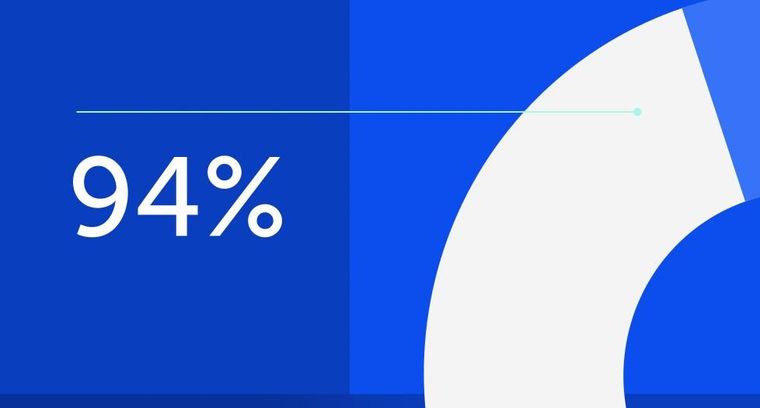
94% of researchers rate our articles as excellent or good
Learn more about the work of our research integrity team to safeguard the quality of each article we publish.
Find out more
ORIGINAL RESEARCH article
Front. Sustain. Food Syst., 08 December 2022
Sec. Agroecology and Ecosystem Services
Volume 6 - 2022 | https://doi.org/10.3389/fsufs.2022.974215
This article is part of the Research TopicThe Forgotten Pollinators: The Importance and Conservation of Wild PollinatorsView all 5 articles
Land use change has been identified as a cause for biodiversity loss and has significant effects on pollinators and their interactions with plants. Interaction network analyses complement diversity estimators by providing information on the stability and functionality of the plant-pollinator community in an ecosystem. However, how land use changes affect insect diversity, and the structure of their plant-insect interaction networks, could depend on the intensity of the disturbance but also may be a matter of scale. Our study was carried out in a tropical highland landscape dominated by intense, yet diverse, small-scale agriculture. We studied the effects of land use, at a landscape scale, and local cover and plant ecological descriptors, at a local scale; on diversity descriptors of insect pollinator communities, the abundance of the most frequent flower visitors, and their interaction networks. Seminatural vegetation favored insect flower visitors at both scales. At the landscape scale, human settlements positively influenced bee diversity, and seminatural areas favored the abundance of frequent hoverfly and bumblebee species. At the local scale, bare soil cover negatively influenced honeybee abundance while flower-rich covers positively related to bumblebee abundance. Only local scale variables had influence on network metrics. Bare soil cover was related to higher network specialization, probably due to a low rate of honeybee interactions. Flower-rich covers negatively influenced network connectance but favored modularity. These results suggest that flower resources, provided by weed areas and flowering crops, promote a high rate of interactions between trophic levels and a non-random structure in the interaction networks that may be helping to sustain network stability. Our results highlight the role of seminatural vegetation, at both scales, in maintaining stable insect pollinator communities and interactions in heterogeneous agricultural landscapes of the tropics.
Croplands and cultivated grasslands occupy ~30% of the surface of the Earth (Asner et al., 2004; Foley et al., 2005). It is estimated that by 2050 one billion hectares of croplands will be necessary to satisfy human food demand (Laurence et al., 2014), which entails a conflict between the amount of land dedicated to crops for human nutrition, and the preservation of natural areas that provide ecological services to humanity and agricultural areas, including animal-mediated pollination (Steffan-Dewenter et al., 2005). Further, insect-mediated pollination is necessary for reproduction and maintenance of plant populations, either wild or cultivated (Ollerton et al., 2011). It has been observed that crop yield generally increases with insect species richness, abundance, and visitation (Garibaldi et al., 2013; Kleijn et al., 2015; Rader et al., 2016; Woodcock et al., 2019). However, despite the fact that bees are perceived as the most important pollinators, both farmers and scientific studies recognize that other floral visitors, such as flies, are also important (Doyle et al., 2020: Raguso, 2020; Osterman et al., 2021). General trends show that insect pollinator populations are declining worldwide as a consequence of habitat loss (Potts et al., 2010; IPBES, 2016; Dicks et al., 2021). Nevertheless, not all pollinator taxa respond equally, which might be due to taxon-specific habitat requirements that may vary at different spatial scales (Jauker et al., 2009; Bates et al., 2011). In that way, understanding taxon-specific habitat requirements is key to developing effective pollinator conservation strategies in agricultural landscapes (Kohler et al., 2008; Jauker et al., 2009).
On the other hand, stable and robust populations of insect pollinators depend upon the availability of floral resources (i.e., pollen, nectar, and non-volatile oils) to feed adults and larvae, and non-floral resources (i.e., resins and nest availability) to protect brood cells of both eusocial and solitary bees (Requier and Leonhardt, 2020). Several studies suggest that flower-rich sites with intermediate levels of perturbation by human activities (e.g., human settlements and agriculture) can have a positive effect on bee and fly abundance and the total diversity of insect pollinators in temperate zones (Kratschmer et al., 2019; Aguilera et al., 2020; Wenzel et al., 2020; Bergholz et al., 2021) and tropical areas (Meng et al., 2012; Landaverde-González et al., 2017; Cusser et al., 2019; Escobedo-Kenefic et al., 2020; Coutinho et al., 2021). In this sense, habitat quality can strongly affect how insect pollinators proceed toward resources that are defined by land use (Grundel et al., 2010; Bates et al., 2011). The provision of high flower diversity to pollinator communities may mitigate the negative impacts of landscape perturbation on pollinator diversity (Bates et al., 2011; Birdshire et al., 2020). In addition, it has been demonstrated that agroforestry systems in the tropics where non-intensive agriculture is performed, play an important role in the conservation of pollinator diversity (Jha and Vandermeer, 2010; Landaverde-González et al., 2017; Vides-Borrell et al., 2019; Armas-Quiñonez et al., 2020) and plant-pollinator interactions (Klein et al., 2008; Perfecto et al., 2014; Escobedo-Kenefic et al., 2020) Also, tropical croplands are immersed in topographically diverse areas that may promote complex interactions among the habitat, pollinator diversity and plant-pollinator interaction systems, at landscape and local scales (Klein et al., 2008; Motzke et al., 2016; Cely-Santos and Philpott, 2019; Wayo et al., 2020).
Studies in tropical agroecosystems have found effects of land use on the diversity of insect pollinators at different scales, probably due to differences in ecological characteristics of the studied species or biological guild (Jauker et al., 2009; Burrascano et al., 2018). Some studies have identified that landscape factors like forest cover proportion (Wayo et al., 2020), crops cover (Cely-Santos and Philpott, 2019) and seminatural vegetation cover (Cusser et al., 2019) can explain pollinator diversity. Other studies have pointed out that small scale factors as habitat type (Tarakini et al., 2020), herbaceous stratum height (Hernández-Villa et al., 2020), flower richness (Landaverde-González et al., 2017; Escobedo-Kenefic et al., 2020; Tarakini et al., 2020; Wayo et al., 2020), and flower abundance (Cely-Santos and Philpott, 2019) can be predictors of pollinator diversity. However, pollinator communities may respond to land use both at landscape and local scales. In temperate regions, Ropars et al. (2020) found that large bee richness (i.e., more than 11.5 mm in body length) was associated negatively to seminatural vegetation areas at landscape scale while local scale plant richness variables were non-significant, and Benjamin et al. (2013) determined that large bees had a negative relationship with agricultural land use at landscape and local scales, while small bees were negatively associated to agricultural lands at local scale but positively related at landscape scale. In the tropics, Motzke et al. (2016) observed that bee abundance increased with flowering-plant cover at local scale whereas small bee abundance increased with closeness to forest. Also, Meng et al. (2012) recorded the highest hoverfly species richness in habitats with vegetation of early successional stages as rice fields and fallows at landscape scale, and found a positive significant relationship among hoverfly richness and weed richness at local scale.
As a complement to diversity descriptors like richness and diversity, ecological network analyses show how interactions among trophic levels affect the structure, composition, and distribution of biological communities (Bascompte and Jordano, 2007). Interaction networks have inherent properties as connectance, nestedness, specialization, generalization, and modularity. These metrics allow to visualize how interactions are assembled at community level, and also to indirectly assess ecosystem functions as pollination stability and robustness (Kaiser-Bunbury and Blüthgen, 2015; Kaiser-Bunbury et al., 2017; Burrascano et al., 2018). Some studies have shown that along with pollinator diversity, plant-pollinator network attributes such as robustness, nestedness and modularity, differ across seasons suggesting spatial and temporal niche partitioning between pollinator species (Souza et al., 2018; Rabeling et al., 2019; Escobedo-Kenefic et al., 2020; Stein et al., 2021; Assunção et al., 2022). Also, genetic diversity in the plant trophic level may act as a buffer to minimize the effect of landscape structure on ecological interaction networks ( Landaverde-González et al.,2021). However, to our knowledge, hitherto no analyses comparing between landscape and local scales have been done to understand which land use variables are important drivers of the topology of ecological interactions in the tropical highlands.
In this study, we investigate the effect of land use (at landscape scale), local cover, and plant population ecological descriptors (at a local scale) on the diversity of flower visitors and their interaction networks. We were interested in testing how landscape and local variables affect (1) the diversity of insect flower visitors, (2) the abundance of the most frequent flower visitor species, and (3) the stability of insect flower visitor interaction networks. We hypothesize that seminatural vegetation at landscape scale, and flowering weed local cover and floral abundance and diversity at local scale, will have a positive effect on the diversity of insect flower visitors and on the stability of the interaction networks between pollinators and flowering plants.
