above facts in view, there is a need to support the indigenous adaptation strategies with a wide range of institutional, policy, and technological support. At the same time, creating opportunities for non-farm income sources is equally important, as these kinds of activities are less sensitive to climate change. In addition, providing climate change information, extension services, and creating access to markets is also crucial in tackling the impact of climate change on agriculture. Furthermore, there is an urgency in improving household heads' adaptive capacity through education and capacity building, and increasing investments in climate-resilient programs by governmental and non-governmental organizations should deserve special attention. Understanding the elements that influence farmers' decisions to choose one of the available adaptive methods might give a strong foundation for drafting policy suggestions that are sensitive to climatic change (Piya et al., 2013).
Despite the high frequency of climate-induced risks in farming, no research has identified the elements influencing farmers' adaptive choices in agricultural production in the rainfed region of South India under changing climate conditions. This study was focused on a better understanding of perceptions and practices followed by the farmers to tackle climate change in four southern states, namely, Andhra Pradesh, Telangana, Tamil Nadu, and Karnataka. As no prior research on these lines was conducted earlier in South India, this study certainly contributes to the existing literature on farmers' perceptions as one of the major critical elements to dealing with climate change and identifying major determinants for practicing various adaptation strategies. Accordingly, the objective of this study was how and in what way the key determinants related to farm households influence their CCAS in the region.
Methodology
Study area and method of data collection
This study was based on the primary and secondary data, conducted in southern states of India, viz., Andhra Pradesh, Telangana, Tamil Nadu, and Karnataka. Nearly half of South India's population is engaged in agriculture, which was largely dependent on monsoon rains. Moreover, South Indian states occupied prominent positions in the cultivation of major crops such as paddy, maize, groundnut, chickpeas, urad, cotton, chilies, sunflower, tobacco, tomato, banana, cashew, coconut, and cardamom in the country. More than half of the gross area sown across these states, viz., Andhra Pradesh (52%), Telangana (63%), Tamil Nadu (54%), and Karnataka (75%), is under rainfed condition. Furthermore, in these states, more than 80 % of farmers belong to marginal and small farmers. From each state, one district was selected purposively, based on the consultations held with local agricultural officers, where it was largely affected by drought and other climate change parameters. In this regard, we purposively selected one district from each state like from Andhra Pradesh (Ananthapuramu, 540 mm), Telangana (Jogulamba Gadwal, 533 mm), Tamil Nadu (Tiruppur, 600 mm), and Karnataka (Chitradurga, 507 mm). From each district, one Mandal was selected (Ananthapuramu (Kalyandurg); Jogulamba Gadwal (Gattu Mandal); Tiruppur (Dharapuram Mandal); and Chitradurga (Challakere Mandal) in accordance with the adoption of CCAS by the farmers and from each Mandal, 100 sample farmers were selected at random. According to Yamane (1967), the minimum sample size in the study should be as follows:
Therefore, this study involved a cross-sectional survey of 400 sample farmers at 100 random farmers from each of the above four districts during 2019–2020 (Table 1). Data were collected relating to farmers' perceptions toward tackling climate change and identification of major determinants of farmers' CCAS (drought coping) in the study area. A structured questionnaire was employed among the sample farmers with assistance from local agricultural officers, who interacted directly with the farmers in their respective working locations. In the present context, two groups of farmers were made, viz., farmers willing to tackle climate change (Yes = 1) and farmers not willing to tackle climate change (No = 0). As per the survey, 256 farmers were willing and practicing CCAS and the remaining 144 farmers were not willing to tackle climate change. The socioeconomic characteristics of sample farmers (Table 2) were hypothesized to contribute to discriminating between the two categories of farmers.
Empirical models
A discriminant and multinomial logit model was employed to assess the factors influencing farmers' adoption and intensity of adoption of CCAS at the farm level in South India.
Discriminant analysis
This multivariate statistical technique was employed (Duong et al., 2017; Tshikororo et al., 2021) to classify the farmers into two (or more) mutually exclusive and exhaustive groups based on a set of independent variables, that is, the discriminant model was used to distinguish between two categories of farmers: (i) willing to tackle climate change and (ii) non-willing to tackle climate change coded as 1 and 0, respectively. These two possible categories were defined by several factors, which simultaneously influence the farmers' willingness to tackle climate change. The information related to independent variables (Table 1) used to calculate discriminant score Z for a given farmer is as follows:
where Z is the discriminant score that maximizes the distinction between the two categories.
