- 1Central Agricultural Research Center, Montana State University, Bozeman, MT, United States
- 2College of Agriculture and Bioresources, University of Saskatchewan, Saskatoon, SK, Canada
- 3Northern Agricultural Research Center, Montana State University, Havre, MT, United States
- 4Department of Animal and Range Sciences, Montana State University, Bozeman, MT, United States
The incorporation of cover crops into cropping systems is important for enhancing soil health in agricultural systems. Soil microbes contribute to soil health by supplying key nutrients and providing protection against plant pests, diseases, and abiotic stress. While research has demonstrated the connection between cover crops and the soil microbiology, less is known regarding the impact of cover crops on the soil microbial community in semi-arid regions of the Northern Great Plains. Our objectives were to evaluate changes in the soil bacterial community composition and community networks in wheat grown after multi-species cover crops. Cover crops were compared to continuous cropping and crop/fallow systems and the effects of cover crop termination methods were also evaluated. Cover crops consisted of a cool season multispecies mix, mid-season multispecies mix, and a warm season multispecies mix, which were grown in rotation with winter wheat. A continuous cropping (wheat/barley) and wheat/fallow system were also included along with cover crop termination by grazing, herbicide application, and haying. Cover crop treatments and termination methods had no significant impact on microbial community alpha diversity. Cover crop termination methods also had no significant impact on microbial community beta diversity. Families belonging to the phyla Actinobacteria, Bacterioidota, and Proteobacteria were more abundant in the cool season cover crop treatment compared to the warm season cover crop treatment. Co-occurrence network analysis indicated that incorporation of cool season cover crops or mid-season mixes in a wheat-based cropping system led to greater complexity and connectivity within these microbial networks compared to the other treatments which suggests these communities may be more resilient to environmental disturbances.
Introduction
The primary dryland grain production system in the Northern Great Plains, USA, is a cereal-fallow system where wheat is grown every other year and the land is left fallow alternating years (Zentner et al., 1991; Sigler et al., 2018; Bourgault et al., 2021). This has become economically untenable and has produced unintended ecological consequences including erosion, persistent weed problems, and loss of biodiversity in the soil (Lupwayi et al., 1998). Incorporating cover crops can mitigate these negative effects and increase the sustainability of agricultural systems (Strickland et al., 2019). Cover crops are used to reduce erosion and provide supplemental forage (Munawar et al., 1990; Unger and Vigil, 1998; Daryanto et al., 2018; Florence et al., 2019). Cover crops are also used to increase plant diversity which can reduce weed pressure through shading the soil surface and inhibiting weed growth (Florence et al., 2019). Cover crops can also assist to decrease plant pathogen pressure (Peralta et al., 2018). Additional benefits include improved soil physical quality, increased organic matter, soil nutrients, and microbial biomass (McDaniel et al., 2014; Adetunji et al., 2020; Haruna et al., 2020).
Microorganisms are essential for ecosystem function and can increase nutrient availability, enhance seedling vigor, improve plant resiliency and productivity, improve soil structure, and aid in pathogen suppression (Balser et al., 2006; Bardgett and van der Putten, 2014; Bonanomi et al., 2016; Banerjee et al., 2019). Studies have evaluated the impact of cover crops on soil microorganisms in different ecoregions and cropping systems, but results have been contradictory (Biederbeck et al., 2005; Calderón et al., 2016; Daryanto et al., 2018). Results from research in the Central Great Plains, USA, showed that cover crops increased microbial biomass compared to fallow but by the next season this change was no longer observed (Calderón et al., 2016). This suggests that benefits to the microbial community associated with cover crops might be short-lived. A meta-analysis of 60 studies assessed the benefit of cover crops to the soil microbiome and found a significant increase in microbial abundance, diversity, and activity when cover crop were used but factors such as termination method, climate, soil taxonomy, and tillage effected the response (Kim et al., 2020). This suggests that the effect of plant species on the microbial community is context-specific and factors such as cropping system, moisture, temperature, and soil physical and chemical parameters must also be considered (Millard and Singh, 2009).
While cover crops have demonstrated benefits in some regions, there are still questions regarding their applicability in semi-arid regions due to concerns of water usage (Bodner et al., 2007) and potential yield reductions (Rosner et al., 2018; Euteneuer et al., 2022) in the cash crop grown the following year (Calderón et al., 2016; Bourgault et al., 2021; Thapa et al., 2021). Information is lacking on the soil health benefits of individual cover crop species and mixes grown in the semi-arid Northern Great Plains and little is known regarding the performance of diverse cover crop mixes in this region. There is also little information available regarding the best practices for cover crop termination. While data are available regarding the agronomic effects of termination timing (Ghimire et al., 2018), less is known about the impact of different termination methods on the soil microbial community. Knowledge gaps exist regarding the effect of cover crops on subsequent wheat yields and the suitability of cover crop mixtures for livestock forage in the dryland wheat growing regions of northern Montana. This has caused reluctance among producers in this region to adopt cover crops as part of their management strategy (Bourgault et al., 2021).
Previously we found that in dryland wheat based cropping systems, cover crops significantly reduced wheat yields compared to fallow but yield differences were not significant between cool, mid, and warm season cover crops (Bourgault et al., 2021). The objectives of this study were to evaluate bacterial community composition and community networks response to cover crop mixes compared to conventional fallow or continuous monoculture cropping practices in the wheat phase of the rotation to determine if cover crop effects persist in the subsequent cash crop. Specifically, we tested the impacts of a winter wheat—cover crop rotation with cover crop mixtures varying in diversity and planting date (early, mid, and warm-season mixtures). Another objective was to evaluate the impact of cover crop termination methods on soil microbial community composition in dryland cropping systems in the Northern Great Plains. We hypothesized that (1) microbial communities in wheat grown after cover crops would have greater alpha and beta diversity compared to fallow and (2) cover crops would contribute to greater microbial network complexity.
Materials and methods
Study site description
This study was part of a larger long-term study evaluating cover crops in a semi-arid region of the Northern Great Plains (Bourgault et al., 2021; Wyffels et al., 2021; DuPre et al., 2022). The study was established in April, 2012 on two adjacent fields at the Northern Agricultural Research Center of Montana State University, Havre, MT, USA, located 48.49689 N, 109.8029 W and 800 m above sea level. Average annual low and high temperatures at the study location were 0°C and 13.4°C, respectively, with an average annual precipitation of 305 mm1. The soil underlying the study area is a mix of Joplin and Telstad clay loam (Fine-loamy, mixed, superactive, frigid Aridic Argiustolls)2. Additional soil parameters are presented in Supplementary Table S1. The soils are relatively deep (>150 cm) with water holding capacities of over 18 centimeters (USDA, 1999). Monthly maximum and minimum temperatures and precipitation, as well as long-term averages (1916-2018) are presented in Supplementary Table S2.
Experimental design
The experiment was designed to evaluate a wheat cover crop rotation with different cover crop mixtures in rotation with winter and spring wheat (Triticum aestivum L.), and terminated with herbicides, grazing, or haying. The experiment was established as two adjacent replicated trials, one for each phase of the rotation, and designed as a restricted-randomization strip-plot with three replicates with termination treatments assigned perpendicular to the fallow and cover crop treatments (Figure 1). This study was conducted in the winter wheat phase of the rotation during the 5th year of the trial. Additional details of the field trials, including experimental design, crop management, treatments, and field sampling procedures, are described in Bourgault et al. (2021), Wyffels et al. (2021), and DuPre et al. (2022). Planting was done with a ConservaPak hoe-type air seeder with 30 cm row spacing at a depth of 2.54 cm for both cover crops and wheat crops. Plots were fertilized with 112 Kg ha−1 of 20:20:20 (N:P:K) at planting. Fields used in this study were managed using no-till practices. Treatments included 3 cover crop mixes, barley, and fallow (Table 1). Barley was included since, after wheat, it is the second most common grain crop in this region (USDA, 2020) and fallow was also included as a treatment since it is still a common producer practice in the region. The cover crops were broadly divided into three groups: cool season crops, warm season crops, and a mixture of cool- and warm season crops. These divisions are based on timing of planting (Bourgault et al., 2021; DuPre et al., 2021). Cool season cover crops were planted 23 April 2016, mid season on 5 May 2016, and warm season on 19 May 2016, which was ~3.5 weeks later than the cool season cover crop mix. Plots were terminated by haying between 23 June and 1 July. Chemical termination (glyphosate, applied at 2,500 g ai per hectare) was applied on 23 June and grazing was performed beginning 23 July. An additional application of herbicide (2,000 g of ai glyphosate per hectare plus 340 g of ai dicamba per hectare) was applied to terminate any regrowth of cover crops and weeds in the grazed and hayed termination treatments ~4 weeks after the initial termination and prior to planting the subsequent wheat crop. Winter wheat was planted on 1 October 2016.