Our study was carried out in a seasonal agriculture area of the department of Chimaltenango, Guatemala, between 2,086 and 2,500 masl. In this area, agricultural cycles are adjusted to the rainy season; consequently, most crops grow through a single season (i.e., usually 6 months or fewer) (Altieri, 2004). Even though traditional-based agricultural practices (e.g., milpa system) are prevalent among local farmers, most of them use fertilizers and pesticides to improve the harvest yield of crops. Also, most of the land that is suitable for crops is being used all year long, even though some crop rotation is practiced. In this sense, agricultural practices are intensive in the study region (Carey, 2009). Agricultural land use is composed of a combination of non-traditional crops (i.e., carrots, cabbage, cauliflower, broccoli, and peas) and traditional crops (i.e., corn, avocado, lima beans, and beans) arranged in a highly heterogeneous mosaic of small areas (1,100–10,000 m2) of different crops and cover types (Guardiola and Bernal, 2009; Escobedo-Kenefic et al., 2014; Gálvez et al., 2014). Human settlements in our study area are predominantly rural in nature and often are surrounded by diverse and heterogeneous land use arrangements that may include housing areas, small forest patches, pastures and small farming plots (e.g., traditional-based milpa system) (Nigh and Diemont, 2013; Hellin et al., 2018). Forests are composed of long-established plants, mostly pine and oak trees, and seminatural vegetation consists of combined recently established plants, mostly small trees, bushes and weeds that comprise the early succession stages of plant community (Escobedo-Kenefic et al., 2014). We randomly selected 8 sites within the study area, separated by a minimum of 2 km (Figure 1; Supplementary Table S1). We selected our study area considering land use composition and configuration (e.g., size and shape of forest, crops, and settlement patches), so that our sites represented the variability of land use composition (i.e., mixture of forest, seminatural vegetation, crops, and settlements areas) of the study region.
Figure 1. Locations of study sites (site names as in Supplementary Table S1) in the highlands of Guatemala. The red dots represent the analysis units, the red circles represent the 2 km buffers used to obtain the landscape scale variables. Dark green = forest, light green = seminatural, yellow dash = seasonal agriculture, gray = human settlements. The location of the Chimaltenango department in the maps is shaded in yellow.
Fieldwork was carried out only once in each study site within a 1-month period (from December 16, 2019, to January 19, 2020). It was not possible to repeat the samplings due to the short duration of the wild vegetation blooming peak and to the COVID-19 lockdown.
To analyze the effect of local and landscape scales on the diversity of flower visitor community and their interaction networks, we calculated first land use composition at both scales using a modification of the methodology created by Cely-Santos and Philpott (2019). To characterize land use at a landscape scale, we obtained the area of each land use within a 1,000 m radius buffer using Google Earth images version 7.3.4.8642 (Google Earth/Maxar Technologies, 2020) with 15 meters resolution and the supervised classification algorithm from the ArcGIS software version (ESRI, 2014). We classified land uses within four categories: (i) forest, (ii) crops, (iii) seminatural vegetation, and (iv) human settlements (as defined above) (Supplementary Table S2).
In each study site, we defined a 100 × 100 m field plot that constituted our analysis units to determine land use composition at local scale. Further, to obtain a detailed description at the local scale, we measured a priori the area of each local cover within each 100 × 100 m field analysis unit using Google Earth images (2020). We defined local cover as each of the different land use types at local scale that we characterized within each 100 × 100 m field plot (Supplementary Figure S1). Subsequently, we field-verified each individual local cover. We classified the local covers within the following categories: (1) non-flowering crops (crops without pollinator-attractive flowers from growth to harvest, e.g., corn, carrots, cabbage, cauliflower, and broccoli), (2) flowering crops (e.g., pumpkin, lima beans, peas, and beans), (3) flowering weeds (i.e., abandoned crops mixed with naturally-grown flowering weeds and regeneration areas with flowering herbaceous plants and bushes), and (4) bare soil and/or dry crops (areas where crops were allowed to dry out, and most of the bare soil was exposed) (Supplementary Table S2). All eight 100 × 100 m field analysis units were located in agricultural lands. Each analysis unit was constituted as a unique combination of different kinds of agriculture and flowering weed areas but did not include any forest patches or human settlements.
Additionally, in each analysis unit we delimited 4 plant-community plots within flowering weeds local cover areas to perform a detailed characterization of plant ecological estimators. We performed 4 replicates of each 4 m2 plot (16 m2 in total per site, see details in Supplementary Figure S2). For each 4 m2 plot we measured (1) floral density (flowers/m2), (2) herbaceous stratum height, (as a measurement of stratum maturity in meters, measured from the floor to the top portion of the plants), and (3) plant species richness and abundance. From these last measurements we calculated Chao1 corrected richness (Chao and Shen, 2003) and Shannon diversity index (Shannon and Weaver, 1949) of weeds. Floral density and herbaceous stratum height were measured in all sites, but plant richness and abundance were measured in seven sites only (Supplementary Table S2). We intended to complete the data during a second sampling round, but this was not possible due to resource limitations and the reasons mentioned before.
Also, we standardized the local cover values (local scale) by converting the raw m2 values to proportions, as land use values (landscape scale) were already measured as proportions. Further, we calculated a land use heterogeneity index (LH) at landscape and local scales, derived from the Shannon diversity index (Supplementary Table S2). Landscape-scale LH was calculated using the land use categories, measured within 1,000 m buffer areas (forest, crops, seminatural vegetation, and human settlements). Local-scale LH was calculated using the local covers, measured within each 100 × 100 m analysis unit (bare soil/dry crops, flowering crops, flowering weeds, and non-flowering crops cover). Additionally, in order to compare land use effects between the two scales, we considered all agricultural use covers (bare soil/dry crops, flowering crops, and non-flowering crops), at local scale as equivalents to the “crops” use the landscape scale, and the “flowering weeds cover” at local scale as an equivalent to “seminatural vegetation” at landscape scale. Since the experiment was designed to measure local-scale variables in agricultural lands only, we do not have local-scale equivalents to the “forest” and “human settlements” landscape-scale categories. Lastly, we used a Pearson test to explore the relationship between the local covers and the plant population ecological estimators (Supplementary Table S3).
Insect samples and interaction records were gathered through observations during an exhaustive 3-h random walk within each 100 × 100 m analysis unit for the eight selected sites. Each flowering plant or patch was observed for 5 min at a time. Flower visitor visits were recorded for individuals that touched or searched for the reproductive parts of any flowering plant in each analysis unit. In the case of highly abundant and recognizable insect species (i.e., Apis mellifera Linnaeus, 1758, Bombus wilmattae Cockerell, 1912, Bombus ephippiatus Say, 1837) and plant species (i.e., Bidens pilosa L., Bidens bicolor Greenm., Lopezia racemosa Cav., Raphanus raphanistrum L.) only a representative number of specimens were collected, and their subsequent interactions were recorded. We collected the flower visitors and visited plants that we were not able to identify during the in situ observations. All insects were collected manually, using entomological nets. We stored unidentified flower visitors and visited plants for later taxonomic identification. Samplings were performed during the flower visitor activity peak (10:00–13:00 h), only on sunny days without rain or perceptible wind.
We calculated abundance, Chao1 corrected richness (Chao and Shen, 2003) and Shannon diversity index (Shannon and Weaver, 1949) to describe the diversity of the complete flower visitor community. We also calculated abundance, Chao1 corrected richness and Shannon diversity index for bees and flies as separate taxonomic groups. All the diversity metrics were calculated using the Past statistics software, version 4.09 (Hammer et al., 2001).
Additionally, we analyzed separately the abundance of the most frequent bee floral visitors in the communities: A. mellifera, B. wilmattae, and B. ephippiatus, considered key floral visitors in the region (Brosi et al., 2008), and the abundance of the most frequent hoverfly species Eristalis sp. (Diptera: Syrphidae). From this data we constructed species/morphospecies-based interaction networks. To obtain network metrics from plant-insect interactions, we constructed an individual quantitative interaction matrix for each 100 × 100 m analysis unit. We defined the abundance of each interaction in the network as the frequency of visits of each insect flower visitor species to the same flowering plant species, sampled from each analysis unit. From each matrix we computed five metrics: specialization (H2′), nestedness (NODF), modularity (Q), connectance, and generalization. For nestedness, we used the NODF metric (Almeida-Neto et al., 2008). NODF calculates the persistence of the interaction at network level based on the tendency of specialist species to interact with the subset of generalist species. For modularity, we used the QuanBiMo algorithm (Dormann and Strauss, 2014). QuanBiMo computes the strength of division of the network in modules based on a hierarchical clustering algorithm. Thus, a module is delineated when species are having more interaction within the module than between modules. For specialization, we used the H2′ metric (Blüthgen et al., 2006). The H2′ metric quantify the reciprocal selectivity of interaction between trophic levels. In contrast, the generalization metric evaluates the reciprocal impression of interactions between trophic levels (Tylianakis et al., 2007). Lastly, the connectance metric measures the proportion of possible links realized among the trophic levels (Jordano, 1987).
Due to the dependence of network metrics on network dimensions, we simulated 1,000 random interaction networks, using the Patefeld's algorithm (Patefeld, 1981) for each site and then Δ-transformed all network metrics (Schleuning et al., 2014; Dalsgaard et al., 2017; Simmons et al., 2018; Escobedo-Kenefic et al., 2020). The Δ-transformed network metrics were calculated as Nobs – Nrammean where Nobs is the observed value of a network metric and Nrammean is the mean value for the 1,000 randomized networks and reflects the degree to which a network metric deviates from a random expectation (see Supplementary Table S4). All the analyses were performed using the bipartite package (Dormann et al., 2009) in RStudio. In addition, we used t-tests to evaluate whether relative metrics are consistently smaller or larger than zero (Supplementary Table S5).
