- School of Management, Guangzhou University, Guangzhou, China
The agricultural sector faces a food crisis and major challenges in green and sustainable development, and digital technology is an important countermeasure. In this paper, “digital technology” is used as a keyword to construct a regional digitalization level indicator. China's provincial panel data from 2013 to 2020 are adopted as samples to explore the impact of regional digitalization levels on agricultural total factor productivity and its mechanism. The study found the following: (1) Regional digitalization can significantly raise agricultural total factor productivity. (2) Regional digitalization can significantly raise agricultural total factor productivity in economically underdeveloped areas but not in economically developed areas. (3) The mitigation of factor market distortion and large-scale production can strengthen the role of regional digitalization in raising agricultural total factor productivity.
Introduction
In order to cope with the food security issue brought about by population growth, government, and researchers have focused on the efficiency of agricultural production. Improving agricultural total factor productivity not only is a key way to solve the problem of global food security but also can advance the agricultural sector and the overall economy by adjusting the allocation of labor (Cao and Birchenall, 2013; Ball et al., 2015). It is particularly important for the economic growth of poor countries or regions (Fuglie, 2018). However, the agricultural production in economically developed regions such as the United States and Europe is slowing down. Although the growth rate of agricultural total factor productivity in developing countries such as China is relatively high (Alston and Pardey, 2014), extensive growth impacts the ecological environment, leading to issues such as excessive fertilization, forest destruction, and water pollution (Ju et al., 2009; Schwarzenbach et al., 2010; Laurance et al., 2014). As such, the transformation of the agricultural production model is imminent. The digital transformation of agriculture reduces the use of chemical products, saves water resources, and increases per unit output and environmental sustainability. It is another technological upgrade following agricultural mechanized production. With the emergence of digital technology, global agriculture faces restructuring and transformation. Digital technology is a strong driving force for environmentally friendly and sustainable agricultural growth.
Digitization is the social technological process of applying digital innovation (Klerkx et al., 2019). Emerging technologies such as big data, the Internet of Things, and artificial intelligence not only have a great impact on the business economy (Chen et al., 2012; Ng and Wakenshaw, 2017) but also will reshape traditional agricultural production. Agricultural digitalization is to integrate advanced digital technologies such as artificial intelligence, big data, and robotics and connect these digital technologies to agricultural production systems through the Internet of things (Lioutas et al., 2021). In addition, digital technology will also play a role in the entire agricultural production chain (Shepherd et al., 2020). Digital technology will gradually become a part of the agricultural production infrastructure. Although it can have a positive effect on agricultural production efficiency, high investment costs and the digital divide between technology adaptors limit the widespread adoption of agricultural digital technology (Benyam et al., 2021). Under these circumstances, government investment in digital construction not only helps popularize digital production technology to farmers but also improves the digital and intelligent levels of the external environment and promotes the deep integration of digital technology with the agricultural real economy. As a result, the agricultural production process and the circulation of elements in the value chain will be optimized, the “digital divide” will be narrowed, and the cost for laborers to adapt to digital technology will be reduced, thereby increasing the agricultural total factor productivity and ultimately pressing ahead with agricultural green and sustainable production.
This study explores the impact of digitalization levels on agricultural total factor productivity through the establishment of a fixed-effect model of provincial samples, and further determines the causal relationship between regional digitalization levels and agricultural total factor productivity through IV-GMM method. The study has found that the overall digitalization of a region not only promotes the digital transformation of agricultural production but also effectively improves market information communication and value chain element circulation, thereby increasing agricultural total factor productivity. The heterogeneity test results show that, in economically underdeveloped areas, digitalization can significantly increase agricultural total factor productivity. In addition, alleviated distortion of market factor allocation and large-scale production can positively enhance the promotion effect of regional digitalization level on agricultural total factor productivity. We believe that digital development is an effective means for countries to transform agricultural production and improve agricultural production efficiency.
The theoretical contributions of this paper mainly include: First, an indicator is developed to measure regional digitalization levels based on the Internet big data search engine. To study the effect of China's digital construction, the level of digitalization must be measured. However, the lack of measurement research on this indicator hinders the development of related theories. The paper measures regional digitalization levels based on the number of keyword searches related to digital technology in prefecture-level cities or municipalities in Baidu News, which provides a reference for subsequent research on digitalization. Second, the impact on agricultural total factor productivity is analyzed from the overall perspective of regional digital construction, breaking the shackles of the current research framework. Previous empirical studies on agricultural total factor productivity are often limited to the impact of input factors or agricultural policies, including natural conditions (Liang et al., 2017), farm size (Rada and Fuglie, 2019), R&D expenditure (Fuglie, 2017), agricultural subsidy policy (Rizov et al., 2013), and agricultural trade policy (Yoo et al., 2012), while this paper incorporates the regional digital environment into the analysis framework, with the focus on the overall environment of agricultural production with government participation, which contributes to the understanding of emerging production technologies and factors affecting agricultural total factor productivity, and enriches the literature on the effect of regional digital development and studies on the growth factors of agricultural total factor productivity.
At a practical level, the impact of digitalization levels on agricultural total factor productivity is analyzed from the perspectives of market factor allocation, large-scale production, and the heterogeneous results caused by differences in economic development levels. The analysis shows that regional digitalization can significantly increase agricultural total factor productivity, especially in regions with lower levels of economic development. In addition, an increase in the efficiency of factor allocation in the external market and a commitment to large-scale production will strengthen the role of regional digitalization in raising agricultural total factor productivity. This provides useful empirical evidence for the government to promote the deep integration of digital construction and agricultural production.
The remaining part of this paper is structured as follows: The second part is the research plan, which elaborates the mechanism of the impact of regional digitalization on agricultural total factor productivity, empirical model construction, data sources, and variable selection and conducts a descriptive statistical analysis. The third part contains the econometric test, endogenous treatment, and robustness test of digitalization on agricultural total factor productivity and analyzes the heterogeneity of the economic development level and the adjustment mechanism of factor configuration distortion and large-scale production. The fourth part provides conclusions and recommendations.
Research Plan
Research Hypothesis
Regional digitalization promotes the optimization of the entire process of agricultural production through agricultural digital technology, thereby increasing the agricultural total factor productivity. Agricultural digital technologies such as big data and the Internet of Things help control agricultural production and improve agricultural efficiency (Wolfert et al., 2017). Food traceability technology derived from blockchain and Internet technology can manage food safety issues, improve the transparency of supply chains, and advance sustainable development (Creydt and Fischer, 2019; Kamble et al., 2020). Information and communication technology can help increase the efficiency of resource use in food businesses as well as the productivity of pesticides and fertilizers (Radoglou-Grammatikis et al., 2020). Technologies such as artificial intelligence, smart robots, and the Internet of Things form smart water conservancy technologies that help the effective use of agricultural water resources (Muangprathub et al., 2019). These technological advances enable the effective use of resources and the improvement of agricultural total factor productivity.
The role of digitalization in raising agricultural total factor productivity is also reflected in optimizing the external market environment and improving agricultural policies. George (George, 2014) pointed out that agricultural total factor productivity in developing countries has increased through investments in irrigation and machinery, but crop production in these countries still lags behind in terms of the progress of agronomy. The important reason behind this is that farmers in developing countries who are poorly educated and risk-averse prefer low-risk, low-return production methods. On top of that, information asymmetry in product markets further locks agricultural growth potential. The asymmetry of agricultural market information leads to abnormal fluctuations in agricultural product prices and sales, which may mislead farmers in planting and production decisions, increase the probability of loss, and affect agricultural output value, reducing agricultural total factor productivity. Therefore, digital construction, mobile Internet, and information sharing can reduce the information asymmetry between agricultural producers and external markets (Lin et al., 2013; Deichmann et al., 2016), thereby creating a low-risk, high-return external market environment and securing stable or predictable market prices and sales. In addition, Otsuka et al. (2016) pointed out that improper agricultural policies have led to distortions in the Japanese market, the loss of agricultural comparative advantages, and reduced large-scale production efficiency in farms. Big data, the Internet, cloud platforms, and other technologies can improve government transparency and public participation. This ensures a successful implementation of policies, timely feedback, and an effective evaluation of the effects of agricultural policies, thereby improving the implementation effects of agricultural policies. In addition, modern technologies such as big data, blockchain, and mobile communications can improve regional digitalization levels and effectively optimize the agricultural production value chain system and the circulation of resource elements, thereby reducing the distortion of market element allocation and promoting agricultural innovation by drastically reducing transaction costs (Deichmann et al., 2016). Based on the above analysis, the following hypothesis is proposed:
Hypothesis 1 (H1). The regional digitalization level is positively correlated with agricultural total factor productivity.