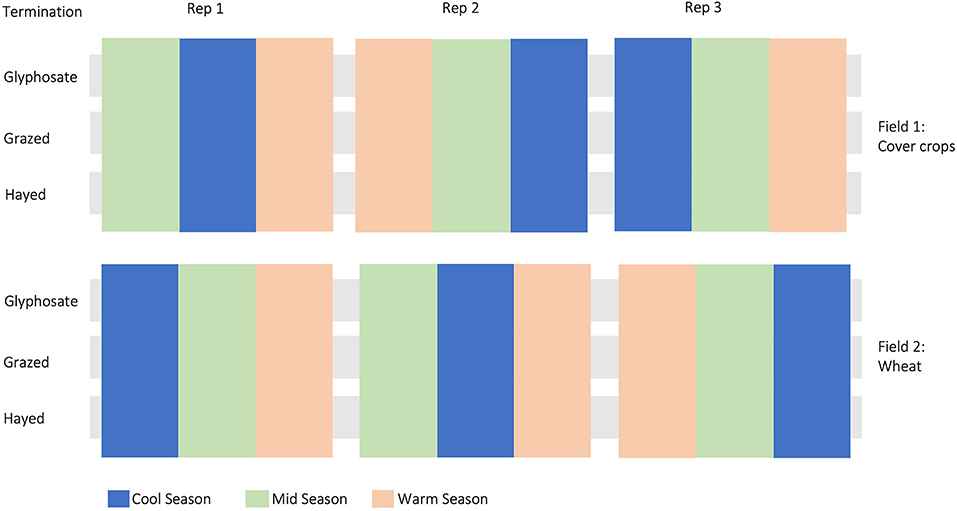
Figure 1. Study design showing randomized treatments (colored bars) and cover crop termination treatments (gray strips). Cover crops and wheat were rotated each year between field 1 and 2. Plot dimensions were 7.3 m x 40.2 m with a 3.6 m wide buffer strip between each plot. Plots are not drawn to scale.
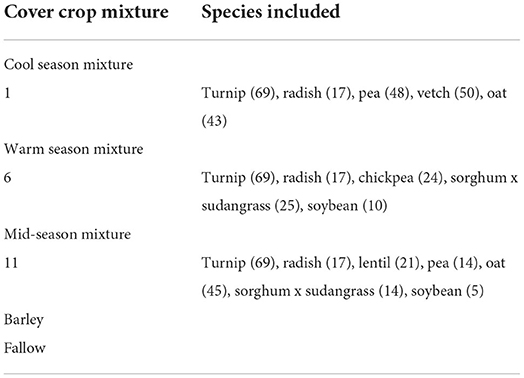
Table 1. List of species in each of treatments evaluated in the cover crop trial. Seeding rates (seeds m−2) are shown in parentheses behind each species.
Bulk soil samples (0–30 cm) were collected from the wheat phase of the study for microbial analysis on 1 August 2017. Five 10 cm depth x 3.54 cm diameter soil cores were collected at random from each plot and homogenized to make one composite sample per plot. Samples were placed in coolers on ice in the field then transported to the lab and stored at −80°C until extraction.
Sample handling and sequencing
For each plot, DNA was extracted from a 250 mg soil subsample using the Qiagen DNeasy PowerSoil DNA Isolation Kit (Qiagen Inc., Germantown, MD, USA). Variable region 4 of the 16S rRNA gene was amplified in triplicate from each DNA extract by PCR using the Platinum HotStart PCR Kit (ThermoFisher Scientific, Waltham, MA) with 10 μl PCR Mastermix, 13 μl molecular-grade water, 0.5 μl each of forward and reverse primers reconstituted at 10 mM concentration, and 1 μl sample DNA. PCR primers were as previously described for 515F and 806R (Caporaso et al., 2011) with Illumina's 5' and 3' adapters, respectively and Golay barcode, primer pads and linkers as described by Caporaso et al. (2012). A unique barcode was assigned to each sample plot. PCR reactions comprised an initial 94°C denaturation for 3 min, followed by 35 cycles of denaturation at 94°C for 45 s, annealing at 50°C for 60 s, elongation at 72°C for 90 s. Amplicons were pooled and purified by agarose gel electrophoresis and then sequenced on an Illumina MiSeq platform (Illumina, San Diego, CA, USA) using an Illumina MiSeq 500 cycle v2 kit. Demultiplexing and trimming of barcodes and adaptors was performed in Illumina MiSeq Reporter software (version 2.5.1) prior to analysis.
Bioinformatics
Sequence reads were processed using the QIIME2 bioinformatics pipeline (Bolyen et al., 2019). After quality filtering, three samples with low total reads (< 5000 sequences) were removed. Quality-filtered paired end reads were assembled into error-corrected amplicon sequence variants (ASVs) using DADA2 (Callahan et al., 2016). Taxonomic assignment was performed using a naive Bayes classifier pre-trained on the Silva 132, database (Quast et al., 2013) with a 97% identity threshold. Alpha (Chao1 and Shannon diversity) analysis was performed using the q2-diversity plugin. Linear regression with a mixed effect model was used to explore the relationships between treatments and alpha diversity using the R package lme4 (Bates et al., 2015). Cover crop treatment, termination method, and interactions among them were included as fixed effects with replication as a random effect in the mixed effects model. Tukey's post-hoc comparison was used to evaluate differences in means between treatments.
Differences in community composition based on cover crop treatment and termination were visualized using unconstrained principal coordinate analysis (PCoA) of the weighted unifrac distances. The betadisper function from the vegan R package (Oksanen et al., 2018) was used to assess the assumption of homogenous dispersion for performing a permutational analysis of variance (PERMANOVA), which was met. Permutational multivariate analysis of variance (Permanova) was performed on the weighted unifrac distance matrices using the adonis2 function with 999 permutations from the R package vegan to test differences in overall community composition between cover crops treatments. Data were stratified by replication to account for repeated measures. Post-hoc contrasts between significant factors were examined using pairwise.adonis2 (Martinez Arbizu, 2020).
Files generated in QIIME2 were imported into R using the package Qiime2R (Bisanz, 2018) for additional analysis. Data were filtered to remove taxa occurring fewer than five times or in less in 20% of the samples. Differential abundance testing was also performed. Taxa significantly different between cover crop treatments were identified using the generalized linear models implemented in the R package DESeq2 (Love et al., 2014). DESeq2 was performed on unrarified sample libraries to determine differential abundance of taxa among treatments (Weiss et al., 2017). The model included replicates and cover crop treatments and the contrast argument of the results function was used to extract comparisons of interest after fitting the model. Differential ASVs were visualized for each cover crop treatment by Bland-Altman plots which show the mean change in abundance (M) vs. average counts (A) (MA plots) (Altman and Bland, 1983). MA plots were constructed using ggpubR (Kassambara, 2020) and plotted with a log2 fold change threshold of 2. Indicator species analysis (ISA), which is a method for identifying taxa associated with a specific environment (Bakker, 2008), was conducted using the multipatt function from the R package indicspecies (De Cáceres et al., 2010) to identify ASVs associated with specific cover crops. ISA was performed with 104 permutations and associations were considered significant at p < 0.05 (Bonferroni corrected).