To answer whether landscape or local variables affect (1) the diversity of insect flower visitors, (2) the abundance of the most frequent flower visitor species, and (3) the stability of flower visitor interaction networks; we ran generalized linear models with Gaussian distribution which included 18 response variables and 15 explanatory variables by following the three steps described below:
1. Definition and standardization of the variables: We defined 18 response variables and categorized them in three groups: (a) nine insect diversity descriptors (abundance, Chao1 corrected richness, Shannon diversity index of the total insect floral visitors, as well as for bees and flies as separate groups); (b) the abundance of four of the most frequent floral visitors (the honeybee A. mellifera, the bumblebees B. ephippiatus and B. wilmattae and the hoverfly Eristalis sp.); and (c) five plant-flower visitor network descriptors (Δ-transformed connectance, Δ-transformed specialization, Δ-transformed generalization, Δ-transformed nestedness and Δ-transformed modularity). Additionally, we defined 15 explanatory variables that were grouped as follows: (A) four landscape scale land use categories, measured within 1,000 m buffer areas (forest, crops, seminatural vegetation, and human settlements) and the landscape-scale land use heterogeneity index; (B) four local-scale land covers measured within each 100 × 100 m analysis unit (bare soil/dry crops, flowering crops, flowering weeds, and non-flowering crops cover) and the local-scale heterogeneity index, as described before; and (C) five plant population ecological estimators obtained from the 4 m2 plant-community plots (flower density, herbaceous stratum height, Chao1 corrected richness, Shannon diversity index, and abundance of weeds).
To compare between equivalent estimators of landscape at landscape and local scale, we analyzed the explanatory variables from groups A (landscape-scale) and B (local-scale) in the same models together, since the variables were measured as areas (although the scales were different), and thus were comparable. Plant population ecological estimators (group C) were analyzed as a separate set, since they were only measured at the local scale and did not have equivalent estimators at the landscape scale. Further, to make the model parameters comparable we standardized all variables: we scaled and centered to zero each raw variable by subtracting its mean value and subsequently dividing it by its standard deviation, as in Moreira et al. (2015) and Coutinho et al. (2021). The response variables that did not follow a normal distribution (B. ephippiatus abundance and Δ-transformed modularity); Shapiro Wilk test (P < 0.05), were normalized by 1 + Log10 transformations.
2. Selection of explanatory variables for model analyses: To identify correlations among explanatory variables, we ran Pearson correlation analyses between all the variables, using the “Hmisc” package version 4.7-1 (Harrell, 2022). Only four local scale variables were uncorrelated (bare soil/dry crops cover, flowering crops cover, flower density and herbaceous stratum height; P > 0.05) whereas all the landscape scale variables were correlated (P > 0.05). Thus, to avoid the inclusion of correlated explanatory variables that could generate spurious effects, we evaluated which of the explanatory variables clustered in the A, B, and C groups (as described previously in the Section Introduction) were best supported for our response variables, through the multi-model inference based on Akaike's information criterion (AIC) and the second order AIC (AICc) from de “AICmodavg” package version 2.3-1 (Mazerolle, 2020). We used the Δ-AICc values to discard the hypotheses (models) with lower likelihood probability, and the same time leave out the explanatory variables with no effect on the response variables, sensu the parsimony principle. In that sense, we chose the hypotheses with Δ-AICc values < 2. In all the hypotheses in the cluster of variables A, B, or C had Δ-AICc values < 2, we select the hypotheses in the lowest AIC (Δ-AICc = 0).
3. Final model analyses: Since correlated variables remained after the application of the AIC criterion on the hypotheses above, we used a stepwise model selection and the backwards method to identify the final set of explanatory variables for each model, using the MASS package version (Venables and Ripley, 2002) version 4.3-58.1, in R. If the final models included correlated explanatory variables, we analyzed each correlated variable in a different model. We used the Δ-AICc parameter to compare the performance among the candidate final models. We report the final models with Δ-AICc < 2 values (Supplementary Table S6). Finally, we tested all model residuals for normality using a Shapiro-Wilk test, using the Past statistics software, version 4.09 (Hammer et al., 2001). The other model assumptions (linearity and homogeneity of variances) were verified visually. All models were run in R 2.3-1 (R Core Team, 2022).
We sampled a total of 1,344 insect specimens and their interactions (complete network graphic in Supplementary Figure S3) and identified 21 species and 38 morphospecies from 33 genera. Altogether, 1,146 specimens were bees (85%), 102 were flies (mostly hoverflies) (8%) and 96 specimens were butterflies, wasps, and beetles (7%) (see Supplementary Figure S4 for details). No other species or morphospecies exceeded 5% of the total specimens sampled. A complete list of species or morphospecies and their abundances is detailed in Supplementary Table S7. The most frequent interactions were: 279 visits registered in Bidens bicolor Greenm. (Asteraceae) (21%), 263 in Raphanus raphanistrum L. (Brassicaceae) (20%), 139 in Lopezia racemosa Cav. (Onagraceae) (10%), 126 in Salvia polystachia Cav. (Lamiaceae) (9%), and 95 in Viguiera mima S. F. Blake (Asteraceae) (7%). Other visited plant species did not exceed 4% of the total observed visits. A complete list of flower visitor-plant interactions and their frequency is described in Supplementary Table S8.
At the landscape scale (1,000 m radii), we did not find any effects of land use categories (forest, seminatural vegetation, crops, and human settlements) on corrected richness and Shannon diversity of the total flower visitors (P > 0.05). Similarly, we did not find any influence of land use categories on the abundance and corrected richness of bee flower visitors, Shannon diversity of flies, or on the abundance of the most frequent flower visitor species, A. mellifera and B. wilmattae (P > 0.05). Human settlements showed a significant positive relationship with the total insect abundance (t = 4.11, P = 0.015) and with the Shannon diversity of bees (t = 2.86, P = 0.029), but had a significant negative relationship with fly abundance (t = −3.045, P = 0.023). Seminatural vegetation showed a significant positive relationship with the total insect abundance (t = 3.18, P = 0.034) and with Eristalis sp. abundance (t = 3.048, P = 0.028). Finally, forest exhibited a significant positive relationship with the corrected fly richness (t = 2.59, P = 0.049) (Table 1).
Table 1. Top general linear models explaining the effects of land use categories at the landscape and local scales (landscape: within 1,000 m radius buffers, local: 100 × 100 m analysis units) on the flower visitor diversity variables (total floral visitor abundance, Shannon diversity index of bees, the abundance and Chao1 corrected richness of flies and the abundance of A. mellifera, B. ephippiatus, B. wilmattae, and Eristalis sp.) and on network topological attributes (connectance and specialization).
At local scale, we did not find significant relationships between local cover variables (flowering crops, non-flowering crops, seminatural vegetation, and bare soil/dry crop) and the total flower visitor diversity (Shannon diversity index and Chao1 corrected richness) (P > 0.05). In addition, we did not find significant relationships between local cover variables (described above) and bee diversity (abundance, Shannon diversity index, and Chao1 corrected richness) (P > 0.05) or with the abundance and Shannon diversity of flies (P > 0.05). However, flowering weeds cover had a significant positive relationship with total flower visitor abundance (t = 4.19, P = 0.014) and B. wilmattae abundance (t = 3.73, P = 0.0097). Bare soil/dry crops showed a significant negative relationship with A. mellifera abundance (t = −2.49, P = 0.047) and a positive significant relationship with Eristalis sp. abundance (t = 2.81, P = 0.038). Flowering crops cover exhibited a significant negative relationship with B. ephippiatus abundance (t = −4.18, P = 0.006). Non-flowering crops had a marginally negative relationship with corrected richness of flies (t = −2.16, P = 0.084) (Table 1; Figure 2).
Figure 2. Insect flower visitor metrics that were affected by land cover variables (local scale), vs. the effects of their equivalent landscape scale variables. Circles represent landscape scale variables; squares represent local scale variables. Equivalent variables are shaded in the same color: “flowering weeds” was defined as the local scale equivalent to “seminatural vegetation” at the landscape scale, and all the agricultural-use local scale variables (flowering crops, non-flowering crops, and bare soil /dry crops) were defined as equivalent to the landscape scale variable “crops”. Positive significant effects are represented by upward-pointing arrows, negative significant effects are represented by downward-pointing arrows (*P < 0.05). The effects of “forest” and “human settlements” are not included in the figure since the analysis units were placed in agricultural lands and did not include local scale equivalents to these variables. **P < 0.01.
Regarding plant population ecological estimators, we did not find any influence of floral density, herbaceous stratum height, weed Shannon diversity, weed abundance or weed Chao1corrected richness on total insect visitor diversity and bee diversity measurements (abundance, Chao1 corrected richness, and Shannon diversity) (P > 0.05). We did not find significant relationships among plant population ecological estimators (previously described) and Shannon diversity of fly flower visitors (P > 0.05).
However, herbaceous stratum height had a positive relationship with B. wilmattae abundance (t = 4.33, P = 0.0049) and a marginal negative relationship with B. ephippiatus abundance (t = −2.58, P = 0.061), respectively. Flower density showed a significant negative relationship with fly abundance (t = −4.84, P = 0.084) and a marginal negative relationship with Eristalis sp. abundance (t = −2.19, P = 0.080). Moreover, weed abundance displayed a marginal positive relationship with A. mellifera abundance (t = 0.30, P = 0.083) while weed corrected richness displayed a significant positive relationship with fly abundance (t = 3.68, P = 0.021) (Table 2; Figure 3).
Table 2. Top general linear models explaining the effects of plant population ecological descriptors on the flower visitor diversity variables (the abundance of flies and the abundance of A. mellifera, B. ephippiatus, B. wilmattae, and Eristalis sp.) and on network topological attributes (connectance and modularity).
Figure 3. Effects of plant ecological estimators on insect flower-visitor metrics. Capitals: Explanatory variables, lowercase: Response variables. Positive significant effects are represented by upward-pointing arrows, negative significant effects are represented by downward-pointing arrows (**P < 0.01, *P < 0.05, .P < 0.1).
