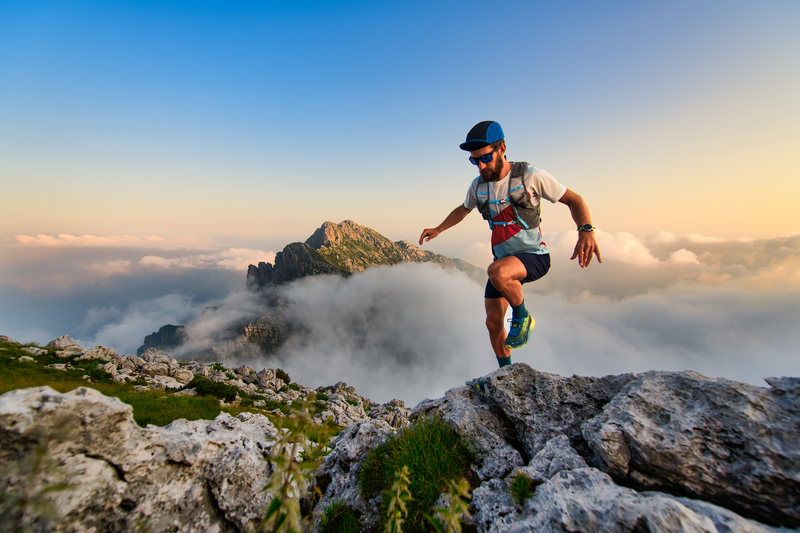
94% of researchers rate our articles as excellent or good
Learn more about the work of our research integrity team to safeguard the quality of each article we publish.
Find out more
POLICY AND PRACTICE REVIEWS article
Front. Sustain. Food Syst. , 19 October 2022
Sec. Social Movements, Institutions and Governance
Volume 6 - 2022 | https://doi.org/10.3389/fsufs.2022.903230
This article is part of the Research Topic Law, Policy and the Governance of Sustainable Food Systems View all 8 articles
With the increasing use of precision agriculture and technological development, the agricultural sector has been majorly transformed. Precision agriculture uses technological innovations such as sensors, drones, and data analysis tools to improve the productivity of resources and management decisions on the farm. Since these technologies collect a large amount of data related to the farm, the farmers are concerned about the privacy of their data. The farmers are worried about unauthorized access, collection, and sharing of their data with third parties by the agricultural technology providers (ATPs). Furthermore, the ambiguity of agreements and legal frameworks around data collection, processing, and sharing may result in uncertainty in data privacy practices. Furthermore, this situation is aggravated by a lack of adoption of best practices and standards for farm data protection. Violation of privacy can cause reluctance among farmers to adopt new technologies which can negatively impact various stakeholders, government, and public. Protecting farmers' privacy and respecting their rights related to the collected data should be addressed collectively by the actors in the farming ecosystem, including farmers, agricultural technology providers, governments, and supply chain stakeholders. This paper aims at providing recommendations on how to minimize privacy risks and concerns for farmers and reviews some of the data governance best practices for data protection.
With the recent technological advancements, farming has been significantly transformed by the adoption of so-called precision agriculture. Precision agriculture uses innovative technology such as sensors, drones, robots, precision machinery, and GPS technology to help farmers increase productivity, sustainability, and profitability (Monteiro et al., 2021). These digital agricultural technologies use large amounts of data from multiple sources to improve the use of farming resources such as fertilizers, pesticide applications, and livestock health and welfare. Farmers use measurement tools provided by precision agriculture technologies to enhance farm-related decision-making. For example, on a big farm, the soil nutrients may vary in each section of the farm, and if a farmer uses the same fertilizing procedure for the entire field, it can be more costly and environmentally harmful. This can be addressed by collecting soil health-related data and analyzing them to make better management decisions related to the use of resources such as fertilizers and water (Caria et al., 2017). The use of technologies in livestock farming has also been growing. Livestock technology helps in improving the welfare and management of livestock animals on a farm. For example, implementing automated feeding systems can provide cost-effective decisions by monitoring the duration of feed, electronic identification of each animal, and measuring the weight of feed consumed (Monteiro et al., 2021).
Data is the core component of digital agricultural technologies. Digital technologies collect, store, integrate, and analyze farm data to predict an event, recommend a solution, build automated tools such as robots to make an automated decision or take an action, or guide farmers to make more informed decisions. To turn farm data into effective decision making, statistical analysis and Artificial Intelligence (AI) models are used (Monteiro et al., 2021). Many of these technologies are data intensive and require large amounts of data to operate accurately and reliably. Massive data collection raises privacy risks for farmers. Privacy risks vary from identification, reputation loss, misuse of data, lack/limited control over data, social engineering, and unauthorized access to data (Wiseman et al., 2019; Linsner et al., 2021). Information privacy has many different definitions which cover technical and process aspects of data processing. But, in general, the objective of data privacy is to protect misuse of data, prevent unauthorized access to data, and enforce greater control of personal data for the individual.