Generally speaking, the situation of regional agricultural production is in line with the level of economic development. Some studies have compared changes in agricultural production efficiency in countries with different levels of development (Alston and Pardey, 2014; Fuglie, 2018). The conclusion of these studies is that the growth of agricultural total factor productivity in economically developed areas is slowing down, while agricultural total factor productivity in developing countries increases relatively fast. These studies show that differences in the level of economic development have an important impact on the agricultural total factor productivity. Santangelo (2018) analyzed foreign land investment in developed and developing countries and found that governments and investors in developed countries pay more attention to the sustainable development of agriculture and the use of advanced technologies to increase the food production of the investees. However, the governments of developing countries usually require investors' goals to be consistent with national interests and government policies. Therefore, investors in developing countries make less responsible investments, which leads to an excessive use of water, pesticides, and fertilizers. This shows that differences in the level of economic development directly affect the behavior of governments and agricultural investors; for example, they have completely different attitudes toward the use of digital technology. The long-term imbalance of economic development in China may mean that underdeveloped regions pay more attention to the input of pesticides, fertilizers, and agricultural machinery, while economically developed regions pay more attention to green and sustainable digital technologies. In addition, the degree of market information asymmetry in regions with different levels of economic development also differs. Information asymmetry in the agricultural market leads to fluctuations in agricultural product prices and trading volumes, which may mislead farmers in their production and planting decisions, leading to losses and reducing agriculture production value and agricultural total factor productivity. As the effect of a rising regional digitalization level on the information asymmetry in the agricultural market differs in regions with different levels of economic development, the impact of rising regional digitalization level on agricultural total factor productivity is also different.
On the other hand, the efficiency of agricultural production in economically developed regions is relatively high, and efficiency growth may have reached a state of convergence. As a result, regional digitalization has a weaker impact on agricultural total factor productivity, or a higher digitalization level is needed to significantly improve agricultural total factor productivity (which means a higher threshold effect value). This means that the effect of digital development on the improvement of agricultural total factor productivity is insignificant temporarily (Zambon et al., 2019). On the other hand, the agricultural production efficiency in underdeveloped regions is relatively low and promises more potential. Therefore, a rising regional digitalization level can optimize the entire agricultural value chain, such as production, market information, and factor circulation, thereby increasing agricultural total factor productivity. Based on this, the following hypothesis is proposed:
Hypothesis 2 (H2). Digitalization levels exert significantly different impacts on agricultural total factor productivity in regions with different levels of economic development.
Factor allocation efficiency is an important factor affecting agricultural total factor productivity. Distortions in the allocation of market factors caused by an underdeveloped market and lagging government supervision or factor market reforms exist in China (Dai and Cheng, 2016). Research on the consequences of market or economic distortions has shown that market distortions lead to a waste of resources (Yin et al., 2018), which means too much input, less output, and a less efficient use of resources brought about by digital technology. Zhu et al. (2011) proved that the distortion of factor allocation significantly reduces the agricultural total factor productivity of China. Reducing distortions in the allocation of market elements can not only alleviates resource waste but also reduces resource redistribution (Dai and Cheng, 2016). The redistribution of labor resources usually means greater costs (Dower and Markevich, 2018). Therefore, reducing the redistribution of resources means reducing the cost of resource allocation. The reduction in resource costs can strengthen the role of digitalization in increasing agricultural total factor productivity. At the same time, the distortion of the labor market limits the ability of labor forces to learn new skills, while digital technology particularly requires the ability to learn and adapt (Kong et al., 2021). Therefore, alleviating the distortion of labor factor allocation will effectively improve the “digital divide” of labor force. In addition, the information asymmetry in the supply chain distorts the capacity allocation among retailers, leading to inefficient capacity shortages and overpricing (Chen et al., 2014). As such, alleviating the distortion of agricultural market factor allocation can also optimize the agricultural production value chain by reducing information asymmetry, thereby enhancing the role of regional digitalization in increasing agricultural total factor productivity.
The scale of farmland also has a significant impact on agricultural productivity. Previous studies have found that there is often an inverse relationship between the scale of farmland and land output or productivity (Feder, 1985; Barrett, 1996; Barrett et al., 2010). The smaller the scale of farmland, the lower the opportunity cost of labor and the cost of labor employment. If agricultural production technology does not bring about increasingly high returns to scale, farmers' enthusiasm for work will reduce, leading to an inverse relationship between farm size and productivity (Barrett, 1996). Digital technologies such as big data, cloud computing, and artificial intelligence will “empower” the supply of raw materials, production, and sales of traditional agriculture. For example, digital production technologies such as intelligent irrigation, intelligent fertilization, and intelligent pest control, as well as digital supply and sales chain technologies such as intelligent logistics, intelligent storage, and intelligent transportation, will greatly increase the return to scale of farmland. On the other hand, large-scale operation can reduce the unit cost of digital technology input, which facilities the acquisition and analysis of big data in agricultural production and the input and application of artificial intelligence technology. Digital technologies such as digital soil maps and intelligent robots can be mainly used for large farms that require substantial investment (Deichmann et al., 2016). Based on this, the following hypotheses is proposed:
Hypothesis 3a (H3a). The lower the degree of distortion in market factor allocation, the greater the promotion effect of digitalization on agricultural total factor productivity.
Hypothesis 3b (H3b). Large-scale production can strengthen the role of digitalization in increasing agricultural total factor productivity.
Research Model
Agricultural Total Factor Productivity Measurement
In this paper, stochastic frontier analysis (SFA) is used to measure the agricultural total factor productivity in various regions of China. Two main methods are used for measuring China's agricultural total factor productivity in the existing literature: parametric and non-parametric methods. The commonly used parametric method is data envelopment analysis (DEA) (Erdem Demirtaş and Fidan Keçeci, 2020), while the widely applied non-parametric method is stochastic frontier analysis (SFA) (Shabanzadeh-Khoshrody et al., 2016). Compared with data envelopment analysis, the advantage of stochastic frontier analysis is that it can fully consider the impact of random factors on the output through parameter estimation, which is more in line with the essential characteristics of agricultural production, and is less sensitive to outliers. Thus, it can reduce the difference between estimated results and actual efficiency levels. Therefore, based on the research of Aigner et al. (1977), Battese and Coelli (1992), the specific form of the model is set as follows:
In Model (1), Yit represents the output of the i-th decision-making unit in period t; Xit represents the input of the i-th decision-making unit in period t; t represents the time trend, reflecting the time variation of technology; f(Xit, t; β) represents a specific functional form, β is the input vector parameter to be estimated; vit represents the influence of climate, natural disasters, measurement errors, and other random factors on the agricultural production frontier, and it is assumed to follow a normal distribution; uit is a non-negative random variable, representing the agricultural production efficiency loss of the sample unit, and it is assumed to follow a truncated normal distribution; the agricultural production technical efficiency of the i-th decision-making unit in the t period is TEit = exp(–uit); vit are uit independent of each other. See Appendix A for more explanation of the SFA model.
In the choice of the form of specific function f(Xit, t; β), a translog production function, which is more flexible than the C-D production function (Coelli and Perelman, 2000), was used. It is widely used in the calculation of agricultural production efficiency. The specific form is as follows:
In Model (2), Yit represents the total agricultural output value of province i in period t, Xit is the factor input, and j and l represent the jth and lth factor inputs, respectively. We mainly selected land (S), labor (L), capital (K), and intermediate product input (M) as input indicators.