The honeybee A. mellifera and the bumblebee B. willmattae were the most abundant species within the interaction network system (Supplementary Table S7). All network metrics were higher than zero (ΔNODF: 33.85 ± 8.04 SE, t-test P < 0.001; connectance: 0.16 ± 0.020 SE, t-test P < 0.001; H2′: 0.091 ± 0.029 SE, t-test P < 0.001; modularity: 3.36 ± 1.29 SE, t-test P < 0.001; generalization: 4.23 ± 0.56 SE, t-test P < 0.001; Supplementary Table S7).
At the landscape scale (1,000 m radii), we did not find any effects of land use area (forest, seminatural vegetation, crops, and human settlements) on the Δ-transformed network descriptors: connectance, generalization, nestedness, specialization and modularity (P > 0.05). At the local scale, we did not find any effects of non-flowering crops or seminatural vegetation local covers on any of our network topological descriptors (P > 0.05). Flowering crops had a significant negative relationship with Δ-transformed connectance (t = −2.51, P = 0.046), and bare soil/dry crops cover had a significant positive relationship with Δ-transformed specialization H2′ (t = 2.54, P = 0.044) (Table 1).
Concerning plant population ecological estimators, we did not find any significant relationships of the herbaceous stratum height, corrected richness, or Shannon diversity of weeds with our network topological descriptors (P > 0.05). Flower density had a significant negative relationship with Δ-transformed connectance (t = −2.56, P = 0.042). Weed abundance had a marginal positive relationship with Δ-transformed modularity (t = 2.13, P = 0.086) (Table 2; Figure 3).
Eight insect-flower visitor response variables (total insect abundance, fly richness, Eristalis sp. abundance, B. wilmattae abundance, B. ephippiatus abundance, A. mellifera abundance, network specialization, and network connectance) were influenced by local-scale explanatory variables. Only two of these insect flower visitor metrics (total insect abundance and B. wilmattae abundance) were affected by landscape-scale variables that were the equivalents to the local-scale variables (Figure 2).
We did not find any effects of local cover (bare soil/dry crops, non-flowering crops, and flowering weeds) on the plant population ecological estimators of weeds (flower density, herbaceous stratum height, Chao1 corrected richness, Shannon diversity index, and abundance). Nonetheless, flowering crops cover had a positive significant relationship with Shannon diversity (P = 0.012) and Chao1 corrected richness of weeds (P = 0.055; Supplementary Table S9).
Regarding our first goal, to investigate how landscape and local variables affect the diversity of insect flower visitors, we observed that human settlements and seminatural vegetation areas, at the landscape scale, and flowering weed areas, at the local scale, influenced positively the total abundance of insect flower visitors. Conversely, human settlement areas had a negative influence on fly abundance. At the local scale, fly abundance was negatively influenced by flower density, but showed a positive relationship with weed richness. Fly richness was positively related to forest at the landscape scale, but negatively influenced by non-flowering crops cover at the local scale. More specifically, regarding our second goal, to assess the effects of land use on the abundance of the most frequent flower visitor species, we observed that bare soil/dry crops cover had a positive influence on the abundance of Eristalis sp., but negatively affected the abundance of A. mellifera. As a complement, A. mellifera abundance was positively influenced by weed abundance. Flowering crops and flowering weeds influenced positively the abundance of B. ephippiatus, and B. wilmattae, respectively. At the landscape scale, only seminatural vegetation had a significant relationship with the abundance of Eristalis sp. Regarding our third goal, to evaluate the influence of land use on network metrics, we observed that only local variables presented a significant influence on the stability of insect flower visitor interaction networks. We discuss the detail of our findings in the next paragraphs.
Insect abundance was positively affected by seminatural areas at both scales (“Seminatural areas” at the landscape scale and “Flowering weeds” at the local scale). This result points toward a general beneficial effect of seminatural vegetation on insect flower visitor abundance, as it has been observed in the tropics (e.g., Tangtorwongsakul et al., 2018). At the landscape scale, we observed that bee diversity was positively affected by settlement areas, although fly abundance was affected negatively. Similarly, human settlements have been found to be beneficial for bee diversity, their visitation rate and pollination service, while negatively affecting Diptera and Lepidoptera (Theodorou et al., 2020a,b). This has been attributed to the increase of heterogeneity, floral resources and nesting places, resulting from the presence of home gardens and traditional agricultural practices normally found in both human settlements and seminatural areas (Landaverde-González et al., 2017; Du Clos et al., 2020; Sexton et al., 2021). These arrangements may provide suitable habitats and resources for flower visiting insects, and thus explain the positive effect of settlement areas on bee abundance. Many other works have shown that human settlement areas tend to negatively affect the abundance and presence of flies (Jauker et al., 2009; Potts et al., 2010; Theodorou et al., 2020b), that hoverflies are frequent floral visitors for many crops, and that floral density favors their richness and abundance (Rader et al., 2011, 2013; Moquet et al., 2018; Raguso, 2020). In addition, forest areas had a positive influence on fly richness, and seminatural areas (both variables at the landscape scale) had a positive influence on Eristalis sp. abundance. The abundance of Eristalis sp. was also positively influenced at the local scale by the bare soil/dry crops area (areas with abandoned dry crops or without any vegetation cover). Syrphid hoverflies have heterogeneous nutritional and ecological requirements during their development that may determine their relationship to seminatural areas, although very little is known about their ecology in tropical agroecosystems (Inouye et al., 2015; Doyle et al., 2020) and their role in pollination (Mayer et al., 2011); which makes our study one of the first to provide information on how the ecological dynamics of hoverflies are affected by land use at different scales. It has been reported that hoverflies are declining less rapidly than bees, thus, although relatively less frequent, flower visiting flies may be aiding the robustness of pollination services in changing landscapes and environments, possibly by niche complementarity, especially where bees may be becoming less abundant (Lucas et al., 2018; Doyle et al., 2020). In accordance with these studies, our results suggest that fly flower visitors may be carrying out an important role as pollinators in semi natural areas.
Regarding the most frequent insect flower visitors, we observed that B. ephippiatus abundance was positively affected by flowering crop areas, and B. wilmattae abundance was positively affected by flowering weed abundance, both at the local scale. Bumblebees have been positively associated with seminatural vegetation and forested areas in previous studies from the Guatemalan highlands (Landaverde-González et al., 2018; Escobedo-Kenefic et al., 2020). At the local scale, bare soil and/or dry crops areas showed a negative influence on A. mellifera abundance. Small areas with high diversity and abundance of flowering plants have been known to maintain bee richness and abundance in agricultural landscapes of the tropics (Landaverde-González et al., 2017; Tangtorwongsakul et al., 2018). The lack of floral resources offered by the bare soil/dry crops cover may explain its negative effect on honeybee abundance. On the contrary, the abundance of Eristalis sp. was observed to be influenced positively by bare soil and/or dry crops areas. As mentioned above, the ecological requirements of hoverflies are highly heterogeneous and vary from one species to another. A more detailed taxonomic identification of the species in the area could provide information on the possible reasons for these results. More insect samplings and further taxonomic efforts are needed to address this supposition. Also, it is necessary to point out that most of our analysis units had very small representation of the bare soil/dry crops local variable, but it was largely represented in at least one unit. This strong difference could be influencing our results, since we had only eight analysis units.
Interestingly, the abundance of B. ephippiatus was negatively influenced by the height of the herbaceous stratum, while B. wilmattae was positively influenced. Bombus ephippiatus and B. wilmattae are two phylogenetically related bumblebee species that coexist sympatrically in the study area (Duennes et al., 2012). Although B. wilmattae has a smaller geographic distribution, in our study area it is the most abundant bumblebee species (Duennes et al., 2012; Escobedo-Kenefic et al., 2014, 2020). Taller vegetation height indicates less frequent mowing and longer regeneration time, which has been associated with bumblebees (Xie et al., 2008), even though other studies have found this variable to be more important to moths and butterflies (Pöyry et al., 2006; Milberg et al., 2016). In this sense, B. wilmattae may be dominating the areas with larger and older flowering resources, while B. ephippiatus could be making use of areas with smaller floral resource availability. Niche partitioning has been theorized as a survival trade-off that allows the coexistence of closely related species (e.g., Kaplan and Denno, 2007; DeMarche et al., 2013). However, a detailed analysis on the ecological dynamics of these species is beyond of the scope of this study and further studies on this subject are necessary.
Regarding other frequent visitors, weed abundance had marginally positive influence on A. mellifera abundance, while weed richness was positively related to fly abundance. A greater preference by different flower visitors for weed plants has already been observed (Balfour and Ratnieks, 2022). Interestingly, flower density negatively influenced fly and Eristalis sp. abundance. This could be explained by competitive behavior, since competition for floral resources between bees and hoverflies has been recently addressed in other studies (e.g., Jeavons et al., 2022). Although, specific experiments are needed to test this hypothesis in our study area.
In our study we did not find any significant effects of landscape variables on flower visitor interaction networks. At the local scale, we observed that network specialization was positively affected by bare soil/dry crop cover, while flowering crops had a negative influence on network connectance. Traveset et al. (2020) found highly specialized plant-pollinator networks in areas with radical habitat loss (vegetation removal leading to bare soil). This network pattern was associated to low species abundance, which results in the disruption of the interactions after the disturbance. The negative impacts of bare soil/dry crop and agricultural areas on the abundance of the most frequent floral visitor A. mellifera could be driving the loss of interactions in our flower visitor systems. A specialized network structure may imply (1) high interaction complementarity among trophic levels, (2) strong differences in species niches, and (3) that more species are needed to accomplish the pollination functions of the ecosystem (Blüthgen, 2010; Blüthgen and Klein, 2011).
Less connected networks showed a relationship with larger areas of flowering crops and with flower density. This outcome may be due to the fact that weeds and flowering crops represent the only floral resource available to floral visitors in agricultural areas, and also because the most frequent and dominant blooming weeds attract the most generalist floral visitors (Vaca-Uribe et al., 2021). Our networks were also less connected when flower density and flowering-crops cover increased, but interestingly the flowering crops cover had a positive relationship with the diversity and richness of weeds. Also, weed abundance had a positive relationship with the abundance of a dominant floral visitor of our networks, the honeybee A. mellifera. This suggests that flowering crops cover constitutes a flower-rich habitat to floral visitors, and that most floral resources attract the most generalist floral visitors in our studied agricultural highlands system. Therefore, less connected network patterns may be due to the presence of a flower-rich habitat and the dominance of a single generalist floral visitor, A. mellifera, in our plant-floral visitor systems.