The data collected from the farms can be broadly grouped into two categories: farm data and personal data. Farm data can include information such as crop data, livestock data, and machine data. Personal data can include personal data related to farmers such as name, email, and location. Personal data can be categorized into two groups: data that can make farmers directly identifiable, such as name and location, or indirectly identifiable such as a combination of farm activities and information about crop and livestock animals. With the substantial amounts of data that are being collected from farms, there is a growing concern about farmers' privacy and farm data protection practices. Generally, farmers have limited control of their farm data by ATPs. This raises concerns about privacy of farm data. Privacy is defined as the right of an individual to control or influence what information related to them is collected, how that data are stored and used, and with whom they are shared or disclosed (Linsner et al., 2021). However, farmers are usually not informed about the purpose of data collection from the farm, how their data is used, and whether their data is shared with the third parties (Linsner et al., 2021). Due to the lack of control and lack of transparency, farmers are unwilling to share their data with ATPs.
Many privacy regulations have been developed for the protection of personal information and preserving individuals' privacy. In most of the privacy regulations, personal information refers to any information related to an identified or identifiable person (Schuster, 2017). Personal information or Personally Identifiable Information (PII) such as name, email, location, or any other information that can disclose the individuals' identity, are the subject of privacy legislation. Some of these regulations such as the General Data Protection Regulation (GDPR) (Schuster, 2017), established European Union (EU), are comprehensive in nature and protect personal information irrespective of the application domain. This may include farming data. However, some other regulations are formulated for the protection of personal information that is collected by commercial businesses and do not protect personal information or PII collected in the farming or agricultural sectors such as California Consumer Privacy Act (CCPA) and Personal Information Protection and Electronic Documents Act in Canada.
With the lack of specific regulations or standards related to farm data (Kaur et al., 2018), farmers have become even more concerned about their privacy. One of the major privacy risks is the identification of farmers which may result in the disclosure of sensitive data without their consent, identity theft, or reputation loss. The identification of farmers can occur through direct identifiers (e.g. name, email address, and location) and indirect identifiers such as PII data related to farm practices. For instance, farm data such as data related to crops, soil type, fertilization strategies, and water consumption may not show the farmers' identity directly. However, using statistical analysis and AI techniques may reveal unique patterns for farm practices that can be traced back to a specific farm and make the farmers identifiable (9). These types of data are referred to as PII. Personally Identifiable Information in the farming sector can potentially include any representation, combination, and analysis of farm data that can disclose farmers' identity.
Furthermore, farmers are concerned about unauthorized access to their farm data. Agricultural technology providers may share their farm data with other technology providers or stakeholders in the supply chain without farmers' consent and awareness. Moreover, the farmers are usually not notified about how their data is collected, used, accessed, or disclosed by the ATPs. These concerns and lack of transparency in how farm data is utilized can result in a loss of trust which may lead to farmers' reluctance to share data with the ATPs (Linsner et al., 2021).
There are existing technical and legal privacy standards and practices that are being used in other domains such as healthcare and e-commerce. However, the adoption of these standards and practices by ATPs and other agriculture stakeholders has not been as extensive as it should have been. This paper provides practical recommendations on how farm data can be protected by different actors' groups in the farming ecosystem. The main stakeholders that contribute to farm data protection can be grouped into four categories: farmers, ATPs, government, and other stakeholders in the food supply chain (Figure 1). Supply chain stakeholders can include processors, transporters, producers, distributors, retailers, farmers' associations, and any organizations that have access to farm technologies. This paper intends to suggest legal, technological, and human-centered recommendations to protect the privacy of farm data and preserve the confidentiality of farmers.
Data is a valuable resource for effective decision-making for farmers. However, if large volumes of farm data are not effectively managed, it can pose privacy risks to the farmers. These risks can be partly mitigated by farmers by taking proactive measures that are cost-effective. This section provides some practical guidelines for the farmers on how to protect privacy and confidentiality of their data.
Data-driven technologies are becoming more complex and connected. To ensure the privacy of the farmers' sensitive data that are collected, stored, and utilized by these technologies (Linsner et al., 2021), farmers must advance their digital literacy. This means that farmers should keep themselves up to date with technological advancements to be able to effectively protect their data. There are organizations or agri-cooperatives that provide educational programs and training for farmers so that farmers can enhance their digital skills. For example, in the EU, COPA-COGECA provides training to farmers to enhance digital skills that are required for digital transformation in rural areas and to make farmers aware of their rights and responsibilities in the digital era. Furthermore, farmers can learn more advanced technical approaches for data integration and analysis (Wilson, 2018). This will result in farmers retaining more control over their data by keeping and using data locally/on-farm.
