- 1Department of Entomology, Center for Pollinator Research, Huck Institutes of the Life Sciences, The Pennsylvania State University, University Park, PA, United States
- 2Intercollege Graduate Degree Program in Ecology, Huck Institutes of the Life Sciences, The Pennsylvania State University, University Park, PA, United States
- 3Fruit Research and Extension Center, Department of Entomology, The Pennsylvania State University, Biglerville, PA, United States
Bees depend on flowering plants for their nutrition, and reduced availability of floral resources is a major driver of declines in both managed and wild bee populations. Understanding the nutritional needs of different bee species, and how these needs are met by the varying nutritional resources provided by different flowering plant taxa, can greatly inform land management recommendations to support bee populations and their associated ecosystem services. However, most bee nutrition research has focused on the three most commonly managed and commercially reared bee taxa—honey bees, bumble bees, and mason bees—with fewer studies focused on wild bees and other managed species, such as leafcutting bees, stingless bees, and alkali bees. Thus, we have limited information about the nutritional requirements and foraging preferences of the vast majority of bee species. Here, we discuss the approaches traditionally used to understand bee nutritional ecology: identification of floral visitors of selected focal plant species, evaluation of the foraging preferences of adults in selected focal bee species, evaluation of the nutritional requirements of focal bee species (larvae or adults) in controlled settings, and examine how these methods may be adapted to study a wider range of bee species. We also highlight emerging technologies that have the potential to greatly facilitate studies of the nutritional ecology of wild bee species, as well as evaluate bee nutritional ecology at significantly larger spatio-temporal scales than were previously feasible. While the focus of this review is on bee species, many of these techniques can be applied to other pollinator taxa as well.
Introduction
Bees depend on flowering plants to meet their nutritional needs, while nearly 80% of flowering plant species, including many economically and nutritionally important agricultural food crops, benefit from the pollination services provided by bees and other animals (Klein et al., 2007; Eilers et al., 2011; Jordan et al., 2021; Rodger et al., 2021). Understanding the nutritional requirements of bees, and how these requirements shape foraging preferences across different flowering plant species, is essential for creating, maintaining, and improving habitat for bee conservation in urban, agricultural, and natural landscapes, as well as to support attraction of bees to agricultural crops (Vaudo et al., 2015). The approaches used to investigate bee nutritional ecology require detailed studies that, ideally, are carried out over broad spatio-temporal scales and span analysis of both foraging preferences and the outcomes of consuming nutritional resources provided by different plant species. However, conducting studies at these broad scales is logistically and economically challenging, and thus most studies fall into one of three experimental categories: identification of floral visitors of selected focal plant species, evaluation of the foraging preferences of adults in selected focal bee species, or evaluation of the nutritional requirements of larval or adult focal bee species in controlled settings.
Developing a holistic understanding of bee nutritional ecology requires studies that integrate across all three of these approaches. Such integrative studies have been, to some extent, conducted with the most commonly managed and commercially available species: honey bees (Apis mellifera) (Wright et al., 2018), bumble bees (Bombus sp.) (Vaudo et al., 2016) and mason bees (Osmia sp.) (Filipiak and Filipiak, 2020). Other species that have been managed for commercial or research purposes that may also be amenable to such integrative studies, including leafcutting bees (Megachile sp.) (Fischman et al., 2017), alkali bees (Nomia sp.) (Kapheim and Johnson, 2017), stingless bees (Tetragonula carbonaria) (Trinkl et al., 2020), squash bees (Eucera pruinosa) (Chan and Raine, 2021), and halictid bees (Lassioglossum albipes) (Kocher et al., 2013). However, the current approaches typically used for investigating nutritional ecology of readily managed bee species are not easily applied to most wild bee species, particularly to solitary, ground-nesting species that represent 70% of the ~20,000 wild bee species in the world (Harmon-Threatt, 2020).
In this review, we discuss the methodologies used in three different approaches, their associated advantages and limitations, and how these methods can be applied to other managed and wild bee species (Figure 1). When possible, we highlight examples of species outside of honey bees, bumble bees, and mason bees. We then explore the emerging technologies which have the potential to improve our ability to assess bee-plant interactions and impacts on bee health across a broader range of species, habitats and communities, and may facilitate integration across the three experimental approaches. To obtain an unbiased view of the field, we used Web of Science (https://www.webofscience.com/wos/woscc/basic-search) to conduct a literature review with the terms: “bee,” “pollen,” “nectar,” and “nutrition.” Additional peer-reviewed literature was identified by examining associated citations and references.
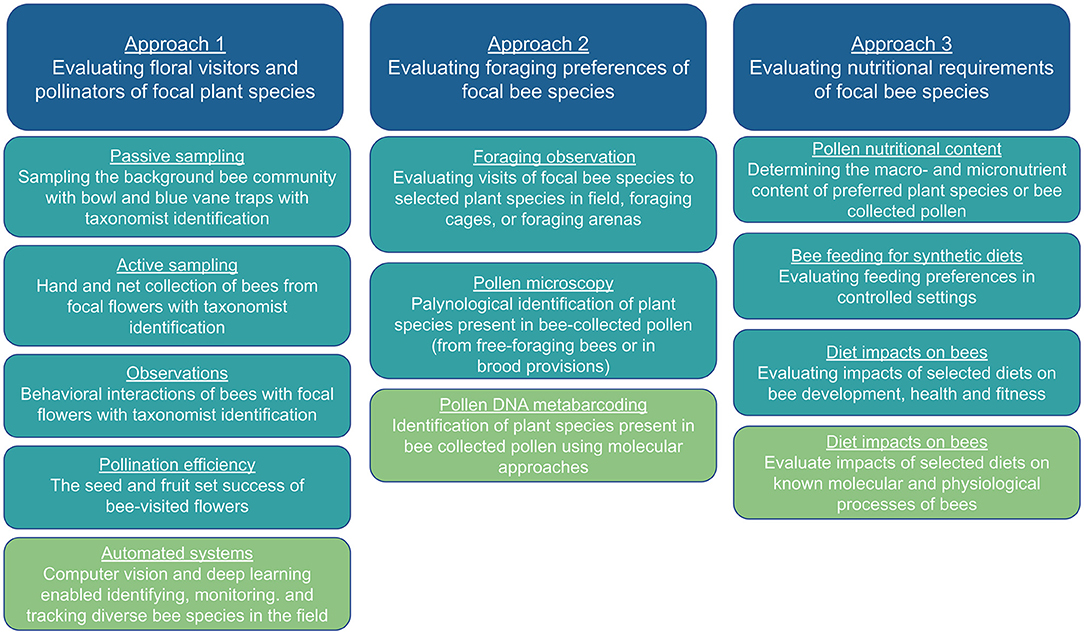
Figure 1. An outline of each research approach in bee nutritional ecology and their main methodologies.
This review focuses on bee pollinators and nutrition provided by pollen, since bees are the primary consumers of pollen, and both dietary preferences (O'Neill and O'Neill, 2011; Hendriksma and Shafir, 2016; Muth et al., 2016; Vaudo et al., 2016, 2020a,b; Vanderplanck et al., 2017; Hendriksma et al., 2019a; Kraus et al., 2019; Trinkl et al., 2020) and bee health outcomes (Kapheim and Johnson, 2017; Smart et al., 2019; Filipiak and Filipiak, 2020; Lawson et al., 2020; Ruedenauer et al., 2020; Austin and Gilbert, 2021) are strongly linked to variation in the composition of pollen (Vaudo and Leonard, 2021). Though nectar is also an important component of bee diets (Leach and Drummond, 2018) that influences pollinator preference (Prasifka et al., 2018), as it provides a source of carbohydrates, amino acids, and vitamins (Nicolson, 2011), nectar foraging choice and nutrition merits a separate review and is outside the scope of this work. Additionally, while we focus on bee pollinators, these approaches also have the potential to be used to study the nutritional needs of other pollinator taxa, such as flies, beetles, and wasps. Though these groups are significant contributors to crop pollination services (Rader et al., 2016), they are historically understudied in comparison to bees. An improved understanding of the nutritional needs of diverse pollinator taxa, and how these can be met by different plant species, can greatly improve the development of pollinator habitat in different landscapes.
Approach 1: Evaluating The Floral Visitors and Pollinators of Focal Plant Species
There is tremendous interest in restoring or creating habitat that can support either focal bee species or diverse pollinator communities. Florally complex habitats can support more diverse wild bee communities in urban (Theodorou et al., 2020), agricultural (Wood et al., 2015; Kammerer et al., 2016), and natural landscapes (Neumüller et al., 2021). However, creating and maintaining these florally diverse plant communities can be quite costly (M'Gonigle et al., 2016). Thus, a detailed understanding of which plant species and cultivars, individually or as a community, can support target bee species, the most diverse bee species, or the most robust and resilient pollinator community, can be a valuable tool to support land management decisions. For example, in evaluations of 25 ornamental herbaceous plant species and cultivars, Erickson et al. (2021) found that planting schemes with the six most attractive cultivars (nested within 3 plant species) could support four times as many bee species, including many specialist and rare bee species, compared to planting schemes with the six least attractive cultivars. In addition, floral enhancement schemes in agricultural areas can provide ecosystem services (e.g., increasing soil structure, reducing surface water flow, and recruitment of other beneficial insects), and trees and shrub rows can provide these services with lower costs and more floral resources per unit area (USDA NRCS Maine, 2012; Wratten et al., 2012; Donkersley, 2019). However, selection of an inappropriate plant species to enhance an agricultural area can lead to ecosystem dis-services, such as pest attraction and competition for water and nutrients between floral enhancement and crop plants (Zhang et al., 2007). Agricultural systems that supply diverse floral resources across time, including before or after bloom of target crops, also have a higher diversity of bee species (Heller et al., 2019). Thus, identifying the key plant taxa that can support pollinators in different habitats and for different outcomes can allow stakeholders to make the most informed and cost-effective land management decisions with regards to pollinators.
