- 1School of Earth and Environment, University of Leeds, Leeds, United Kingdom
- 2The Brazilian Agricultural Research Corporation (EMBRAPA) Arroz e Feijão, Santo Antônio de Goiás, Brazil
- 3Alliance of Biodiversity International and CIAT, Rome, Italy
- 4CGIAR Research Program on Climate Change, Agriculture and Food Security (CCAFS), Cali, Colombia
- 5Plant Production Systems Group, Wageningen University, Wageningen, Netherlands
Irrigation strategies are keys to fostering sustainable and climate-resilient rice production by increasing efficiency, building resilience and reducing Greenhouse Gas (GHG) emissions. These strategies are aligned with the Climate-Smart Agriculture (CSA) principles, which aim to maximize productivity whilst adapting to and mitigating climate change. Achieve such mitigation, adaptation, and productivity goals- to the extent possible- is described as climate smartness. Measuring climate smartness is challenging, with recent progress focusing on the use of agronomic indicators in a limited range of contexts. One way to broaden the ability to measure climate-smartness is to use modeling tools, expanding the scope of climate smartness assessments. Accordingly, and as a proof-of-concept, this study uses modeling tools with CSA indicators (i.e., Greenhouse Intensity and Water Productivity) to quantify the climate-smartness of irrigation management in rice and to assess sensitivity to climate. We focus on a field experiment that assessed four irrigation strategies in tropical conditions, Continuous Flooding (CF), Intermittent Irrigation (II), Intermittent Irrigation until Flowering (IIF), and Continuous soil saturation (CSS). The DNDC model was used to simulate rice yields, GHG emissions and water inputs. We used model outputs to calculate a previously developed Climate-Smartness Index (CSI) based on water productivity and greenhouse gas intensity, which score on a scale between−1 (lack of climate-smartness) to 1 (high climate smartness) the climate-smartness of irrigation strategies. The CSS exhibited the highest simulation-based CSI, and CF showed the lowest. A sensitivity analysis served to explore the impacts of climate on CSI. While higher temperatures reduced CSI, rainfall mostly showed no signal. The climate smartness decreasing in warmer temperatures was associated with increased GHG emissions and, to some extent, a reduction in Water Productivity (WP). Overall, CSI varied with the climate-management interaction, demonstrating that climate variability can influence the performance of CSA practices. We conclude that combining models with climate-smart indicators can broaden the CSA-based evidence and provide reproducible research findings. The methodological approach used in this study can be useful to fill gaps in observational evidence of climate-smartness and project the impact of future climates in regions where calibrated crop models perform well.
Introduction
To maintain sustainable rice production, farmers need to adapt to climate change and reduce Greenhouse Gas (GHGs) emissions in rice systems. In this sense, the Climate-Smart Agriculture (CSA) approach have been promoting irrigation strategies to simultaneously achieve mitigation, adaptation, and productivity in rice crops (Wassmann et al., 2019). Such strategies have been placed in attendance to the 2030 Agenda for Sustainable Development, intending to achieve goals 2 (Zero hunger), 6 (Clean water and sanitation), 12 (Responsible consumption and production) and 13 (Climate action; United Nations Organization, 2015).
Irrigation practices like mild-season drainage or Alternate Wetting and Drying (AWD) can reduce GHG emissions by up to 60% and save water by up to 30% without affecting productivity (Carrijo et al., 2017; Jiang et al., 2019; Liu et al., 2019). However, a key challenge is that these objectives often cannot all be achieved to the full extent because the effectiveness of irrigation practices varies according to the context, which results in trade-offs and synergies between CSA objectives.
Several approaches have been developed to assess and monitor the performance of CSA strategies and bring a quantitative measure of their effectiveness or “climate-smartness” (van Wijk et al., 2020). A first instance is the use of so-called climate-smart indicators, which are qualitative or quantitative variables (agro-climatic, biophysical socio-economic, among others) that inform the performance of the agricultural systems and work as a benchmark for decision-making in CSA-oriented projects and programs. Some publications as the “Climate-Smart Agriculture Indicators” by the World Bank (2016); the “National level indicators for gender, poverty, food security, nutrition and health in Climate-Smart Agriculture (CSA) activities” (Duffy et al., 2017) or the “CSA Programming and Indicator Tool” (Quinney et al., 2016) they listed and categorized CSA indicators used in different dimensions of the agriculture (i.e., social, economic, biophysical).
Among the available methodological approaches that use and combine different climate-smart indicators, the Climate-Smartness Index (CSI) is a metric that brings a quantitative measure of climate-smartness. The CSI is a composite index based on agronomic indicators of CSA, normalized, and aggregated to represent the synergy/trade-off between water productivity and the greenhouse gas intensity in cropping systems under water-oriented adaptation strategies (Arenas-Calle et al., 2019).
The CSI was applied by Arenas-Calle et al. (2019) to compare several independent studies with paired comparisons of conventional irrigation and the Alternate Wetting and Drying (AWD) irrigation in different contexts. The CSI identified trends in AWD treatments across geographical locations and quantified the climate-smartness of AWD treatments. To date, the use of climate-smart indices based on field data is limited to the spatial and temporal scales of the underlying measurements (i.e., historical trials at the field scale). The use of crop model simulations with climate-smart indices has the potential to vastly broaden the range of places and periods where CSI can be calculated. This approach could be used to identify CSA practices, inform the robustness of future interventions, or estimate trade-offs across spatial and temporal scales that could undermine scaling up efforts (Pringle, 2011; Nowak et al., 2019).
This study presents the first logical step in using climate-smart indices with a process-based model: to calculate the index based on process-based model outputs, and thus provide an assessment of simulated climate-smart practices in climatic scenarios beyond the environment that have been tested in the field. We a climate-smartness assessment based on model simulations and CSI for water management strategies in rice. The assessment was aaplied to a a 5-year experiment that evaluates four irrigation strategies in rice following two steps: (1) modeling of rice yield, GHG emissions and water inputs, and (2) calculation of CSI from simulated output indicators for irrigation treatments during 2014-2019 cropping seasons and sensitivity analysis outcomes.
Materials and Methods
A simulation-based climate-smartness assessment was developed for several water management strategies using irrigated rice in the Brazilian tropical region as case study. First, the DNDC v.9.5 model was parameterized and evaluated using field data from two cropping seasons (2016–2018). Rice yield, water inputs and GHG emissions were simulated for all irrigation treatments during 2014 to2019, and the Climate-Smartness Index (CSI) was calculated. The simulations were re-run for the same period under different rainfall and temperature scenarios created based on the observed climate data. The application of modeling tools to simulate CSA indicators, the model performance and CSA assessment results were analyzed and discussed.
Field Experiment
This study used data from a 5-year experiment carried out in the Embrapa Arroz e Feijão (Brazilian Agricultural Research Corporation, Unit Rice and Beans) experimental station “Palmital”, municipality of Goianira, Goiás State, at the Brazilian Midwest region (16°26′8.45″S−49°23′38.31″O, altitude 729 m). The location has a tropical climate with a well-defined dry and wet season. The annual mean temperature is 23°C, with the minimum mean temperature reported in June (12.8°C) and the maximum temperatures in September (32.3°C), and annual precipitation of 1485 mm distributed across wet periods in October to April (220 to 270 mm/month) and dry periods in May-September (6.6. to 11 mm/month; INMET, https://www.gov.br/agricultura/pt-br/assuntos/inmet).
The experiment assessed different irrigation strategies as follows: Continuous Flooding (otherwise described as conventional irrigation) (CF); Intermittent Irrigation (II); Continuous Soil Saturation, where the soil kept saturated or above field capacity (CSS); and Intermittent Irrigation until Flowering where the continuous flooding conditions were maintained until harvesting (IIF). The N fertilization consisted of basal dressing application at sowing and two split doses: the first at the beginning of the tillering (25-28 days after sowing) and the second at effective tillering (40–45) days after sowing.
The rice genotype cultivated was BRS Catiana, a modern size with erect leaves, high tillering and robust stems, with resistance to plant lodging and tolerant to the main rice diseases, in special to blast (Fragoso et al., 2021). This genotype presents high yielding potential, with mean flowering days of 89 (104) and a cycle of 116 (131) days after emergence in the tropical (subtropical) environment. This cultivar was commercially released in 2016 and it is recommended for cropping in the North, South, Southeast, Northeast and Midwest regions of Brazil (de Morais et al., 2016). A detailed description of the field experiment has been published by Barbosa (2018).
Weather data (min. temperature, max. temperature, precipitation, humidity, solar radiation, and wind speed) were available for the whole period assessed (2014–2019, https://www.cnpaf.embrapa.br/climacnpaf/). Yield data were available for all treatments during the assessed period except for season 2015/2016. As part of the experiment, methane (CH4) and nitrous oxide (N2O) emissions were measured during 2015/2016 and 2016/2017 seasons in the plots under CF, II and CSS treatments. Water inputs were available for the 2016/2017 and 2017/2018 cropping seasons in all irrigation treatments.
The static closed chamber technique was used to measure the CH4 and N2O fluxes from the soil. The static chambers used had a circular shape with a plastic lid (20 cm height and 17.5 cm radius), inserted into the soil at 10 cm. The chambers included a 38 cm high extender to adjust the chamber height as plants grown. Gas samplings were performed in the morning, between 9:00 and 11:00 h, according to recommendations from Jantalia et al. (2008), at the pre-established time intervals of 0, 15, and 30 min after closing the chambers. Water was added to the top of the basis and extenders (junctions) to avoid air leaking. Before and after each air sampling, the temperatures inside the chamber, soil, and air were monitored with a digital thermometer. The concentrations of GHG gases were determined using a gas chromatograph GC 2014 “Greenhouse” (Shimadzu Co., Tokyo, Japan). In addition, fertilizer was applied inside the base of the installed chambers; the N fertilizer applied inside the chamber base was adjusted in the proportion of the chamber area. In all seasons, direct seeding was carried out before irrigation.
