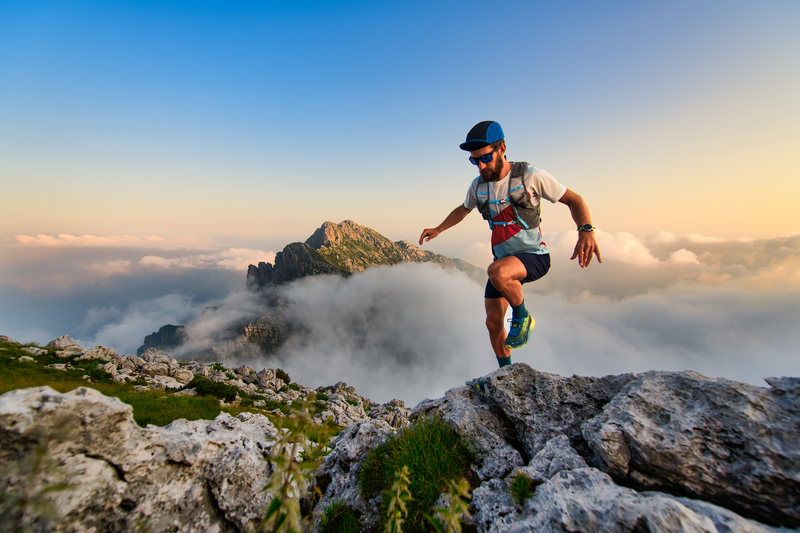
95% of researchers rate our articles as excellent or good
Learn more about the work of our research integrity team to safeguard the quality of each article we publish.
Find out more
ORIGINAL RESEARCH article
Front. Sustain. Food Syst. , 26 October 2022
Sec. Social Movements, Institutions and Governance
Volume 6 - 2022 | https://doi.org/10.3389/fsufs.2022.997549
International food chains and trade usually support many vulnerable and food-insecure developing countries to ensure stable access to food and sufficient resources to maintain and enhance economic growth. However, the smooth transition of uninterrupted food trade and supply is one of the major concerns for almost every country. Based on the economic threshold model grouping tactics, the study evaluates the influencing factors of global food imports and how different countries foster food imports in different conditions. The empirical data has been comprised of panel data from 91 countries along the “Belt and Road” for the last 21 years. The results show that: (i) Population size and arable land endowment have single and double threshold effects on food imports. (ii) Economic development has different effects on the food imports of each group of countries. It has an inverted U-shaped relationship with countries with large populations and high arable land endowments and a “U-shaped relationship” with countries with low arable land endowments. There is a linear relationship between the food imports of countries with small populations and medium arable land endowments, and there is no significant impact on food imports of countries with large populations and medium arable land endowments. (iii) The impact of infrastructure, technological progress, food stocks, and industrial structure on food imports varies from country to country, but tariff policies have no significant impact on food imports. All member countries should utilize the platform of “Belt and Road Initiatives” to capture the resource endowment and exchange associated science and technology of food production, processing, transport, and storage. Food productivity and self-dependency on food should also be increased.
The food supply chain comprises all the stages that food products go through, from production to consumption activities including how foods are produced, processed, distributed, consumed, and disposed of (Marsden et al., 2000). With the rapid development of the global economy, there is a clear change in food demand patterns triggering consumers to move from the initial food and clothing to balanced and diverse food types (Vorst, 2000). Nowadays, food is transported over longer distances, across continents (Nguyen, 2018). Therefore, the international trade of food becomes more extensive, and almost every country needs to import food from other countries to meet domestic demand (Ahumada and Villalobos, 2009). According to Food and Agriculture Organization of the United Nations (FAO) data, from 2000 to 2016, the total global food imports increased from 214 million tons to 378 million tons, an average annual increase of 2.59% (Smyth et al., 2016). As the world's most populous agricultural country, China has created a miracle of supporting nearly 20% of the world's population with less than 9% of its arable land (Cui and Shoemaker, 2018). However, to ease domestic pressure regarding diverse food in recent years, China is also one of the top countries in food imports (Liu et al., 2021). For a long since, several continents have operated some regional cooperation for food, technology, and other necessary trading relations. Improving the regional organization of food flow requires well-structured and long-term cooperation among the associated parties. It is also required for facilitating an equitable and sustainable manner of food supply.
China has a long history of forming specialized organizations from ancient maritime Silk Road initiatives to the “One Belt One Road (OBOR)” policy or “Belt and Road Initiatives (BRI).” In 2013, China proposed a belt and road initiative to open up the international market, deepen international cooperation and increase the source of China's food imports (Erokhin and Gao, 2018; Wang et al., 2021). At the same time, the initiative also provides opportunities for the participating countries to carry out socio-economic exchanges and food trade, helping each other to solve food security issues, eliminate regional poverty, and jointly build “a community with a shared future for mankind” (Chen et al., 2018). According to the World Bank and the United Nations Food and Agriculture Organization's database, the “Belt and Road initiatives” is the most important food production area globally, and the output of the food accounts for more than 40% of the world's total output (Syed and Ying, 2019). However, these countries have low self-sufficiency and a high import dependence rate on food (Hughes et al., 2020). The increasing number of member countries of BRI has expanded the scope of food trade with more convenient channels, but the increasing dependence on food imports in countries means that the stability of the food supply is vulnerable to the international market (Sternberg et al., 2020). Therefore, it is necessary to accurately grasp the determinants of food imports and their long-term evolution trends, which will help countries along the route, adopt targeted macro-control policies to ensure food supply and ensure food security in countries along with BRI.
Extensive research can be found on the influencing factors of food import trade are more extensive (Gürkan et al., 2003; Erokhin, 2017; Mwangi, 2021), which can be summarized as economic growth, international markets, and domestic resource endowments (Knight et al., 2007b; Atif et al., 2017; Suanin, 2021). In terms of economic growth, the existing literature established the following assumptions: economic development has a positive impact on food imports (Pingali, 2007; Norton et al., 2021), and the level of economic development is negatively correlated with food imports (Fukase and Martin, 2020; Ren et al., 2021). While different stages of economic development will have varying degrees of impact on food imports, an “inverted U-shaped” curve law has presented between economic growth and food imports (Abay et al., 2020). In terms of international market factors, existing studies have shown that both the exchange rate and international food prices have a negative impact on food imports (Ikuemonisan et al., 2018). In terms of domestic resource endowments, population growth can be an important reason for the significant growth of the food trade in the future (Schneider et al., 2011). It is also consistent with the research conclusions of some related studies (for e.g., Tukker et al., 2016; Dorninger et al., 2021, and Qingjie, 2021). In addition, some scholars pointed out that the difference in factor endowments between different countries is the main factor affecting the food trade (Koo and Kennedy, 2005; Li, 2012; Abad, 2013). The advancement of science and technology fostered by infrastructure development has saved both parties' trade costs and promoted the development of the food trade (Geng et al., 2007). Some studies also found that the endowment of cultivated land, water resources, food yield per capita, and per capita food consumption are the main factors affecting food imports (Maslak et al., 2020; Han and Li, 2021).
