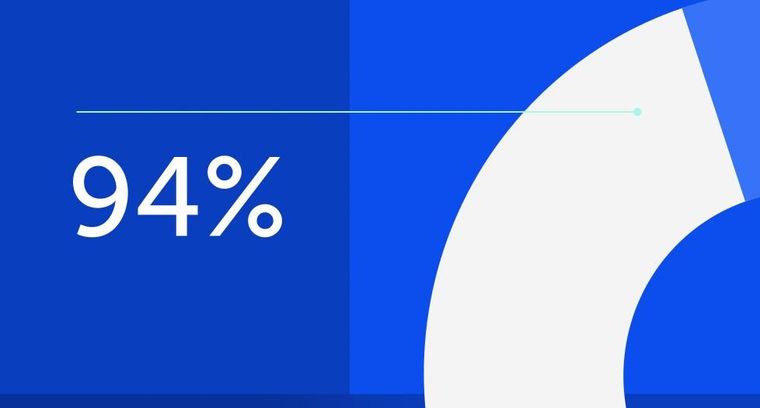
94% of researchers rate our articles as excellent or good
Learn more about the work of our research integrity team to safeguard the quality of each article we publish.
Find out more
ORIGINAL RESEARCH article
Front. Sustain. Food Syst., 07 September 2022
Sec. Agro-Food Safety
Volume 6 - 2022 | https://doi.org/10.3389/fsufs.2022.984493
This article is part of the Research TopicEmerging Clean Label Approaches to Food SafetyView all 4 articles
The blockchain-based traceability in agri-food marketing has brought a disruptive paradigm shift by removing the inherent information asymmetry problem. Likewise, revealing sufficient product quality and attributes information could break agricultural markets' “Lemon Market” dilemma. This study takes the fresh fruit with blockchain traceability QR label as a case and systematically investigates the influence of consumers' food control risk attitude on information-seeking intentions. We utilized online survey data of 1,058 fresh fruit buyers and simultaneously applied ordinary least square (OLS), ordered logit model (Ologit), and propensity score matching (PSM) approaches to overcome the potential self-selection biases and confounding factors. The results show that risk attitude significantly negatively impacts consumers' information seeking fresh fruits. The stronger consumers' risk preference, the lower the probability of information seeking. Furthermore, we used PSM to overcome potential sample selectivity bias; therefore, PSM reinforces the significance of OLS and Ologit results. The sub-sample estimation results show that young individuals with high school and below education have stronger blockchain information-seeking intentions. The study provides new insights into the role of food control risk attitude and agri-food information traceability and offers several measures for policy and practice to realize a border trajectory in agri-food information disclosure.
In developing countries, most consumers have greater access to safe, diversified, and affordable food. The issue is that consumer attitude toward food safety regarding the new processes and control infrastructure is often not positive (Frewer et al., 2011; Bearth and Siegrist, 2016). Traditional supply chains have several loopholes and non-compliance in the legislation, which shows the weaknesses in safeguarding public health and further undermines consumer confidence in the food control measures (Kleef et al., 2006). The key to solving food quality and safety is formulating an effective management mechanism to reveal the quality and safety information with the lowest management cost to restore consumer confidence in the food products (Gong et al., 2014). It requires integrated systems and information technology to increase the supply of food quality and safety information (Wang et al., 2013). The food traceability system has been proved to be an important means to solve the problem of information and trust in food safety and ensure food quality and safety (Rodriguez-Salvador and Dopico, 2020; Islam and Cullen, 2021).
In recent years, blockchain technology is gradually applied to the food traceability system and has become a potential solution to the dilemma of food traceability due to the multiple interconnections between its characteristics and agricultural product supply chain management (Lin et al., 2019; Niknejad et al., 2021). Blockchain, with its distributed storage, common mechanism, encryption algorithm, timestamp, and other technical characteristics (i.e., immutability, anonymity) (Creydt and Fischer, 2019; Dey et al., 2021), can generate a unique electronic label for each product, store the data on the distributed nodes and connect them by chain (Collart and Canales, 2022). The consumer can read the Quick Response (QR), reconstruct the product's history, and view any relevant certifications and events associated with the product (Baralla et al., 2019). Blockchain application can effectively solve the shortcomings of the current traditional traceability system and ensure the integrity and authenticity of product information traceability from “farm to table.” In particular, the newly emerging blockchain traceability can provide safer, transparent, and accurate information sharing (Valoppi et al., 2021), and help improve the trust among supply chain participants (Köhler and Pizzol, 2020; Markovic et al., 2020). Thus, it ensures the authenticity and credibility of food characteristics and related information in the value chain (Galvez et al., 2018).
In the case of information asymmetry, information seeking is an important part of consumers' purchasing decision-making (Kotler and Keller, 2013). Consumers can obtain product information by seeking necessary information to help them make judgments and reduce the uncertainty in purchasing. Labels are one of the channels for food information-seeking sources (Lioutas, 2014). Traceable QR code, a key labeling tool for transmitting characteristic product information, turn information search attributes into trust element attached through background detailed agricultural products information (Jin and Zhou, 2014; Lin et al., 2022). Consumers can obtain the whole industrial chain information about agricultural products (e.g., planting, harvesting, processing, transportation, and retail) at a low cost. It effectively discloses the agricultural products' internal attribute information and sufficiently reduces the information asymmetry between buyers and sellers. Some studies have discussed the blockchain and QR codes from a technological perspective. To make the food production data easily accessible, traceable, and verifiable by consumers, Dey et al. (2021) have created a FoodSQRBlock, which can digitize the food production information so that the consumers can trace the food product information easily accessible using the QR code. Baralla et al. (2019) proposed a generic European agri-food supply chain traceability system based on blockchain technology and QR code, which increases trust between organizations and individuals by reading a QR code. Köhler and Pizzol (2020) focused on analyzing six cases of blockchain-based technologies (namely, blockchain technology, data communication, etc.) in the food supply chain using a technology assessment framework. Among them, most cases provide product information through scanning QR codes. Dey et al. (2022) proposed a blockchain-based multi-layered framework utilizing cloud computing, QR code, and reinforcement learning to reduce food waste in different phases of the supply chain. However, little research about consumers' intention to trace QR labels based on blockchain technology is known. Therefore, as a new traceability solution, the question remained unanswered whether the consumers are willing to use or scan QR labels based on blockchain technology. Furthermore, what factors stimulate consumers' intentions to scan QR labels for the information seeking need to be further verified.
A global litany of food scandals and income growth in developing countries has raised consumers' food control risk attitudes. Since food safety work as a credence food attribute, it potentially leaves room for information asymmetry and permeates the consumers' food control risk attitude, especially for agricultural products (Prashar et al., 2020; Hoffmann et al., 2021). However, food information with blockchain traceability has emerged as a well-established market mechanism to mitigate this problem (Lin et al., 2022). Apart from the fact that traceability has improved information disclosure, we need to study to systematically trace how food control risk attitude influences information seeking, coupled with the impact of information availability through blockchain-based traceability system, on consumer risk attitude. Yet, little is known about the consumer food control risk attitude regarding food blockchain traceability information, especially for fresh fruits. To this end, this study provides new insights for a better understanding of the impacts of food control risk attitude on blockchain traceable information-seeking intention.