Moreover, farmers can create a set of guidelines that can be followed by the farm workers for protecting farm data. A data protection guideline is an internal document used in an operation, e.g. farm, to set standards for protecting sensitive data. A simple approach to this can be, for example, to provide a set of practices in the form of a checklist for farm staff. Another strategy is training farm workers about farm data protection practices. This exercise is not limited to the farm workers that directly use farm data; all farmworkers that have access to digital devices and computer systems on the farm need to be trained. Data breaches occur due to unauthorized access to sensitive data which can happen in many ways including opening a phishing email, visiting an untrustworthy website, or easy access to a device by an intruder or an internal staff with malicious intent. Farmers and farmworkers should learn how to take preventive measures such as using strong passwords, updating software, and backups, and detecting and avoiding phishing emails to ensure protection from such threats. All farm staff should also have unique and valid credentials to access the computing infrastructure at farms.
Legal agreements are also important in protecting farm data. Studies show that 74% of the farmers are not aware of the terms of use and data license agreements (Wiseman et al., 2019). In fact, 55% of farmers have declared that they sign data contracts with ATPs without seeking clarifications on-farm data usage, sharing, and other important terms related to data protection and use (Schuster, 2017). It is highly recommended that farmers read data and terms of use agreements before accepting them and seek clarification for any unclear or ambiguous practices. Important data practices that require attention are data collection, data sharing, data security, data retention, data control, data access and portability, and data erasure. Table 1 lists the questions that farmers can ask the ATPs regarding their farm data collection, use, and protection. These questions are derived from regulations, privacy management best practices, and comprehensive research that has been performed on these legal documents (OECD., 2013; Guntamukkala et al., 2015; GDPR., 2018; Kaur et al., 2018; Office of the Privacy Commissioner of Canada., 2018; Wiseman et al., 2019). For instance, some regulations such as GDPR have provided comprehensive sets of recommendations on the content of privacy policies and data agreements. Furthermore, agriculture codes of conduct have also commented on what farmers should expect in the ATPs data agreements.
The lack of standardized data protection practices in farming has resulted in inconsistent legal data and use agreements created by ATPs (Linsner et al., 2021). This deficiency has resulted in farmers' lack or limited control of their data. Research also shows that farm' data are shared with third parties without farmers' consent. This is due to the power imbalance between farmers and ATPs which has led to the farmers' inability to negotiate stronger control of their farm data. This power imbalance emphasizes the need for farmers to educate themselves about the legal terms and data practices that are included in the data agreements to understand how their data is used and shared and how long their data is retained and stored. Farmers should inquire about their options, e.g., opt-in or opt-out of the data agreements and ATPs' service, before signing the contract. Additionally, farmers can seek clarification about regulations that apply to their farm data. Privacy laws depend on the regulatory regimes in a region.
Since most of the privacy policies and terms of use agreements use legal terminology, it can be difficult for the farmers to fully understand the content and learn about ATPs data practices (Guntamukkala et al., 2015; Kaur et al., 2018). Therefore, it is recommended that farmers seek professional help to better understand legal terminology and data protection/usage methodologies included in the documents (Audich et al., 2021). Seeking legal advice can flag ambiguous terms in the agreements. For instance, some ATPs may state that “you own the data” in the agreement. This statement may give the impression that farmers have the right to control with whom and under what condition farm data is shared. However, a privacy expert may realize that data ownership does not lend itself to greater control due to other conflicting and ambiguous data sharing statements, e.g. “we will share your farm data with our partners to enhance your experience.” Such legal services exist in some regions. Janzen Agricultural Law LLC, for instance, is a United States non-profit firm that reviews and validates terms and data practices in farm data agreements (McIntosh, 2018) and informs farmers about potential concerns (Guntamukkala et al., 2015). Ag Data Transparent also offers a certification program in which legal agreements get a transparency seal if they abide by the core principles of the Privacy and Security Principles for Farm Data (Ag Data Transparent., 2022). Farmers can use such services to check farm data and terms of use agreements to make an informed decision about the ATPs service and their rights.
Farmers should also safeguard farm data with apply security practices. One approach is enabling data encryption. Encryption is a process in which data are converted into encoded information, and they can only be decoded using a unique decryption key. Encryption helps ensure the integrity of data by protecting it from unauthorized access. Farmers can ask ATP to encrypt data end-to-end. De-identification/anonymization of data can be enabled by ATPs if the data is transferred and processed out of the farm. This approach removes identifiable information such as name, location, email or even PII if known in advance from farm data before the data is transferred, stored in the cloud, or shared with other partners (IAPP., 2022). It is also possible to fully anonymize farm data at the source so that the identity of farmers is not revealed when integrated with other data sources or when processed.