Modeling of Rice Yields, Direct GHG Emissions and Water Inputs Using DNDC Model
The DNDC model (https://www.dndc.sr.unh.edu/) is a process-based biogeochemistry model (DNDC) that simulates carbon and nitrogen cycles in agroecosystems. The model is based on two main modules. One module contains the soil climate, crop growth, and decomposition sub-models to simulate physical and chemical soil proprieties. A second module comprises nitrification, denitrification, and fermentation sub-models that simulate plant-soil gas exchange (Li, 2000). Although DNDC is commonly used to modeling carbon and nitrogen dynamics in the soil, the model also can simulate crop growth using a GDD-based sub-model (Zhang and Niu, 2016). We used the DNDC v.9.5 to simulate rice yield, water inputs and GHG emissions (CH4 and N2O) for the irrigation strategies assessed in the experiment described in Section Field Experiment.
Input Data and Calibration of Cultivar Parameters in DNDC Model
The input requirements in the DNDC model consist of (1) climate data, (2) soil data, (3) crop parameters, and (4) agronomic management such as fertilization, tillage, irrigation or flooding, as well as dates of sowing and harvesting. Daily weather data, namely, maximum and minimum temperature (°C), precipitation (cm), wind speed (m s−1), solar radiation (MJ m−2) and humidity (%), were obtained from the local meteorological station located at the experimental station for the period 2014–2019. Average climate parameters for the five cropping seasons are summarized in Table 1. Daily variation of temperature and rainfall distribution can be consulted in Supplementary Figure 5.
Soil parameters such as texture, clay portion (%), bulk density (g/cm3), organic matter (g kg−1, OM) and total carbon (%), and pH were obtained from soil analysis of the experimental site. Porosity was calculated based on the bulk density and the soil particle density (2.65 g cm3) as is shown in equation 1. The water-filled pore space (WFPS %) at field capacity and wilting point were calculated based on gravimetric soil water content at 33 and 1,500 kPa and bulk density according to Equations 2 and 3. Table 2 shows the soil initial condition parameters.
where BD, bulk density (g /cm3); PD, particle density (2.65 g /cm3); θ at 33 kPa, gravimetric soil water content at field capacity and θ at 1500 kPa, gravimetric soil water content at wilting point.
The parametrization of the irrigation management used the treatments description provided by Barbosa (2018) and the records of water inflows estimated by the hydrometers installed in the field. The irrigation started on the same date in all treatments at 17–18 days after emergence. Treatments started to differentiate after the water from the first flood drained (~1 week). After the first irrigation, the CF management kept flooded until 1 or 2 days before harvesting. The II had maintained intermittent irrigation with re-flooding approximately every 5 to 7 days. The IIF had a similar irrigation schedule to II until the flowering stage when a continuous flood was maintained until harvesting. As SCC was in theory saturated soil, the hydrometers showed a few flood events during the cropping season. The urea-based fertilization consisted of one base application during the sowing and a top-dressing fertilizer (80 kg ha−1 N) split into two doses. Table 3 shows the agronomic data for the two seasons (2016/207 and 2017/2018) to validate the model.
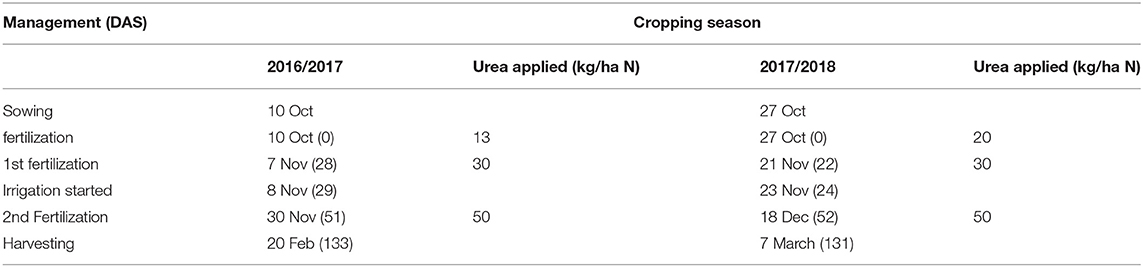
Table 3. Agronomic management in 2016/2017 and 2017/2018 cropping seasons [numbers in brackets next to dates indicated days after sowing (DAS)].
Cultivar BRS-Catiana was calibrated based on traits reported in the literature and field data. Thermal degree days for maturity (TDD) was calibrated based on the range of TDD values from the five cropping seasons. From this range, the TDD average from the five seasons obtained the yield and Leaf Area Index (LAI) with the lowest RMSE. Maximum grain biomass was manually calibrated based on independent experiments reported by dos Santos et al. (2017) and Rangel et al. (2019). Biomass fractions and optimum temperature were taken from de Castro (2020), who optimized the calibration of BRS-Catiana in the Oryza2000 model using an independent experiment of BRS-Catiana carried out in the experimental station “Palmital”. Thus, the default rice crop parameters in DNDC were modified as follows: the TDD was modified from 3,800 to 1,943, the maximum grain biomass from 5,200 to 4,531 kg C/ha/yr; biomass fraction at maturity of grain/leaf/stem/root from 0.4/0.22/0.22/0.16 to 0.48/0.07/0.25/0.2, and the optimum temperature from 25 to 34°C.
Evaluation of the DNDC Model
To evaluate the DNDC model it were used the yield and water input observed in CF, II, IIF and CSS and the GHG fluxes in all the irrigation treatments except IIF during the 2016/2017 and 2017/2018 seasons. The observed data from both seasons were selected to validate the model because they had the information needed to calculate the CSI.
The Total Water Input based on rainfall and irrigation (TWI) was estimated using the daily water balance simulations (Tian et al., 2021). Cumulative fluxes of N2O and CH4 were calculated from the sum of the daily fluxes during the cropping season. The net global warming potential (expressed as CO2-equivalent) resulted from the sum of CH4 and N2O cumulative fluxes after their conversion to CO2-eq by multiplying their 100-year time horizon global warming potentials (GWP); 28 for CH4 and 265 for N2O (Myhre et al., 2013). The Water productivity (WP; kg/m3) was calculated by dividing the rice yield (kg/ha) by total water input (m3 Equation 4) and the GHGI by dividing the cumulative fluxes or the area-based GWP expressed in CO2-eq /ha by rice yield (kg CO2-eq / kg grain; Equation 5).
Calculation of the Climate-Smartness Index
The water-oriented Climate-Smartness Index (CSI) proposed by Arenas-Calle et al. (2019) was calculated for the modeling outcomes to quantify the climate-smartness of irrigation treatments. The CSI provide a measurable and replicable metric of climate-smartness. Given the high crop water requirements and the significant contribution of rice systems to GHG emissions, one indicative of climate-smartness in such systems is their potential to achieve rice with high water use efficiency and low carbon footprint.
The CSI is calculated using water productivity (WP), based on irrigation and rainfall, and Greenhouse Gas Intensity (GHGI) that can be calculated as is shown in Equations 4 and 5. The Climate-Smartness Index (CSI) was calculated based on values of WP and GHGI that were normalized on a scale of 0–1, as is shown in Equations 10 and 11. For the normalization of WP and GHGI, we used the same maximum and minimum references reported by Arenas-Calle et al. (2019) in their literature review. To obtain such reference values the authors consulted 113 studies that reported WP and GHGI in rice systems around the world from were obtained 381 data points of WP and 499 of GHGI
GHGImin= 0.01-kg CO2-eq/kg grain, GHGmax = 7.8 kg CO2-eq/kg grain, WPmin = 0.1 kg grain m−3, WPmax = 3.7 kg grain m−3. The Equation 12 shows the calculation of the Climate-Smartness Index (CSI) from the substation of GHG (N) to WP (N).
CSI has a scale between −1 and 1, from lowest to highest climate-smartness. Negative CSI values indicate a lack of climate smartness when the GHG emissions per kilogram of grain (GHGI) are proportionately high than the amount of rice produced per unit of water applied (WP). On the contrary, when the system reports higher production per unit of water than GHG emissions produced per kilogram of grain, the CSI take positive values indicating climate smartness. Between higher the GHGI with respect to WP, the CSI will become negarive and vice versa. CSI was calculated for all irrigation treatments and seasons. Moreover, the CSI was compared against the temperature and precipitation scenarios set in the sensitivity analysis. Results of the CSI were compared, analyzed, and discussed.
Statistical Analysis
The coefficient of determination (R2, Equation 6), the root mean square error (RMSE; Equation 7), the normalized RMSE (Equation 8) and the relative deviation (RD, %); Equation 9) were calculated for the yield, cumulative GHGs and water inputs, and CSI to quantify the goodness fit between simulated and observed values.
Where Obsi is the observed value in the field; is the average of observed values; SMi is the simulated value is the average of simulated values; n is the number of measured values.
Simulation of Yield, Water Use and Greenhouse gas Emissions for the 2014–2019 Period
DNDC simulations for the four irrigation treatments evaluated under the experimental conditions described in Section Field Experiment were assessed to describe the climate-smartness of different irrigation strategies in irrigated rice under tropical conditions,. The simulated yields, water inputs and GHG emissions were used to analyse the trade-offs and synergies between these agronomic indicators among irrigation treatments and calculate the CSI.
Sensitivity Analysis
A sensitivity analysis was conducted to evaluate the response of the CSI to the variation in climate. Temperature was varied between −2 and 2°C by 1°C rate and the rainfall were varied from −25 to 25% at 5% increments. CSI was calculated for all irrigation treatments and seasons. The CSI was compared against the temperature and precipitation scenarios set in the sensitivity analysis.