This study explores the consumers' information-seeking intention for scanning the traceability QR label based on blockchain technology. It also examines the impact of risk attitude on information-seeking intention. Identifying consumers' intention for information seeking, especially in the wake of frequent food quality and safety incidents, holds sufficient insights to guide policymakers. In this work, we take blockchain-certified traceable fresh fruits with QR label as a case to study consumers' information-seeking intentions and risk attitudes. Doing so provides cues on promoting the blockchain traceability certification and mitigating information asymmetry vis-à-vis restoring consumer confidence in advanced value chain systems.
The remainder of this study is arranged as follows. The next section is literature review, followed by empirical strategy and detailed information on the data source, variable selection, and empirical models. Next, empirical results and discussion are presented. Finally, the study conclusions, policy implications, new insights, limitations, and future research direction are acknowledged.
The consumer is an important stakeholder in the market mechanism (Bojnec et al., 2019). Yet, the literature on consumers' willingness toward blockchain traceability information is limited. For example, Thomasson (2019) pointed out that consumers significantly accepted the products traced by the blockchain and were willing to spend up to 90 s reading the source information obtained by scanning the code. Lin et al. (2021) studied the factors influencing the intention of the organic food blockchain traceability system by building a conceptual framework based on the information success model and the theory of planned behavior. Their results showed that attitude and perceived behavior control, system quality, information quality, and service quality were significantly and positively related to consumer trust. And, trust significantly influences the willingness to use the blockchain traceability system. In addition, Dionysis et al. (2022) studied consumer willingness to purchase blockchain traceable coffee based on online survey data. It shows that attitude, perceived behavior control, and environmental protection measures promote blockchain traceable coffee purchase intention. Most recent studies mainly focus on meat and coffee buying through blockchain traceability. Therefore, a need exists to explore common products, especially fresh fruits, which account for a substantial proportion of households' daily food consumption.
The risk attitude literature differentiates between the perceived and actual risks related to unhygienic food conditions. The consumers' judgment of actual risk is inherent through risk attitude (Schroeder et al., 2007). Evidence shows that food control risk perception determines consumers' consumption of unsafe food (Lagerkvist et al., 2013; Evans et al., 2020), promoting information-seeking behavior (Lusk and Coble, 2005). Information seeking is important for learning, social behavior, and decision-making (Kelly and Sharot, 2021). A recent strand of the literature indicated various drivers of information seeking. Inter alia, trust and risk perception (Jin et al., 2020), information source characteristics and demographics (Desta et al., 2019), social psychological (Chang et al., 2017), cognitive and affective factors (Zhao and Liu, 2021), consumer social network (Lee, 2014), social media exposure (He and Li, 2021), social environment (Yang and Kahlor, 2013), and cognitive barriers to information seeking (Savolainen, 2015) are major ones. According to Boot and Meijman (2010), these driving factors include psychology, mass communication, library and information science, and medical science. However, regarding food consumption, based on the risk attitude, consumers decide to buy various products, namely, pork (Liu and Wu, 2013), genetically modified food (Zhu et al., 2018), and fresh food (Zhang and Liu, 2010).
Food control risk attitude refers to technical concepts of intuitive psychology capturing various temperaments that can be exhibited under different situations (Baccelli, 2018), which plays an important role in decision-making. Studies pointed out that risk attitude influences online shopping (Wu and Chang, 2007), street food consumption (Choi et al., 2013), organic foods consumption (Saba and Messina, 2003), healthy eating (Szabo et al., 2019), purchase intention toward GM food (Hassan et al., 2016), and poultry purchase intention (Heikkila et al., 2013). Most existing studies utilize the risk perception attitude framework to evaluate the relationship between risk attitude and information seeking and mainly focus on individuals' risk perception and consumer efficacy (Turner et al., 2006; Deng and Liu, 2017). Given this framework, each individual is categorized for each scenario as responsive to evaluate the given risk level(s). For example, higher risk, higher efficacy (avoidant), higher risk, lower efficacy (proactive), lower risk, higher efficacy (indifferent), lower risk, lower efficacy, and the likelihood of information seeking are different for four scenarios (Grasso and Bell, 2015). Likewise, Zhu et al. (2018) noted that risk perception is a significant determinant of information seeking, and risk attitude is determined by consumer perception and information seeking. Some scholars found that health self-efficacy significantly moderates the relationship between perceived risk and information seeking (Deng and Liu, 2017).
The study contributes to the strand of literature on consumer behavior in many ways. First, the research object is novel. The existing studies mainly focus on the traditional product traceability system. Blockchain traceability belongs to the technological frontier, which has not been popularized for a long time, particularly for fresh fruits. Therefore, we choose fresh fruit as a case to explore the agricultural product supply chain and traceability as the core, describing the research status and development of blockchain technology in the agricultural field, and further exploring solutions to different application problems. Second, the data used are quite interesting, especially for suggesting coherent policy actions. The sampled areas in this work belong to traceable pilot cities in China. Likewise, the economic development levels of the sampled cities are different, ensuring the sample's representativeness and data validity, which is an important factor that has been overlooked in prior studies. Third, we study the interplay between the combination of information seeking and blockchain traceability as a major feature in this article, which has not been explored so far. Furthermore, the study provides robust estimates by simultaneously employing three empirical methods—OLS, Ologit, and PSM—to overcome the plethora of econometric challenges. The study findings may serve as a guide for researchers and practitioners interested in blockchain technology, enhance the comprehension of information disclosure, and consequently encourage the development and implementation of agri-food traceability.
The study data set comes from the online survey conducted in Nanjing, Beijing, Xi'an, and Fuzhou in 2021 (Figure 1). In the survey, we considered factors such as regional economic development and residents' consumption habits to include respondents from four pilot cities, namely, Nanjing, Beijing, Xi'an, and Fuzhou. These four cities are part of traceability systems pilot cities implemented in the People's Republic of China. According to National Bureau of Statistics (2020), the per capita disposable income of urban residents in Beijing, Nanjing, Fuzhou, and Xi'an is $10,996, $7614, $6794, and $4962, respectively. It represents the regions with different economic development levels, enhancing the data credibility and results produced. Furthermore, we consider the harvesting time of fruits and thus list one after another in summer. The final online survey was conducted in April and May of 2021.
