- 1Professorship of Ecological Services, Bayreuth Center of Ecology and Environmental Research, University of Bayreuth, Bayreuth, Germany
- 2Environmental Sciences, Faculty of Geosciences, Copernicus Institute of Sustainable Development, Utrecht University, Utrecht, Netherlands
- 3Environmental Geography Group, Faculty of Science, Institute for Environmental Studies, Vrije Universiteit Amsterdam, Amsterdam, Netherlands
- 4Department Landscape Ecology, Helmholtz Centre for Environmental Research-UFZ, Leipzig, Germany
A debate about cultivation and trading of soy has emerged among scientists, policymakers, and the public in recent years. Export-orientated soy production in regions of South America is associated with large-scale ecosystem destruction. Since soy is an important source of animal fodder, policymakers are developing schemes to support and enhance sustainable domestic soy cultivation, especially in the EU. Expanded soy cultivation should ideally provide high yields and at the same time promote environmental benefits. For this purpose, we applied a multi-objective optimization algorithm that selects areas with maximum soy suitability, minimum erosion risk, need for low fertilizer input due to water quality issues, and need for diversification of monotonous crop rotations. We use the state of Bavaria in Germany as a case study, modeling full self-sufficiency of soy. The results of the optimization indicate synergies between plantation suitability with need for low fertilization input and crop variation, which implies that the environmental benefit of nitrogen fixation and rotation diversification from soy plants can easily be reconciled with food productivity. However, slight trade-offs occur between erosion risk and the three other objectives, i.e., locations with better soy production might be more prone toward erosion risk. As a potential consequence of expanded soy cultivation in Bavaria, we identified winter wheat, grain maize, potatoes, and sugar beet as those crops that have the highest share of displaced cultivation area. To reduce such land use conflicts and ensure self-sufficiency in relevant crops, we recommend to limit the use of soy as animal feed. Nevertheless, we propose to explicitly incorporate the local need for the environmental benefits of soy cultivation in the planning for soy expansion. In doing so, domestic soy can turn into a real sustainable alternative to imported plant protein.
Introduction
Demographic growth, increasing gross domestic products, and urbanization have driven food consumption and dietary preferences worldwide toward a rising meat consumption with annual increases of 1.15 % (de Visser et al., 2014; Sans and Combris, 2015). In the European Union (EU), the most important protein source for livestock feed can be attributed to the soy complex, consisting of meal, beans, and seeds. Despite strong fluctuations of the soy price over the last years, the EU heavily relies on soy imports, originating mainly from Brazil, Argentina, and the United States, while only 5 % of the soy-based protein is produced domestically (de Visser et al., 2014; European Commission, 2018). This dependency represents not only an economic risk, but the industrialized soy production also contributes to deforestation, biodiversity loss, and land degradation in soy exporting countries. Therefore, a growing self-sufficiency in the European animal feed market could increase food security and lessen the environmental damage from agricultural expansion in producing countries (Boerema et al., 2016; Zander et al., 2016; Zabel et al., 2019).
Against this background, the EU has been working toward a joint protein plan through consultations of stakeholders, thematic workshops, and several research programs to examine the protein crop complex (European Commission, 2018). The declared aims include support of farmers who are growing legumes through farm subsidies, research and innovation financing, market monitoring, and plant protein promotion (European Commission, 2018). In order to put the process of increased protein plant cultivation into effect, European policy-makers support research regarding the supply chain possibilities (European Commission, 2018), the crop science (Wilbois et al., 2014), or feed management (LfL, 2019a). As a transnational initiative, DonauSoja supports the added value soy in the countries of the Danube basin (DonauSoja, 2022). In Germany, soy promotion associations (e.g., Sojaförderring, 2022) endorse the expansion of soy cultivation. Also, the federal state of Bavaria launched a protein initiative to solicit the uptake of protein crop cultivation and to inform and advice farmers as well as the public. The objectives of this federal initiative include a reduced dependence from overseas protein imports for animal feed, support for local grassland and legumes, and strengthened economic value added by domestic protein plants (LfL, 2020a).
However, few studies have engaged with the environmental aspects of soy cultivation in Central Europe. Previous research has focused on the influence of climate change on protein plant production in Europe (Mitter et al., 2015) and the interplay between the cultivation of grain legumes and ecosystem functions in general (Reckling et al., 2016; Watson et al., 2017). In reports from policymakers of various scales, the potential environmental and climatic benefits regarding domestic protein plant cultivation are only mentioned briefly, while the focus is clearly set on agronomic considerations and feed management. Yet, one declared objective remains the sustainable production of domestic protein plants (European Commission, 2018; LfL, 2020a).
Planning for such sustainable cultivation should consider and evaluate the effects of legumes on the local ecosystems, i.e., by taking into account the various ecosystem services they provide: the provision of human food and animal feed, supporting services due to biological nitrogen fixation, and regulating services from their impact on soil microbes. An introduction of grain legume into crop rotations can further enhance biodiversity and suppress weeds or diseases (Watson et al., 2017). While many publications characterized the various environmental impacts of different legumes (e.g., Nemecek et al., 2008; Zander et al., 2016), locations and situations remain to be delineated where they can be cultivated to promote economic as well as environmental benefits (Reckling et al., 2016).
In this context, our study aims to contribute to the current efforts by applying a multi-objective spatial optimization for Bavaria, Germany. This analysis intents to identify ecologically and biophysically optimal land allocations for enhanced soy cultivation. We chose four objectives that are relevant for sustainable soy cultivation. They include best climatic and soil suitability for planting soy to ensure successful production. Minimum erosion risk is included to limit soil damage. To find locations that would benefit from soy as a nitrogen-fixing and rotation diversifying legume, maximum nitrate pollution and minimum crop diversity are set as objectives. Those two objectives help to allocate soy in places with environmental problems from current management that are supposed to be lessened by introducing soy. With these objectives, we address important environmental issues of European agriculture. Further, we analyze trade-offs and synergies between the objectives and evaluate the consequences of domestic production in terms of changes in cultivation patterns of other important crops in this region and the emerging land-use conflicts. This study focusses on soy due to its dominating role in the animal feed sector. Other pulses like faba bean, pea or lupins used for livestock are grown largely in the EU (European Commission, 2018), hence, they are not as charged with the environmental and economic consequences of large-scale import as soy.
Materials and methods
Study area
The German federal state of Bavaria is located in southern Germany. It lies in the warm-temperate climate zone with a multi-annual average (1971–2000) temperature of 7.9°C and precipitation of 941 mm, respectively (StMUV, 2021). The soil types north of the Danube River represent a mosaic of cambisols, podzols, stagnosols, and rendzina, while the soils south of the river are dominated by cambisols (UBA, 2010). The federal state covers about 70,500 km2 of which 46.4 % are agriculturally used as of 2018 (Figure 1). Within the agricultural sector, livestock production has a high significance. The State of Bavaria is a net exporter of animal products with milk being its economically most important product, accounting for 26.7 % of the agricultural gross output in 2018 (StMELF, 2020a).
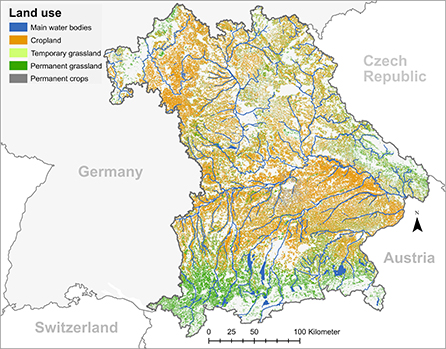
Figure 1. Major agricultural land use categories in Bavaria, based on data of the Integrated Administration and Control System (IACS) for the year 2020 (EU Regulation, 2014) as well as main water bodies.
Due to Bavaria's protein initiative, the cultivation area of legumes like soy, lupin, field pea, faba bean and field bean have doubled since the initiative started in 2011 reaching 600 km2 in 2019 (LfL, 2019a, 2020a; StMELF, 2019a). Soy cultivation covered about 86 km2 (29.928 tons) in 2017 and reached approximately 155 km2 (50.220 tons) in 2019 (LfL, 2019b; StMELF, 2019a, 2020b).
Optimization problem
To identify ecologically and biophysically ideal allocations of soy production, several aspects have to be considered: (1) Soy productivity depends on the climatic and soil suitability of a site for soy cultivation (Geisler, 1980; Heyland, 1996; Diepenbrock et al., 2016). Therefore, we used a suitability factor combining temperature, precipitation and soil requirements of soy plants. (2) Soil might be more susceptible to water erosion under soy plantation, because the plants are sown with a lower density than for instance summer cereal crops (Kistler et al., 2013). Hence, soy should be located on arable fields that are least prone to erosion to ensure an intact soil throughout and after the plantation. (3) Fertilizer application can be reduced with nitrogen fixation from soy (Mitter et al., 2015). To allocate soy in areas that most need this benefit, the spatial optimization is set to favor sites with currently high nitrate pollution. Finally, (4) soy cultivation can enrich crop rotations at sites where the rotational diversity is low, i.e., where currently the lowest number of unique crop types can be found. The addition of soy into crop rotations is said to inhibit the spread of weeds through a more diverse range of natural competitors, as well as increase the soil fertility and overall biodiversity (Smith et al., 2008; Pistrich et al., 2014; Isbell et al., 2017). Considering points (1) to (4), we formulated four objectives for the optimization, which describe the desired characteristics of those locations where soy should be planted:
(1) maximum soy suitability (to ensure good biophysical growth conditions),
(2) minimum erosion risk (since soy is an erosion-prone crop),
(3) maximum current nitrate pollution (to allocate soy where reduced fertilizer input is needed),
(4) minimum current crop diversity (to allocate soy where crop rotation needs diversification).