The Influence of Digitalization Level on Agricultural Total Factor Productivity
The core issue studied in this paper is the impact of digitalization levels in various regions of China on the agricultural total factor productivity. The fixed-effect regression model of the provincial samples is constructed as follows:
Model (3) is the main regression model of this paper, and the explained variable TFPit represents the agricultural total factor productivity of the province i in the period t, which is calculated by Model (2); the explanatory variable DIGit is the digitalization level of province i in period t; Controlit is the control variables of the model, including the installed capacity of hydropower stations, labor quality, effective irrigation area, the share of financial support for agriculture in fiscal expenditure, and per capita GDP; γt is the time effect; εit is the individual fixed effect and the random error term of the model; the coefficient α1 is the core parameter that this paper focuses on and is expected to be positive. This is to say, digitalization can promote agricultural total factor productivity.
Data Sources, Variable Selection, and Descriptive Analysis
Data Sources
In 2012, the Chinese government proposed to adhere to the road to informatization and agricultural modernization, promote the deep integration of informatization and industrialization, and realize smart governance by using modern science and technology. Since then, various regions in China have been advancing digitalization. The data used in this paper are from 2013 to 2020, and the sample objects are 31 provinces or municipalities in China. The research data come from the China Statistical Yearbook and China Rural Statistical Yearbook. Some data are missing. Since China's agricultural development has been in a state of steady growth (Cao and Birchenall, 2013), we used the average growth rate method to supplement the missing data.
Variable Selection and Descriptive Statistics
(1) Explained variable (TFP). First, with regard to the calculation of agricultural total factor productivity, we selected the total output value of agriculture, forestry, animal husbandry, and fishery as the output index Y. In terms of factor input, the sown area of crops at the end of the year (S) is used in this paper as the index of land input, and the number of employees in the primary industry (L) is used as the index of labor input. In view of the availability of data and reference to the previous literature (Quan, 2009), the total power of agricultural machinery (K) and the consumption of chemical fertilizers (M) are used as alternative input indicators for agricultural capital and intermediate input, respectively. At the same time, in order to avoid the multicollinearity between the high-order terms (quadratic terms and interaction terms) and the low-order terms (first-order terms) in the translog production function as much as possible, as well as the impact of differences in their dimensions, the input and output indexes are standardized in this paper.
(2) Core explanatory variable (DIG). The core explanatory variable in this paper is the digital technology level of 31 provinces or municipalities in Mainland China (excluding Taiwan, Hong Kong, and Macau). The quantitative evaluation of this indicator is a frontier issue that has not yet been involved in the academic community. This paper draws on the practice of Han et al. (2017) to use keywords to count the cumulative number of corresponding industrial policy documents in various provinces and cities as an indicator of industrial policy intensity, and at the same time refers to the idea of Li et al. (2020) to use crawler technology to construct the financial technology development level index based on the number of Baidu news search results. Web crawler technology is used to count the digital-related news volume in prefecture-level cities and above in China to arrive at the digitalization level index of each province and city. Most of the digital construction activities are monitored in various regions of China. The more digital construction activities there are, the greater the amount of relevant news reports. Therefore, the number of relevant news reports represents the digitalization level in each region to a certain extent. News reports on Baidu, the world's largest and most comprehensive Chinese search engine, can be accurately searched by keywords. Therefore, we used Baidu to search for relevant news. Specifically, this paper refers to the “White Paper on China's Digital Economy Development (2020)”, “2020 Digital Trends Report,” and other research reports, as well as the “14th Five-Year Plan” report, the report of the 19th CPC National Congress, relevant policy documents and news reports of various local governments, and classic literature on Chinese digitization research (Wu et al., 2021) and extracts keywords related to digitization through Python's Jieba word segmentation function. We matched these keywords with prefecture-level cities or municipalities in China, searched for prefecture-level cities or municipalities + keywords, such as “Guangzhou + Big Data” in the Baidu news search, and used web crawler technology to count the search results. The total search volume was obtained by summing up the search volume of all keywords in a prefecture-level city or municipality. Because this kind of data has a typical “right-biased” characteristic, we conducted logarithmic processing to obtain the digitalization level (DIG) index. Table 1 shows the characteristic words related to the digitalization level.
As can be seen in Table 1, a series of high-frequency vocabulary is filtered out through the Python-based Jieba Chinese word segmentation function. These digital technology keywords can be roughly divided into big data technology, Internet, Internet of Things technology, smart technology, cloud, cloud computing technology, information technology, and blockchain technology, which is in line with the current understanding of digitization on mainstream literature (Klerkx et al., 2019; Lioutas et al., 2021).
(3) Control variables. According to the existing literature and the actual situation (Quan, 2009; Fuglie, 2018; Zhang et al., 2019), the control variables of this paper consider the two aspects of agricultural production conditions and the socio-economic environment. Agricultural production conditions directly affect the growth and changes of agricultural total factor productivity. Agricultural production conditions generally adapt to the socio-economic environment and can affect agricultural total factor productivity. Specifically, the installed capacity of hydropower stations (Elect), the labor quality (Labqua), and the effective irrigation area (Irrigation) are used to control agricultural production conditions. The social and economic environment is controlled by the share of financial support for agriculture in fiscal expenditure (Finsup) and the level of regional economic development (GDP). At the same time, in order to eliminate the problem of heteroscedasticity, the data are processed logarithmically. The definitions of the input and output variables, dependent variables, independent variables, and control variables of the benchmark model are shown in Table 2.
Descriptive Statistical Analysis
Descriptive statistical analysis of the variables included in the agricultural total factor productivity measurement model and the benchmark regression model is performed, and the results are shown in Table 3.
Among the descriptive statistical results in Table 3, the input and output indexes are statistically described in the form of raw data, but they are logarithmically processed and standardized in the subsequent model calculation process.
The descriptive statistical results show that there is a large difference between the minimum and maximum values and the mean values of various input and output indexes. For example, the minimum value of the total output value of agriculture, forestry, animal husbandry, and fishery (Toutput) among output indexes is about 12.8 billion yuan, the maximum value is about 1.0191 trillion yuan, and the mean value is about 356.5 billion yuan. The minimum value (S) of land input among input indexes is 88.6 thousand hectares, the maximum value is 14910.1 thousand hectares, and the mean value is 5535.025 thousand hectares. This means that there are substantial differences between agricultural output and agricultural input in various regions in China. These differences are mainly due to unbalanced regional economic development and the heterogeneity of the population and industrial structure. For example, compared with other provinces, fewer laborers engage in the primary industry in Shanghai, as there is less arable land, the level of economic development is high, and the tertiary industry is the main industry. Meanwhile, more laborers engage in the primary industry in Guangxi Province, as there is more arable land, and the level of economic development is low. In addition, the maximum values of the independent variable agricultural total factor productivity (TFP) and the core explanatory variable digital technology level (DIG) are 0.978 and 10.448, and the minimum values are 0.274 and 3.714, respectively, indicating that there are substantial differences between the agricultural total factor productivity and digitalization levels in various regions in China. Such differences include differences in regional natural conditions, agricultural production infrastructure, labor quality, and financial input, exerting an impact on agricultural total factor productivity.
Econometric Test of Digitalization Level on Agricultural Total Factor Productivity
The Calculation of Agricultural Total Factor Productivity
Table 4 shows the maximum likelihood estimation results of the stochastic frontier production function model (2). It can be seen that the parameter estimation results of the four main input items of land (S), labor (L), mechanical power (K), and fertilizer (M) are all significant at the 1% level, and most of the remaining parameters have also passed the T test. Among them, the coefficient of γ is 0.986 and passes the T test at a significance level of 1%. The γ value is very close to 1, which shows that technical inefficiency is the main component of the joint random disturbance term, accounting for 98.6%, while the influence of the random error term on the inefficiency is only 1.4%, indicating that it is appropriate to use the stochastic frontier production function model in this paper.