In recent years, the existing literature regarding food import and trade has become matured. However, a huge gap in research triggers a unified theoretical framework and empirical guidance within this crucial field. Especially the empirical research on the food trade of the BRI countries is still not explored sufficiently. Countries along with the BRI have large gaps in economic development levels and different resource endowments. Most of the existing studies have homogenized countries, ignoring the heterogeneity of different economies in terms of institutional environment and resource structure (Shevchuk, 2014; Sertoglu and Dogan, 2016; Mwangi, 2021). A specific “category” or a country with a certain “characteristic” may alter the whole patterns of food imports. Therefore, the role of the classic trade theory cannot be brought into full play. In response to the above shortcomings, this article describes the food-importing countries along BRI with different characteristics in specific groups by employing a panel threshold model. Likewise, the study evaluates the impact of different countries' food imports based on clarifying goal test models for different groups combined with actual economic backgrounds. The evaluation of factors affecting food trade and import will provide baseline references for countries with different characteristics to formulate food security strategies. The study will be one of the first attempts to explore food imports and trade factors within BRI countries.
The factors affecting grain import can be assessed from the two aspects of demand and supply (Fader et al., 2013). When a country's demand exceeds supply, grain import will become an important supply channel to meet its grain demand. Likewise, when demand is less than supply, it will be converted to grain export or food reserves (Porkka et al., 2017). However, the level of economic development determines the structure of grain demand and when the level of economic development is low, the demand for traditional grain crops such as wheat, corn, soybean, and rice is large, and the total demand for grain imports is also large (Mostenska et al., 2022). With the continuous development of the economy, the adjustment of the food consumption structure has been accelerated, the consumption of rations of these grains has dropped significantly, and the consumption of non-staple food has increased significantly (Rush and Obolonkin, 2020). The food consumption pattern has begun to be dominated by non-staple food, and the demand for grain imports has gradually changed. When the economy grows to a certain level, the purchasing power of the population is also continuously expanding, arable land resources are gradually scarce, and the industrial structure gradually changes dominated by industry and service industries (Distefano et al., 2018). At this time, the further upgrade of the dietary structure will drive the growth of food demand, which will lead to the total import of food (Attrey, 2017).
Conversely, population growth can bring about an almost proportional increase in demand (van Dijk et al., 2021). The larger population also possesses a higher demand for varieties of food, and the dependence on food imports will gradually intensify. In addition, constrained by the endowment of cultivated land, under certain agricultural production conditions and production technologies, there is an extreme value of land output (Zhao et al., 2021). Therefore, the endowment of cultivated land is one of the most important factors in determining a country's grain output. The richer the cultivated land resources, the more helpful to meet the food needs of the population, thereby reducing food imports (Lau et al., 2018; Tian et al., 2020). Similarly, various studies have shown that infrastructure is necessary and a prerequisite for smooth trade (Bougheas et al., 1999; Limão and Venables, 2001; Donaubauer et al., 2018). The level of infrastructure has a significant negative impact on transportation costs, which in turn has a significant negative impact on import and export trade volumes. The development of trade infrastructure among countries along the “Belt and Road” is relatively lagging, which restricts the development of trade and the economic development of countries along the route (Limão and Venables, 2001).
Similarly, international grain prices have a very important impact on grain imports. According to international trade theory, the price has a direct and significant impact on trade quantity and share (He et al., 2016). When international food prices rise, a government will reduce the number of food imports, relieve the pressure of food shortages by releasing food stocks, and at the same time, appropriately increase food exports to obtain the highest economic benefits. Grain stocks play an important role in maintaining national food security, when the level of grain production increases, a government can increase exports and increase stock for grain sales or strategic grain reserves (Huang et al., 2017). When frequent natural disasters, wars, and other reasons lead to a reduction in grain production and a decline in global grain reserves, it is easy to cause international grain prices to rise in certain years. According to the theory of economic supply and demand, an increase in international grain prices will inevitably lead to a decrease in demand (Karbekova et al., 2022). Since the demand for grain is rigid demand, when a country reduces its grain imports due to rising international grain prices (Zhan, 2021; 1949–2019), its government usually responds to the impact of rising international grain prices by releasing grain stocks, to alleviate the external dependence on grain and reduce the purposes of food imports (Meloni and Swinnen, 2022).
Agricultural modernization may also play an important role in rectifying any country's agroeconomic development and influences the level of food imports (Liu and Zhou, 2021). The higher the level of agricultural modernization, the higher the efficiency of grain production, which is more conducive to increasing grain output (Liu and Zhou, 2021). At the same time, it is beneficial to save costs, improve the comparative and competitive advantages of agriculture, and thus play a role in stabilizing grain imports. Luo and Tanaka (2021) pointed out that grain import can also stimulate domestic supply and technological progress in reverse, which is helpful to improve grain output and quality. Seemingly, the industrial structure mainly represents the economic structure such as the proportion of agricultural industry and the intensity of agricultural resource elements (Bozsik et al., 2022). Eventually, countries with agriculture as the mainstay of the national economy have a low level of economic development and a large demand for food. Only by expanding food imports and adjusting the agricultural structure to supplement the shortage of domestic food production can the problem of insufficient food supply be solved (Lu et al., 2022). Based on the above assumptions the study outlines its theoretical framework as shown in Figure 1.
The core economic activities such as the import and export of agricultural products are usually interlinked with several decisive factors such as socioeconomic conditions, local industry structure, consumption patterns, and governmental policy directions (Mwangi, 2021), and those factors are largely influenced by other several mediating and external factors (Bui and Chen, 2017). Therefore, it is challenging to explore how and to what extent the primary and secondary factors are interconnected. To comprehensively evaluate the relationship between the influencing factors, and identify the most important influencing factors can be determined by the multiple correlation analysis between those associated factors (Okpe and Ikpesu, 2021). Therefore, the study utilized the multivariate non-linear regression model to observe the non-linear relationship between the level of economic development and the total amount of grain imports. The multiple non-linear regression model refers to the regression model of a dependent variable (grain import volume) and multiple independent variables (economic development level, population size, arable land resource endowment, infrastructure level, etc.). Several studies with similar setups (Okolo and Obidigbo, 2015; Ivanova et al., 2018; Maaouane et al., 2021) have advocated multiple regression analysis as it can accurately measure the degree of correlation between various factors and the degree of regression fit. However, according to Majumdar et al. (2017), it is more suitable for actual economic problems and is used when it is affected by multiple factors. The specific steps of the analysis are as follows: first, the multiple regression model is constructed, and the factors which are analyzed in theory are put into the model. After that, we divide the 91 countries into five groups according to their resource endowment and population size by using the threshold panel model, and the stationarity of the model is tested. And finally, carry out multiple regression in groups and analyze the results.
Belt and Road Initiatives involve a total of 139 countries (Shakib et al., 2021). However, among them, the study selects 91 countries' panel data ranging from 1996 to 2016 (21 years) due to the limitations and availability of the associated data. The main reason for excluding the other country is the unavailability of full data set of those countries' food imports. The basic data needed for the research are extracted from the FAOSTAT database (https://www.fao.org/faostat) of the United Nations Food and Agriculture Organization (FAO), the United Nation's Commodity Trade Statistics database (https://comtrade.un.org), the World Bank's World Development Index (https://datatopics.worldbank.org/world-development-indicators/) and the United States Department of Agriculture (https://www.usda.gov). Moreover, some supplementary data are derived from several associated countries' government databases, reports, and agendas.
Total food imports (Impit) has been derived from the total amount of food imports in various countries, including 15 categories of wheat, rice, corn, barley, rye, oats, biscuits, sorghum, and other forms of grain.