A primary goal of planting schemes and habitat support strategies in agricultural areas is to increase wild bee populations and improve pollination services (Pufal et al., 2017). Improving pollination services of focal crops first requires identification of the most common floral visitors to crop plants in each geographic region (Venturini et al., 2017; Garibaldi et al., 2020), and subsequently identification of which visitors are the most effective at transferring pollen to support fruit and seed set (Sihag, 2018). This can be particularly important in areas where invasive species may rob crops of resources without providing pollination services (Smith-Ramirez et al., 2021). Identifying the most effective and important pollinators can be achieved by comparing seed set or fruit production in bee pollinated, hand-pollinated, and closed flowers (Benjamin and Winfree, 2014), or by quantifying the number of transferred pollen grains after virgin inflorescences are pollinated by an individual bee (Rader et al., 2016). The most important pollinator for a particular crop may vary substantially depending on the context: for example, honey bees are poor pollinators of blueberries but can be highly effective when bumble bees are also present, and the most important pollinators of blueberries can vary with geographic region and blueberry cultivar (see BOX 1 for further discussion). Subsequently, planting schemes can be identified that attract and support these pollinator species in the target agricultural system. However, it is also possible that high abundance of attractive flowering plant species that flower at the same time as the target crop system can reduce pollination services, and thus care must be taken to select plants that flower before and after the target crops (Isaacs et al., 2017). Note, however, that these pollinator dilution effects are context dependent (Sardiñas and Kremen, 2015) and are more likely to occur when there are multiple simultaneously-mass-blooming crops (Holzschuh et al., 2011; Grab et al., 2017) versus due to supplementation with wildflowers (Sidhu, 2013; Lundin et al., 2017; Knapp et al., 2019).
Box 1. Identification of key pollinators of agricultural crops: a case study in blueberries.
Pollination research for blueberry cropping systems can be used as a prime example in addressing the goals of (1) identifying key pollinators and (2) determining pollination efficacy of target agricultural crops. When examining pollination by key visitors of rabbiteye blueberry (Vaccinium ashei), Sampson et al. (2004) found that honey bees frequently visit blueberry flowers to “rob” nectar and are unable to collect large amounts of pollen due to their inability to buzz pollinate. However, the sheer number of visiting honey bees that pick up loosened pollen from complementary pollinators (e.g., buzz pollinating bumble bee species), and their high propensity to switch between cultivars, results in effective cross-pollination of blueberry during honey bee nectar collection (Hoffman et al., 2018; Estravis-Barcala et al., 2021). Note, however, that this is not true for all cropping systems with differences in floral morphology. Similar studies in other blueberry cropping systems have found that wild pollinators of blueberry vary across geographic regions, production types, blueberry species, and cultivar. For example, in highbush blueberry, production in Michigan relies on bumble bees, mining bees (Andrena sp.), and bees from the subfamily Halictidae (Tuell et al., 2009), while production in Washington and Oregon is almost completely reliant on honey bees (Gibbs et al., 2016; Hoffman et al., 2018). In addition, southern production of rabbiteye blueberry relies heavily on the southeastern blueberry bee (Hapropoda laboriosa) and bumble bees (Cane and Payne, 1993). Therefore, detailed context-specific studies are needed for cropping systems to understand how to best support the relevant pollinator communities. Aside from nutritional support, land managers can also support target bee species by providing nesting materials (e.g., paper straws and nesting boxes for cavity nesting bees) or implementing cultural practices (e.g., using no-till strategies to conserve ground nesting bees) (Ullmann et al., 2016).
Pollinator visitation patterns to selected plant species (either experimentally placed in a landscape or naturally present in the landscape) are used to evaluate pollinator plant preferences and are often used as a proxy for evaluating nutritional preferences and requirements. However, pollinators may be foraging across a diversity of plants in the landscape and thus supplementing their nutrition from other sources, and thus the most attractive plant in a particular context may not be the most nutritionally optimal plant (Kraus et al., 2019). Thus, integrating studies of plant visitation preferences with evaluation of foraging preferences of focal bee species or controlled studies of the effects of diets on these bees can provide a more holistic understanding of how these attractive plants contribute to bees' nutritional requirements. Below we discuss current and emerging methods to monitor pollinator attraction to selected flowering plants or sites with selected flowering plants species.
Current Methods: Bowls, Blue Vane Traps, Hand- Or Net-Collections and Observations
Methods to identify pollinator visitation patterns include passive sampling techniques (which sample bees and other insects that are present at a particular site, and not necessarily visiting the focal flowering plant species), hand/net collection of pollinators on focal plants, and direct observations of pollinator interaction with the flowers on the focal plant species. Though these methods may be used with all bee species, they vary in benefits and disadvantages, which include biases toward different taxa, time constraints, and false detection.
The most commonly used passive sampling tools in pollination research are bee bowls and blue vane traps. Bee bowls, or pan traps, are containers of soapy water colored in blue, yellow, or white to attract and collect bees (Droege et al., 2017). This method is inexpensive, requires no training to use, and can sample continuously over time. However, bowl traps are biased in that they are more likely to attract pollinators when the surrounding floral community is less dense (Kuhlman et al., 2021), are less likely to capture larger bees (Rhoades et al., 2017), and their efficacy can vary depending on bowl size and the degree of ultraviolet reflection, which can vary among manufacturers (Prendergast et al., 2020). Like bee bowls, blue vane traps (BVTs) are also used as a passive sampling method, where a base is attached to a UV reflective blue vane top to attract and trap bees. BVTs are particularly useful for broadly sampling the insect community (Gibbs et al., 2017), are more successful in capturing larger bees and attract less non-bee bycatch species than bee bowls, but are less likely to capture small, parasitic species such as Nomada and Sphecodes (Joshi et al., 2015). Because BVTs are less likely to be disturbed by wildlife scavenge for water, researchers can use diluted ethylene glycol as a killing and preserving fluid rather than soapy water. Preservation with ethylene glycol rather than soapy water prevents bee degradation, which allows researchers to only check traps periodically rather than daily. However, though BVTs have many benefits, it has been suggested that these traps may oversample areas and negatively impact pollinator populations (Gibbs et al., 2017). Regardless of the method used, passive sampling still has limitations in determining focal pollinators and the background pollinator community. For example, a bee species may not be detected if more attractive flowers are nearby (Baum and Wallen, 2011; Kuhlman et al., 2021). In addition, passive trapping methods under-sample certain bee species, such as cleptoparasitic species (Mackert, 2019), and trap other insect species, such as beetles, ants, and flies (Prendergast et al., 2020; Montgomery et al., 2021). Passive sampling methods also cannot confirm that captured species are actually visiting focal plant species near traps. Therefore, these methods should be used with caution and used in supplement with other detection methods.
Active methods include direct observation and targeted collection directly from flowers. Researchers can capture bees visiting flowers by sweep net, insect-vacuum, or by hand with vials. Collection alone does not indicate that bees are visiting the focal plant, as sweep netting and insect-vacuums can collect individuals that are near flowers but not collecting nectar or pollen. Direct observations are required to determine if pollinators are collecting nectar and/or pollen from a focal flowering plant. A benefit of active methods is that unlike passive sampling, these methods confirm that a particular bee species actually visited the focal plant. However, active methods take more time and trained collectors or observers, are biased toward slow-flying bees, and lack the potential to continuously sample as passive traps do (Wilson et al., 2008; Prendergast et al., 2020). Observations may also be biased depending on the level of expertise of the observer, and identification of more cryptic genera such as Andrena or Lasioglossum is not possible without immobile specimens and a microscope (Biddinger et al., 2013b; Sutherland et al., 2015). Time is also a variable that must be taken into consideration. Studies often only collect or observe bees at each focal plant for a few minutes before moving to the next, potentially missing important visitors. For example, Vaudo et al. (2014) found that certain plants had high visitation rates at particular times of the day, which could have been missed if visitation had not been consistently observed in a controlled setting (this study took place in a greenhouse). Some crops such as pumpkin also have long bloom periods and may be dependent on different pollinator taxa throughout this period. As such, preliminary work should be conducted to determine bee foraging schedules and floral bloom periods before designing optimal sampling or observation strategies.
Factors other than nutrition also impact which bees may visit a focal plant, as nutrition is not the only driver of bee populations in a study area. Certain species require particular nesting materials to persist in a given area (e.g., small carpenter bees Ceratina require hollow, pithy stems, and mason bees require a choice of soil textures] (Pinilla-Gallego et al., 2018; Rehan, 2020). Management practices such as pesticide application can also negatively affect bee populations, with differential pesticide sensitivities across species (Biddinger et al., 2013a; Woodcock et al., 2016; Manjon et al., 2018). Tilling can also reduce populations of ground nesting bees (Ullmann et al., 2016). Therefore, a bee species that is expected to be present in a study system may not be due to lack of nesting resources or other stressors, such as pesticide exposure, and not due to the nutritional content of the focal plant. Floral rate of visitation may also be confounded by the surrounding plant-pollinator community (Essenberg et al., 2012; Valido et al., 2019). Attractive plants nearby or competition among bee species may alter visitation rates to the focal plant species. Accounting for variation bee populations, species richness, and visitation rate due to variance in other resources, environmental stressors, and the background community of plants and pollinators requires studies with diverse background communities in different habitats or geographic regions, which can be time consuming and costly. Determining visitation of certain plants may also be difficult due to challenges in sampling from or observing focal plant species. For example, tall trees such as black cherry (Prunus serotina) have blooms that are several meters above the ground, which require a lift to allow researchers to access (McLaughlin et al., 2021).
Even if care is taken to prevent bias from collection methodology, observers, timing, and landscape factors, there are still significant constraints when using the above methods. First, there is the significant challenge of insect identifications, as it may be quite time-consuming, and requires highly trained scientists who can identify the hundreds of bee species that may be present in a given region (Jamieson et al., 2019; Kilpatrick et al., 2020; Engel et al., 2021). When trained scientists are not available, bees may be identified to morpho-taxa, or easily identified species and groups of bees with common morphological traits. Common groups used include: honey bees, bumble bees, large carpenter bees, hairy legged bees, small black bees, large black bees, and small green bees, but these classifications differ (and may be more specific) based on research goals and the geographic area of interest (Ullman et al., 2008). Identification to morpho-taxa, while easier, can only give information on very broad ranges of pollinators and does not provide detailed taxonomic classifications that aid in practical application of results. Sorting Hymenoptera by morpho-taxa can also underestimate species richness by 12%, and non-specialists have been found to correctly group only 44% of taxonomic species (Derraik et al., 2010).
