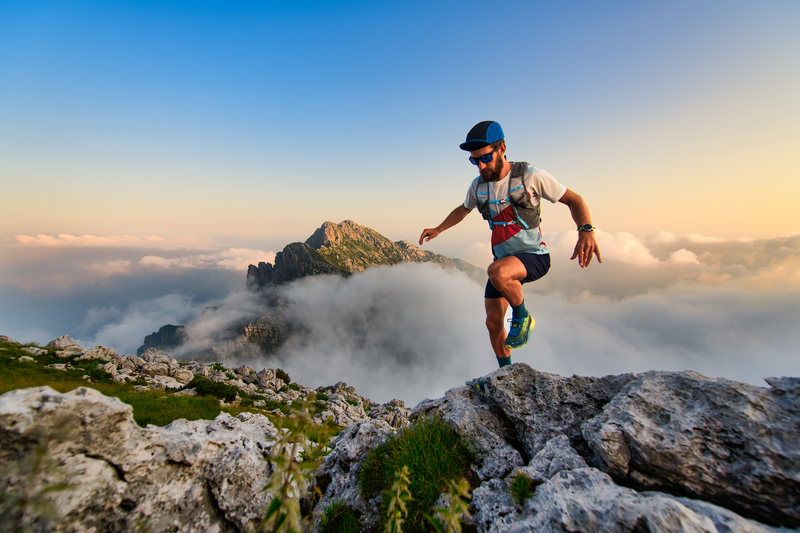
94% of researchers rate our articles as excellent or good
Learn more about the work of our research integrity team to safeguard the quality of each article we publish.
Find out more
ORIGINAL RESEARCH article
Front. Sustain. Food Syst. , 05 October 2022
Sec. Land, Livelihoods and Food Security
Volume 6 - 2022 | https://doi.org/10.3389/fsufs.2022.965767
This article is part of the Research Topic Improving Investment in Research and Innovation to Transform Agrifood Systems in the Global South View all 13 articles
This paper provides estimates of the global investment gap in agricultural research and development (R&D) and innovation. The investment gap is defined as the additional annual investments required to end hunger in 2030 (Sustainable Development Goal SDG2) and to put agriculture on the pathway to the Paris Agreement target for 1.5◦C increase over pre-industrial temperature levels. The investment gap is projected relative to a reference scenario with projections to 2030 using an integrated economic-biophysical model of the global agri-food system. In addition to showing the impacts on hunger, the modeling results are used to simulate the effect of the gap-closing investments on greenhouse gas (GHG) emissions from agriculture. In addition to projecting the impacts of overall investment in agricultural R&D on productivity and environmental outcomes, the analysis assesses the contributions of different types of innovative technologies and farming systems to the environmental outcomes, especially technologies that contribute to sustainability outcomes. Sustainability-oriented technologies and management practices examined include conservation tillage, nitrogen-use efficiency, improved livestock management, and other climate-smart technologies. The projected results show that additional agricultural R&D investments of USD 4 billion per year above baseline investments together with USD 6.5 billion per year invested in technical climate-smart options, can reduce hunger to 5% globally and achieve 2030 GHG emission reductions consistent with the Paris Agreement 2◦C and 1.5◦C pathways to 2030.
If the world is to achieve the Sustainable Development Goal 2 (SDG2) (United Nations 2022), to end hunger and succeed in stabilizing global warming at below 2◦C, and adapt to the climate change that this warming will bring, agricultural systems must transform significantly by 2030. This will not be easy. A rising global population, rapid income growth, and urbanization are having profound effects on the demand and patterns of agricultural production (Godfray et al., 2010; Hawkes et al., 2017; Rosegrant et al., 2017). While hunger persists for too many people, diets continue to shift toward convenience foods and fast foods (Ruel et al., 2017; Fan et al., 2019). Developments in consumption patterns are positive in some respects but negative in many others. There is increased consumption of fruits and vegetables; growing demand for sugar, fats, and oils; and rapid growth in meat consumption and therefore higher demand for feed grains or other livestock feeds (Godfray et al., 2010; Kearney, 2010; Thornton, 2010; Rosegrant et al., 2017). As these demands put pressure on food systems and sustainable food production growth also faces challenges from climate change, with higher temperatures, changing precipitation patterns, as well as a likely increase in weather variability (Smith et al., 2018; Mbow et al., 2019).
Concurrently, agriculture itself is a major contributor to greenhouse gas (GHG) emissions, so sustainable intensification needs to contribute to climate change solutions by reducing GHG emissions and sequestering carbon (Smith et al., 2018; Mbow et al., 2019). Agriculture needs to use less land if the world is to reverse deforestation and halt the global collapse in biodiversity.
A transformation this large and rapid will require investment in innovations for sustainable agriculture intensification. These are innovations that seek to produce the food needed to meet changing human needs while simultaneously ensuring the long-term productive potential of natural resources, such as water and land resources, and the associated ecosystems and their functions. This research pulls together multiple modeling techniques to estimate the size of that investment.
Specifically, this paper uses integrated economic and biophysical modeling and assessment of climate-smart and resource-saving technical options to identify the innovation investment gap that needs to be filled to ensure that sustainable agriculture intensification supports the achievement of specific global goals:
• Ensuring that less than 5% of the world's population is at risk of hunger by 2030 (SDG2, using the FAO threshold for zero hunger) (FAO et al., 2015).
• Reducing and sequestering emissions in agriculture and stopping emissions from land-use change for food production, on a trajectory consistent with stabilizing temperature increase below 2◦C (Paris Agreement).
For this study, innovation for sustainable agriculture intensification is defined as the creation, development, and implementation of new technologies, techniques, and management practices for sustainable productivity growth, climate mitigation, and water resource improvement that drive progress toward achieving the above goals and trajectories. The specific innovation investments that are analyzed follow:
• Public and private investments in agricultural research and development (R&D) for the Global South, which consists of sub-Saharan Africa, Latin America and the Caribbean, Pacific Islands, and the low- and middle-income countries in Asia, the Middle East, and North Africa.
• Investments to support the adoption of technical mitigation options for climate change mitigation in agriculture through carbon payments or other forms of targeted subsidies or payments of environmental services.
The analysis of agricultural R&D investments covers the key actors for the Global South, the international public research institutions of the CGIAR (a global partnership of international agricultural research centers, formerly known as the Consultative Group for International Agricultural Research), national agricultural research systems (NARS), and the private sector. The CGIAR operates through research partnerships at 15 different international agricultural research centers. CGIAR has more than 9,000 staff working in 89 countries around the world (CGIAR, 2022). NARS are national public research institutes based in the Global South, which primarily conduct locally relevant research for the benefit of their own nations. Private sector investments included here are those directly allocated to the Global South, including expenditures by international companies and national companies in the Global South. Analysis of the investment requirements and investment gap up to 2030 uses model-based investment scenarios combined with analysis of specific climate-smart and resource-saving technical options as well as management practices that can reduce GHG emissions and increase GHG sequestration. The SDG2 (zero hunger) and the Paris Agreement (UNFCCC, 2020) on climate change provide the specific sustainability context in which the investment gaps are evaluated. The targets and indicators of progress used to assess the effectiveness of investments in addressing the gaps follow.
SDG2. End hunger by 2030 (part of SDG target 2.1). The target of ending hunger is defined as the reduction of hunger to a 5% share of the population by 2030. This target is based on the FAO et al. (2015) Achieving Zero Hunger report, which adopted “a prudential threshold of five percent of the population” as indicating ending hunger. The methodology is based on the reduction in hunger due to increased calorie availability for consumption. This target, together with the mitigation in line with the Paris Agreement climate trajectories described below, are the measures that determine the agricultural innovation investment gap. For the other targets, we measure progress based on the indicators described below, where the investment target defined by meeting the investment gap is achieved. Progress is measured relative to the outcomes under the reference scenario (REF_HGEM).
Paris Agreement. The Paris Agreement provides broad targets for mitigation. It calls for “a long-term goal of keeping the increase in global average temperature to well below 2◦C above pre-industrial levels; and to aim to limit the increase to 1.5◦C, since this would significantly reduce risks and the impacts of climate change.” Wollenberg et al. (2016), drawing upon the results of leading integrated assessment models, estimated a global requirement of reducing non-carbon dioxide (CO2) GHG emissions from agriculture by 1,000 million tons of CO2 equivalents (MtCO2eq)/year by 2030 to limit warming in 2100 to 2◦C above pre-industrial levels. This target was estimated based on the findings of leading integrated assessment models: Reisinger et al. (2013) estimated a requirement for non-CO2 mitigation of 930 MtCO2eq/year in 2030; van Vuuren et al. (2011) estimated 1,370 MtCO2eq/year; and Wise et al. (2014) estimated 920 MtCO2eq/year (all cited in Wollenberg et al., 2016). We adopt this target as the mitigation requirement for investment in sustainable agriculture intensification. Target estimates for non-CO2 mitigation in 2050 are not available and targets for a 1.5◦C pathway are also unavailable. Targets have been estimated for CO2 emissions that are consistent with the 1.5◦C pathway, but not for a 2◦C pathway. Rogelj et al. (2018) estimated targets for these CO2 emissions consistent with the 1.5◦C pathway based on the set of scenarios outlined by the Intergovernmental Panel on Climate Change (IPCC, 2018). The target is to sequester 100 MtCO2/year by 2030, and 2,300 MtCO2/year by 2050. These estimates are based on a low-overshoot scenario and are at the upper end of the required reductions outlined in these scenarios (Rogelj et al., 2018; McKinsey and Company, 2020). SDG13 is also related to climate change. It sets forth targets for climate action focused primarily on policies for adaptation: to strengthen resilience and adaptive capacity to climate-related disasters; integrate climate change measures into policy and planning; build knowledge and capacity to meet climate change; implement the UN Framework Convention on Climate Change; and promote mechanisms to raise capacity for planning and management. This paper does not address these policies directly.
The total investment gap includes the required investment in agricultural R&D and the required investment in climate-smart and resource-saving technical options and management practices. In addition to showing the impacts of the gap-closing investments on hunger and GHG emissions—including CO2 and non-CO2 [methane (CH4) and nitrous oxide (N2O)] emissions—the analysis shows the impacts of these investments on per capita income, gross domestic product (GDP), and food prices. Results are reported both for 2030 and 2050 to show the longer-term impacts of potential gap-closing investments.
The primary tool for the scenario analysis is IFPRI's International Model for Policy Analysis of Agricultural Commodities and Trade (IMPACT) framework, an integrated modeling system that combines information from climate models (Earth System Models, ESMs), crop simulation models (Decision Support System for Agrotechnology Transfer—DSSAT), and river basin level hydrological and water supply and demand models. This information is linked to a global, partial equilibrium, multimarket model focused on the agriculture sector (Robinson et al., 2015) with a high level of disaggregation across 158 countries, 154 water basins, and 60 commodities. ESMs provide monthly rainfall and temperature data under alternative climate change scenarios, the results of which were downscaled to the pixel level for input into the crop models. The hydrological and water supply and demand models then determine the runoff available for crop production based on the downscaled rainfall data along with interactions across other economic sectors (industrial, domestic, livestock, and irrigation).