Results
DNDC Model Validation
The DNDC model generated a good estimation of rice yields. Simulated and observed yields showed a good correlation (R2 = 0.68) and RMSE = 533 kg/ha, which represents an nRMSE (%) <10%. The comparison of observed and simulated Leaf Area Index (LAI) until the initiation of senescence also showed a good fit, these data are available in Supplementary Figure 1. The Relative Difference RD (%) between simulated and observed yield was 1.3% for CF, II, and IIF and 5% for CSS in 2016-2017. The RD (%) in the 2017/2018 season was higher; simulated yields in II and IIF treatments were 6 and −8.4% lower than the observed yields. Yield under CSS was underestimated by 19%, being the poorest estimation among the four treatments in both seasons. The simulation of Total Water Inputs (TWI) showed different responses. Overall, the model presented a poor simulation, especially CF treatment during the 2016/2017 season where TWI was overestimated by 74% and IIF treatment by 117%. The 2017/2018 season showed better performance with RD (%) between 0.3 and 28%. In both seasons the differences among treatments were similar; the highest TWI in CF treatments followed by IIF, II and CSS (Figure 1).
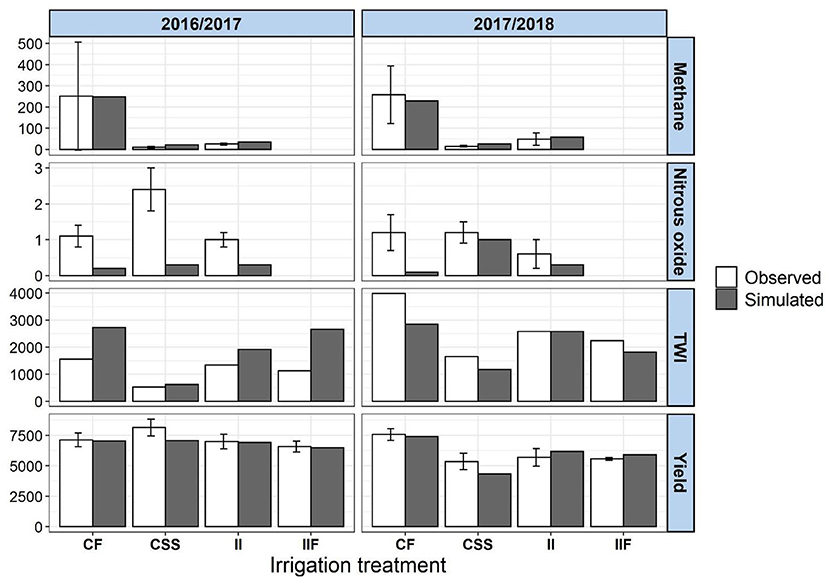
Figure 1. Observed (white bars) and simulated (gray bars) data of Yield (kg ha−1), methane (CH4, kg CH4 ha−1 season−1), Nitrous oxide (N2O, kg N2O ha−1 season−1) and Total Water Inputs (TWI, mm) for the 2016/20127 and 2017/2018 cropping seasons. Error bars represent the standard error.
The comparison of GHG emissions between simulated and observed data showed a good simulation of CH4 emissions but an underestimation of N2O emissions in all treatments (Supplementary Figures 3, 4). Overall, the model produced a poor simulation of N2O fluxes, which showed a low correlation with observed data (R2 <0.1). The DNDC model could capture the peaks of N2O generated during fertilization, but DNDC assumes zero N2O emissions during flooding periods, which disagreed with observed fluxes. In treatments with prolonged flooding conditions like CF, the model underestimated N2O cumulative fluxes by up to 90%.
Despite that N2O was underestimated, net Global Warming Potential presented a high correlation with the observed data (R2 = 0.9). The RD (%) of net GWP was <25% in all treatments except for CSS-2016/2017 (RD%−40%). These results evidence the main contribution of methane in the overall GHG emissions, in contrast with treatments under predominantly aerobic conditions such as CSS, where the N2O represents the principal contributor to overall GHG emissions. Water productivity (WP) and Greenhouse Gas Intensity (GHGI) indicators were calculated using simulated data and compared with the observed values (Figure 2). Both indicators showed a good correlation with observed data (R2 = 0.8 and R2 = 0.9, respectively). The poor simulation of TWI for the 2016/2017 season resulted in an underestimation of WP of 52% for the CF treatment (Figure 1). The Relative Difference (RD %) in WP between observed and simulated data varied between−36 to−5% in the 2017/2018 season. Greenhouse Gas Intensity (GHGI) simulations showed a better fit than WP, with RD% ranging between−3 to 12%, except in the case of the GHGI in CSS- 2017/2018, which was overestimated by 60%.
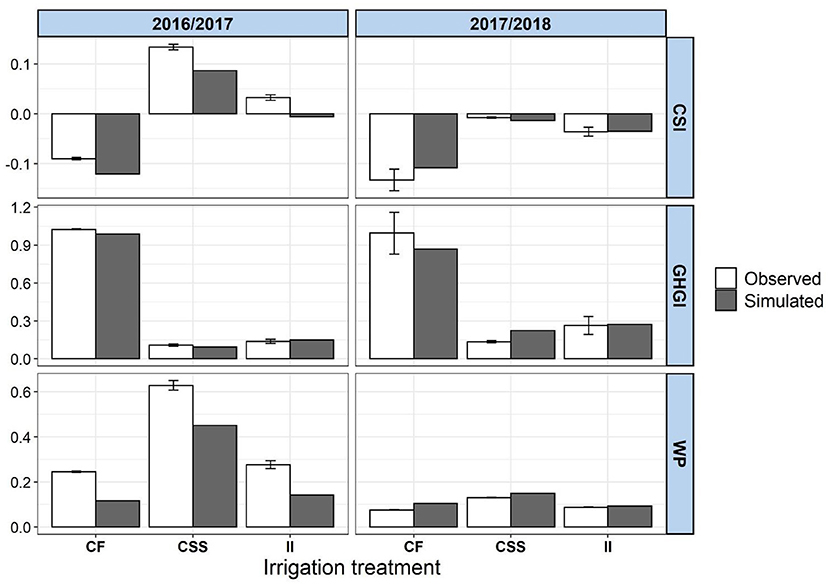
Figure 2. Observed (white bars) and simulated (gray bars) data of Water productivity (WP; kg/m3); Greenhouse Gas Intensity (GHGI; kg CO2-eq kg grain−1); Climate-Smartness Index (CSI) for the Continuous flooding (CF), Intermittent Irrigation (II) and Continuous Soil Saturation (CSS) for the seasons 2016/2017 and 2017/2018. Error bars represent the standard error.
DNDC Outputs From 2014/2015 to 2018/2019 Crop Seasons
The simulations showed differences in CSA outputs under different irrigation management across the five seasons (Figure 3). The season with the highest accumulated rainfall (2017/2018) presented the highest TWI for CF and IIF treatments. The lowest TWI occurred in the driest season (2018/2019). The differences in the TWI among the treatments showed that IIF used 4% less water than CF treatments, while II can save 20% more water than CF. Finally, CSS treatment presented the highest water-saving potential; 60% less water than CF (Figure 3A).
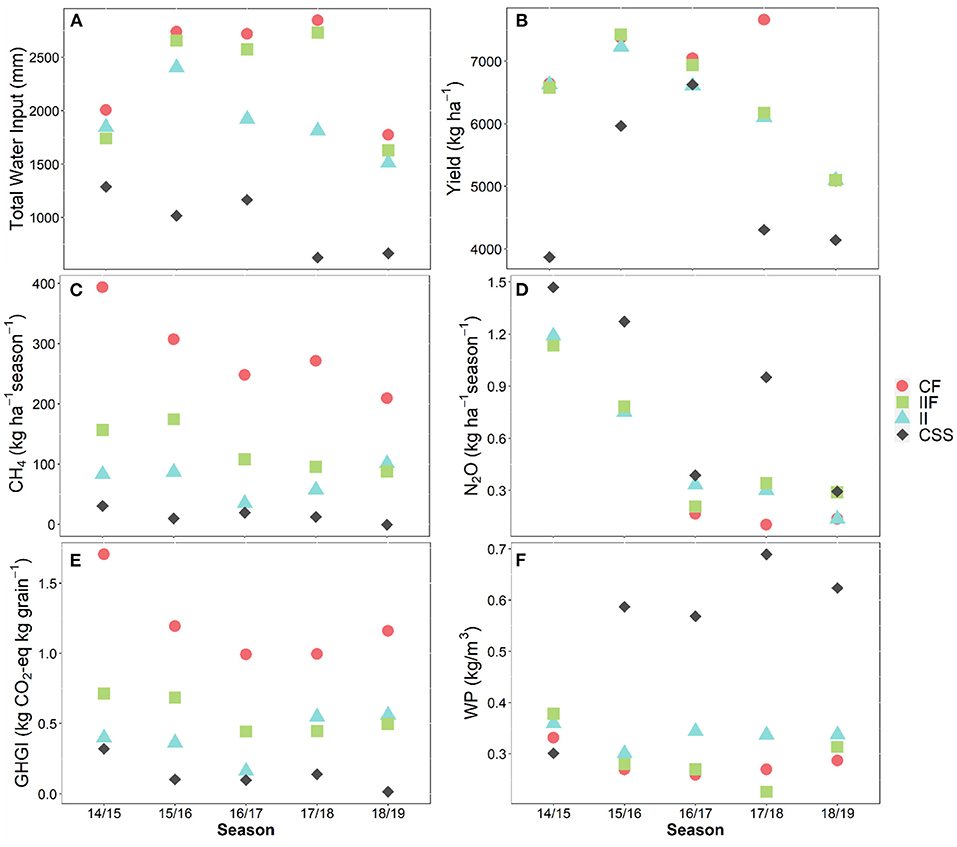
Figure 3. Simulations of (A) Total Water Input (TWI); (B) rice yield; (C) methane (CH4); (D) nitrous Oxide (N2O); (E) Water productivity (WP); (F) Greenhouse Gas Intensity (GHGI) under Continuous Flooding (CF), Intermittent Irrigation (II); Intermittent Irrigation until Flowering (IIF) and Continuous Soil Saturation (CSS) for the period 2014-2019.