The key reasons for conducting the online survey were the followings: purposively reach fresh buyers, cover four major pilot cities, and overcome mobility issues due to the spread of COVID-19. Most cities in China have implemented strict epidemic prevention policies to restrict or prohibit mobility, especially in areas at risk of positive infection during the COVID-19 epidemic. At all stages of questionnaire development and online survey, we tried to overcome self-selection bias as 71.6% of Chinese are frequent mobile internet users (China Internet Network Information Center., 2021); hence it helps overcome this problem (Olsen, 2009; Windle and Rolfe, 2011). Likewise, compared with email, telephone, and face-to-face questionnaire interviews, online surveys are increasingly popular among researchers due to their low cost and high efficiency (Hu and Veeman, 2006; Fleming and Bowden, 2009). Furthermore, many research studies fully support the suitability of online surveys for the current study objectives (Loo et al., 2011; Nielsen, 2011; Tonsor and Shupp, 2011).
To ensure the validity of the online survey, we systematically performed various measures. First, before the formal survey, the research team conducted an offline pre-investigation in Nanjing in April 2021, randomly intercepting respondents in three representative places by visiting supermarkets, communities, and shopping malls. Second, seven graduate students who were later well-trained and familiar with the questionnaire's content were selected. The investigator(s) randomly selected one consumer from every three who came into sight as the potential sample. Those willing to participate and complete the survey were taken as valid respondents. The questionnaire survey was mainly conducted by scanning two-dimensional code, supplemented by a paper questionnaire (for those who are not convenient to fill in with a mobile phone). As a pre-test, 33 valid samples were finally obtained. Based on the pre-testing information, the survey questionnaire was revised to make it more inclusive and insightful, and the formal online survey was implemented. Second, we set screening rules for respondents at the beginning of the survey questionnaire. Per se, respondents must meet three screening conditions before they can formally answer the question, (i) the respondents must be the main household food buyers, (ii) they purchased fruit in the past month, and (iii) they are above 18 years old above. Notably, we used trap questions as attention seekers (i.e., to test whether the respondents paid attention to the questionnaire. Likewise, we added another question after using the 5-point Likert scale to measure risk attitude: please choose somewhat disagree.) and maintained a minimum time limit for each part of the questionnaire. The formal questionnaire was divided into three parts: (1) respondents' attention to current food quality and safety, risk perception, and traceability awareness; (2) preference for fruit blockchain traceability information, purchase intention, and scan intention; and (3) socio-demographic information.
In the formal survey, the “Questionnaire Star Company” (https://www.wjx.cn/) was responsible for randomly distributing questionnaires into the databases of four cities and data collection from April 12 to May 20, 2021. A total of 1,126 questionnaires were collected. After eliminating the questionnaires with missing answers, wrong answers, or answers with obvious logical errors, we got 1058 valid questionnaires, with an effective rate of 91%. Among them, the valid samples of Nanjing, Beijing, Xi'an, and Fuzhou are 284 (including, pre-survey samples), 257, 261, and 256, respectively.
Labels can inform consumers about certain characteristics of foods (i.e., protected designation of origin, protected geographical indication, and organic label) (Bojnec et al., 2019). In this study, information seeking refers to consumers' search behavior for the QR label of fresh fruits. QR code in digital form has really good resistance against defacement or error correction (Dey et al., 2022).
The particularity of the blockchain traceability label is that it requires smartphones to scan the QR code to obtain related product information (Figure 2). Therefore, we call this behavior—whether consumers are likely to scan the blockchain traceable QR code—an information-seeking intention.
The questionnaire measures consumers' information-seeking intention by asking the question (if you see the “blockchain traceability QR code” on fresh fruit, how likely are you to scan the code to query traceable information) and taking their responses on a 5-point Likert scale (5 extremely unlikely to 1 extremely likely). Survey results indicate that consumers answered “extremely unlikely,” accounting for 1.13%; answered “unlikely,” accounting for 3.97%; those who answered “neither likely nor unlikely” are 251 consumers, accounting for 23.72%; who answered “likely,” accounting for 49.24%; those answered “extremely likely,” accounting for 21.93%. Most consumers are willing to seek traceable information by scanning blockchain traceability QR code.
Referring to existing research (Pennings et al., 2002; Lusk and Coble, 2005; Jin et al., 2017; Ha et al., 2020), the questionnaire measures consumers' risk attitudes as independent variables by asking four questions in our study: (1) although I often hear bad news regarding pesticide residues and illegal use of preservatives and industrial waxes, this does not affect my fresh fruit purchasing behavior, (2) I do not care about production origin when buying fresh fruits, (3) when eating fresh fruits, I never worry about pesticide residues, preservatives, or industrial waxes, and (4) I can accept the health risks caused by eating fresh fruits containing chemicals. Responses to these four questions were noted on a 5-point Likert scale (1 strongly disagree to 5 strongly agree). Finally, the average value of the four question options represents the consumers' food control risk attitude. The higher the value, the stronger the risk preference. Otherwise, the lower the value, the stronger the risk aversion. According to the descriptive statistics in Table 1, the average risk attitude of consumers is 2.45. It implies that most people have a weaker tolerance level for food control risks.
Other potential factors affecting consumers' information-seeking intention are introduced; such as individual basic characteristics, namely, gender, education, age, and marriage. Family characteristics include the demographic structure and family income. Furthermore, individual cognition characteristics include food safety perception, origin concern, information demand, and traceability cognition, food poisoning experience.
Among them, by referring to the existing relevant research experience (Michaelidou and Hassan, 2010; Xu et al., 2019), food safety perception is measured by four questions. (1) How concerned are you about the excessive pesticide residues in food? (2) How concerned are you about the illegal use of additives in food? (3) How concerned are you about the overuse of antibiotics in food? (4) How concerned about food-borne disease outbreaks (e.g., disease-causing bacteria in fresh fruits and vegetables)? Responses to these four questions were taken on a 5-point Likert scale (1 not at all concerned to 5 extremely concerned). Finally, the average value of the four question options represents the consumers' food safety perception level. The larger the value, the stronger the food safety perception. The specific definitions and descriptive statistics of all variables are shown in Table 1.
Since consumers' information-seeking intention variables belong to typical sorting data, following the literature (Wooldridge, 2010; Li et al., 2020; Vita et al., 2022), an Ordered logit model (hereinafter referred to as Ologit) is constructed. The specific setting of the model is as follows:
In formula (1), is the latent variable of consumers' information-seeking intention, Riski is consumers' risk attitude; Controlim is m control variables that may affect consumer information-seeking intention; α0 is a constant term, γ1 and βm are the parameters to be estimated in the model, εi is the residual disturbance term, and it is assumed to follow the standard normal distribution.
In formula (2), Infseri represents the consumer information seeking, and C1~C5 are the cut points. When , consumers are extremely unlikely to seek information (); when , consumers are unlikely to seek information (); when , consumers are uncertain about information seeking (); when , consumers are likely to seek information (), and when , consumers are extremely likely to seek information (). From equation (2), the likelihood function of the sample can be obtained, and the MLE estimator can be obtained, which is the Ologit model. Since the economic meaning of the estimated coefficients of the Ologit model is not intuitive and the amount of information given is limited, this study mainly reports the marginal effect of each explanatory variable on the information-seeking intention ().