DSSAT, developed by Jones et al. (2003), and frequently updated since then, integrates crop, soil, and weather databases into standard formats for use by crop models and other applications. Weather statistics, including the availability of water from rainfall and runoff and temperature from climate models are incorporated in order to estimate crop yield impacts under existing and various future climate scenarios. The biophysical models are then used to estimate the impacts of climate change (through changing temperature and water availability) on crop yields. All crop yield simulations, both in the reference case and any alternative scenarios, are within bounds of known biophysical limits through an iteration check against the Global Yield Gap Atlas (van Ittersum et al., 2013). Biophysical yield shocks from climate change are then input into the IMPACT partial equilibrium model. Climate change shocks induce economic feedback effects in the model. Taking the example of an initial negative impact on yields, the drop in supply will induce higher commodity prices, which in turn generate yield and area increases in the model, which partly compensate for the initial biophysical shock.
IMPACT is linked to a global general equilibrium model, GLOBE (Willenbockel et al., 2018). The link with the GLOBE model enables the assessment of the economy-wide impacts of climate change and agricultural investments, including GDP and per capita income. Linking IMPACT and GLOBE allows quantitative analyses of the impact of changes in investment in innovation in the agricultural sector on the rest of the economy. The feedback from GLOBE to IMPACT captures the endogenous effect of changes in income on food demand, food prices, and hunger. The output from the above modeling provides the drivers for further analyses that estimate the effects of alternative scenarios on the share and number of hungry people and GHG emissions.
The GHG emissions post-processor gives the GHG impacts generated by modeled changes in crop and livestock production systems caused by agricultural productivity growth in the different scenarios. The empirical approach to estimating GHG emissions uses IPCC Tier 1 factors for GHG emissions (IPCC, 2006; Yan et al., 2009). The Tier 1 method, which provides a default emission factor and scaling factors, is applied to countries in which country-specific emission factors do not exist. The Tier 2 method is the same as the Tier 1 method but requires that country-specific emission factors and/or scaling factors be used. The Tier 1 method is the most feasible for application at a global scale for a modeling analysis such as this paper because Tier 2 factors are not available for most countries (Yan et al., 2009). The GHG emissions are estimated from three subcategories: synthetic fertilizers (N2O), rice cultivation (CH4), and enteric fermentation (CH4) in livestock. To simulate emissions, we employ the IPCC Tier 1 factors for direct N2O emissions arising from mineral nitrogen (N) fertilizer application to managed soils. The CO2 equivalent (CO2eq) for these emissions is computed by multiplying the amount of the GHG by its global warming potential.
The IPCC Tier 1 default factors for direct N2O emissions arising from mineral N fertilizer application are 0.01 kg N2O-N per kg N fertilizer applied to managed soils and 0.003 kg N2O-N per kg N fertilizer applied to irrigated rice. These factors are multiplied by the N fertilizer consumption projections for each country and each crop/commodity. Note that the N2O emissions we estimate exclude the indirect N2O emissions from N leaching and runoff and atmospheric N deposition.
To estimate CH4 emissions from rice production, we combine crop/commodity yield projections with Tier 1 emission factors from Yan et al. (2009), enhanced by scaling factors. Emissions factors for this approach include the baseline emission factor for continuously flooded fields without organic amendments, a scaling factor for differences in the water regime during the cultivation period (e.g., single drainage and multiple drainages), and a scaling factor for both the type of organic amendment applied (e.g., rice straw and farmyard manure) and the amount. These CH4 emissions from rice production are first calculated for a unit of area and then multiplied by rice production areas projected by IMPACT.
Livestock production is responsible for CH4 emissions from enteric fermentation and both CH4 and N2O emissions from livestock manure management systems. Among several species of livestock, ruminants such as cows, buffaloes, camels, and goats are important sources of CH4 in many countries because their ruminant digestive systems have high CH4 emission rates (IPCC, 2006). Thus, CH4 emissions from ruminants are estimated based on animal number projections (both slaughtered cattle and dairy animals) and emission numbers from the enteric fermentation section of FAOSTAT. To estimate emissions from the entire herd of ruminants, the projected numbers of each type of animal (slaughter cattle, dairy cows, goats, sheep, camels, and buffaloes) are multiplied by the emission value obtained from FAOSTAT for per-head emissions from enteric fermentation.
Finally, the GHG emissions from changes in land cover driven by changes in crop area harvested and pastureland are computed. The relationship between changes in crop area and livestock production and total cropland and forest area are derived from simulations that linked IMPACT and LandSHIFT, a land use and land cover change model (Schaldach et al., 2011). The estimated changes in forest area driven by changes in area and livestock production under alternative scenarios are then multiplied by a coefficient for estimated GHG sequestration per unit of forest area to compute the carbon sequestration generated by the investment scenarios. This coefficient varies by type of forest and region. Sequestration rates for afforestation/reforestation range within 0.8–2.4 t/ha/year in boreal forests, 0.7–7.5 t/ha/year in temperate regions, and 3.2–10 t/ha/year in the tropics (Brown et al., 1996). Given these ranges, we utilize a medium level of sequestration per hectare of forests of 6 MtCO2/ha/year to compute global sequestration of CO2 under the agricultural R&D investment scenarios.
The scenarios utilize key elements of IPPC scenarios. The IPCC scenarios are defined by two major components. First, Shared Socioeconomic Pathways (SSPs) are global pathways that represent alternative futures for economic and population growth (O'Neill et al., 2014, 2015). Population growth and GDP growth assumptions in the reference scenario are drawn from SSP2, which is a middle-of-the-road scenario based on historical trends and potential changes in trajectories in economic and demographic growth. The SSP2 scenario corresponds to the medium variant of IIASA-VID-Oxford population projections, where global population reaches 8.3 billion by 2030 with a global GDP of US$143 trillion. Under SSP2, expected changes in population and economic growth vary substantially by region. Population growth is concentrated in the developing world, where population grows at more than one percent per year adding, by 2030, almost 1.4 billion people globally, compared to only 0.1 billion in developed countries. Economic growth is also faster in developing countries, with an average annual growth rate of 5% compared to 2% in developed countries (Mason-D'Croz et al., 2019).
The second key component in the IPCC scenarios is the Representative Concentration Pathways (RCPs), which represent potential GHG emission levels in the atmosphere and the subsequent increase in solar energy that would be absorbed (radiative forcing). There are four RCPs, named according to the approximate level of radiative forcing in 2100, which ranges from 2.6 watts per square meter (W/m2) to 8.5 W/m2. Through 2030, there are limited differences in atmospheric concentration of GHGs across the four RCPs ranging from concentration levels between 445 ppm and 480 ppm in 2030 compared to approximately 375 ppm in 2005. Radiative forcing in 2030 ranges from 2.9 W/m2 to 3.3 W/m2 compared to 1.9 W/m2 in 2005. The RCPs begin to diverge more significantly by mid- and end-of-century. For this analysis we use RCP 8.5, the most severe of the RCPs, which has a radiative forcing pathway leading to 8.5 W/m2 (approximately 1,370 ppm CO2 eq) by 2100.
Following the establishment of the reference scenario, additional scenarios are run to assess the gap in public and private agricultural R&D investment, defined as the additional annual investments above the business-as-usual reference scenario required to end hunger in 2030. Increased agricultural R&D affects hunger by boosting crop and livestock yields, reducing food prices, and increasing farm income and economy-wide GDP through multiplier effects on the non-agricultural sectors. The lower prices and higher incomes boost food consumption. In addition to showing the projected impacts on hunger, the modeling results provide estimates of the effect of the gap-closing investments on GHG emissions from agriculture and deforestation.
The impact of overall agricultural R&D investments is captured in the model in terms of productivity gains and subsequent impacts on environmental and other outcomes. In this article we build on previous work on cost estimation, such as Nelson et al. (2010), using data on research costs (investments) collected by the Agricultural Science and Technology Indicators program, as well as literature on the economic and productivity returns to investments in agricultural research (e.g., Evenson and Gollin, 2003; Alston et al., 2011; Nin-Pratt et al., 2015; Nin-Pratt, 2016). This literature establishes a quantitative relationship between changes in the stock of investment in agricultural R&D and changes in agricultural productivity. The baseline private sector investment in agricultural R&D is estimated based on Pardey et al. (2006) and Fuglie (2016).
Beyond the reference scenario, we have developed an R&D investment–yield model to assess the investment required to achieve projected growth in agricultural productivity under the alternative investment scenarios. Investments in research take time to bear fruit, as new ideas can take years to develop and spread. To capture these lags, we utilize an investment–yield estimation model based on the perpetual inventory method, in which research investments contribute to the stock of knowledge over time. Knowledge decays as older technologies become obsolete or irrelevant. Productivity grows if the stock of knowledge grows at a faster rate than it decays. The lag structure in the perpetual inventory method used here follows a gamma distribution in which R&D investments reach peak impact 10–15 years after initial investment and then decline over time to zero impact 10–15 years after peak impact. With regionally differentiated elasticities of yield with respect to research and decay rates, these imputed lag structures vary by region according to existing R&D capacity and the potential trajectories for each region. The elasticity of yield with respect to research measures the percentage change in yields with respect to the stock of knowledge, which is explained in the paragraph above. See Appendix J of Rosegrant et al. (2017) for parameters used in the perpetual inventory method. This approach allows us not only to estimate the baseline costs in research implied under the reference scenario to 2050 but also to estimate the additional investments needed to adapt to climate change and make progress toward selected SDGs. Improvements in agricultural productivity in the reference scenario are represented by exogenous growth rates for each commodity and country, based on historical trends as well as expert opinion about future changes.
Accounting for both public and private investments, the first component of the investment gap is computed as the difference in investments between the reference scenario and the level of investments required to end hunger (SDG2 calorie-based target) in 2030. Investments in the scenario analysis focus on agricultural R&D. In addition to food security impacts, the impact on emissions of CO2, non-CO2, and those due to long-term productivity growth in agriculture are projected based on the outcomes of the investment scenarios. In the IMPACT modeling system, investments in agricultural R&D for productivity growth also influence projected GHG emissions by reducing commodity prices, crop area harvested, animal numbers, and fertilizer use due to improved N use efficiency (using less N per unit of output), and by changing cropping and livestock production patterns.
Along with a reference business-as-usual scenario, alternative agricultural R&D investment scenarios are analyzed in this article. The scenarios for higher investments in agricultural R&D include international public research institutions of the CGIAR, national agricultural research systems (NARS), research efficiency investments, and the private sector (Table 1).