Also, we observed a tendency of flowering weed abundance increasing modularity, although this was just marginally significant. Similarly, a tendency to generate modules due to trait-matching between interaction partners has been observed in Neotropical plant-pollinator networks (Olesen et al., 2007; Vizentin-Bugoni et al., 2018), as well as a change in abundance in the most generalist floral visitors in the network (Watts et al., 2016). In addition, interactions between non-crop plants and bees have been observed to be modular and robust in tropical agroecosystems in Brazil, which suggested spatial habitat partitioning among bee species (Assunção et al., 2022). The coexistence of low connected and highly modular networks with high nested structures has been described for other mutualistic networks (Fortuna et al., 2010; Landaverde-González et al., 2021). However, high weed abundance favored the presence of A. mellifera in our sites. The presence of A. mellifera could propitiate the disassembling of interactions between the trophic levels in our plant-flower visitor systems. This may result in the loss of interactions among modules and consequently the increase of modularity (Valido et al., 2019). The existence of modules of interacting partners give rise to a non-random interaction link structure among the trophic levels (Olesen et al., 2007; Chacoff et al., 2012). Also, high modularity and high specialization may be allowing the isolation of perturbations and thus maintaining its stability (Thébault and Fontaine, 2010).
As a caveat to our results, it must be considered that they were obtained from eight analysis units (seven units for some plant ecological estimators). It also must be considered that our analysis units were located in agricultural lands only, which did not allow us to directly compare the effects of two important landscape-scale variables (human settlements and forest) with equivalent effects at a local scale. Because of this, we could be missing important information on species that are associated to these landscape-scale variables. For instance, native social bee species like stingless bees are known to be dependent on forest in the Guatemalan highlands as well (Escobedo-Kenefic et al., 2020). Although at least six stingless bee species have been reported close to our study area (Escobedo-Kenefic et al., 2014), the only one observed in three of our analysis units was Partamona bilineata (Say, 1837). Species-specific functional traits, like nesting place and body size may determine stingless bee abundance in disturbed areas (Lichtenberg et al., 2017). Since P. bilineata bees can also build their nests in various substrates, including man-made structures and materials (e.g., Camargo and Pedro, 2003), they are more adaptable to disturbed areas, like our sampling units. On the other hand, tree-nesting bee species may be prone to remain in forest patches that provide them with nesting places (Brosi, 2009) and to avoid disturbed areas (Lichtenberg et al., 2017). Even though our analysis units were located only in agricultural areas, the forest remnants at our study sites are ravines that are impossible to cultivate, and thus, constitute small but relatively well-conserved primary forest remnants that provide nest and food resources, and may be serving as refuge to forest-dependent species. Overall, these may be the reasons why P. bilineata was observed during our samplings while other stingless bees were absent, but additional effort including local forest patches and temporal analysis will be necessary to test this supposition.
However, the fact that we were able to detect significant effects points toward an important influence of local variables on insect visitor diversity. Also, to our knowledge, this is the first study to detect significant influence of local variables, like land covers and plant ecological estimators, on insect visitor diversity and their interactions in a tropical highland agroecosystem.
Our study highlights the relevance of local context in the maintenance of flower visitor communities and their interactions. Both landscape and local scales revealed contradictory effects on different taxa. For instance, human settlement areas seem to be positive for bee diversity but negative for flower visiting fly abundance. This pattern has been previously observed and attributed to the availability of floral resources and nesting sites for bees in human settlements, while hoverflies may have complex development requirements that seem to be present in seminatural areas, but not in settlement areas. Further, local scale variables better explained the abundance of flower visiting insects, including the most frequent social bee species (honeybees and bumblebees). Seminatural vegetation, represented in our local scale by flowering weed areas, showed a positive influence on insect abundance, highlighting the role of flower resource availability on maintaining flower visiting insect populations in agroecosystems. Also, the abundance of the three most frequent bee species was influenced by local scale variables in different ways: B. ephippiatus and B. wilmattae were influenced positively by flowering crops and flowering weeds, respectively, while A. mellifera was negatively influenced by bare soil/dry crops area, which suggests possible species-specific effects of the different local covers.
Interaction network metrics were only affected by local scale variables, suggesting that land cover at a local scale is an important driver of ecological interaction networks in tropical highland agroecosystems. Network specialization was positively affected by the presence of bare soil/dry crops cover, probably due to the lower abundance of flower visitors and therefore less interactions. Modularity was found to be positively associated with flowering weed abundance, which was already observed in Neotropical agroecosystems. This effect suggests that modularity may benefit network stability by isolating perturbations when specialization increases. These findings suggest susceptibility of the interaction network to small-scale changes and highlight the importance of promoting agricultural practices that allow land cover heterogeneity. Finally, our results point out the complementary roles that the most abundant insect flower visitors carry out in the study area, and the importance of studying the biology and ecological dynamics of lesser-known pollinators.
The original contributions presented in the study are included in the article/Supplementary material, further inquiries can be directed to the corresponding authors.
Written informed consent was obtained from the individuals that appear in any identifiable images or data included in this article.
NE-K, QC-A, EE, and PL-G wrote first draft. NE-K, QC-A, and EC designed the study. QC-A, NE-K, EC, AM-C, and DE-G collected and recorded flower visitor interactions. QC-A and DE-G identified insects. EC, QC-A, and AM-C identified plant specimens. QC-A, NE-K, and PL-G contributed to data analysis. NE-K and QC-A made the figures. All authors reviewed, edited, and approved the final version of the manuscript.
This research was co-financed by the General Direction of Research of the University of San Carlos de Guatemala (DIGI) through the Grant B32-2020 and by the Early Career Fellowship Program, Organization for Women in Science for the Developing World (OWSD) through the Grant 45004272284 (2021–2022).
The authors thank the Conservationist Studies Center (CECON) of the University of San Carlos de Guatemala and especially the Unidad de Biodiversidad team for providing continued support in the form of human resources, equipment, and logistics. We thank Javier Morales and Navil Ventura for field work support. We also thank Beatriz Jo, who enthusiastically collaborated with this project and to whose memory we dedicate this work.
The authors declare that the research was conducted in the absence of any commercial or financial relationships that could be construed as a potential conflict of interest.
All claims expressed in this article are solely those of the authors and do not necessarily represent those of their affiliated organizations, or those of the publisher, the editors and the reviewers. Any product that may be evaluated in this article, or claim that may be made by its manufacturer, is not guaranteed or endorsed by the publisher.
The Supplementary Material for this article can be found online at: https://www.frontiersin.org/articles/10.3389/fsufs.2022.974215/full#supplementary-material
Aguilera, G., Roslin, B., Miller, K., Tamburini, G., Birkhofer, K., Caballero-Lopez, B., et al. (2020). Crop diversity benefits carabid and pollinator communities in landscapes with semi-natural habitats. J. Appl. Ecol. 57, 2170–2179. doi: 10.1111/1365-2664.13712
Almeida-Neto, M., Guimarães, P. R. J., Loyola, R. D., and Ulrich, W. (2008). A consistent metric for nestedness analysis in ecological systems: reconciling concept and measurement. Oikos 117, 1227–1239. doi: 10.1111/j.0030-1299.2008.16644.x
Altieri, M. (2004). Linking ecology and traditional farmers in the search for sustainable agriculture. Front. Ecol. Environ. 2, 35–42. doi: 10.1890/1540-9295(2004)002[0035:LEATFI]2.0.CO;2
Armas-Quiñonez, G., Ayala-Barajas, R., Avendaño-Mendoza, C., Lindig-Cisneros, R., and Del-Val, E. (2020). Bee diversity in secondary forests and coffee plantations in a transition between foothills and highlands in the Guatemalan Pacific Coast. PeerJ 8, e9257. doi: 10.7717/peerj.9257