Food imports are mainly determined by domestic supply and demand. Among them, the national economic development level and population size are the key factors that affect demand. Cultivated land endowments, food stocks, technological progress, and industrial structure are the main factors that affect supply. In addition, infrastructure (de Lima et al., 2018; Chernova and Noha, 2019), tariff policies (Elsheikh et al., 2015; Mgeni et al., 2018), and international food prices (McMichael, 2000; Clapp, 2009) can also have a significant impact on food imports. This article sets up explanatory variables from the above aspects and compiles them in Table 1.
The impact of economic levels on food imports is a dynamic process (Williams et al., 2017; Jaworska, 2018). When economic development is low, people's dietary structure is relatively simple (Pedersen et al., 2018; Olayungbo, 2021), and there is a large demand for traditional ration crops and low-income limits on imports, which can only be achieved by maximizing domestic production levels to meet demand (Knudsen et al., 2006). With economic growth, the industrial structure has gradually changed from being dominated by agriculture to being dominated by industry and service industries (Gandhi and Zhou, 2014; Alrobaish et al., 2021). The further upgrade of the dietary structure has driven food demand growth, surpassing the domestic production rate, resulting in a rise in total food imports (Bakari and Mabrouki, 2017). When the economy continues to grow, the country will invest more in agricultural technology research, improve the food-planting environment, and increase the efficiency of arable land production (Porkka et al., 2017). At this time, domestic food production will continue to increase, ensuring national demand and food imports may decrease. After this stage, urbanization, industrialization, occupation of arable land, and over-utilization of arable land may lead to insufficient food supply again, and imports may increase again at this time (Knight et al., 2007a). Therefore, economic development may non-linearly affect food imports. The larger the population, the higher the ability to consume food, and the greater the dependence on food imports (Mercken et al., 2020).
Under certain agricultural production conditions and technologies, the richer cultivated land resources are, the more helpful it is to meet the population's food needs, reducing food imports (Kummu et al., 2012). Food stocks play an important role in safeguarding national food security (Wright and Cafiero, 2011). When the international food market is turbulent, stocks can meet domestic demand and reduce imports. The main manifestation of scientific and technological progress in food production is known as agricultural modernization. The improvement of agricultural modernization will drive the improvement of food production efficiency, help increase food production, and at the same time help save costs, and play a role in stabilizing food imports (Prosekov and Ivanova, 2018; Müller et al., 2020). A well-developed infrastructural system is necessary for unconstrained trade, and it can negatively impact transportation costs significantly. Transportation costs have a significant negative impact on import and export trade [59, 60], so well-structured infrastructure may positively affect food imports. Likewise, the industrial structure mainly refers to the economic structure, such as the proportion of agricultural industry and the intensity of agricultural resource elements. Generally, countries with lower agricultural development via modern technology and innovation are lagging in food production efficiency (Abdul-Rahaman et al., 2021; Konieczna et al., 2021). Therefore, food imports need to supplement domestic food production. The role of tariff policy is to raise the price of imported food through taxation, reduce its market competitiveness, reduce the adverse impact on domestic products in the market (Yu and Jensen, 2005; Mousavi and Esmaieli, 2011), and help protect domestic food from the impact of foreign food market prices (Zhao et al., 2021), which has a negative impact on food imports.
Considering that there may be a non-linear relationship between some variables and food imports, and the economic threshold model can more accurately explore this relationship between variables, the study adopted the theory and practice of “Threshold Autoregression” proposed by Hansen (Hansen, 2011) and built the following single threshold panel model to explore whether each variable has a threshold value:
If there are multiple thresholds, the formula (1) can be transformed into a multi-threshold panel model:
Whereas, β1, β2 denoting each coefficient to be estimated in different sections of the threshold variable, qit is a variable threshold, μit unknown threshold, εit the individual fixed effects of disturbance term , and I(•) denotes the indicator function. Its object has been set according to the sample segmenting threshold.
The study sets the following benchmark equation to explore the factors affecting food imports in various countries:
Where Impit is the food import volume of the ith country in tth year; Pgdpit is the GDP per capita of the ith country in tth year. Given the possible non-linear relationship between the level of economic development and the total amount of food imports, the following steps have been taken: (i) In the setting of the regression model, first set the cubic curve form; (ii) if the cubic curve form is not significant, remove the cubic term, and then check the quadratic form; (iii) and finally if the quadratic form is still insignificant, remove the quadratic term. In order to reduce the heteroscedasticity, each index adopts the natural logarithm form and is presented in Table 1.
In order to overcome the arbitrariness and subjectivity of the traditional grouping standard, the study uses the threshold effect model to identify the endogenous characteristics of the data automatically. It uses the correlation coefficient between the explained variable and its influencing factors to determine the threshold value instead of exogenous grouping to divide the sample effectively, as suggested by Savvides and Stengos (Savvides and Stengos, 2000) and Masters and McMillan (Masters and McMillan, 2001). The correlation coefficient matrix in Table 2 shows that the top factors influencing food imports are population size, food inventory level, infrastructure level, arable land endowment, and economic development level. Through the correlation between variables, it can be found that the correlation coefficients of per capita GDP are −0.395 and 0.354, respectively. Seemingly, the correlation coefficients of infrastructure and per capita GDP are 0.771, and the correlation coefficients of economic development level, population size, and cultivated land endowment are −0.351 and −0.304, respectively. Finally, the population size and cultivated land endowment are selected as the threshold grouping variables, as suggested by Tian et al. (2020).
Table 3 reports the test results with population size and cultivated land endowment as the threshold based on the threshold regression method. The results show that the population size is single.
Table 4 represented the grouping results of threshold regression of the selected countries. The threshold rejects the null hypothesis at a significance level of 10% and indicates that there is a significant difference between β1; β2 in formula (1), and the threshold effect is significant. While the dual threshold is not significant, indicating that there is no dual-threshold effect. Therefore, the single threshold estimated by the population size and the value is 16.553. Similarly, there is a significant double threshold effect for cultivated land endowment, and the double threshold values are −0.505 and −0.175. Therefore, the countries along the investigated countries can be divided into two groups according to the population size: “large” (1,544.506 and +∞) and “small” (0 and 1,544.506); according to the endowment of cultivated land, they can be classified as “high” (0.839 and +∞), “medium” (0.603 and 0.839), and “low” (0 and 0.603).
The threshold panel model generally requires that each explanatory variable be a stationary variable. To avoid spurious regression and ensure that the estimation results are valid, the stationarity of each panel sequence must be tested (Table 5). According to the LLC and ADF test results, only “lnPop, lnAst, lnPcland, and lnInfra” in group 1 significantly rejected the null hypothesis that there is a unit root, and none of the other variables could be rejected. The first-order difference of each variable finds that most variables reject the null hypothesis at a significance level of 1%, and the sequence is a first-order single integer sequence. Similarly, each variable in group 3, group 4, and group 5 is the first-order difference.
Since each variable is a first-order single-integration sequence, various indicators may have a long-term stable cointegration relationship. Therefore, the Cointegration test might be useful to evaluate the dataset as suggested by Westerlund and Edgerton (2007) and Leitão and Lorente (2020). In the article, the Kao cointegration test (Kao, 1999) determines whether there is a significant relationship between variables. It might be an effective test option for international trade economics study, as suggested by Iheonu et al. (2021) and Essandoh et al. (2020). Table 6 showed that three items are presented in group 1 at the 5% significance level. Therefore, the null hypothesis cannot be rejected (the null hypothesis is a non-existent Cointegration relationship). However, the cointegration test results of the quadratic term reject the null hypothesis as it does not fit in any group, indicating a long-term stable equilibrium relationship among the variables in the group and the regression residuals of the equation are stable (Bujang et al., 2013).