The mathematical formulation of these objectives can be found in the Supplementary material 1.
Input data
For the optimization process, we created an input map of fields that are eligible for soy cultivation according to their objective scores. This map consists of grid cells (150 × 150 m) that were joined to patches (~0.05 to 50 km2) that serve as decision variables. We established the patches by assigning the scores for the four objectives to Bavaria's agricultural fields from the data of the Integrated Administration and Control System (IACS) (EU Regulation, 2014). We combined fields with the same four scores into one patch. The resulting patches were of diverging sizes, hence we split patches larger than 100 km2 into five patches to avoid abrupt land-use changes when these patches change from soy plantation to no soy plantation or vice versa. Further, we merged patches smaller than 0.1 km2 with patches of similar objective scores to limit the number of decision variables in the optimization in order to reduce computation time (Strauch et al., 2019). Hereafter, we transformed fields into grid cells holding the patch number of the resulting 473 patches. Further information on the pre-processing is summarized in the Supplementary material 2.
Our first objective for the optimization is the sites' suitability for soy plantation, which is determined by the requirements of the plant. During germination, the air temperature must not drop below 8–10°C. Soy is susceptible to frost and depends on warm temperatures during the main growth period (Diepenbrock et al., 2016). The plants can endure dry periods but exhibit water demand during flowering and grain development. Therefore, the water holding capacity of the soil is fundamental (Geisler, 1980; Diepenbrock et al., 2016). While soy can be cultivated on various soils if they are easily warmed, waterlogged or clayey soils are unsuitable for soy cultivation (Heyland, 1996). We calculated the input data for suitability from the soil survey map at scale 1:1000000 published by the Federal Agency for Geology and Resources (BGR) and daily climate data spanning the years 1981 to 2010 from the German Meteorological Service (DWD). We interpolated these datasets and aggregated them into classes, which we assigned scores and weighted among each other as documented in Schätzl et al. (2015). The sum of the scores resulted in a final suitability factor ranging from 1 to 11. Only fields with medium to high suitability scores between 5 and 11 are included in the optimization, because these are considered to have worthwhile cultivation conditions for soy (LfL, 2019c).
The second objective refers to minimum erosion risk on the potential soy fields. The average long-term potential water erosion is calculated by the authorities based on soil erodibility (K-factor) and slope (S-factor) from the Universal Soil Loss Equation (LfL, 2020b). We aggregated the data to median values per fields (IACS data for 2019). For the optimization process, we classified the erosion potential as specified in the standard DIN 19708 (Schäfer et al., 2017). We derived five classes of erosion risk spanning from soil loss of below 5 t/ha/a to very high losses of more than 30 t/ha/a (see Table 1). For the optimization process, we used integer values per DIN class, stretching from 1 to 5.
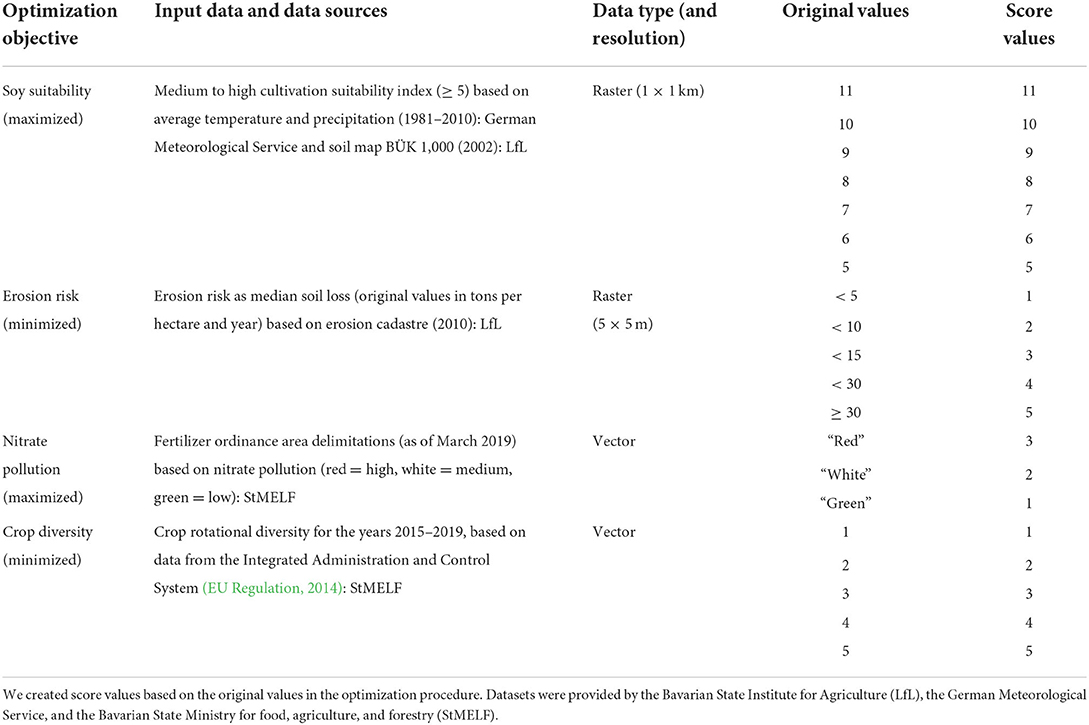
Table 1. Summary of input data for the optimization objective including their considered ranges (values are listed for each objective in descending favorability).
For the third objective, we used areas currently showing a high level of nitrate pollution. To approximate those, we followed the designated areas as defined by the federal fertilizer ordinance (Fertilizer ordinance, 2020; LfL, 2020c). Farms in so-called “red areas” are facing special obligations to reduce the high nitrate pollution, while farms in “green areas” on the contrary can get exemptions from generally applied regulations. For farms in “white areas” none of these two options applies (Execution ordinance AVDüV, 2018; LfL, 2020d). For the optimization, we assigned integer values from 1 to 3 as scores to each area type (see Table 1).
To calculate the crop rotational diversity for the fourth objective, we extracted the information on crops planted in the years 2015 to 2019 from the IACS data of the respective years. For each agricultural field, we summed up the number of unique crop types over the 5-year period, while omitting the records of permanent grassland occupation to exclude the option of grassland transformation into cropland.
The scores for each objective were treated as integer values to streamline the computation process. Per objective, we transformed the integer score values to grid cells, since the algorithm requires the score maps in addition to the patch map. All data sources, the original values and the derived scores are compiled in Table 1. Further explanation, the maps of the input datasets, and a list of the soil types and their classification can be found in the Supplementary materials 3, 4.1, 4.3. The data preparation was performed in ArcMap 10.2.2, ArcGIS Pro 2.9, and RStudio 4.1.1.
Optimization process
The spatial optimization problem was solved using the tool CoMOLA (Constrained Multi-objective Optimization of Land use Allocation), which is based on the Non-dominated Sorting Genetic Algorithm-II (NSGA-II) (Deb et al., 2002; Strauch et al., 2019). The patches serve as decision variables, which can take on values corresponding to a land use category, in our case fields with soy and fields without soy. For the first generation of optimization solutions, a set of maps with patch selections (individuals) is generated randomly from the input map. The performance of each of those maps regarding the objective functions (i.e., fitness values) is evaluated by means of user-defined models, e.g., process-based or statistical models written in R (in this study) or Python (Strauch et al., 2019). According to these fitness values, the algorithm sorts the solutions based on their performances and uses the best solutions to generate the next generation by crossover and mutation. This process is repeated until a stopping criterion, e.g., the maximum number of generations, is met (Figure 2). In total, seven optimization runs were completed, each with a population size of 100 individuals and 250 generations, which proved to be a sufficient number of generations (see Supplementary material 4.4).
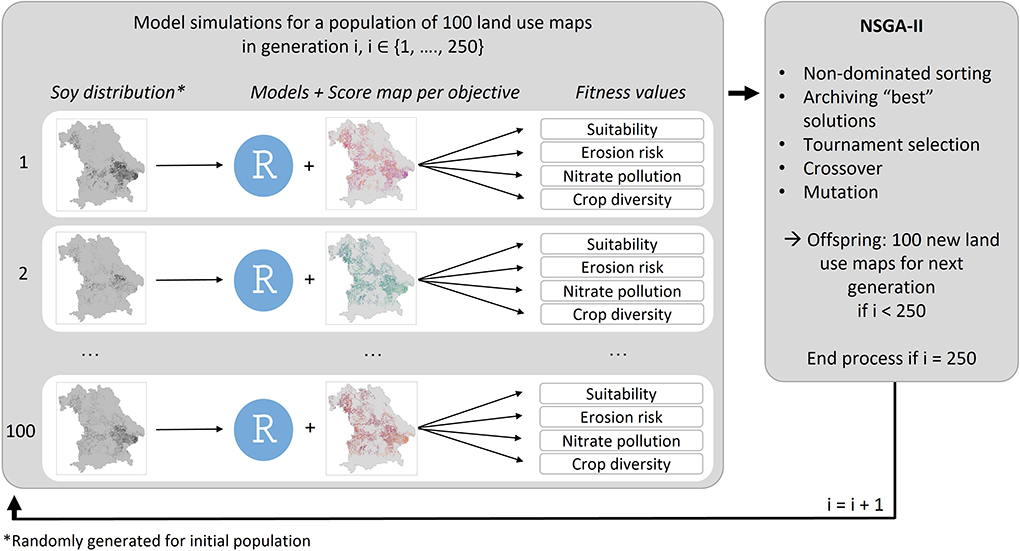
Figure 2. Workflow of the optimization process with CoMOLA (adapted from Kaim et al., 2020). For the initial population, the algorithm randomly generates maps of soy distribution. The fitness values of each map are calculated by models in RStudio based on the score maps. The NSGA-II (Non-dominated Sorting Genetic Algorithm-II) sorts the spatial solutions according to their fitness values (non-dominated sorting), compares the ranked solutions (tournament selection), and creates the next generation by combining and altering the previous best solutions (crossover and mutation). The algorithm terminates after 250 generations.