Asner, G., Elmore, A., Olander, L., Martin, R., and Harris, T. (2004). Grazing system, ecosystem responses, and global change. Annu. Rev. Environ. Resour. 29, 261–299. doi: 10.1146/annurev.energy.29.062403.102142
Assunção, R. M., Camargo, N. F., Souza, L. S., Rocha, E. M., Tostes, G. M., Sujii, E. R., et al. (2022). Landscape conservation and local interactions with non-crop plants aid in structuring bee assemblages in organic tropical agroecosystems. J Insect Conserv. (Unpublished manuscript) doi: 10.1007/s10841-022-00438-8
Balfour, N. J., and Ratnieks, F. L. (2022). The disproportionate value of ‘weeds’ to pollinators and biodiversity. J. Appl. Ecol. 59, 1209–1218. doi: 10.1111/1365-2664.14132
Bascompte, J., and Jordano, P. (2007). Plant-animal mutualistic networks: the architecture of biodiversity. Annu. Rev. Ecol. Evol. Syst. 38, 567–593. doi: 10.1146/annurev.ecolsys.38.091206.095818
Bates, A. J., Sadler, J. P., Fairbrass, A. J., Falk, S. J., Hale, J. D., and Matthwes, T. J. (2011). Changing bee and hoverfly pollinator assemblages along an urban-rural gradient. PLoS ONE. 6, e23459. doi: 10.1371/journal.pone.0023459
Benjamin, E. F. R, Reilly, J., and Winfree, R. (2013). Pollinator body size mediates the scale at which land use drives crop pollination services. J. Appl. Ecol. 51, 440–449. doi: 10.1111/1365-2664.12198
Bergholz, K., Sittel, L. P., Ristow, M., Jeltsch, F., and Weiss, L. (2021). Pollinator guilds respond contrastingly at different scales to landscape parameters of land-use intensity. Ecol. Evol. 12, e8708. doi: 10.22541/au.163428515.53420959/v1
Birdshire, K. R., Carper, A. L., and Briles, C. (2020). Bee community response to local and landscape factors along an urban-rural gradient. Urban Ecosyst. 23, 689–702. doi: 10.1007/s11252-020-00956-w
Blüthgen, N. (2010). Why network analysis is often disconnected from community ecology: a critique and an ecologist's guide. Basic Appl. Ecol. 11, 185–195. doi: 10.1016/j.baae.2010.01.001
Blüthgen, N., and Klein, A. M. (2011). Functional complementarity and specialisation: the role of biodiversity in plant—Pollinator interactions. Basic Appl. Ecol. 12, 282–291. doi: 10.1016/j.baae.2010.11.001
Blüthgen, N., Menzel, F., and Blüthgen, N. (2006). Measuring specialization in species interaction networks. BMC Ecol. 6, 1–12. doi: 10.1186/1472-6785-6-9
Brosi, B. J. (2009). The complex responses of social stingless bees (Apidae: Meliponini) to tropical deforestation. For. Ecol. Mang. 258, 1830–1837. doi: 10.1016/j.foreco.2009.02.025
Brosi, B. J., Daily, G. C., Shih, T. M., Oviedo, F., and Durán, G. (2008). The effects of forest fragmentation on bee communities in tropical countryside. J. Appl. Ecol. 45, 773–783. doi: 10.1111/j.1365-2664.2007.01412.x
Burrascano, S., de Andrade, R. B., Paillet, Y, Ódor, P., Antonini, G., Bouget, C., et al. (2018). Congruence across taxa and spatial scales: are we asking too much of species data? Global. Ecol. Biogeogr. 27, 980–990. doi: 10.1111/geb.12766
Camargo, J. M. F., and Pedro, S. R. M. (2003). Neotropical Meliponini: the genus Partamona Schwarz, 1939 (Hymenoptera, Apidae, Apinae)—bionomy and biogeography. Rev. Bras. Entomol. 47, 311–372. doi: 10.1590/S0085-56262003000300001
Carey, D. (2009). Guatemala's green revolution: synthetic fertilizer, public health, and economic autonomy in the Mayan Highland. Agric. Hist. 83, 283–322. doi: 10.1215/00021482-83.3.283
Cely-Santos, M., and Philpott, S. (2019). Local and landscape habitat influences on bee diversity in agricultural landscapes in Anolaima, Colombia. J. Insect Conserv. 23, 133–146. doi: 10.1007/s10841-018-00122-w
Chacoff, N. P., Vázquez, D. P., Lomáscolo, S. B., Stevani, E. L., Dorado, J., and Padrón, B. (2012). Evaluating sampling completeness in a desert plant–pollinator network. J. Anim. Ecol. 81, 190–200. doi: 10.1111/j.1365-2656.2011.01883.x
Chao, A., and Shen, T. S. (2003). Non parametric estimation of Shanonn's index of diversity when there are unseen species in a sample. Environ. Ecol. Stat. 10, 429–443. doi: 10.1023/A:1026096204727
Coutinho, J. G. E., Hipólito, J., Santos, R. L. S., Moreira, E. F., Boscolo, D., and Viana, B. F. (2021). Landscape structure is a major driver of bee functional diversity in crops. Front. Ecol. Evol. 9, 624835. doi: 10.3389/fevo.2021.624835
Cusser, S., Grando, C., Zucchi, M. I., López-Uribe, M. M., Pope, N. S., Ballare, K., et al. (2019). Small but critical: semi-natural habitat fragments promote bee abundance in cotton agroecosystems across both Brazil and the United States. Landsc. Ecol. 34, 1825–1836. doi: 10.1007/s10980-019-00868-x
Dalsgaard, B., Schleuning, M., Maruyama, P. K., Dehling, D. M., Sonne, J., Vizentin-Bugoni, J., et al. (2017). Opposed latitudinal patterns of network derived and dietary specialization in avian plant-frugivore interaction systems. Ecography 40, 1395–1401. doi: 10.1111/ecog.02604
DeMarche, M., Rice, K., and Sexton, J. (2013). Niche partitioning between close relatives suggests trade-offs between adaptation to local environments and competition. Ecol. Evol. 3, 512–522. doi: 10.1002/ece3.462
Dicks, L. V., Breeze, T. D, Ngo, T. H., Senapathi, D., An, J., et al. (2021). A global scale expert assesment of drivers and risk associated with pollinator decline. Nat. Ecol. Evol. 5, 1453–1461. doi: 10.1038/s41559-021-01534-9
Dormann, C. F., Frund, J., Blüthgen, N., and Gruber, B. (2009). Indices, graphs and null models: analyzing bipartite ecological networks. Open Ecol. J. 2, 7–24. doi: 10.2174/1874213000902010007
Dormann, C. F., and Strauss, R. (2014). A method for detecting modules in quantitative bipartite networks. Methods Ecol. Evol. 5, 90–98. doi: 10.1111/2041-210X.12139
Doyle, T., Hawkes, W., Massy, R., Powney, G. D., Menz, M., and Wotton, K. R. (2020). Pollination by hoverflies in the Anthropocene. Proc. R. Soc. B. 287, 20200508. doi: 10.1098/rspb.2020.0508
Du Clos, B., Drummond, F. A., and Loftin, C. S. (2020). Noncrop habitat use by wild bees (Hymenoptera: Apoidea) in a mixed-use agricultural landscape. Environ. Entomol. 49, 502–515. doi: 10.1093/ee/nvaa001
Duennes, M. A., Lozier, J. D., Hines, H. D., and Cameron, S. A. (2012). Geographical patterns of genetic divergence in the widespread Mesoamerican bumble bee Bombus ephippiatus (Hymenoptera: Apidae). Mol. Phylogenet. Evol. 64, 219–231. doi: 10.1016/j.ympev.2012.03.018
Escobedo-Kenefic, N., Dardón, M., López, J., Martínez, O., and Cardona, E. (2014). Efecto de la configuración del paisaje en las comunidades de abejas (Apoidea) de un mosaico de pino-encino y áreas agrícolas de Sacatepéquez y Chimaltenango, Guatemala. Ciencia Tecnología y Salud. 1, 13–20. doi: 10.36829/63CTS.v1i1.1
Escobedo-Kenefic, N., Landaverde-González, P., Theodorou, P., Cardona, E., Dardón, M. J., Martínez, O., et al. (2020). Disentangling the effects of local resources, landscape heterogeneity and climatic seasonality on bee diversity and plant-pollinator networks in tropical highlands. Oecologia 194, 333–344. doi: 10.1007/s00442-020-04715-8
ESRI (2014). ArcGis for Desktop. Version 10.2.1. Redlands, CA: Environmental Systems Research Institute.
Foley, J., DeFries, R., Asner, G., Barford, C., Bonan, G., Carpenter, S., et al. (2005). Global consequences of land use. Science. 309, 570–574. doi: 10.1126/science.1111772
Fortuna, M. A., Stouffer, D. B., Olesen, J. M., Jordano, P., Moullot, D., Krasnov, K., et al. (2010). Nestedness versus modularity in ecological networks: two sides of the same coin? J. Appl. Ecol. 79, 811–817. doi: 10.1111/j.1365-2656.2010.01688.x
Gálvez, J., Buch, M., Pineda, P., Carrera Cruz, J., Ortiz, A., Carrera, L. J., et al. (2014). Perfil del agro y la ruralidad de Guatemala 2014: Situación actual y tendencias. eds C. Cleaves (Guatemala: Universidad Rafael Landívar/Instituto de Agricultura, Recursos Naturales y Ambiente IARNA. Instituto Interamericano de Cooperación para la Agricultura IICA, Del Altiplano de Guatemala).
Garibaldi, L. A., Steffan-Dewenter, I., Winfree, R., Aizen, M. A., Bommarco, R., Cunningham, S. A., et al. (2013). Wild pollinators enhance fruit set of crops regardless of honey bee abundance. Science 339, 1608–1611. doi: 10.1126/science.1230200
Google Earth/Maxar Technologies (2020). Chimaltenango, Guatemala. 14.66579893 N,−90.93334258 W, elevation 2100 m. [Satellite image]. Available online at: https://earth.google.com/web/@14.66579893,-90.93334258,2115.90214558a,19448.64180487d,35y,0.1707h,0t,0r?utm_source=earth7andutm_campaign=vineandhl=es-419 (accessed November 1, 2020).
Grundel, R., Jean, R. P., Frohnapple, K. J., Glowacki, G. A., Scott, P. E., and Pavlovic, N. B. (2010). Floral and nesting resources, habitat structure, and fire influence bee distribution across an open-forest gradient. Ecol. Appl. 20, 1678–1692. doi: 10.1890/08-1792.1
Guardiola, J., and Bernal, J. (2009). Factores influyentes en la adopción de cultivos no tradicionales: El caso de Guatemala. Agroalimentaria. 15, 27–37.
Hammer, Ø., Harper, D. A. T., and Ryan, P. D. (2001). PAST paleontological statistics software package for education and data analysis. Paleontol. Electron. 4, 1–9. Available online at: https://palaeo-electronica.org/2001_1/past/past.pdf (accessed September 12, 2022).
Harrell, F. E. Jr. (2022). Hmisc: Harrell Miscellaneous (Version 4.7-1). Cran. Available online at: https://cran.r-project.org/web/packages/Hmisc/index.html (accessed October 18, 2022).