For the reference scenario, REF_HGEM, investments in agricultural R&D by CGIAR are projected to average USD 1.7 billion per year during 2015–2050 in real 2005 dollars, while annual NARS investment in the Global South averages USD 6.4 billion per year (Table 2). The largest investments are projected in sub-Saharan Africa (SSA) (USD 2.2 billion per year) and Latin America and the Caribbean (LAC) (USD 1.8 billion per year). In most regions, the largest contribution to agricultural research will come from investments from NARS. The exception is SSA, where about half of the investments will come from CGIAR.
Table 2. Average annual investments in the Global South in the reference scenario (REF_HGEM), 2015–2050 (billion 2005 USD).
Four alternative scenarios seek to enhance agricultural productivity through increased investment in agricultural R&D. These four scenarios vary in level, source, and efficiency of investment (Table 2). Each of these scenarios also uses SSP2 and RCP8.5, so that the results reflect changes in investment, not changes in underlying socioeconomic conditions and climate change. Yield effects for these alternative scenarios are implemented by increasing yield growth rates relative to reference yield growth rates. The HIGH R&D scenario incorporates yield gains from increasing investments in CGIAR R&D and was developed in collaboration with all 15 CGIAR centers through the Global Futures and Strategic Foresight program, an initiative of the Policies, Institutions, and Markets (PIM) research program of the CGIAR (see Prager and Wiebe, 2021). As a starting point, each center quantified potential yield gains for their respective commodities (including crops, livestock, and fish) in the Global South across SSA, LAC, South Asia (SAS), East Asia and the Pacific (EAP), and the Middle East and North Africa (MEN) with increased agricultural R&D investment. The HIGH scenario adds USD 2.1 billion annually to the reference costs for CGIAR investment in REF_HGEM, heavily concentrated in SSA.
In the scenario HIGH+NARS, the increased investment by CGIAR is complemented by an increase in NARS spending in the Global South of USD 1 billion per year. The largest shares of this increase are in SSA and MEN, which contribute almost two-thirds of additional NARS investments.
Scenario HIGH+NARS+REFF adds investments in higher research efficiency. Research efficiency is gained through advances in breeding techniques, including in genome editing technology, genomics and bioinformatics, and high-throughput gene sequencing, as well as more effective regulatory and intellectual property rights systems that reduce the lag times from discovery to deployment of new varieties (Waltz, 2018; Pourkheirandish et al., 2020; Zhu et al., 2020). For example, Lenaerts et al. (2018) show that a reduction in time of breeding through one technique, rapid generation advance, can generate an increase in economic benefits of 26%, 36%, and 47% with a saving of 3, 4, and 5 years, respectively, at a discount rate of 8%. Falck Zepeda et al. (2012) show that regulatory costs and time lags of 2 years delay would reduce the net present benefit for the adoption of various crop varieties by 23–71%; eliminating those delays and excess costs would increase profits by comparable amounts. Based on these studies, research efficiency is assumed to increase the yield impact of investments by 30%, and the maximum yield improvement is achieved by 2040, 5 years earlier than in the HIGH scenario. Investment in increased research efficiency adds another USD 0.42 billion per year to this scenario.
Scenario HIGH+NARS+REFF+PRIV, the most extensive R&D scenario, adds an increase in private sector investments of 30% to the higher CGIAR, NARS, and research efficiency investments. This adds USD 0.52 billion per year in private investment, with nearly 40% spent in EAP and SAS. Combining all the above R&D costs, the HIGH+NARS+REFF+PRIV investment scenario requires an additional USD 4 billion per year above the reference scenario, an increase of 41%. The private sector accounts for 13% of the additional investments in this scenario.
The analysis of technical options for GHG emissions reduction draws on the available evidence in the literature regarding the potential impact of adopting climate-smart techniques and management practices on GHG emissions, the cost of adoption for these practices, and the adoption potential of technical options. The four agricultural activities included in the analysis are management of cropland, rice, pasture, and livestock, as defined in IPCC publications (Smith et al., 2007, 2014; IPCC, 2014).
The second part of the investment gap is therefore calculated as the additional investment required in technical mitigation options to achieve the targets for non-CO2 and CO2 emission reductions and sequestration in agriculture in 2030 that are consistent with 2◦C and 1.5◦C climate change trajectories. Restoration of agricultural soils is not included. Following IPCC guidelines for accounting for GHG emissions in agriculture (IPCC, 2006), upstream and downstream emissions such as the production of fertilizer and other inputs and value chain emissions are not included. The technical options considered follow:
• Improved cropland management. This is an important potential method to reduce N2O emissions and sequester CO2. These can be achieved through agronomy (crop rotation and cover crops); conservation tillage and residue management; improved water management to reduce fertilizer runoff; and improved nutrient management through precision agriculture, advanced types of fertilizer, new N-use-efficient crop varieties, and stabilized N sources (polymer-coated urea and nitrification inhibitors).
• Improved rice management for reduction of CH4 emissions. This includes mid-season drainage of rice paddies and alternate wetting and drying.
• Pasture management, which can reduce GHG emissions through improved grasses and pasture management, improved manure management, and the use of legumes.
• Livestock management, which reduces CH4 emissions with improved feeding practices and feed additives, improved manure management systems, and breeding and long-term management.
The assessment of technical options for GHG mitigation is based on data and research outcomes available from IPCC documents and other publications. Sources consulted include Smith et al. (2008, 2018), Del Grosso and Cavigelli (2012), Smith et al. (2013), Havlík et al. (2014), IPCC (2014); Beach et al. (2015), Herrero et al. (2016), Wollenberg et al. (2016), Frank et al. (2018), IPCC (2018), and EPA (2019). Key parameters considered in the assessment include the potential savings in tCO2eq per hectare or per animal unit from the adoption of technical options, the rate of adoption of technical options in terms of percentage of area or herd, and the cost of investment in mitigation from each technical option in USD per tCO2eq. Investment costs include incremental annualized capital costs where applicable (many of the mitigation practices are more focused on changes in practices and inputs than capital expenditures) and estimated incremental changes in the annual costs of agricultural labor, fertilizer, and other inputs. Following the practice in these sources, the technologies are assumed to be yield-neutral, so the costs do not include revenue changes for farmers due to possible productivity increases or decreases related to the application of a technology (Frank et al., 2018). For any given technology, this assumption could lead to over- or under-estimation of the cost of GHG emission reductions. Future research that rigorously assesses the yield impacts could allow this assumption to be dropped. The assumptions regarding the range of values for the key parameters are shown in Table 3.
Table 3. Assumptions for analysis of technical climate mitigation potential and costs: range of values used.
In agriculture, there is a relationship between the amount paid for GHG emission reductions (i.e., the price per tCO2eq) and the level of mitigation realized. The economic potential for mitigation options in agriculture increases as the carbon price rises. For this analysis, we assess the potential for GHG mitigation from the adoption of technical mitigation options at a carbon price of USD 70 per tCO2eq. This carbon price was chosen for assessment based on a review of the literature as a carbon price that would potentially generate GHG emissions reductions that would be consistent with the Paris Agreement pathways (see Del Grosso and Cavigelli, 2012; Smith et al., 2014; Beach et al., 2015; Frank et al., 2018).
Based on these parameters we compute the annual costs of potential mitigation in MtCO2eq and of investment in mitigation in 2030 and 2050 in million USD. The key parameters vary across sources and different ranges are reported in many of the sources. To capture this variability, calculations are made for a series of combinations of the parameters to assess a distribution of potential outcomes. These results allow us to compute the investment required to generate GHG emissions reductions consistent with the Paris Agreement pathways. The investment requirements represent the total carbon payments or payments for environmental services that need to be paid to induce the adoption of the technical options needed to generate mitigation consistent with a 2◦C climate trajectory.
Projected percentage increases in crop and livestock production under the investment scenarios relative to the reference scenario (REF_HGEM) are shown in Figures 1, 2. The regions assessed are EAP, East Asia and Pacific; SAS, South Asia; SSA, sub-Saharan Africa; MEN, Middle East and North Africa; LAC, Latin America and the Caribbean; and DVG, Global South (the total of the other regions). Agricultural production growth in SSA has lagged significantly behind the rest of the world, but with the heavy concentration of investment in agricultural R&D in this region in the investment scenarios, both crop and livestock production growth in SSA are projected to grow faster relative to the reference scenario than in other regions. Crop and livestock production in SAS will also grow rapidly. There is strong growth in MENA in crop production in percentage terms, from a low reference level; LAC has substantial growth in livestock production; and EAP has relatively slow growth in both crop and livestock production, from very high reference levels.
Figure 1. Percent changes in total crop production under alternative R&D investment scenarios compared to the reference scenario. WLD, world; DVG, Global South; EAP, East Asia and Pacific; SAS, South Asia; SSA, sub-Saharan Africa; MEN, Middle East and North Africa; and LAC, Latin America and the Caribbean. Source: IMPACT model.
Figure 2. Percent changes in total livestock production under alternative R&D investment scenarios compared to the reference scenario. WLD, world; DVG, Global South; EAP, East Asia and Pacific; SAS, South Asia; SSA, sub-Saharan Africa; MEN, Middle East and North Africa; and LAC, Latin America and the Caribbean. Source: IMPACT model.
The share of people at risk of hunger is the percentage of the total population in a country that is at risk of suffering from undernourishment. This calculation is based on the empirical correlation between the share of undernourished within the total population and the relative availability of food and is adapted from the work done by Fischer et al. (2005) in the International Institute for Applied Systems Analysis (IIASA) World Food System used by IIASA and FAO. This approach is equivalent to the FAO prevalence of undernourishment metric (FAO, 2008). The number of hungry people is then computed as the share of people at risk of hunger multiplied by the population. The results for the impact of the investment scenarios are shown in Table 4.
The rise in productivity growth under the increased investment in agricultural R&D scenarios boosts per capita income and results in lower food prices, which in turn increases the demand for food, particularly for lower-income groups. The result is that for the Global South, the population at risk of hunger is reduced by 22% under the HIGH+NARS+REFF+PRIV scenario relative to the reference scenario in 2030, less than half its 2010 level. The biggest reductions in hungry people to 2030 are in SAS. The HIGH+NARS+REFF+PRIV and HIGH+NARS+REFF scenarios achieve the SDG2.1 target at the 5% share at risk of hunger in EAP, SAS, and LAC.
However, SSA remains well above the SDG2.1 target with an 11.8% share at risk of hunger in 2030, although this is a major improvement relative to its 24.3% share at risk of hunger in 2010. After 2030, the number of hungry people in SSA falls sharply as the effects of agricultural productivity growth accumulate, and by 2050 the region reaches a share of 5.3% at risk of hunger. Given the lags from investment in R&D to impacts on productivity and hunger, it is not feasible to design an even higher R&D investment scenario to try to achieve the 5% target for SSA by 2030 while still improving performance elsewhere. Moreover, other types of investment and policies are needed to address persistent hunger, including income transfers and social safety nets (World Bank, 2012).