This article uses a combination of the F-test and Hausman test and found that Group 1 and Group 3 should use the random-effects model (RE). In statistics, a random-effects model, also called a variance components model, is a statistical model where the model parameters are random variables (Bell and Jones, 2015). However, Group 2 only contains Ukraine, and therefore, we use the Ordinary least-squares (OLS) model for regression. In statistics, ordinary least squares (OLS) is a linear least-squares method for estimating the unknown parameters in a linear regression model (Oh, 2001). Finally, as many countries represent Group 4 and Group 5, we use the fixed-effects models (FE). A fixed-effects model is a statistical model whose parameters are fixed or non-random quantities (Nicita, 2013). The regression results are shown in Table 7 and outlined as follows:
i. Countries with “large populations and highly cultivated land endowments” are represented as group 1 (Kazakhstan and Russia). Their population size has a significant positive impact on food imports. If the population size increased by 1%, then the number of food imports increased by 2.821%, and the number of food imports increased by 2.821%. The primary and secondary terms of GDP per capita have significant positive and negative effects on food imports, respectively, indicating an “inverted U-shaped” relationship between the level of economic development and the number of food imports. The volume of food imports showed a trend of rising first and then falling. According to calculations, the inflection point value is 8.744, and the corresponding GDP per capita is US$6,272.937. In 2016 Kazakhstan and Russia's per capita GDP crossed the “inverted U-shaped” type inflection point, with future food imports will show a downward trend in economic growth potential. Per capita arable land area and industrial structure positively affect food imports at the 1% significant level. When the Per capita arable land area increased by 1%, the food imports increased by 2.68%. Seemingly, the proportion of agricultural added value increased by 1%, and food imports increased by 1.092%. The reason is that the higher the proportion of agricultural added value in GDP and the greater the proportion of agriculture in the overall national economic development, the backward development of industrialization, the level of scientific and technological progress and infrastructure, and the output of the low food. Seemingly, it is necessary to import different categories of food and ensure the balance of domestic supply and demand. Food stocks have a significant negative impact on food imports. When stocks increase by 1%, imports will decrease by 0.073%. The reason is that stocks play a key role in regulating the gap between supply and demand. When stocks are abundant, domestic food demand can be met by releasing stocks, thereby reducing imports. However, infrastructure, tariff policies, international food prices, and technological progress significantly impact food imports.
ii. Countries with a “large population and medium endowment of arable land” (group 2). This group only includes Ukraine. The results show that the level of infrastructure, technological progress, and food stocks all significantly impact the country's food imports. The infrastructure level has increased by 1%, and food imports have increased by 0.178% due to the decrease in infrastructure level. Reduce the cost of food transportation, which promotes food imports. A 1% increase in the level of science and technology and a decrease of 0.650% in food imports indicate that technological advancement can improve the efficiency of food production and improve the comparative advantage and competitive advantage of agriculture, thereby reducing food imports and their impact. When food stocks increased by 1%, the food imports decreased by 0.568%, indicating that stocks can be combined with production to meet domestic demand to a certain extent, reducing the need for imports.
iii. Countries with a “small population and medium endowment of cultivated land” (group 3). This group only includes two countries, Uruguay and Lithuania. However, there is no inverted “U-shaped” or inverted “N-shaped” relationship between the level of economic development and food imports (the second and third tests reject the original hypothesis). It indicates there is a linear relationship between the two. The level of economic development has a positive impact on food imports, with per capita GDP increasing by 1% and food imports by 2.274%. While food stocks still have a significant negative impact on food imports. The impact of other variables on Uruguay and Lithuania is not significant.
iv. Countries with a “large population and low cultivated land endowment” (group 4). This group includes 38 countries, including China, Algeria, Bangladesh, Myanmar, and so on. The results show that other variables significantly impact the country's food imports in addition to infrastructure and tariff policies. Among them, there is a significant “inverted N-shaped” relationship between the level of economic development and the number of food imports. The two inflection points are −17.027 and 5.694, respectively. However, the per capita GDP in the “inverted N-shaped” curve has practical significance when greater than 0, so it is a “U-shaped” curve. The analysis found that only Ethiopia, Myanmar, and Mozambique did not cross the threshold in some years, and the rest of the countries have entered the downward range of the “U-Shaped” curve. Therefore, the economic growth of those countries will continue to reduce the total amount of food imports. In addition, population size and industrial structure have a significant positive impact on food imports. Cultivated land endowments, international food prices, technological progress, and food stocks all significantly negatively impact food imports.
v. Countries with “small population size and low cultivated land endowments” (group 5). This group includes 48 countries, including Albania, Austria, and Bahrain. The results show that the population size significantly impacts this group's countries' food imports. If the population size increased by 1%, it increased the food imports by 1.119%. While, there is a significant “U–shaped” relationship between the level of economic development and food imports with an inflection point of US$ 2,371.21. Belarus crossed this inflection point in 1997, Albania and Congo (Brazzaville) in 2001, Azerbaijan in 2004, Georgia in 2006, and Bolivia in 2015. The remaining countries crossed this point before the start of this study. The possible reason is that these countries have shifted to the secondary and tertiary industries after the economy reaches a certain level, resulting in insufficient investment in agricultural production and relying on imports to meet domestic demand. The per capita arable land area has a significant negative impact on food imports, and countries with higher arable land endowments are more capable of achieving the balance of food supply and demand through themselves, curbing food imports and foreign dependence. In addition, infrastructure, technological progress, and industrial structure all significantly impact food imports.
Based on 21 years of panel data from 91 countries along the “Belt and Road,” the article divides countries with the same “characteristics” into one group through the panel threshold model and then examines the influencing factors of each group of food imports according to the groupings. The 91 countries along the “Belt and Road” are divided into five distinct groups. The study found that population size and the arable land endowment have significant single and double-threshold effects on food imports, respectively. Among them, the population size has no significant impact on the food import of countries with medium cultivated land endowments but has no significant effect on cultivated land. Countries with high and low endowments have a significant positive impact. Seemingly, per capita-arable land area has no significant impact on food imports from countries with medium arable land endowments but has a significant positive effect on countries with high arable land endowments and a significant negative impact on countries with low arable land endowments.
Countries with different population sizes, economic levels, and arable land endowments have different food imports. There is an inverted U-shaped relationship between economic level and food imports in countries with large populations and high cultivated land endowments. A U-shaped relationship has been found between the two groups of countries with low cultivated land endowments, related to the small population size and medium cultivated land endowment. There is a positive linear relationship between food imports in countries with medium-cultivated land endowments, but it has no significant impact on food imports in countries with large populations and medium-cultivated land endowments. The impact of infrastructure, technological progress, food stocks, and industrial structure on the food imports of countries with different population sizes and arable land endowments has been found different from each other. However, tariff policies and international food prices have no significant impact on countries' food imports. Based on the research conclusions, the following policy implications are put forward:
(i) For countries like China with a large population but low arable land endowments, the level of economic development has a U-shaped relationship with food imports and has now entered an upward range. First of all, domestic investment in agricultural science and technology should be strengthened, and farmland water conservancy and other facilities should be improved. The im-port dependencies should be reduced as much as possible by increasing productivity and efficiency. In addition, countries should use the “Belt and Road Initiatives” platform to broaden the prospects of import channels to minimize barriers and tariffs imposed by the international market.