Since the estimation results of the OLS regression and the ordered selection model are highly consistent (Ferrer-I-Carbonell and Frijters, 2004), this study estimated the OLS model and Ologit model simultaneously to use the OLS estimates as a reference. The results are shown in Table 2; only the core explanatory variable risk attitude is introduced in columns (1) and (4), the control variables are introduced in columns (2) and (5), and the control variables and regional dummy variable are introduced in columns (3) and (6).
Comparing the regression results, among the six regression results, the impact of risk attitude on consumer information seeking is significantly negative at a 1% level. It indicates the stronger the degree of risk preference of consumers, in other words, the greater the tolerance of food quality and safety risk, the less they seek information by scanning the blockchain traceable QR code on the packaging of agricultural products. These findings are consistent with the research conclusions of Quan and Zeng (2013). Hence, the higher the degree of food safety risk aversion, the higher the amount of consumer information seeking, because high perception may increase consumers' uncertainty in seeking information to evaluate risks (Verbeke, 2005a; Kuttschreuter, 2006). Besides, higher risk perception regarding food control risks makes people feel more anxious and threatened, and expands the gap between the information they need and their relevant knowledge, which will further stimulates information-seeking attitude (Yang et al., 2020). Based on the estimate in column (6), consumers with high-risk preferences have a 5.7% lower probability of information seeking than consumers with low-risk preferences.
In terms of other control variables, most variables significantly impact consumers' information seeking; these findings also endorse prior results (Dinpanah and Lashgarara, 2011; Quan and Zeng, 2013). Compared to men, women's information-seeking intention for fresh fruits is weaker. This finding supports the strand of literature on the role of gender in online shopping. Prior studies indicated that women have less trust in online shopping than men (Rodgers and Harris, 2003). In terms of age, middle-aged and young people have stronger information-seeking intentions. It implies that the higher the consumer's age, the lower the acceptance of new knowledge and skills, and the weaker the intention to obtain product information by scanning blockchain traceable QR codes. From the perspective of marginal effect, young people have the strongest information-seeking intention. According to Demirci et al. (2021), young people are more inclined toward the latest technologies, processes, and information seeking. Regarding family size, families with fewer children have stronger intentions to seek information, and families with more children do not heed information seeking. The presence of children in the household drives parents' motivation to seek adequate nutrition and wholesome food (Verbeke, 2005b). In terms of income, middle and high-income groups pay more attention to product quality and safety and have a stronger intention to obtain product information attributes. Regarding the marginal effect, high-income groups have the strongest intention to seek information. These results also align with Beaudoin and Hong (2011) findings, suggesting that income is positively related to information seeking. The stronger consumers' perception of food safety, the more they are inclined to seek information related to product quality.
The results of individual cognitive characteristics indicate that the more consumers are likely to pay more attention to the origin of products, the more they scan the blockchain traceable QR code to seek product information, especially the information related to the origin of products. Information demand and traceability cognition have a significant positive impact on consumers' information seeking. It implies that the higher consumers' information demand and cognition of traceable agricultural products, the stronger the intention to obtain product information by scanning blockchain traceable QR codes.
We use the propensity score matching to correct the possible presence of self-selection bias and improve the robustness of the empirical results. First, according to the variable characteristics of the PSM model, the variable of consumer risk attitude is reconstructed, the value of consumer risk attitude ≥2.5 is considered risk preference type, with a value of 1, and the value <2.5 is taken risk aversion type, with a value of 0. Furthermore, consumers are divided into risk preference and risk aversion. The propensity scores of the two groups of samples are matched. The probability distribution of the propensity scores of the two groups before and after matching is shown in Figure 3. Before matching, the difference between risk preference and risk aversion consumers is significant, decreasing significantly after matching, indicating that the matching effect is good.
Figure 3. Probability distribution of propensity score value between pre-processing group and control group.
This study calculates the average treatment effect on the treated (ATT) of the matched risk preference and risk aversion samples; the results are shown in Table 3. Whether it is nearest neighbor matching, local linear matching, or spline matching with 500 iterations of the autonomous sampling method, the results of ATT show that after eliminating the observable systematic differences between samples, the intention of risk preference consumers to seek product information is weaker. This implies that consumers with strong risk preferences are less likely to scan blockchain traceable QR codes.
Furthermore, the ATT calculated by nearest neighbor matching (1:1) is significant at the statistical level at 5%, and the other three matching results are significant at 1%. The ATT value obtained after spline matching is the largest, which is −0.202, and the ATT value obtained after nearest neighbor matching (1:1) is the smallest, which is −0.171. The ATT values obtained by different matching methods have little difference, with a mean value of −0.189. Thus, risk preference consumers have weaker information-seeking intentions than risk-averse consumers. These results endorse the robustness of the above empirical estimates based on the OLS and Ologit model.
Heterogeneity analysis generally divides the total sample into different groups according to a certain standard, to explore the differences in the results of the same study in different samples. The above analysis confirms the impact of consumers' risk attitude on fresh fruit information seeking from the full sample level; it does not consider the differences between different consumer groups. Therefore, we further investigate the age and education heterogeneity of the impact of risk attitude on consumers' information seeking. The estimated results are shown in Table 4.
Among the age group, the risk attitude of the elderly is not related to information seeking, which may be due to the small sample size of the elderly group, only 35 samples. For young and middle-aged people, risk attitude significantly negatively impacts their information seeking at the statistical level of 1% and 10%, respectively. The marginal effect indicates that the probability of young people with a strong risk preference for information seeking is significantly lower than young people with a weak risk preference by 5.9%. Likewise, the probability of middle-aged people with strong risk preference seeking information with blockchain QR codes is significantly lower than middle-aged people with weak risk preference by 4.5%. It indicates that young people with strong risk preferences have a weaker intention to seek information. It suggests that the risk attitude greatly impacts young people's information seeking. The possible reason is that the information-seeking motivation of young people is stronger than the elderly (Demirci et al., 2021).
From the grouping of education level, the risk attitude of the group with graduate education or above has no significant impact on information seeking, which may be related to the small sample size of this group, only 106 samples. For groups with high school and below education and university education, the impact of risk attitude on information seeking is significantly negative at the statistical level of 1%. From the perspective of marginal effect, the probability of information seeking in high school and below educated groups with strong risk preference is significantly lower than those with weak risk preference by 13.9%. The probability of information seeking in college-educated groups with strong risk preference is significantly lower than that of those with weak risk preference by 5.4%, which indicates that the intention of information seeking in high school and below educated groups with strong risk preference is weaker. The risk attitude has a greater impact on information seeking of groups with high school education and below. Because people with a lower education level perceived the information to be significantly more complex than higher educated participants (Rutsaert et al., 2015), it is more necessary for them to seek traceable information to make rational decisions.