Along with the progress in achieving global hunger goals, the investment scenarios generate large economic returns. The R&D investment alone adds USD 1.7 trillion to the GDP of the Global South in 2030, and USD 9.1 trillion in 2050. In these countries, investment raises national average per capita income by 1.9% in 2030 and 5.9% in 2050 relative to business as usual (Tables 5, 6). The increases in investment in SSA generate the highest proportional per capita income gains among the various regions: 8% by 2030 and 23.5% by 2050.
Table 5. Average per capita incomes in the reference scenario (thousand 2005 USD per person) and percent differences under alternative investment scenarios in 2030 and 2050.
Table 6. Regional increase in GDP under different investment scenarios in 2030 and 2050 compared to REF_HGEM (trillion 2005 USD).
Across all the alternative investment scenarios, increases in yields and production drive a reduction in food prices in 2030 and 2050 relative to the reference scenario (Table 7). Climate change reduces yields relative to a counterfactual climate scenario that follows a no-climate change (NoCC) pathway, assuming a constant climate after 2015, with atmospheric concentration of GHGs remaining at 2015 levels. Including both the biophysical impacts and the induced responses described in the methodology section, climate change as specified in REF_HGEM reduces global average crop yields compared to NoCC in 2050 scenario by 8.8% for cereals and oilseeds, 6.5% for roots and tubers, 2.9% for fruits and vegetables, and 1.9% for pulses. The reduced yields due to climate change result in increasing prices under REF_HGEM. Cereal prices increase by 43% between 2015 and 2050, oilseeds by 32%, roots and tubers by 42%, fruits and vegetables by 39%, and pulses by 21%. The scenarios with higher investment in R&D result in substantially lower prices for all commodities compared to the reference scenario. For example, under HIGH+NARS+REFF+PRIV the price for oil crops decreases on average by about 21% compared to REF_HGEM in 2050, whereas the decrease is over 43% for roots and tubers, 39% for cereals, and 36% for meat (Table 7). Thus, this level of investment is projected to eliminate or greatly reduce the commodity price increases from 2015 to 2050 caused by climate change.
Table 7. Global aggregated commodity prices, percent difference relative to REF_HGEM in 2030 and 2050.
Although this paper focuses on SSP2 and RCP8.5, a sensitivity analysis run for another recent publication using IMPACT shows that the impacts of investments in agricultural R&D on hunger have a robust effect across the range of potential climate and socioeconomic futures. Sulser et al. (2021) ran scenarios with combinations of socioeconomic assumptions for SSP1, SSP2, and SSP3, and climate assumptions with RCP4.5 and RCP8.5, using the Global Circulation Models HadGEM2-ES (Jones et al., 2011), IPSL-CM5A-LR (Dufresne et al., 2013), MIROC-ESM (Watanabe et al., 2011), NorESM1-M (Bentsen et al., 2013; Iversen et al., 2013), and GFDL-ESM2M (Dunne et al., 2012) for agricultural R&D investment scenarios similar to the HIGH, HIGH+NARS and HIGH+NARS+REFF scenarios in this paper. The results for these investment scenarios show a reduction in the population at risk of hunger in the developing world of 15% and 30% in 2030 and 2050, respectively, relative to the reference scenario. The results from this study are consistent with these results, with reductions in the population at risk of hunger in the developing world in 2030 of 15–28%, relative to the reference scenario, and of 20–31% in 2050 for the HIGH, HIGH+NARS, and HIGH+NARS+REFF scenarios (Table 4).
Total global GHG emissions from all sources were 52,000 MtCO2eq in 2015 (Crippa et al., 2021). Direct GHG emissions from agricultural production, together with related emissions from land-use change and forestry, account for nearly one-quarter of global GHG emissions (IPCC, 2014). According to FAO (2021a), direct agricultural emissions were about 5,450 MtCO2eq in 2015. IPCC (2014) estimates the total direct agricultural emissions to be in the range of 4,300–5,300 MtCO2eq/year, with 95% confidence interval of 3,900–7,000 MtCO2eq/year. According to the Food Security Chapter of the IPCC Climate Change Land Special Report (Mbow et al., 2019), about 21–37% of total GHG emissions are attributable to the food system, including emissions from agriculture and land use, storage, transport, packaging, processing, retail, and consumption. Crippa et al. (2021) provide a higher estimate of 34%, with a range of 25–42%. Crop and livestock activities within the farm gate account for 9–14% of total global GHG emissions, consistent with the FAOSTAT and IPCC estimates of direct agricultural emissions above (Mbow et al., 2019). Agriculture is also responsible for 5–14% of total GHG emissions through its impact on land use and land-use change including deforestation and peatland degradation, and 5–10% from supply chain activities (Mbow et al., 2019). The focus of this article is on direct agricultural emissions and the impact of investments on land-use change. Changes in GHG emissions from supply chain activities are not analyzed in this article. Although agricultural land also generates large CO2 fluxes both to and from the atmosphere via photosynthesis and respiration, this flux is nearly balanced on existing agricultural land. Substantial carbon releases, however, result from the conversion of forested land, which is accounted for under the land-use change category.
In the reference scenario, deforestation due to agricultural production for 2015–2030 is projected to be 9 million hectares (Mha)/year, and 7.3 Mha/year for 2030–2050. The reduction in deforestation is due to agricultural R&D expenditures, which increase crop yields and thus reduce the rate of crop area expansion. Taken together, these two estimates give an overall projected rate of deforestation due to agricultural production of 8.15 Mha/year, which is consistent with the available evidence. According to FAO (2020), the annual global rate of deforestation was 10 Mha/year for 2015–2020, and it is estimated that 80% of global deforestation, 8 Mha/year, is caused by agricultural activities (Kissinger et al., 2012). Under the HIGH+NARS+REFF+PRIV scenario, the projected average annual reduction in deforestation is 925,000 ha/year for 2015–2030 and 1 Mha/year for 2030–2050. Thus, under HIGH+NARS+REFF+PRIV, the projected annual average rate of deforestation is 8.1 Mha/year for 2015–2030 and 6.3 Mha/year for 2030–2050.
The HIGH+NARS+REFF+PRIV scenario contributes to non-CO2 emission reductions of 291 MtCO2eq/year by 2030, relative to the reference scenario. This is due to lower N2O release from fertilizer use and reduced CH4 from rice and livestock production (Table 8). The scenario also achieves CO2 emission reductions of 111 Mt/year from the prevention of deforestation due to productivity growth that results in slower expansion of cropland.
Table 8. Projected GHG emissions reductions and sequestration per year from agriculture due to investments in productivity growth, HIGH+NARS+REFF+PRIV, relative to REF_HGEM. Numbers in parentheses are percent change compared to REF_HGEM.
The results from the agricultural R&D scenarios show that, in addition to coming close to meeting the SDG2 target of ending hunger, the investments of HIGH+NARS+REFF+PRIV make important contributions to reducing GHG emissions, but do not achieve the CO2 or non-CO2 emission reductions necessary for agriculture's contribution to a 2◦C or 1.5◦C climate trajectory. Therefore, additional investments are required to promote the adoption of climate-smart and resource-conserving technical options that can achieve GHG emission reduction outcomes consistent with the Paris Agreement and SDG13, when combined with the reductions achieved through investment in agricultural R&D. It is assumed that the GHG emission reductions from technical options are additive to the GHG emissions reductions generated by the agricultural R&D scenarios. Figures 3A–C show the distribution of estimated potential mitigation of GHG emissions from technical options at a carbon price of USD 70 per tCO2eq. Figure 4 shows the investment required to generate this level of mitigation.
Figure 3. Global agriculture sector technical mitigation potential for (A) non-CO2, (B) CO2 and (C) Total for 2030 and 2050, at a carbon price of USD 70 per tCO2eq. In these box-and-whisker diagrams, the box shows the upper and lower quartiles of the distribution of the results, and the whiskers (the lines extending from the boxes) indicate variability outside the upper and lower quartiles (defined here as highest and lowest values within 1.5 times the interquartile range). Any dots outside the whiskers represent outliers. The means of the distribution are shown as “x” within the boxes and the medians are shown as horizontal lines in the boxes. Source: IMPACT model.
Figure 4. Global cost of technical mitigation, 2030 and 2050, million USD per year, at a carbon price of USD 70 per tCO2eq. Source: Author estimates based on parameters derived from the literature.
The mean non-CO2 technical mitigation economic potential in 2030 is 714 MtCO2eq/year, with a range of 606–815 MtCO2eq/year; the mean potential in 2050 is 783 MtCO2eq/year, with a range of 647–901 MtCO2eq/year (Figure 3A). Comparisons with the literature are not precise because of the different methods employed for these estimations but are nevertheless useful. Del Grosso and Cavigelli (2012) estimate that the potential for non-CO2 agricultural mitigation from technical options at a carbon price of USD 50 per tCO2eq is 693 MtCO2eq/year in 2030; EPA (2019) estimates savings of 593 MtCO2eq/year in 2030 at “increasing prices;” Frank et al. (2018) estimate that adoption of technical options in 2030 can deliver direct non-CO2 emission savings of 500 and 800 MtCO2eq/year at USD 40 and 100 per tCO2eq, respectively, and about 850 MtCO2eq/year at USD 100 per tCO2eq in 2050. Thus, the estimates of economic potential made here are within the range found in the literature. The total cost (investment required in carbon payments) to generate this level of mitigation is shown in Figure 4. The mean cost of technical mitigation is USD 6.5 billion per year in 2030, with a range of USD 5.4–7.9 billion per year. We also ran a sensitivity analysis of the non-CO2 technical mitigation economic potential with respect to carbon prices of USD 50 and 100 per tCO2eq. Although the total cost of mitigation at USD 50 per tCO2eq is of course lower than for USD 70 per tCO2eq, at USD 3.1 billion per year, the mean potential savings is only 483 MtCO2eq/year in 2030, far below the non-CO2 agricultural mitigation needed to be consistent with the 2◦C climate change pathway. At USD 100 per tCO2eq the mean potential non-CO2 technical mitigation economic potential in 2030 is 887 MtCO2eq/year, at a cost of USD 11.6 billion.