(ii) Countries with high arable land endowments should give full support to their resource advantages, combine with sustainable production, and strengthen the interactions of various science and technology to maximize the efficiencies like modern irrigation technology, pest control technology, and sustainable intensifications. They need to increase the cooperation among the countries with BRI to tap food production potential further, meet domestic demand, and increase exports to achieve resource-sharing superiority.
(iii) Countries with small populations should make overall arrangements according to local conditions, adjust the industrial structure while increasing agricultural scientific research, and reduce excessive dependence on food imports. In particular, countries with low arable land endowments should appropriately control the population size and implement production maximization under the banner of sustainable land, water, and other critical resource management. They should strengthen the food reserves to avoid large-scale food imports in emergencies.
The study also possessed some limitations. This article tries to select as many countries and longer periods as possible. However, due to the long period, political and social instability, and time lag in major databases, many countries have missing data for many years, resulting in limited data comprehensiveness. Future research can appropriately control and screen samples, expand the scope of indicator analysis, and expand the national scope of the study. Moreover, the study excludes the impact of environmental factors and agricultural overseas resource development on grain import and export trade. It might be interesting if appropriate ecological indicators along with resource endowment factors could have been compiled and incorporated into the model. One of the major challenges of this study is the type and range of selected grains. In future research, multiple agricultural products with a large proportion of trade can be considered to be included in the study.
Publicly available datasets were analyzed in this study. This data can be found here: The basic data needed for the research are extracted from the FAOSTAT database (https://www.fao.org/faostat) of the United Nations Food and Agriculture Organization (FAO), the United Nation's Commodity Trade Statistics database (https://comtrade.un.org), the World Bank's World Development Index (https://datatopics.worldbank.org) and the United States Department of Agriculture (https://www.usda.gov).
Conceptualization and methodology: YW and AS. Software: AS. Both authors have read and agreed to the published version of the manuscript.
This research was funded by the National Natural Science Foundation of China, grant numbers 71673222 and 72064009. Humanities and Social Science Fund of Ministry of Education of China, grant number 15XJA790005. The Shaanxi Provincial Key R&D Program Project, grant number 2020KW-029. China Scholarship Council Funded Project, grant number 202106300001.
The authors declare that the research was conducted in the absence of any commercial or financial relationships that could be construed as a potential conflict of interest.
All claims expressed in this article are solely those of the authors and do not necessarily represent those of their affiliated organizations, or those of the publisher, the editors and the reviewers. Any product that may be evaluated in this article, or claim that may be made by its manufacturer, is not guaranteed or endorsed by the publisher.
Abad, L. A. (2013). Persistent Inequality? Trade, Factor Endowments, and Inequality in Republican Latin America. J. Econ. History 73, 38–78. doi: 10.1017/S0022050713000028
Abay, K. A., Ibrahim, H., Breisinger, C., and Bayasgalanbat, N. (2020). Food Policies and Their Implications on Overweight and Obesity Trends in Selected Countries in Near East and North Africa Region. IFPRI MENA Working Paper RP.
Abdul-Rahaman, A., Issahaku, G., and Zereyesus, Y. A. (2021). Improved rice variety adoption and farm production efficiency: accounting for unobservable selection bias and technology gaps among smallholder farmers in Ghana. Technol. Soc. 64, 101471. doi: 10.1016/j.techsoc.2020.101471
Ahumada, O., and Villalobos, J. R. (2009). Application of planning models in the agri-food supply chain: a review. Eur. J. Oper. Res. 196, 1–20. doi: 10.1016/j.ejor.2008.02.014
Alrobaish, W. S., Vlerick, P., Luning, P. A., and Jacxsens, L. (2021). Food safety governance in Saudi Arabia: challenges in control of imported food. J. Food Sci. 86, 16–30. doi: 10.1111/1750-3841.15552
Atif, R. M., Haiyun, L., and Mahmood, H. (2017). Pakistan's agricultural exports, determinants and its potential: an application of stochastic frontier gravity model. J. Int. Trade. Econ. Dev. 26, 257–276. doi: 10.1080/09638199.2016.1243724
Attrey, D. P. (2017). “Chapter 37 - Food safety in international food trade—imports and exports,” in Food Safety in the 21st Century, eds R. K. Gupta, Dudeja, and Singh Minhas (San Diego: Academic Press), 455–468. doi: 10.1016/B978-0-12-801773-9.00037-6
Bakari, S., and Mabrouki, M. (2017). Impact of exports and imports on economic growth: new evidence from Panama. J. Smart Econ. Growth 2, 67–79. Available online at: https://jseg.ro/index.php/jseg/article/view/70
Bell, A., and Jones, K. (2015). Explaining fixed effects: random effects modeling of time-series cross-sectional and panel data*. Polit. Sci. Res. Methods 3, 133–153. doi: 10.1017/psrm.2014.7
Bougheas, S., Demetriades, P. O., and Morgenroth, E. L. W. (1999). Infrastructure, transport costs and trade. J. Int. Econ. 47, 169–189. doi: 10.1016/S0022-1996(98)00008-7
Bozsik, N. T. J. P. C., Stalbek, B., Vasa, L., and Magda, R. (2022). Food security management in developing countries: influence of economic factors on their food availability and access. PLoS ONE 17, e0271696. doi: 10.1371/journal.pone.0271696
Bui, T. H. H., and Chen, Q. (2017). An analysis of factors influencing rice export in Vietnam based on gravity model. J. Knowl. Econ. 8, 830–844. doi: 10.1007/s13132-015-0279-y
Bujang, I., Hakim, T. A., and Ahmad, I. (2013). Tax structure and economic indicators in developing and high-income OECD countries: panel cointegration analysis. Procedia Econ. Finance 7, 164–173. doi: 10.1016/S2212-5671(13)00231-1