Referring to existing research (Zhai et al., 2022), we follow a range of robustness measures to test the robustness of the aforementioned results. First, we applied the change model and replaced the Ologit model with the Oprobit model for regression. Second, we transformed the core explanatory variable and used the binary risk attitude variable in the above PSM analysis to conduct the regression of Ologit and Oprobit, respectively. Third, transformed the explained variable and combined the “extremely unlikely” and “unlikely” in the information seeking of the explained variable into “unlikely,” “likely” and “extremely likely” into “likely,” assigning values of 1 and 3, respectively. Likewise, we assign values of “neither likely nor unlikely” to 2 to form a new explained variable and carry out the regression of the Ologit model and Oprobit model. The robustness test results are shown in Table 5. The risk attitude variable is significantly negative at the statistical level of 1%. Thus, it supports the conclusion that risk attitude significantly and negatively impacts consumers' information seeking.
Blockchain traceability information is making headway in the marketing sector to mitigate information asymmetry and optimize processes and efficiencies. As the agri-food sector embraces the benefits of blockchain technology, the need to stay competitive would ultimately drive the transformation to information traceability. The nature of markets mandates competitors to stay relevant to survive, which results in broader agri-food information disclosure and consumer welfare. Likewise, information seeking is feasible for consumers to screen product quality to ensure food quality and safety. This study takes fresh fruit with blockchain traceability QR labels as an example to systematically investigate the impact of risk attitude on consumers' information seeking. This study used online survey data of 1,058 fresh fruit buyers from four traceability pilot project cities in China. The study provides several insights into promoting the broader trajectory of the food blockchain traceability system.
The study findings provide coherent policy measures for food suppliers to realize a broader agri-food information traceability. First, the findings show that risk attitude negatively impacts consumers' information-seeking intention. It implies that the stronger consumers' risk preference, the probability of “extremely likely” seeking information through scanning blockchain traceable QR code is significantly reduced by 5.7%. Second, we used propensity score matching to correct the possible presence of self-selection bias and improve the robustness of the empirical results. The PSM results reinforce the OLS results above and justify the appropriateness of the study estimates. It suggests that the stronger the risk preference, the weaker the consumers' information-seeking intention.
Third, the sub-sample estimate shows that risk attitude's impact on consumer information seeking is heterogeneous in age and education. Specifically, the impact of the risk attitude of young people on information seeking is stronger than others. Likewise, the risk attitude of high school and below education groups on information seeking is stronger than that of university education groups. These findings imply that blockchain traceability information improves the consumers' confidence in agri-food products derived from encrypted information and certification. Likewise, findings endorse the notion that young, educated, high-income, and men have stronger positive intentions for blockchain traceable information. Therefore, improving the trust component and expectations about blockchain operations to create a stronger technology-based information system is apparent.
Furthermore, the heterogeneity in consumers' characteristics might be an opportunity to tap the world's biggest and most diversified Chinese population. The findings suggest that organizations and marketing endeavors should concentrate more on creating awareness about the effectiveness and transparency of blockchain traceability. The study inculcates that the blockchain enterprises' concentration should be directed to influence the household decision-makers to prefer blockchain traceable foods. Moreover, the blockchain traceability system should upgrade anticounter measures for stakeholders in the manufacturing domain to overcome loopholes and distrust elements along with the supply chain traceability system.
This work helps blockchain food enterprises better understand the role of consumers' food control risk attitude in seeking traceable agri-food information. Based on the conclusions, the following policy implications are suggested.
First, it is apparent to ensure the effectiveness of the blockchain traceability system to revamp the consumer trust in the new value chain and advanced systems. Consumers' information seeking intends to obtain more information about the quality and safety of agricultural products through the blockchain traceability system, which can convert the trust attributes of food into the search attributes to reduce consumers' risk perception, which is conducive to better product diagnosis and purchase decisions. Therefore, agricultural enterprises should improve the attractiveness and perceived usefulness of the blockchain traceability system. QR codes can become the main label of blockchain traceability authentication products. Consumers can easily access, track and verify the traceability information by scanning the label through smartphones, thus reducing the learning cost of consumers on the blockchain traceability system of fresh agricultural products.
Second, blockchain traceability enterprises should use correct information to guide consumers' risk attitudes toward food quality and safety. In such a way, it would become an effective means to build consumer confidence in traceable food quality and safety information. Simultaneously, it might help regulate market mechanisms to ensure food quality and safety through blockchain traceability, and therefore, can break the dilemma of the “lemon market” of agricultural products. Third, at the regional level, new traceable certification—like blockchain traceability—is suggested in the regions with relatively high economic development to realize a rapid and broader acceptance of such profound information disclosing system. Furthermore, there is a dire need to promote blockchain traceability, especially by targeting highly educated and young groups, individuals, and areas with the upper class and elite living to help promote blockchain traceability on a wider scale.
The study rigorously addresses the proposed questions, yet it has some limitations, which might be avenues for future research. First, this study does not further discuss the impact of risk attitude on consumers' actual information-seeking costs and associated benefits. Likewise, this study overlooked the deviation between consumers' information-seeking intention and behavior. Future studies can build a theoretical framework to analyze the factors influencing this deviation to put forward more practical strategies for promoting blockchain traceability systems. Second, we take the fresh fruit as the research case; although it has relevance, it may lack general application. Whether consumers are willing to use blockchain information labels may vary according to different categories of agricultural products. In the future, it can be extended to more categories of agricultural products, such as pork, rice, and vegetables. It would help explore the impact of risk attitude using blockchain traceability systems for different agricultural products to develop specific blockchain traceability information systems solutions to meet consumers' needs. Last, the traceability information seeking studied in this article does not specifically refer to the type of information searched. Further studies can investigate consumers' preferences for traceability information of locally produced or short-chain food.
The raw data supporting the conclusions of this article will be made available by the authors, without undue reservation.
Ethical review and approval was not required for the study on human participants in accordance with the local legislation and institutional requirements. The patients/participants provided their written informed consent to participate in this study.
QZ, AS, QL, and CC: conceptualization, writing the original draft, reviewing, editing, and resources. QZ and CC: formal analysis and investigation. QZ, AS, and QL: methodology. CC: supervision and funding acquisition. All authors contributed to the article and approved the submitted version.
This research was supported by the Earmarked Fund for China Agriculture Research System (CARS-30).
The authors declare that the research was conducted in the absence of any commercial or financial relationships that could be construed as a potential conflict of interest.
All claims expressed in this article are solely those of the authors and do not necessarily represent those of their affiliated organizations, or those of the publisher, the editors and the reviewers. Any product that may be evaluated in this article, or claim that may be made by its manufacturer, is not guaranteed or endorsed by the publisher.
Baccelli, J. (2018). Risk attitudes in axiomatic decision theory: a conceptual perspective. Theor. Decis. 84, 61–82. doi: 10.1007/s11238-017-9636-6
Baralla, G., Pinna, A., and Corrias, G. (2019). “Ensure traceability in European food supply chain by using a blockchain system,” in 2019 IEEE/ACM 2nd International Workshop on Emerging Trends in Software Engineering for Blockchain (WETSEB). (Montreal. Canada), 40–47.