For Approach 1, researchers have traditionally identified and evaluated the key floral visitors of focal plants by using passive and active collection or observation methods. Though these methods can be applied to all bee species, there have been limitations in extensively sampling bees across time and landscapes. Properly identifying wild bees that visit focal plants has also constrained these studies. Below, we discuss how automated monitoring and identification technologies will allow researchers to address questions of floral visitation at much larger spatio-temporal scales than has previously been feasible.
Emerging Methods: Automated Monitoring and Identification Technologies
Cameras, automated monitoring systems, and automated identification technologies have rapidly advanced since their inception (Devillers, 2014; Preti et al., 2021). Using cameras to monitor insect behavior is not a new concept and was first conducted by Kondo et al. in 1994 (Reviewed in Preti et al., 2021). However, using cameras to monitor insect plant-pollinator interactions was not demonstrated until 2009 (Marten-Rodriguez and Fenster, 2008). Then, in Lihoreau et al. (2012), motion-sensitive cameras were used for the first time to track pollinator movement. Researchers have also furthered the field by developing automated data processing and artificial intelligence programs that present unique opportunities to study plant-pollinator interactions (Høye et al., 2021).
The development of automated monitoring and identification systems will allow researchers to study plant-pollinator interactions across greater spatio-temporal scales than has previously been feasible. Installation of cameras at focal plants can allow researchers to collect exceptionally large datasets to answer questions that would be nearly impossible, or extremely time consuming, to answer by human observation or sampling alone (Høye et al., 2021). Plant-pollinator interactions may be recorded continuously across multiple days by cameras, and cameras may also be placed in many geographic regions or landscape types to monitor all sites simultaneously. Klomberg et al. (2022) were able to simultaneously monitor plant-pollinator interactions at an elevational gradient along 6 different transects. Similar studies conducted by human observations can only capture the community of insect visitors during a snapshot of time, which can miss key pollinators, such as squash bees that forage very early in the morning and retreat to below-ground nests in the evening. Frequency and richness of insect visitation is also dependent upon weather and time of day, and studies with human observation alone could be biased by observation period (Goodwin et al., 2021). Finally, plant-pollinator community dynamics change throughout seasons, between seasons, and vary by year (Mora et al., 2020). Even if constant human observation is possible, sampler fatigue interferes with observation after a short period, and samplers may be biased to notice larger, showy species more than smaller, fast-moving species (Biddinger et al., 2013b). Continuous, reliable monitoring by cameras across seasons and years can uncover more fine-scale nuances in a changing pollinator community.
However, the collection of these large data sets is only beneficial if coupled with artificial-intelligence powered computer-vision based automated identification systems. On a basic level, computer vision and deep learning systems can identify when a pollinator is present at the focal flower and only record these small bursts of activity, thereby drastically decreasing data storage and battery power of field equipment (Pegoraro et al., 2020). These short bursts of video or photos can then be viewed by a researcher to identify the pollinator and behavior exhibited. For example, Steen (2017) monitored 24-h forager activity rhythms using automated camera monitoring and data processing. This study would have been extremely labor intensive without automated systems, as researchers would have to parse through data manually to identify useful photos. This technology may also be used in conjunction with automated identification systems to determine which insects are present (Raitoharju et al., 2018). These systems are still limited in that they require training with large image libraries before they can successfully identify subjects, which involves hours of researcher observation and outlining of pollinators in photos. While the development of large, shared image libraries can facilitate the development of automated identification systems, often these systems require images that are taken in similar contexts as the functional camera systems. In addition, certain bee taxa are easier to distinguish between than others. For example, Spiesman et al. (2021) used convolutional neural network classification models to distinguish between 36 different species of bumble bees in North America with a ~92% accuracy rate, but many species have different phenotypes by region, such as Bombus rufocinctus, and were often falsely identified as other species. Therefore, current automated identification systems require distinct physical characteristics that are visible to cameras and easily measured, which may not be possible with more cryptic bee genera that are distinguished by minute details, such as Andrena, Lassioglossum, or Melissodes. However, cameras could be combined with other monitoring tools, such as radar to monitor wing beat frequency, which has been used to identify moths (Wang et al., 2017). Bees that may not be identified by computer systems may also have the potential to be identified by experts, depending on freeze-frame image quality. This may be particularly useful for fast-moving species that are difficult to tell apart by observing by eye alone, and visual attractants could be used to pull bees to a single camera. In Box 2, we briefly discuss how bee identification may be used in parallel with molecular methods in Approach 2. Artificial intelligence systems can also track bees as they move throughout the camera frame, allowing more complex behaviors to be quantified. Ratnayake et al. (2021) utilized this image-based tracking software to track unmarked honey bee foragers in the field. This software can account for multiple floral choices by the same insect rather than counting each bee as a single visitation event. Previous image-based tracking methods have required barcoded tags, which require a large time investment to apply to many individuals (Greenwald et al., 2015; Gernat et al., 2018; Jones et al., 2020).
Box 2. Using DNA metabarcoding for bee identification.
DNA metabarcoding is a molecular approach used to identify organisms by targeting conserved molecular markers across species (see Approach 2 for more details). Though we primarily discuss using DNA metabarcoding to identify the plants that bee-collected pollen originated from, this approach may also be used to identify bees. Morphology has traditionally been used to identify bees to species, but DNA metabarcoding can resolve cryptic species or ambiguous species complexes where morphology cannot (e.g., Nomada, Andrena, and Ceratina) (Darby et al., 2020). This method has also been successfully used to simultaneously identify Lepidoptera hosts and Hymenoptera parasitoids (Šigut et al., 2017), aquatic macroinvertebrate communities (Serrana et al., 2019; Turunen et al., 2021), and pest species (aphids and psyllids) (Batovska et al., 2021). DNA metabarcoding estimates higher diversity than traditional morphotyping, particularly in more cryptic taxa (Šigut et al., 2017), but is a poor detector of overall abundance (Darby et al., 2020). As such, DNA metabarcoding could be combined with other methods, such as computer vision and deep learning enabled automated identification (Høye et al., 2021) or morphological identification (Batovska et al., 2021) to most accurately determine species richness and abundance. However, developing libraries of the barcoding sequences for bee species is time consuming and costly, as multiple sequences are needed per bee, and there are over 4,000 bee species in the United States alone (Danforth, 2019). As more insects are sequenced, there will likely be greater ability to distinguish among insect populations and cryptic species, though identification of cryptic species can require integration of molecular information, morphological information, and natural history (Hubert and Hanner, 2015).
Though these technologies present intriguing possibilities, there are still challenges to applying them broadly across bee species. First, automated identification will still have limitations at the genus and species level for many taxa regardless of how vast the reference library is, as some minute details may only be viewed under a dissecting microscope or discerned by an expert (e.g., wing venation, scopal hair structure, facial fovea, etc.). Thus, investment in improving current online bee identification keys (e.g., Discover Life, https://www.discoverlife.org/mp/20q?guide=Bee_genera), which are more geared toward those already trained in taxonomy, should occur concurrently, as it will be necessary for anyone using automated identification technology to assess the accuracy of their results. Future integration of algorithm and camera systems that allow for recording when movement is detected, automated identification of insects, and behavioral tracking of individual bees will expand the spatio-temporal scales at which plant-pollinator interactions can be studied.
Approach 2: Evaluating The Foraging Preferences of Focal Bee Species
While monitoring visitation rate patterns to focal plant species can provide some understanding of the preferences of selected bee species among those focal plant species, bees are typically foraging broadly across many plant species present in an ecological community, and their interactions with a focal plant species may be influenced by the presence of other flowering plants and co-foraging pollinators (Baracchi, 2019). Thus, detailed studies evaluating the broader foraging and nutritional preferences of a focal bee species are necessary to comprehensively understand the requirements of that species.
Bee species may be broadly categorized as pollen generalists or pollen specialists. Pollen generalists are referred to as polylectic and collect pollen from a diversity of plant genera to meet their nutritional needs. Bees that are pollen specialists may be categorized as oligolectic, or those that collect pollen from a small number of plant genera, while bees that only forage on a single plant genus or species are categorized as monolectic (only a few dozen described species fall into this category) (Cane, 2021). Within these categories are parameters for “broad” or “narrow” dietary tendencies to further characterize pollen preference and obligate associations, but for the purposes of this review we will refer to only the main three categories (Cane and Sipes, 2006).
Determining the individual preferences of bee species will allow land managers to design planting schemes that will support the nutritional needs of many species. Aside from containing plants that satisfy focal species' needs, one of the most important tenants of designing planting schemes is to attract a diverse community of bee species by planting a diversity of species from different plant families (Fründ et al., 2010; Heller et al., 2019; Theodorou et al., 2020). Diverse floral communities can simultaneously support the needs of both specialist and generalist bee species through nested designs (Vaudo et al., 2015). Diverse floral communities allow polylectic bees to forage for pollen across multiple plant taxa, which vary in nutritional concentrations, and potentially to balance pollen nutrients (i.e., proteins and lipids) to optimum ratios (Vaudo et al., 2020a). Integrating plants used by specialist species within these communities can also support oligolectic and monolectic bee species that may have limited foraging distances and phenologies due to environmental constraints (Vaudo et al., 2015). In addition to providing optimal nutritional resources, a diverse plant community also provides a mechanism for polylectic and oligolectic bees to dilute toxic secondary plant compounds in pollen from certain plant species that would otherwise lead to adverse health effects (Eckhardt et al., 2014; Treanore et al., 2019). Indeed, it is clear that diverse floral communities are needed to support the majority of individual bee species and diverse bee communities. However, as noted above, it can be costly to create and manage a plant community that consists of a large number of plant species, genera and families, and thus identifying the most important plant species can improve adoption and maintenance.