Table 4 shows that the input parameters of land, mechanical power, and fertilizer are significantly positive, indicating that, for every 1% increase in the sown area of crops, the agricultural output will increase by 0.5291%. For every 1% increase in agricultural machinery input, agricultural output will increase by 0.1382%. For every 1% increase in fertilizer input, agricultural output will increase by 0.5067%. This result is consistent with the fact that large-scale, mechanized, and scientific agricultural production in China brings high yields and high returns. The labor input coefficient is significantly negative, indicating that the increase in labor input actually reduces agricultural output. The result is the same as the study by Zhang et al. (2019). The negative correlation between labor input and agricultural output is mainly due to the fact that labor income is much higher than agricultural income, which leads to the transfer of rural labor. At the same time, agricultural machinery has a substitution effect on labor (Otsuka et al., 2016). At the moment, China's agriculture is currently transforming into large-scale and mechanized production. According to the data released by the Ministry of Agriculture and Rural Affairs in the 2020 press conference, the comprehensive mechanization level of the cultivation and harvest of China's main crops has exceeded 70% since the 13th Five-Year Plan. In the limited accommodating space, agricultural machinery replaces a substantial amount of manpower. As such, too much manpower input will cause labor redundancy, which will negatively affect large-scale and mechanized agricultural production. In addition, with the increase in labor costs, excessive labor input will reduce the profit rate of agricultural production. Therefore, labor input and agricultural output are negatively correlated, but this does not negate the benefits brought by high-quality agricultural labor input.
Benchmark Regression Results
In order to verify Hypothesis 1 (H1), Benchmark Model (3) is first used to study the overall impact of digitalization levels on agricultural total factor productivity. Column (1) of Table 5 is the case where no control variables are added to study the basic relationship between the digitalization levels and the total factor productivity of agriculture. Column (2) is the regression result after adding various core control variables to control the interference of other important factors on the research conclusion.
At the same time, in order to verify Hypothesis 2 (H2), that is, to explore the heterogeneous results produced by differences in regional economic development levels, per capita GDP is used to measure regional economic development levels and constructs a dummy variable for economic development. If the per capita GDP of a region is greater than the annual mean value, then it is an economically developed area, that is, Developed = 1; otherwise, it is an economically underdeveloped area, that is, Developed = 0.
Table 5 shows that, regardless of whether the control variables are considered, regional digitalization can significantly increase agricultural total factor productivity (both are positively correlated at the 1% significance level). Thus, Hypothesis 1 is verified. Specifically, improving the digitalization level can greatly boost the upgrading of agricultural technology, and the use of modern production technology can effectively improve agricultural total factor productivity; on the other hand, regional digital development can further optimize the external conditions of agricultural production including information elements and capital elements, alleviate the adverse effects of asymmetric market supply and demand caused by factors such as distorted resource allocation and information communication failure, thereby improving agricultural total factor productivity. After various control variables are added, the coefficient of the core explanatory variable DIG drops slightly, which shows that the control variables considered in Benchmark Model (3) in this paper are reasonable. Specifically, the Finsup parameter is estimated to be significantly positive at the level of 1%, indicating that financial support for agriculture has achieved good results. For developing countries, backward agricultural production equipment is an important factor restricting agricultural production efficiency. Providing farmers with funds to purchase mechanical and digital equipment helps popularize mechanized and digital production equipment, while financial subsidies can stimulate farmers' enthusiasm for production and alleviate the decline in working-age population in rural areas. To sum up, financial support for agriculture can significantly improve agricultural total factor productivity. In addition, labor quality (Labqua) and agricultural total factor productivity are positively correlated at a significance level of 10%, which indicates that the improvement of labor quality can significantly improve agricultural total factor productivity. The reason may be that agricultural labor forces cannot use agricultural machinery and equipment, digital equipment and other agricultural input factors, or acquire and understand market information without certain knowledge. Labor forces with higher quality can better adapt to digital technology, better use the “digital dividend” and timely access to market information with the help of digital platforms. As the improvement of labor quality can effectively alleviate the “digital divide” problem, labor quality is positively correlated with agricultural total factor productivity. This result is also consistent with the reality.
However, the installed capacity of hydropower stations (Elect) and the effective irrigation area (Irrigation) are significantly negatively correlated with agricultural total factor productivity. This is mainly due to the fact that hydropower is not the main source of electricity in China and is closely related to geographical conditions, and China's power supply situation also varies greatly with regional characteristics. The effective irrigation area is related to the area of arable land. The arable land area in China is unevenly distributed, and the arable land area and the effective irrigation area are significantly smaller in economically developed areas. For example, in economically developed areas such as Beijing and Shanghai, there is less hydropower, electric power is mainly used for the tertiary industry and residents' lives, and the effective irrigation area is relatively small. However, a high labor quality, high mechanical power input, and a high digitalization level can also ensure high agricultural total factor productivity. At the same time, the effective irrigation area is related to the scale of farmland, and previous studies have found that there is an inverse relationship between the scale of farmland and land productivity (Feder, 1985; Barrett, 1996; Barrett et al., 2010). The results of this paper are similar to those of previous studies. On top of that, the level of regional economic development (GDP) and agricultural total factor productivity are also negatively correlated at a significance level of 1%, which reflects the inherent characteristics of economically developed regions; that is, economically developed regions are mainly driven by the tertiary industry, and the more developed the economy, the smaller the proportion of agricultural production. Compared with the primary industry, the tertiary industry can bring higher added value and economic transmission effects. Therefore, the main goal of agricultural production in these regions is to maintain the food supply security line and meet the basic needs of the people in the region. This is in line with China's reality.
Columns (3) and (4) of Table 5 are the heterogeneous results caused by differences in economic development levels. It can be seen that, under the sub samples of economically developed regions, the estimated coefficient of the digitalization level is negative but not significant, which shows that digitalization has no significant effect on the agricultural total factor productivity of economically developed regions. In the sub samples of economically underdeveloped regions, the regression coefficient of digitalization level is positive at the 1% significance level, indicating that digitalization can significantly improve the agricultural total factor productivity in economically underdeveloped regions. The reason may be that a high labor quality, infrastructure construction, and agricultural subsidies in economically developed regions lead to high agricultural production efficiency benchmarks. Moreover, efficiency growth in economically developed regions may have reached a state of convergence. As a result, a higher digitalization level is needed to significantly improve agricultural total factor productivity (which means a higher threshold effect value). In other words, digital development cannot significantly promote agricultural total factor productivity in a short period of time, which makes it impossible for us to clarify the promotion effect of digital development on agricultural total factor productivity at the moment. In addition, the fact that the focus of economically developed areas is not on the primary industry, the decline in agricultural labor forces and the loss of farmland lead to a skewed allocation of resources, so currently the radiation effect of the digitalization level may act more on secondary or tertiary industries, and the agricultural sector can hardly be promoted by the digitalization. In economically underdeveloped regions, many laborers engage in the primary industry, the labor quality is poor, the infrastructure is underdeveloped (Gorelick and Walmsley, 2020), agricultural subsidies are lacking, and agricultural production is still growing. Therefore, a rising regional digitalization level in economically underdeveloped regions can optimize the entire agricultural value chain, such as production, market information, and factor circulation, making up for the deficiency of insufficient investment in related facilities, and significantly improving the agricultural total factor productivity.
Endogenous Treatment and Robustness Test
Endogenous Treatment
In this paper, there is no reverse causality between the digitalization level at the provincial level and the agricultural total factor productivity, but there may still be errors in the results due to missing variables and measurement errors, resulting in endogenous problems. Compared with the two-stage least squares method (2SLS), the generalized method of moments (GMM) can better deal with the autocorrelation and heteroscedasticity of the data (Lin and Du, 2018). Thus, the instrumental variable-generalized method of moments (IV-GMM) is adopted to test Benchmark Model (3) and eliminate the possible impact of endogeneity.
Specifically, considering that there may be a certain lag in the effect of digitalization at the regional level on agricultural production, this paper refers to the practice of Chong et al. (2013) of choosing a digitalization level lagging by one stage and the mean value index of digitalization level (DIG_IV) of provinces with similar economic development levels in the same year as the instrumental variable and to test Model (3) with IV-GMM. As far as the relevance of the instrumental variable is concerned, scientific research, infrastructure construction, and practical applications of regional digital technology require financial support, which means that the regional digitalization level is largely affected by the level of economic development. That is to say, regions with similar economic development levels have similar levels of technological development (Li et al., 2020), and the corresponding inputs differ little, so the digital technology levels are also similar. This instrumental variable is relevant. In addition, due to the geographical difference, it is difficult for the digitalization level of each region to affect the agricultural production of other provinces. This is consistent with the exogenous nature of the instrumental variables. Table 6 reports the IV-GMM regression results.