Farmers can inquire about data retention practices that ATP use. For instance, how long the ATP keeps the data or what happens to farm data when farmers terminate their service with ATP. Farmers can ask about their ability to access and download their farm data. Finally, farmers can select ATPs that have strong privacy and security protocols if there are multiple service providers that they can choose from.
Agricultural technology providers collect, process, or govern enormous amounts of farm data to enhance efficiency in farm operations (Yaqot and Menezes, 2021; Yaqot et al., 2021). Agricultural technology providers role as stewards of data and technology and their ability to control farm IT assets enable them to perform a critical role in protecting farm data and building trust with the farmers. This section provides recommendations to ATPs on how they can contribute to the protection of farm data and establish the “long lost” trust with the farmers.
One of the key aspects of building trust with farmers is transparency (Jakku et al., 2019). Transparency and legitimate data processing can encourage farmers to share data with ATPs and other stakeholders. Transparency is one of the most important practices in privacy. Agricultural technology providers should be transparent about the collection, use, sharing, and disclosure of the farmers' data. This can be achieved by creating clear, complete, and unambiguous data agreements that discuss the data practices and terms of use. Furthermore, these legal documents should be written in simple language so that it is easy to understand.
Agricultural technology providers should follow the privacy by design approaches while designing and developing technology products for farms or working with large-scale networked farm data systems (Amiri-Zarandi et al., 2022). Privacy by design is an approach which encourages data protection to be integrated into a product or service from the very early stages of development. Privacy by design covers seven foundational principles which ATPs should include in their business' core values (Cavoukian, 2009). For example, the ATPs should embed privacy protection functionalities into the system that are user centric and give more control to the farmers. Agricultural technology providers should provide farmers with the choice to give or withdraw consent for the use of farm data. Agricultural technology providers should also embed user-friendly privacy features in the farm data collection and processing system. User friendly features contribute to transparent privacy practices and make them more understandable for farmers. To achieve this, farmers can be engaged, through interviews, during the design and development of farm systems. Testing the farming technologies at the farm is also recommended to examine privacy and security loopholes and to proactively resolve them before production. Furthermore, educating farmers to work with farm equipment can result in a lower rate of technical issues and privacy and security violations in the system.
Farm legal data agreements content should cover a comprehensive set of data protection practices related to farm data (Ferris, 2017). They should include different topics such as data collection, data sharing, data access, data retention, data security, policy change, purpose, choice, contact information of the organization or data steward, and several other sections. Regulations and privacy principles such as GDPR and Organization for Economic Co-operation and Development (OECD) have provided recommendations about the content of data licenses and terms of use agreements. They have also considered several rights for individuals regarding the collection and use of their personal data such as the right to an explanation or automated individual decision making, including profiling, right to erasure, and right to object (OECD., 2013; Office of the Privacy Commissioner of Canada., 2018). The applicability of these rights to farmers' personal data can be investigated by ATPs. For instance, the right to automated individual decision-making is a right that could allow individuals to request clarification on data processing. For applications such as carbon credits or carbon taxes, for instance, farmers may need to know how algorithms arrive at decisions about their carbon footprint. Agricultural technology providers can design their systems in a way that the outcomes of algorithms are explainable. It is also recommended that ATPs pay particular attention to the content of data agreements. For instance, data agreements should clearly specify the data collection process, list of personal and farm data that are collected at the farms and inform the farmers how their data is used. These agreements should also include the intention or purpose of collection, sharing, and usage of farmers' personal information. The farmers should have the right to access data and opt out of the service whenever they wish.
Effective measures should be taken by ATPs to preserve the privacy of farmers while processing their data (Zaman et al., 2017). The collection and processing of personal data may result in an invasion of farmers' privacy. This issue can be mitigated by de-identifying the personal data from the source by removing identifiable information such as name, address, phone number, and location wherever applicable (Jakku et al., 2019). While collecting data, ATPs should avoid collecting sensitive or farmers personal data if the data are not needed for analytical purposes. Furthermore, ATPs should clearly specify the purpose of data collection and use in their data agreements and must strictly adhere to those purposes (Janzen, 2021). If ATPs use the data for other purposes which are not disclosed to farmers before data collection, this may lead to violation of farmers privacy.
One of the strongest approaches to strengthen ATPs' data practices is obtaining consent before collecting, using, sharing, or disclosing farm or personal information of the farmers. With explicit and informed consent, the farmers will be aware of the data practices that ATPs will implement and make informed decisions about the services ATPs provide. Furthermore, obtaining consent should be a dynamic and ongoing process. This would mean that the farmers should be notified if there are any changes made in the data collection, use and sharing practices, and ATPs should obtain consent before making any changes in privacy practices.