The area covered by patches selected for soy distribution by the algorithm is limited to minimum 10% and maximum 11% of the total modeled area. This proportion is based on the fact that Bavaria imports approximately 500,000 t/year, assuming an average soy yield of 2.98 t/ha (as between 2015 and 2019 in Bavaria) and current production of 155 km2 in 2019, an area of 1813.6 km2 or 10.7% of Bavaria's total crop area (StMELF, 2018a, 2020b) would be required to compensate soy imports by local production.
Post-processing of optimization results
The result of a multi-objective optimization is a set of non-dominated optimal solutions called Pareto-frontier. A solution is defined to be non-dominated if there exists no other feasible solution that improves the value of one objective without simultaneously decreasing the value of another objective. The solutions can be analyzed in terms of their fitness values. As part of the analysis, the ten maps with the best fitness values for each objective were consolidated to one map of optimal soy allocation for each objective, respectively. This decision is based on the fact that two solutions can have very similar fitness values for a given objective, but the optimization algorithm chose patches at very different locations to compile the respective soy distributions. Therefore, the best 10 solution maps vary little in the respective fitness values per objective and still capture the spatial distribution of regions that are favorable for soy cultivation according to our objectives. For a compromise between the objectives, we picked from all the Pareto-optimal solutions the one whose fitness values deviate the least from the means of the fitness values of all four objectives.
To judge potential impacts of the expanded soy cultivation, we evaluated which crops have the highest share of being displaced by each objectives' best solution and the compromise solution. This is calculated by analyzing the average crop distribution between 2015 and 2019 on the fields with the largest overlap per grid cell that has been selected for soy cultivation by the optimization algorithm. Since it was assumed that soy would cover the area of the entire grid cell, also the replaced crop was assumed to be replaced by the respective area. In addition, we estimated if certain crops are displaced disproportionally. For this, we compared the displaced area to the average cover area [km2] of the respective crops in Bavaria between 2015 and 2019. Those percentages point out if the optimized soy distributions would simply displace an average crop mix or affect specific crops disproportionally.
Results
The resulting solutions of the optimization have fitness values per objective, which are derived from averaging the integer scores of all the fields that are selected for soy cultivation by a given solution. Figure 3 illustrates how the fitness value distribution of all solutions is influenced by the percentage of area available per score. Suitability and nitrate pollution are maximized by the optimization, hence the algorithm has included areas with high scores. Erosion risk and crop diversity are minimized by the optimization, therefore areas with low scores were selected.
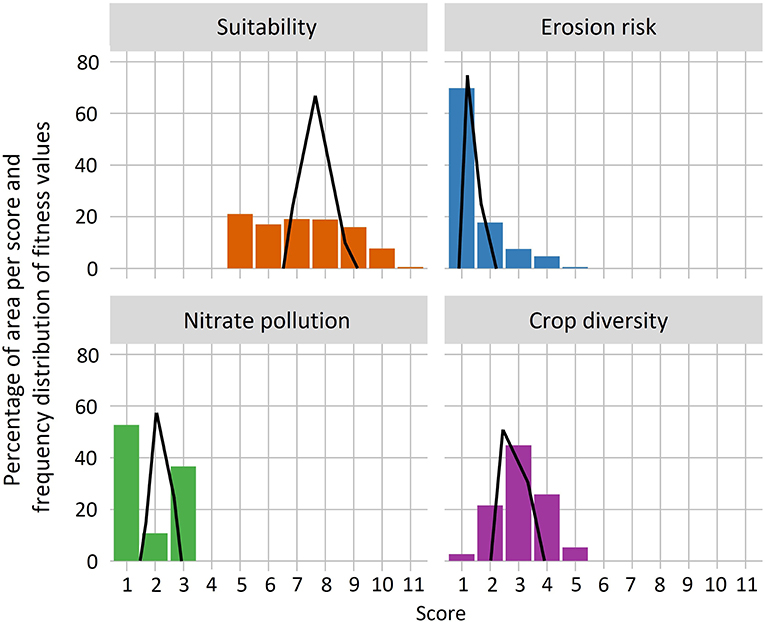
Figure 3. Percentage of area per score of the input data (bars) and distribution of fitness value scores of all non-dominated solutions (black lines) for each objective. The fitness value range along the scores is displayed as percentage of fitness value frequency. This number demonstrates in relative terms how many of all optimal solutions have a certain fitness value.
Regarding our four chosen objectives, the results of the spatial optimization reveal both synergies and trade-offs (Figure 4). The seven initiated optimization runs resulted in 992 Pareto-optimal (non-dominated) solutions. When comparing the fitness values of these solutions, crop diversity has a slightly positive relationship with suitability, while presenting a trade-off with erosion risk. Erosion risk is negatively correlated with all objectives, displaying the largest trade-off with suitability. Nitrate pollution has a near-zero correlation with crop diversity and a slightly positive relationship with suitability. However, as the scatterplots indicate, a linear regression does not seem to be the most appropriate representation of the relationship between those objectives (non-linearity).
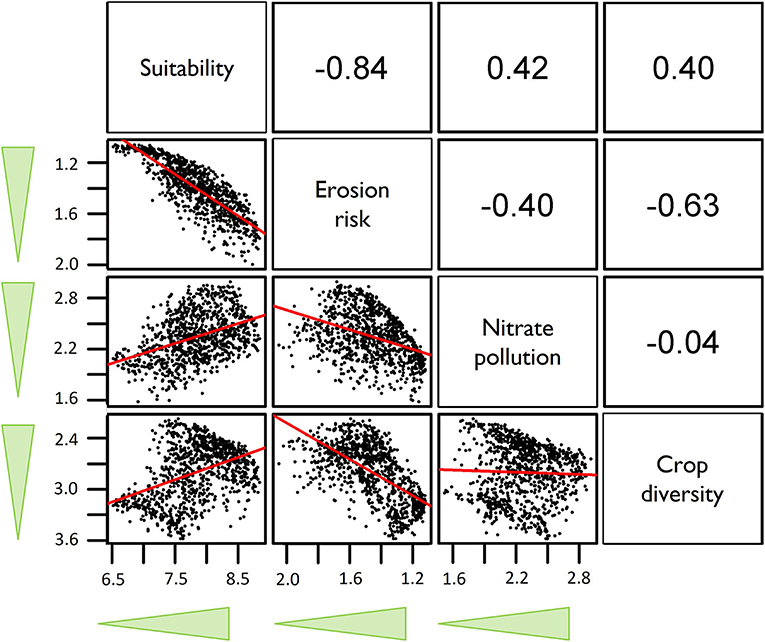
Figure 4. Scatter plots of dual relationships between the objectives of the 992 Pareto-optimal solutions of the spatial optimization. All solutions are displayed as dots, each with one fitness value per objective. A linear regression line is added in red as well as correlation coefficients after Pearson. Positive correlation coefficients indicate a synergy, negative coefficients a trade-off. Fitness values for soy suitability range from 5 to 11 (most desirable: 11), for erosion risk from 1 to 5 (most desirable: 1), for nitrate pollution from 1 to 3 (most desirable: 3), and for crop diversity from 1 to 5 (most desirable: 1). The green triangles indicate where desirable fitness values are in the scatter plots with their two-edged side. The pointy end of the triangle indicates the less desirable fitness values.
In the spatial distribution of the optimized soy allocations, trade-offs and synergies are also evident (Figure 5). Large overlaps in the maps of the 10 best solutions depict the spatial synergies among the objectives, since the respective compilations share large areas where both objectives can be achieved. Maps with small overlapping areas point to trade-offs among the respective objectives due to low amount of shared optimal areas. The relatively large overlapping areas of the ten best suitability solutions with the ones from crop diversity (5E) and nitrate pollution (5F) confirm the positive relationship found by the correlation coefficients. In addition, all objectives share small areas with the best solutions of erosion risk (5A, 5B, 5C). Here, the negative correlation coefficients are confirmed again by the spatial distribution of the best ten solutions. Lastly, the spatial comparison of crop diversity and nitrate pollution (5D) shows a larger overlap than the near-zero correlation coefficient suggested. This could be due to the possible non-linear relationship between the objectives. The maps of each objectives' single best solution can be found in the Supplementary material 4.5.