Rice yield was 3.8% higher in CF IIF, while the difference between II and CSS was 6 and 26% higher, respectively (Figure 3B). Rice yield also showed temporal differences; the Sustainable Yield Index (SYI) indicated that CF, IIF, and IIF presented similar stability (ranged from 0.76 to 0.77), while CSS showed less yield stability (SYI = 0.59). The lowest yields occurred in 2018/2019 during the lowest cumulative rainfall in a cropping season. The highest yield occurred in the 2017/2018 season under CF treatment; however, II and IIF presented the highest yields during 2015/2016 which is the season with the second-highest cumulative rainfall.
The GHG emissions showed differences among treatments and seasons. The CH4 emissions were from high to low in the following order: CF>IIF>II>CSS (Figure 1). Continuous Flooding (CF) treatment ranged between 209 to 393 kg CH4 ha−1 season−1 while IIF treatments present on average, 56% fewer emissions (87 to 174 kg CH4 ha−1 season−1). The CF treatment was 74% higher than II and 95% than CSS where the methane emissions ranged between (−0.5 to 30 CH4 ha−1 season−1). The lowest methane emissions for CF, IIF, and CSS treatments occurred during 2018/2019, and the highest was in the 2014/2015 season (Figure 3C).
Seasonal N2O emissions were lower in CF than in IIF and II treatments (16.5 and 18%, respectively; Figure 3D). The irrigation treatment with the highest N2O emissions was CSS, with 87% more N2O emissions than CF. Despite the differences in N2O emissions among treatments, the emissions were generally low, ranging from 0.46 to 0.87 kg N2O ha−1 season−1. The N2O emissions also showed temporal differences: the lowest N2O emissions occurred during the 2018/2019 season and the highest during 2014/2015. Although CSS treatment presented the highest N2O emissions, the lowest N2O among seasons occurred during the drier year (2018/2019; Figure 3D).
The relationship between water input and yields was consistent across CF, IIF, and II treatments, reflected in water productivity ranging between 0.13 and 0.11 kg m−3 among treatments. In contrast, CSS presented the highest WP (0.33 kg m−3, during 2018/2019: Figure 3F) despite having the lowest yields across the seasons (Figure 3E). The results suggest that II and IIF treatments are effective strategies to save water and maintain rice yields; however, they could be insufficient to increase the efficiency of the rice crop. The water productivity showed seasonal variability, with the highest WP in CF, IIF and II during 2014/2015, while the highest WP achieved under CSS occurred during 2018/2019. Based on the WP and GHGI results (Figures 3E,F), it is possible to elucidate the climate smartness of the different irrigation treatments. Treatments with low GHGI such as CSS express 514 higher climate-smartness than treatments with high GHGI (e.g., CF treatment); similarly, relatively 515 high WP increases the climate-smartness over other treatments with lower WP.
In all seasons, the mitigation potential of II, IIF and CSS treatments reduce the Greenhouse Gas Intensity (GHGI) compared with CF, despite these treatments also reported reductions in yield (Figure 3F). While CF treatment showed an average GHGI of 1.2 kg CO2-eq per kilograms of grain, IIF showed the half (0.55 kg CO2-eq kg grain−1) and II treatment a GHGI 66% lower (0.4 kg CO2-eq kg grain−1) than CF. The CSS treatment with the highest impact on CH4 showed the lowest mean GHGI (0.13 kg CO2-eq kg grain−1). The GHGI also showed seasonal differences within the treatments. The highest GHGI occurred during 2014/2015 for CF, IIF and CSS treatments, while the lowest GHGI for CF, IIF and II treatments occurred during 2016/2017. The GHGI in CSS showed the lowest value during 2018/2019 but also coincided with a relatively low GHGI during 2016/2017.
The net Global Warming Potential (GWP) in CF treatments was, on average, 53% higher than IIF and 66% more than in II treatments. CSS irrigation had the lowest GWP (92% lower than CF treatments) across all seasons. The differences among the irrigation managements also varied among the seasons; IIF treatment emits between 42 and 63% less GHG emissions than CF, and II between 51 and 84% less than IIF. Differences in the GHGs cumulative fluxes among the treatments evidenced the contribution of each non-GHGs to the net GWP (Figure 4). Methane is the main contributor to net GWP in CF (95.6%), IIF (85.3%), and II (76.9%) treatments. For its part, methane represents 37.9% of net GWP in CSS treatment, whereas nitrous oxide represents the main contribution (62%).
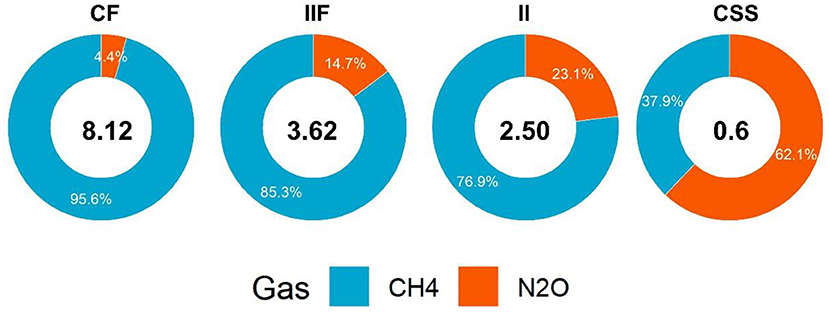
Figure 4. Mean percentage contribution of CH4 and N2O in the overall emissions of rice fields under Continuous Flooding (CF), Intermittent Irrigation (II), and Intermittent irrigation until Flowering (IIF) and Continuous Soil Saturation (CSS) for the period 2014–2019. Numbers in the center of each plot indicate the total seasonal emissions expressed in ton CO2-eq/ha/season.
CSI Values and Intercomparison of Water Management Options Based on DNDC
The performance of the agronomic/biophysical indicators simulated in this study was contrasted among irrigation strategies. The lower GHGs are indicative of the mitigation potential of the strategies, while the low water inputs as the potential indicator of water-saving. The most effective synergy among the irrigation strategies occurred in CSS, where the water-saving and the CH4 mitigation (showed the highest values among treatments). Conversely, CSS presented the lowest N2O mitigation potential. In contrast, the high yields (compared with the other irrigation treatments) and the mitigation of N2O were, on average, the most representative impact of CF treatment. The II and IIF irrigation treatments presented an intermediate methane mitigation potential with a similar impact on yield to CF (Figure 5).
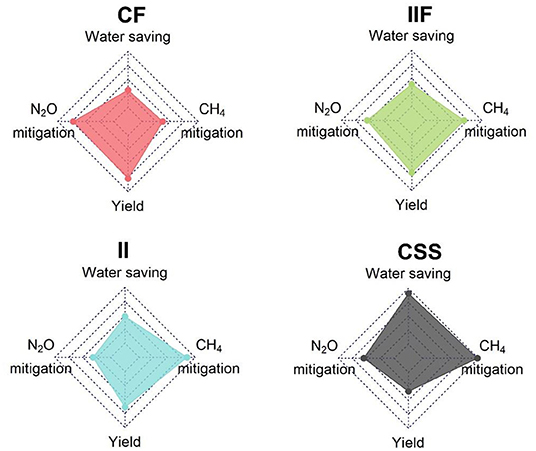
Figure 5. Radar plots of 4 Climate-Smart indicators (Water Saving, yield and, N2O and CH4 mitigation) under Continuous Flooding (CF), Intermittent Irrigation (II), intermittent irrigation until Flowering (IIF) and Continuous Soil Saturation (CSS). The points of the radar plots were calculated using a 0–1 normalization method based on the highest and lowest mean value of each indicator among irrigation treatments and seasons.
To provide a quantitative measure of climate-smartness based on WP and GHGI, the Climate-Smartness Index (CSI) was calculated (Figure 6). The CSI can provide a quantitative measure of the relation between WP and GHGI, where the climate-smartness is expressed by relatively lower GHG emissions, high productivity and high water use efficiency. Based on CSI results, the climate-smartness of irrigation treatments from high to low is in the following order: CSS>II>IIF>CF. The CF treatment presents the lowest climate-smartness (−0.14, with the lowest CSI scores reported in the 2014/2015 season: CSI = −0.2). The IIF and II also score negative CSI values, ranging between −0.083 to −0.054 for IIF and −0.061 to −0.02 for II. On the contrary, CSS presented the highest CSI scores ranging between −0.027 to 0.1 and was the only treatment to score positive CSI. The Climate-Smartness Index (CSI) varied among cropping seasons. The CSS treatment showed the highest climate-smartness during the 2016/2017 and 2018/2019 seasons, while for II and IIF, the highest CSI occurred during the 2016/2017 season. The Continuous Flooding (CF) treatment expressed the lowest climate-smartness in the 2014/2015 season, which improved during the 2016/2017 season.
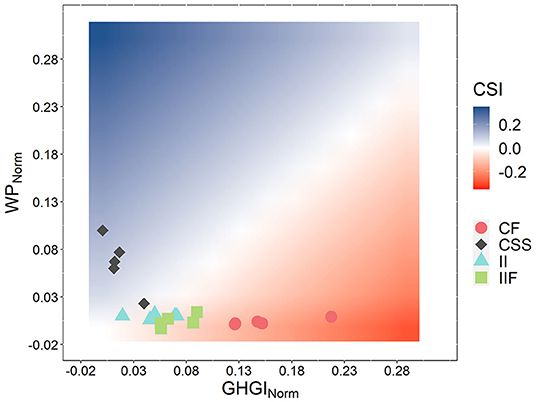
Figure 6. Heatmap of Climate-smartness Index (CSI) scores of the Continuous flooding (CF), Intermittent irrigation until Flowering (IIF), intermittent irrigation (II) and Continuous soil saturation (CSS) treatments.