Direct comparators for global CO2 sequestration potential at specific carbon prices are not available, but comparators for combined CO2 and non-CO2 mitigation potential are discussed below. The potential for global CO2 sequestration from the implementation of the same technical mitigation options described above for non-CO2 emissions reductions is shown in Figure 3B. Combined total technical mitigation potential includes the values for non-CO2 GHG and CO2 emissions (Figure 3C). The total CO2 technical mitigation potential has a mean of 1,868 MtCO2eq/year in 2030, with a range of 1,613–2,417 MtCO2eq/year; and the corresponding values for 2050 are 2,148 and 1,733–2,511 MtCO2eq/year. In 2030, cropland management accounts for 49% of the total CO2 emission reduction potential, rice management 10%, grasslands 22%, and livestock 19%. The 2030 values fall between the Smith et al. (2014) estimates of mitigation potential in 2030 for the four categories of technical options analyzed here (management of cropland, rice, pasture, and livestock) of approximately 1,575 and 1,950 MtCO2eq/year at USD 50 and 100 per tCO2eq, respectively (estimated from Smith et al., 2014: figure 11.13).
The mean results for total CO2 GHG emissions reductions from Figure 3C are further broken down by technical options in Table 9. The results show that the projected economic mean potential non-CO2 GHG emissions reductions from technical options in agriculture are 1,868 and 2,148 MtCO2eq/year in 2030 and 2050, respectively.
Table 9. Summary of mean potential total emissions reductions from technical options in agriculture at a carbon price of USD 70 per tCO2eq.
Numerous estimates have been made of the cost of achieving various development goals, such as ending hunger, although methods and targets are often specified differently. Estimates vary depending on the specific questions being asked (Fan et al., 2018); the objective of the study; sectors and investments covered; whether climate change is considered; the methods, models, and assumptions used; geographical coverage; and numerous other factors (Mason-D'Croz et al., 2019). Estimates are therefore not directly comparable, but can provide useful context. Results from these studies are summarized in Table 10.
ZEF and FAO (2020) use a marginal cost curve approach to estimate the cost of ending hunger by 2030, finding that total additional annual investments of about USD 39–50 billion are required. Investments and policies considered include agricultural R&D, agricultural extension services, agricultural information systems, small-scale irrigation expansion in Africa, female literacy improvement, child nutrition programs, scaling up existing social protection programs, crop protection, integrated soil fertility management, the African Continental Free Trade Agreement, and fertilizer use efficiency.
FAO et al. (2015) focus on the investments needed to ensure that people have adequate income and resources to get the food they need. To achieve this by 2030 would cost an additional USD 265 billion per year for social protection and pro-poor investments and expenditures, both public and private, in agriculture and rural development. This study looks at the broadest set of investments, including additional public investment in social protection and targeted pro-poor investments in rural areas combined with public and private efforts to raise investment levels in productive sectors.
Laborde et al. (2016), using the MIRAGRODEP dynamic global model, estimate that hunger can be ended by 2030 with additional annual investments of USD 11 billion for 2015–2030. These new public expenditures would fund three categories of interventions: (1) social safety nets directly targeting consumers through cash transfers and food stamps; (2) farm support to expand production and increase farmers' incomes; and (3) rural development that reduces inefficiencies along the value chain and enhances rural productivity.
In a subsequent study, Laborde et al. (2020) find that USD 33 billion annually is needed to end hunger, double the incomes of small-scale producers by 2030, and maintain agricultural GHG emissions below the commitments made in the Paris Agreement. The study includes investments in interventions related to social protection, institutions such as farmers' organizations, and education through vocational training. It also includes interventions provided directly to farmers, including farm inputs, R&D, improved livestock feed, and irrigation infrastructure. Other interventions considered in this study include interventions to reduce post-harvest losses, improve returns from sales, and support the mix of services provided by small- and medium-sized enterprises, such as cooperatives, traders, and processors.
Baldos et al. (2020) examine the required R&D investment costs to adapt to climate change, based on climate-driven crop yield projections generated from extreme combinations of crop and global circulation models. They find that offsetting crop yield losses projected by climate and crop models for 2006–2050 would require increased R&D adaptation investments for 2020–2040 totaling USD 187–1,384 billion (in 2005 USD purchasing power parity). The R&D-led climate adaptation could therefore offer favorable economic returns and deliver gains in food security and environmental sustainability by mitigating food price increases and slowing cropland expansion.
Dalberg (2021) provides an analysis of investment in innovation in agriculture, but they do not link the investment to hunger and climate outcomes. They estimate that the annualized innovation spending on agriculture in the Global South for 2000–2019 was USD 50–70 billion in 2019 constant dollars. Classifying spending estimates by innovation area, Dalberg finds that the areas with the largest shares of funding are public and private R&D funding with 20%, marketing extension and behavior change with 33%, institutional and infrastructure with 20%, and product development with 15%. Although they are not conceptually identical, the Dalberg estimate of USD 10–14 billion for R&D can be compared to the USD 9.8 billion of agricultural R&D investment in the reference scenario in this paper.
Finally, previous studies using IFPRI's IMPACT model analyzed a broader set of investments to assess the impact of boosting agricultural productivity on food security and the environment in the context of climate change. Rosegrant et al. (2017) found that increased global investments in agricultural research, resource management, and infrastructure (irrigation and rural roads), with the aim of increasing agricultural productivity and nearly ending hunger by 2030, would cost an average of USD 52 billion annually for 2015–2030. This is much higher than the cost estimated in this paper due to the inclusion of infrastructure. A comparison between the two papers indicates that shifting additional spending to agricultural R&D may be more cost-effective in addressing hunger than large increases in infrastructure investment relative to recent trends. Nevertheless, expenditures on infrastructure remain important, with substantial investments in irrigation infrastructure and rural roads built into the reference scenario.
Overall, previous studies have higher estimates of investment gaps to end hunger. These higher costs are generally because previous studies target multiple goals and/or because they include investments in broader development initiatives, including infrastructure such as rural roads and irrigation, rural development programs, and social protection programs. The comparative magnitude of these gap estimates with the estimate in this article indicates that investment in innovation may have especially high impacts on ending hunger while also improving the performance of climate change mitigation. Careful targeting of interventions to the hunger goal can also reduce the cost relative to the impact. The study of Laborde et al. (2016) has a relatively low-cost estimate for ending hunger by 2030, at USD 11 billion annually, arrived at by combining the targeting of consumers with cash transfers and food stamps with farm support to expand production and increase farmers' incomes. Nevertheless, broader investments in social protection, infrastructure, and value chains, together with reforms in the areas of gender-responsive policies, agricultural extension, finance for small farmers, and water management, remain essential for sustainable agriculture intensification and economic development.
In common with the studies cited above, our analysis relies on many assumptions and estimated agricultural and economic relationships. As a global economic-biophysical model, IMPACT relies primarily on aggregate national statistics, together with sub-national down-scaling of climate and water resources and must therefore represent economic behavior in a relatively aggregated approach (Mason-D'Croz et al., 2019). The linkage of a disaggregated agricultural partial equilibrium model like IMPACT with GLOBE advances the assessment of economy-wide impacts of investments with feedback to agricultural incomes. Additional disaggregation would further enrich the analysis. The analysis focuses on innovation investments in sustainable intensification of agricultural production, rather than on the full food system. In this analysis, hunger is defined as the SDG 2.1 calorie-based target to end hunger. Future work should also focus on dietary and nutritional security and quality.
The study focuses on SDG 2 and the Paris Agreement pathways. Many other SDGs are also important, such as water resources and biodiversity. Future work on the policies and potentials to improve performance in meeting additional SDGs would be valuable but are beyond the scope of the current analysis.
As with the other studies on ending hunger, we focus on calorie-based hunger. This afflicted 689 million people in 2019, an increase of 10 million from 2018 and nearly 60 million from 2014 (FAO, 2021b). Projections of other aspects of nutrition and food security, such as micronutrient malnutrition and childhood stunting are more complex, as is a distributional analysis by groups of people within countries. It is likely that the cost of addressing these aspects of hunger in addition to calorie-based hunger would be considerably higher than the estimates here. As noted in Rosegrant et al. (2021a), broader malnutrition problems, together with the continued transformation of food systems in developing countries, require wider-ranging approaches and interventions to improve nutritional outcomes than have been used historically. Reducing the impact of these factors would require changes beyond the agricultural sector, including planning, transportation, public health, food production, and marketing (Caballero, 2007; Ruel et al., 2017). Interventions and policies should take into account the need for more sustainable diets that would include a sufficient supply of micronutrient-rich foods without excessive consumption of energy-dense, nutrient-poor foods (Kearney, 2010). In promoting nutrition and health-driven policies, it will be important to target those most in need, particularly children and marginalized populations underserved by essential health services. Furthermore, filling the knowledge gaps through research, scaling innovation solutions, and promoting partnerships across health, nutrition, and agriculture will be important (Fan et al., 2019).
Weak governance, fragile states, extreme climate events, and conflict can reduce the potential gains from investment in agricultural R&D (Mason-D'Croz et al., 2019). The SSPs focus their narratives on long-running trends in the global economy, which, although helpful for exploring scenarios around climate change and long-term drivers in the food system like agricultural R&D, do not include other drivers that are important to global food security. For example, extreme social and environmental events, such as the COVID-19 pandemic and extreme climate shocks, result in year-to-year variability and alter trajectories, at least in the short run. Although the projections here do not assume effective or improved governance, a worsening of governance and conflict can slow the projected growth (Mason-D'Croz et al., 2019).
The focus of our GHG emissions analysis is on emissions in agriculture consistent with a sustainable agriculture intensification trajectory for closing the investment gap. It does not focus on a food systems trajectory, including changes in cold storage and diets, emissions from transportation, downstream processing of food, the manufacture of tractors and fertilizer, expansion of solar power use in agriculture, or other relevant inputs. Emissions from these sources are included by IPCC guidelines in other, non-agricultural sectors such as transport and energy (Mbow et al., 2019). Land-use change and deforestation emissions driven by agriculture were accounted for to the extent that they are generated by the investments analyzed in this article.
Analysis of potential food security improvements and GHG emissions reductions from improvement in agricultural value chains would be a particularly important extension of this analysis. Innovations and investments in the value chain would improve the prospects for meeting the SDG2 and Paris Agreement targets. Infrastructure investments, including in rural roads, electricity cell phone towers, markets, cold chains, and processing facilities have important impacts on input and output markets, reduce marketing margins and post-harvest losses of food, thereby generating production and income gains and potentially significantly reducing hunger and reducing GHG emissions (Rosegrant et al., 2021a). Innovations and investments in the value chain can make the outputs of agricultural R&D investments more profitable for farmers and generate higher social returns to agricultural R&D investments. Expanded investments in these items will likely require partnerships with the private sector. Aggregating mechanisms could be put in place, for example, through cooperatives that can help ensure that economies of scale for inspection, packaging, food safety regimes and quality management are achieved competitively. Such cooperatives can also lower costs for agricultural inputs such as seeds and chemicals and support microfinance services (Otsuka et al., 2016). Farmers need timely and reliable information about markets. In addition to information on prices, a whole range of business-related information is essential, such as who the buyers are and what their terms and conditions for doing business are. Digital information systems linked to farmer mobile phones can increase access to timely information, improve links between farmers and processors, and reduce transport costs, thereby reducing post-harvest losses (USAID, 2018). In addition to market information services, advanced digital technologies—such as satellite imaging, remote sensing, and in-field sensors—can support precision farming based on observation of, and response to, intra-field variations that guide the efficient application of inputs and improve productivity and farm income (Aker et al., 2016).