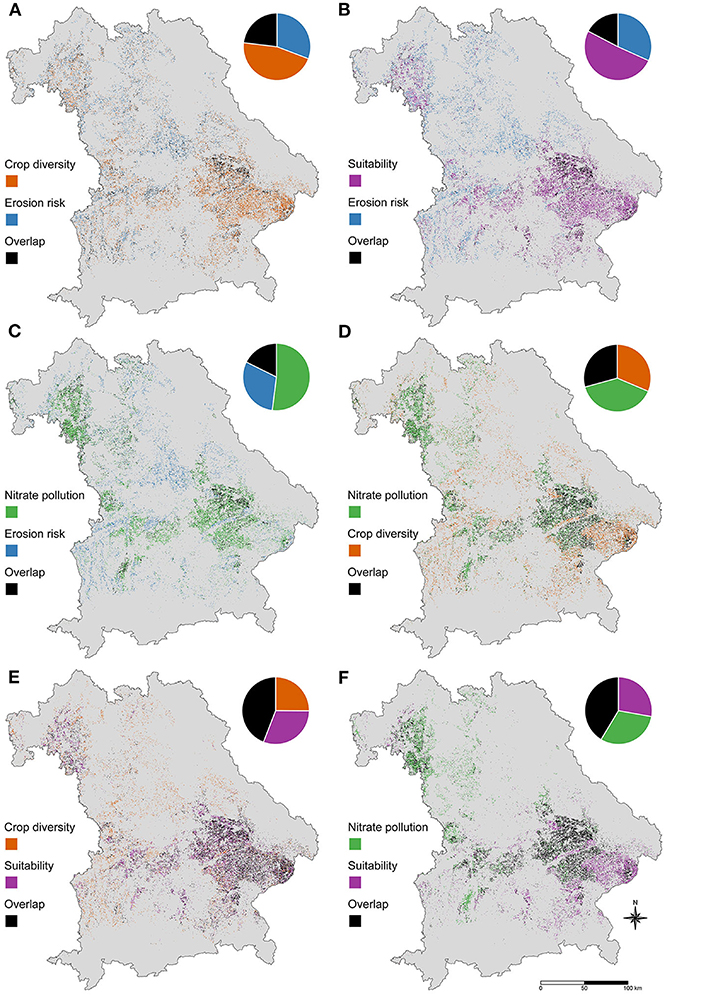
Figure 5. Pairwise comparison of spatial overlaps of the 10 best out of 992 Pareto-optimal solutions per objective of (A) crop diversity and erosion risk (overlap 23%) (B) suitability and erosion risk (overlap 17%) (C) nitrate pollution and erosion risk (overlap 18%), (D) nitrate pollution and crop diversity (overlap 29%), (E) crop diversity and suitability (overlap 44%), (F) nitrate pollution and suitability (overlap 41%). The pie chart depicts the share of individual patch selection by each objective and the overlap (black).
The generated compromise solution between all four objectives still exhibits relatively high fitness values for all of them. Compared to the optimal solutions for each individual objective, the compromise solution achieves still high shares of the best possible values: 88% (7.80 compared to 8.84) suitability, 81% (1.24 compared to 1.04) erosion risk, 79% (2.26 compared to 2.89) nitrate pollution, 72% (2.78 compared to 2.18) for crop diversity. In terms of the spatial soy distribution, the compromise solution most strongly resembles the best 10 solutions for nitrate pollution (overlap of 67%) and least the best 10 solutions for erosion risk (overlap of 54%). The overlaps with suitability (overlap of 65%) and crop diversity (overlap of 63%) range in between. Compared to the soy distribution in 2019, the compromise solution performs similarly good for suitability (-0.04%) and better regarding all other objectives (+18% for erosion risk, +15% for nitrate pollution and +21% for crop diversity). Regarding the spatial distribution, the compromise solution partly overlaps with the focus regions of the current soy cultivation (Figure 6). However, our compromise solution shifts the current most frequent soy cultivation territory a little more toward the North-East of Bavaria. Judging from the fitness value and the spatial distribution, soy cultivation in 2019 makes use of biophysically highly suitable areas, but due to its small spatial extent, it only covers 8.5% of the soy area in the optimized solutions. In the compromise solution, territories are added that are currently rarely selected for soy cultivation, because the optimization selected patches to achieve the desired values for all the four objectives and the self-sufficiency in soy production.
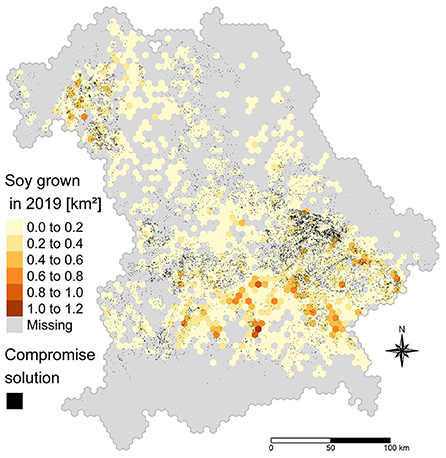
Figure 6. Area of optimized soy allocation according to our compromise solution in comparison to the soy grown in Bavaria based on data of the Integrated Administration and Control System (IACS) for the year 2019 (EU Regulation, 2014). The compromise solution harmonizes the four optimization objectives in terms of their fitness values.
The area of crops that would be displaced if the soy distribution would be expanded according to our optimization solutions is shown in Figure 7. Substantial shares of the cultivation area of starch potatoes (cultivation area on average 2015–2019 in Bavaria 132 km2), sugar beet (645 km2), grain maize (1,240 km2), winter wheat (4,711 km2), and potatoes (278 km2) would experience shifts to soy for all selected solutions. The distinct spatial distributions of the solutions are shown by the different shares of crops displaced by them. The crops depicted in the diagram would experience more than 20% displacement in at least one of the solutions. Further crops with lower displacement share can be found in the Supplementary material 4.6.
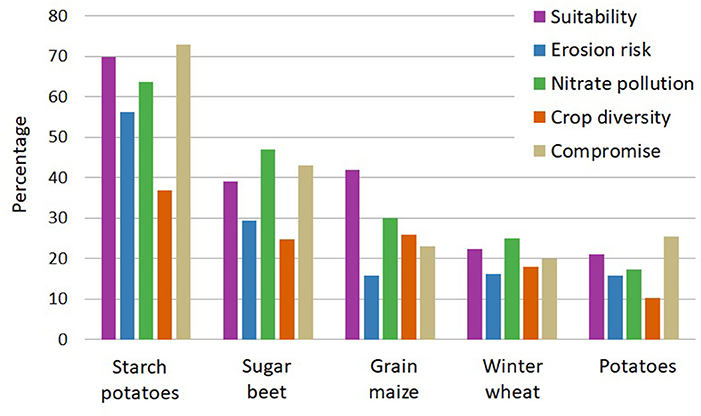
Figure 7. Share of crop cover from the Bavarian average between 2015 and 2019 that are most affected by a shift toward soy cultivation according to each single best solution per objective and the compromise solution. For example, for the solution with the highest fitness value for soy suitability, 70% of the area used for cultivating starch potatoes in Bavaria is displaced by soy production.
Discussion
Synergies and trade-offs between biophysical and ecological objectives
The strongest synergies we identified, are between crop diversity and suitability as well as between nitrate pollution and suitability. These objectives share larger areas of desirable scores. Therefore, a soy expansion scenario according to the best suitability solutions would proceed on fields with good growth opportunities and a need for biological fertilization from nitrogen fixation and diversified crop rotations. It appears that the fields in biophysically suitable areas for soy are currently under intensive agricultural management, which is characterized, among other factors, by high agrochemical input and low crop diversity (Tscharntke et al., 2021). In addition to the environmental benefits from diversifying rotations with soy like improved biodiversity and soil health, other measures like enhanced natural and semi-natural structures and overall landscape complexity would be needed to fully achieve the ecological objectives (Kormann et al., 2015; van der Plas et al., 2019).
The optimization results also revealed rather strong trade-offs among erosion risk and the other objectives. From this we could potentially derive that soy expansion scenarios that satisfy the other three objectives utilize fields with high erosion risk. However, a closer look at the fitness values for erosion risk shows that any solution contains only fields with fitness values between 1 and 2, which refers to a potential soil loss up to 10 tons per hectare per year. Therefore, even though the mathematical trade-off suggests erosion problems for many solutions, the selected fields would experience erosion risk at the lower end of the scale. According to the German Federal Environmental Agency, erosion of 10 tons per hectare refer to one millimeter of soil loss per year. However, the actual impact on crop yield from the loss of fertile soil under this erosion risk depends on soil type, soil density and on-site farming practices (UBA, 2022).
We selected the objectives maximum nitrate pollution and minimum crop diversity to represent the need for environmental benefits from soy as a legume. However, the potential for making use of these benefits depends on local farming practices. After the soy harvest (usually in October), there is a danger of nitrate leaching depending on weather conditions and soil type. However, long-term experiments showed that certain measures can limit the nitrate losses. They include the cultivation of winter crops with high nitrogen uptake, e.g., winter wheat or the cultivation of winter hardy greening. In addition, it is recommended to apply a mulch sowing procedure and abstain from plowing to minimize the mineralization of crop residues (LTZ, 2018, 2020). Since the nitrogen fixation abilities of soy spare the use of additional fertilizer (Eder et al., 2019) and nitrate leaching can be prevented by appropriate management, in the long term, soy plantation in areas with high levels of nitrate pollution is a desirable option. The magnitude of benefits from diversifying a crop rotation with soy depends on the implementation on farm level. Including soy too frequently in crop rotations could impair the yields due to the long survival of pathogens (Watson et al., 2017). Experts recommend a 3 to 4 year gap to other legumes, rapeseed, and sunflower to avoid infestation of Sklerotinia (Eder et al., 2019). In contrast, intercropping of winter cereals with legumes might improve yields and promote nutrients exchange (Watson et al., 2017; LTZ, 2019).
Limitations
The chosen calculation of suitability for Bavaria closely matches a cultivation suitability study for Germany published by Rossberg and Recknagel (2017). It slightly differs in the classification of heat units and precipitation sums into scores. In the suitability calculation for Bavaria, radiation is not included, since no radiation deficits are expected in Southern Germany (Rossberg and Recknagel, 2017). The chosen suitability approach does not apply Liebig's law of the minimum, hence, the potential limitations for soy growth due to low values in either one of the included factors are not reflected in the suitability calculation. Additionally, the chosen suitability approach does not reflect a reduction of the suitability above a certain optimum. Other suitability calculations over larger spatial scales utilized temperature and precipitation with an optimum (Zabel et al., 2014) or corrected the potential maximum yield with temperature and water deficit reduction factors as well as combinations of soil type and slope (Fischer et al., 2021). However, we chose the approach that classifies temperature as the most limiting factor due to its high importance during the crucial periods of germination and flowering (Diepenbrock et al., 2016; Karges et al., 2022). Regarding the climatic conditions in Bavaria, temperature is the limiting factor at the lower end, while soy cultivation under the highest reached temperature can still be successful when utilizing irrigation. On sandy soils, experimental studies found that irrigation improved grain and protein yield in soy. The magnitude of improvement depends also on the reaction of different cultivars to the changes in the environmental conditions (Karges et al., 2022). Lower precipitation can also partly be compensated through suitable soil (Rossberg and Recknagel, 2017). On the other hand, strong precipitation can limit the yield through strong vegetative development and impeded maturity of the grains (Diepenbrock et al., 2016; Rossberg and Recknagel, 2017), but these conditions are accounted for with the exclusion of waterlogging soils. In the selected suitability approach, the specific interactions between temperature, precipitation and soil regarding soy suitability in Bavaria are reflected in the scoring. The resulting suitability map resembles the spatial distribution of the suitability index as modeled by the Global Agro-Ecological Zones (GAEZ, 2022), see the latter in the Supplementary Figure 4.2.