Data security is considered an important practice in protecting the privacy of farmers (Qi et al., 2020; Yaqot et al., 2021; Hazrati et al., 2022). Proactive measures must be taken by the ATPs to check for data leakages such as loss, unauthorized access or use, destruction, modification, or unintended and inappropriate disclosure of data so that the farmers' data are kept secure. It is recommended for the ATPs to take proactive data security measures to protect the privacy of farmers rather than taking remedial measures when a problem arises in the farm system (Cavoukian, 2009). Proactive measures can include finding loopholes and potential vulnerabilities in the system. This can be achieved by monitoring threats, performing intrusion detection, and training staff for privacy protection. To protect sensitive farm information, end-to-end data encryption is recommended. This will help in encoding the data that can only be read when the data are decrypted. Moreover, personal information stored by ATPs on laptops and portable hard drives should be protected by using technological safeguards such as encryption and password protection. Other cyber security approaches can be using two-layer authentication and network security for data transfer (Gupta et al., 2020).
If farm data are shared with third parties or industry partners, consent must be obtained from farmers and other actors who contribute to data generation. Agricultural technology provider must ensure that third parties and industry partners comply with the same legal terms and conditions that have been presented to farmers in terms of processing, sharing, and retention of data collected from farms. Agricultural technology providers should develop appropriate audit procedures to ensure compliance with data agreements and consent by third parties and avoid conflicts in intellectual property.
Table 2 summarizes additional recommendations that can contribute to farmers' privacy protection.
Finding a balance between protecting farm data confidentiality, while supporting supply chain stakeholders' growth and economic gain, is a challenge for policy makers and governments (Jouanjean et al., 2020). The legal frameworks that govern farm data are fragmented and do not protect farm data as it is expected by the farmers. The government's intervention in farm data protection and establishment of standards for farm data practices can address some of the existing governance challenges of farm data (Jouanjean et al., 2020). This section discusses the possible measures that policy makers can take for protecting farm data.
There is legislation related to the protection of an individuals' personal information by the private sector or businesses. For example, Personal Information Protection and Electronic Documents Act (PIPEDA) in Canada provides 10 fair information principles such as accountability, identifying purpose, consent, limiting collection, limiting use, disclosure and retention, accuracy, safeguards, openness, individual access, and challenging compliance (Office of the Privacy Commissioner of Canada., 2019). Regulations such as GDPR and CCPA also mandate the protection of individuals' information privacy. These regulations can strengthen users control over their data. These privacy laws enforce privacy principles or rights such as the right to be informed of the data gathered and how they are be used, the right to remove personal information that are collected, the right to withdraw from a service and erase the collected data, and the right to object to processing. Some of these regulations such as GDPR and the new bill 64 in the province of Quebec in Canada can be applied to protect farmers' most sensitive data, e.g. location data (GDPR., 2018). However, even those policies may not protect farmers against privacy risks such as identifiability (e.g., through PII; Ferris, 2017). This highlights the need for laws and policies that provide more comprehensive protection of farm data exclusively and extensively.
In addition to regulations, governments can implement strict measures to ensure compliance and accountability. These measures include non-compliance fines or other penalties including data processing suspension. Non-compliance penalties have been implemented by GDPR for the protection of personal information in private and public sectors. Policy enforcement is vital for the protection of farmers' right to privacy.
Given the existing gaps in laws and regulations pertaining to farm data, codes of conduct have been formulated by some non-profit organizations. These codes provide guidelines for protecting farm data. For example, the American Farm Bureau has drafted a set of principles referred to as Privacy and Security Principles or Core principles (Wiseman et al., 2019). These principles provide a benchmark for good practices in farm data governance including collecting, storing, using, and transferring farmer's data. Furthermore, this code of conduct encourages transparency on how farmers' data are used and processed and even secured. Other codes of conduct are New Zealand Farm Data Code of Practice, The European Union Code of Conduct on Agricultural Data Sharing by Contractual Agreement (“EU Code of Conduct”) and the Australian Farm Data Code. These codes of conduct are voluntary to follow and are self-regulatory which may reduce their effectiveness.
Governments can provide incentives for agricultural co-operatives to serve as trusted actors in the farming ecosystem. Agricultural co-operatives can foster a sustainable environment for farm data accessibility and use (Jouanjean et al., 2020). Data co-operative platforms can be established as a governance structure to facilitate farm data storage and processing by technology providers and other stakeholders. They can also facilitate access and collection of agricultural data for public research and innovation (Canadian Centre for the Study of Co-Operatives., 2017). Some examples of farm data cooperatives are Ag Data Coalition (ADC), Grower Information Services Cooperative (GISC), and JoinData. With the right legal frameworks and protocols, data co-operatives can empower farmers to manage data and build more confidence in using digital technologies on their farms. Data co-operatives can potentially resolve some of the farm data governance issues including data access; however, other challenges such as privacy (e.g., identifiability) and interoperability of data still need to be resolved. Governments can be instrumental in mitigating some of these challenges.