Before running a discriminant analysis, the data used must be independent and normally distributed (Khemakhem nd Boujelbene, 2015). Therefore, the Kolmogorov–Smirnov test was employed to prove the data are normally distributed (Supplementary Table 4). Furthermore, multicollinearity among the independent variables was tested by computing Pearson's correlation matrix (Supplementary Table 5). As the highest absolute value of the correlation coefficient between each variable is < 0.7, the multicollinearity problem was ruled out in this study. In the next step, a discriminant analysis (direct method) was applied to the sample data on explanatory variables.
Multinomial logit model
To analyze the determinants of practicing different climate change adaptation strategies such as crop diversification (a shift toward drought-resistant crops), integrating crops with livestock, changing planting date, and adoption of soil and water conservation practices, the multinomial logistic model was employed (Asrat and Simane, 2018; Diallo et al., 2020; Aryal et al., 2021; Kosoe and Ahmed, 2022). These strategies were prioritized based on the informal discussions held with local Agricultural Department officials of selected Mandals. The description and expected signs of explanatory variables used in this study are presented in Table 3. The estimation of the multinomial logistic model was conducted by normalizing one category, which is named as the “base category.” The adaptation measures were grouped into four major categories because farmers used more than one strategy, and the base category was “No adaptation strategy,” that is, climate change adaptation strategy—the dependent variable (Dummy), 4 = crop diversification, 3 = integrating crop with livestock, 2 = change planting date, 1 = adoption of soil and water conservation practices, and 0 = no adaptation strategy. These measures were identified based on the discussions held with local agricultural officers and officials from the Department of Agriculture. The officials were recommending these strategies for the farmers to combat the climate change scenario. Not all the farmers were the adopters of these four strategies. Therefore, we assigned ranks to the strategies depending on their priority in the study area as emphasized by local agricultural officers. These adaptation strategies refer to changes in practices followed by the farmers to moderate potential damages or to benefit from opportunities associated with climate change. These prioritized CCAS can help decrease climate risk via major risk factors, viz., low rainfall, unseasonal rainfall, vulnerability, and exposure. Therefore, the adverse impacts of climate risks may be reduced with the help of these strategies and thus ensure farmers' resilience, reduce vulnerability, and at the same time lead to stabilized annual income. They further guide the farmers to respond to the impacts of climate change that were already affecting them, as well as prepare for future impacts.
Results and discussion
Descriptive statistics of the farmers
The description of both the dependent and the explanatory variables included in the model (see Supplementary Tables 1–3) estimations is presented in this section. The dependent variables were the adoption and determinants of adaptation strategies employed by farmers in rainfed regions of South India. The study stretches its empirical description from the studies of determinants of the adoption of CCAS (Abdulai and Huffman, 2014; Kibue et al., 2016; Mulwa et al., 2017; Ojo and Baiyegunhi, 2019). The description of explanatory variables and their respective means and the standard deviation is presented in Table 4. The socioeconomic attributes, viz., age, literacy level, farm size, farm income, and off-farm income, were included in the model to control farm household heterogeneity. The descriptive analysis revealed a mean age of 45 years with a standard deviation (SD) of 7.97 for farmers who are practicing climate adaptation strategies and a mean age of 44 years and an SD of 8.09 for farmers not willing to take up climate adaptation strategies. Among the factors such as the number of training (TRG) received on the importance of climate adaptation strategies, access to contacts with local extension officers (AEC), farming experience (FE), and farm size (FS), the results did not reveal much variation between the two categories of adoption. However, it is interesting to note that both off-farm income and farm income of farmers practicing climate adaptation strategies [Rs.26,503 (US$ 355.94) and Rs. 120,717 (US$1,621.23), respectively], were considerably higher compared to farmers not willing to practice climate adaptation strategies [Rs. 19,811 (US$266.06) and Rs. 119,900 (US$1,610.26), respectively]. Thus, it was evident that the farmers practicing climate adaptation strategies benefitted by getting higher off-farm income and farm income. On average, the respondents had 45 years of age with 13 years of FE and derived ~83% of their annual income from agriculture and the remaining 17 % from off-farm sources.
Relative significance of the discriminating variables
Table 5 represents the summary data for the discriminant analysis, and the analysis yielded one discriminant function for two categories of climate change adaptation. The findings include both unstandardized and standardized discriminant (canonical) function coefficients, and they were meant for evaluating the relative contribution of each of the predictor variables as discriminators between two categories. When predictors were measured in different units, the magnitude of an unstandardized coefficient provides little indication of its relative contribution to the discriminant function. Hence, standardizing the coefficients was necessary, to have a common scale of measurement for comparative purposes as all the predictor variables (Kumari et al., 2017).