In terms of the results, the resulting fitness values of the optimized solutions are driven by the availability of patches with desirable scores. Figure 3 shows that e.g., for suitability, there is only a small area with high scores (10 and 11). Hence, the optimization algorithm also has to select patches with mediocre suitability scores to create a solution. The same applies for erosion risk, where the high abundance of patches with scores 1 and 2 resulted in the averaged fitness values being mainly distributed between these two numbers. In addition, assigning the input data values to scores (Table 1) led to disproportional distribution of value ranges within the scores. For example, high erosion risk values between 30 and 150 tons per hectare per year were assigned score 5, while fields with potential soil loss between 5 and 10 tons per hectare per year were assigned score 2. Further, the optimization is based on the selection of patches that are derived from unique combinations of scores. However, the uneven abundance of fields with each score combination resulted in patches of strongly varying size. Since the optimization algorithm was limited to only choose 10 to 11% of the available area in patches, patch combinations could not be realized if they exceeded this limit.
We approximated the total soy yield, which serves as the basis for the area where soy can be allocated, from the average yield in Bavaria from 2015 to 2019. Locally, the soy yield could vary, leading to different outcomes in the total soy production. However, local yield data is not yet available for entire Bavaria. The optimized maps still suggest that a roughly similar allocation of soy production as currently grown can be accomplished by utilizing the average yield as a proxy. For the objectives, we used data specific to the region of Bavaria and, to our knowledge, the best available data to reach the level of complexity that fitted the aim of our study. The time periods of the input data can vary due to data availability and purpose of use. Other important factors for finding optimal locations for expanded soy cultivation like actual fertilizer application and observed farmland biodiversity can be included in the objectives if such data is available in further studies.
Potential land use conflicts caused by soy expansion
As a potential consequence of soy expansion, other crops can be displaced when soy is introduced to the rotation. According to the best single solutions and compromise solution from our optimization, winter wheat, grain maize, sugar beet, potatoes, and starch potatoes have high shares of their cultivation area selected for soy in the respective scenarios (Figure 7). In Bavaria, many factors determine the distribution of crops, among them are the plants' requirements. Grain maize and soy plants in general have similar demands on their cultivation region. Both depend on high temperature levels during germination and main growth period. The plants require sufficient water in order to develop flowers and fill grains (Geisler, 1980; Heyland, 1996; Diepenbrock et al., 2016). Consequentially, the distribution of grain maize is similar to the maximum suitability solution for soy cultivation from this optimization, leading to 42% of this crop being replaced by this solution. Sugar beet and starch potatoes appear to be cultivated to larger spatial extents in areas selected by all the best solutions and the compromise solution.
The high shares of displacement of certain crops is very relevant, if environmental consequences of different crop allocation scenarios are evaluated in global contexts (see Kreidenweis et al., 2016). In case of constant consumption levels, imports of particular crops could increase, leading to a translocation of its cultivation. In the case of maize, imports to Bavaria come mainly from Austria, France and the Netherlands, as well as from Hungary, Czech Republic and Poland, while potatoes and sugar beet are imported largely from France, Belgium and Austria (LfStaD, 2020). An increase in the crop cultivation in the respective countries can lead to various environmental problems. In Eastern Europe, maize cultivation could be enlarged due to higher potential for yield increase, as predicted in a modeling study by Schils et al. (2018). The authors warn against declining local water quality due to higher nitrogen fertilizer application from attempts to close the gap between actual and potential yield. In the Netherlands, intensive crop cultivation including potato and sugar beet can have detrimental effects on public health due to the involved pesticide application (Brouwer et al., 2017).
The potential change in cultivation pattern due to soy expansion to 10.7% of crop fields in Bavaria might also implicate shifts in the purpose of the field usage. Soy that is predominantly used for animal feed (European Commission, 2018), can replace crops like potatoes, sugar beet, and winter wheat that are mainly intended for human consumption. In Germany, sugar beet is mainly used for human consumption and industrial applications, while a small part of approximately 5% is used for bioenergy. Potatoes serve to 70% for human consumption, to 13% for industrial purposes, and to 3% for animal feed. Lastly, around 10% of wheat are used for bioenergy production, while approximately 38% of the total use go into animal feed and 39% into food production, leaving the remaining share for seed propagation and industrial applications (BMEL, 2020). Grain maize is essential for animal feed as 75–85% of pig feed components are grain maize and cereal products (LfL, 2021). Hence, expanded soy cultivation could introduce local conflicts between animal feed and human food production as well as shifts within feed production, from grain maize as roughage or energy component to soy as protein feed. The development of volume and distribution of these crops in case of soy expansion is tied to changes in consumption preferences (plant-based vs. animal foods) and trade patterns (domestic consumption vs. export). Soy itself could also be replaced by other grain legumes like faba bean, pea or lupins depending on land use policies directed at the whole food system, as well as future environmental and commercial attractiveness.
Support and barriers of soy expansion in Bavaria
The realization of expanded Bavarian soy cultivation in the future depends on various factors. On one hand, climate change might alter the spatial distribution of suitable areas for soy cultivation. According to various global crop models, climate change leads to reduced yield in todays' main producing countries like the United States and Brazil. Large yield gains up to 30% are projected for higher latitudes, including parts of China, as well as Austria, Hungary and Southern Germany (Jägermeyr et al., 2021). In these areas, soy is predicted to benefit both from higher temperatures and CO2 concentration due to climate change (Guilpart et al., 2020). At European level, simulations for soy production under climate change scenarios exhibit positive as well as negative effects with regional disparities (Mitter et al., 2015). In Bavaria, climate change has already improved the soy yield due to warmer temperatures according to the final report of the Bavarian crop production in 2020 (Bavarian Agricultural Report, 2020). However, yields are predicted to peak in the middle of the twenty-first century and decrease toward to end of the century (Jägermeyr et al., 2021). In order to establish successful soy production in Bavaria, the grain quality under climate change should also be tested and accounted for in projections. In the case of wheat, Asseng et al. (2018) presented model results that point to decreasing grain protein yield under climate change for unadapted varieties. In turn, breeding efforts and research on new varieties are an undeniable necessity to enable stable and high-quality soy returns in the future. This becomes even more clear when checking for the potential of adapted cultivars in major crop types. Compared to maize, wheat, and rice, soy yields could face the highest potential losses due to the lack of varieties that are suited for climate change (Zabel et al., 2021). For Bavaria, developments in soy breeding can firstly expand the range of suitable areas for soy cultivation (LfL, 2017). In addition, climate change is predicted to increase the pressure from weeds and diseases on soy. Other studies found diverse crop rotations to be more resistant to pests (Mitter et al., 2015), where soy could be a valuable addition to the crop rotation. Bearing in mind all these potential influences of future climate on the potentials for soy cultivation, repeating our analysis under climate change scenarios is certainly an interesting task for future studies.
Another factor in estimating the potential for expanded soy in Europe are farmers perspectives. According to surveys from different European countries, farmers perceive yield instability as a major constraint to enlarge their grain legume production. However, there is a lack of experience and knowledge about local prospects for legume cultivation (Zander et al., 2016). Since many ecosystem services are hard to monetize, the potential benefits from introducing legumes into crop systems are rarely evaluated by farmers (Reckling et al., 2016). The acknowledgment of the environmental benefits usually only follows after years of practice and shared knowledge within the peer group. If a higher share of legumes in the plantations is reached, cereals can be substituted and even the whole cropping system can be re-designed (Mawois et al., 2019).