Since data is a useful resource for stakeholders, disagreements can occur regarding the status or ownership of data between farmers and other stakeholders. A range of policies or standards should be developed for well-balanced governance of farm data. This can be achieved by established innovation platforms for food system stakeholders that foster collaboration and knowledge sharing. Furthermore, governments can provide guidance on what should be performed or avoided in the contracts (Jouanjean et al., 2020).
Data-driven technologies improve efficiency of the entire supply chain, resulting in reduced concerns regarding food safety and security. Food supply chain stakeholders include crops, livestock, or other agriculture sector's actors, resource producers such as feed, fertilizing, and pesticide providers, as well as producers, distributors, retailers, and consumers. With the technological revolution and adoption of data-driven technologies, the agricultural sector has become well connected and offers benefits to the entire supply chain. This means that supply chain stakeholders' data practices influence protection of farm data. This section briefly discusses the measures that the stakeholders in the food supply chain can take to protect farmers data.
Data-driven technologies for food safety and sustainability require collaboration among food supply chain stakeholders through data sharing and integration. Food traceability and provenance, for instance, need data tracking systems such as digital identity management, Radio Frequency Identification (RFID), to integrate data from farms to consumers in order to validate the origin of food products (Chen et al., 2008). Food recall, supply management, and many other applications rely on access and integration of farm data. These interconnected actors and processes need responsible data sharing and integration practices and tools to protect farmers' privacy and data assets.
Standardized data practices and tools are needed to be shared and used among the stakeholders to effectively manage data provenance, integration, and processing while preserving the privacy of all the stakeholders. Explicit consent and transparent data agreements are also required to ensure all the stakeholders are fully aware of the terms and conditions of data collection, sharing, integration, and use. Additionally, supply chain stakeholders should ensure the protection of farmers' identities by anonymization or de-identification of farmers' data. Other recommendations include securing data end-to-end through security standards and platforms (e.g., blockchain) (Al-Farsi et al., 2021; Li et al., 2022). Automated auditing procedures can help ensure ethical and responsible access and use of data through the value chain.
The success of precision agriculture is highly reliant on the massive amounts of data that are now possible to collect and process by big data technologies. With this comes the responsibility for data privacy and confidentiality which has imposed challenges on the farming system. Fragmented legal frameworks, regulations and contractual obligations, lack of standards and best practices for protecting farm data, and lack of appropriate business models for co-creation, and sharing value of data are only some of the challenges. These issues in addition to limited adoption of privacy best practices by ATPs and other stakeholders in the supply have resulted in farmers' reluctance to share data or even adopt new technologies.
This paper provides practical recommendations for the main stakeholders in the farming ecosystem, on using existing best practices to better preserve farmers' privacy and data confidentiality. We believe if these recommendations are adopted in a proactive manner by all the stakeholders in the agriculture sector, farmers data will be better protected, and may encourage them to share farm data. This can help in strengthening trust among farmers and other stakeholders which may result in increased adoption and usage of technology in the agriculture sector.
This paper is one of the first attempts to gather best practices for protecting privacy and confidentiality of farm data. Future research can examine the social, legal, and economic impact of farm data privacy breaches and misuse and evaluate the impact and effectiveness of the existing privacy preserving methods and protocols.
SH, JK, and RD contributed to conception of the study. All authors have contributed to writing the paper. All authors contributed to manuscript revision, read, and approved the submitted version.
This research was funded by a Natural Sciences and Engineering Research Council of Canada (NSERC) Discovery Grant and Ontario Ministry of Agriculture Food and Rural Affairs, Alliance Tier I, funding awarded to R. Dara.
The authors declare that the research was conducted in the absence of any commercial or financial relationships that could be construed as a potential conflict of interest.
All claims expressed in this article are solely those of the authors and do not necessarily represent those of their affiliated organizations, or those of the publisher, the editors and the reviewers. Any product that may be evaluated in this article, or claim that may be made by its manufacturer, is not guaranteed or endorsed by the publisher.
Ag Data Transparent. (2022). Ag Data Transparent.Available online at: https://www.agdatatransparent.com/about (accessed February 18, 2022).
Al-Farsi, S., Rathore, M. M., and Bakiras, S. (2021). Security of blockchain-based supply chain management systems: challenges and opportunities. Appl. Sci. 11:5585. doi: 10.3390/app11125585
Amiri-Zarandi, M., Hazrati, M., Yousefinaghanis, S., Kaviani, M., and Dara, R. (2022). A platform approach to smart farm information processing. Agriculture 12:838. doi: 10.3390/agriculture12060838
Audich, D. A., Dara, R., and Nonnecke, B. (2021). Improving readability of online privacy policies through doop: a domain ontology for online privacy. Digital 1, 198–215. doi: 10.3390/digital1040015
Canadian Centre for the Study of Co-Operatives. (2017). Digital Technologies and the Big Data Revolution in the Canadian Agricultural Sector: Opportunities, Challenges, and Alternatives. Available online at: https://usaskstudies.coop/documents/big-data-in-canadian-agriculture-report-fultonetal.pdf (accessed February 22, 2022).