Co-occurrence networks
Network analysis was performed using the SpiecEasi R package (Kurtz et al., 2015) to identify microbial co-occurrence patterns across the cover crop treatments. The Meinshausen and Bühlmann (MB) neighborhood selection framework was used with pulsar.params set to 999 replications. The nodes of the resulting network represent ASVs while edges represent correlations between pairs of ASVs. The output was transformed with the adj2igraph script to transform the matrix into an igraph object and the resulting network files were visualized in Cytoscape v3.8 using the NetworkAnalyzer tool to calculate network topology parameters (Lotia et al., 2013). Network topological parameters can be used as biological indicators of microbial community resilience to environmental disturbances and can be used to identify important taxa within microbial communities and the degree of connectedness within these communities (Williams et al., 2014; Price et al., 2020). The number of nodes indicates the number of co-occurrence relationships in each treatment while edges indicate significant (p < 0.05) correlations between taxa. Edge betweenness centrality, which is used to describe the number of shortest paths that go through an edge in a network (Girvan and Newman, 2002), was represented by edge thickness. The clustering coefficients indicated the probability that the adjacent nodes of any given node are connected (Ma et al., 2016), while the closeness centrality represents the average distance of a given node to any other node and indicates the central importance of the node (Berry and Widder, 2014; Banerjee et al., 2019).
Results
Microbial community analysis
A total of 8.2 x 106 sequences were obtained. Following quality filtering and feature table construction with DADA2, three low quality samples (< 5,000 reads) were removed from the data set. The number of sequences per sample in the remaining data set ranged from 129,059 to 298,372 with a median of 152,114. A total of 28,773 amplicon sequence variants (ASVs) were identified across all samples. The average relative abundance of six most abundant phyla across all cover crop treatments were Acidobacteria (25.0%), Proteobacteria (24.9%), Bacteroidetes (14.6%), Verrucomicrobia (8.1%), Actinobacteria (7.7%), and Nitrospirae (3.2%).
Alpha diversity, a measure of community richness, was not significantly different between any of the cover crop treatments or termination methods when compared by Chao1 richness index or Shannon's diversity index (p > 0.05) (Figure 2). Unconstrained principal coordinate analysis (PCoA) of the weighted unifrac distances showed little separation between cover crop treatments or termination methods (Supplementary Figure S1). No statistically significant differences in beta diversity, as measured by weighted unifrac distances, were found between cover crop termination methods (Table 2). Permanova pair-wise comparison of cover crop treatments showed significant differences (p = 0.0462). Pair-wise contrasts showed significant differences between the cool and warm season cover crop Treatments (p < 0.05, FDR corrected) (Table 3).
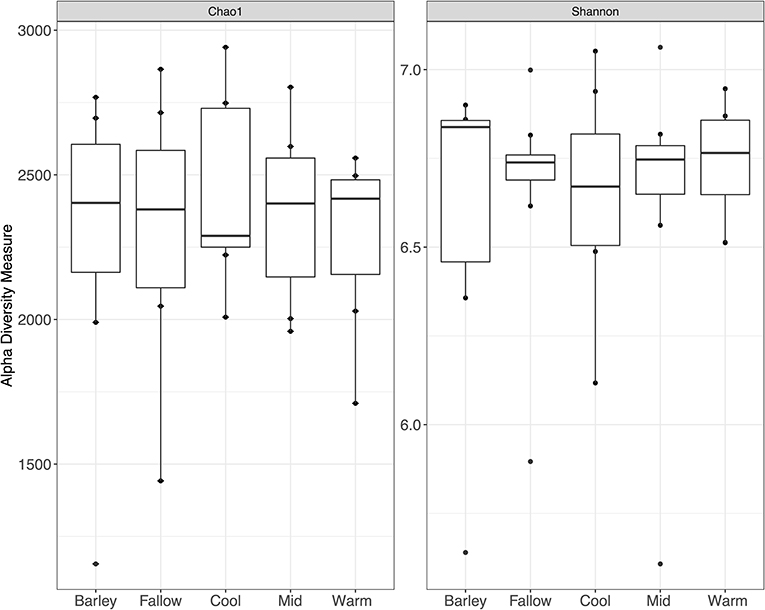
Figure 2. Chao1 and Shannon diversity indices for cover crop treatments. Mean values shown are averages across all termination methods since no significant differences were observed between termination methods. Differences in alpha diversity were also not significant between cover crop treatments.

Table 2. Pair-wise permutational analysis of variance (PERMANOVA) for multivariate (β-diversity) group significance for each cover crop termination method.
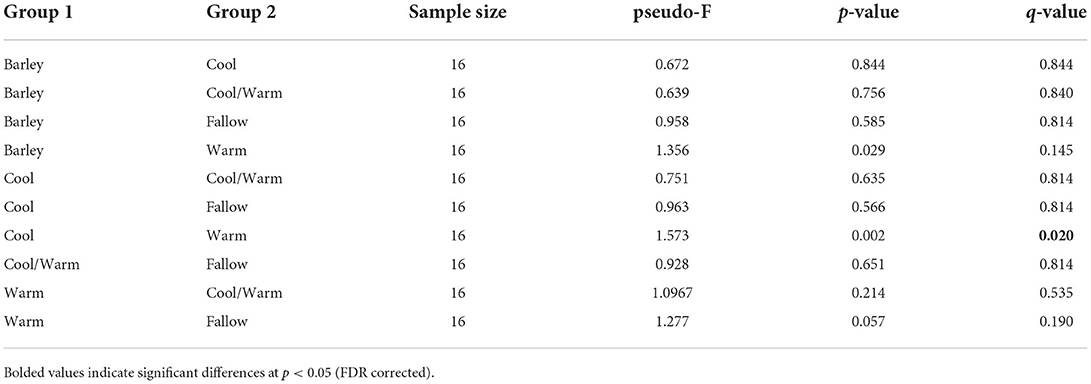
Table 3. Pair-wise permutational analysis of variance (PERMANOVA) for multivariate (β-diversity) group significance for cover crop treatments.
Differential ASVs were visualized for each cover crop treatment by Bland-Altman plots which show the mean change in abundance (M) vs. average counts (A) (MA plots) of the DESeq analysis (Supplementary Figure S2). Differentially abundant ASVs were identified at the phylum and family taxonomic ranks for each cover crop treatment combination (Supplementary Table S4) and were visualized at the phylum and family level for each treatment (Figure 3). A total of 23 ASVs were differentially abundant between cool and warm season treatments while 11 ASVs were differentially abundant between warm season cover crops and barley (Figure 3; Supplementary Tables S4, S5). The remaining treatments had 3 or fewer ASVs that were differentially abundant. Families belonging to the phyla Actinobacteria, Bacterioidota, and Proteobacteria had the greatest log2 fold change in abundance in the cool season cover crop treatment compared to the warm season cover crop treatment (Figure 3; Supplementary Table S4). In contrast, the phyla with the greatest log2 fold change in abundance in the warm season cover crop and barley comparison were all Actinobacteriota except for one undefined family belonging to the phylum Proteobacteria (Figure 3; Supplementary Table S5).