In most analyses of technical options for mitigation that we draw upon here, some of the options have low or even negative costs in specific regions. This occurs when the net revenues associated with an option are positive, indicating that the practice would be profitable even in the absence of mitigation incentives such as carbon payments or targeted subsidies (Beach et al., 2015). It is therefore necessary to also address potential barriers to be overcome to achieve adoption options that have low or negative costs, and that also hinder adoption of higher-cost options even with mitigation incentives. These barriers may include institutional problems, lack of property rights, regulatory and legal issues, farmer risk aversion, and market failures (Beach et al., 2015). Giller et al. (2009), for example, point to farm-level constraints to the adoption of conservation tillage for soil sequestration in Africa. These can include lower yields in some cases, increased labor and fertilizer requirements when herbicides are not used, limited access to external inputs, and a lack of mulch due to both low productivity and the priority given to feeding livestock with crop residues.
Constraints can also arise in the implementation of carbon sequestration programs (Pannell, 2021). With successful soil sequestration, soil carbon increases to a new equilibrium level after about 20–30 years and then stops. However, the soil sequestration methods need to be continued to avoid releasing the sequestered carbon. Costs continue without new benefits. Sequestration programs also need to ensure additionality, so that management options are additional to what farmers would do anyway. Monitoring and measuring soil carbon stored in soils is expensive, requiring regular soil testing to determine that carbon has been sequestered (Pannell, 2021). Measurement is costlier when it needs to be done for multiple small farms. Innovations in measurement through advances in information and communications technologies, including less-expensive soil testing and remote sensing, could help reduce the costs. Managing carbon sequestration for groups of farmers rather than individual farmers could also be more cost-effective.
This article estimated the investment gap in research and innovation for sustainable agriculture intensification in the Global South. Agricultural R&D investments of USD 4 billion per year have the potential to nearly end hunger by 2030. Another USD 6.5 billion per year, invested in technical climate-smart options, can achieve 2030 GHG emission reductions consistent with the Paris Agreement 2◦C and 1.5◦C pathways. Therefore, the estimated innovation investment gap to reduce hunger to 5% globally and reduce GHG emissions to a level consistent with the Paris pathways is USD 10.5 billion annually.
The USD 4 billion of additional yearly R&D investments incorporates international public R&D by CGIAR, national R&D by NARS, advances in research efficiency, and private agricultural R&D, which together reduce the risk of hunger below the targeted 5% of the population in EAP, SAS, and LAC—an impressive achievement in the short time remaining until 2030. These investments in SSA, however, fail to achieve the SDG2.1 target by 2030 where the share at risk of hunger is still projected to be nearly 12%, though this is an important improvement over baseline levels.
The agricultural productivity growth generated, along with the adoption of technical mitigation options, achieves non-CO2 GHG emissions savings of 1,010 MtCO2eq/year in 2030, a reduction in line with agriculture's contribution to a 2◦C climate pathway. Technical options and avoided land-use change also achieve ample CO2 emissions reduction and sequestration, totaling 1,200 MtCO2eq/year in 2030—far higher than the estimated 100 MtCO2eq/year needed to support a 2◦C climate trajectory. These investments do not achieve zero land-use change from agriculture but do reduce the rate of deforestation by an average of 925,000 ha/year by 2030.
Along with achieving global goals, the investment scenarios generate large economic returns. The R&D investment adds USD 1.7 trillion to the GDP of the Global South in 2030, and USD 9.1 trillion in 2050. In these countries, investment raises per capita income by 2% in 2030 and nearly 6% in 2050 relative to business as usual. The highest agricultural R&D investment scenario reduces food commodity prices by 16% globally in 2030.
These results show that increased investment in innovation could have powerful impacts on key sustainable development and climate goals between now and 2030, with the potential to bring us within reach of ending hunger in many parts of the world, achieve globally significant reductions in GHG emissions, and generate strong economic benefits for the Global South. Improvements in supporting policies and investments would further enhance the impact of the investments and improve the prospects for meeting global goals in 2030 and beyond. These enabling conditions include improved value chains, finance, extension, gender-responsive policies and investments, social protection, water management, implementation of carbon payments and smart subsidies, and agroecological and landscape approaches.
In addition to reforms and investments in these enabling conditions, the results suggest that more transformational policies and investments are needed to reverse deforestation and boost carbon sequestration and mitigation, especially beyond 2030. Greater targeting of agricultural R&D on the development of climate-smart varieties and breeds, and on lower-cost climate-smart farming systems and practices, could change the relative prices, costs, and benefits of different interventions. This, in turn, could substantially improve climate mitigation by making the adoption of climate-smart technology cheaper. If the targeted funding is taken from the existing or projected investment portfolio, careful monitoring and assessment of the impact of such a reallocation are needed to determine if there is a trade-off with the food security target—for example, if newly developed climate-smart technology reduces yields and farm profitability. Evaluation of alternative investment portfolios with prospective transformational technologies and policies would provide additional insights into the future of sustainable agriculture intensification.
Modeling assumptions and inputs are available at https://github.com/IFPRI/IMPACT. All other data necessary for this analysis are presented directly in the article.
MR conceived the approach to the research and led all aspects. TS and KW contributed to the modeling efforts and write-up of the manuscript. All authors have approved the final manuscript.
The research was co-financed by the UK's Foreign, Commonwealth & Development Office (FCDO) and CoSAI. This research was also supported by Funders contributing to the CGIAR Trust Fund (https://www.cgiar.org/funders/). The Commission on Sustainable Agriculture Intensification (CoSAI) was initiated and is supported by the CGIAR Research Program on Water, Land and Ecosystems (WLE), funded by the CGIAR Trust Fund and other donors. CoSAI Commissioners are independent. CoSAI is facilitated by a Secretariat based at the International Water Management Institute (IWMI) headquarters in Colombo, Sri Lanka.
This article is based on a working paper (Rosegrant et al., 2021b) hosted on the CoSAI website and https://cgspace.cgiar.org/. The research was guided by an Oversight Group, chaired by Donald Menzies (FCDO) and involving Rachel Lambert, Anna De Palma, both from FCDO; Julia Compton, Ruben Echeverria, Rodomiro Octavio, Uma Lele and Vara Prasad, all from CoSAI; Dhanush Dinesh from CCAFS; and Jim Jones, from the University of Florida. Study management and administration were provided by David Shearer and Josefina Achaval-Torre from the CoSAI Secretariat.
The authors declare that the research was conducted in the absence of any commercial or financial relationships that could be construed as a potential conflict of interest.
All claims expressed in this article are solely those of the authors and do not necessarily represent those of their affiliated organizations, or those of the publisher, the editors and the reviewers. Any product that may be evaluated in this article, or claim that may be made by its manufacturer, is not guaranteed or endorsed by the publisher.
Aker, J. C., Ghosh, I., and Burrell, J. (2016). “The promise (and pitfalls) of ICT for agriculture initiatives.” Agric. Econ. 47, 35–48. doi: 10.1111/agec.12301
Alston, J. M., Andersen, M. A., James, J. S., and Pardey, P. G. (2011). The economic returns to U.S. public agricultural research. Am. J. Agric. Econ. 93, 1257–1277. doi: 10.1093/ajae/aar044
Baldos, U. L. C., Fuglie, K. O., and Hertel, T. W. (2020). The research cost of adapting agriculture to climate change: a global analysis to 2050. Agric. Econ. 51, 207–220. doi: 10.1111/agec.12550
Beach, R. H., Creason, J., Ohrel, S. B., Ragnauth, S., Ogle, S., Li, C., et al. (2015). Global mitigation potential and costs of reducing agricultural non-CO2 greenhouse gas emissions through 2030. J. Integr. Environ. Sci. 12, 87–105. doi: 10.1080/1943815X.2015.1110183
Bentsen, M., Bethke, I., Debernard, J. B., Iversen, T., Kirkevåg, A., Seland, Ø., et al. (2013). The Norwegian earth system model, NorESM1-M – part 1: description and basic evaluation of the physical climate. Geosci. Model Dev. 6, 687–720. doi: 10.5194/gmd-6-687-2013
Brown, S., Sathaye, J., Cannell, M., and Kauppi, P. E. (1996). Mitigation of carbon emissions to the atmosphere by forest management. Commonw. For. Rev. 75, 80–91.
Caballero, B. (2007). The global epidemic of obesity: an overview. Epidemiol. Rev. 29, 15. doi: 10.1093/epirev/mxm012
CGIAR (2022). CGIAR Funding. Available online at: https://www.cgiar.org/funders/ (accessed May 15, 2022).
Crippa, M., Solazzo, E., Guizzardi, D., Monforti-Ferrario, F., Tubiello, F., and Leip, A. (2021). Food systems are responsible for a third of global anthropogenic GHG emissions. Nature Food 2, 198–209. doi: 10.1038/s43016-021-00225-9
Dalberg (2021). Investment Landscape for Transforming Agricultural Innovation Systems for People, Nature and Climate. Hong Kong, China: Dalberg.
Del Grosso, S. J., and Cavigelli, M. J. (2012). Climate stabilization wedges revisited: can agricultural production and greenhouse gas reduction goals be accomplished? Front. Ecol. Environ. 10, 571–578. doi: 10.1890/120058
Dufresne, J.-L., Foujols, M.-A., Denvil, S., Caubel, A., Marti, O., Aumont, O., et al. (2013). Climate change projections using the IPSL-CM5 Earth System Model: from CMIP3 to CMIP5. Clim. Dyn. 40, 2123–2165. doi: 10.1007/s00382-012-1636-1
Dunne, J. P., John, J. G., Adcroft, A. J., Griffies, S. M., Hallberg, R. W., Shevliakova, E., et al. (2012). GFDL's ESM2 global coupled climate-carbon earth system models. Part I: physical formulation and baseline simulation characteristics. J. Clim. 25, 6646–6665. doi: 10.1175/JCLI-D-11-00560.1
EPA (2019). Global Non-CO2 Greenhouse Gas Emission Projections and Mitigation 2015–2030. Washington, DC, United States: United States Environmental Protection Agency (EPA).