Hellin, J., Ratner, B. D., Meizen-Dick, R., and López-Ridaura, S. (2018). Increasing social-ecological resilience within small-scale agriculture in conflict-affected Guatemala. Ecol. Soc. 23, 5. doi: 10.5751/ES-10250-230305
Hernández-Villa, V., Vibrans, H., Uscanga.Mortera, E., and Aguirre-Jaimes, A. (2020). Floral visitors and pollinator dependence are related to floral display size and plant height in native weeds of central Mexico. Flora. 262, 151505. doi: 10.1016/j.flora.2019.151505
Inouye, D. W., Larson, B. M. H., Ssymank, A., and Kevan, P. G. (2015). Flies and flower III: ecology of foraging and pollination. J. Poll. Ecol. 16, 115–133. doi: 10.26786/1920-7603(2015)15
IPBES (2016). “The assessment report of the Intergovernmental Science-Policy Platform on Biodiversity and Ecosystem Services on pollinators, pollination and food production,” in Secretariat of the Intergovernmental Science-Policy Platform on Biodiversity and Ecosystem Services, eds S.G. Potts, V. L. Imperatriz-Fonseca, and H. T. Ngo (Bonn: IPBES), 552.
Jauker, F., Diekötter, T., Schwarzbach, F., and Wolters, F. (2009). Pollinator dispersal in an agricultural matrix: opposing responses of wild bees and hoverflies to landscape structure and distance from main habitat. Landsc. Ecol. 24, 547–555. doi: 10.1007/s10980-009-9331-2
Jeavons, E., Chevrie, O., Le Lann, C., Renault, D., Floch, M., et al. (2022). Exploitative competition for floral resources reduces sugar intake but differently impacts the foraging behaviour of two non-bee flower visitors. Oikos, 2022:e08576. doi: 10.1111/oik.08576
Jha, S., and Vandermeer, J. (2010). Impacts of coffee agroforestry management on tropical bee communities. Biol. Conserv. 146, 1423–1431. doi: 10.1016/j.biocon.2010.03.017
Jordano, P. (1987). Patterns of mutualistic interactions in pollination and seed dispersal: connectance, dependence asymmetries, and coevolution. Am. Nat. 129, 657–677. doi: 10.1086/284665
Kaiser-Bunbury, C. N., and Blüthgen, N. (2015). Integrating network ecology with applied conservation: a synthesis and guide to implementation. AoBP 7, plv076. doi: 10.1093/aobpla/plv076
Kaiser-Bunbury, C. N., Mougal, J., Whittington, A., Valentine, T., Gabriel, R., Olensen, J. M., et al. (2017). Ecosystem restoration strengthens pollination network resilience and function. Nature 542, 223–227. doi: 10.1038/nature21071
Kaplan, I., and Denno, R. F. (2007). Interspecific interactions in phytophagous insects revisited: a quantitative assessment of competition theory. Ecol. Lett. 10, 977–994. doi: 10.1111/j.1461-0248.2007.01093.x
Kleijn, D., Winfree, R., Bartomeus, I., Carvalheiro, L. G., Henry, M., Isaacs, R., et al. (2015). Delivery of crop pollination services is an insufficient argument for wild pollinator conservation. Nat. Commun. 6, 7414. doi: 10.1038/ncomms8414
Klein, M. A., Cunningham, S., Bos, M., and Steffan-Dewenter, I. (2008). Advances in pollination ecology from tropical plantation crops. Ecology 89, 935–943. doi: 10.1890/07-0088.1
Kohler, F., Verhulst, J., Van Klink, R., and Kleijn, D. (2008), At what spatial scale do high-quality habitats enhance the diversity of forbs pollinators in intensively farmed landscapes? J. App. Ecol. 45, 753–762. doi: 10.1111/j.1365-2664.2007.01394.x
Kratschmer, S., Pachinger, B., Schwantzer, M., Paredes, D., Guzmán, G., Gómez, J. A., et al. (2019). Response of wild bee diversity, abundance, and functional traits to vineyard inter-row management intensity and landscape diversity across Europe. Ecol. Evol. 9, 4103–4115. doi: 10.1002/ece3.5039
Landaverde-González, P., Baltz, L., Escobedo-Kenefic, N., Mérida, J., Paxton, R. J., and Husemann, M. (2018). Recent low levels of differentiation in the native Bombus ephippiatus (Hymenoptera: Apidae) along two Neotropical mountain-ranges in Guatemala. Biodivers. Conserv. 27, 3513–3531. doi: 10.1007/s10531-018-1612-0
Landaverde-González, P., Enríquez, E., and Núñez-Farfán, J. (2021). The effect of landscape on Cucurbita pepo-pollinator interaction networks varies depending on plants' genetic diversity. Arthropod. Plant Interact. 15, 917–928 doi: 10.1007/s11829-021-09872-y
Landaverde-González, P., Quesada-Euán, J., Theodorou, P., Murray, T., Husemann, M., Ayala, R., et al. (2017). Sweat bees on hot chilies: provision of pollination services by native bees in traditional slash-and-burn agricultural in Yucatán Peninsula of Tropical Mexico. J. Appl. Ecol. 54, 1814–1824. doi: 10.1111/1365-2664.12860
Laurence, W., Sayer, J., and Cassman, K. (2014). Agricultural expansion and its impact on tropical nature. Trends Eco. Evol. 29, 107–116. doi: 10.1016/j.tree.2013.12.001
Lichtenberg, E. M., Mendenhall, C. D., and Brosi, B. (2017). Foraging traits modulate stingless bee community disassembly under forest loss. J. Anim. Ecol. 86, 1404–1416. doi: 10.1111/1365-2656.12747
Lucas, A., Bodger, O., Brosi, B. J., Ford., C. R, Forman, D. W., Greig, C., et al. (2018). Floral resource partitioning by individuals within generalised hoverfly pollination networks revealed by DNA metabarcoding. Sci. Rep. 8, 5133. doi: 10.1038/s41598-018-23103-0
Mayer, C., Adler, L., Armbruster, W. S., Dafni, A., Eardley, C., Huang, S. Q., et al. (2011). Pollination ecology in the 21st Century: key questions for future research. J. Pollinat. Ecol. 3, 8–23. doi: 10.26786/1920-7603(2011)1
Mazerolle, M. J. (2020). AICcmodavg: Model Selection and Multimodel Inference Based on (Q)AIC(c). R package version 2.3-1. Available online at: https://cran.r-project.org/package=AICcmodavg (accessed October 18, 2022).
Meng, L. Z., Martin, K., Liu, J. X., Burger, F., and Chen, J. (2012). Contrasting responses of hoverflies and wild bees to habitat structure and land use change in a tropical landscape (southern Yunnan, SW China). Insect Sci. 19, 666–676. doi: 10.1111/j.1744-7917.2011.01481.x
Milberg, P., Bergman, K. O., Cronvall, E., Eriksson, Å., Glimskär, A., Islamovic, A., et al. (2016). Flower abundance and vegetation height as predictors for nectar-feeding insect occurrence in Swedish semi-natural grasslands. Agric. Ecosyst. Environ. 230, 47–54. doi: 10.1016/j.agee.2016.05.029
Moquet, L., Laurent, E., Bacchetta, R., and Jacquemart, A. L. (2018), Conservation of hoverflies (Diptera, Syrphidae) requires complementary resources at the landscape local scales. Insect. Conserv. Divers. 11, 72–87. doi: 10.1111/icad.12245
Moreira, E. F., Boscolo, D., and Viana, B. F. (2015). Spatial heterogeneity regulates plant-pollinator networks across multiple landscape scales. PLoS ONE 10, e0123628. doi: 10.1371/journal.pone.0123628
Motzke, I., Klein, M. A., Saleh, S., Wanger, T. C., and Tscharntke, T. (2016). Habitat management on multiple spatial scales can enhance bee pollination and crop yield in tropical homegardens. Agric. Ecosyst. Environ. 223, 144–151. doi: 10.1016/j.agee.2016.03.001
Nigh, R., and Diemont, S. A. (2013). The Maya milpa: fire and the legacy of living soil. Front. Ecol. Environ. 11, e45–e54. doi: 10.1890/120344
Olesen, J. M., Bascompte, J., Dupont, Y. L., and Jordano, P. (2007). The modularity of pollination networks. PNAS 104, 19891–19896. doi: 10.1073/pnas.0706375104
Ollerton, J., Winfree, R., and Tarrant, S. (2011). How many flowering plants are pollinated by animals? Oikos 120, 321–326. doi: 10.1111/j.1600-0706.2010.18644.x
Osterman, J., Landaverde-González, P., Garratt, M. P. D., Gee, M., Mandelik, Y., Langowska, A., et al. (2021). On-farm experiences shape farmer knowledge, perceptions of pollinators, and management practices. Glob. Ecol. Conserv. 32, e01949. doi: 10.1016/j.gecco.2021.e01949
Patefeld, W. M. (1981). Algorithm AS 159: an efficient method of generating random R x C tables with given row and column totals. J. R. Stat. Soc. C-Appl. 30, 91–97. doi: 10.2307/2346669
Perfecto, I., Vandermeer, J., and Philpott, S. M. (2014). Complex ecological interactions in coffee agroforestry systems. Annu. Rev. Ecol. Evol. Syst. 45, 137–158. doi: 10.1146/annurev-ecolsys-120213-091923
Potts, S. G., Biesmeijer, J. C., Kremer, C., Neumann, P., Schwieger, O., and Kunin, W. (2010). Global pollinator declines: trends, impacts and drivers. Trends Eco. Evol. 25, 345–353. doi: 10.1016/j.tree.2010.01.007
Pöyry, J., Luoto, M., Paukkunen, J., Pykälä, J., Raatikainen, K., and Kuussaari, M. (2006). Different responses of plants and herbivore insects to a gradient of vegetation height: an indicator of the vertebrate grazing intensity and successional age. Oikos 115, 401–412. doi: 10.1111/j.2006.0030-1299.15126.x
R Core Team (2022). R: A Language and Environment for Statistical Computing. Vienna: R Foundation for Statistical Computing. Available online at: https://www.R-project.org/ (accessed October 18, 2022).