Column (1) of Table 6 is the regression results of the first stage. It can be seen that the estimated coefficients of the selected instrumental variables are positively correlated with the digitalization level DIG at the significance levels of 1 and 5%, which proves that the instrumental variables are in line with the correlation condition. In addition, the F statistic of the first stage estimation result is >10, indicating that there is no weak instrumental variable problem. The results of the second stage show that, after dealing with the endogenous problem, the digitalization level and agricultural total factor productivity are positively correlated at a significance level of 1%, and the estimated coefficient has risen from 0.0098 to 0.03. This indicates that some endogeneity factors previously reduced the coefficient of explanatory variables, and the estimated parameters of explanatory variables in this paper are revised after overcoming certain endogeneity factors. At the same time, underidentification and over-identification tests are carried out. The test results show that there is no underidentification or over-identification of instrumental variables, so the selection of instrumental variables is reasonable.
Robustness Test
(1) The PSM-DID method. The core conclusion of this paper is that digitalization has significantly promoted agricultural total factor productivity. In 2016, the National Development and Reform Commission approved the establishment of national big data comprehensive pilot zones in eight regions of 10 provinces or municipalities directly under the Central Government. To further verify the validity of this conclusion, considering that the regional digital development policy is an excellent quasi-natural experiment, the PSM-DID method is used to investigate the impact of regional digital construction on agricultural total factor productivity. The PSM-DID method can effectively correct the selective bias problem through propensity score matching (PSM), alleviate the endogeneity problem through difference in differences (DID), and reduce the omitted variable bias to a certain extent (Wu et al., 2021). This means the conclusions of this paper are robust.
The establishment of national big data comprehensive pilot zones is a reform policy proposed by the State Council to press ahead with regional digital construction and better integrate digital technology with economic production. The impact of changes in regional digital technology levels on agricultural total factor productivity before and after the implementation of the policy can prove the core conjectures of this paper to a certain extent.
The economic level and agricultural production were specifically selected for the following covariates: the per capita GDP, the share of financial support for agriculture in fiscal expenditure, the effective irrigation rate, the total power of agricultural machinery (taking the natural logarithm), and the number of employees in the primary industry (taking the natural logarithm). The entropy matching method does not need to set the specific form of the model and can perform high-order matching without losing samples. In view of the sample size in this study, it is an efficient matching method (Yang and Hou, 2020) and fits this situation well. Thus, the entropy matching method is used for matching. The entropy matching results are shown in Table 7.
Table 7 shows that the p value of each covariate before matching is <0.01, which indicates that there is a significant difference between the experimental group and the control group. After entropy matching, the absolute value of the standard deviation of each covariate is 0%, there is no significant difference, and the deviation is reduced by 100%. This shows that the variables selected in this study are appropriate. It also shows that PSM regression has made the experimental group and the control group roughly similar, and the model is robust. In addition, the dummy variable yeardummy was set before the implementation of the policy and was cross-multiplied with the experimental group or the control group (Treat × yeardummy) after it was incorporated into Model (4) for the equilibrium tendency test. The test results are shown in Table A1 of Appendix A. The test results show that the coefficient of the cross-product term (Treat × yeardummy) are not significant, indicating that the experimental group and the control group meet the equilibrium tendency before the implementation of the policy.
The regions approved for the construction of national big data comprehensive pilot zones in 2016 are Guizhou, Beijing, Tianjin, Hebei, Guangdong, Shanghai, Henan, Chongqing, Liaoning, and Inner Mongolia. These regions are included in the experimental group, and the remaining regions are in the control group. The difference-in-differences model is adopted on the experimental group and the control group before and after the implementation of the digital development policy to effectively eliminate the internal differences between individuals and the bias caused by the time trend unrelated to the experimental group and obtain the “net effect” of the digital development policy on agricultural total factor productivity. The measurement model constructed is
In model (4), when a sample is in the experimental group, Treat is 1, and when a sample is in the control group, Treat is 0. When the year is ≥2016, Post is 1; otherwise, it is 0. The coefficient of cross-product term α1 of Treat and Post reflects the change in agricultural total factor productivity before and after the implementation of the digital development policy in a region and is a key parameter to be evaluated in the model. Controlit represents other control variables, and the specific meaning remains unchanged. θi is an individual fixed effect of the model. τt is the time fixed effect, and εit is the random error term of the model. The regression results are shown in Column (1) of Table 8.
(2) The time lag effect. Taking into account that digital technology may have a certain time lag effect on agricultural production, the core explanatory variables and explained variables are further treated with a one-period lag and a one-period lead, respectively. The test results are shown in Columns (2) and (3) of Table 8.
(3) GMM dynamic panel analysis. The agricultural total factor productivity has a certain degree of sustainability, that is, serial correlation. To solve this problem, dynamic panel data are further constructed, the lag term of the explained variable is used to control the influence of initial conditions on the agricultural total factor productivity, and the first-order difference generalized method of moments (FD-GMM) is adopted to perform regression. The regression results are shown in Column (4) of Table 8.
Column (1) of Table 8 is the regression result of PSM-DID. It can be found that the regression coefficient of treat × post is significantly positive at the level of 5%, which indicates that, after the implementation of the digital development strategy, the regional digitalization level was promoted, and the agricultural total factor productivity significantly increased. Columns (2) and (3) are the test results after considering the time lag effect. It can be seen that the digitalization level and agricultural total factor productivity are still positively correlated at a significance level of 1%. This shows that the promotion effect of the digitalization on agricultural total factor productivity has not decayed over time, but this reflects that the development of digital technology in various regions can exert a duplicate effect on agricultural total factor productivity in a longer time series. This further proves the correctness of the core viewpoints of this paper.
Column (4) is the regression result of the GMM dynamic panel. The p value of AR(1) test is close to 0, and the p value of AR(2) test is 0.648, which means that the model has a first-order serial correlation but no second-order serial correlation. This satisfies the moment condition of GMM estimation. At the same time, p values in Sangan's test and the Hansen J test are >0.1, which shows that the model is effective. The regression coefficient of the digitalization level (DIG) is still significantly positive at the 1% level, indicating that, after considering the characteristics of agricultural total factor productivity serial correlation (controlling the explanatory variable lagging one period and the resulting endogenity), regional digitalization can significantly promote agricultural total factor productivity.
The Influence Mechanism of the Digitalization Level on Agricultural Total Factor Productivity
In order to verify Hypothesis 3a (H3a), that is the adjustment mechanism of factor allocation distortion, this paper refers to the research of Hsieh and Klenow (2009), Zhu et al. (2011), and Gai et al. (2015). In this paper, factor distortion is defined as the comparison between factor return and actual return. If the factor distortion index is <1, the factor return is less than the actual price, and the factor price is positively distorted. Otherwise, it is negatively distorted. First, under the CD production function equation, that is, Y = ASαLβKγMδ, where Y represents total output, S, L, K, and M represent land, labor, capital, and intermediate product inputs, respectively, and α, β, γ, and δ represent the output elasticity of each input, respectively, A is the total factor productivity, which also indicates technological progress. Since the input of the intermediate products can be adjusted freely and does not face flow limitations (Wang and Li, 2021), it is assumed that there is no distortion in the input of intermediate products. At the same time, based on the actual situation of agricultural production, it is generally believed that land use is free (Zhu et al., 2011). Therefore, this paper only considers the distortions of the capital and labor markets. The marginal outputs of capital and labor are expressed as follows:
Assuming that the capital price is the benchmark loan interest rate R and the labor price is the average wage W, the degree of distortion in the capital and labor markets and the overall degree of distortion in the market are, respectively,
This paper establishes a panel data model to estimate the element elasticity of each region:
Here, Y is the total output value of the primary industry, S, L, K, and M are again, respectively, land, labor, capital, and intermediate product inputs, θt represents the fixed effect of controlling time, ρi represents the individual effect of controlling province, and εit is the random error term of the model. After estimating the elasticity of factors in each region from Model (10), the overall distortion of the market can be estimated by model (7)–(9). After the overall market distortion degree (DisT) is obtained, the cross-product term (DIG × DisT) between it and the explanatory variable DIG is added to Benchmark Model (3). The coefficient of the cross-product term is the adjustment effect of the distortion of market factor allocation. The test results are shown in Table 9.