It is important to note that bee foraging preferences are not simply a result of a bee's nutritional requirements. The co-evolution of flowering plants and bees has resulted in intricate associations between floral traits and bees' sensory ecology (e.g., ability to detect chemical and visual cues), anatomy (e.g., long vs. short tongued bees), cognitive ecology (e.g., learning and memory, floral handling strategies such as buzz-pollination), and foraging strategies (e.g., floral constancy, traplining, and solitary vs. eusocial strategies) (Chittka and Thomson, 2001). For instance, Vaudo et al. (2020b) found that Osmia cornifrons in the northeastern United States collected pollen from plant species that originated in their native range (Japan) as well as plant species that are native to the United States that this species did not co-evolve with. The bees' ability to collect pollen from plants that they did not co-evolve with may be a result of nutritional requirements, similarities in floral traits between co-evolved and non-co-evolved plant species, or some combination of these factors.
The methods described below can be used to identify the plant species and genera that both managed and wild bees preferentially collect pollen from, and this information can be used to both optimally design pollinator habitat and provide insights into how different floral traits influence bee pollen foraging (Erickson et al., 2022). These approaches can be used with both pollen collected from foraging adults or from larval provisions within the nest.
Current Methods: Foraging Observation
One of the key methods used to assess plant or pollen preference of a focal bee species is by observation of visitation. Researchers may test particular plants in an open field setting to see which plants bee species prefer (Nicholls and Hempel de Ibarra, 2017), but this type of method is more often used with plants as the species of interest rather than bees, as described Approach 1. Observations may also be conducted in an enclosed or controlled setting, such as a hoophouse, foraging arena, or flight cage. These methods have been used with honey bees (Hendriksma et al., 2019a), bumble bees (Russell et al., 2016), mason bees (Boff et al., 2021), and wild bees (Dukas and Real, 1991; Howard et al., 2021). Hoophouses or flight cages can be quite large (>10 ft in width or length) to conduct studies where many bees are permitted to forage, while foraging arenas tend to be smaller sized and maintained with the lab for foraging trials with individual bees. Foraging arenas typically consist of a box with a plexiglass wall for viewing and a forager entrance. Preferences can be assessed by offering flowers, dishes of pollen, or even artificial diets to understand bee nutritional requirements (Vaudo et al., 2016). Real flowers offer more realistic scenarios and can be used for floral trait choice rather than nutritional preference alone, but also come with confounding variables such as floral humidity and volatiles that play a role in attraction (Arx et al., 2012; Burkle and Runyon, 2019). Imitation flowers, pollen, and artificial diets do not have these confounding variables, but are less field-realistic. Any foraging choices should have randomized locations within the enclosure that are spatially rearranged between trials to prevent bias from forager learning and trap lining (Saleh and Chittka, 2007; Ohashi and Thomson, 2009). Choices should also be regularly replaced to prevent chemical scent deposition from interfering with future trials (Saleh et al., 2006).
Using enclosed spaces to observe bee foraging patterns allows for more control than field observations. In enclosed settings, individual bee responses, and thus, variation in responses within species, population or colony, can be observed. Foraging arenas also make it possible to observe individuals as “naive foragers,” which allows researchers to understand if preferences are innate or learned (Raine and Chittka, 2007). In addition, extraneous variables are removed from the study system. For example, there is no interference from focal bees visiting other flowers, or from other pollinators competing with the focal bee species (Russell et al., 2017; Adler et al., 2020). Furthermore, the timing of foraging can be controlled such that observations are initiated as bee foraging initiates (thus, the bees are not visiting flowers outside of the observation period) and the bees are allowed to forage when all flowers are open (see Vaudo et al., 2016) and so are choosing among all flowers. The difficulty associated with enclosed foraging studies is that they are primarily amenable to bee species that can be managed in an indoor or semi-field setting. However, it is possible to collect wild bees from a natural setting and transfer them to a foraging arena for a short period, though there are few examples of this method in the literature (see Howard et al., 2021 for Lassioglossum and Dukas and Real, 1991 for Xylocopa). Even managed bees do not always forage in an enclosed setting, and it is likely that researchers will require some understanding of how a focal bee species perceives its environment before arriving at a successful arena design. For example, UV ray intensity has been shown to impact indoor bee foraging, positively or negatively, depending upon species (Kendall et al., 2021). Even with previously used managed bees, each flight cage or foraging arena design may also require additional troubleshooting during preliminary data collection. Bee behavior can be influenced by variables such as light source, light intensity, and color, and each experimental protocol will require adjustments that are appropriate for the questions and surrounding environmental context (Chittka and Thomson, 2001; Erickson and Russell, pers. comm.). Regardless of optimization, there will be cases where a forager will not complete a foraging bout, so many trials may be necessary to meet statistical significance. As such, these studies can take a significant amount of time to complete.
Current Methods: Species Identification of Bee-Collected Pollen
Bee floral preferences may also be determined by examining whole bee pollen provisions sampled from bees foraging under natural conditions. Because these samples can be collected from bees foraging in the field, it is possible to assess preferences across the broader plant community at the field or landscape scale, versus providing bees with a small number of focal plants and assessing visitation through direct observation. The plant species bees visit, and the proportion of visits to each species, can be determined by examining pollen via microscopy (Campos et al., 2021). Pollen can be removed from bees collected from field sites, as they forage on flowers or return to the nest, or pollen can be collected from stores and larval provisions within nests. Pollen provisions have previously been collected from nests in wood, stems, soil, and even empty snail shells, and thus these methods should be amenable to use with any wild bee species (Müller et al., 2006). Pollen microscopy has been used to identify pollen sources in provisions from honey bees (Lau et al., 2019), bumble bees (Whittington et al., 2004), mason bees (Kraemer and Favi, 2005), and wild bees (Larkin et al., 2008). Pollen samples can be used if samples are dry, frozen (Pinilla-Gallego and Isaacs, 2018), suspended in ethanol or in insect tissues (Jones, 2012, 2014), and thus this method is feasible to also use with museum or stored samples, and is not impacted by differences in amounts of nectar or water mixed into larval pollen provisions (e.g., dry, doughy provisions of Osmia cornifrons vs. the soupy provisions of Osmia pumilla) (Wood et al., 2019). The benefit to these methods is that the scale of studies can be drastically increased (from a set of focal plants to the landscape scale, and across sites), and foraging preferences of individual bees can be evaluated, rather than averaging across individuals from a set of observations. This also prevents personnel from having to follow bees as they forage in the field, which can be quite difficult. Determining the plants visited in a landscape can also be used to examine entire plant-pollinator networks to understand how communities are interacting, and if these community networks change between seasons due to plant or bee cycles within particular species (Arceo-Gómez et al., 2020). Examining whole pollen collections of many individuals from the same species can also show trends in visitation that observations alone may not detect (Pornon et al., 2017), or individual variation within a colony or species (Klein et al., 2017; Hendriksma et al., 2019a). However, though examining whole pollen provisions is a useful strategy to employ, it is important to consider what choices bees made in the landscape based on available flowering plant species. Conducting community plant surveys to determine what plants were most and least common can help researchers understand if bees are collecting what happens to be most abundant or if they are seeking out rare species. Determining broad trends in plant preference can lead to choice tests between individual plant species and cultivars to narrow down the best planting choices for developing pollinator forage habitat, or to eliminate competitive species around a crop that is the target of pollination.
Researchers have traditionally used microscopy to view bee-collected pollen and identify these samples to plant taxa the sample was derived from Campos et al. (2021). Pollen acetolysis, a process wherein pollen is treated with powerful acids to remove cellular content, is often used to make the morphological features of pollen grains more visible (Jones, 2014; Halbritter et al., 2018). Staining with safranin O during slide mounting also improves contrast of features. One of the main benefits of using microscopy over other methods is that pollen species may be identified even if the available quantity is quite low, which is necessary when recovering pollen from bee tissues. However, pollen slide preparation requires a significant time investment and is not a high-throughput method. Proper pollen identification also requires experience and training. Identification keys are freely available from the Global Pollen Project database or natural history museums, but the vast majority of plants are not documented in these libraries, and museums often have fragmented collections based on geographic location (Martin and Harvey, 2017). Automated pollen identification programs are also available (Cao et al., 2020; Aperio ImageScope - Pathology Slide Viewing Software, 2021). These programs can make identification less time consuming, as though slide mounting pollen and taking photos of pollen grains is necessary, extensive prior knowledge of pollen morphological traits is not needed. This technique is 98% effective at accurately identifying pollen to species, including species previously considered indistinguishable from one another by palynologists (species in Fagaceae and Asteraceae) (Sevillano et al., 2020). One caveat to this system is that though it has a high success rate, the authors were only able to train and test the system to discern between 46 different species, and training the system to identify pollen from all the flowering plants in the world (~450,000) would take a significant amount of time (Pimm and Joppa, 2015).
For Approach 2, studies evaluating foraging choices in enclosed settings are feasible primarily with managed bee species that forage under such conditions. However, pollen loads of foragers of any bee species can be readily assessed, though it is only possible to collect pollen samples used to feed developing larvae in bees where nests can be managed or easily identified and harvested. Below, we detail how DNA metabarcoding has made examining pollen provisions more high throughput, allowing for additional comparisons across time, bee species, and landscapes.
Pollen Species Identification Using DNA Metabarcoding
The plant species that pollen has been derived from may also be identified by using DNA metabarcoding. This method has widened the breath of species that may be investigated, which include honey bees (Milla et al., 2021), bumble bees (Leidenfrost et al., 2020), mason bees (Vaudo et al., 2020b), and several wild bee species (Gous et al., 2019; Potter et al., 2019; Casanelles-Abella et al., 2021). This technique involves amplifying loci in pollen that are common across plant taxa, but vary in sequence across species, genera, or families. Loci are amplified through multiple rounds of PCR in a nested design (PCR1 amplifies target regions with generic primers, PCR2 attaches next-generation sequencing read-priming oligonucleotides, ad PCR3 adds dual multiplex indices) which can then be sequenced and compared to a reference database such as MetaClassifier (Richardson et al., 2019; Sponsler et al., 2020). This nested design is not required, but initial amplification in PCR improves the robustness of the assay by minimizing any amplification biases and mistagging events (Richardson et al., 2019). Plant-pollinator networks created from DNA metabarcoding are more complete, more accurate, and show fewer specialization linkages than those created with observations alone (Wood et al., 2016; Arstingstall et al., 2021). This technique is high throughput and allows for larger sampling efforts across years, which can help account for variation in plant blooming phenology due to differences in growing degree day accumulation each year. In Box 2, we briefly discuss how DNA metabarcoding may also be applied to bee identification.