In the derived function, the sign indicates the direction of the relationship, and the magnitude indicates the extent of contribution to the group discrimination. It is important to note that the larger the standardized coefficient (b), the larger the respective variable's unique contribution to the group discrimination (irrespective of the sign of the coefficient). All the predictors except TRG were positively influencing the discrimination of groups. It was further apparent from the analysis that off-farm income (b5 = 0.658), farm income (b7 = 0.558), and farming experience (b1 = 0.517) were the highest discriminating variables with the largest contributions. This implies that appropriate attention should be given to promoting off-farm employment opportunities. Furthermore, the farming experience should be considered in practicing/implementing climate change adaptation strategies. Therefore, by using the variables and the standardized coefficients, the required discriminant equation (discriminator) is shown as follows:
The classification results (Table 6) reveal that 79 % of respondents were classified correctly into “Willing” or “Non-willing” groups, and this overall predictive accuracy of the discriminant function represents the “hit ratio” (based on cross-validated set of data). Farmers willing to tackle climate change were classified with slightly better accuracy (84.1%) than their counterparts (74.7%).
Structure matrix
In addition to standardized coefficients, the structural matrix was also employed (Table 7) to check the relative importance of the predictors. This provides another way to study the usefulness of each predictor variable in the discriminant function. This indicates the product–moment correlations between the discriminating variables and discriminant function. Factor loadings of ≥0.30 were used as the cutoff between important and less important variables, that is, if the structure coefficient was ≥0.30, it was considered meaningful (Duong et al., 2017; Halagundegowda et al., 2017; Kumari et al., 2017). The findings indicated that the structure coefficients with the highest relationship to function 1 were OFFI (0.846), FI (0.789), and FE (0.730). These three predictors had a positive correlation with the function. Squaring the coefficient of a predictor will explain the proportion of variation in the dependent variable. For instance, OFFI can explain 72% (=0.8462) variation in the dependent variable. With 0.30 as the cutoff point, the other predictors, viz., TRG, AGE, AEC, and FS, were not loaded on the discriminant function, that is, these predictors are, therefore, not significantly associated with climate change adaptation strategies. AEC was the weakest predictor and suggests that it was not associated with adaptation strategies but a function of other unassessed factors.
The group centroids were the averages of Z-values calculated by the estimated model, which can be used to evaluate the expected position of the concerned farmers' categories (Uddin et al., 2013). As can be seen in Table 8, the centroid of the non-willing category was −0.447, and the centroid of the “willing” category was 0.252. This implies that if someone's score on the discriminant function was positive (closer to 0.252), then that respondent was probably willing to tackle climate change. On the contrary, if a person's score on the discriminant function was negative (closer to −0.447), then the data probably came from the “non-willing” category. On calculating the cut score (halfway between the two centroids), that is, −0.097 and if a person's score on the discriminant function (calculated by plugging in their scores on predictor variables) was above −0.097, then the respondent was probably from the “willing” category. On the contrary, if the discriminant function score was below −0.097, then the respondent was probably from the “non-willing” category.
Finally, the performance of the model was studied using the receiver operating characteristic (ROC) curve to compare sensitivity vs. specificity across a range of values for the ability to predict a dichotomous outcome. The area under the ROC curve was another measure of test performance (Figure 3). The results showed a large area under the curve (AUC) of 71.4%, significant at the 5% level, which further affirmed that the model was correctly specified.