On a political level, the strategies, declarations and pronounced plans from Germany and the EU support the aims of the Bavarian plant protein production. Existing and emerging proscriptions and payment schemes are shaping the prospects for legume cultivation as well (LfL, 2019a). On EU scale, protein plant cultivation is supported by different Common Agricultural Policy instruments. Nitrogen-fixing plants have been allowed to be grown on Ecological Focus Areas (EFA) in the past funding period and rural development measures as Agri-Environment-Climate Measures (AECM) support crop rotations if legumes are included. In addition, several countries like Croatia, Italy or Hungary have own policies in place (European Commission, 2018; Balázs et al., 2021). In Bavaria, two agri-environmental payment schemes reward the cultivation of soy since it is a flowering crop and a grain legume. Especially the establishment of soy as a diversifying crop could be carried forward with these subsidy payments (StMELF, 2018b, 2019b). In addition, the market situation in terms of high soy prices and rising sales of plant-based protein food influences the potential for soy expansion (Alandia et al., 2020). EU-scale policies increasingly stimulate plant-based diets for reasons of public health, food security, and environmental impact (Moschitz et al., 2021). Additionally, consumers in the EU are becoming increasingly aware of deforestation, animal welfare, and genetically modified feed and therefore endorse Non-GM fed and organic animal products (European Commission, 2018). The economic potential of soy from EU countries could grow, considering that 80% of the soy worldwide is genetically modified, but only Non-GM seeds are allowed in Germany and Austria, (LfL, 2020e). Lastly, the competitiveness of soy depends on the prices. For instance, soy can be competitive with wheat in Bavaria if the soy price is twice as large as the wheat price (Danube Soya Congress, 2013). The higher average costs for soy seeds are compensated by the fact that farmers have to spend less on fertilizer, pesticides and sometimes even on machine costs (LfL, 2017). The spatial distribution of soy would then follow mainly economic principles and allocate soy where the profit can be maximized. Hence, yield, market access, and trade conditions would determine the allocation of enhanced soy (Mauser et al., 2015). However, in order to make soy and other protein plants more competitive, the current patchwork of policies need to be streamlined and extended to include all relevant stakeholders (Alandia et al., 2020; Balázs et al., 2021). In Bavaria, as in Europe in total, soy is mainly used as feed for livestock farming (European Commission, 2018). However, a high amount of concentrate feed in animal rations competes with direct human food crop production due to a less effective protein conversion by ruminants compared to protein gain if humans were consuming the respective crops directly themselves (Wilkinson, 2011). Schader et al. (2015) showed that the global food system would be more sustainable if livestock was fed less food-competing feed. Additionally, Dentler et al. (2020) conclude that “high nutrient use efficiency, high resource use efficiency and high food production efficiency within dairy farming can be fostered by a more extensive farming system based on grassland, with low concentrate inputs.” With these aspects in mind, the target of self-sufficiency with soy at the current consumption level and export of dairy and meat products should be critically reflected.
In conclusion, the future of domestic protein supply for animal feed and human consumption is of interest for many scientific fields in the EU. With a focus on the conditions for a sustainable implementation, our research applied a spatial optimization approach to allocate soy cultivation to ecologically and biophysically desirable areas, quantified as areas with high climatic and soil suitability, low erosion risk, high need for reduced fertilizer input, and for diversification of crop rotation. We found synergies and trade-offs among our objectives and presented a compromise solution to achieve all of them to an acceptable degree. While the results are subject to the definition and selection of input data, the approach helps to delineate favorable regions where soy cultivation can be successfully and sustainably realized under appropriate local farming practices. Our discussion pointed toward the multiple factors on which domestic soy cultivation expansion depends in Bavaria. Those include the consequences of climate change, farmers' knowledge, political and economic settings, as well as feed management. Thus, protein plant production in Central Europe is a complex subject and requires more attention to solve the issues of sustainable nutrition in the future. Spatial optimization approaches like in this study can help to find locations that attain ecological objectives while assuring productivity from protein crop cultivation.
Data availability statement
The datasets analyzed in this study were inquired from public institutions for the purpose of this study. The points of contact for those datasets, as well as the generated data of this study, and R Scripts will be provided by the corresponding author upon reasonable request.
Author contributions
SG performed the data collection and data processing, the analysis of the optimization results, and writing. MH contributed the study idea, assisted with the processing of input data, and took part in the writing. CS took part in the scientific discussion, the placement of this study into the current knowledge, and editing the manuscript. AK contributed to the set-up and usage of CoMOLA, shared mathematical understanding of the optimization process, and took part in the writing. All authors contributed to the article and approved the submitted version.
Funding
This research is part of the LandKlif project (www.landklif.biozentrum.uni-wuerzburg.de) funded by the Bavarian Ministry of Science, Research and the Arts via the Bavarian Climate Research Network bayklif (www.bayklif.de). Additionally, this work was partly supported by the German Ministry for Education and Research (BMBF) funding the SUSALPS project (Sustainable use of alpine and pre-alpine grassland soils in a changing climate) [Grant Numbers: 031B0027C and 031B0516C]. It was also partly funded by the Netherlands Organization for Scientific Research (NWO) through the SusCrop ERA-Net project LegumeGap.
Acknowledgments
We would like to acknowledge the data providers (Wolfgang Janssen, German Meteorological Service - DWD; Robert Knöferl, Bavarian State Ministry of Food, Agriculture and Forestry - StMELF; Robert Schätzl, Bavarian State Research Center for Agriculture - LfL) for the processed climate data for soy suitability calculation, the fertilizer ordinance delimited areas, the soil map with expert judgement regarding soy cultivation, and the Bavarian State Ministry of Food, Agriculture and Forestry (StMELF) for the data access of the Integrated Administration and Control System (IACS), provided by the Bavarian State Research Center for Agriculture (LfL). In addition, we thank the Bavarian State Research Center for Agriculture (LfL), the Bavarian Ministry of Food, Agriculture and Forestry (StMELF) and the Center for Agricultural Technology Augustenberg (LTZ) for sharing their knowledge about soy cultivation in and imports to Germany, M. Versluis, R. Riebl and B. Uniyal (all Professorship of Ecological Services, Bayreuth Center of Ecology and Environmental Research, University of Bayreuth, Bayreuth, Germany) for their support in processing the IACS data, M. Strauch (Department of Computational Landscape Ecology, Helmholtz Centre for Environmental Research-UFZ, Leipzig, Germany) for his support with the tool CoMOLA, and T. Koellner (Professorship of Ecological Services, Bayreuth Center of Ecology and Environmental Research, University of Bayreuth, Bayreuth, Germany) as well as our reviewers for their helpful comments on the manuscript.
Conflict of interest
The authors declare that the research was conducted in the absence of any commercial or financial relationships that could be construed as a potential conflict of interest.
Publisher's note
All claims expressed in this article are solely those of the authors and do not necessarily represent those of their affiliated organizations, or those of the publisher, the editors and the reviewers. Any product that may be evaluated in this article, or claim that may be made by its manufacturer, is not guaranteed or endorsed by the publisher.
Supplementary material
The Supplementary Material for this article can be found online at: https://www.frontiersin.org/articles/10.3389/fsufs.2022.916003/full#supplementary-material
References
Alandia, G., Pulvento, C., Sellami, M.H., Hoidal, N., Anemone, T., Nigussie, E., et al. (2020). “Grain legumes may enhance high-quality food production in Europe”. in Emerging Research in Alternative Crops, eds, A. Hirich, R. Choukr-Allah, R. Ragab (Environment and Policy, Springer, Cham), 25–53. doi: 10.1007/978-3-319-90472-6_2
Asseng, S., Martre, P., Maiorano, A., Rötter, R. P., O'Leary, G. J., Fitzgerald, G. J., et al. (2018). Climate change impact and adaptation for wheat protein. Glob. Change Biol. 25, 155–173. doi: 10.1111/gcb.14481
Balázs, B., Kelemen, E., Centofanti, T., Vasconcelos, M.W., and Iannetta, P.P.M. (2021). Integrated policy analysis to identify transformation paths to more sustainable legume-based food and feed value-chains in Europe. Agroecol. Sustain. Food Syst. 45, 931–953. doi: 10.1080/21683565.2021.1884165
Bavarian Agricultural Report (2020). Bayerischer Pflanzenspiegel. Available online at: https://www.lfl.bayern.de/mam/cms07/ipz/dateien/lfl_bayerischer-pflanzenbauspiegel-2020.pdf (accessed November 28, 2020).
BMEL (2020). Bundesministerium für Ernährung und Landwirtschaft. Available online at: https://www.bmel-statistik.de/tabellen-finden/suchmaske/?stw=Versorgung (accessed October 20, 2020).
Boerema, A., Peeters, A., Swolfs, S., and Vandevenne, F. (2016). Soybean trade: balancing environmental and socio-economic impacts of an intercontinental market. PLoS ONE. 11, e0155222. doi: 10.1371/journal.pone.0155222
Brouwer, M., Kromhout, H., Vermeulen, R., Duyzer, J., Kramer, H., Hazeu, G., et al. (2017). Assessment of residential environmental exposure to pesticides from agricultural fields in the Netherlands. J. Expo. Sci. Environ. Epidemiol. 28,173–181. doi: 10.1038/jes.2017.3
Danube Soya Congress (2013). Micro economics of soya production—soya bean cultivation under the aspect of crop rotation and economy. Available online at: https://www.donausoja.org/fileadmin/user_upload/DS_Congress/Congress_2013/Presentations_2013/Day_1_Forum_I/Schaetzl_Halama-Micro_Economics_of_Soya_Production_DS2013.pdf (accessed September 10, 2020).
de Visser, C. L. M., Schreuder, R., and Stoddard, F. (2014). The EU's dependency on soya bean import for the animal feed industry and potential for EU produced alternatives. Oilseeds and fats, Crops and Lipids. 21, D407. doi: 10.1051/ocl/2014021
Deb, K., Pratap, A., Agarwal, S., and Meyarivan, T. (2002). A fast and elitist multiobjective genetic algorithm: NSGA-II. IEEE Trans. Evol. Comput. 6, 182–197. doi: 10.1109/4235.996017
Dentler, J., Kiefer, L., Hummler, T., Bahrs, E., and Elsaesser, M. (2020). The impact of low-input grass-based and high-input confinement-based dairy systems on food production, environmental protection and resource use. Agroecol. Sustain. Food Syst. 44, 1089–1110. doi: 10.1080/21683565.2020.1712572
Diepenbrock, W., Ellmer, F., and Léon, J. (2016). Ackerbau, Pflanzenbau und Pflanzenzüchtung. 4 Edition. Ulmer, Stuttgart: UTB GmbH. doi: 10.36198/9783838546070
DonauSoja (2022). DonauSoja. Available online at: https://www.donausoja.org/en/home/ (accessed March 4, 2022).