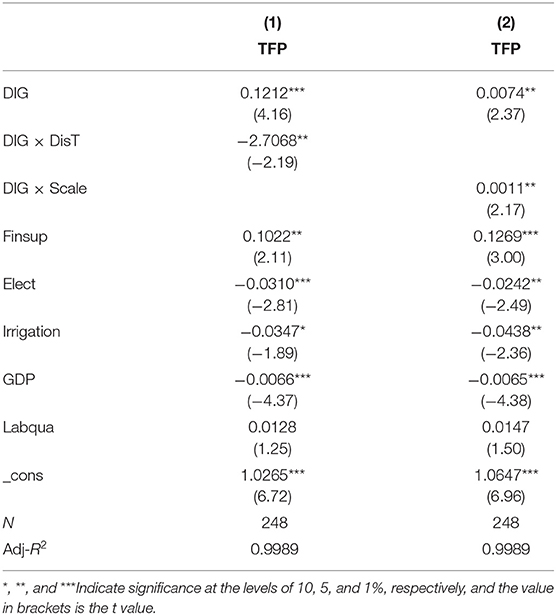
Table 9. The influence mechanism of the digitalization level on agricultural total factor productivity.
In addition, in order to verify Hypothesis 3b (H3b), that is, the adjustment mechanism of scale, this paper defines large-scale production (Scale) as the ratio of the sown area of crops to the number of laborers, that is, the average sown area of labor (taking the logarithm to ease measurement errors and heteroscedasticity issues), and adds its cross-product term with the digital technology level (DIG) to Benchmark Model (3). The coefficient of the cross-product term DIG × Scale is the adjustment effect of large-scale production. The test results are shown in Table 9.
Column (1) of Table 9 is the regression result of the adjustment effect of the distortion of market factor allocation. The coefficient of the interaction term between the digitalization level and the distortion of market factor allocation (DIG × DisT) is significantly negative at the 5% level, indicating that, when the market factor allocation is distorted, or the market factor allocation is not efficient, the promotion effect of digitalization on agricultural total factor productivity will be significantly weakened. On the other hand, when the efficiency of market factor allocation is improved, that is, after the distortion of market factor allocation is improved, the promotion effect of digitalization on agricultural total factor productivity will be significantly strengthened. Hypothesis 2 is thus verified. The mechanism of action may be as follows: Resources tend to be input in urban enterprises rather than the agricultural sector due to market distortions (Au and Henderson, 2006), which aggravates the negative consequences brought by the decline in agricultural labor forces and the loss of farmland, seriously hindering the improvement of agricultural production efficiency. Alleviating market distortions will effectively improve resource input bias and guide resources to enter the agricultural sector, thereby enhancing the role of digitalization in promoting agricultural total factor productivity. Specifically, alleviating price distortions in the agricultural market can help correct the profits of the agricultural sector, stimulate the enthusiasm of producers, and effectively enhance the promotion effect of digital technology on agricultural total factor productivity; alleviating the distortion of capital allocation can reduce the ineffective allocation of capital, increase the capital inflow to the agricultural sector, provide financial support for agricultural digital equipment and offer labor forces with the opportunity to learn digital technology, thereby enhancing the promotion effect of digital technology on agricultural total factor productivity. At the same time, after the distortion of the allocation of labor factors in the market is alleviated, wages of the agricultural sector will be raised to introduce high-quality talents (Li and Ma, 2021), thereby improving the adaptability of agricultural labors to digital technology, easing the “digital divide” problem in the agricultural sector, and further enhancing the role of regional digitalization in promoting agricultural total factor productivity. In addition, the reduction in the degree of market distortion indicates an improvement in resource allocation efficiency, whether it is a capital element or a labor element. Improving the allocation efficiency can further enhance the role of digital technology in promoting agricultural total factor productivity and optimizing the agricultural production value chain.
Column (2) of Table 9 is the regression result of the adjustment effect of large-scale production. The coefficient of the cross-product term DIG × Scale is significantly positive at the 5% level, indicating that large-scale production has significantly enhanced the role of digitalization in promoting agricultural total factor productivity. That is, the larger the scale of farmland production, the greater the promotion effect of digitalization on agricultural total factor productivity. Hypothesis 3 is thus verified. The reasons for this may be as follows: First, in terms of technology input cost, large-scale production is conducive to reducing the unit input cost of digital technology. Cost reduction contributes to the application of digital production technology in the agricultural sector both in depth and in breadth, and wider application and more advanced digital technology means a greater improvement in agricultural total factor productivity. Second, at the level of technological adaptation, the application of agricultural digital technology is more suitable for large-scale production of land. Agriculture land fragmentation limits the application of digital technology, and satellite imagery, smart water conservancy and other technologies are more suitable for large-scale production, so large-scale farmland and digital technology have better synergistic effects. Third, large-scale production is conducive to the acquisition of digital information for agricultural production, and these digital information will become agricultural digital information assets, providing a reference for subsequent agricultural production-related decisions. Based on the above reasons, large-scale agricultural production can positively enhance the promotion effect of regional digitalization on agricultural total factor productivity. Previous studies have found that there may be an inverse relation between farm scale and production efficiency, and the results of this study may also indicate that digital technology is an important support condition for large-scale production, that is, the expansion of farmland production on the basis of improving regional digitalization level can significantly promote the growth of agricultural total factor productivity. Digital technology and agricultural machinery have the same labor substitution effect, ensuring better results for large-scale production. This conclusion provides empirical evidence for the policy guidance of large-scale production.
Conclusions and Recommendations
As populations grow, slowdowns in agricultural productivity growth and the extensive development of agriculture lead to unsustainable development in some parts of the world. Under the circumstance, food security is challenged, and agricultural transformation is imminent. This paper uses data from 30 provinces or municipalities in Mainland China from 2013 to 2020, identifies keywords related to digitization, adopts the number of Internet searches using “prefecture-level cities or municipalities + keywords related to digital technology” to measure regional digitalization levels, discusses the impact of regional digitalization levels on agricultural total factor productivity and the heterogeneity of economic development level, and further analyzes the adjustment mechanism of market factor distortion and large-scale production. The following conclusions can be drawn:
First, regional digitalization can significantly increase agricultural total factor productivity. Digital technology can make agricultural production, the supply of consumables, the transportation and sales of finished products, and other value chains more efficient (Wolfert et al., 2017; Creydt and Fischer, 2019). Regional digital construction can alleviate the shortage of agricultural digital technology input and the “digital divide” of laborers, which are important reasons behind inefficient agricultural production (Benyam et al., 2021).
Second, the impact of regional digitalization levels on agricultural total factor productivity is heterogeneous. Regional digitalization can significantly raise agricultural total factor productivity in economically underdeveloped regions but not in economically developed regions. However, the fact that digitalization does not have a significant effect on agricultural total factor productivity in economically developed regions does not mean that these regions should pay less attention to digital construction. On the contrary, since digital technology is still not widely applied in agricultural production and external markets, these regions should continue to develop digital technology in order to break the threshold for the actual utility of digital technology. This study points out that an important means of improving agricultural total factor productivity in economically underdeveloped regions or developing countries is the promotion of regional digitalization. It is also an important route to green and sustainable agricultural growth.