Chen, D., Yu, Q., Hu, Q., Xiang, M., Zhou, Q., and Wu, W. (2018). Cultivated land change in the belt and road initiative region. J. Geogr. Sci. 28, 1580–1594. doi: 10.1007/s11442-018-1530-9
Chernova, V. Y., and Noha, V. I. (2019). A study of the characteristics of food import dependence of the countries. Amazonia Investiga 8, 484–492. Available online at: https://amazoniainvestiga.info/index.php/amazonia/article/view/1012
Clapp, J. (2009). Food price volatility and vulnerability in the global south: considering the global economic context. Third World Q. 30, 1183–1196. doi: 10.1080/01436590903037481
Cui, K., and Shoemaker, S. P. (2018). A look at food security in China. npj Sci. Food 2, 4. doi: 10.1038/s41538-018-0012-x
de Lima, D. P., Fioriolli, J. C., Padula, A. D., and Pumi, G. (2018). The impact of Chinese imports of soybean on port infrastructure in Brazil: a study based on the concept of the “Bullwhip Effect.” J. Commodity Markets 9, 55–76. doi: 10.1016/j.jcomm.2017.11.001
Distefano, T., Laio, F., Ridolfi, L., and Schiavo, S. (2018). Shock transmission in the International Food Trade Network. PLoS ONE 13, e0200639. doi: 10.1371/journal.pone.0200639
Donaubauer, J., Glas, A., Meyer, B., and Nunnenkamp, P. (2018). Disentangling the impact of infrastructure on trade using a new index of infrastructure. Rev. World Econ. 154, 745–784. doi: 10.1007/s10290-018-0322-8
Dorninger, C., von Wehrden, H., Krausmann, F., Bruckner, M., Feng, K., Hubacek, K., et al. (2021). The effect of industrialization and globalization on domestic land-use: a global resource footprint perspective. Glob. Environ. Change 69, 102311. doi: 10.1016/j.gloenvcha.2021.102311
Elsheikh, O. E., Elbushra, A. A., and Salih, A. A. A. (2015). Economic impacts of changes in wheat's import tariff on the Sudanese economy. J. Saudi Soc. Agric. Sci. 14, 68–75. doi: 10.1016/j.jssas.2013.08.002
Erokhin, V. (2017). Factors influencing food markets in developing countries: an approach to assess sustainability of the food supply in Russia. Sustainability 9, 1313. doi: 10.3390/su9081313
Erokhin, V., and Gao, T. (2018). “Competitive advantages of China's agricultural exports in the outward-looking belt and road initiative,” in China's Belt and Road Initiative: Changing the Rules of Globalization Palgrave Studies of Internationalization in Emerging Markets, eds W. Zhang, I. Alon, and C. Lattemann (Cham: Springer International Publishing), 265–285. doi: 10.1007/978-3-319-75435-2_14
Essandoh, O. K., Islam, M., and Kakinaka, M. (2020). Linking international trade and foreign direct investment to CO2 emissions: any differences between developed and developing countries? Sci. Tot. Environ. 712, 136437. doi: 10.1016/j.scitotenv.2019.136437
Fader, M., Gerten, D., Krause, M., Lucht, W., and Cramer, W. (2013). Spatial decoupling of agricultural production and consumption: quantifying dependences of countries on food imports due to domestic land and water constraints. Environ. Res. Lett. 8, 014046. doi: 10.1088/1748-9326/8/1/014046
Fukase, E., and Martin, W. (2020). Economic growth, convergence, and world food demand and supply. World Dev. 132, 104954. doi: 10.1016/j.worlddev.2020.104954
Gandhi, V. P., and Zhou, Z. (2014). Food demand and the food security challenge with rapid economic growth in the emerging economies of India and China. Food Res. Int. 63, 108–124. doi: 10.1016/j.foodres.2014.03.015
Geng, S., Ren, T., and Wang, M. (2007). Technology and infrastructure considerations for E-commerce in Chinese agriculture. Agric. Sci. China 6, 1–10. doi: 10.1016/S1671-2927(07)60010-8
Gürkan, A. A., Balcombe, K., and Prakash, A. (2003). Food import bills: experiences, factors underpinning changes and policy implications for food security of least developed and net food-importing developing countries. Commodities and Trade Division Food and Agriculture Organization of the United Nations Rome 2003, 19. Available online at: https://scholar.google.com.au/scholar?hl=en&as_sdt=0%2C5&q=G%C3%BCrkan%2C+A.+A.%2C+Balcombe%2C+K.%2C+and+Prakash%2C+A.+Q16+%282003%29.+Food+import+bills%3A+experiences%2C+factors+underpinning+changes+and+policy+implications+for+food+security+of+least+developed+and+net+food-importing+developing+countries.+Commodities+and+Trade+Division+Food+and+Agriculture+Organization+of+the+United+Nations+Rome+2003%2C+19.&btnG=
Han, M., and Li, S. (2021). Transfer patterns and drivers of embodied agricultural land within China: Based on multi-regional decomposition analysis. Land 10, 213. doi: 10.3390/land10020213
Hansen, B. E. (2011). Threshold autoregression in economics. Stat. Interface 4, 123–127. doi: 10.4310/SII.2011.v4.n2.a4
He, M., Huang, Z., and Zhang, N. (2016). An empirical research on agricultural trade between China and “The Belt and Road” countries: competitiveness and complementarity. Modern Econ. 07, 1671. doi: 10.4236/me.2016.714147
Huang, J., Wei, W., Cui, Q., and Xie, W. (2017). The prospects for China's food security and imports: will China starve the world via imports? J. Integr. Agric. 16, 2933–2944. doi: 10.1016/S2095-3119(17)61756-8
Hughes, A. C., Lechner, A. M., Chitov, A., Horstmann, A., Hinsley, A., Tritto, A., et al. (2020). Horizon scan of the belt and road initiative. Trends Ecol. Evol. 35, 583–593. doi: 10.1016/j.tree.2020.02.005
Iheonu, C. O., Anyanwu, O. C., Odo, O. K., and Nathaniel, S. P. (2021). Does economic growth, international trade, and urbanization uphold environmental sustainability in sub-Saharan Africa? Insights from quantile and causality procedures. Environ. Sci. Pollut. Res. 28, 28222–28233. doi: 10.1007/s11356-021-12539-z
Ikuemonisan, E., Ajibefun, I., and Mafimisebi, T. E. (2018). Food price volatility effect of exchange rate volatility in nigeria. Rev. Innov. Competitiveness : J. Econ. Soc. Res. 4, 23–52. doi: 10.32728/ric.2018.44/2
Ivanova, V. N., Tatochenko, A. L., Jazev, G. V., Zaitseva, N. A., Larionova, A. A., and Vikhrova, N. O. (2018). The Use of Regression Analysis to Estimate the Prospects for the Food Industry Development in the Russian Federation. Revista ESPACIOS 39. Available online at: http://www.revistaespacios.com/a18v39n22/18392205.html (accessed September 13, 2022).