Rabeling, S. C., Lim, J. L., Tidon, R., Neff, J. L., Simpson, B. B., and Pawar, S. (2019). Seasonal variation of a plant-pollinator network in the Brazilian Cerrado: implications for community structure and robustness. PLoS ONE 14, e0224997. doi: 10.1371/journal.pone.0224997
Rader, R., Bartomeus, I., Garibaldi, L. A., Garratt, M. P. D., Howlett, B. G., Winfree, R., et al. (2016). Non-bee insects are important contributors to global crop pollination. PNAS 113, 146–151. doi: 10.1073/pnas.1517092112
Rader, R. A., Edwards, W., Wescott, D. A., Cunningham, S. A., and Howlett, B. G. (2011). Pollen transport differs among bees and flies in a human-modified landscape. Diver. Distrib. 17, 519–529. doi: 10.1111/j.1472-4642.2011.00757.x
Rader, R. A., Edwards, W., Wescott, D. A., Cunningham, S. A., and Howlett, B. G. (2013). Diurnal effectiveness of pollination by bees and flies in agricultural Brassica rapa: Implications for ecosystem resilience. Basic Appl. Ecol. 14, 20–27. doi: 10.1016/j.baae.2012.10.011
Raguso, R. A. (2020). Don't forget the flies: dipteran diversity and its consequences for floral ecology and evolution. Appl Entomol Zool 55, 1–7. doi: 10.1007/s13355-020-00668-9
Requier, F., and Leonhardt, S. D. (2020). Beyond flowers: including non-floral resources in bee conservation schemes. J. Insect Conserv. 24, 5–16. doi: 10.1007/s10841-019-00206-1
Ropars, L., Affre, L., Schurr, L., Flachner, F., Genoud, D., Mutillod, C., et al. (2020). Land cover composition, local plant community composition and honeybee colony density affect wild bee species assemblages in a Mediterranean biodiversity hot-spot. Acta Oecol. 104, 103546. doi: 10.1016/j.actao.2020.103546
Schleuning, M., Ingmann, L., Strauß, R., Fritz, S. A., Dalsgaard, B., Dehling, D. M., et al. (2014). Ecological, historical and evolutionary determinants of modularity in weighted seed-dispersal networks. Ecol. Lett. 17, 454–463. doi: 10.1111/ele.12245
Sexton, A. N., Benton, S., Browning, A. C., and Emery, S. M. (2021). Reproductive patterns of solitary cavity-nesting bees responsive to both local and landscape factors. Urban Ecosyst. 24, 1271–1280. doi: 10.1007/s11252-021-01116-4
Shannon, C. E., and Weaver, W. (1949). The Mathematical Theory of Communication. Champaign, IL: University of Illinois Press.
Simmons, B. I., Sutherland, W. J., Dicks, L. V., Albrecht, J., Farwig, N., García, D., et al. (2018). Moving from frugivory to seed dispersal: incorporating the functional outcomes of interactions in plant-frugivore networks. J. Anim. Ecol. 87, 995–1007. doi: 10.1111/1365-2656.12831
Souza, C., Maruyama, P. K., Aoki, C., Sigrist, M. R., Raizer, J., Gross, C. L., et al. (2018). Temporal variation in plant-pollinator networks from seasonal tropical environments: higher specialization when resources are scarce. J. Ecol. 106, 2409–2420. doi: 10.1111/1365-2745.12978
Steffan-Dewenter, I., Potts, S. G., and Packer, L. (2005). Pollinator divesity and crop pollination services are at risk. Trends Eco. Evol. 20, 651–652. doi: 10.1016/j.tree.2005.09.004
Stein, K., Coulibaly, D., Balima, L. H., Goetze, D., Linsenmair, K. E., Porembski, S., et al. (2021). Plant-Pollinator Networks in Savannas of Burkina Faso, West Africa. Diversity 13, 1. doi: 10.3390/d13010001
Tangtorwongsakul, P., Warrit, N., and Gale, G. A. (2018). Effects of landscape cover and local habitat characteristics on visiting bees in tropical orchards. Agr. Forest Entomol. 20, 28–40. doi: 10.1111/afe.12226
Tarakini, G., Cheruma, A., Tarakini, T., and Musundire, R. (2020). Drivers of diversity and community structure of bees in an agroecological region of Zimbabwe. Ecol. Evol. 11, 6415–6426. doi: 10.1002/ece3.7492
Thébault, J., and Fontaine, C. (2010). Stability of ecological communities and the architecture of mutualistic and trophic networks. Science 329, 853–856. doi: 10.1126/science.1188321
Theodorou, P., Herbst, S. C., Kahnt, B., Landaverde-González, P., Baltz, L. M., Osterman, J., et al. (2020a). Urban fragmentation leads to lower floral diversity, with knock-on impacts on bee biodiversity. Sci. Rep. 10, 21756. doi: 10.1038/s41598-020-78736-x
Theodorou, P., Radzevičiute, R., Lentendu, G., Kahnt, B., Husemann, M., Bleidorn, C., et al. (2020b). Urban areas as hotspots for bees and pollination but not a panacea for all insects. Nat. Commun. 11, 576. doi: 10.1038/s41467-020-14496-6
Traveset, A., Castro-Urgal, R., Rotllán-Puig, X., and Lázaro, A. (2020). Effects of habitat loss on the plant – fl ower visitor network structure of a dune community. Oikos 127, 45–55. doi: 10.1111/oik.04154
Tylianakis, J., Tscharntke, T., and Lewis, O. (2007). Habitat modification alters the structure of tropical host–parasitoid food webs. Nature 445, 202–205. doi: 10.1038/nature05429
Vaca-Uribe, J. L., Figueroa, L. L., Santamaría, M., and Poveda, K. (2021). Plant richness and blooming cover affect abundance of flower visitors and network structure in Colombian orchards. Agric. For. Entomol. 23, 546–555. doi: 10.1111/afe.12460
Valido, A., Rodríguez-Rodríguez, M. C., and Jordano, P. (2019). Honeybees disrupt the structure and functionality of plant-pollinator networks. Sci. Rep. 9, 4711. doi: 10.1038/s41598-019-41271-5
Venables, W. N, and Ripley, B. D.. (2002). Modern Applied Statistics with S. New York, NY: Springer. p. 271–300. doi: 10.1007/978-0-387-21706-2
Vides-Borrell, E., Porter-Bolland, L., Fergunson, B. G., Gasselin, P., Vaca, R., Valle-Mora, J., et al. (2019). Polyculture, pastures and monocultures: effects of land use intensity on wild bee diversity in tropical landscapes of southeastern Mexico. Biol. Conserv. 236, 269–280. doi: 10.1016/j.biocon.2019.04.025
Vizentin-Bugoni, J., Maruyama, P. K., Souza, C. S., Ollerton, J., Rech, A. R., and Sazima, M. (2018). “Plant pollinator networks in the tropics: a review,” in Ecological Networks in the Tropics, eds W. Dáttilo, and V. Rico- Gray (Cham: Springer), 73–91.
Watts, S., Dormann, C. F., Martín González, A. M., and Ollerton, J. (2016). The influence of floral traits on specialization and modularity of plant–pollinator networks in a biodiversity hotspot in the Peruvian Andes. Ann. Bot. 118, 415–429. doi: 10.1093/aob/mcw114
Wayo, K., Sritongchuay, T., Chuttong, B., Attasopa, K., and Bumrungsri, S. (2020). Local and landscape compositions influence stingless bee communities and pollination networks in tropical mixed fruit orchards, Thailand. Diversity 12, 428. doi: 10.3390/d12120482
Wenzel, A., Grass, I., Belavadi, V. V., and Tscharntke, T. (2020). How urbanization is driving pollinator diversity and pollination—a systematic review. Biol. Conserv. 241, 108321. doi: 10.1016/j.biocon.2019.108321
Woodcock, B. A., Garrant, M. P. D., Powney, G. D., Shaw, R. F., Osborne, J. L., Soroka, J., et al. (2019). Meta-analysis reveals that pollinator functional diversity and abundance enhance crop pollination and yield. Nat. Commun. 10, 1481. doi: 10.1038/s41467-019-09393-6
Keywords: land use, hoverflies, small-scale agriculture, pollination, bumblebees, honeybees
Citation: Escobedo-Kenefic N, Casiá-Ajché QB, Cardona E, Escobar-González D, Mejía-Coroy A, Enríquez E and Landaverde-González P (2022) Landscape or local? Distinct responses of flower visitor diversity and interaction networks to different land use scales in agricultural tropical highlands. Front. Sustain. Food Syst. 6:974215. doi: 10.3389/fsufs.2022.974215
Received: 21 June 2022; Accepted: 14 November 2022;
Published: 08 December 2022.
Edited by:
Celia A. Harvey, Monteverde Institute, Costa RicaReviewed by:
Alistair John Campbell, Embrapa Amazônia Oriental, BrazilCopyright © 2022 Escobedo-Kenefic, Casiá-Ajché, Cardona, Escobar-González, Mejía-Coroy, Enríquez and Landaverde-González. This is an open-access article distributed under the terms of the Creative Commons Attribution License (CC BY). The use, distribution or reproduction in other forums is permitted, provided the original author(s) and the copyright owner(s) are credited and that the original publication in this journal is cited, in accordance with accepted academic practice. No use, distribution or reproduction is permitted which does not comply with these terms.
*Correspondence: Natalia Escobedo-Kenefic, ZXNjb2JlZG8ubmF0YWxpYUB1c2FjLmVkdS5ndA==; Patricia Landaverde-González, cGF0eWxhbmRhdnJAZ21haWwuY29t
†These authors have contributed equally to this work and share first authorship
Disclaimer: All claims expressed in this article are solely those of the authors and do not necessarily represent those of their affiliated organizations, or those of the publisher, the editors and the reviewers. Any product that may be evaluated in this article or claim that may be made by its manufacturer is not guaranteed or endorsed by the publisher.
Research integrity at Frontiers
Learn more about the work of our research integrity team to safeguard the quality of each article we publish.