Determinants for climate change adaptation strategies
The findings of the multinomial logistic model (Table 9) revealed that farming experience, farm income, access to climate information, and education (at 1% level) and off-farm income and Access to market (at 5% level) were significantly influencing the farmers to practice crop diversification toward less-water consuming and drought-resistant crops. For integrating crops with livestock, farming experience, farm income, access to market, livestock ownership (at 1% level) access to extension contacts, and farm size (at 5% level) were the most significant factors. Change in planting date was significantly influenced by farming experience, farm income, access to climate information (at 1% level), and training and education (at 5% level). Regarding the adoption of soil and water conservation practices, it was significantly influenced by farming experience, off-farm income, farm income, and access to climate information (at 1% level) and education (at 5% level). A close perusal of the table further revealed that farming experience and farm income were the crucial factors that promote the farmers to take up the climate change adaptation strategies in the southern states. The marginal effects of farming experience indicated that, for every 1-year increase, the probabilities of practicing crop diversification, integrating crops with livestock, changing planting dates, and adopting soil and water conservation practices were increased by 1.06, 0.03, 1.83, and 1.48%, respectively. Similarly, the marginal effects of farm income indicated that a unit increase in income could increase the likelihood of practicing crop diversification, integrating crops with livestock, changing planting dates, and adopting soil and water conservation practices by 9.15, 8.51, 9.33, and 4.13%, respectively. Access to climate information is another important variable that contributed to the adaptation options among the selected farmers. As expected, the findings showed that the farmers' access to climate change information had affected the likelihood of adaptation to climate change by practicing crop diversification (1.47%), change in planting date (2.36%), and adoption of soil and water conservation practices (1.17%). This implies that the farmers who enjoy better access to climate change information (i.e., seasonal or mid-term forecasting) made better-informed adaptation decisions. These findings were similar to the findings from various studies (Belay et al., 2017; Halagundegowda et al., 2017; Adeagbo et al., 2021; Tshikororo et al., 2021). As expected by the researchers, education and livestock rearing had a positive association across all climate change adaptation strategies. Other determinants like access to market (on crop diversification −1.61% and integrating crop with livestock 1.84%), farm size (on integrating crop with livestock −2.67%), and training (on change in planting date −3.02%) have exerted a significant positive influence on the adoption of climate change adaptation strategies.
Conclusion and policy implications
Climate change is one of the biggest challenges to agriculture, particularly for smallholder farming in developing countries like India. Therefore, it is inevitable for the farmers to plan and resort to different climate change adaptation strategies to stabilize and diversify agricultural production and augment farm income on a sustainable basis. The variables such as off-farm income, farm income, and farming experience emerged as determining factors to adopt CCAS. Thus, policy efforts on these significant variables are to be given high priority to motivate the farmers for practicing climate change adaptation strategies and to instill confidence among the farmers in farming. Moreover, creating off-farm employment/income opportunities for the farmers deserve special attention through rural non-farm activities, which are less sensitive to climate change. The policy implications that emerge from this study are as follows: The role of institutions is crucial in the capacity building of smallholders to safeguard their interests through a wide range of policy, research, and technological interventions. Aspects such as crop diversification, linking farmers to agricultural markets, improving access to climate change information, application of ICTs and artificial intelligence in information dissemination, and knowledge about various climate change adaptation strategies (long-term drought-proofing measures) should be included in the current formal agricultural extension system in the country inculcate spirit in the farming community. In a nutshell, R&D focus on climate-resilient agriculture as well as promoting the development of the non-farm sector in rural areas is crucial to sustaining their livelihoods as these serve as potential mitigation strategies to impart resilience to climate-sensitive sectors like agriculture, and water in rainfed regions of the country.
Data availability statement
The raw data supporting the conclusions of this article will be made available by the authors, without undue reservation.
Ethics statement
Ethical review and approval was not required for the study on human participants in accordance with the local legislation and institutional requirements. Written informed consent from the participants was not required to participate in this study in accordance with the national legislation and the institutional requirements.
Author contributions
KK, MR, and KR conceived the study, sourced and analyzed the data, and wrote the draft manuscript. VP, MB, TK, RK, and DR validated the results, edited the draft, and improved the content. All authors approved the final draft.
Acknowledgments
We thank the financial support provided by ANGRAU, Bapatla, Andhra Pradesh, and PJTSAU, Telangana. The authors are also thankful to ICAR–Central Tobacco Research Institute, Rajahmundry, Andhra Pradesh, and ICAR–Central Coastal Agricultural Research Institute, Old Goa, Goa, India, for providing the necessary facility.
Conflict of interest
The authors declare that the research was conducted in the absence of any commercial or financial relationships that could be construed as a potential conflict of interest.
The handling editor RG declared a past co-authorship with one of the authors KR.
Publisher's note
All claims expressed in this article are solely those of the authors and do not necessarily represent those of their affiliated organizations, or those of the publisher, the editors and the reviewers. Any product that may be evaluated in this article, or claim that may be made by its manufacturer, is not guaranteed or endorsed by the publisher.