Eder, J., Gehring, K., Urbatzka, P., Schätzl, R., Heigl, L., and Schäffler, M. (2019). Eiweißpflanze Soja. Erfolgreich in Bayern produzieren. Available online at: https://www.lfl.bayern.de/mam/cms07/publikationen/daten/informationen/eiweisspflanze-soja_lfl-information.pdf (accessed December 10, 2021).
EU Regulation (2014). Commission Implementing Regulation (EU) No 809/2014. Available online at: https://eur-lex.europa.eu/legal-content/en/ALL/?uri=CELEX%3A02014R0809-20191101 (accessed November 5, 2021).
European Commission (2018). Report from the Commission to the Council to the European Parliament on the development of plant proteins in the European Union.
Execution ordinance AVDüV (2018). Ausführungsverordnung Düngeverordnung GVBl. S. 722, BayRS 7820-1-L. Available online at: https://www.gesetze-bayern.de/Content/Document/BayAVDueV/true?AspxAutoDetectCookieSupport=1 (accessed June 9, 2020).
Fertilizer ordinance. (2020). Düngeverordnung. Verordnung über die Anwendung von Düngemitteln, Bodenhilfsstoffen, Kultursubstraten und Pflanzenhilfsmitteln nach den Grundsätzen der guten fachlichen Praxis beim Düngen (BGBl. I S. 1305).
Fischer, G., Nachtergaele, F.O., van Velthuizen, H.T., Chiozza, F., Franceschini, G., Henry, M., et al. (2021). Global Agro-Ecological Zones v4 – Model documentation. Rome: FAO. doi: 10.4060/cb4744en
GAEZ. (2022). GAEZ v4 Data Portal. Global Agro-Ecological Zones. Available online at: https://gaez-services.fao.org/apps/theme-4/ (accessed June 9, 2022).
Geisler, G. (1980). Pflanzenbau: Ein Lehrbuch – Biologische Grundlagen und Technik der Pflanzenproduktion, Parey, Berlin, Hamburg.
Guilpart, N., Iizumi, T., and Makowski, D. (2020). Data-driven yield projections suggest large opportunities to improve Europe's soybean self-sufficiency under climate change. bioRxiv. doi: 10.1101/2020.10.08.331496
Isbell, F., Adler, P.R., Eisenhauer, N., Fornara, D., Kimmel, K., et al. (2017). Benefits of increasing plant diversity in sustainable agroecosystems. J. Ecol. 105, 871–879. doi: 10.1111/1365-2745.12789
Jägermeyr, J., Müller, C., Ruane, A. C., Elliott, J., Balkovic, J., Castillo, O., et al. (2021). Climate impacts on global agriculture emerge earlier in new generation of climate and crop models. Nat. Food. 2, 873–885. doi: 10.1038/s43016-021-00400-y
Kaim, A., Strauch, M., and Volk, M. (2020). Using Stakeholder Preferences to Identify Optimal Land Use Configurations. Front. Water. 2, 579087. doi: 10.3389/frwa.2020.579087
Karges, K., Bellingrath-Kimura, S.D., Watson, C.A., Stoddard, F.L., Halwani, M., and Reckling, M. (2022). Agro-economic prospects for expanding soybean production beyond its current northerly limit in Europe. Eur. J. Agron. 133, 126415. doi: 10.1016/j.eja.2021.126415
Kistler, M., Brandhuber, R., and Maier, H. (2013). Wirksamkeit von Erosionsschutzmaßnahmen. Ergebnisse einer Feldstudie. Bericht zum Forschungsvorhaben, “Evaluierung der Cross Compliance Bestimmungen zum Erosionsschutz in Bayern.” Schriftenreihe der Bayerischen Landesanstalt für Landwirtschaft.
Kormann, U., Rösch, V., Batáry, P., Tscharntke, T., Orci, K.M., Samu, F., et al. (2015). Local and landscape management drive trait-mediated biodiversity of nine taxa on small grassland fragments. Diversity and Distributions. 21, 1204–1217. doi: 10.1111/ddi.12324
Kreidenweis, U., Lautenbach, S., and Koellner, T. (2016). Environmental Modelling and Software Regional or global? The question of low-emission food sourcing addressed with spatial optimization modelling. Environ. Model. Softw. 82, 128–141. doi: 10.1016/j.envsoft.2016.04.020
LfL (2017). Wettbewerbsfähigkeit der Sojabohne gegenüber Vergleichsfrüchten 2017. Bayerische Landesanstalt für Landwirtschaft. Available online at: https://www.lfl.bayern.de/mam/cms07/iba/dateien/wettbewerbsf%C3%A4higkeit_der_sojabohne_gegen%C3%BCber_vergleichsfr%C3%BCchten_2017.pdf (accessed July 6, 2020).
LfL (2019a). Bayerische Eiweißinitiative: Zwischenbilanz 2017. Bayerische Landesanstalt für Landwirtschaft. Available online at: https://www.lfl.bayern.de/schwerpunkte/eiweissstrategie/189581/index.php (accessed February 18, 2022).
LfL (2019b). Anbauflächenentwicklung von Leguminosen 2019. Bayerische Landesanstalt für Landwirtschaft. Available online at: https://www.lfl.bayern.de/schwerpunkte/eiweissstrategie/225206/index.php (accessed September 17, 2021).
LfL (2019c). Anbaueignung für Sojabohnen in Bayern. Bayerische Landesanstalt für Landwirtschaft. Available online at: https://www.lfl.bayern.de/iba/pflanze/119165/index.php (accessed August 24, 2020).
LfL (2020a). Die Bayerische Eiweißinitiative. Bayerische Landesanstalt für Landwirtschaft. Available online at: https://www.lfl.bayern.de/schwerpunkte/eiweissstrategie/121662/index.php (accessed June 15, 2020).
LfL (2020b). Erosionsschutz-App: Erosionsschutz-Planung mit Hilfe der “ABAG interaktiv”. Bayerische Landesanstalt für Landwirtschaft. Available online at: https://www.lfl.bayern.de/iab/boden/203640/index.php (accessed June 27, 2020).
LfL (2020c). Düngeverordnung. Bayerische Landesanstalt für Landwirtschaft. Available online at: https://www.lfl.bayern.de/iab/duengung/032364/ (accessed: July 12, 2020).
LfL (2020d). Ausführungsverordnung DüV–rote Gebiete, gelbe Gebiete. Bayerische Landesanstalt für Landwirtschaft. Available online at: https://www.lfl.bayern.de/iab/duengung/207027/index.php (accessed July 4, 2020).
LfL (2020e). Sojabohne-Standortansprüche und Sortenwahl. Bayerische Landesanstalt für Landwirtschaft. Available online at: https://www.lfl.bayern.de/ipz/oelfruechte/062694/index.php (accessed May 23, 2020).
LfL (2021). Futtermittel für Schweine/Bewertungssysteme. Bayerische Landesanstalt für Landwirtschaft. Available online at: https://www.lfl.bayern.de/ite/schwein/036198/index.php (accessed: March 4, 2022).
LfStaD (2020). Statistik Außenhandel. Bavarian State Office of Statistics and Data Processing. Available online at: https://www.statistikdaten.bayern.de/genesis/online/data?operation=statisticandlevelindex=0andlevelid=1606723529604andcode=51000 (accessed October 30, 2021).
LTZ (2018). Soja in Wasserschutzgebieten. Landwirtschaftliches Technologiezentrum Augustenberg. Available online at: https://ltz.landwirtschaft-bw.de/pb/site/pbs-bw-mlr/get/documents_E-1400771619/MLR.LEL/PB5Documents/ltz_ka/Arbeitsfelder/Landwirtschaft%20und%20Umwelt/Wasserschutz/Wasserschutzgebiete/Soja%20im%20Wasserschutzgebiet.%20Herbstnitratgehalte%20nach%20dem%20Anbau%20von%20Soja.pdf (accessed November 22, 2021).
LTZ (2019). Optimierung des Anbaus von Sojabohnen: Bestimmung des Vorfruchtwertes und der N2-Fixierungsleistung sowie Reduzierung der Bodenbearbeitung. Landwirtschaftliches Technologiezentrum Augustenberg. Available online at: https://orgprints.org/id/eprint/36906/1/36906-14EPS019_020-ltz-bwl-butz-2019-sojaanbau.pdf (accessed November 22, 2021).
LTZ (2020). Hinweise zum Pflanzenbau. Landwirtschaftliches Technologiezentrum Augustenberg. Available online at: https://ltz.landwirtschaft-bw.de/pb/site/pbs-bw-mlr/get/documents_E1193327853/MLR.LEL/PB5Documents/ltz_ka/Arbeitsfelder/Eiwei%C3%9Fpflanzen/Anbauanleitungen/Hinweise%20zum%20Pflanzenbau_Ackerbohne_2020.pdf (accessed November 22, 2021).
Mauser, W., Klepper, G., Zabel, F., Delzeit, R., Hank, T., Putzenlechner, B., et al. (2015). Global biomass production potentials exceed expected future demand without the need for cropland expansion. Nat. Commun. 6, 8946. doi: 10.1038/ncomms9946
Mawois, M., Vidal, A., Revoyron, E., Casagrande, M., Jeuffroy, M.-H., and Le Bail, M. (2019). Transition to legume-based farming systems requires stable outlets, learning, and peer-networking. Agron. Sustain. Dev. 39, 14. doi: 10.1007/s13593-019-0559-1
Mitter, H., Schmid, E., and Sinabell, F. (2015). Integrated modelling of protein crop production responses to climate change and agricultural policy scenarios in Austria. Clim. Res. 65, 205–220. doi: 10.3354/cr01335
Moschitz, H., Muller, A., Kretzschmar, U., Haller, L., de Porras, M., Pfeifer, C., et al. (2021). How Can the EU Farm to Fork Strategy Deliver on Its Organic Promises? Some critical reflections. EuroChoices. 20, 30–36. doi: 10.1111/1746-692X.12294
Nemecek, T., von Richthofen, J.S., Dubois, G., Casta, P., Charles, R., and Pahl, H. (2008). Environmental impacts of introducing grain legumes into European crop rotations. Eur. J. Agron. 28, 380–393. doi: 10.1016/j.eja.2007.11.004
Pistrich, K., Wendtner, S., and Janetschek, H. (2014). Versorgung Österreichs mit pflanzlichem Eiweiß – Fokus Sojakomplex. Endbericht des Projektes Nr. AWI/167/09 “Versorgungssicherheit mit pflanzlichem Eiweiß in Österreich” Schriftenreihe 107 der Bundesanstalt für Agrarwirtschaft, Wien.