Sensitivity Analysis of Climate-Smartness Index
The CSI was calculated to assess the sensitivity of CSI to changes in climate for the four treatments and five seasons under different temperature and rainfall scenarios (Figure 7). According to the results, CSI is sensitive to changes in temperature: warmer conditions reduce the climate-smartness in all treatments, while a reduction in temperature improve the CSI scores. The Climate-Smartness Index (CSI) in CSS treatment presents the lowest sensitivity to temperature, followed by the II treatment. Continuous Flooding (CF) and IIF treatment showed higher sensitivity to changes in temperature, where +1°C reduced the CSI up to 26% and +2°C up to 42%. Climate-smartness increased between 1 to 25% in CF and IIF treatments when the temperature decreased to −1°C and 11 to 34% at −2°C. The CSI in CSS treatment increased between 0.6 to 11% with temperature −1°C to ambient and 4–17% at −2°C.
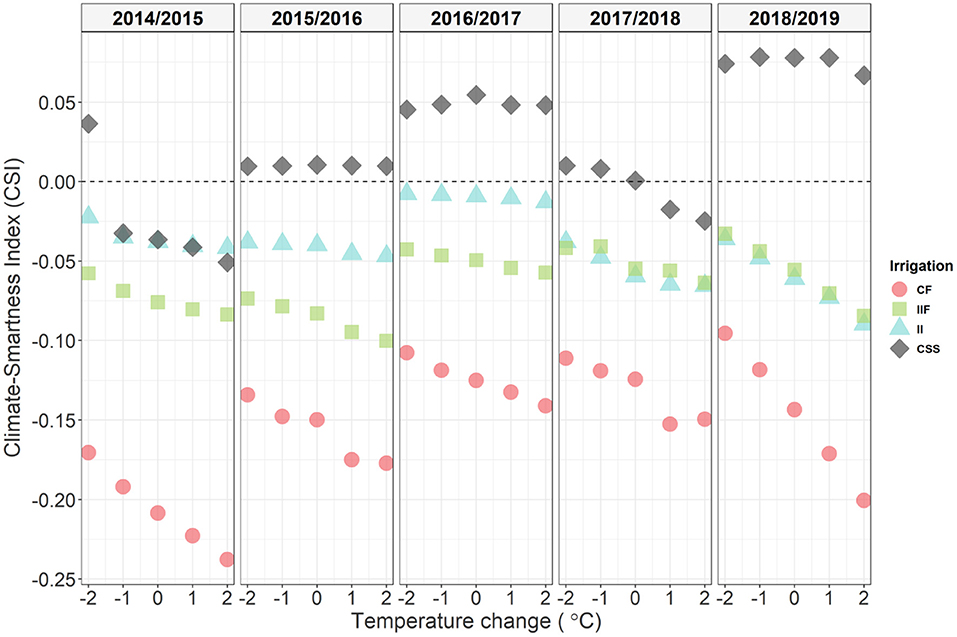
Figure 7. Sensitivity analysis of Climate-Smartness Index (CSI) to changes on temperature of rice crop under Continuous flooding (CF), Intermittent Irrigation until Flowering (IIF), Intermittent Irrigation (II) and Continuous soil saturation (CSS) treatments.
The sensitivity of CSI to rainfall was lower than the temperature (Figure 8). The CSI decreased when the precipitation increased for all treatments during 2014/2015 and 2017/2018, which correspond to the highest cumulative rainfall seasons. During 2015/2016 and 2016/2017, CSI slightly increased (0–1%) in all treatments except for CSS, where CSI decreased in scenarios with more rainfall. The treatment with the highest sensitivity to rainfall changes was the CSS; CSI decreased between 7 and 15% for each 5% increase in rainfall. Irrigation demand decreased proportionally with the increase in rainfall. In the DNDC model, the increment of rainfall reduces the irrigation demand; thus water productivity (WP) presented negligible changes in treatments like CF, IIF, and II. On the contrary, the WP in CSS treatments decreased in the scenarios with increased rainfall because the TWI increases were higher than yield gains.
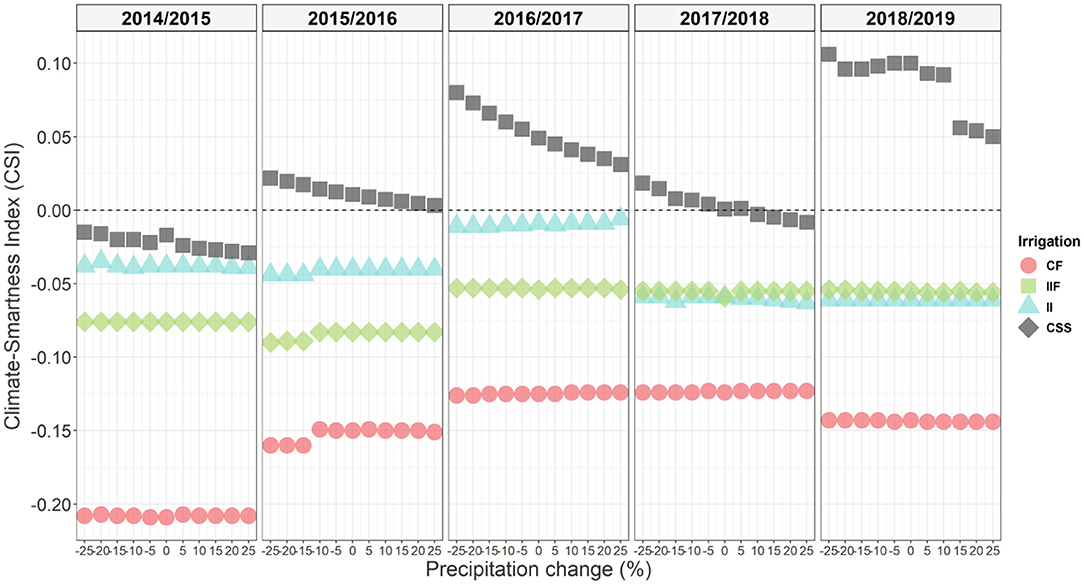
Figure 8. Sensitivity of Climate-Smartness Index (CSI) to changes on rainfall of rice crop under Continuous flooding (CF), Intermittent irrigation until flowering (IIF), intermittent irrigation (II) and Continuous soil saturation (CSS) treatments.
Greenhouse gas emissions showed negligible sensitivity to rainfall in CF, II, and IIF treatments. However, the yields increased between 0 and 3% for each 5% increase in rainfall. This yield/ rainfall synergy reduce the GHGI; increasing the climate-smartness of CF, IIF, and II treatments during 2015/2016 and 2016/2017. GHG emissions were more sensitive in CSS treatment than in CF, II and IIF treatments. Although CH4 emissions increased by <1% for each +5% increase in rainfall, N2O increased up to 18% while drier conditions reduced N2O and CH4 emissions. As the GHG emissions and yields increased proportionally across the increased rainfall scenarios, the GHGI was stable; thus, the climate-smartness was mainly affected by the reduction in WP in CSS treatments.
Discussion
Use of the DNDC Model to Simulate Climate-Smart Water Management Options
This study used the DNDC model with the Climate-Smartness Index (CSI) to assess the climate-smartness of irrigation management strategies in irrigated rice systems. Driven by field data, the DNDC model simulated various irrigation strategies. The model outputs were used to develop a climate-smartness assessment and evaluate the sensitivity of CSI to temperature and rainfall. Process-based models are useful tools to evaluate the performance of agronomic strategies in a wide range of contexts and climate scenarios (Xiong et al., 2014). Thus, the modeling approach represents a cost-efficient method to evaluate climate-smart strategies, explore the effectiveness of scaling up CSA interventions and interpret the trade-offs and synergies between mitigation, adaptation, and productivity across different time and spatial scales.
The DNDC model has been used to simulate GHG emissions and soil carbon dynamics in a wide range of crops and agronomic management at the site and regional scales. The list of published studies that use DNDC is available on the Global DNDC network webpage. Most of the studies that have applied DNDC to rice fields have focused on the modeling of GHG emissions; however, several published papers reported the use of DNDC to simulate rice yields. Studies such as Tian et al. (2018), Pandey et al. (2021) and Shi et al. (2021) modeled the trade-offs between GHG emissions and yields. Moreover, Tian et al. (2021) simulated the relationship between yields, GHG emissions and TWI. In this study, the first step toward modeling climate-smartness metrics was to evaluate the performance of the DNDC model to simulate GHG emissions, yield, and water input. The model validation indicates that the DNDC model performed well in simulating rice yields and seasonal CH4 emissions; however, it underestimated N2O emissions and showed discrepancies between observed and simulated Total Water Inputs (TWI).
Although DNDC is not a crop model, the yield simulations achieve reasonable results. Zhang and Niu (2016) drew similar conclusions from their review, which summarized the application of the DNDC model to crop modeling. The authors remarked that rice, maize, barley, rapeseed, soybean and sugar beets are the main crops simulated in DNDC and count with validations in several geographical contexts. Ku et al. (2019) obtained similar validation results for rice yields [nRMSE (%) = 15–19%] under different fertilization schemes using DNDC. Similarly, Pandey et al. (2021) reported a consistent RMSE with this study for flooded rice systems with organic fertilization. It is necessary to adjust the default crop parameters (optimum crop yield, biomass fraction, and biomass C/N ratio) to improve yield estimations in DNDC; this is also important for improving the fit of GHG emissions simulations (Nie et al., 2019).