Supplementary material
The Supplementary Material for this article can be found online at: https://www.frontiersin.org/articles/10.3389/fsufs.2023.1010527/full#supplementary-material
References
Abdulai, A., and Huffman, W. (2014). The adoption and impact of soil and water conservation technology: an endogenous switching regression application. Land Econ. 90, 26–43. doi: 10.3368/le.90.1.26
Adeagbo, O. A., Ojo, T. O., and Adetoro, A. A. (2021). Understanding the determinants of climate change adaptation strategies among smallholder maize farmers in South-West, Nigeria. Heliyon 7, e06231. doi: 10.1016/j.heliyon.2021.e06231
Agrawala, S., and Lemos, M. C. (2015). Adaptive development. Nat. Clim. Change 5, 185–187. doi: 10.1038/nclimate2501
Almaraz, J. J., Mabood, F., Zhou, A., Gregorich, E. G., and Smith, D. L. (2008). Climate change, weather variability and corn yield at a higher latitude locale: south-western Quebec. Clim. Change 88, 187–197. doi: 10.1007/s10584-008-9408-y
Anik, A. R., Rahman, S., Sarker, J. R., and Al Hasan, M. (2021). Farmers' adaptation strategies to combat climate change in drought prone areas in Bangladesh. Int. J. Disaster Risk Reduct. 65, 102562. doi: 10.1016/j.ijdrr.2021.102562
Aryal, J. P., Sapkota, T. B., Rahut, D. B., Marenya, P., and Stirling, C. M. (2021), Climate risks and adaptation strategies of farmers in East Africa and South Asia. Sci. Rep. 11, 10489, doi: 10.1038/s41598-021-89391-1
Asafu-Adjaye, J. (2008), Factors affecting the adoption of soil conservation measures: a case study of Fijian cane farmers. J. Agric. Resourc. Econ. 33.
Asrat, P., and Simane, B. (2018). Farmers' perception of climate change and adaptation strategies in the Dabus watershed, North-West Ethiopia. Asrat Simane Ecol. Proc. 7, 7. doi: 10.1186/s13717-018-0118-8
Auffhammer, M., and Schlenker, W. (2014), Empirical studies on agricultural impacts and adaptation. Energy Econ. 46, 555–561. Available online at: http://refhub.elsevier.com/S0308-521X(22)00201-3/rf0030,
Barzola Iza, C. L., and Dentoni, D. (2020). How entrepreneurial orientation drives farmers' innovation differential in Ugandan coffee multi-stakeholder platforms. J. Agribus. Dev. Emerg. Econ. 10, 629–650. doi: 10.1108/JADEE-01-2020-0007
Belay, A., Recha, J. W., Woldeamanuel, T., and Morton, J. F. (2017). Smallholder farmers' adaptation to climate change and determinants of their adaptation decisions in the Central Rift Valley of Ethiopia. Agric. Food Secur. 6, 1–13. doi: 10.1186/s40066-017-0100-1
Campbell, B. M., Vermuelen, S. J., Aggarwal, P. K., Corner-Dolloff, C., Givertz, E., Loboguerrerro, A. M., et al. (2016). Reducing risks to food security from climate change. Glob. Food Sec. 11, 34–43. doi: 10.1016/j.gfs.2016.06.002
CIE (2014). Analysis of the Benefits of Improved Seasonal Climate Forecasting for Agriculture, Canberra ACT 2609. The Centre for International Economics.
Connoly-Boutin, L. C., and Smit, B. (2015). Climate change, food security, and livelihood in Sub-Saharan Africa. Reg. Environ. Change 16, 385–399. doi: 10.1007/s10113-015-0761-x
Croppenstedt, A., Knowles, M., and Lowder, S. (2018). Social protection and agriculture: introduction to the special issue. Glob. Food Sec. 16, 65–68. doi: 10.1016/j.gfs.2017.09.006
Das, H. P. (2005). “Agro meteorological impact assessment of natural disasters and extreme events and agricultural strategies adopted in areas with high weather risk,” in: Natural Disaster and Extreme Events in Agriculture, eds M. V. K. Shivakumar„ P. R. Motha, H. P. Das (Berlin; New York, NY: Springer-Verlag), 93–118. doi: 10.1007/3-540-28307-2_6
Diallo, A., Donkor, E., and Owusu, V. (2020). Climate change adaptation strategies, productivity and sustainable food security in southern Mali. Clim. Change 159, 309–327. doi: 10.1007/s10584-020-02684-8
Duong, N. T., Ha, D. T. T., and Ngoc, N. B. (2017). The application of discriminant model in managing credit risk for consumer loans in Vietnamese commercial bank. Asian Soc. Sci. 13, 176–186. doi: 10.5539/ass.v13n2p176
Easterling, W. E., Aggarwal, P. K., Batima, P., Brander, K. M., Erda, L., Howden, S. M., et al. (2007). Food, fibre and forest products. Clim. Change 273–313.