Jaworska, M. (2018). “Food imports and food security of main global market players,” in Proceedings of the International Scientific Conference “Economic Sciences for Agribusiness and Rural Economy.” Available online at: https://js.wne.sggw.pl/index.php/esare/article/view/1521 (accessed December 17, 2021). doi: 10.22630/ESARE.2018.2.32
Kao, C. (1999). Spurious regression and residual-based tests for cointegration in panel data. J. Econ. 90, 1–44. doi: 10.1016/S0304-4076(98)00023-2
Karbekova, A. B., Sadyraliev, Z., Mamyralieva, A. T., and Tolmachev, A. V. (2022). “A SWOT analysis of agricultural improvement in food-importing countries: a view from the standpoint of sustainable development of agricultural entrepreneurship,” in Sustainable Agriculture: Circular to Reconstructive, Volume 2 Environmental Footprints and Eco-design of Products and Processes, eds E. G. Popkova and B. S. Sergi (Singapore: Springer Nature), 107–115. doi: 10.1007/978-981-19-1125-5_13
Knight, J., Holdsworth, D., and Mather, D. (2007b). Determinants of trust in imported food products: perceptions of European gatekeepers. Br. Food J. 109, 792–804. doi: 10.1108/00070700710821331
Knight, J. G., Holdsworth, D. K., and Mather, D. W. (2007a). Country-of-origin and choice of food imports: an in-depth study of European distribution channel gatekeepers. J. Int. Bus. Stud. 38, 107–125. doi: 10.1057/palgrave.jibs.8400250
Knudsen, M. T., Halberg, N., Olesen, J. E., Byrne, J., Iyer, V., and Toly, N. (2006). “Global trends in agriculture and food systems,” in Global Development of Organic Agriculture-challenges and Prospects (Wallingford: CABI Publishing), 1–48. doi: 10.1079/9781845930783.0001
Konieczna, A., Roman, K., Roman, M., Sliwiński, D., and Roman, M. (2021). Energy efficiency of maize production technology: evidence from polish farms. Energies 14, 170. doi: 10.3390/en14175574
Koo, W., and Kennedy, P. L. (2005). International trade and agriculture. Manage. Environ. Qual.: Int. J. 16, 566–567. doi: 10.1002/9780470759189
Kummu, M., de Moel, H., Porkka, M., Siebert, S., Varis, O., and Ward, P. J. (2012). Lost food, wasted resources: global food supply chain losses and their impacts on freshwater, cropland, and fertiliser use. Sci. Tot. Environ. 438, 477–489. doi: 10.1016/j.scitotenv.2012.08.092
Lau, Y., Tam, K., Ng, A. K. Y., Fu, X., Jing, Z., and Feng, J. (2018). Effects of the ‘Belt and Road' initiative on the wine import logistics of China. Maritime Policy Manage. 45, 403–417. doi: 10.1080/03088839.2017.1405291
Leitão, N. C., and Lorente, D. B. (2020). The linkage between economic growth, renewable energy, tourism, CO2 emissions, and international trade: the evidence for the European Union. Energies 13, 4838. doi: 10.3390/en13184838
Li, X. (2012). Technology, factor endowments, and China's agricultural foreign trade: a neoclassical approach. China Agric. Econ. Rev. 4, 105–123. doi: 10.1108/17561371211196801
Limão, N., and Venables, A. J. (2001). Infrastructure, geographical disadvantage, transport costs, and trade. World Bank Econ. Rev. 15, 451–479. doi: 10.1093/wber/15.3.451
Liu, F., Xiao, X., Qin, Y., Yan, H., Huang, J., Wu, X., et al. (2021). Large spatial variation and stagnation of cropland gross primary production increases the challenges of sustainable grain production and food security in China. Sci. Tot. Environ. 811, 151408. doi: 10.1016/j.scitotenv.2021.151408
Liu, Y., and Zhou, Y. (2021). Reflections on China's food security and land use policy under rapid urbanization. Land use policy 109, 105699. doi: 10.1016/j.landusepol.2021.105699
Lu, Y., Zhang, Y., Hong, Y., He, L., and Chen, Y. (2022). Experiences and lessons from agri-food system transformation for sustainable food security: a review of China's practices. Foods 11, 137. doi: 10.3390/foods11020137
Luo, P., and Tanaka, T. (2021). Food import dependency and national food security: a price transmission analysis for the wheat sector. Foods 10, 1715. doi: 10.3390/foods10081715
Maaouane, M., Zouggar, S., Krajačić, G., and Zahboune, H. (2021). Modelling industry energy demand using multiple linear regression analysis based on consumed quantity of goods. Energy 225, 120270. doi: 10.1016/j.energy.2021.120270
Majumdar, J., Naraseeyappa, S., and Ankalaki, S. (2017). Analysis of agriculture data using data mining techniques: application of big data. J. Big Data 4, 20. doi: 10.1186/s40537-017-0077-4
Marsden, T., Banks, J., and Bristow, G. (2000). Food supply chain approaches: exploring their role in rural development. Sociol. Ruralis 40, 424–438. doi: 10.1111/1467-9523.00158
Maslak, N., Lei, Z., and Xu, L, . (eds.) (2020). Analysis of agricultural trade in China based on the theory of factor endowment. Agricultural and Resource Economics: International Scientific E-Journal. 6, 50–61. doi: 10.51599/are.2020.06.01.04
Masters, W. A., and McMillan, M. S. (2001). Climate and scale in economic growth. J. Econ. Growth 6, 167–186. doi: 10.1023/A:1011398431524
McMichael, P. (2000). A global interpretation of the rise of the East Asian food import complex. World Dev. 28, 409–424. doi: 10.1016/S0305-750X(99)00136-9
Meloni, G., and Swinnen, J. (2022). Globalization and political economy of food policies: insights from planting restrictions in colonial wine markets. Appl. Econ. Perspect. Policy 44, 766–787. doi: 10.1002/aepp.13192
Mercken, E., Van Damme, I., Serradell, A., and Gabriël, S. (2020). Presence of Anisakidae in commercial fish species imported into the Belgian food markets: a systematic review and meta-analyses. Int. J. Food Microbiol. 318, 108456. doi: 10.1016/j.ijfoodmicro.2019.108456
Mgeni, C. P., Sieber, S., Amjath-Babu, T. S., and Mutabazi, K. D. (2018). Can protectionism improve food security? Evidence from an imposed tariff on imported edible oil in Tanzania. Food Sec. 10, 799–806. doi: 10.1007/s12571-017-0746-3
Mostenska, T. L., Mostenska, T. G., Yurii, E., Lakner, Z., and Vasa, L. (2022). Economic affordability of food as a component of the economic security of Ukraine. PLoS ONE 17, e0263358. doi: 10.1371/journal.pone.0263358
Mousavi, S. H., and Esmaieli, A. (2011). The impacts of import tariff policy on domestic rice market in Iran. J. Agric. Econ. Res. 3, 1–20. Available online at: https://www.cabdirect.org/cabdirect/abstract/20113376205 (accessed December 17, 2021).
Müller, B., Hoffmann, F., Heckelei, T., Müller, C., Hertel, T. W., Polhill, J. G., et al. (2020). Modelling food security: Bridging the gap between the micro and the macro scale. Glob. Environ. Change 63, 102085. doi: 10.1016/j.gloenvcha.2020.102085
Mwangi, E. N. (2021). Determinants of agricultural imports in Sub-Saharan Africa: a gravity model. Afr. J. Econ. Rev. 9, 271–287. Available online at: https://www.ajol.info/index.php/ajer/article/view/207157
Nguyen, H. (2018). Sustainable Food Systems: Concept and Framework. Rome, Italy: Food and Agriculture Organization of the United Nations. Available online at: https://www.fao.org/3/ca2079en/CA2079EN.pdf (accessed December 9, 2022).