Bearth, A., and Siegrist, M. (2016). Are risk or benefit perceptions more important for public acceptance of innovative food technologies: a meta-analysis. Trends Food Sci. Tech. 49, 14–23. doi: 10.1016/j.tifs.2016.01.003
Beaudoin, C. E., and Hong, T. (2011). Health information seeking, diet and physical activity: an empirical assessment by medium and critical demographics. Int. J. Med. Inform. 80, 586–595. doi: 10.1016/j.ijmedinf.2011.04.003
Bojnec, S., Petrescu, D. C., Petrescu-Mag, R. M., and Radulescu, C. V. (2019). Locally produced organic food: Consumer preferences. Amfiteatru Econ. 21, 209–227. doi: 10.24818/EA/2019/50/209
Boot, C. R. L., and Meijman, F. J. (2010). The public and the internet: Multifaceted drivers for seeking health information. Health Inf. Manag. J. 16, 145–156. doi: 10.1177/1460458210364786
Chang, L., Yen, C. C., Xue, L., Tai, B. C., Chan, H. C., Duh, H. B., et al. (2017). Factors associated with mobile health information seeking among Singaporean women. J. Women Aging 29, 75–86. doi: 10.1080/08952841.2015.1065144
China Internet Network Information Center. (2021). The 48th Statistical Report on China's Internet Development. Available online at: http://www.cnnic.net.cn/hlwfzyj/hlwxzbg/hlwtjbg/202109/P020210915523670981527.pdf (accessed August 5, 2022).
Choi, J., Lee, A., and Ok, C. (2013). The effects of consumers' perceived risk and benefit on attitude and behavioral intention: a study of street food. J. Travel Tour. Mark. 30, 222–237. doi: 10.1080/10548408.2013.774916
Collart, A. J., and Canales, E. (2022). How might broad adoption of blockchain-based traceability impact the U.S. fresh produce supply chain? Appl. Econ. Perspect. 44, 219–236. doi: 10.1002/aepp.13134
Creydt, M., and Fischer, M. (2019). Blockchain and more-algorithm driven food traceability. Food Control 105, 45–51. doi: 10.1016/j.foodcont.2019.05.019
Demirci, S., Ugurluoglu, O., Konca, M., and Cakmak, C. (2021). Socio-demographic characteristics affect health information seeking on the Internet in Turkey. Health Inf. Libr. J. 38, 304–312. doi: 10.1111/hir.12358
Deng, Z., and Liu, S. (2017). Understanding consumer health information-seeking behavior from the perspective of the risk perception attitude framework and social support in mobile social media websites. Int. J. Med. Inform. 105, 98–109. doi: 10.1016/j.ijmedinf.2017.05.014
Desta, A. G., du Preez, M., and Ngulube, P. (2019). Factors affecting the information-seeking behavior of postgraduate students at the University of South Africa Ethiopia Regional Learning Centre. Inf. Dev. 35, 362–373. doi: 10.1177/0266666917744824
Dey, S., Saha, S., Singh, A. K., and McDonald-Maier, K. (2021). FoodSQRBlock: Digitizing food production and the supply chain with blockchain and QR code in the cloud. Sustainability 13, 3486. doi: 10.3390/su13063486
Dey, S., Saha, S., Singh, A. K., and McDonald-Maier, K. (2022). SmartNoshWaste: Using blockchain, machine learning, cloud computing and QR code to reduce food waste in decentralized web 3.0 enabled smart cities. Smart Cities 5, 162–176. doi: 10.3390/smartcities5010011
Dinpanah, G., and Lashgarara, F. (2011). Factors influencing the information seeking knowledge of wheat farmers in Iran. Afr. J. Agric. Res. 6, 3419–3427. doi: 10.5897/AJAR11.164
Dionysis, S., Chesney, T., and McAuley, D. (2022). Examining the influential factors of consumer purchase intentions for blockchain traceable coffee using the theory of planned behaviour. Br. Food J. doi: 10.1108/BFJ-05-2021-0541
Evans, K. S., Teisl, M. F., Lando, A. M., and Liu, S. (2020). Risk perceptions and food-handling practices in the home. Food Policy 95, 101939. doi: 10.1016/j.foodpol.2020.101939
Ferrer-I-Carbonell, A., and Frijters, P. (2004). How important is methodology for the estimates of the determinants of happiness?. Econ. J. 114, 641–659. doi: 10.1111/j.1468-0297.2004.00235.x
Fleming, C. M., and Bowden, M. (2009). Web-based surveys as an alternative to traditional mail methods. J. Environ. Manage. 90, 284–292. doi: 10.1016/j.jenvman.2007.09.011
Frewer, L. J., Bergmann, K., Brennan, M., Lion, R., Meertens, R., Rowe, G., et al. (2011). Consumer response to novel agri-food technologies: implications for predicting consumer acceptance of emerging food technologies. Trends Food Sci. Tech. 22, 442–456. doi: 10.1016/j.tifs.2011.05.005
Galvez, J. F., Mejuto, J. C., and Simal-Gandara, J. (2018). Future challenges on the use of blockchain for food traceability analysis. TRAC-Trend Anal. Chem. 107, 222–232. doi: 10.1016/j.trac.2018.08.011
Gong, X., Wang, M., Zhang, Y., and Zhang, G. (2014). Risk perception and information search behavior with Shanghai residents in the purchase of pork. J. Shanghai Bus. School. 15, 80–85, 101. Available online at: https://mall.cnki.net/magazine/article/SHSS201406018.htm
Grasso, K. L., and Bell, R. A. (2015). Understanding health information seeking: a test of the risk perception attitude framework. J. Health Commun. 20, 1406–1414. doi: 10.1080/10810730.2015.1018634
Ha, T. M., Shakur, S., and Do, K. H. P. (2020). Risk perception and its impact on vegetable consumption: a case study from Hanoi, Vietnam. J. Clean. Prod. 271, 122793. doi: 10.1016/j.jclepro.2020.122793
Hassan, S. H., Kua, J. S. B., and Harun, H. (2016). Muslim consumers' perception and purchase intention toward GM food. Int. Food Res. J. 23, 806–815. Available online at: http://www.ifrj.upm.edu.my/23%20(02)%202016/(47).pdf
He, R., and Li, Y. (2021). Media exposure, cance beliefs, and cancer-related information-seeking or avoidance behavior patterns in China. Int. J. Environ. Res. Public Health 18, 3130. doi: 10.3390/ijerph18063130
Heikkila, J., Pouta, E., Forsman-Hugg, S., and Makela, J. (2013). Heterogeneous risk perceptions: The case of poultry meat purchase intentions in Finland. Int. J. Environ Res. Public Health 10, 4925–4943. doi: 10.3390/ijerph10104925
Hoffmann, V., Moser, C. M., and Herrman, T. J. (2021). Demand for aflatoxin-safe maize in Kenya: Dynamic response to price and advertising. Am. J. Agric. Econ. 103, 275–295. doi: 10.1111/ajae.12093
Hu, W., and Veeman, A. (2006). Labeling context and reference point effects in models of food attribute demand. Am. J. Agric Econ. 88, 1034–1049. doi: 10.1111/j.1467-8276.2006.00914.x
Islam, S., and Cullen, J. M. (2021). Food traceability: a generic theoretical framework. Food Control 123, 107–848. doi: 10.1016/j.foodcont.2020.107848
Jin, H., Wang, H., Gong, C., and Liu, L. (2020). A study on the influencing factors of consumer information-seeking behavior in the context of ambient intelligence. J. Ambient. Intell. Hum. Comput. 11, 1397–1404. doi: 10.1007/s12652-018-1005-y
Jin, S., Zhang, Y., and Xu, Y. (2017). Amount of information and the willingness of consumers to pay for food traceability in China. Food Control 77, 163–170. doi: 10.1016/j.foodcont.2017.02.012
Jin, S., and Zhou, L. (2014). Consumer interest in information provided by food traceability systems in Japan. Food Qual. Prefer. 36, 144–152. doi: 10.1016/j.foodqual.2014.04.005
Kelly, C. A., and Sharot, T. (2021). Individual differences in information-seeking. Nat. Commun. 12, 7062. doi: 10.1038/s41467-021-27046-5
Kleef, E. V., Frewer, L. J., Chryssochoidis, G. M., Houghton, J. R., Korzen-Bohr, S., Krystallis, T., et al. (2006). Perceptions of food risk management among key stakeholders: Results from a cross-European study. Appetite 47, 46–63. doi: 10.1016/j.appet.2006.02.002
Köhler, S., and Pizzol, M. (2020). Technology assessment of blockchain-based technologies in the food supply chain. J. Clean. Prod. 269, 122193. doi: 10.1016/j.jclepro.2020.122193
Kotler, P., and Keller, K. L. (2013). Marketing Management, 14th Edn. Upper Saddle River, NvJ: Pearson Education International.