Food and Agriculture Organization. (2011). Crop Prospects and Food Situation (no. 4, December, 2011). Available online at: http://www.fao.org/docrep/014/al983e/al983e00.pdf
Gornall, J., Betts, R., Burke, E., Clark, R., Camp, J., Willett, K., et al. (2010). Implications of climate change for agricultural productivity in the early twenty-first century. Philos. Trans. R. Soc. B Biol. Sci. 365, 2973–2989. doi: 10.1098/rstb.2010.0158
Government of India (2017). “Climate, climate change, and agriculture,” in Economic Survey. New Delhi: Ministry of finance, Government of India, 96–97.
Halagundegowda, G. R., Singh, A., and Meenakshi, H. K. (2017). Discriminant analysis for prediction and classification of farmers based on adoption of drought coping mechanisms. Agric. Update 12, 635–664. doi: 10.15740/HAS/AU/12.TECHSEAR(3)2017/635-640
IPCC (2013). The Physical Science Basis: Working Group I Contribution to the Fifth Assessment Report of the Intergovernmental Panel on Climate Change 1. New York, NY: Cambridge University Press, 535–541.
IPCC. (2012). “Managing the risks of extreme events and disasters to advance climate change adaptation,” in A Special Report of Working Groups I and II of the Intergovernmental Panel on Climate Change, eds C. B. Field, V. Barros, T. F. Stocker, D. Qin, D. J. Dokken, K. L. Ebi, M. D. Mastrandrea, K. J. Mach, G. -K. Plattner, S. K. Allen, M. Tignor, and P. M. Midgley (Cambridge; New York, NY: Cambridge University Press), 1–582.
IPCC. (2014). “Climate change 2014: Mitigation of climate change,” in Contribution of Working Group III to the Fifth Assessment Report of the Intergovernmental Panel on Climate Change, eds O. R. Edenhofer, R. Pichs-Madruga, Y. Sokona, E. Farahani, S. Kadner, K. Seyboth, A. Adler, I. Baum, S. Brunner, P. Eickemeier, B. Kriemann, J. Savolainen, S. Schlömer, C. von Stechow, T. Zwickel, and J. C. Minx (Cambridge; New York, NY: Cambridge University Press).
Khanal, A. R., and Mishra, A. K. (2017). Enhancing food security: food crop portfolio choice in response to climatic risk in India. Glob. Food Sec. 12, 22–30. doi: 10.1016/j.gfs.2016.12.003
Khemakhem, S., and Boujelbene, Y. (2015).Credit risk prediction: A comparative study between discriminant analysis and the neural network approach. Account. Manag. Inform. Syst. 14, 1–60.
Kibue, G. W., Liu, X., Zheng, J., Pan, G., Li, L., and Han, X. (2016). Farmers' perceptions of climate variability and factors influencing adaptation: evidence from Anhui and Jiangsu, China. Environ. Manage. 57, 976–986. doi: 10.1007/s00267-016-0661-y
Kosoe, E. A., and Ahmed, A. (2022), Climate change adaptation strategies of cocoa farmers in the Wassa East District: implications for climate services in Ghana. Clim. Serv. 26, 100289. doi: 10.1016/j.cliser.2022.100289
Kumari, M., Singh, K. M., Mishra, R. R., Sinha, D. K., and Ahmad, N. (2017). Role of socio-economic variables in adoption of crop insurance: a discriminant function approach. Econ. Affairs 62, 361–365. doi: 10.5958/0976-4666.2017.00045.6
Lobell, D. B., Schlenker, W., and Costa-Roberts, J. (2011). Climate trends and global crop production since 1980. Science. 333, 616–620. doi: 10.1126/science.1204531
Lotze-Campen, H., and Schellnhuber, H. J. (2009). Climate impacts and adaptation options in agriculture: what we know and what we don't know. J. Verbr. Lebensm. 4, 145–150. doi: 10.1007/s00003-009-0473-6
Malek, K., Adam, J. C., Stöckle, C. O., and Peters, R. T. (2018). Climate change reduces water availability for agriculture by decreasing non evaporative irrigation losses. J. Hydrol. 561, 444–460.