Third, the distortion of market factors and large-scale production have a significant regulatory effect. The lower the degree of market factor distortion, the greater the effect of digitalization in promoting agricultural total factor productivity; the larger the scale of farmland production, the greater the role of digitalization in promoting agricultural total factor productivity. The inefficient allocation of market elements indicates high information asymmetry between the supply and demand parties. Invalid allocation further causes a waste of resources, hinders market development, and affects agricultural production. Alleviating the distortion of market factor rationing will help digital technology to better promote the connection between supply and demand in the agricultural market and will further strengthen the role of digitalization in promoting agricultural total factor productivity. At the same time, this is an important foundation for creating a low-risk, high-return market. Some studies have found that farm scale and production efficiency are often inversely related (Feder, 1985; Barrett, 1996). However, with the development of agricultural production technology, the adaptation scenarios of these studies have changed greatly. For example, Otsuka et al. (2016) found that, as machinery replaces a large number of labors in Asia, farm scale and production efficiency have shown a positive correlation. This research reflects that the application of agricultural machinery technology has changed the current agricultural production scene. This paper is based on China's digital construction, which may mean that digital construction is also an important support condition for large-scale agricultural production in developing countries. Developing countries can popularize agricultural machinery while improving the level of regional digitalization, and bottlenecks in digital technology investment costs proposed by Benyam et al. (2021) can also be alleviated in large-scale production. This will have a more significant promotion effect on agricultural total factor productivity. In short, our empirical evidence shows that large-scale production and digital technology complement each other.
Based on the above conclusions, the following recommendations are put forward: First, governments should work hard to promote digitalization as a way to increase agricultural total factor productivity. Digital construction in economically developed regions is the subsequent growth driver for agricultural production, while digital construction is an opportunity for economically underdeveloped regions to turn to green and sustainable development. At the same time, it is necessary to work hard to solve the “digital divide” of laborers and the problem of digital technology adaptation, to strengthen the practical application of digital technology, to achieve industry–university–research cooperation, and to popularize digital technology education in order to improve the labor quality and promote the transformation of the workforce. Second, government regulatory agencies should establish an information exchange platform for the supply and demand parties of the agricultural market through digital technology to optimize the external environment of the market and the efficiency of factor allocation and to reduce the waste of resources caused by ineffective allocation. In addition, it is necessary to improve government transparency and citizen participation and to obtain effective feedback on policy effects, thereby solving the problem of market distortion caused by ineffective policies.
Data Availability Statement
The original contributions presented in the study are included in the article/Supplementary Material, further inquiries can be directed to the corresponding authors.
Author Contributions
WF and RZ: conceptualization, writing—original draft preparation, and writing—review and editing. WF: data curation and formal analysis. Both authors have read and agreed to the published version of the manuscript.
Funding
This study was approved by National Social Science Fund from the National Office for Philosophy and Social Sciences, China (Grant No. 20BGL076).
Conflict of Interest
The authors declare that the research was conducted in the absence of any commercial or financial relationships that could be construed as a potential conflict of interest.
Publisher's Note
All claims expressed in this article are solely those of the authors and do not necessarily represent those of their affiliated organizations, or those of the publisher, the editors and the reviewers. Any product that may be evaluated in this article, or claim that may be made by its manufacturer, is not guaranteed or endorsed by the publisher.
Supplementary Material
The Supplementary Material for this article can be found online at: https://www.frontiersin.org/articles/10.3389/fsufs.2022.860780/full#supplementary-material
References
Aigner, D., Lovell, C. A. K., and Schmidt, P. (1977). Formulation and estimation of stochastic frontier production function models. J. Econ. 6, 21–37. doi: 10.1016/0304-4076(77)90052-5
Alston, J. M., and Pardey, P. G. (2014). Agriculture in the global economy. J. Econ. Perspect. 28, 121–146. doi: 10.1257/jep.28.1.121
Au, C. C., and Henderson, J. V. (2006). Are Chinese cities too small? Rev. Econ. Stud. 73, 549–576. doi: 10.1111/j.1467-937X.2006.00387.x
Ball, E. V., Wang, S. L., Nehring, R., and Mosheim, R. (2015). Productivity and economic growth in U.S. Agriculture 38, 30–49. doi: 10.1093/aepp/ppv031
Barrett, C. B. (1996). On price risk and the inverse farm size-productivity relationship. J. Dev. Econ. 51, 193–215. doi: 10.1016/S0304-3878(96)00412-9
Barrett, C. B., Bellemare, M. F., and Hou, J. Y. (2010). Reconsidering conventional explanations of the inverse productivity–size relationship. World Dev. 38, 88–97. doi: 10.1016/j.worlddev.2009.06.002
Battese, G. E., and Coelli, T. J. (1992). Frontier production functions, technical efficiency and panel data: with application to paddy farmers in India. J. Productivity Analysis 3, 153–169. doi: 10.1007/BF00158774
Benyam, A., Soma, T., and Fraser, E. (2021). Digital agricultural technologies for food loss and waste prevention and reduction: global trends, adoption opportunities and barriers. J. Clean. Prod. 323:129099. doi: 10.1016/j.jclepro.2021.129099
Cao, K. H., and Birchenall, J. A. (2013). Agricultural productivity, structural change, and economic growth in post-reform China. J. Dev. Econ. 104, 165–180. doi: 10.1016/j.jdeveco.2013.06.001
Chen, H., Chiang, R. H., and Storey, V. C. (2012). Business intelligence and analytics: from big data to big impact. MIS Q 36, 1165–1188. doi: 10.2307/41703503
Chen, Y. J., Deng, M., and Huang, K. W. (2014). Hierarchical screening for capacity allocation in supply chains: the role of distributors. Prod. Oper. Manag. 23, 405–419. doi: 10.1111/poms.12063
Chong, T. T. L., Lu, L., and Ongena, S. (2013). Does banking competition alleviate or worsen credit constraints faced by small- and medium-sized enterprises? Evidence from China. J. Bank. Financ. 37, 3412–3424. doi: 10.1016/j.jbankfin.2013.05.006
Coelli, T., and Perelman, S. (2000). Technical efficiency of European railways: a distance function approach. Appl. Econ. 32, 1967–1976. doi: 10.1080/00036840050155896
Creydt, M., and Fischer, M. (2019). Blockchain and more - Algorithm driven food traceability. Food Control 105, 45–51. doi: 10.1016/j.foodcont.2019.05.019
Dai, X., and Cheng, L. (2016). Market distortions and aggregate productivity: Evidence from Chinese energy enterprises. Energy Policy 95, 304–313. doi: 10.1016/j.enpol.2016.05.009
Deichmann, U., Goyal, A., and Mishra, D. (2016). Will digital technologies transform agriculture in developing countries? Agric. Econ. 47, 21–33. doi: 10.1111/agec.12300
Dower, P. C., and Markevich, A. (2018). Labor misallocation and mass mobilization: Russian Agriculture during the great war. Rev. Econ. Stat. 100, 245–259. doi: 10.1162/REST_a_00726
Erdem Demirtaş, Y., and Fidan Keçeci, N. (2020). The efficiency of private pension companies using dynamic data envelopment analysis. Quant. Finance Econ. 4, 204–219. doi: 10.3934/QFE.2020009
Feder, G. (1985). The relation between farm size and farm productivity: the role of family labor, supervision and credit constraints. J. Dev. Econ. 18, 297–313. doi: 10.1016/0304-3878(85)90059-8
Fuglie, K. (2017). RandD Capital, RandD Spillovers, and Productivity Growth in World Agriculture. Appl. Econ. Perspect. Policy 40, 421–444. doi: 10.1093/aepp/ppx045
Fuglie, K. O. (2018). Is agricultural productivity slowing? Glob. Food Secur. 17, 73–83. doi: 10.1016/j.gfs.2018.05.001
Gai, Q., Zhu, X., Cheng, M., and Shi, Q. (2015). Factor market's distortion, markup and TFP. Econ. Res. J. 50, 61–75.
George, T. (2014). Why crop yields in developing countries have not kept pace with advances in agronomy. Glob. Food Secur. 3, 49–58. doi: 10.1016/j.gfs.2013.10.002
Gorelick, J., and Walmsley, N. (2020). The greening of municipal infrastructure investments: technical assistance, instruments, and city champions. Green Finance 2, 114–134. doi: 10.3934/GF.2020007
Han, Y., Huang, L., and Wang, X. (2017). Do industrial policies promote industrial structure upgrading? - Theory and evidence from China's development-oriented local government. Econ. Res. J. 52, 33–48.