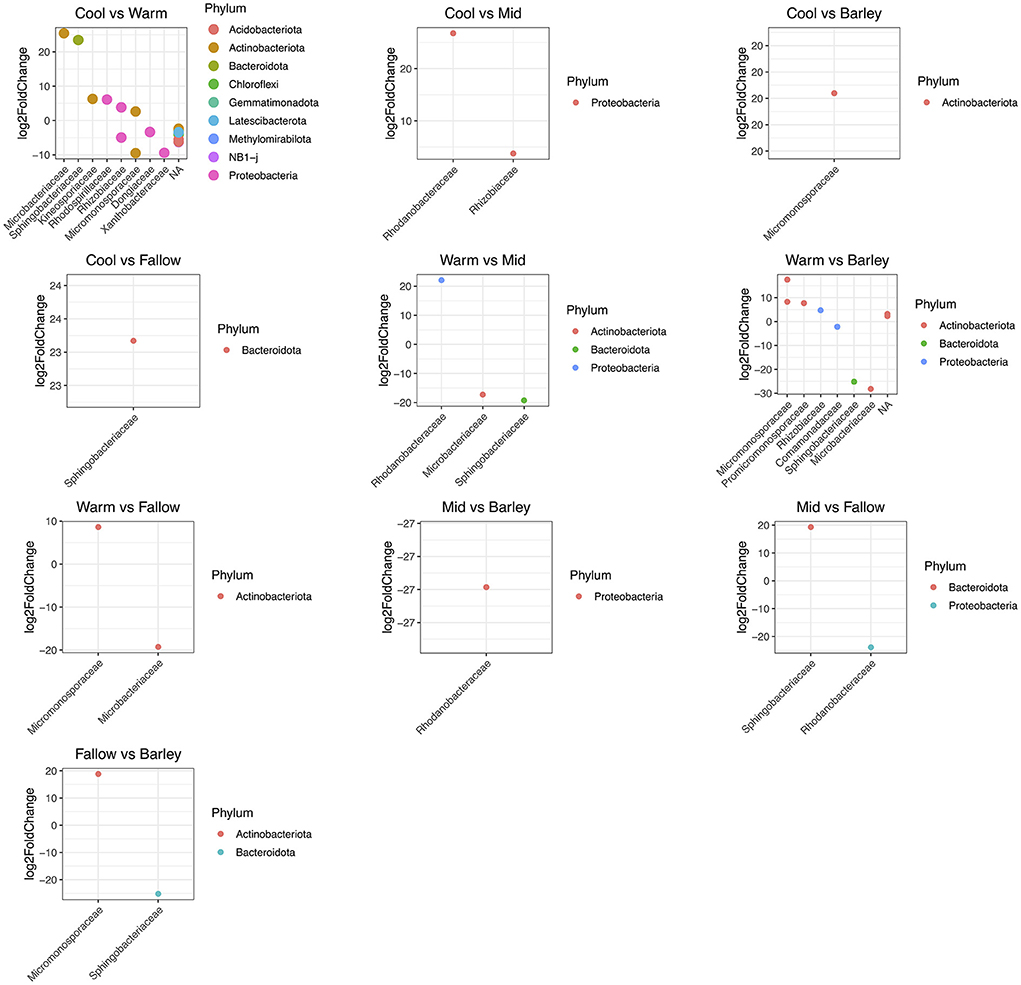
Figure 3. Plot of differentially abundant taxa. Cover crop treatment comparisons are shown above each plot. Significantly different (p < 0.05, FDR corrected) taxa are colored by phylum while the taxonomic rank at the family level is shown on the x-axis.
Indicator species analysis (ISA), which can be used as ecological indicators of community types (De Cáceres et al., 2010), identified 4 significant (p < 0.05) ASVs associated with cool season cover crops and 9 significant (p < 0.05) ASVs associated with warm season cover crops (Table 4). Significant indicator species in the cool season cover crops was from the family Cytophagaceae and represented 0.5% relative abundance (Table 4). ISA analysis identified a significant indicator species from the phylum Gemmatimonadetes which represented 0.06% relative abundance (Table 4). Relative abundance of these putative indicator taxa was not significantly different between treatment (Data not shown). In contrast to the cool and warm season cover crop mixes, there were no indicator species unique to the mid-season cover crop mix, barley, or fallow.
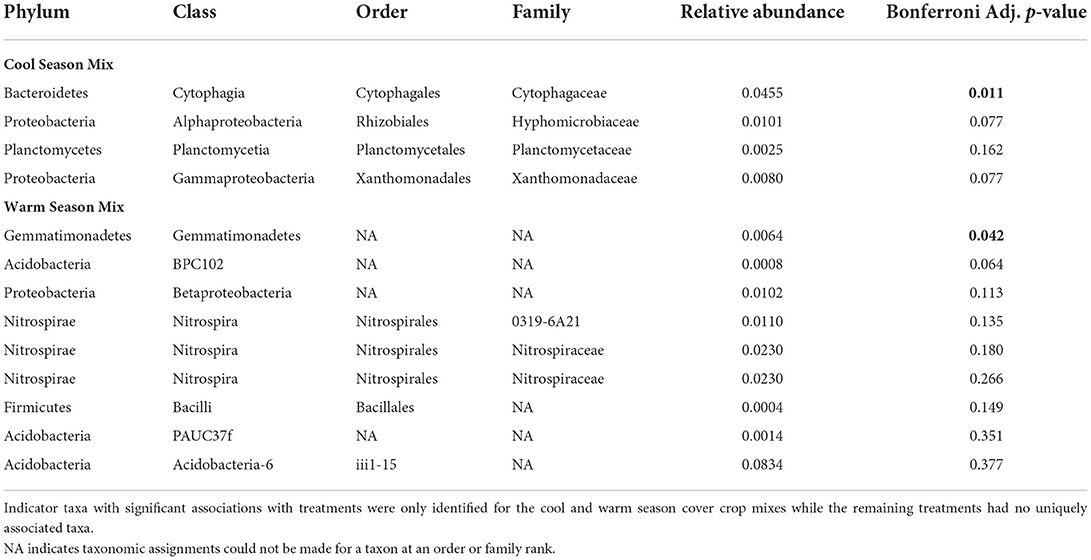
Table 4. Indicator species analysis (ISA) based on taxonomic assignment with the Silva 132 database.
Co-occurrence networks
Co-occurrence networks were generated for each cover crop mix, and barley and fallow controls (Figure 4). From these co-occurrence networks, network topological parameters were calculated (Table 5). Nodes were colored by phylum and abundance of connected taxa was represented by node diameter. Edge betweenness centrality was represented by edge thickness. The number of nodes and edges were greatest for the cool and mid-season cover crop mixes with cool season having 68.7% more edges and mid-season having over twice as many edges as fallow even though the number of nodes was similar (Table 5). Barley and fallow both had nodes that were not connected to the network (Figure 4). Average connectivity and clustering coefficients were also higher for the cool and mid-season cover crop mixes. Cool and mid-season cover crop mixes had the greatest number of edges with high betweenness centrality scores (Figures 4A,C).
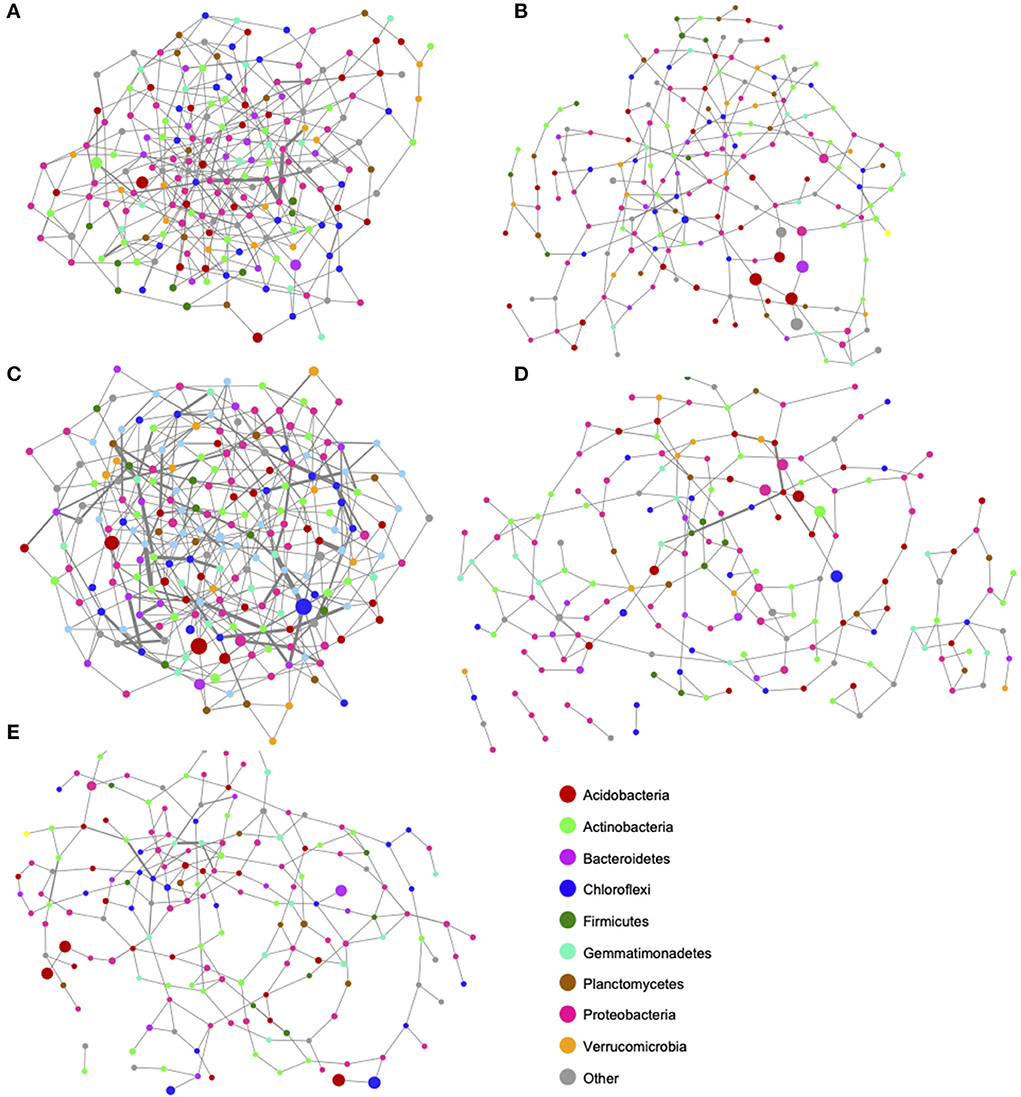
Figure 4. Co-occurrence networks of bacterial communities across five cover crop treatments and controls. (A) Cool Season, (B) Warm Season, (C) Cool/Warm Season mix, (D) Barley, and (E) Fallow. Co-occurrence networks indicate bacterial taxa that frequently occur together in a particular treatment or environment. Network nodes are colored by phylum and node size represents the number of connected taxa. Taxa with many correlations are in the most densely connected areas of the network. Edges (lines connecting nodes) represent significant (p < 0.05) positive correlations between taxa. thickness is proportional to the correlations between pairwise nodes.