Mendelsohn, R., Basist, A., Kurukulasuriya, P., and Dinar, A. (2006). Climate change and rural income. Clim. Change 81, 101–118. doi: 10.1007/s10584-005-9010-5
Misra, A. K. (2012). Climate change impacts, mitigation and adaptation strategies for agricultural and water resources, in Ganga plain (India). Mitigat. Adapt. Strat. Glob. Change 18, 673–689. doi: 10.1007/s11027-012-9381-7
Misra, A. K. (2014). Climate change and challenges of water and food security. Int. J. Sustain. Built Environ. 3, 153–165. doi: 10.1016/j.ijsbe.2014.04.006
Mjelde, J. W., Sonka, S. T., and Pell, D. S. (1989). The Socioeconomic Value of Climate and Weather Forecasting: A Review. Midwestern Climate Center, Climate & Meteorology Section Illinois State Water Survey.
Motha, R. P., and Murthy, V. R. K. (2007). “Agrometeorological services to cope with risks and uncertainties,” in Managing Weather and Climate Risks in Agriculture, eds V. K. Sivakumar and R. P. Motha (Berlin; Heidelberg: Springer), 435–462.
Mulwa, C., Marenya, P., and Kassie, M. (2017). Response to climate risks among smallholder farmers in Malawi: a multivariate probit assessment of the role of information, household demographics, and farm characteristics. Clim. Risk Manag. 16, 208–221. doi: 10.1016/j.crm.2017.01.002
Mushore, T. D., Mhizha, T., Manjowe, M., Mashawi, L., Matandirotya, E., Mashonjowa, E., et al. (2021). Climate change adaptation and mitigation strategies for small holder farmers: a case of Nyanga District in Zimbabwe. Front. Clim. 3, 676495. doi: 10.3389/fclim.2021.676495
Mwinkom, F. X., Damnyag, L., Abugre, S., and Alhassan, S. I. (2021), Factors influencing climate change adaptation strategies in North-Western Ghana: evidence of farmers in the Black Volta Basin in Upper West region. SN Appl. Sci. 3, 548. doi: 10.1007/s42452-021-04503-w
Nabikilo, D., Bashaasha, B., Mangheni, M. N., and Majaliwa, J. G. M. (2012). Determinants of climate change adaptation among male and female headed farm households in Eastern Uganda. Afr. Crop. Sci. J. 20, 203–212.
Narain, S., Ghosh, P., Saxena, N., Parikh, J., and Soni, P. (2009). Climate Change—Perspectives From India. United Nations Development Programme (UNDP).
Ndamani, F., and Watanabe, T. (2016). Determinants of farmers' adaptation to climate change: A micro level analysis in Ghana. Agrometeorol. Sci. Agric. 73, 201–208. doi: 10.1590/0103-9016-2015-0163
Nelson, G. C., Rosegrant, M. W., Koo, J., Robertson, R., Sulser, T., Zhu, T., et al. (2009). Climate Change: Impact on Agriculture and Costs of Adaptation. Washington, DC: International Food Policy Research Institute–IFPRI.
Niles, M. T., Brown, M., and Dynes, R. (2016). Farmer's intended and actual adoption of climate change mitigation and adaptation strategies. Clim. Change 135, 277–295. doi: 10.1007/s10584-015-1558-0
Ogen, O. (2007). The Agricultural Sector and Nigeria's Development: Comparative Perspectives From the Brazilian Agro-industrial Economy. Nebula, 1960–1995.
Ojo, T. O., and Baiyegunhi, L. J. S. (2019). Determinants of climate change adaptation strategies and its impact on the net farm income of rice farmers in south-west Nigeria. Land Use Policy 95, 103946. doi: 10.1016/j.landusepol.2019.04.007
Pino, G., Toma, P., Rizzo, C., Miglietta, P., Peluso, A., and Guido, G. (2017). Determinants of farmers' intention to adopt water saving measures: evidence from Italy. Sustainability 9, 77. doi: 10.3390/su9010077
Piya, L., Maharjan, K. L., and Joshi, N. J. (2013). Determinants of adaptation practices to climate change by chepang households in the rural mid-Hills of Nepal. Reg. Environ. Change 13, 437–447. doi: 10.1007/s10113-012-0359-5
Reidsma, P., Ewert, F., Lansink, A. O., and Leemans, R. (2009). Vulnerability and adaptation of European farmers: a multi-level analysis of yield and income responses to climate variability. Reg. Environ. Change 9, 25–40. doi: 10.1007/s10113-008-0059-3
Rosenzweig, C., and Tubiello, F. N. (2007). Adaptation and mitigation in agriculture: an analysis of potential synergies. Mitig. Adapt. Strat. Glob. Change 12, 855–873. doi: 10.1007/s11027-007-9103-8
Shrestha, S., and Bui, T. T. T. (2015). Assessment of climate change impacts and evaluation of adaptation measures for paddy productivity in Quangnam province, Vietnam. Paddy Water Environ. 13, 241–253. doi: 10.1007/s10333-014-0434-2
Shrestha, U. B., Gautam, S., and Bawa, K. S. (2012). Widespread climate change in the Himalayas and associated changes in local ecosystems. PLoS ONE. 7, 36741. doi: 10.1371/journal.pone.0036741
Singh, N. P., Anand, B., Singh, S., and Khan, A. (2019). Mainstreaming climate adaptation in Indian rural developmental agenda: a micro-macro convergence. Clim. Risk Manage. 24, 30–41. doi: 10.1016/j.crm.2019.04.003
Sivakumar, M. V. K. (2011). “Operational agro meteorological strategies in different regions of the world,” in Challenges and Opportunities in Agrometeorology, eds S. D. Attri, L. S. Rathore, M. V. K. Sivakuma, and S. K. Dash, editors (Berlin; New York, NY: Springer-Verlag), 551–571. doi: 10.1007/978-3-642-19360-6_43
St Clair, S. B., and Lynch, J. P. (2010). The opening of Pandora's Box: climate change impacts on soil fertility and crop nutrition in developing countries. Plant Soil. 35, 101–115.