Caria, M., Schudrowitz, J., Jukan, A., and Kemper, N. (2017). “Smart farm computing systems for animal welfare monitoring,” in 2017 40th International Convention on Information and Communication Technology, Electronics and Microelectronics (MIPRO) (Opatija), 152–157. doi: 10.23919/MIPRO.2017.7973408
Castelluccia, C., Cunche, M., le Metayer, D., and Morel, V. (2018). “Enhancing transparency and consent in the IoT,” in 2018 IEEE European Symposium on Security and Privacy Workshops (EuroS PW) (London), 116–119. doi: 10.1109/EuroSPW.2018.00023
Cavoukian, A. (2009). Privacy by design: the 7 foundational principles. Inform. Priv. Commiss. Ont. Canada 5:12.
Chen, R. S., Chen, C. C., Yeh, K. C., Chen, Y. C., Kuo, C. W., et al. (2008). Using RFID technology in food produce traceability. WSEAS Trans. Inform. Sci. Appl. 5, 1551–1560.
Ferris, J. L. (2017). Data privacy and protection in the agriculture industry: is federal regulation necessary. Minn. JL Sci. Tech. 18:309.
GDPR. (2018). “Art. 33 GDPR – notification of a personal data breach to the Supervisory Authority,” in General Data Protection Regulation (GDPR), 29-Mar-2018. Available online at: https://gdpr-info.eu/art-33-gdpr/ (accessed February 18, 2022).
Guntamukkala, N., Dara, R., and Grewal, G. (2015). “A machine-learning based approach for measuring the completeness of online privacy policies,” in 2015 IEEE 14th International Conference on Machine Learning and Applications (ICMLA) (Miami, FL), 289–294.
Gupta, M., Abdelsalam, M., Khorsandroo, S., and Mittal, S. (2020). Security and privacy in smart farming: challenges and opportunities. IEEE Access. 8, 34564–34584. doi: 10.1109/ACCESS.2020.2975142
Hazrati, M., Dara, R., and Kaur, J. (2022). On-farm data security: practical recommendations for securing farm data. Front Sustain Food Syst. 6:884187. doi: 10.3389/fsufs.2022.884187
Hert, P. D., Papakonstantinou, V., Malgieri, G., Beslay, L., and Sanchez, I. (2017). The right to data portability in the GDPR: towards user-centric interoperability of digital services. Comput Law Secur. Rev. 34, 193–203. doi: 10.1016/j.clsr.2017.10.003
IAPP. (2022). De-Identification of Personal Information. Available online at: https://iapp.org/resources/article/de-identification-of-personal-information/ (accessed February 18, 2022).
Jakku, E., Taylor,. B., Fleming, A., Mason, C., Fielke, S., Sounness, C., et al. (2019). ‘If they don't tell us what they do with it, why would we trust them?' Trust, transparency and benefit-sharing in Smart Farming. NJAS Wagen. J. Life Sci. 90–91:100285. doi: 10.1016/j.njas.2018.11.002
Janzen, T. (2021). “Secondary use of AG data is a privacy violation,” in Precision Farming Dealer, 30-Apr-2021. Available online at: https://www.precisionfarmingdealer.com/articles/4647-secondary-use-of-ag-data-is-a-privacy-violation (accessed February 18, 2022).
Jouanjean, M.-A., Casalini, F., Wiseman, L., and Gray, E. (2020). Issues Around Data Governance in the Digital Transformation of Agriculture: The Farmers' Perspective. Paris: OECD Publishing. doi: 10.1787/18156797
Kaur, J., Dara, R. A., Obimbo, C., Song, F., and Menard, K. (2018). A comprehensive keyword analysis of online privacy policies. Inform. Secur. J. Glob. Perspect. 27, 260–275. doi: 10.1080/19393555.2019.1606368
Li, F., Yu, X., Ge, R., Wang, Y., Cui, Y., Zhou, H., et al. (2022). BCSE: blockchain-based trusted service evaluation model over big data. Big Data Min. Anal. 5, 1–14. doi: 10.26599/BDMA.2020.9020028
Linsner, S., Kuntke, F., Steinbrink, E., Franken, J., and Reuter, C. (2021). The role of privacy in digitalization-analyzing perspectives of german farmers. Proc. Priv. Enhanc. Technol. 2021, 334–350. doi: 10.2478/popets-2021-0050
McIntosh, M. (2018). “The legal mess of farm data ownership,” in Farmtario, 24-Sep-2018. Available online at: https://farmtario.com/machinery/the-legal-mess-of-farm-data-ownership/ (accessed February 18, 2022).