DNA metabarcoding can be a powerful approach for screening pollen samples, but it is not as effective in determining the amount of each pollen type as microscopy (Richardson et al., 2015) and is limited in its taxonomic resolution. In most studies, identification is limited to the genus level, though some biomarkers (ITS) perform better than others (rbcL, matK) (Kolter and Gemeinholzer, 2021). ITS2 can provide much better resolution for plant classification than ITS1, but ITS2 use may result in fungal co-amplification (Cheng et al., 2016; Gous et al., 2019). Additional loci that are commonly used are trnL and psbA-trnH (Dormontt et al., 2018). Recent studies have demonstrated that species-level resolution can be improved if the genomic reference libraries are expanded to include sequences from more species. Kolter and Gemeinholzer (2021) demonstrated that species identification was improved by 19% (from 43 to 62%) for the ITS biomarker when the number of ITS sequences/species in the reference library was increased from 1 to 7. Furthermore, the inclusion of additional loci to the analysis can improve taxa resolution, but the identification of these loci can be challenging, as they must be conserved enough to allow binding from a conserved primer set but variable enough to allow for species sequence differences (Kadlec et al., 2017). Johnson et al. (2019) identified 353 single-copy protein coding genes from the One Thousand Plant Transcriptome Initiative and developed a set of 75,151 probes that target these genes that function over 600 angiosperms; this provides tremendous resolution for species identification and phylogenetic analyses. Alternatively, sequencing of organelle (chloroplast) genomes or whole genome skimming using high throughput sequencing can provide a substantial amount of sequence data, but analysis requires that the reference library contains the full sequence for the target species (Johnson et al., 2019).
Though DNA metabarcoding is a high-throughput method, there are still significant limitations in processing the substantial number of samples that are typically necessary for these studies, which can be particularly large if the goal is to create plant-pollinator networks, compare across species, or compare across different land use patterns, which can require hundreds of samples (Cullen et al., 2021). Conducting nested PCR on individual samples also takes a significant amount of time (and thus higher personnel costs) and material required. Microfluidics approaches can significantly reduce handling time and allow for more high-throughput parallel processing but require access to expensive equipment (Gostel et al., 2020). Alternatively, pull-down approaches (where sheared DNA from the samples binds to sequence-specific probes) can allow for multi-sequence amplifications within a single reaction, which can be especially useful if larger numbers of loci are used (Hale et al., 2020). Nonetheless, in all cases, adequate representation of the target species in the reference library is necessary to bioinformatically identify the samples. With efforts to sequence the genomes of plants and animals expanding (Lewin et al., 2018), reference libraries are also expanding, providing new opportunities for improving approaches to study the nutritional ecology of wild bees.
Though bees may visit certain plants, plant visitation cannot necessarily be equated to quality nutrition. For example, honey bees will often prioritize pollen quantity over quality (Leonhardt and Blüthgen, 2012). In addition, solitary bees may sacrifice pollen quality for quantity in poor landscapes, as they must make many small trips to collect resources while conserving energy rather than flying long distances (Ne'eman et al., 2006; Greenleaf et al., 2007; Biddinger et al., 2013a). Therefore, visitation rates, determined by foraging observations or examining pollen provisions, should be carefully considered with choice assays for particular nutrient levels or plant traits.
Approach 3: Evaluating The Nutritional Requirements Of Focal Bee Species
While many studies have evaluated the visitation patterns of different wild bee species to focal flowering plants, or the foraging preferences of wild bee species within a collection or community of plant species, few studies have evaluated how these interactions satisfy wild bee nutritional needs or how plant nutritional properties influence these preferences. Pollen provides the macronutrients protein and lipids, as well as several micronutrients such as potassium, magnesium, calcium, phosphorus, and iron (Campos et al., 2008). The concentrations and ratios of macronutrients (Ruedenauer et al., 2019; Vaudo et al., 2020a) and micronutrients (Mărgăoan et al., 2014; Kostić et al., 2015, 2017) can vary greatly between plant species. The effect of this variation can be evaluated by assessing (1) bee preferences for collecting pollen from different plant species that vary in their nutritional content, (2) bee preferences when consuming synthetic diets that vary in their nutritional content, and (3) impacts on bee development, health, and fitness outcomes from consuming diets that vary in their nutritional content. A truly comprehensive understanding of bee nutritional ecology requires studies that cross all three levels of evaluation.
Current Methods: Evaluating the Nutritional Content of Bee-Collected Pollen and Preferred Plant Species
Nutritional preferences may be determined by measuring nutrient levels of bee-collected pollen, or of pollen from plant species that bees prefer. Methods have been developed to assess the concentrations of different micro- and macronutrients (namely, protein and lipids) in pollen, which are described in detail in Box 3. This approach is useful because it allows the investigator to understand the broad nutritional needs of a bee species rather than plant visitation rates alone. For example, recent studies have evaluated whether protein and lipid content are associated with different foraging preferences in bumble bees and found a preference for plants providing pollen with a specific protein: lipid ratio (Vaudo et al., 2016). These dietary preferences also shift when bumble bee colonies have brood to provision (Kraus et al., 2019). Honey bee collected pollen has also been screened for nutritional content (Ghosh et al., 2020), but few studies have assessed the nutritional composition of bee-collected pollen, or the nutritional composition of plants preferred by bees, in species outside of honey bees, bumble bees, and mason bees (Weiner et al., 2010; Belina-Aldemita Ma et al., 2019).
Box 3. Analytical approaches for evaluating pollen micro- and macronutrient content.
Micronutrients are detected by using high-performance liquid chromatography (HPLC), where metabolites are identified by differences in molecular mass, fragmentation patterns, isotope distribution, and retention time (Chakrabarti et al., 2019). There are few studies that investigate pollen micronutrients, perhaps due to the high cost per sample, the significant amount of time needed to prepare samples, the inability to run multiple samples simultaneously (samples must be processed individually by the HPLC), and large amounts of data that can be difficult to interpret. Data are given as concentrations of different compounds, including alkaloids, glycosides, phenols, terpenes, amino acids, and different classes of lipids (Jackson, 1991). The vast number of variables can make it difficult to compare the nutritional values of diets, and data must be displayed in multivariate formats such as PCA plots or heat maps. See Nicolson (2011) and Wright et al. (2018) for more details on pollen and nectar micronutrient content.
Protein concentration of pollen may be determined by the Bradford assay, the bicinchoninic acid assay (BCA), or HPLC. The most commonly used approach is the Bradford assay. The Bradford assay quantifies crude protein concentration by detecting the color intensity of dye bound to denatured proteins in solution. Protein concentration can be determined by recording this absorbance with a spectrophotometer and comparing sample absorbance values to a standard curve of known protein concentrations (Bradford, 1976; Green et al., 2012). A full protocol can be found in Vaudo et al. (2020b). The BCA assay detects protein by targeting chelated Cu+2 ions from protein backbones, which are then reduced to Cu+1 ions by BCA molecules. These copper ions then form a purple reaction product, and the intensity of this color can be measured by a spectrophotometer and compared to a standard curve (Green et al., 2012).
There have been mixed opinions on which of these methods is best to use for pollen protein detection. The BCA assay detects only polypeptides (whole proteins) rather than nitrogen, or crude protein as the Bradford assay does. Some studies suggest that this method offers more accuracy, as there is non-protein nitrogen in pollen that the Bradford assay may detect, and polypeptide concentration is more relevant to bee nutrition than overall crude protein levels (Vanderplanck et al., 2014). HPLC may also be used to determine levels of amino acids, which is a measure considered total protein content (Weiner et al., 2010; Nicolson and Human, 2013). HPLC may offer more accuracy in protein detection than the Bradford or BCA assays, but there are still the difficulties of high cost, time consuming protocols, and the inability to test many samples simultaneously.
The standard approaches to detecting lipids and carbohydrates are the sulfo-phospho-vanillin and anthrone assays, respectively. These methods are modified from a protocol created for the detection of macronutrients in mosquitoes (Van Handel and Day, 1988). This method has the convenience of separating lipids and glycogen (carbohydrates) in one protocol, allowing users to conduct multiple assays from one sample. Lipids are detected by binding with vanillin, and carbohydrates are detected by binding with anthrone reagent. This results in color intensity changes that can be measured by a spectrophotometer and compared to standard curves of known concentrations (Giri et al., 2019). Full protocols can be found in Vaudo et al. (2020b).
On a broader scale, evaluation of 82 plant species demonstrated that there is a wide range of pollen protein: lipid ratios, and pollen collected from three bee species (honey bees, bumble bees, mason bees) exhibited distinct protein: lipid ratios (Vaudo et al., 2020a). This study then suggests that different bee species may have different nutritional preferences, and thus forage preferentially on different plant species in the field, thereby effectively niche partitioning according to pollen nutrition. However, bees may be able to mix pollen from different plant species to achieve their preferred macronutrient ratios, and thus it remains to be determined if, for habitat restoration practices, it is preferable to provide plants that provide pollen that match a target bee species' protein: lipid ratio or provide plants that span a broader nutritional range, so many species can forage to meet their targets. Ruedenauer et al. (2019) assessed 387 different plant species belonging to 229 genera and 75 different families, and found that pollen protein concentration and protein: lipid ratios vary by phylogeny, suggesting that pollinator preference over time has impacted pollen nutritional content. However, the relationships between pollen nutrition, floral traits, and bee preference have yet to be fully investigated.