Evenson, R. E., and Gollin, D. (2003). Assessing the impact of the Green Revolution, 1960 to 2000. Science 300, 758–762. doi: 10.1126/science.1078710
Falck Zepeda, J., Yorobe, J. Jr, Amir Husin, B., Manalo, A., Lokollo, E., Ramon, G., et al. (2012). Estimates and implications of the costs of compliance with biosafety regulations in developing countries. GM Crops and Food: Biotechnology in Agriculture and the Food Chain 1, 1–8 doi: 10.4161/gmcr.18727
Fan, S., Headey, D., Laborde, D., Mason-D'Croz, D., Rue, C., Sulser, T. B., et al. (2018). Quantifying the Cost and Benefits of Ending Hunger and Undernutrition: Examining the Differences among Alternative Approaches. Washington, DC, United States: IFPRI.
Fan, S., Yosef, S., and Pandya-Lorch, R. (2019). “The way forward for nutrition-driven agriculture,” in Agriculture for Improved Nutrition: Seizing the Momentum, eds S. Fan, S. Yosef, and R. Pandya-Lorch (Wallingford, United Kingdom: IFPRI and CABI), 209–214. doi: 10.1079/9781786399311.0209
FAO (2008). FAO Methodology for the Measurement of Food Deprivation: Updating the Minimum Dietary Energy Requirements. Rome, Italy: FAO Statistics Division.
FAO (2021a). FAOSTAT Database of Greenhouse Gas Emissions From Agriculture. Available online at: http://www.fao.org/faostat/en/#data/GT (accessed July 7, 2021).
FAO (2021b). FAOSTAT Food Security Database. Available online at: http://www.fao.org/faostat/en/#data/FS (accessed July 10, 2021).
FAO International Fund for Agricultural Development (IFAD), and World Food Programme (WFP). (2015). Achieving Zero Hunger: The Critical Role of Investments in Social Protection and Agriculture. Rome, Italy: FAO.
Fischer, G., Shah, M., Tubiello, F. N., and van Velhuizen, H. (2005). Socio-economic and climate change impacts on agriculture: an integrated assessment. Philos. Trans. Royal Soc. B 360, 2067–2083. doi: 10.1098/rstb.2005.1744
Frank, S., Beach, R., Havlík, P., Valin, H., Herrero, M., Mosnier, A., et al. (2018). Structural change as a key component for agricultural non-CO2 mitigation efforts. Nat. Commun. 9, 1060. doi: 10.1038/s41467-018-03489-1
Fuglie, K. (2016). The growing role of the private sector in agricultural research and development world-wide. Glob. Food Sec. 10, 29–38. doi: 10.1016/j.gfs.2016.07.005
Giller, K. E., Witter, E., Corbeels, M., and Tittonell, P. (2009). Conservation agriculture and smallholder farming in Africa: the heretics' view. Field Crops Res. 114, 23–34. doi: 10.1016/j.fcr.2009.06.017
Godfray, H. C. J., Beddington, J. R., Crute, I. R., Haddad, L., Lawrence, D., Muir, J. F., et al. (2010). Food security: the challenge of feeding 9 billion people. Science 327, 812–818. doi: 10.1126/science.1185383
Havlík, P., Valin, H., Herrero, M., Obersteiner, M., Schmid, E., Rufino, M. C., et al. (2014). Climate change mitigation through livestock system transitions. Proc. Natl. Acad. Sci. USA. 111, 3709–3714. doi: 10.1073/pnas.1308044111
Hawkes, C., Harris, J., and Gillespie, S. (2017). “Changing diets: urbanization and the nutrition transition,” in 2017 Global Food Policy Report, eds S. Fan, J. Falik, R. Pandya-Lorch, K. Park, P. Stedman-Edwards, K. von Grebmer, S. Yosef, and L. Zseleczky (Washington, DC, United States: IFPRI), 34–41.
Herrero, M., Henderson, B., Havlík, P., Thornton, P. K., Conant, R. T., Smith, P., et al. (2016). Greenhouse gas mitigation potentials in the livestock sector. Nat. Clim. Chang. 6, 452–461. doi: 10.1038/nclimate2925
IPCC (2006). “Emissions from livestock and manure management,” in 2006 IPCC Guidelines for National Greenhouse Gas Inventories Volume 4: Agriculture, Forestry, And Other Land Use, eds S. Eggleston, L. Buendia, K. Miwa, T. Ngara, and K. Tanabe (Cambridge, United Kingdom: Cambridge University Press), Chapter 10.
IPCC (2014). Climate Change 2014: Synthesis Report. Contribution of Working Groups I, II and III to the Fifth Assessment Report of the Intergovernmental Panel on Climate Change. Geneva, Switzerland: Intergovernmental Panel on Climate Change (IPCC).
IPCC (2018). “Summary for policymakers,” in: Global Warming of 1.5◦C. An IPCC Special Report on the Impacts of Global Warming of 1.5◦C Above Pre-industrial Levels and Related Global Greenhouse Gas Emission Pathways, in the Context of Strengthening the Global Response to the Threat of Climate Change, Sustainable Development, and Efforts to Eradicate Poverty, eds V. Masson-Delmotte, P. Zhai, H.-O. Pörtner, D. Roberts, J. Skea, P. R. Shukla, A. Pirani, W. Moufouma-Okia, C. Péan, R. Pidcock, S. Connors, J. B. R. Matthews, Y. Chen, X. Zhou, M. I. Gomis, E. Lonnoy, T. Maycock, M. Tignor, and T. Waterfield (Geneva, Switzerland: IPCC).
Iversen, T., Bentsen, M., Bethke, I., Debernard, J. B., Kirkevåg, A., Seland, Ø., et al. (2013). The Norwegian earth system model, NorESM1-M – Part 2: Climate response and scenario projections. Geosci. Model Dev. 6, 389–415. doi: 10.5194/gmd-6-389-2013
Jones, C. D., Hughes, J. K., Bellouin, N., Hardiman, S. C., Jones, G. S., Knight, J., et al. (2011). The HadGEM2-ES implementation of CMIP5 centennial simulations. Geosci. Model Dev. 4, 543–570. doi: 10.5194/gmd-4-543-2011
Jones, J., Hoogenboom, G., Porter, C., Boote, K., Batchelor, W., Hunt, L., et al. (2003). The DSSAT Cropping System Model. Eur. J. Agron. 18, 235–265. doi: 10.1016/S1161-0301(02)00107-7
Kearney, J. (2010). Food consumption trends and drivers. Philos. Trans. Royal Soc. B Biol. Sci. 365, 2793–2807. doi: 10.1098/rstb.2010.0149
Kissinger, G., Herold, M., and De Sy, V. (2012). Drivers of Deforestation and Forest Degradation: A Synthesis Report for REDD+ Policymakers. Vancouver, Canada: Lexeme Consulting.
Laborde, D., Bizikova, L., Lallemant, T., and Smaller, C. (2016). Ending Hunger: What Would It Cost? Winnipeg, Canada: International Institute for Sustainable Development (IISD).
Laborde, D., Parent, M., and Smaller, C. (2020). Ending Hunger, Increasing Incomes, and Protecting the Climate: What would it cost donors? Winnipeg, Canada: IISD and IFPRI.
Lenaerts, B., de Mey, Y., and Demont, M. (2018). Global impact of accelerated plant breeding: evidence from a meta-analysis on rice breeding. PLoS ONE 13, e0199016. doi: 10.1371/journal.pone.0199016
Mason-D'Croz, D., Sulser, T. B., Wiebe, K., Rosegrant, M. W., Lowder, S. K., Nin-Pratt, A., et al. (2019). Agricultural investments and hunger in Africa modeling potential contributions to SDG2 – Zero Hunger. World Dev. 116, 38–53. doi: 10.1016/j.worlddev.2018.12.006
Mbow, C., Rosenzweig, C., Barioni, L. G., Benton, T. G., Herrero, M., Krishnapillai, M., et al. (2019). “Food security,” in Climate Change and Land: an IPCC Special Report on Climate Change, Desertification, Land Degradation, Sustainable Land Management, Food Security, and Greenhouse Gas Fluxes in Terrestrial Ecosystems, eds. P. R. Shukla, J. Skea, E. Calvo Buendia, V. Masson-Delmotte, H.-O. Pörtner, D. C. Roberts, P. Zhai, R. Slade, S. Connors, R. van Diemen, M. Ferrat, E. Haughey, S. Luz, S. Neogi, M. Pathak, J. Petzold, J. Portugal Pereira, P. Vyas, E. Huntley, K. Kissick, M. Belkacemi, and J. Malley (Geneva, Switzerland: IPCC), Chapter 5.
McKinsey and Company (2020). Agriculture and Climate Change: Reducing Emissions Through Improved Farming Practices. New York, United States: McKinsey and Company.
Nelson, G. C., Rosegrant, M. W., Palazzo, A., Gray, I., Ingersoll, C., Robertson, R., et al (2010). Food Security, Farming, and Climate Change to 2050. Washington, DC, United States: IFPRI. Available online at: http://www.ifpri.org/sites/default/files/publications/rr172.pdf
Nin-Pratt, A. (2016). “Inputs, productivity and agricultural growth in sub-Saharan Africa,” in Productivity and Efficiency Analysis, eds W. H. Greene, L. Khalaf, R. C. Sickles, M. Veall, and M. C. Voia (Berlin, Germany: Springer), 175–201. doi: 10.1007/978-3-319-23228-7_11
Nin-Pratt, A., Falconir, C., Ludena, C., and Martel, P. (2015). Productivity and the Performance of Agriculture in Latin America and the Caribbean: From the Lost Decade to the Commodity Boom. Washington, DC, United States: Inter-American Development Bank (IDB). Available online at: http://cdm15738.contentdm.oclc.org/cdm/ref/collection/p15738coll5/id/5105
O'Neill, B. C., Kriegler, E., Ebi, K. L., Kemp-Benedict, E., Riahi, K., Rothman, D. S., et al. (2015). The roads ahead: narratives for shared socioeconomic pathways describing world futures in the 21st century. Glob. Environ. Chang. 42, 169–180. doi: 10.1016/j.gloenvcha.2015.01.004
O'Neill, B. C., Kriegler, E., Riahi, K., Ebi, K. L., Hallegatte, S., Carter, T. R., et al. (2014). A new scenario framework for climate change research: the concept of shared socioeconomic pathways. Clim. Chang. 122, 387–400. doi: 10.1007/s10584-013-0905-2
Otsuka, K., Nakano, Y., and Takahashi, K. (2016). “Contract farming in developed and developing countries.” Ann. Rev. Res. Econ. 8, 353–376. doi: 10.1146/annurev-resource-100815-095459
Pannell, D. (2021). Soil Carbon is a Highly Flawed Climate Policy, Parts 1–3. Available online at: https://www.pannelldiscussions.net/2021/04/346-soil-carbon-1/ (accessed on July 30, 2021).
Pardey, P., Beintema, N., Steven, D., and Wood, S. (2006). Agricultural Research: A Growing Global Divide? Washington, DC, United States: IFPRI.