Kuttschreuter, M. (2006). Psychological determinants of reactions to food risk messages. Risk Anal. 26, 1045–1057. doi: 10.1111/j.1539-6924.2006.00799.x
Lagerkvist, C. J., Hess, S., Okello, J., and Karanja, N. (2013). Consumer willingness to pay for safer vegetables in urban markets of a developing country: The case of Kale in Nairobi, Kenya. J. Dev. Stud. 49, 365–382. doi: 10.1080/00220388.2012.724165
Lee, S. H. (2014). The role of consumers' network positions on information-seeking behavior of experts and novices: a power perspective. J. Bus. Res. 67, 2853–2859. doi: 10.1016/j.jbusres.2012.07.002
Li, Q., Wang, J., Wang, X., and Wang, Y. (2020). The impact of alternative policies on livestock farmers' willingness to recycle manure: evidence from central china. China Agr. Econ. Rev. 12, 583–594. doi: 10.1108/CAER-09-2019-0158
Lin, Q., Wang, H., Pei, X., and Wang, J. (2019). Food safety traceability system based on blockchain and epcis. IEEE Access 7, 20698–20707. doi: 10.1109/ACCESS.2019.2897792
Lin, W., Ortega, D. L., Ufer, D., Caputo, V., and Awokuse, T. (2022). Blockchain-based traceability and demand for US beef in China. Appl. Econ. Perspect. 44, 253–272. doi: 10.1002/aepp.13135
Lin, X., Chang, S., Chou, T., Chen, S., and Ruangkanjanases, A. (2021). Consumers' intention to adopt blockchain food traceability technology towards organic food products. Int. J. Environ. Res. Public Health 18, 912. doi: 10.3390/ijerph18030912
Lioutas, E. D. (2014). Food consumer information behavior: need arousal, seeking behavior, and information use. J. Agric. Food Inf. 15, 81–108. doi: 10.1080/10496505.2014.880655
Liu, R., and Wu, L. (2013). Study on factors affecting consumers' food safety information search behavior: taking pork as an example. Lanzhou Acad. J. 34, 104–110. Available online at: https://mall.cnki.net/magazine/article/LZXK201311018.htm
Loo, E. J. V., Caputo, V. Jr, R. M. N., Meullenet, J., and Ricke, S. C. (2011). Consumers' willingness to pay for organic chicken breast: evidence from choice experiment. Food Qual. Prefer. 22, 603–613. doi: 10.1016/j.foodqual.2011.02.003
Lusk, J. L., and Coble, K. H. (2005). Risk perceptions, risk preference, and acceptance of risky food. Am. J. Agric. Econ. 87, 393–405. doi: 10.1111/j.1467-8276.2005.00730.x
Markovic, M., Jacobs, N., Dryja, K., Edwards, P., and Strachan, N. J. C. (2020). Integrating internet of things, provenance, and blockchain to enhance trust in last mile food deliveries. Front. Sustain. Food Syst. 4, 563424. doi: 10.3389/fsufs.2020.563424
Michaelidou, N., and Hassan, L. M. (2010). Modeling the factors affecting rural consumers' purchase of organic and free-range produce: a case study of consumers' from the Island of Arran in Scotland, UK. Food Policy 35, 130–139. doi: 10.1016/j.foodpol.2009.10.001
National Bureau of Statistics. (2020). China Statistical Yearbook. Yearbook Editorial Board: Beijing, China. Available online at: http://www.stats.gov.cn/ (accessed April 5, 2021).
Nielsen, J. S. (2011). Use of the internet for willingness-to-pay surveys: a comparison of face-to-face and web-based interviews. Resour. Energy Econ. 33, 119–129. doi: 10.1016/j.reseneeco.2010.01.006
Niknejad, N., Ismail, W., Bahari, M., Hendradi, R., and Salleh, A. Z. (2021). Mapping the research trends on blockchain technology in food and agriculture industry: a bibliometric analysis. Environ. Technol. Inno. 21, 101272. doi: 10.1016/j.eti.2020.101272
Olsen, S. B. (2009). Choosing between internet and mail survey modes for choice experiment surveys considering non-market goods. Environ. Res. Econ. 44, 591–610. doi: 10.1007/s10640-009-9303-7
Pennings, J. M., Wansink, B., and Meulenberg, M. T. (2002). A note on modeling consumer reactions to a crisis: the case of the mad cow disease. Int. J. Res. Mark. 19, 91–100. doi: 10.1016/S0167-8116(02)00050-2
Prashar, D., Jha, N., Jha, S., Lee, Y., and Joshi, G. P. (2020). Blockchain-based traceability and visibility for agricultural products: a decentralized way of ensuring food safety in india. Sustainability 12, 3497. doi: 10.3390/su12083497
Quan, S., and Zeng, Y. (2013). Study on consumer's behavior of food safety information searching: Based on the investigation of the consumers in Beijing. J. Agrotech. Econ. 32, 43–52. doi: 10.13246/j.cnki.jae.2013.04.004
Rodgers, S., and Harris, M. A. (2003). Gender and e-commerce: an exploratory study. J. Advert. Res. 43, 322–329. doi: 10.1017/S0021849903030307
Rodriguez-Salvador, B., and Dopico, D. C. (2020). Understanding the value of traceability of fishery products from a consumer perspective. Food Control 112, 107–142. doi: 10.1016/j.foodcont.2020.107142
Rutsaert, P., Barnett, J., Gaspar, R., Marcu, A., Pieniak, Z., Seibt, B., et al. (2015). Beyond information seeking: Consumers' online deliberation about the risks and benefits of red meat. Food Qual. Prefer. 39, 191–201. doi: 10.1016/j.foodqual.2014.07.011
Saba, A., and Messina, F. (2003). Attitudes towards organic foods and risk/benefit perception associated with pesticides. Food Qual. Prefer. 14, 637–645. doi: 10.1016/S0950-3293(02)00188-X
Savolainen, R. (2015). Cognitive barriers to information seeking: a conceptual analysis. J. Inf. Sci. 41, 613–623. doi: 10.1177/0165551515587850
Schroeder, T. C., Tonsor, G. T., Pennings, J. M. E., and Mintert, J. (2007). Consumer food safety risk perceptions and attitudes: impacts on beef consumption across countries. BE J. Econ. Anal. Policy 7, 65. doi: 10.2202/1935-1682.1848
Szabo, K., Piko, B. F., and Fitzpatrick, K. M. (2019). Adolescents' attitudes towards healthy eating: The role of self-control, motives and self-risk perception. Appetite 143, 104416. doi: 10.1016/j.appet.2019.104416
Thomasson, E. (2019). Carrefour Says Blockchain Tracking Boosting Sales of Some Products. Available online at: https://www.reuters.com/article/us-carrefour-blockchain-idUSKCN1T42A5 (accessed December 10, 2021).