Current Methods: Bee Preferences When Consuming Synthetic Diets
Rather than simply evaluating the nutritional content of pollen collected from foraging bees or in the plant species that bees prefer to forage from, it is also possible to conduct detailed bioassays using synthetic or altered pollen diets to evaluate the nutritional preferences for different quantities and ratios of key nutrients, including protein and lipids. The Geometric Framework for Nutrition provides a conceptual and technical approach for these studies (Simpson and Raubenheimer, 2012). For these studies, animals are offered diets that vary in the ratios of two macro- or micronutrients. If the animal eats from only one of these diets, its nutritional intake would fall along the “rail” (represented as a line plotted in a graph representing nutritional space) of that diet, where the ratio is kept constant as the quantity of both micro or macronutrients increases. However, if the animal feeds selectively from more than one diet, its consumption will not fall along the rails for these diets, fall somewhere in between. This preferred ratio is considered the “intake target” of the animal. This intake target can vary depending on the development or physiological state of the animal and can vary between species. In addition, determining which nutrients an animal will compromise in favor of obtaining the correct amounts of another can give insight as to which macro- or micronutrient is most important (Simpson and Raubenheimer, 2012). Such studies in honey bees have demonstrated that pollen protein: carbohydrate ratios (Hendriksma et al., 2019a), protein: lipid ratios (Stabler et al., 2021), and amino acid content (Hendriksma and Shafir, 2016) impact dietary preference, and that micronutrient requirements vary by caste (Filipiak et al., 2017). In addition to the previously mentioned studies on bumble bees, studies also indicate that bumble bees prioritize carbohydrates over protein (Hendriksma et al., 2019a), prefer diets with particular amino acid concentrations and sources (Stabler et al., 2015), and use lipids as a nutritional cue when assessing diet quality (Ruedenauer et al., 2020). Fewer studies have been conducted with solitary bees, but it has been demonstrated that carbohydrates are prioritized over protein in mason bees (Austin and Gilbert, 2021).
Current Methods: Measuring Impacts on Bee Development, Health, and Fitness Outcomes
The approaches above examine bees' nutritional preferences with their assumption that these preferences reflect the optimal diets, but to fully test this hypothesis it is necessary to conduct studies in which bees are fed diets with different nutritional content, and their development, health, and fitness are measured. These studies can be conducted within controlled laboratory settings or in the field. Most field studies that involve habitat restoration by introducing planting schemes are limited in that they often show that managed sites have higher abundance of wild bees, but do not measure background population levels before and after site restoration. The increase in abundance of bees to the flowers or study site may reflect increased attraction to the site, and not necessarily an overall increase in the background population (Kleijn et al., 2011).
While many different measurements of success can be used in laboratory settings to assess the effect of nutrition on wild bee health and fitness outcomes, most wild bee species have yet to be successfully captured and maintained (able to feed on lab diets for an extended time period), reared (raised from immature stage to adult), or bred (raised from immature stage to adult with reproduction) in a lab setting. Bee species used in these studies thus far include honey bees (Hendriksma et al., 2019b), bumble bees (Treanore et al., 2019), mason bees (Filipiak and Filipiak, 2020), small carpenter bees (Lawson et al., 2020), and sweat bees (Roulston and Cane, 2002). Measures of success used in a nutritional assay also depend on life history traits of the focal bee species. For instance, social species can be monitored for colony growth and reproduction (e.g., wax production, resource allocation, and brood levels) (Treanore et al., 2019; Ruedenauer et al., 2020). Cavity-nesting, solitary species do not produce wax or store long-term resources for a colony but can be monitored in trap nests for offspring production and sex ratio, which is a key indicator of fitness and environmental quality in solitary bees (Eckhardt et al., 2014; Filipiak and Filipiak, 2020). Offspring production and sex ratios of ground nesting species can be assessed by taking soil cores of nests, or by pouring talcum powder in tunnels to make removing excess soil around nests easier (Leonard and Harmon-Threatt, 2019). Other measures of success that can be used for any bee species include measuring bee body size (e.g., intertegular distance, head capsule width, body mass, etc.) (Grab et al., 2019), developmental time (Lawson et al., 2020), and survival (Cane, 1987; Praz et al., 2008; Bramke et al., 2019; Fortuin et al., 2021). These physiological methods are beneficial in that they can be determined by examining individuals externally and do not require expensive equipment, but they do not provide evidence as to the underlying nutritional qualities that make a diet good or bad for bee health.
For Approach 3, the nutritional content of collected pollen can be assessed in any bee species in which foragers collect substantial pollen loads (most commonly used methods require ~6 mg of pollen for macronutrient analysis, Vaudo et al., 2020a) or where nests can be managed or easily identified and harvested. Studies of bee preferences for synthetic diets or studies of diet impacts on bee health, development and reproduction can only be completed in bee species that are amenable to some level of management and rearing under laboratory or semi-field conditions, which we detail in Table 1. Box 4 discusses the benefits and constraints of using different species for nutritional assays. Below, we discuss emerging molecular tools to assess the impact of diets on physiology, and how these methods may be applied to additional wild bee species.
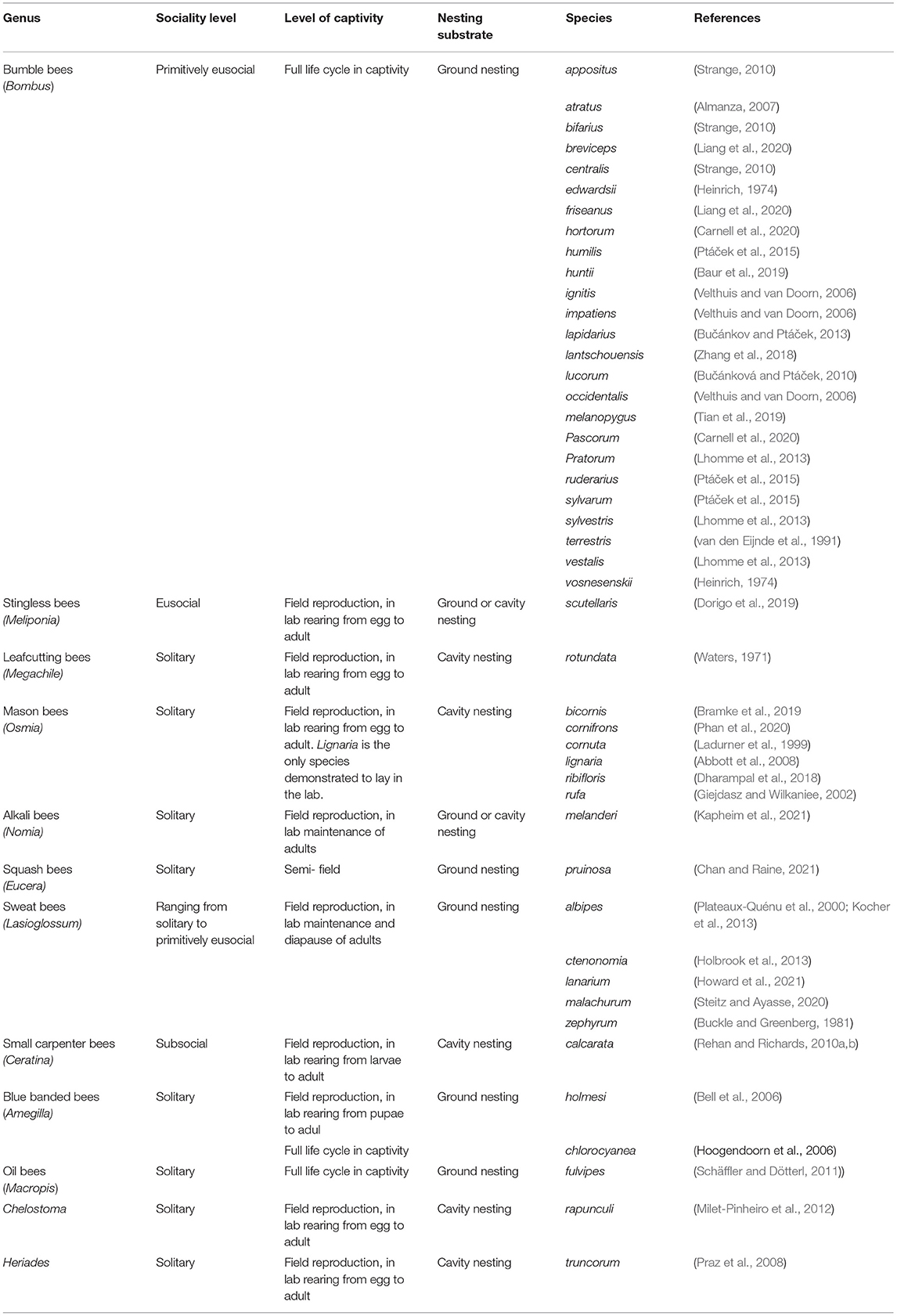
Table 1. List of wild bee species that have been successfully propagated in controlled lab or semi-field settings and have the potential to be used for controlled assays of foraging preferences and impacts of diets on health, development, and fitness.
Box 4. Benefits and constraints of conducting nutritional experiments with species that vary in their natural history.
Nesting habitat
Ground nesting—Ground nesting bees include eusocial, primitively eusocial, and solitary species. Bees may live in preexisting burrows, such as bumble bees., or may dig a narrow tunnel like Andrena sp. (Danforth, 2019). Some solitary species can be managed in the field with “bee beds,” or mounds of curated soil for a particular bee species to nest in, but little is known about the soil preferences of most ground nesting species (Lybrand et al., 2020). The difficulty with conducting experiments with ground nesting bees is that larvae are delicate and can be difficult to transfer from bee beds to a lab environment. Due to this constraint, only Nomia sp. are currently available for purchase, and researchers who would like to work with other species must find their own nesting aggregations to collect from.
Cavity nesting—Cavity nesting bees may use many different nesting materials, including hollow reeds or sticks, pre-existing cavities in trees, and even crevices in manmade structures, such as between bricks in buildings (Cane et al., 2006). These bees can be managed in the field by releasing females to nest in provided trap nests, or by trapping a local population. Eggs and immature larvae may be easily transferred to different diets and finish their life cycle in the lab. Though there are few cavity nesting species that are actively managed, it is possible to develop new trapping strategies by determining the preferred nesting substrate, shading, orientation of nest entrance (Martins et al., 2012), and the ideal diameter of the nesting cavity (Fye, 1965) to replicate these conditions and attract a species of interest.