Reckling, M., Bergkvist, G., Watson, C. A., Stoddard, F. L., Zander, P. M., Walker, R. L., et al. (2016). Trade-Offs between Economic and Environmental Impacts of Introducing Legumes into Cropping Systems. Front. Plant Sci. 7, 669. doi: 10.3389/fpls.2016.00669
Rossberg, D., and Recknagel, J. (2017). Untersuchungen zur Anbaueignung von Sojabohnen in Deutschland. Journal für Kulturpflanzen. 69, 137–145. doi: 10.1399/JfK.2017.04.02
Sans, P., and Combris, P. (2015). World meat consumption patterns: An overview of the last fifty years (1961–2011). Meat Sci. 109, 106–111. doi: 10.1016/j.meatsci.2015.05.012
Schader, C., Muller, A., El-Hage Scialabba, N., Hecht, J., Isensee, A., Erb, K.-H., et al. (2015). Impacts of feeding less food-competing feedstuffs to livestock on global food system sustainability. J. R. Soc. Interface. 12, 20150891. doi: 10.1098/rsif.2015.0891
Schäfer, W., Sbresny, J., and Thiermann, A. (2017). Methodik zur Einteilung von landwirtschaftlichen Flächen nach dem Grad ihrer Erosionsgefährdung durch Wassergemäß § 6Abs. 1 der Agrarzahlungen-Verpflichtungenverordnung in Niedersachsen. Landesamt für Bergbau, Energie und Geologie.
Schätzl, R., Maier, H., Janssen, W., Halama, M., and Aigner, A. (2015). LfL (Hrsg.) Anbaueignung für Sojabohnen in Bayern. Tagungsband Soja-Tagung 2015 in Freising. Available online at: https://lfl.bayern.de/mam/cms07/iba/dateien/anbaueignung_f%C3%BCr_sojabohnen_in_bayern__tagungsbandbeitrag_.pdf (accessed April 2, 2022).
Schils, R., Olesen, J.E., Kersebaum, K-C., Rijk, B., Oberforster, M., Kalyada, V., et al. (2018). Cereal yield gaps across Europe. Eur. J. Agron. 101, 109–120. doi: 10.1016/j.eja.2018.09.003
Smith, R.G., Gross, K.L., and Robertson, G.P. (2008). Effects of crop diversity on agroecosystem function: Crop yield response. Ecosystems. 11, 355–366. doi: 10.1007/s10021-008-9124-5
Sojaförderring (2022). Available online at: https://www.sojafoerderring.de/ (accessed January 8, 2022).
StMELF (2018a). Weniger Soja-Importe aus Übersee. Bayerisches Staatsministerium für Ernährung, Landwirtschaft und Forsten. Available online at: https://www.StMELF.bayern.de/service/presse/pm/2018/197802/index.php (accessed June 4, 2020).
StMELF (2018b). Merkblatt Agrarumwelt- und Klimamaßnahmen. Bayerisches Staatsministerium für Ernährung, Landwirtschaft und Forsten. Available online at: https://www.StMELF.bayern.de/mam/cms01/agrarpolitik/dateien/m_aum_verpflichtungszeitraum_2019_2023.pdf (accessed July 4, 2020).
StMELF (2019a). Bayerische Eiweißinitiative. Bayerisches Staatsministerium für Ernährung, Landwirtschaft und Forsten. Available online at: http://www.StMELF.bayern.de/agrarpolitik/001128/ (accessed July 14, 2020).
StMELF (2019b). Kulturenliste. Bayerisches Staatsministerium für Ernährung, Landwirtschaft und Forsten. Available online at: https://www.StMELF.bayern.de/mam/cms01/agrarpolitik/dateien/kulap_b43_kulturenliste.pdf (accessed, July 3 2020).
StMELF (2020a). Agriculture and Forestry in Bavaria-Graphics and Tables 2020. Bayerisches Staatsministerium für Ernährung, Landwirtschaft und Forsten. Available online at: https://www.stmelf.bayern.de/agrarpolitik/daten_fakten/index.php (accessed December 14, 2021).
StMELF (2020b). LfL-Deckungsbeiträge und Kalkulationsdaten–Sojabohnen. Bayerisches Staatsministerium für Ernährung, Landwirtschaft und Forsten. Available online at: https://www.stmelf.bayern.de/idb/sojabohne.html (accessed June 28, 2022).
StMUV (2021). Bayerns Klima im Wandel. Heute und in der Zukunft. Bayerisches Staatsministerium für Umwelt und Verbraucherschutz. Available online at: https://www.bestellen.bayern.de/application/applstarter?APPL=eshopandDIR=eshopandACTIONxSETVAL(artdtl.htm,APGxNODENR:1325,AARTxNR:lfu_klima_00173,AARTxNODENR:358787,USERxBODYURL:artdtl.htm,KATALOG:StMUG,AKATxNAME:StMUG,ALLE:x)=X (accessed February 18, 2022).
Strauch, M., Cord, A.F., Pätzold, C., Lautenbach, S., Kaim, A., et al. (2019). Environmental Modelling and Software Constraints in multi-objective optimization of land use allocation–repair or penalize? Environ. Model. Softw. 118, 241–251. doi: 10.1016/j.envsoft.2019.05.003
Tscharntke, T., Grass, I., Wanger, T.C., Westphal, C., and Batáry, P. (2021). Beyond organic farming–harnessing biodiversity-friendly landscapes. Trends Ecol. Evol. 36, 919–30. doi: 10.1016/j.tree.2021.06.010
UBA (2010). Die Böden Deutschlands. Sehen, Erkunden, Verstehen. Ein Reiseführer. Umweltbundesamt. Available online at: https://www.umweltbundesamt.de/sites/default/files/medien/publikation/long/4161.pdf (accessed December 14, 2021).
UBA (2022). Erosion. Umweltbundesamt. Available online at: https://www.umweltbundesamt.de/en/topics/soil-agriculture/land-a-precious-resource/erosion#what-are-the-consequences-of-water-erosion (accessed January 17, 2022).
van der Plas, F., Allan, E., Fischer, M., Alt, F., Arndt, F., Binkenstein, J., et al. (2019). Towards the Development of General Rules Describing Landscape Heterogeneity–Multifunctionality Relationships. J. Appl. Ecol. 56, 168–179. doi: 10.1111/1365-2664.13260
Watson, C.A., Reckling, M., Preissel, S., Kuhlman, T., Nemecek, T., et al. (2017). Grain legume production and use in European agricultural systems. Adv. Agron. 144, 235–303. doi: 10.1016/bs.agron.2017.03.003
Wilbois, K.-P., Spiegel, A.-K., Asam, L., Balko, C., Becker, H., et al. (2014). Ausweitung des Sojaanbaus in Deutschland durch züchterische Anpassung sowie pflanzenbauliche und verarbeitungstechnische Optimierung. Bundesprogramm Ökologischer Landbau und andere Formen nachhaltiger Landwirtschaft.
Wilkinson, J.M. (2011). Re-defining efficiency of feed use by livestock. Animal. 5, 1014–1022. doi: 10.1017/S175173111100005X
Zabel, F., Delzeit, R., Schneider, J., Seppelt, R., Mauser, W., and Václavík, T. (2019). Global impacts of future cropland expansion and intensification on agricultural markets and biodiversity. Nat. Commun. 10, 2844. doi: 10.1038/s41467-019-10775-z
Zabel, F., Müller, C., Elliott, J., et al. (2021). Large potential for crop production adaptation depends on available future varieties. Glob. Change Biol. 27, 3870–3882. doi: 10.1111/gcb.15649
Zabel, F., Putzenlechner, B., and Mauser, W. (2014). Global agricultural land resources – a high resolution suitability evaluation and its perspectives until 2100 under climate change conditions. PLoS ONE. 9, e107522. doi: 10.1371/journal.pone.0114980
Keywords: soy cultivation, multi-objective optimization, spatial land-use allocation, trade-off analysis, ecosystem services
Citation: Gebhardt S, Haensel M, Schulp CJE and Kaim A (2022) Ecologically and biophysically optimal allocation of expanded soy production in Bavaria, Germany. Front. Sustain. Food Syst. 6:916003. doi: 10.3389/fsufs.2022.916003
Received: 08 April 2022; Accepted: 25 July 2022;
Published: 17 August 2022.
Edited by:
Matteo Dainese, Eurac Research, ItalyReviewed by:
Florian Zabel, Ludwig Maximilian University of Munich, GermanyQiangyi Yu, Chinese Academy of Agricultural Sciences (CAAS), China
Copyright © 2022 Gebhardt, Haensel, Schulp and Kaim. This is an open-access article distributed under the terms of the Creative Commons Attribution License (CC BY). The use, distribution or reproduction in other forums is permitted, provided the original author(s) and the copyright owner(s) are credited and that the original publication in this journal is cited, in accordance with accepted academic practice. No use, distribution or reproduction is permitted which does not comply with these terms.
*Correspondence: Swantje Gebhardt, cy5nZWJoYXJkdEB1dS5ubA==