Hsieh, C. T., and Klenow, P. J. (2009). Misallocation and manufacturing TFP in China and India. Q. J. Econ. 124, 1403–1448. doi: 10.1162/qjec.2009.124.4.1403
Ju, X. T., Xing, G. X., Chen, X. P., Zhang, S. L., Zhang, L. J., Liu, X. J., et al. (2009). Reducing environmental risk by improving N management in intensive Chinese agricultural systems. Proc. Natl. Acad. Sci U.S.A. 106, 3041–3046. doi: 10.1073/pnas.0813417106
Kamble, S. S., Gunasekaran, A., and Sharma, R. (2020). Modeling the blockchain enabled traceability in agriculture supply chain. Int. J. Inf. Manag. 52:101967. doi: 10.1016/j.ijinfomgt.2019.05.023
Klerkx, L., Jakku, E., and Labarthe, P. (2019). A review of social science on digital agriculture, smart farming and agriculture 4.0: New contributions and a future research agenda. NJAS - Wagening. J. Life Sci. 91:100315. doi: 10.1016/j.njas.2019.100315
Kong, Q., Tong, X., Peng, D., Wong, Z., and Chen, H. (2021). How factor market distortions affect OFDI: An explanation based on investment propensity and productivity effects. Int. Rev. Econ. Financ. 73, 459–472. doi: 10.1016/j.iref.2020.12.025
Laurance, W. F., Sayer, J., and Cassman, K. G. (2014). Agricultural expansion and its impacts on tropical nature. Trends Ecol. Evol. 29, 107–116. doi: 10.1016/j.tree.2013.12.001
Li, C., Yan, X., Song, M., and Yang, W. (2020). Fintech and corporate innovation - evidence from Chinese NEEQ-listed companies. China Ind. Econ. 81–98.
Li, T., and Ma, J. (2021). Does digital finance benefit the income of rural residents? A case study on China. Quantitative Finance Econ. 5, 664–688. doi: 10.3934/QFE.2021030
Liang, X. Z., Wu, Y., Chambers, R. G., Schmoldt, D. L., Gao, W., Liu, C., et al. (2017). Determining climate effects on US total agricultural productivity. Proc. Natl. Acad. Sci. USA 114, E2285–E2292. doi: 10.1073/pnas.1615922114
Lin, B., and Du, Z. (2018). A Study of China's Urban Vehicle Energy Consumption and Public Transport Efficiency. Econ. Res. J. 53, 142–156.
Lin, M., Prabhala, N. R., and Viswanathan, S. (2013). Judging Borrowers by the Company They Keep: Friendship Networks and Information Asymmetry in Online Peer-to-Peer Lending. Manag. Sci. 59, 17–35. doi: 10.1287/mnsc.1120.1560
Lioutas, E. D., Charatsari, C., and De Rosa, M. (2021). Digitalization of agriculture: A way to solve the food problem or a trolley dilemma? Technol. Soc. 67, 101744. doi: 10.1016/j.techsoc.2021.101744
Muangprathub, J., Boonnam, N., Kajornkasirat, S., Lekbangpong, N., Wanichsombat, A., and Nillaor, P. (2019). IoT and agriculture data analysis for smart farm. Comput. Electron. Agric. 156, 467–474. doi: 10.1016/j.compag.2018.12.011
Ng, I. C. L., and Wakenshaw, S. Y. L. (2017). The Internet-of-Things: Review and research directions. Int. J. Res. Mark. 34, 3–21. doi: 10.1016/j.ijresmar.2016.11.003
Otsuka, K., Liu, Y., and Yamauchi, F. (2016). Growing advantage of large farms in Asia and its implications for global food security. Glob. Food Secur. 11, 5–10. doi: 10.1016/j.gfs.2016.03.001
Quan, J. (2009). An Empirical Analysis of China's Agricultural Total Factor Productivity Growth: 1978~2007-Based on the Stochastic Frontier Analysis (SFA) Method. China Rural. Econ. 36–47.
Rada, N. E., and Fuglie, K. O. (2019). New perspectives on farm size and productivity. Food Policy 84, 147–152. doi: 10.1016/j.foodpol.2018.03.015
Radoglou-Grammatikis, P., Sarigiannidis, P., Lagkas, T., and Moscholios, I. (2020). A compilation of UAV applications for precision agriculture. Comput. Netw. 172, 107148. doi: 10.1016/j.comnet.2020.107148
Rizov, M., Pokrivcak, J., and Ciaian, P. (2013). CAP Subsidies and Productivity of the EU Farms. J. Agric. Econ. 64, 537–557. doi: 10.1111/1477-9552.12030
Santangelo, G. D. (2018). The impact of FDI in land in agriculture in developing countries on host country food security. J. World Bus. 53, 75–84. doi: 10.1016/j.jwb.2017.07.006
Schwarzenbach, R. P., Egli, T., Hofstetter, T. B., Von Gunten, U., and Wehrli, B. (2010). Global Water Pollution and Human Health. Annu. Rev. Environ. Resour. 35, 109–136. doi: 10.1146/annurev-environ-100809-125342
Shabanzadeh-Khoshrody, M., Azadi, H., Khajooeipour, A., and Nabavi-Pelesaraei, A. (2016). Analytical investigation of the effects of dam construction on the productivity and efficiency of farmers. J. Clean. Prod. 135, 549–557. doi: 10.1016/j.jclepro.2016.06.145
Shepherd, M., Turner, J. A., Small, B., and Wheeler, D. (2020). Priorities for science to overcome hurdles thwarting the full promise of the 'digital agriculture' revolution. J. Sci. Food Agric. 100, 5083–5092. doi: 10.1002/jsfa.9346
Wang, Y., and Li, N. (2021). Liberalization of trade in intermediate goods and distortion of factor markets. China Ind. Econ. 43–61. doi: 10.19581/j.cnki.ciejournal.2021.09.009
Wolfert, S., Ge, L., Verdouw, C., and Bogaardt, M.-J. (2017). Big data in smart farming – a review. Agric. Syst. 153, 69–80. doi: 10.1016/j.agsy.2017.01.023
Wu, F., Hu, H., Lin, H., and Ren, X. (2021). Enterprise digital transformation and capital market performance: empirical evidence from stock liquidity. J. Manag. World 37, 130–144+110. doi: 10.19744/j.cnki.11-1235/f.2021.0097
Yang, G., and Hou, Y. (2020). The usage of industry robots, technology upgrade and economic growth. China Ind. Econ. 138–156. doi: 10.19581/j.cnki.ciejournal.2020.10.004
Yin, J., Wang, S., and Gong, L. (2018). The effects of factor market distortion and technical innovation on China's electricity consumption. J. Clean. Prod. 188, 195–202. doi: 10.1016/j.jclepro.2018.03.294
Yoo, C. K., Gopinath, M., and Kim, H. (2012). Trade policy reform, productivity growth and welfare in South Korean agriculture. Appl. Econ. Perspect. Policy 34, 472–488. doi: 10.1093/aepp/pps019
Zambon, I., Cecchini, M., Egidi, G., Saporito, M. G., and Colantoni, A. (2019). Revolution 4.0: industry vs agriculture in a future development for SMEs. Processes 7:36. doi: 10.3390/pr7010036
Keywords: digitalization level, agricultural total factor productivity, factor market distortion, large-scale production, heterogeneity
Citation: Fu W and Zhang R (2022) Can Digitalization Levels Affect Agricultural Total Factor Productivity? Evidence From China. Front. Sustain. Food Syst. 6:860780. doi: 10.3389/fsufs.2022.860780
Received: 23 January 2022; Accepted: 07 March 2022;
Published: 28 March 2022.
Edited by:
Ping Zhu, Royal Observatory of Belgium, BelgiumReviewed by:
LiPing Xu, Hunan University, ChinaRazieh Pourdarbani, University of Mohaghegh Ardabili, Iran
Copyright © 2022 Fu and Zhang. This is an open-access article distributed under the terms of the Creative Commons Attribution License (CC BY). The use, distribution or reproduction in other forums is permitted, provided the original author(s) and the copyright owner(s) are credited and that the original publication in this journal is cited, in accordance with accepted academic practice. No use, distribution or reproduction is permitted which does not comply with these terms.
*Correspondence: Rongwu Zhang, cnd6aGFuZ0Bnemh1LmVkdS5jbg==