Degree of sociality
Solitary—Solitary bee species work as individuals to provision their own offspring, and though they may live in gregarious populations, they do not work together to rear offspring. The benefit of working with solitary bees is that replication of maternal genetic diversity may be easily managed by collecting individual nesting tubes from source populations. Each adult female will provision separate nesting tubes at field sites, and though an adult female may produce more than one full tube in her lifetime, a researcher can be fairly confident that all reeds collected on the same day (if reeds are collected daily) are from different females (Danforth, 2019). Collecting reeds daily can also minimize usurpation by other bees, which could otherwise interfere with control for maternal genetic diversity. In contrast, eusocial bee colonies take up much more room in the lab and require a higher degree of management, so while genetic diversity can be managed, it can be much more costly and time consuming. Unfortunately, unlike eusocial species, protocols for managing entire life cycles of solitary bees in the lab have only been developed for a few genera, including Macropis and Amegilla. For most of these genera, adults may emerge from cocoons and mate in the lab but must be in a field or large semi-field setting to collect pollen and lay eggs (but, see Fortuin and Gandhi, 2021).
Subsocial—Subsocial species have overlapping generations and provide care to offspring throughout development. In some cases, foundresses may work with a dwarf eldest daughter (DED) to rear offspring (Rehan and Richards, 2010a,b). Though these DEDs do not have the ability to reproduce and exhibit more docile behavior than other offspring that share the nest, these species are not considered eusocial because they do not have an entire class of workers, and the nest established by the foundress is shared with other adult daughters that provision their own offspring (Lawson et al., 2017). This behavioral plasticity can impede nutritional experiments. If the nest has only a foundress and an adult dwarf daughter, a researcher can control for maternal genetic diversity, similarly to solitary bees. However, if a nest is shared by other reproductive daughters this is not possible. Another confounding factor of rearing these bees in the lab from egg to adult is that larvae will not experience maternal care, which may allow for transfer of microbes that are necessary to develop into healthy adult bees. Thus, lack of maternal care may impact nutritional needs or gene expression.
Ranging from primitively eusocial to solitary—These bees exhibit characteristics of both eusocial and solitary bees and can range on the continuum of sociality (Michener, 2007). Some species have populations that vary in eusociality due to behavioral plasticity, such as Halictus rubicundus, which change their degree of sociality based on how much time is left in the season to produce offspring (Field et al., 2012). Other species, such as Lasioglossum albipes, differ in social behavior due to genetic differences between populations (Kocher et al., 2018). This variance in behavior could lead to interesting research exploring differences in nutritional needs of reproductive and non-reproductive females, or perhaps differences in foraging behavior. However, managing these bees also comes with the constraint of having to standardize the degree of sociality in lab populations, as differences in eusociality could lead to differential gene expression and behavior, which may then impact nutritional needs.
Eusocial and primitively eusocial—Eusocial and primitively eusocial species have structured colonies where non-reproductive workers with overlapping generations care for the offspring of one reproductive queen (da Silva, 2021). Maintaining these bees in the lab for their entire life cycle is less difficult than maintaining solitary bees, and as adults maintain environmental cleanliness and feed brood. However, genera such as bumble bees present the difficulty of disentangling adult and larval nutritional needs. For example, when colonies rear brood they will adjust their macronutrient intake to suit brood nutritional needs rather than prioritizing their own (Kraus et al., 2019). Adults may be reared on diets separately from brood, but it can be difficult to rear brood without the presence of workers to provide constant care.
Emerging Methods: Molecular and Physiological Tools
Molecular methods may be used to measure bee development, health and reproduction, and may also provide insights into the mechanisms underlying the impact of nutritionally varied diets on bees. These methods can be used with any bee species (Grozinger and Zayed, 2020), but to our knowledge have thus far only been used to study the impacts of variation in pollen-based nutrition with honey bees (Crone and Grozinger, 2021) and bumble bees (Stabler et al., 2015). Expression levels of genes can be evaluated across the genome, using transcriptomics/RNA-seq, or for candidate genes using quantitative PCR. These studies can monitor changes in genes involved in detoxification pathways (Colgan et al., 2019), growth and development (Castelli et al., 2020), or immune function (Brunner et al., 2014; Tritschler et al., 2017). Gene expression may also be assessed when pairing diet with a stressor to understand how diet and stress interact to impact overall health. For example, Brunner et al. (2014) measured how different diets impacted bumble bee response to the gut parasite Crithidia bombi and found that lack of dietary protein impacted immune response, resulting in failure to produce antimicrobial peptides.
The lack of information currently available on wild bee gene expression patterns and genome characterization has likely been a limiting factor of using molecular and physiological methods in wild bee studies. These studies require that the genes underlying physiological processes are well-described, and thus a particular expression pattern is known to be associated with variation in levels of nutritional resources, detoxification, immunocompetence, or reproductive potential. While the function of some genes are well-conserved across insects (and arthropods in general), such as those involved in immune response, functional characterization is typically necessary to obtain baseline information about how genes in a particular species and at a particular developmental or physiological stage are responding to a given nutritional stressor (Palmer and Jiggins, 2015). Efforts are increasing to characterize genomes equally across insect taxa, and this will facilitate more in-depth studies concerning wild bee nutrition (Hotaling et al., 2021).
Rather than measuring expression of specific genes, it is also possible to measure levels of nutrient stores (proteins, lipids, and glycogen) in the bee's body, which can be measured through hemolymph (as a measure of readily available nutrients) (Stabler et al., 2015), or through body tissues (as a measure of long term nutrient storage) (Amsalem et al., 2015; Woodard et al., 2020). Measuring body nutrient levels can indicate the level of nutrient that was stored for use, and the excesses that may have been excreted (Lee, 2007).
Molecular methods may also be used to measure genetic diversity in bee populations by evaluating microsatellites, or non-coding regions of DNA that vary based on relatedness between individuals (Maebe et al., 2015). This technique would be useful when measuring a change in bee population numbers and diversity at field sites where supplemental forage is provided. Population dynamics can be measured within foraging distance of these resources and the surrounding areas to determine if bee populations are increasing due to more resources or if more bees are simply attracted to these enhanced habitats. Using this method with wild bee species would first require sequencing and development of microsatellite targets (see Khidr et al., 2014 for an example), so evaluating many bee species at once could be quite costly and time consuming.
Conclusions and Future Directions
Bee nutritional ecology has traditionally been investigated using three different approaches, which are (1) identification of floral visitors of selected focal plant species, (2) evaluation of the foraging preferences of selected focal bee species, and (3) evaluation of the nutritional requirements of focal bee species in controlled settings. New technologies are available within these different approaches to further the field, but development of new technologies is not enough to gain a holistic understanding of bee nutritional ecology. Complete understanding of the nutritional needs of a focal bee species and how these needs are met using pollinator habitat design requires an integrative, iterative process, in which the foraging preferences of a bee species are evaluated using field-based studies, followed by evaluation of preferred plants in a controlled setting (in the absence of competition with other pollinators and plants). Bees' health, reproduction, and fitness outcomes would then be evaluated on diets provided by these plants. Finally, these plants would be incorporated into field settings and evaluated to determine if floral preferences are maintained, and if these plants positively impact bee populations or communities.
An improved understanding of the nutritional needs of diverse pollinator taxa, and how these can be met by different plant species will allow land managers to optimize the design of planting schemes for pollinator communities. Plant communities can be designed with a specific end goal (e.g. improving the population of a particular species or improving diversity of a pollinator community), and selecting a group of plant species that would support these goals, and potentially support other ecosystem services (e.g., nitrogen fixation, water runoff management, pest management). Though native, pollinator-friendly planting lists are widely available, these lists do not take nutritional properties into account outside of pollen and nectar availability, and updated lists would need to be compiled by extension specialists (Planting Pollinator Friendly Gardens, 2017; Pollinator Partnership, 2022; Finding Pollinator Attractive Plants Protecting Bees, 2022).
In addition to integration of approaches, use of newer technologies will lead to an increase in the extent and scale of the questions that are able to be answered, including across landscapes, bee species, seasons, and years. As an example, we have discussed that one of the main limitations of automated insect identification through machine learning is that bees have very minute morphological differences that cannot be viewed from a camera alone. Høye et al. (2021) has suggested that automated bee identification may be combined with bee DNA metabarcoding to increase the taxonomic resolution of insects that visit camera observation sites. Use of both cameras and molecular techniques would allow for simultaneous screening of multiple sites, and fine-scale identification of sampled insects in a shorter time span than has previously been feasible. Therefore, these new technologies, with integration across approaches and taxa, have the potential to allow us to achieve a more comprehensive and holistic understanding of pollinator nutritional ecology than was possible before.
Author Contributions
MC and CG developed the conceptual framework for the manuscript. MC preformed the literature review and developed the initial manuscript drafts. MC and CG wrote the manuscript with input from DB. All authors approved of the final manuscript.
Funding
This material is based upon work supported by the National Science Foundation Graduate Research Fellowship Program under Grant No. DGE1255832 and research supported by funding from Wyman's of Maine to the Penn State Center for Pollinator Research. Any opinions, findings, and conclusions or recommendations expressed in this material are those of the authors and do not necessarily reflect the views of the National Science Foundation.
Conflict of Interest
The authors declare that the research was conducted in the absence of any commercial or financial relationships that could be construed as a potential conflict of interest.
Publisher's Note
All claims expressed in this article are solely those of the authors and do not necessarily represent those of their affiliated organizations, or those of the publisher, the editors and the reviewers. Any product that may be evaluated in this article, or claim that may be made by its manufacturer, is not guaranteed or endorsed by the publisher.
Acknowledgments
The authors would like to thank Gabriela Quinlan and other members of the Grozinger lab for critically reviewing the manuscript.
References
Finding Pollinator Attractive Plants Protecting Bees (2022). Available online at: https://protectingbees.njaes.rutgers.edu/find-plants/ (accessed Febraury 22, 2022).
Planting Pollinator Friendly Gardens (2017). Available online at: https://ento.psu.edu/research/centers/pollinators/public-outreach/cert/cert-steps-step1 (accessed February 22, 2022) Finding Pollinator Attractive Plants Protecting Bees (2022). Available online at: https://protectingbees.njaes.rutgers.edu/find-plants/. (Accessed: 2/22/2022)."