Discussion
Significant differences in alpha diversity between fallow and different cover crop treatments were not observed, which is consistent with other reports. Castle et al. (2021) found that alpha diversity was not affected by cover crop treatments consisting of winter rye or pennycress, although specific bacterial taxa showed differential abundance in cropping systems containing penny cress cover crops. Permanova pair-wise comparison of cover crop treatments showed a significant difference in community composition only between cool and warm season cover crop Treatments (Table 3). In contrast, we previously found that under the cover crop phase of the wheat—cover crop rotation, cover crops had significant differences in four of the eight most abundant phyla when compared to fallow (Ouverson et al., 2022). This suggests that cover crops may have transient effects on the structure of the soil microbial community and these effects may no longer be apparent under the subsequent cash crop.
Differentially abundant taxa were observed between the cover crop treatments. Differences between cover crop mixes were primarily among taxa that had low relative abundance (< 0.1% at a family level) or were poorly characterized. This is consistent with other studies which have found small but significant differences among rarer taxa in semi-arid cropping systems in the Northern Great Plains (Ishaq et al., 2020; Ouverson et al., 2021). Because these low abundance taxa are poorly characterized, it is difficult to link bacterial community response to specific cover crop characteristics. More work is needed to functionally characterize rare community members.
Indicator species analysis (ISA) provided further evidence of limited community differences between treatments. Indicator species are species that are used as ecological indicators of specific habitats or community types (De Cáceres et al., 2010). This concept has been broadly applied in ecology (Bakker, 2008) but more recently been used to identify taxa within microbial communities that are indicative of specific ecological conditions (Sun et al., 2018; Brisson et al., 2019). Only a single indicator species was identified in cool and warm season cover crop treatments (Table 4) which suggests few differences between multispecies cover crop mixes. These results suggest that in general, the composition of soil bacterial communities is minimally altered due to the introduction of cover crop mixes in dryland wheat-based cropping systems of the Northern Great Plains.
Microorganisms do not function in isolation but form complex network associations. Network analysis has been applied to human social networks, computer networks, food webs, and ecosystems (Krause et al., 2003; Barberan et al., 2012; Williams et al., 2014). Network robustness is defined as the resilience of a network to perturbation, and more specifically in biological systems, as the ability of a community to tolerate species loss or environmental disturbances (Chan and Akoglu, 2016; Evans et al., 2016; Price et al., 2020). Studies have shown that ecological networks have similar patterns of robustness as other complex networks (Gilbert, 2009; Pocock et al., 2012). More recently these concepts have been extended to microbial communities to develop a framework for characterizing interactions between microorganisms in complex communities (Bissett et al., 2013; Berry and Widder, 2014). Recent studies have demonstrated that microbial communities with lower network complexity may be more vulnerable to environmental stress (Ludwig et al., 2018; Banerjee et al., 2019). Network complexity and associated robustness can be inherent to the system such as the rhizosphere which contains a more robust network than bulk soil (Fan et al., 2018). Network robustness can also be influenced by agricultural practices. It has also been demonstrated that agronomic practices, such as increased tillage, fertilizer, and pesticide use, can reduce microbial network complexity and result in the loss of keystone species and network complexity (Banerjee et al., 2019; Zhang et al., 2021). Cover crops in particular, have been linked to increased functional redundancy and complementarity with bacterial communities (Alahmad et al., 2018) which can further increase the resilience of these systems to stressors such as drought, nutrient limitation, and disease. Similarly, the results of our work showed greater community complexity than monoculture (barley) or fallow.
Understanding the complexity of microbial networks is important for determining how resilient the community is to perturbations (Bissett et al., 2013). The results of this work indicated that the cool season cover crop and mid-season mix had more robust networks compared to the other treatments (Table 5). Specifically, average connectivity and clustering coefficients were also higher for the cool and mid-season cover crop mixes. Cool and mid-season cover crop mixes had the greatest number of edges with high betweenness centrality scores (Figures 4A,C). An edge with a high betweenness centrality score suggests the edge is an important connection between two parts of a network and implies greater redundancy in the community (Radicchi et al., 2004; Lu and Zhang, 2013). The fact that sampling occurred during the winter wheat phase of the rotation suggests that the benefit of cover crops to greater network robustness persists and may benefit the subsequent cash crop. Collectively these results suggest that cool season and mid-season mixes contributed to greater complexity and connectivity within the microbial networks associated with these cover crops compared to fallow.
Surprisingly, the warm season mix had similar network characteristics to the barley and fallow controls even though the species diversity was similar to the mid-season cover crop mix (Table 1). In the Northern Great Plains, precipitation is erratic and extended dry periods are common (Carr et al., 2020). This has made warm season crops challenging to grow in this region as the late planting dates result in lower biomass since crops cannot take advantage of spring moisture.
Consistent with previous findings (Banerjee et al., 2019; Gao et al., 2022), our work suggests that the number of taxa within a community is less important in determining network complexity than the number of associations among taxa with more associations leading to greater resilience in the community. Our work suggests that incorporating cover crops in a cropping system can in some cases increase the network complexity which should in theory make the system more robust to environmental perturbations, however more work is needed to empirically determine the biological relevance of network components (Faust and Raes, 2012). Additional work is also needed to empirically establish links among microbial taxa and community functional response to disturbance (Allison and Martiny, 2008).
Cover crop termination method also did not significantly alter the microbial community composition. In contrast, a meta-analysis found small but significant differences in the microbial community abundance and diversity associated with cover crop termination method and concluded this was a significant moderator of cover crop effects on the soil microbiome (Kim et al., 2020). However, this study was limited to comparing chemical and mechanical termination methods where mechanical termination included undercutting or mulching (Kim et al., 2020). Soil disturbance from undercutting could contribute to some of the differences in community composition that were observed given the significant impact tillage has on the soil microbiome (Legrand et al., 2018; Ouverson et al., 2021). Another study found that cover crop termination methods (frost, rolling, and glyphosate) resulted in significant changes in microbial community structure while cover crop mixtures had a minor but significant effect (Romdhane et al., 2019). The difference in termination results may be explained in part by termination timing. In our study, all termination methods were initiated at the same time while Rhomdhane et al., performed the glyphosate termination several months after the other termination treatments (Romdhane et al., 2019).
Warm season crops are not grown extensively in this region of the Northern Great Plains (Carr et al., 2020) due in part to the low temperatures and short growing season. Given the predicted increase in temperatures and expected longer growing season, warm season crops, particularly forages are becoming a viable option (Meccage et al., 2019). While results of this study suggest some potential benefits for the soil bacterial community, more work is needed to disentangle the interaction between crop species, planting date, and climatic factors.