Nicita, A. (2013). Exchange rates, international trade and trade policies. Int. Econ. 135, 47–61. doi: 10.1016/j.inteco.2013.10.003
Norton, G. W., Alwang, J., and Masters, W. A. (2021). Economics of Agricultural Development: World Food Systems and Resource Use. 4th Edn. London: Routledge. doi: 10.4324/9780429316999
Oh, J. (2001). International trade in film and the self-sufficiency ratio. J. Media Econ. 14, 31–44. doi: 10.1207/S15327736ME1401_03
Okolo, C. V., and Obidigbo, C. (2015). Food security in Nigeria: an examination of food availability and accessibility in Nigeria. Int. J. Econ. Manage. Eng. 9, 3171–3179. Available online at: https://publications.waset.org/10002471/food-security-in-nigeria-an-examination-of-food-availability-and-accessibility-in-nigeria
Okpe, A. E., and Ikpesu, F. (2021). Effect of inflation on food imports and exports. J. Dev. Areas 55, 1–10. doi: 10.1353/jda.2021.0075
Olayungbo, D. O. (2021). Global oil price and food prices in food importing and oil exporting developing countries: a panel ARDL analysis. Heliyon 7, e06357. doi: 10.1016/j.heliyon.2021.e06357
Pedersen, S., Aschemann-Witzel, J., and Thøgersen, J. (2018). Consumers' evaluation of imported organic food products: the role of geographical distance. Appetite 130, 134–145. doi: 10.1016/j.appet.2018.08.016
Pingali, P. (2007). Agricultural growth and economic development: a view through the globalization lens. Agric. Econ. 37, 1–12. doi: 10.1111/j.1574-0862.2007.00231.x
Porkka, M., Guillaume, J. H. A., Siebert, S., Schaphoff, S., and Kummu, M. (2017). The use of food imports to overcome local limits to growth. Earth's Future 5, 393–407. doi: 10.1002/2016EF000477
Prosekov, A. Y., and Ivanova, S. A. (2018). Food security: the challenge of the present. Geoforum 91, 73–77. doi: 10.1016/j.geoforum.2018.02.030
Qingjie, L. (2021). Resource endowments in emerging market countries. Glob. J. Emerg. Market Econ. 13, 287–310. doi: 10.1177/09749101211004338
Ren, D., Yang, H., Zhou, L., Yang, Y., Liu, W., Hao, X., et al. (2021). The Land-Water-Food-Environment nexus in the context of China's soybean import. Adv. Water Resour. 151, 103892. doi: 10.1016/j.advwatres.2021.103892
Rush, E., and Obolonkin, V. (2020). Food exports and imports of New Zealand in relation to the food-based dietary guidelines. Eur. J. Clin. Nutr. 74, 307–313. doi: 10.1038/s41430-019-0557-z
Savvides, A., and Stengos, T. (2000). Income inequality and economic development: evidence from the threshold regression model. Econ. Lett. 69, 207–212. doi: 10.1016/S0165-1765(00)00293-7
Schneider, U. A., Havlík, P., Schmid, E., Valin, H., Mosnier, A., Obersteiner, M., et al. (2011). Impacts of population growth, economic development, and technical change on global food production and consumption. Agric. Syst. 104, 204–215. doi: 10.1016/j.agsy.2010.11.003
Sertoglu, K., and Dogan, N. (2016). Agricultural trade and its determinants: evidence from bounds testing approach for Turkey. Int. J. Econ. Financial Issues 6, 450–455. Available online at: https://dergipark.org.tr/en/pub/ijefi/issue/31978/352461?publisher=http-www-cag-edu-tr-ilhan-ozturk
Shakib, M., Yumei, H., Rauf, A., Alam, M., Murshed, M., and Mahmood, H. (2021). Revisiting the energy-economy-environment relationships for attaining environmental sustainability: evidence from Belt and Road Initiative countries. Environ. Sci. Pollut. Res. 29, 3808–3825. doi: 10.1007/s11356-021-15860-9
Shevchuk, V. (2014). Modelling of agricultural import demand in Ukraine. Inform. Sys. Manage. 3, 201–211. Available online at: https://www.infona.pl//resource/bwmeta1.element.desklight-f05d09c1-98eb-4ea5-ac97-8927e1fd0538
Smyth, S. J., Phillips, P. W. B., and Kerr, W. A. (2016). EU failing FAO challenge to improve global food security. Trends Biotechnol. 34, 521–523. doi: 10.1016/j.tibtech.2016.04.003
Sternberg, T., McCarthy, C., and Hoshino, B. (2020). Does China's belt and road initiative threaten food security in Central Asia? Water 12, 2690. doi: 10.3390/w12102690
Suanin, W. (2021). Demand elasticity of processed food exports from developing countries: a panel analysis of US imports. J. Agric. Econ. 72, 413–429. doi: 10.1111/1477-9552.12409
Syed, J., and Ying, Y.-H. (2019). “Introduction: diversity, convergence and opportunities along the belt and road,” in China's Belt and Road Initiative in a Global Context: Volume I: A Business and Management Perspective Palgrave Macmillan Asian Business Series, eds J. Syed and Y.-H. Ying (Cham: Springer International Publishing), 1–19. doi: 10.1007/978-3-030-14722-8_1
Tian, R., Yang, Z., and Shao, Q. (2020). Effects of host country resource endowment and labor cost on China's investment in overseas cultivated land. Environ. Sci. Pollut. Res. 27, 45282–45296. doi: 10.1007/s11356-020-10373-3
Tukker, A., Bulavskaya, T., Giljum, S., de Koning, A., Lutter, S., Simas, M., et al. (2016). Environmental and resource footprints in a global context: Europe's structural deficit in resource endowments. Glob. Environ. Change 40, 171–181. doi: 10.1016/j.gloenvcha.2016.07.002
van Dijk, M., Morley, T., Rau, M. L., and Saghai, Y. (2021). A meta-analysis of projected global food demand and population at risk of hunger for the period 2010–2050. Nat. Food 2, 494–501. doi: 10.1038/s43016-021-00322-9
Vorst, J. G. A. J., and van der. (2000). Effective Food Supply Chains : Generating, Modelling and Evaluating Supply Chain Scenarios. Available online at: https://research.wur.nl/en/publications/effective-food-supply-chains-generating-modelling-and-evaluating- (accessed November 27, 2021).
Wang, Y., Sarkar, A., Ma, L., Wu, Q., and Wei, F. (2021). Measurement of investment potential and spatial distribution of arable land among countries within the “belt and road initiative.” Agriculture 11, 848. doi: 10.3390/agriculture11090848
Westerlund, J., and Edgerton, D. L. (2007). A panel bootstrap cointegration test. Econ. Lett. 97, 185–190. doi: 10.1016/j.econlet.2007.03.003
Williams, G. W., Capps, O., and Hanselka, D. (2017). The national economic benefits of food imports: the case of U.S. Imports of Hass Avocados From Mexico. null 29, 139–157. doi: 10.1080/08974438.2016.1266570
Wright, B., and Cafiero, C. (2011). Grain reserves and food security in the Middle East and North Africa. Food Sec. 3, 61–76. doi: 10.1007/s12571-010-0094-z
Yu, W., and Jensen, T. V. (2005). Tariff preferences, WTO negotiations and the LDCs: the case of the ‘Everything But Arms' initiative. World Econ. 28, 375–405. doi: 10.1111/j.1467-9701.2005.00682.x
Zhan, S. (2021). The political economy of food import and self-reliance in China: 1949-2019. Glob. Food History 0, 1–19. doi: 10.1080/20549547.2021.2012082
Keywords: belt and road initiatives (BRI), food import, influencing factors, panel threshold model, food trade, sustainable food trade
Citation: Wang Y and Sarkar A (2022) Evaluating the influencing factors of food imports within belt and road initiatives (BRI) countries: An economic threshold model approach. Front. Sustain. Food Syst. 6:997549. doi: 10.3389/fsufs.2022.997549
Received: 19 July 2022; Accepted: 03 October 2022;
Published: 26 October 2022.
Edited by:
Andrea Pieroni, University of Gastronomic Sciences, ItalyReviewed by:
Justice Gameli Djokoto, Central University, GhanaCopyright © 2022 Wang and Sarkar. This is an open-access article distributed under the terms of the Creative Commons Attribution License (CC BY). The use, distribution or reproduction in other forums is permitted, provided the original author(s) and the copyright owner(s) are credited and that the original publication in this journal is cited, in accordance with accepted academic practice. No use, distribution or reproduction is permitted which does not comply with these terms.
*Correspondence: Yameng Wang, d3ltQG53YWZ1LmVkdS5jbg==
Disclaimer: All claims expressed in this article are solely those of the authors and do not necessarily represent those of their affiliated organizations, or those of the publisher, the editors and the reviewers. Any product that may be evaluated in this article or claim that may be made by its manufacturer is not guaranteed or endorsed by the publisher.
Research integrity at Frontiers
Learn more about the work of our research integrity team to safeguard the quality of each article we publish.