Tang, X., Li, J., Ma, Y., Hao, X., and Li, X. (2008). Phosphorus efficiency in long-term (15 years) wheat-maize cropping systems with various soil and climate conditions. Field Crops Res. 108, 231–237. doi: 10.1016/j.fcr.2008.05.007
Tesfahunegn, G., Mekonen, K., and Tekle, A. (2016). Farmers' perception on causes, indicators and determinants of climate change in northern Ethiopia: implication for developing adaptation strategies. Appl. Geogr. 73, 1–12. doi: 10.1016/j.apgeog.2016.05.009
Tshikororo, M., Thaba, K., Nefale, T. A., and Tshikororo, M. (2021). Perception driven from farmers' socio-economic characteristics towards tackling climate change. J. Agric. Sci. 13, 41–47. doi: 10.5539/jas.v13n6p41
Turpie, J., and Visser, M. (2013). The impact of climate change on South Africa's rural areas. Fin. Fiscal Comm. 14, 100–160.
Uddin, M. S., Shah, M. A. R., Khanom, S., and Nesha, M. K. (2013). Climate change impacts on the Sundarbans mangrove ecosystem services and dependent livelihoods in Bangladesh. Asian J. Conserv. Biol. 2, 152–156. Available online at: https://www.ajcb.in/journals/full_papers_dec_2013/AJCB-Vol2-No2-Shams-Uddin%20et%20al.pdf
Verchot, L. V., Noordwijk, M. V., Kandji, S., Tomich, T., Ong, C., Albrecht, A., et al. (2007). Climate change: linking adaptation and mitigation through agroforestry. Mitigat. Adapt. Strateg. Glob. Chang. 12, 901–918.
Von Braun, J., Swaminathan, M. S., and Rosegrant, M. W. (2005). Agriculture, Food Security, Nutrition and the Millennium Development Goals. International Food Policy Research Institute.
Keywords: climate change, climate resilience, rainfed ecosystem, small farm holders, sustainability
Citation: Kumar KNR, Reddy MJM, Reddy KV, Paramesha V, Balasubramanian M, Kumar TK, Kumar RM and Reddy DD (2023) Determinants of climate change adaptation strategies in South India: Empirical evidence. Front. Sustain. Food Syst. 7:1010527. doi: 10.3389/fsufs.2023.1010527
Received: 03 August 2022; Accepted: 03 January 2023;
Published: 15 February 2023.
Edited by:
Rupak Goswami, Ramakrishna Mission Vivekananda Educational and Research Institute, IndiaReviewed by:
Sanjit Maiti, National Dairy Research Institute (ICAR), IndiaUttam Khanal, Tribhuvan University, Nepal
Copyright © 2023 Kumar, Reddy, Reddy, Paramesha, Balasubramanian, Kumar, Kumar and Reddy. This is an open-access article distributed under the terms of the Creative Commons Attribution License (CC BY). The use, distribution or reproduction in other forums is permitted, provided the original author(s) and the copyright owner(s) are credited and that the original publication in this journal is cited, in accordance with accepted academic practice. No use, distribution or reproduction is permitted which does not comply with these terms.
*Correspondence: K. Viswanatha Reddy, vishu.uas@gmail.com; Venkatesh Paramesha,
parameshagron@gmail.com