Conclusions
Our work showed that in the semi-arid regions of the Northern Great Plains, incorporating multispecies cover crop mixes into a winter wheat cropping system did not lead to a significant difference in the soil bacterial community abundance in the wheat crop the following year. Small but significant differences were observed in some taxa, but in general differences in community composition between treatment were minimal. Significant differences were observed in the microbial network complexity which suggests that some cover crop mixes may lead to a more robust and resilient microbial community compared to fallow and this benefit may persist to the subsequent cash crop.
Data availability statement
The datasets presented in this study can be found in online repositories. The names of the repository/repositories and accession number(s) can be found below: https://www.ncbi.nlm.nih.gov/bioproject/839381.
Author contributions
DB, JD, CY, and PL contributed to the conception and design of the study. MB, JD, PL, and SW performed data collection. JE performed data analysis and wrote the first draft of the manuscript. All authors contributed to manuscript revision, read, and approved the submitted version.
Funding
This work was supported by the Montana Wheat and Barley Committee, USDA NRCS, Montana Research and Economic Development Initiative, the Montana Agricultural Experiment Station (MONB00512), and the Montana INBRE Bioinformatics and Biostatistics core via the National Institute of General Medical Sciences of the National Institutes of Health (NIH-NIGMS; award number P20GM103474).
Acknowledgments
The authors would like to acknowledge the contributions of Northern Agricultural Research Center staff who helped with various aspects of data collection over the years. Particularly, sincere thanks are due to Roger Hybner for the technical support related to this project, Tom Allen for planting and spraying operations, and Cory Parsons and the livestock crew for putting up electrical fences and bringing cows and bulls in for grazing. Numerous short-term employees and student interns have also helped with soil sample collection, residue counts, infiltration measurements, and sample processing and cleaning are also acknowledged.
Conflict of interest
The authors declare that the research was conducted in the absence of any commercial or financial relationships that could be construed as a potential conflict of interest.
Publisher's note
All claims expressed in this article are solely those of the authors and do not necessarily represent those of their affiliated organizations, or those of the publisher, the editors and the reviewers. Any product that may be evaluated in this article, or claim that may be made by its manufacturer, is not guaranteed or endorsed by the publisher.
Supplementary material
The Supplementary Material for this article can be found online at: https://www.frontiersin.org/articles/10.3389/fsufs.2022.948220/full#supplementary-material
Footnotes
References
Adetunji, A. T., Ncube, B., Mulidzi, R., and Lewu, F. B. (2020). Management impact and benefit of cover crops on soil quality: A review. Soil Tillage Res. 204, 104717. doi: 10.1016/j.still.2020.104717
Alahmad, A., Decocq, G., Spicher, F., Kheirbeik, L., Kobaissi, A., Tetu, T., et al. (2018). Cover crops in arable lands increase functional complementarity and redundancy of bacterial communities. J. Appl. Ecol. 56, 651–664. doi: 10.1111/1365-2664.13307
Allison, S. D., and Martiny, J. B. (2008). Colloquium paper: resistance, resilience, and redundancy in microbial communities. Proc. Natl. Acad. Sci. USA. 105(Suppl 1), 11512–11519. doi: 10.1073/pnas.0801925105
Altman, D. G., and Bland, J. M. (1983). Measurement in medicine: the analysis of method comparison studies. The Statistician 32, 1–48. doi: 10.2307/2987937
Bakker, J. D. (2008). Increasing the utility of indicator species analysis. J. Appl. Ecol. 45, 1829–1835. doi: 10.1111/j.1365-2664.2008.01571.x
Balser, T. C., McMahon, K. D., Bart, D., Bronson, D., Coyle, D. R., Craig, N., et al. (2006). Bridging the gap between micro - and macro-scale perspectives on the role of microbial communities in global change ecology. Plant and Soil 289, 59–70. doi: 10.1007/s11104-006-9104-5
Banerjee, S., Walder, F., Buchi, L., Meyer, M., Held, A. Y., Gattinger, A., et al. (2019). Agricultural intensification reduces microbial network complexity and the abundance of keystone taxa in roots. ISME J. 13, 1722–1736. doi: 10.1038/s41396-019-0383-2
Barberan, A., Bates, S. T., Casamayor, E. O., and Fierer, N. (2012). Using network analysis to explore co-occurrence patterns in soil microbial communities. ISME J. 6, 343–351. doi: 10.1038/ismej.2011.119
Bardgett, R. D., and van der Putten, W. H. (2014). Belowground biodiversity and ecosystem functioning. Nature 515, 505–511. doi: 10.1038/nature13855
Bates, D., Mächler, M., Bolker, B., and Walker, S. (2015). Fitting linear mixed-effects models using lme4. J. Stat. Software 67, 1–48. doi: 10.18637/jss.v067.i01
Berry, D., and Widder, S. (2014). Deciphering microbial interactions and detecting keystone species with co-occurrence networks. Front. Microbiol. 5, 219. doi: 10.3389/fmicb.2014.00219
Biederbeck, V. O., Zentner, R. P., and Campbell, C. A. (2005). Soil microbial populations and activities as influenced by legume green fallow in a semiarid climate. Soil Biol. Biochem. 37, 1775–1784. doi: 10.1016/j.soilbio.2005.02.011
Bisanz, J. E. (2018). Qiime2r: Importing QIIME2 Artifacts and Associated Data into R Sessions. V. 0.99. Available online at: https://github.com/jbisanz/qiime2R/ (accessed January 01, 2022).
Bissett, A., Brown, M. V., Siciliano, S. D., and Thrall, P. H. (2013). Microbial community responses to anthropogenically induced environmental change: towards a systems approach. Ecol. Lett. 16(Suppl 1), 128–139. doi: 10.1111/ele.12109
Bodner, G., Loiskandl, W., and Kaul, H. P. (2007). Cover crop evapotranspiration under semi-arid conditions using FAO dual crop coefficient method with water stress compensation. Agric. Water Manage. 93, 85–98. doi: 10.1016/j.agwat.2007.06.010
Bolyen, E., Rideout, J. R., Dillon, M. R., Bokulich, N. A., Abnet, C. C., Al-Ghalith, G. A., et al. (2019). Reproducible, interactive, scalable and extensible microbiome data science using QIIME 2. Nat. Biotechnol. 37, 852–857. doi: 10.1038/s41587-019-0209-9
Bonanomi, G., De Filippis, F., Cesarano, G., La Storia, A., Ercolini, D., and Scala, F. (2016). Organic farming induces changes in soil microbiota that affect agro-ecosystem functions. Soil Biol. Biochem. 103, 327–336. doi: 10.1016/j.soilbio.2016.09.005
Bourgault, M., Wyffels, S. A., Dafoe, J. M., Lamb, P. F., and Boss, D. L. (2021). Introducing cover crops as fallow replacement in the Northern Great Plains: II. impact on following wheat crops. Renewable Agric. Food Sys. 37, 303–312. doi: 10.1017/S1742170521000508
Brisson, V. L., Schmidt, J. E., Northen, T. R., Vogel, J. P., and Gaudin, A. C. M. (2019). Impacts of maize domestication and breeding on rhizosphere microbial community recruitment from a nutrient depleted agricultural soil. Sci. Rep. 9, 15611. doi: 10.1038/s41598-019-52148-y
Calderón, F. J., Nielsen, D., Acosta-MartÍNez, V., Vigil, M. F., and Lyon, D. (2016). Cover crop and irrigation effects on soil microbial communities and enzymes in semiarid agroecosystems of the Central Great Plains of North America. Pedosphere 26, 192–205. doi: 10.1016/S1002-0160(15)60034-0