Despite the reasonably good results that have been obtained by DNDC simulating rice yields, the model presents limitations modeling detailed physiological and phenological processes Tian et al. (2018). Moreover, the modeling of yields at regional scales using DNDC may be limited by the calibration approach used for the model where only one rice cultivar can be calibrated regardless of the area covered (Zhang and Niu, 2016). An alternative to overcome such limitations could be the coupling with crop models such as ORYZA200O, DSSAT or CERES-Rice
The observed and simulated GHGs emissions data resulted in a good fit of Net-GWP despite the underestimation of N2O emissions; except in the case of the CSS treatment during the 2016/2017 season, in which the N2O represents the main contributor of overall GWP. Similar results were reported by Zhang et al. (2019) argue that, despite discrepancies in the N2O simulation, owing to the strong agreement with methane fluxes and the low contribution of N2O the model can be used to estimate GWPs from tropical paddy fields. The poor performance of the model in simulating N2O may occur because the model assumes homogeneous microbial distribution and over/underestimates the soil moisture under different soil drainage conditions (Tonitto et al., 2010). Moreover, the DNDC model simulates suppressed rates of nitrification in anoxic soil conditions (i.e., during continuous flooding periods), assuming zero N2O emissions (Babu et al., 2006; Hao et al., 2016).
In addition, nitrification and denitrification occurred simultaneously in the soil during a redox condition window between well-drained and saturated soil conditions, thus inaccuracies in the parametrization of water affect the estimation of Eh and the concentrations of and in the soil (Simmonds et al., 2015). Our results confirm that poor N2O simulation may not affect the net GWP of treatments with negligible N2O emissions; however, the accuracy of N2O gains relevance when assessing the mitigation potential of water management strategies that are prone to increase N2O emissions as the case of AWD (Lagomarsino et al., 2016) or mid-season drainage (Liu et al., 2019).
Flooding was modeled based on the irrigation schedule and the duration of flooding events evidenced by the records in the hydrometer. This parametrization approach may lead to discrepancies in the amount of water used and the water column. The parametrization of irrigation treatments can be complex when is consider the approach to modeling soil hydrology in DNDC. The model uses a tipping bucket water flow model that drains the soil profile to field capacity, which could generate an underestimation of soil moisture in treatments like CSS where soil keep saturated or above field capacity. Moreover, the fact that DNDC can underestimate rainfall drainage could lead to the systematic overestimation of TWI during the 2017/2018 season that showed the highest cumulative rainfall (Kiese et al., 2005; Kröbel et al., 2010; Uzoma et al., 2015). Although discrepancies in the estimations, simulated TWI, were consistent among treatments and comparable with TWI observed for the same treatments in other studies (Li et al., 2005; Tian et al., 2021).
Climate-Smartness Water Management Options and Its Sensitivity to Climate
The simulated CH4 and N2O emissions in this study were consistent with those observed in other studies. The net-GWP observed in the CF treatments are within the emissions range observed by Jiang et al. (2019) in their meta-analysis, which also found a similar percent reduction between CF and controlled CF (53%) that in this study is equivalent to II treatment. The CSS treatment showed the highest climate-smartness; however, the reduction in yield could discourage farmers from adopting it. These results evidenced that CSS management enhance agronomic efficiency; however, the economic implications of implement CSS needs to be considered before to recommend its adoption as a climate-smart practice. An example is an economic study reported by Ishfaq et al. (2021), which recognized that aerobic rice systems have a low cost of implementation and high potential as a water-saving technique; however, the net returns are also lower compared with CF conditions.
When the yields are analyzed in function of the net returns, the CSS management could incur in a higher cost per unit of product. Cost/benefits analyses are needed to integrate economic indicators within the climate-smartness assessments. Identifying when irrigation strategies show promising mitigation and adaptation potential are economically feasible for small farmers and subsistence farming is equally important to agronomic performance. This type of analysis will contribute to finding better strategies to overcome adoption barriers.
The sensitivity analysis evidenced that the performance of irrigation managements can vary depending on changes in climate. The extent of these impacts depend of the interaction between soil parameters and climate. For instance, the adoption of severe and mild AWD strategies in sandy soils could lead to yield penalties compared with CF treatments; moreover, the water-saving potential of irrigation strategies might differ among wet and dry seasons due to soil factors such as percolation rates and SOC content Carrijo et al. (2017). These results show the importance of irrigation suitability assessments, such as those developed by Nelson et al. (2015), who used a water balance model to determine the areas climatically suitable for AWD.
Reductions in climate smartness in warmer temperatures are associated with the increase of GHG emissions and- to some extent- by the reduction of WP. High temperatures will increase crop evapotranspiration and the water demand. Thus, WP will decrease either way, by an increment of water requirements to maintain yields or by a reduction of yield by water stress. Accordingly, rice cultivation in warmer conditions would require additional water (Hossain et al., 2021), which, in turn, could increase methane emissions. Conversely, cooler temperatures result in lower GHG emissions and water demand, reflected in the higher CSI scores in irrigated rice compared with warmer temperatures. These results agreed with studies reported by Minh et al. (2015) and Nie et al. (2019), where DNDC model simulate the sensitivity of methane emissions to climate and found that, while increased precipitation has a negligible impact on the CH4 emissions, warmer temperatures significantly elevate them.
Deng et al. (2016) reported similar results regarding the impact of precipitation and temperature in N2O emissions. The authors argue that precipitation could stimulate microbial activity (Giltrap et al., 2010), particularly in dry soils. Rainfall in dry soil can generate a optimal “soil moisture window” to the nitrification/denitrification processes. Several studies reported such optimal conditions; Liu et al., 2022 found the higher net N2O emissions between wetter (80–100% WHC) and drier (20–60%) soils. For its part, Ciarlo and Bartoloni (2007) reported in their incubation experiment that Overall N2O daily emissions were highest at 80% WFPS. N2O emissions under 100% WFPS treatment were significantly greater than those soils under 40% WFPS. Although the N2O production also depends on soil texture and organic matter content, several studies coincide with the optimal water content for N2O production under field capacity conditions (from approx. 60% to 100% WFPS%). Soil moisture conditions become less optimum for N2O production from wilting point (approximately 15% WFPS in coarse soil texture and 32 to 26% in fine texture soils), where water scarcity can constraint biological activity (Wang et al., 2021). These results might explain the lower N2O emissions obtained during the driest season in 2019 and the reduction of climate-smartness (by the increasing of GWP) in the rainfall scenarios above 10% of rainfall increase.
This finding may explain the highest sensitivity of N2O emissions to precipitation in CSS treatment during the driest season (2018-2019) of the period assessed, where N2O increased up to 18% when precipitation increased by 25%. Minamikawa et al. (2016) attribute the increment of CH4 under warmer temperatures to the acceleration of SOM decomposition and N mineralization driven by a stimulation of biological activity in the soil. The authors also pointed out that the effect of temperature on GHG emissions may vary among climates zones, having a higher sensitivity in low temperatures compared to warmer places. Given that mineralization rates may increase under warmer conditions, the SOM become a relevant parameter for mitigation in rice systems. In this sense, modeling-based assessments would be more suitable to elucidate a wider view of soil carbon in the long term in rice fields.
Although the CSI showed a low sensitivity to rainfall, the irrigation demand was lower in all treatments with higher rainfall. This occurred because in DNDC if the crop water demand is the same, the larger the proportion of rainfall the crop will be less dependent on irrigation. A reduction of irrigation demand is desirable and could represent a contribution to climate-smartness if it contributes to increasing water-use efficiency. The sensitivity of climate-smartness to temperature and rainfall reinforces the idea of the strong context-dependency of climate-smart agriculture. For instance, CSS proved to be the irrigation management with the highest climate-smartness in the study site; however, climate change could bring about changes in the climate-smartness of CSS, potentially even reducing it to negative values of CSI.
This sensitivity analysis assumed a constant concentration of atmospheric CO2 across cropping seasons. However, it is worth mentioning that rising atmospheric CO2 trigger an increment in the photosynthetic rate in the plants, resulting in higher biomass accumulation (Lv et al., 2020). In the case of the rice crop, Ainsworth (2008) reported from their meta-analysis, elevated CO2 increased rice yields by 23%, as a response to increased grain mass, panicle, and grain number. Moreover, the stomatal conductance reduction under elevated CO2 can increase water productivity and indirectly reduce greenhouse gas intensity. Although elevated CO2 might potentially improve climate-smartness indicators, severe changes in temperature and rainfall can overshadow yield gains. For instance, Krishnan et al. (2007) simulated the impact of the interaction between elevated CO2 and temperature on rice yields, finding a trade-off between both parameters. Under elevated CO2 (25% on average) yield increase until the temperature increased between +3°C to +5°C, resulting in yield penalties between −10.5 and −34%.
Conclusions
In this study, we used the DNDC model to simulate cumulative CH4 and N2O fluxes, rice yields and water inputs from tropical irrigated rice systems under several irrigation managements. The DNDC model simulations showed a good fit with the methane and yield observations. Nitrous oxide fluxes and water inputs were poorly simulated, evidencing the need for an adequate parameterization of hydrological parameters.
Results demonstrate that seasonal variability in climate may influence the performance of irrigation management practices. Temperature increases can reduce- even reverse- the mitigation potential of irrigation management. Thus water-oriented strategies must be able to be adjusted responsively to the climate if they are to be an effective adaptation measure.
Combining models and CSI can offer spatial and temporal continuity to climate-smartness analyses, strengthening the discussion around the context-dependency of CSA. Modeling agronomic and biophysical indicators bring valuable information, fills data gaps in existing experiments and generates evidence from scenarios that otherwise will be technically impossible to measure (e.g., climate projections or hypothetical socio-economic scenarios). Furthermore, CSI can synthesize model output and thus facilitate the interpretation of model results.