Pourkheirandish, M., Golicz, A. A., Bhalla, P., and Singh, M. B. (2020). Global role of crop genomics in the face of climate change. Front. Plant Sci. 11, 922. doi: 10.3389/fpls.2020.00922
Prager, S. D., and Wiebe, K. (2021). Strategic foresight for agriculture: past ghosts, present challenges, and future opportunities. Glob. Food Secur. 28, 100489. doi: 10.1016/j.gfs.2020.100489
Reisinger, A., Havlík, P., Riahi, K., van Vliet, O., Obersteiner, M., and Herrero, M. (2013). Implications of alternative metrics for global mitigation costs and greenhouse gas emissions from agriculture. Clim. Chang. 117, 677–690. doi: 10.1007/s10584-012-0593-3
Robinson, S., Mason-D'Croz, D., Islam, S., Sulser, T. B., Robertson, R., Zhu, T., et al (2015). The International Model for Policy Analysis of Agricultural Commodities and Trade (IMPACT): Model Description, Version 3. Washington, DC: IFPRI. Available online at: http://ebrary.ifpri.org/cdm/ref/collection/p15738coll2/id/129825 doi: 10.2139/ssrn.2741234
Rogelj, J., Shindell, D., Jiang, K., Fifita, S., Forster, P., Ginzburg, V., et al. (2018). “Mitigation pathways compatible with 1.5◦C in the context of sustainable development,” in Global Warming of 1.5◦C. An IPCC Special Report on the Impacts of Global Warming of 1.5◦C Above Pre-Industrial Levels and Related Global Greenhouse Gas Emission Pathways, in the Context of Strengthening the Global Response to the Threat of Climate Change, Sustainable Development, and Efforts to Eradicate Poverty. (Geneva, Switzerland: IPCC), 93–174.
Rosegrant, M. W., Fan, S., and Otsuka, K. (2021a). “Global issues in agricultural development,” in Agricultural Development: New Perspectives in a Changing World, eds K. Otsuka, and S. Fan (Washington, DC, United States: IFPRI), 35–78. doi: 10.2499/9780896293830_02
Rosegrant, M. W., Sulser, T. B., Dunston, S., Cenacchi, N., Wiebe, K., and Willenbockel, D. (2021b). Estimating the Global Investment Gap in Research and Innovation for Sustainable Agriculture Intensification in the Global South. Colombo, Sri Lanka: Commission on Sustainable Agriculture Intensification, 75.
Rosegrant, M. W., Sulser, T. B., Mason-D'Croz, D., Cenacchi, N., Nin-Pratt, A., Dunston, S., et al (2017). Quantitative Foresight Modeling to Inform the CGIAR Research Portfolio. Washington DC, United States: IFPRI. Available online at: https://www.ifpri.org/publication/foresight-modeling-agricultural-research
Ruel, M. T., Garrett, J. L., and Yosef, S. (2017). “Food security and nutrition: growing cities, new challenges,” in 2017 Global Food Policy Report, eds S. Fan, J. Falik, R. Pandya-Lorch, K. Park, P. Stedman-Edwards, K. von Grebmer, S. Yosef, and L. Zseleczky (Washington, DC, United States: IFPRI), 24–33.
Schaldach, R., Alcamo, J., Koch, J., Kölking, C., Lapola, D. M., Schüngel, J., et al. (2011). An integrated approach to modelling land-use change on continental and global scales. Environ. Model. Softw. 26, 1041–1051. doi: 10.1016/j.envsoft.2011.02.013
Smith, P., Bustamante, M., Ahammad, H., Clark, H., Dong, H., Elsiddig, E. A., et al. (2014). “Agriculture, forestry and other land use (AFOLU),” in Climate Change 2014: Mitigation of Climate Change. Contribution of Working Group III to the Fifth Assessment Report of the Intergovernmental Panel on Climate Change, eds O. Edenhofer, R. Pichs-Madruga, Y. Sokona, E. Farahani, S. Kadner, K. Seyboth, A. Adler, I. Baum, S. Brunner, P. Eickemeier, B. Kriemann, J. Savolainen, S. Schlömer, C. von Stechow, T. Zwickel, and J. C. Minx (Cambridge, United Kingdom: Cambridge University Press), 811–922.
Smith, P., Haberl, H., Popp, A., Erb, K.-H., Lauk, C., Harper, R., et al. (2013). How much land-based greenhouse gas mitigation can be achieved without compromising food security and environmental goals? Glob. Chang. Biol. 19, 2285–2302. doi: 10.1111/gcb.12160
Smith, P., Martino, D., Cai, Z., Gwary, D., Janzen, H., Kumar, P., et al. (2007). “Agriculture,” in Climate Change 2007: Mitigation. Contribution of Working Group III to the Fourth Assessment Report of the Intergovernmental Panel on Climate Change, eds B. Metz, O. R. Davidson, P. R. Bosch, R. Dave, and L. A. Meyer (New York, United States: Cambridge University Press), 498–540.
Smith, P., Martino, D., Cai, Z., Gwary, D., Janzen, H., Kumar, P., et al. (2008). Policy and technological constraints to implementation of greenhouse gas mitigation options in agriculture. Agric. Ecosyst. Environ. 118, 6–28. doi: 10.1016/j.agee.2006.06.006
Smith, P., Nkem, J., Calvin, K., Campbell, D., Cherubini, F., Grassi, G., et al. (2018). Chapter 6: Interlinkages between Desertification, Land Degradation, Food Security and GHG Fluxes: Synergies, Trade-offs and Integrated Response Options. In: Special Report on Climate Change and Land. Geneva, Switzerland: IPCC.
Sulser, T. B., Wiebe, K. D., Dunston, S., Cenacchi, N., Nin-Pratt, A., Mason-D'Croz, D., et al. (2021). Climate Change and Hunger: Estimating Costs of Adaptation in the Agrifood System. Washington, DC, United States: IFPRI. doi: 10.2499/9780896294165
Thornton, P. K. (2010). Livestock production: recent trends, future prospects. Philosophical Transactions of the Royal Society B: Biological Sciences 365, 1554. doi: 10.1098/rstb.2010.0134
UNFCCC (2020). The Paris Agreement. Available online at: https://unfccc.int/process-and-meetings/the-paris-agreement/the-paris-agreement (accessed May 15, 2022).
USAID. (2018). Digital Farmer Profiles: Reimagining Smallholder Agriculture. Washington, DC: United States Agency for International Development (USAID).
van Ittersum, M., Cassman, K. G., Grassini, P., Wolf, J., Tittonell, P., and Hochman, Z. (2013). Yield gap analysis with local to global relevance—a Review. Field Crops Res. 143, 4–17. doi: 10.1016/j.fcr.2012.09.009
van Vuuren, D. P., Stehfest, E., den Elzen, M. G. J., Kram, T., van Vliet, J., Deetman, S., et al. (2011). RCP2.6: exploring the possibility to keep global mean temperature increase below 2◦C. Clim. Chang. 109, 95–116. doi: 10.1007/s10584-011-0152-3
Waltz, E. (2018). With a free pass, CRISPR-edited plants reach market in record time. Nat. Biotechnol. 36, 6–7. doi: 10.1038/nbt0118-6b
Watanabe, S., Hajima, T., Sudo, K., Nagashima, T., Takemura, T., Okajima, H., et al. (2011). MIROC-ESM 2010: model description and basic results of CMIP5-20c3m experiments. Geosci. Model Dev. 4, 845–872. doi: 10.5194/gmd-4-845-2011
Willenbockel, D., Robinson, S., Mason-D'Croz, D., Rosegrant, M. W., Sulser, T. D. S., and Cenacchi, N. (2018). Dynamic Computable General Equilibrium Simulations in Support of Quantitative Foresight Modeling to Inform the CGIAR Research Portfolio: Linking the IMPACT and GLOBE Models. Washington, DC, United States: IFPRI. Available online at: http://ebrary.ifpri.org/cdm/ref/collection/p15738coll2/id/132757
Wise, M., Calvin, K., Kyle, P., Luckow, P., and Edmonds, J. (2014). Economic and physical modeling of land use in GCAM 3.0 and an application to agricultural productivity, land, and terrestrial carbon. Clim. Chang. Econ. 5, 145003. doi: 10.1142/S2010007814500031
Wollenberg, E., Richards, M., Smith, P., Havlík, P., Obersteiner, M., Tubiello, F. N., et al. (2016). Reducing emissions from agriculture to meet the 2◦C target. Glob. Chang. Biol. 22, 3859–3864. doi: 10.1111/gcb.13340
World Bank (2012). Managing Risk, Promoting Growth: Developing Systems for Social Protection in Africa. In the World Bank's Africa Social Protection Strategy 2012-2022. Washington, DC, United States: World Bank, 103.
Yan, X., Akiyama, H., Yagi, K., and Akimoto, H. (2009). Global estimations of the inventory and mitigation potential of methane emissions from rice cultivation conducted using the 2006 Intergovernmental Panel on Climate Change Guidelines. Glob. Biogeochem. Cyc. 23, GB2002. doi: 10.1029/2008GB003299
ZEF and FAO (2020). Investment Costs and Policy Action Opportunities for Reaching a World Without Hunger (SDG 2). Bonn, Germany: ZEF (Center for Development Research).
Keywords: agricultural research and innovation, climate change, hunger, investment, sustainable development goals (SDGs)
Citation: Rosegrant MW, Sulser TB and Wiebe K (2022) Global investment gap in agricultural research and innovation to meet Sustainable Development Goals for hunger and Paris Agreement climate change mitigation. Front. Sustain. Food Syst. 6:965767. doi: 10.3389/fsufs.2022.965767
Received: 10 June 2022; Accepted: 13 September 2022;
Published: 05 October 2022.
Edited by:
Julia Compton, Independent Researcher, Cardiff, United KingdomReviewed by:
Ruediger Schaldach, University of Kassel, GermanyCopyright © 2022 Rosegrant, Sulser and Wiebe. This is an open-access article distributed under the terms of the Creative Commons Attribution License (CC BY). The use, distribution or reproduction in other forums is permitted, provided the original author(s) and the copyright owner(s) are credited and that the original publication in this journal is cited, in accordance with accepted academic practice. No use, distribution or reproduction is permitted which does not comply with these terms.
*Correspondence: Mark W. Rosegrant, bS5yb3NlZ3JhbnRAY2dpYXIub3Jn
Disclaimer: All claims expressed in this article are solely those of the authors and do not necessarily represent those of their affiliated organizations, or those of the publisher, the editors and the reviewers. Any product that may be evaluated in this article or claim that may be made by its manufacturer is not guaranteed or endorsed by the publisher.
Research integrity at Frontiers
Learn more about the work of our research integrity team to safeguard the quality of each article we publish.