Callahan, B. J., McMurdie, P. J., Rosen, M. J., Han, A. W., Johnson, A. J., and Holmes, S. P. (2016). DADA2: High-resolution sample inference from Illumina amplicon data. Nat Methods. 13, 581–583. doi: 10.1038/nmeth.3869
Caporaso, J. G., Lauber, C. L., Walters, W. A., Berg-Lyons, D., Huntley, J., Fierer, N., et al. (2012). Ultra-high-throughput microbial community analysis on the Illumina HiSeq and MiSeq platforms. ISME J. 6, 1621–1624. doi: 10.1038/ismej.2012.8
Caporaso, J. G., Lauber, C. L., Walters, W. A., Berg-Lyons, D., Lozupone, C. A., Turnbaugh, P. J., et al. (2011). Global patterns of 16S rRNA diversity at a depth of millions of sequences per sample. Proc. Natl. Acad. Sci. USA. 108(Suppl 1), 4516–4522. doi: 10.1073/pnas.1000080107
Carr, P. M., Boss, D. L., Chen, C., Dafoe, J. M., Eberly, J. O., Fordyce, S., et al. (2020). Warm-season forage options in Northern Dryland Regions. Agron. J. 112, 3239–3253. doi: 10.1002/agj2.20261
Castle, S. C., Samac, D. A., Gutknecht, J. L., Sadowsky, M. J., Rosen, C. J., Schlatter, D., et al. (2021). Impacts of cover crops and nitrogen fertilization on agricultural soil fungal and bacterial communities. Plant and Soil 466, 139–150. doi: 10.1007/s11104-021-04976-z
Chan, H., and Akoglu, L. (2016). Optimizing network robustness by edge rewiring: A general framework. Data Mining and Knowledge Discovery 30, 1395–1425. doi: 10.1007/s10618-015-0447-5
Daryanto, S., Fu, B., Wang, L., Jacinthe, P.-A., and Zhao, W. (2018). Quantitative synthesis on the ecosystem services of cover crops. Earth-Sci. Rev. 185, 357–373. doi: 10.1016/j.earscirev.2018.06.013
De Cáceres, M., Legendre, P., and Moretti, M. (2010). Improving indicator species analysis by combining groups of sites. Oikos 119, 1674–1684. doi: 10.1111/j.1600-0706.2010.18334.x
DuPre, M. E., Seipel, T., Bourgault, M., Boss, D. L., and Menalled, F. D. (2022). Predicted climate conditions and cover crop composition modify weed communities in semiarid agroecosystems. Weed Res. 62, 38–48. doi: 10.1111/wre.12514
DuPre, M. E., Weaver, D. K., Seipel, T. F., and Menalled, F. D. (2021). Impacts of dryland cropping systems on ground beetle communities (coleoptera: carabidae) in the Northern Great Plains. J. Insect. Sci. 21, 1–8. doi: 10.1093/jisesa/ieab008
Euteneuer, P., Wagentristl, H., Neugschwandtner, R. W., Pauer, S., Keimerl, M., Piepho, H.-P., et al. (2022). Cover crops affect soybean (Glycine max) yield components, but not grain quality. Agron. J. doi: 10.1002/agj2.21158
Evans, D. M., Kitson, J. J. N., Lunt, D. H., Straw, N. A., Pocock, M. J. O., and Poisot, T. (2016). Merging DNA metabarcoding and ecological network analysis to understand and build resilient terrestrial ecosystems. Func. Ecol. 30, 1904–1916. doi: 10.1111/1365-2435.12659
Fan, K., Weisenhorn, P., Gilbert, J. A., and Chu, H. (2018). Wheat rhizosphere harbors a less complex and more stable microbial co-occurrence pattern than bulk soil. Soil Biol. Biochem. 125, 251–260. doi: 10.1016/j.soilbio.2018.07.022
Faust, K., and Raes, J. (2012). Microbial interactions: from networks to models. Nat. Rev. Microbiol. 10, 538–550. doi: 10.1038/nrmicro2832
Florence, A. M., Higley, L. G., Drijber, R. A., Francis, C. A., and Lindquist, J. L. (2019). Cover crop mixture diversity, biomass productivity, weed suppression, and stability. PLoS ONE 14, e0206195. doi: 10.1371/journal.pone.0206195
Gao, C., Xu, L., Montoya, L., Madera, M., Hollingsworth, J., Chen, L., et al. (2022). Co-occurrence networks reveal more complexity than community composition in resistance and resilience of microbial communities. Nat. Commun. 13, 3867. doi: 10.1038/s41467-022-31343-y
Ghimire, R., Ghimire, B., Mesbah, A. O., Idowu, O. J., O'Neill, M. K., Angadi, S. V., et al. (2018). Current status, opportunities, and challenges of cover cropping for sustainable dryland farming in the Southern Great Plains. J. Crop Improve. 32, 579–598. doi: 10.1080/15427528.2018.1471432
Gilbert, A. J. (2009). Connectance indicates the robustness of food webs when subjected to species loss. Ecol. Indicat. 9, 72–80. doi: 10.1016/j.ecolind.2008.01.010
Girvan, M., and Newman, M. E. (2002). Community structure in social and biological networks. Proc. Natl. Acad. Sci. USA. 99, 7821–7826. doi: 10.1073/pnas.122653799
Haruna, S. I., Anderson, S. H., Udawatta, R. P., Gantzer, C. J., Phillips, N. C., Cui, S., et al. (2020). Improving soil physical properties through the use of cover crops: A review. Agrosys. Geosci. Environ. 3, 307–317. doi: 10.1002/agg2.20105
Ishaq, S. L., Seipel, T., Yeoman, C. J., and Menalled, F. D. (2020). Soil bacterial communities of wheat vary across the growing season and among dryland farming systems. Geoderma 358, 113989. doi: 10.1016/j.geoderma.2019.113989
Kassambara, A. (2020). “ggpubr: ‘ggplot2' Based Publication Ready Plots.” 0.4.0 ed. Available online at: https://CRAN.R-project.org/package=ggpubr (accessed January, 2022).
Kim, N., Zabaloy, M. C., Guan, K., and Villamil, M. B. (2020). Do cover crops benefit soil microbiome? A meta-analysis of current research. Soil Biol. Biochem. 142, 107701. doi: 10.1016/j.soilbio.2019.107701
Krause, A. E., Frank, K. A., Mason, D. M., Ulanowicz, R. E., and Taylor, W. W. (2003). Compartments revealed in food-web structure. Nature 426, 282–285. doi: 10.1038/nature02115
Kurtz, Z. D., Muller, C. L., Miraldi, E. R., Littman, D. R., Blaser, M. J., and Bonneau, R. A. (2015). Sparse and compositionally robust inference of microbial ecological networks. PLoS Comput. Biol. 11, e1004226. doi: 10.1371/journal.pcbi.1004226
Legrand, F., Picot, A., Cobo-Díaz, J. F., Carof, M., Chen, W., and Le Floch, G. (2018). Effect of tillage and static abiotic soil properties on microbial diversity. Appl. Soil Ecol. 132, 135–145. doi: 10.1016/j.apsoil.2018.08.016
Lotia, S., Montojo, J., Dong, Y., Bader, G. D., and Pico, A. R. (2013). Cytoscape app store. Bioinformatics 29, 1350–1351. doi: 10.1093/bioinformatics/btt138
Love, M. I., Huber, W., and Anders, S. (2014). Moderated estimation of fold change and dispersion for RNA-seq data with DESeq2. Genome Biol. 15, 550–550. doi: 10.1186/s13059-014-0550-8
Lu, L., and Zhang, M. (2013). “Edge Betweenness Centrality,” in Encyclopedia of Systems Biology, eds W. Dubitzky, O. Wolkenhauer, K.-H. Cho and H. Yokota. (New York, NY: Springer New York), 647–648. doi: 10.1007/978-1-4419-9863-7_874
Ludwig, M., Wilmes, P., and Schrader, S. (2018). Measuring soil sustainability via soil resilience. Sci. Total Environ. 626, 1484–1493. doi: 10.1016/j.scitotenv.2017.10.043
Lupwayi, N. Z., Rice, W. A., and Clayton, G. W. (1998). Soil microbial diversity and community structure under wheat as influenced by tillage and crop rotation. Soil Biol. Biochem. 30, 1733–1741. doi: 10.1016/S0038-0717(98)00025-X
Ma, B., Wang, H., Dsouza, M., Lou, J., He, Y., Dai, Z., et al. (2016). Geographic patterns of co-occurrence network topological features for soil microbiota at continental scale in eastern China. ISME J. 10, 1891–1901. doi: 10.1038/ismej.2015.261
Martinez Arbizu, P. (2020). pairwiseAdonis: Pairwise multilevel comparison using adonis. R package version 0.4 ed. Available online at: https://github.com/pmartinezarbizu/pairwiseAdonis (accessed January, 2022).