Monteiro, A., Santos, S., and Gonçalves, P. (2021). Precision agriculture for crop and livestock farming—brief review. Animals 11:2345. doi: 10.3390/ani11082345
OECD. (2013). OECD Guidelines on the Protection of Privacy and Transborder Flows of Personal Data. Available online at: https://www.oecd.org/sti/ieconomy/oecdguidelinesontheprotectionofprivacyandtransborderflowsofpersonaldata.htm; https://www.priv.gc.ca/en/privacy-topics/collecting-personal-information/02_05_d_56_tips2/ (accessed February 18, 2022).
Office of the Privacy Commissioner of Canada. (2018). Ten Tips for a Better Online Privacy Policy and Improved Privacy Practice Transparency. Office of the Privacy Commissioner of Canada, 30-Nov-2018. Available online at: https://www.priv.gc.ca/en/privacy-topics/collecting-personal-information/02_05_d_56_tips2/ (accessed February 18, 2022).
Office of the Privacy Commissioner of Canada. (2019). PIPEDA in Brief. Office of the Privacy Commissioner of Canada, 31-May-2019. Available online at: https://www.priv.gc.ca/en/privacy-topics/privacy-laws-in-canada/the-personal-information-protection-and-electronic-documents-act-pipeda/pipeda_brief/ (accessed February 18, 2022).
Qi, L., Hu, C., Zhang, X., Khosravi, M., Sharma, S., Pang, S., et al. (2020). Privacy-aware data fusion and prediction with spatial-temporal context for smart city industrial environment. IEEE Trans. Indus. Inform. 17, 4159–4167. doi: 10.1109/TII.2020.3012157
Wilson, M. (2018). “3 Skills tomorrow's farmer will need,” in Farm Progress, 08-Dec-2018. Available online at: https://www.farmprogress.com/technology/3-skills-tomorrow-s-farmer-will-need (accessed February 18, 2022).
Wiseman, L., Sanderson, J., Zhang, A., and Jakku, E. (2019). Farmers and their data: an examination of farmers' reluctance to share their data through the lens of the laws impacting smart farming. NJAS – Wagen. J. Life Sci. 90–91:100301. doi: 10.1016/j.njas.2019.04.007
Yaqot, M., and Menezes, B. C. (2021). “Unmanned Aerial Vehicle (UAV) in precision agriculture: business information technology towards farming as a service,” in 1st International Conference on Emerging Smart Technologies and Applications (eSmarTA) (Sana'a), 1–7. doi: 10.1109/eSmarTA52612.2021.9515736
Yaqot, M., Menezes, B. C., and Al-Ansari, T. (2021). Unmanned aerial vehicles in precision agriculture towards circular economy: a process system engineering (PSE) assessment. Comput. Aid. Process Eng. 50, 1559–1565. doi: 10.1016/B978-0-323-88506-5.50241-2
Zaman, A. N. K., Obimbo, C., and Dara, R. A. (2017). “An improved data sanitization algorithm for privacy preserving medical data publishing,” in Advances in Artificial Intelligence. Canadian AI 2017. Lecture Notes in Computer Science, Vol. 10233, eds M. Mouhoub and P. Langlais, (Cham: Springer), 64–70.
Keywords: precision agriculture, information privacy, farm data protection, privacy legislation, data agreements, farm data governance
Citation: Kaur J, Hazrati Fard SM, Amiri-Zarandi M and Dara R (2022) Protecting farmers' data privacy and confidentiality: Recommendations and considerations. Front. Sustain. Food Syst. 6:903230. doi: 10.3389/fsufs.2022.903230
Received: 24 March 2022; Accepted: 03 October 2022;
Published: 19 October 2022.
Edited by:
Rosalind Malcolm, University of Surrey, United KingdomReviewed by:
Brenno Menezes, Hamad Bin Khalifa University, QatarCopyright © 2022 Kaur, Hazrati Fard, Amiri-Zarandi and Dara. This is an open-access article distributed under the terms of the Creative Commons Attribution License (CC BY). The use, distribution or reproduction in other forums is permitted, provided the original author(s) and the copyright owner(s) are credited and that the original publication in this journal is cited, in accordance with accepted academic practice. No use, distribution or reproduction is permitted which does not comply with these terms.
*Correspondence: Rozita Dara, ZHJveml0YUB1b2d1ZWxwaC5jYQ==
†These authors have contributed equally to this work
Disclaimer: All claims expressed in this article are solely those of the authors and do not necessarily represent those of their affiliated organizations, or those of the publisher, the editors and the reviewers. Any product that may be evaluated in this article or claim that may be made by its manufacturer is not guaranteed or endorsed by the publisher.
Research integrity at Frontiers
Learn more about the work of our research integrity team to safeguard the quality of each article we publish.