Tonsor, G. T., and Shupp, R. S. (2011). Cheap talk scripts and online choice experiments: “looking beyond the mean.” Am. J. Agr. Econ. 93, 1015–1031. doi: 10.1093/ajae/aar036
Turner, M. M., Rimal, R. N., Morrison, D., and Kim, H. (2006). The role of anxiety in seeking and retaining risk information: testing the risk perception attitude framework in two studies. Hum. Commun. Res. 32, 130–156. doi: 10.1111/j.1468-2958.2006.00006.x
Valoppi, F., Agustin, M., Abik, F., de Carvalho, D. M., Sithole, J., Bhattarai, M., et al. (2021). Insight on current advances in food science and technology for feeding the world population. Front. Sustain. Food Syst. 5, 626227. doi: 10.3389/fsufs.2021.626227
Verbeke, W. (2005a). Agriculture and the food industry in the information age. Eur. Rev. Agric. Econ. 32, 347–368. doi: 10.1093/eurrag/jbi017
Verbeke, W. (2005b). Consumer acceptance of functional foods: socio-demographic, cognitive and attitudinal determinants. Food Qual. Prefer. 16, 45–57. doi: 10.1016/j.foodqual.2004.01.001
Vita, G. D., Zanchini, R., Spina, D., Maesano, G., Via, G. L., and D'Amico, M. (2022). Exploring purchasing determinants for a low fat content salami: Are consumers willing to pay for an additional premium? Front. Sustain. Food Syst. 6:794533. doi: 10.3389/fsufs.2022.794533
Wang, H., Xiao, J., Xie, K., and Wu, J. (2013). Food safety governance: a study combing information technology and institution arrangement. China Ind. Econ. 31, 98–110. doi: 10.19581/j.cnki.ciejournal.2013.03.009
Windle, J., and Rolfe, J. (2011). Comparing responses from internet and paper-based collection methods in more complex stated preference environmental valuation surveys. Econ. Anal. Policy 41, 83–97. doi: 10.1016/S0313-5926(11)50006-2
Wooldridge, J. M. (2010). Econometric Analysis of Cross Section and Panel Data, 2nd Edn. London, England: MIT press.
Wu, W., and Chang, M. (2007). The role of risk attitude on online shopping: Experiencing, customer satisfaction, and repurchase intention. Soc. Behav. Personal. 35, 453–468. doi: 10.2224/sbp.2007.35.4.453
Xu, S., Xia, K., and Cai, Y. (2019). Study on the influential factors of consumer acceptance for the food traceability system-based on an extended technology acceptance model perspective. J. Guizhou Univ. Financ. Econ. 37, 82–92. Available online at: https://gcxb.gufe.edu.cn/CN/Y2019/V37/I01/82
Yang, Z., and Kahlor, L. (2013). What, me worry? The role of affect in information seeking and avoidance. Sci. Commun. 35, 189–212. doi: 10.1177/1075547012441873
Yang, Z., Paudel, K. P., Wen, X., Sun, S., and Wang, Y. (2020). Food safety risk information-seeking intention of wechat users in China. Int. J. Environ. Res. Public Health 17, 2376. doi: 10.3390/ijerph17072376
Zhai, Q., Sher, A., and Li, Q. (2022). The impact of health risk perception on blockchain traceable fresh fruits purchase intention in China. Int. J. Environ. Res. Public Health 19, 7917. doi: 10.3390/ijerph19137917
Zhang, L., and Liu, G. (2010). Empirical analysis on consumers information searching behavior of fresh food quality and safety: Based on the fresh food consumption survey in Shanghai. J. Agrotech. Econ. 29, 97–103. doi: 10.13246/j.cnki.jae.2010.02.002
Zhao, S., and Liu, Y. (2021). The more insufficient, the more avoidance? Cognitive and affective factors that relates to information behaviors in acute risks. Front. Psychol. 12, 730068. doi: 10.3389/fpsyg.2021.730068
Keywords: risk attitude, information-seeking, blockchain traceability, fresh fruit, ordered logit model, heterogeneity
Citation: Zhai Q, Sher A, Li Q and Chen C (2022) Consumers' food control risk attitude for blockchain traceable information seeking: Evidence from fresh fruit buyers in China. Front. Sustain. Food Syst. 6:984493. doi: 10.3389/fsufs.2022.984493
Received: 02 July 2022; Accepted: 12 August 2022;
Published: 07 September 2022.
Edited by:
Hsin-Bai Yin, Agricultural Research Service (USDA), United StatesReviewed by:
Štefan Bojnec, University of Primorska, SloveniaCopyright © 2022 Zhai, Sher, Li and Chen. This is an open-access article distributed under the terms of the Creative Commons Attribution License (CC BY). The use, distribution or reproduction in other forums is permitted, provided the original author(s) and the copyright owner(s) are credited and that the original publication in this journal is cited, in accordance with accepted academic practice. No use, distribution or reproduction is permitted which does not comply with these terms.
*Correspondence: Chao Chen, Y2NoZW5AbmphdS5lZHUuY24=
Disclaimer: All claims expressed in this article are solely those of the authors and do not necessarily represent those of their affiliated organizations, or those of the publisher, the editors and the reviewers. Any product that may be evaluated in this article or claim that may be made by its manufacturer is not guaranteed or endorsed by the publisher.
Research integrity at Frontiers
Learn more about the work of our research integrity team to safeguard the quality of each article we publish.