Data Availability Statement
The raw data supporting the conclusions of this article will be made available by the authors, without undue reservation.
Author Contributions
LA-C performed process-based model simulations, analyzed the data from the field experiment, and wrote the paper with the critical feedback provided by all authors. AH, MS, and AS performed the field measurements and processed the experimental data. JR-V, SW, and AC supervised the findings of this work and helped shape the research, analysis, and manuscript writing. All authors contributed to the article and approved the submitted version.
Funding
This work was implemented as part of the CGIAR Research Program on Climate Change, Agriculture and Food Security (CCAFS), which is carried out with support from the CGIAR Trust Fund and through bilateral funding agreements. For details please visit https://ccafs.cgiar.org/donors.
Author Disclaimer
The views expressed in this document cannot be taken to reflect the official opinions of these organizations.
Conflict of Interest
AH, MS, and AS were employed by the Brazilian Agricultural Research Corporation (EMBRAPA) Arroz e Feijão.
The remaining authors declare that the research was conducted in the absence of any commercial or financial relationships that could be construed as a potential conflict of interest.
Publisher's Note
All claims expressed in this article are solely those of the authors and do not necessarily represent those of their affiliated organizations, or those of the publisher, the editors and the reviewers. Any product that may be evaluated in this article, or claim that may be made by its manufacturer, is not guaranteed or endorsed by the publisher.
Supplementary Material
The Supplementary Material for this article can be found online at: https://www.frontiersin.org/articles/10.3389/fsufs.2022.873957/full#supplementary-material
References
Ainsworth, E. A.. (2008). Rice production in a changing climate: A meta-analysis of responses to elevated carbon dioxide and elevated ozone concentration. Global Change Biol. 14, 1642–1650. doi: 10.1111/j.1365-2486.2008.01594.x
Arenas-Calle, L. N., Whitfield, S., and Challinor, A. J. (2019). A Climate Smartness Index (CSI) based on greenhouse gas intensity and water productivity: application to irrigated rice. Front. Sustain. Food. syst. 3, 1–13. doi: 10.3389/fsufs.2019.00105
Babu, Y. J., Li, C., Frolking, S., Nayak, D. R., and Adhya, T. K. (2006). Field validation of DNDC model for methane and nitrous oxide emissions from rice-based production systems of India. Nutr. Cycling Agroecosyst. 74, 157–174. doi: 10.1007/s10705-005-6111-5
Barbosa, L.. (2018). Manejo Alternativo De Irrigação Para O Arroz (Ph.D. Thesis). Instituto Federal De Edução, Cîencia E Tecnologia Goiano – Campus Ceres. https://repositorio.ifgoiano.edu.br/handle/prefix/91
Carrijo, D. R., Lundy, M. E., and Linquist, B. A. (2017). Rice yields and water use under alternate wetting and drying irrigation: a meta-analysis. Field Crops Res. 203, 173–180. doi: 10.1016/j.fcr.2016.12.002
Ciarlo, E. A., and Bartoloni, N. G. (2007). The effect of moisture on nitrous oxide emissions from soil and the N2O /(N 2O + N2) ratio under laboratory conditions. Biol. Fertil. Soils. 43, 675–681 doi: 10.1007/s00374-006-0147-9
de Castro, J. R.. (2020). Avalidação de metodos de calibração do modelo ORYZA para estimar produtividade do arroz irrigado (Doctoral thesis). Universidade Federal de Goiás. Available online at: http://repositorio.bc.ufg.br/tede/handle/tede/10763
de Morais, O. P., Torga, P. P., Cordeiro, A. C. C., Pereira, J. A., de Magalhaes Júnior, A. M., and Colombari Filho, J. M. (2016). BRS Catiana: Cultivar de Arroz Irrigado de Elevada Produtividade e Ampla Adaptação. Santo Antônio de Goiás: Embrapa Arroz e Feijão. Comunicado técnico 233. GO. ISSN. 1678-961X. Available online at: http://ainfo.cnptia.embrapa.br/digital/bitstream/item/139116/1/CNPAF-ComTec233.pdf
Deng, Q., Hui, D., Wang, J., Yu, C. L., Li, C., Reddy, K. C., et al. (2016). Assessing the impacts of tillage and fertilization management on nitrous oxide emissions in a cornfield using the DNDC model. J. Geophys. Res. Bio-geosci. 121, 337–349. doi: 10.1002/2015JG003239
dos Santos, M. P., Zanon Junior, A., Cuadra, S. V., Steinmetz, S., de Castro, J. R., and Heinemann, A. B. (2017). Yield and morphophysiological indices of irrigated rice genotypes in contrasting ecosystems. Pesquisa Agropecuaria Trop. 47, 253–264. doi: 10.1590/1983-40632016v4745955
Duffy, C., Murray, U., Nowak, A., Girvetz, E., Corner-Dolloff, C., Twyman, J., et al. (2017). National Level Indicators for Gender, Poverty, Food Security, Nutrition and Health in Climate-Smart Agriculture (CSA) activities. Report number: CCAFS Working Paper no. 195. Copenhagen, Denmark: CGIAR Research Program on Climate Change.
Fragoso, D. de B., Rangel, P. H. N., Carvalho, R. N., and Cardoso, E. A. (2021). Contribuição das cultivares de arroz da embrapa na produção de arroz irrigado no estado do Tocantins. Revista Agri-Environ. Sci. 7, e021005. doi: 10.36725/agries.v7i2.5440
Giltrap, D. L., Li, C., and Saggar, S. (2010). DNDC: a process-based model of green-house gas fluxes from agricultural soils. Agricult. Ecosyst. Environ. 136, 292–300. doi: 10.1016/j.agee.2009.06.014
Hao, Q., Jiang, C., Chai, X., Huang, Z., Fan, Z., Xie, D., et al. (2016). Drainage, no-tillage and crop rotation decreases annual cumulative emissions of methane and nitrous oxide from a rice field in Southwest China. Agricult. Ecosyst. Environ. 233, 270–281. doi: 10.1016/j.agee.2016.09.026
Hossain, M. B., Roy, D., Maniruzzaman, M., Biswas, J. C., Naher, U. A., Haque, M. M., et al. (2021). Response of crop water requirement and yield of irrigated rice to elevated temperature in Bangladesh. Int. J. Agron. 2021, 11. doi: 10.1155/2021/9963201
Ishfaq, M., Akbar, N., Zulfiqar, U., Ali, N., Shah, F., Anjum, S. A., et al. (2021). Economic assessment of water-saving irrigation management techniques and continuous flooded irrigation in different rice production systems. Paddy Water Environ. 20, 37–50. doi: 10.1007/s10333-021-00871-6
Jantalia, C. P., dos Santos, H. P., Urquiaga, S., Boddey, R. M., and Alves, B. J. R. (2008). Fluxes of nitrous oxide from soil under different crop rotations and tillage systems in the south of Brazil. Nutr. Cycl. Agroecosyst. 82, 161–173. doi: 10.1007/s10705-008-9178-y
Jiang, Y., Carrijo, D., Huang, S., Chen, J., Balaine, N. van Groeni-gen, K. J., Linquist, B., et al. (2019). Water management to mitigate the global warming potential of rice systems: a global meta-analysis. Field Crops Res. 234, 47–54. doi: 10.1016/j.fcr.2019.02.010
Kiese, R., Li, C., Hilbert, D. W., Papen, H., and Butterbach-Bahl, K. (2005). Regional application of PnET-N-DNDC for estimating the N2O source strength of tropical rain forests in the Wet Tropics of Australia. Global Change Biol. 11, 128–144. doi: 10.1111/j.1365-2486.2004.00873.x
Krishnan, P., Swain, D. K., Bhaskar, B. C., Nayak, S. K., and Dash, R. N. (2007). Impact of elevated CO2 and temperature on rice yield and methods of adaptation as evaluated by crop simulation studies. Agricult. Ecosyst. Environ. 122, 233–242. doi: 10.1016/j.agee.2007.01.019
Kröbel, R., Sun, Q., Ingwersen, J., Chen, X., Zhang, F., Müller, T., et al. (2010). Modeling water dynamics with DNDC and DAISY in a soil of the North China Plain: A comparative study. Environ. Model. Softw. 25, 583–601. doi: 10.1016/j.envsoft.2009.09.003
Ku, H. H., Ryu, J. H., Bae, H. S., Jeong, C., and Lee, S. E. (2019). Modeling a long-term effect of rice straw incorporation on SOC content and grain yield in rice field. Arch. Agron. Soil Sci. 65, 1941–1954. doi: 10.1080/03650340.2019.1583330
Lagomarsino, A., Agnelli, A. E., Linquist, B., Adviento-Borbe, M. A. Agnelli, A., Gavina, G., Ravaglia, S., and Ferrara, R. M. (2016). Alternate wetting and drying of rice reduced CH4 emissions but triggered N2O peaks in a clayey soil of central italy. Pedosphere. 26, 533–548. doi: 10.1016/S1002-0160(15)60063-7
Li, C.. (2000). Modeling trace gas emissions from agricultural ecosystems. Nutr. Cycl. Agroecosyst. 58, 259–276. doi: 10.1023/A:1009859006242
Li, C., Frolking, S., Xiao, X., Moore III, B., Boles, S., Qiu, J., et al. (2005). Modeling impacts of farming management alternatives on CO2, CH4, and N2O emissions: A case study for water management of rice agriculture of China. Glob. Biogeochem. Cycles. 19, GB3010. doi: 10.1029/2004GB002341
Liu, H., Zheng, X., Li, Y., Yu, J., and Ding, H. (2022). Soil moisture determines nitrous oxide emission and uptake. Sci. Total Environ. 822, 153566. doi: 10.1016/j.scitotenv.2022.153566