McDaniel, M. D., Tiemann, L. K., and Grandy, A. S. (2014). Does agricultural crop diversity enhance soil microbial biomass and organic matter dynamics? A meta-analysis. Ecol. Appl. 24, 560–570. doi: 10.1890/13-0616.1
Meccage, E., Carr, P. M., Bourgault, M., McVay, K., and Boss, D. (2019). Potential of annual forages in the Northern Great Plains. Crops and Soils 52, 18–22. doi: 10.2134/cs2019.52.0101
Millard, P., and Singh, B. K. (2009). Does grassland vegetation drive soil microbial diversity? Nutr. Cycling Agroecosys. 88, 147–158. doi: 10.1007/s10705-009-9314-3
Munawar, A., Blevins, R. L., Frye, W. W., and Saul, M. R. (1990). Tillage and cover crop management for soil water conservation. Agronomy 82, 773–777. doi: 10.2134/agronj1990.00021962008200040024x
Oksanen, J., Blanchet, F. G., Friendly, M., Kindt, R., Legendre, P., McGlinn, D., et al. (2018). “Vegan: community ecology package,” in R Package Version 2.5–3. Available online at: https://github.com/vegandevs/vegan (accessed January, 2022).
Ouverson, T., Boss, D., Eberly, J., Seipel, T., Menalled, F. D., and Ishaq, S. L. (2022). Soil bacterial community response to cover crops, cover crop termination, and predicted climate conditions in a dryland cropping system. Front. Sustain. Food Sys. 6, 911199. doi: 10.3389/fsufs.2022.911199
Ouverson, T., Eberly, J., Seipel, T., Menalled, F. D., and Ishaq, S. L. (2021). Temporal soil bacterial community responses to cropping systems and crop identity in dryland agroecosystems of the Northern Great Plains. Front. Sustain. Food Sys. 5, 624242. doi: 10.3389/fsufs.2021.624242
Peralta, A., Sun, Y., McDaniel, M. D., and Lennon, J. T. (2018). Crop rotational diversity increases disease suppressive capacity of soil microbiomes. Ecosphere 9, 1–16. doi: 10.1002/ecs2.2235
Pocock, M. J., Evans, D. M., and Memmott, J. (2012). The robustness and restoration of a network of ecological networks. Science 335, 973–977. doi: 10.1126/science.1214915
Price, G. W., Langille, M. G. I., and Yurgel, S. N. (2020). Microbial co-occurrence network analysis of soils receiving short- and long-term applications of alkaline treated biosolids. Sci. Tot. Environ. 751, 141687. doi: 10.1016/j.scitotenv.2020.141687
Quast, C., Pruesse, E., Yilmaz, P., Gerken, J., Schweer, T., Yarza, P., et al. (2013). The SILVA ribosomal RNA gene database project: improved data processing and web-based tools. Nucleic Acids Res. 41, D590–596. doi: 10.1093/nar/gks1219
Radicchi, F., Castellano, C., Cecconi, F., Loreto, V., and Parisi, D. (2004). Defining and identifying communities in networks. Proc. Natl. Acad. Sci. USA. 101, 2658–2663. doi: 10.1073/pnas.0400054101
Romdhane, S., Spor, A., Busset, H., Falchetto, L., Martin, J., Bizouard, F., et al. (2019). Cover crop management practices rather than composition of cover crop mixtures affect bacterial communities in no-till agroecosystems. Front. Microbiol. 10, 1618. doi: 10.3389/fmicb.2019.01618
Rosner, K., Bodner, G., Hage-Ahmed, K., and Steinkellner, S. (2018). Long-term soil tillage and cover cropping affected Arbuscular Mycorrhizal fungi, nutrient concentrations, and yield in sunflower. Agron. J. 110, 2664–2672. doi: 10.2134/agronj2018.03.0177
Sigler, W. A., Ewing, S. A., Jones, C. A., Payn, R. A., Brookshire, E. N. J., Klassen, J. K., et al. (2018). Connections among soil, ground, and surface water chemistries characterize nitrogen loss from an agricultural landscape in the upper Missouri River Basin. J. Hydrol. 556, 247–261. doi: 10.1016/j.jhydrol.2017.10.018
Strickland, M. S., Thomason, W. E., Avera, B., Franklin, J., Minick, K., Yamada, S., et al. (2019). Short-term effects of cover crops on soil microbial characteristics and biogeochemical processes across actively managed farms. Agrosys. Geosci. Environ. 2, 1–9. doi: 10.2134/age2018.12.0064
Sun, W., Xiao, E., Krumins, V., Haggblom, M. M., Dong, Y., Pu, Z., et al. (2018). Rhizosphere microbial response to multiple metal(loid)s in different contaminated arable soils indicates crop-specific metal-microbe interactions. Appl. Environ. Microbiol. 84, e00701–e00718. doi: 10.1128/AEM.00701-18
Thapa, V. R., Ghimire, R., Acosta-Martínez, V., Marsalis, M. A., and Schipanski, M. E. (2021). Cover crop biomass and species composition affect soil microbial community structure and enzyme activities in semiarid cropping systems. Appl. Soil Ecol. 157, 103735. doi: 10.1016/j.apsoil.2020.103735
Unger, P. W., and Vigil, M. F. (1998). Cover crop effects on soil water relationships. J. Soil. Water Conserv. 53, 200–207.
USDA. (1999). Soil Taxonomy: A Basic System of Soil Classification for Making and Interpreting Soil Surveys, 2nd Edn. Washington, DC: Natural Resources Conservation Service; U.S. Department of Agriculture.
USDA. (2020). Montana Farm Service Agency 2020 Reported Crops. Bozeman, MT: U.S. Farm Services Agency, Montana Office. Available online at: https://www.fsa.usda.gov/state-offices/Montana/resources/index (accessed June 01, 2021).
Weiss, S., Xu, Z. Z., Peddada, S., Amir, A., Bittinger, K., Gonzalez, A., et al. (2017). Normalization and microbial differential abundance strategies depend upon data characteristics. Microbiome 5, 27. doi: 10.1186/s40168-017-0237-y
Williams, R. J., Howe, A., and Hofmockel, K. S. (2014). Demonstrating microbial co-occurrence pattern analyses within and between ecosystems. Front. Microbiol. 5, 358. doi: 10.3389/fmicb.2014.00358
Wyffels, S. A., Bourgault, M., Dafoe, J. M., Lamb, P. F., and Boss, D. L. (2021). Introducing cover crops as a fallow replacement in the Northern Great Plains: I. evaluation of cover crop mixes as a forage source for grazing cattle. Renew. Agric. Food Sys. 37, 292–302. doi: 10.1017/S1742170521000417
Zentner, R. P., Tessier, S., Peru, M., Dyck, F. B., and Campbell, C. A. (1991). Economics of tillage systems for spring wheat production in southwestern Saskatchewan (Canada). Soil Tillage Res. 21, 225–242. doi: 10.1016/0167-1987(91)90022-P
Keywords: cover crops, semi-arid environments, continuous cropping, cropping systems, microbiome, co-occurrence networks, soil health
Citation: Eberly JO, Bourgault M, Dafo JM, Yeoman CJ, Wyffels SA, Lamb PF and Boss DL (2022) Soil bacterial community response to cover crop introduction in a wheat-based dryland cropping system. Front. Sustain. Food Syst. 6:948220. doi: 10.3389/fsufs.2022.948220
Received: 19 May 2022; Accepted: 17 October 2022;
Published: 02 November 2022.
Edited by:
Johann G. Zaller, University of Natural Resources and Life Sciences, AustriaReviewed by:
Abraham Loera Muro, Centro de Investigaciones Biológicas del Noroeste (CIBNOR), MexicoPia Euteneuer, University of Natural Resources and Life Sciences, Austria
Copyright © 2022 Eberly, Bourgault, Dafo, Yeoman, Wyffels, Lamb and Boss. This is an open-access article distributed under the terms of the Creative Commons Attribution License (CC BY). The use, distribution or reproduction in other forums is permitted, provided the original author(s) and the copyright owner(s) are credited and that the original publication in this journal is cited, in accordance with accepted academic practice. No use, distribution or reproduction is permitted which does not comply with these terms.
*Correspondence: Jed O. Eberly, amVkLmViZXJseUBtb250YW5hLmVkdQ==