Liu, X., Zhou, T., Liu, Y., Zhang, X., Li, L., Pan, G., et al. (2019). Effect of mid-season drainage on CH4 and N2O emission and grain yield in rice ecosystem: a meta-analysis. Agricult. Water Manage. 213, 1028–1035. doi: 10.1016/j.agwat.2018.12.025
Lv, C., Huang, Y., Sun, Y., Yu, L., and Zhu, J. (2020). Response of rice yield and yield components to elevated [CO2]: a synthesis of updated data from face experiments. Eur. J. Agron. 112, 125961. doi: 10.1016/j.eja.2019.125961
Minamikawa, K., Fumoto, T., Iizumi, T. Cha-un, N., Pimple, U., Nishimori, M., Ishigooka, Y., and Kuwagata, T. (2016). Prediction of future methane emission from irrigated rice paddies in central Thailand under different water management practices. Sci. Total Environ. 566–567, 641–651. doi: 10.1016/j.scitotenv.2016.05.145
Minh, N., Trnh, M. V., Wassmann, R., Sander, B. O., Hoa, T., Trang, N. L., et al. (2015). Simulation of methane emission from rice paddy fields in VuGia-Thu Bn River Basin of Vietnam Using the DNDC model: field validation and sensitivity analysis. VNU J. Sci. Earth Environ. Sci. 31. http://repository.vnu.edu.vn
Myhre, G., Shindell, D., Br eon, F-. M., Collins, W., Fuglestvedt, J. J., Huang, D., et al. (2013). Anthropogenic and natural radiative forc-ing. In: Stocker, T., Qin, D., Plattner, K., Tignor, M., Allen, S., Boschung, J., Nauels,A., Xia, Y.and Bex, V., and Midgley, P., editors. Climate Change 2013 the Physical Science Basis: Working Group I Contribution to the Fifth Assessment Report of the Intergovernmental Panel on Climate Change (Cambridge, United Kingdom and New York, NY, USA: Cambridge University Press). p. 659–740. https://www.ipcc.ch/site/assets/uploads/2018/02/WG1AR5_Chapter08_FINAL.pdf
Nelson, A., Wassmann, R., Sander, B. O., and Palao, L. K. (2015). Climate-determined suitability of the water saving technology “alternate wetting and drying” in rice systems: A scalable methodology demonstrated for a province in the Philippines. PLoS ONE. 10, 1–19. doi: 10.3390/plants10010031
Nie, T., Zhang, Z., Qi, Z., Chen, P., Sun, Z., Liu, X., et al. (2019). Characterizing spatiotemporal dynamics of CH4 fluxes from rice paddies of cold region in Heilongjiang province under climate change. Int. J. Environ. Res. Public Health. 16, 692. doi: 10.3390/ijerph16050692
Nowak, A., Rosenstock, T., and Wilkes, A. (2019). Measurement and Reporting of Climate-Smart Agriculture: Technical Guidance for a Country-Centric Process Measurement and Reporting of Climate-Smart Agriculture: Technical Guidance for a Country-Centric Process. CCAFS Working Paper. Available online at: https://hdl.handle.net/10568/101686
Pandey, A., Dou, F., Morgan, C. L., Guo, J., Deng, J., Schwab, P., et al. (2021). Modeling organically fertilized flooded rice systems and its long-term effects on grain yield and methane emissions. Sci. Total Environ. 755, 142578. doi: 10.1016/j.scitotenv.2020.142578
Pringle, P.. (2011). AdaptME: Adaptation Monitoring and Evaluation. UKCIP, Oxford, UK. Available online at: https://www.ukcip.org.uk/wizard/adaptme-toolkit/
Quinney, M., Bonilla-Findji, O., and Jarvis, A. (2016). CSA Programming and Indicator Tool: 3 Steps for Increasing Programming Effectiveness and Outcome Tracking of CSA Interventions. CCAFS Tool Beta version. Copenhagen, Denmark: CGIAR Research Program on Climate Change, Agriculture and Food Security (CCAFS).
Rangel, P. H. N., Torga, P. P., de Morais, O. P., Fragoso, D. d. B., Filho, J. M. C., Cordeiro, A. C. C., et al. (2019). Brs catiana: irrigated rice cultivar with high yield potential and wide adaptation. Crop Breed. Appl. Biotechnol. 19, 368–372. doi: 10.1590/1984-70332019v19n3c51
Shi, Y., Lou, Y., Zhang, Y., and Xu, Z. (2021). Quantitative contributions of climate change, new cultivars adoption, and management practices to yield and global warming potential in rice-winter wheat rotation ecosystems. Agricult. Syst. 190, 103087. doi: 10.1016/j.agsy.2021.103087
Simmonds, M. B., Li, C., Lee, J., Six, J., Van Kessel, C., Linquist, B. A., et al. (2015). Modeling methane and nitrous oxide emissions from direct-seeded rice systems. J. Geophy. Res. Biogeosci. 120, 2011–2035. doi: 10.1002/2015JG002915
Tian, Z., Fan, Y., Wang, K., Zhong, H., Sun, L., Fan, D., et al. (2021). Searching for “Win-Win” solutions for food-water-GHG emissions trade-offs across irrigation regimes of paddy rice in China. Resour. Conserv. Recycl. 16, 094038 doi: 10.1016/j.resconrec.2020.105360
Tian, Z., Niu, Y., Fan, D., Sun, L., Ficsher, G., Zhong, H., et al. (2018). Maintaining rice production while mitigating methane and nitrous oxide emissions from paddy fields in China: Evaluating tradeoffs by using coupled agricultural systems models. Agricult. Syst. 159, 175–186. doi: 10.1016/j.agsy.2017.04.006
Tonitto, C., David, M. B., and Drinkwater, L. E. (2010). Application of the DNDC model to the Rodale Institute Farming Systems Trial: Challenges for the validation of drainage and nitrate leaching in agroecosystem models replacing bare fallows with cover crops in fertilizer-intensive cropping systems: a meta-analysis of crop yield and N dynamics. Nutr. Cycl. Agroecosyst. 87, 483–494. doi: 10.1007/s10705-010-9354-8
United Nations Organization (2015). UN General Assembly, Transforming our world: the 2030 Agenda for Sustainable Development, 21 October 2015, A/RES/70/1. Available online at: https://www.refworld.org/docid/57b6e3e44.html
Uzoma, K. C., Smith, W., Grant, B., Desjardins, R. L., Gao, X., Hanis, K., et al. (2015). Assessing the effects of agricultural management on nitrous oxide emissions using flux measurements and the DNDC model. Agricult. Ecosyst. Environ. 206, 71–83. doi: 10.1016/j.agee.2015.03.014
van Wijk, M. T., Merbold, L., Hammond, J., and Butterbach-Bahl, K. (2020). Improving assessments of the three pillars of climate smart agriculture: current achievements and ideas for the future. Front. Sustain. Food Syst. 4, 558483. doi: 10.3389/fsufs.2020.558483
Wang, C., Amon, B., Schulz, K., and Mehdi, B. (2021). Factors that influence nitrous oxide emissions from agricultural soils as well as their representation in simulation models: A review. Agronomy 11, 770. doi: 10.3390/agronomy11040770
Wassmann, R., Villanueva, J., Khounthavong, M., Okumu, B. O., Vo, T. B. T., Sander, B. O., et al. (2019). Adaptation, mitigation and food security: multi-criteria ranking system for climate-smart agriculture technologies illustrated for rainfed rice in Laos. Global Food Secur. 23, 33–40. doi: 10.1016/j.gfs.2019.02.003
World Bank (2016). Climate Smart Agriculture Indicators. World Bank Group Report No. 105162-GLB. Washington, DC: World Bank.
Xiong, W., van der Velde, M., Holman, I. P., Balkovic, J., Lin, E., Skalsk y, R., et al. (2014). Can climate-smart agriculture reverse the recent slowing of rice yield growth in China? Agricult. Ecosyst. Environ. 196, 125–136. doi: 10.1016/j.agee.2014.06.014
Zhang, X., Bi, J., Sun, H., Zhang, J., and Zhou, S. (2019). Greenhouse gas mitigation potential under different rice-crop rotation systems: from site experiment to model evaluation. Clean Technol. Environ. Policy. 21, 1587–1601. doi: 10.1007/s10098-019-01729-6
Keywords: climate-smart agriculture, climate-smartness, crop model, climate-smart indicators, water productivity, greenhouse gas intensity, DNDC
Citation: Arenas-Calle LN, Heinemann AB, Soler da Silva MA, dos Santos AB, Ramirez-Villegas J, Whitfield S and Challinor AJ (2022) Rice Management Decisions Using Process-Based Models With Climate-Smart Indicators. Front. Sustain. Food Syst. 6:873957. doi: 10.3389/fsufs.2022.873957
Received: 11 February 2022; Accepted: 21 June 2022;
Published: 22 July 2022.
Edited by:
Bruno José Rodrigues Alves, Brazilian Agricultural Research Corporation (EMBRAPA), BrazilReviewed by:
Aung Zaw Oo, Japan International Research Center for Agricultural Sciences (JIRCAS), JapanNobuko Katayanagi, National Agriculture and Food Research Organization (NARO), Japan
Copyright © 2022 Arenas-Calle, Heinemann, Soler da Silva, dos Santos, Ramirez-Villegas, Whitfield and Challinor. This is an open-access article distributed under the terms of the Creative Commons Attribution License (CC BY). The use, distribution or reproduction in other forums is permitted, provided the original author(s) and the copyright owner(s) are credited and that the original publication in this journal is cited, in accordance with accepted academic practice. No use, distribution or reproduction is permitted which does not comply with these terms.
*Correspondence: Laura N. Arenas-Calle, ZWVsbmFjQGxlZWRzLmFjLnVr; bG5hcmVuYXNjQGdtYWlsLmNvbQ==