Abbott, V. A., Nadeau, J. L., Higo, H. A., and Winston, M. L. (2008). Lethal and sublethal effects of Imidacloprid on Osmia lignaria and Clothianidin on Megachile rotundata (Hymenoptera: Megachilidae). J. Econ. Entomol. 101, 784–796. doi: 10.1603/0022-0493(2008)101784:LASEOI2.0.CO;2
Adler, L. S., Barber, N. A., Biller, O. M., and Irwin, R. E. (2020). Flowering plant composition shapes pathogen infection intensity and reproduction in bumble bee colonies. Proc. Natl. Acad. Sci. U.S.A. 117, 11559–11565. doi: 10.1073/pnas.2000074117
Almanza, M. T. (2007). Management of Bombus atratus bumblebees to pollinate Lulo (Solanum quitoense), a native fruit from the Andes of Colombia. [Dissertation]. [Bonn, Germany]: ZEF Bonn.
Amsalem, E., Grozinger, C. M., Padilla, M., and Hefetz, A. (2015). The physiological and genomic bases of bumble bee social behaviour. Adv. Insect Physiol. 37–93. doi: 10.1016/bs.aiip.2015.01.001
Aperio ImageScope - Pathology Slide Viewing Software (2021). Available online at: https://www.leicabiosystems.com/us/digital-pathology/manage/aperio-imagescope/ (accessed February 22, 2022)
Arceo-Gómez, G., Barker, D., Stanley, A., Watson, T., and Daniels, J. (2020). Plant-pollinator network structural properties differentially affect pollen transfer dynamics and pollination success. Oecologia 192, 1037–1045. doi: 10.1007/s00442-020-04637-5
Arstingstall, K. A., DeBano, S. J., Li, X., Wooster, D. E., Rowland, M. M., Burrows, S., et al. (2021). Capabilities and limitations of using DNA metabarcoding to study plant-pollinator interactions. Mol. Ecol. 30, 5266–5297. doi: 10.1111/mec.16112
Arx, M., von, Goyret, J., Davidowitz, G., and Raguso, R. A. (2012). Floral humidity as a reliable sensory cue for profitability assessment by nectar-foraging hawkmoths. Proc. Natl. Acad. Sci. U.S.A. 109, 9471–9476. doi: 10.1073/pnas.1121624109
Austin, A. J., and Gilbert, J. D. J. (2021). Solitary bee larvae prioritize carbohydrate over protein in parentally provided pollen. Funct. Ecol. 35, 1069–1080. doi: 10.1111/1365-2435.13746
Baracchi, D. (2019). Cognitive ecology of pollinators and the main determinants of foraging plasticity. Curr. Zool. 65, 421–424. doi: 10.1093/cz/zoz036
Batovska, J., Piper, A. M., Valenzuela, I., Cunningham, J. P., and Blacket, M. J. (2021). Developing a non-destructive metabarcoding protocol for detection of pest insects in bulk trap catches. Sci. Rep. 11, 7946. doi: 10.1038/s41598-021-85855-6
Baum, K. A., and Wallen, K. E. (2011). Potential bias in pan trapping as a function of floral abundance. J. Kans. Entomol. Soc. 84, 155–159. doi: 10.2317/JKES100629.1
Baur, A., Strange, J. P., and Koch, J. B. (2019). Foraging economics of the hunt bumble bee, a viable pollinator for commercial agriculture. Environ. Entomol. 48, 799–806. doi: 10.1093/ee/nvz075
Belina-Aldemita Ma, D., Opper, C., Schreiner, M., and D'Amico, S. (2019). Nutritional composition of pot-pollen produced by stingless bees (Tetragonula biroi Friese) from the Philippines. J. Food Composit. Anal. 82, 103215. doi: 10.1016/j.jfca.2019.04.003
Bell, M. C., Spooner-hart, R. N., and Haigh, A. M. (2006). Pollination of greenhouse tomatoes by the Australian bluebanded bee amegilla (Zonamegilla) holmesi (Hymenoptera: Apidae). J. Econ. Entomol. 99, 437–442. doi: 10.1093/jee/99.2.437
Benjamin, F. E., and Winfree, R. (2014). Lack of pollinators limits fruit production in commercial blueberry (Vaccinium corymbosum). Environ. Entomol. 43, 1574–1583. doi: 10.1603/EN13314
Biddinger, D. J., Joshi, N. K., Rajotte, E. G., Halbrendt, N. O., Pulig, C., Naithani, K. J., et al. (2013b). An immunomarking method to determine the foraging patterns of Osmia cornifrons and resulting fruit set in a cherry orchard. Apidologie. 44, 738–49. doi: 10.1007/s13592-013-0221-x
Biddinger, D. J., Robertson, J. L., Mullin, C., Frazier, J., Ashcraft, S. A., Rajotte, E. G., et al. (2013a). Comparative toxicities and synergism of orchard pesticides to Apis mellifera (L.) and Osmia cornifrons (Radoszkowski). PLoS ONE 8, e72587. doi: 10.1371/journal.pone.0072587
Boff, S., Scheiner, R., Raizer, J., and Lupi, D. (2021). Survival rate and changes in foraging performances of solitary bees exposed to a novel insecticide. Ecotoxicol. Environ. Saf. 211, 111869. doi: 10.1016/j.ecoenv.2020.111869
Bradford, M. M. (1976). A rapid and sensitive method for the quantitation of microgram quantities of protein utilizing the principle of protein-dye binding. Anal. Biochem. 72, 248–254. doi: 10.1016/0003-2697(76)90527-3
Bramke, K., Müller, U., McMahon, D. P., and Rolff, J. (2019). Exposure of larvae of the solitary bee osmia bicornis to the honey bee pathogen Nosema ceranae affects life history. Insects 10, 380. doi: 10.3390/insects10110380
Brunner, F. S., Schmid-Hempel, P., and Barribeau, S. M. (2014). Protein-poor diet reduces host-specific immune gene expression in Bombus terrestris. Proc. R. Soc. B Biol. Sci. 281, 20140128. doi: 10.1098/rspb.2014.0128
Bučánkov,á, A., and Ptáček, V. (2013). A test of bombus terrestris cocoon and other common methods for nest initiation in B. lapidarius and B. hortorum. J. Apicult. Sci. 56, 37–47. doi: 10.2478/v10289-012-0022-x
Bučánková, A., and Ptáček, V. (2010). Experiences in rearing of Bombus lucorum L. (Hymenoptera:Apoidea) in captivity. Úroda 12, 621–624. Available online at: https://www.semanticscholar.org/paper/Experiences-in-rearing-of-Bombus-lucorum-L.-in-Bu%C4%8D%C3%A1nkov%C3%A1-Pt%C3%A1%C4%8Dek/d856f408a2125f0ed038db0c83c7c870047255e7 (accessed June 1, 2021).
Buckle, G. R., and Greenberg, L. (1981). Nestmate recognition in sweat bees (Lasioglossum zephyrum): does an individual recognize its own odour or only odours of its nestmates? Anim. Behav. 29, 802–809. doi: 10.1016/S0003-3472(81)80014-0
Burkle, L. A., and Runyon, J. B. (2019). Floral volatiles structure plant-pollinator interactions in a diverse community across the growing season. Funct. Ecol. 33, 2116–2129. doi: 10.1111/1365-2435.13424
Campos, M. G., Anjos, O., Chica, M., Campoy, P., Nozkova, J., Almaraz-Abarca, N., et al. (2021). Standard methods for pollen research. J. Apic. Res. 60, 1–109. doi: 10.1080/00218839.2021.1948240
Campos, M. G. R., Bogdanov, S., de Almeida-Muradian, L. B., Szczesna, T., Mancebo, Y., Frigerio, C., et al. (2008). Pollen composition and standardisation of analytical methods. J. Apic. Res. 47, 154–161. doi: 10.1080/00218839.2008.11101443
Cane, J., and Sipes, S. (2006). “Floral specialization by bees: analytical methodologies and a revised lexicon for oligolecty,” in Plant-Pollinator Interactions: From Specialization to Generalization, eds N. Waser and J. Ollerton (University of Chicago Press), 99–122.
Cane, J. H. (1987). Estimation of bee size using intertegular span (Apoidea). J. Kans. Entomol. Soc. 60, 145–147.
Cane, J. H. (2021). A brief review of monolecty in bees and benefits of a broadened definition. Apidologie 52, 17–22. doi: 10.1007/s13592-020-00785-y
Cane, J. H., Minckley, R. L., Kervin, L. J., Roulston, T. H., and Williams, N. M. (2006). Complex responses within a desert bee guild (Hymenoptera: Apiformes) to urban habitat fragmentation. Ecol. Appl. 16, 632–644. doi: 10.1890/1051-0761(2006)0160632:CRWADB2.0.CO;2
Cane, J. H., and Payne, J. A. (1993). Regional, annual, and seasonal variation in pollinator guilds: intrinsic traits of bees (Hymenoptera: Apoidea) underlie their patterns of abundance at Vaccinium ashei (Ericaceae). Ann. Entomol. Soc. Am. 86, 577–588. doi: 10.1093/aesa/86.5.577
Cao, N., Meyer, M., Thiele, L., and Saukh, O. (2020). “Automated pollen detection with an affordable technology,” in Proceedings of the 2020 International Conference on Embedded Wireless Systems and Networks EWSN '20 (Junction Publishing), 108–119.
Carnell, J. D., Page, S., Goulson, D., and Hughes, W. O. H. (2020). Trialling techniques for rearing long-tongued bumblebees under laboratory conditions. Apidologie 51, 254–266. doi: 10.1007/s13592-019-00707-7
Casanelles-Abella, J., Müller, S., Keller, A., Aleixo, C., Alós Orti, M., Chiron, F., et al. (2021). How wild bees find a way in European cities: pollen metabarcoding unravels multiple feeding strategies and their effects on distribution patterns in four wild bee species. J. Appl. Ecol. 59, 457–470. doi: 10.1111/1365-2664.14063
Castelli, L., Branchiccela, B., Garrido, M., Invernizzi, C., Porrini, M., Romero, H., et al. (2020). Impact of nutritional stress on honeybee gut microbiota, immunity, and Nosema ceranae infection. Microb. Ecol. 80, 908–919. doi: 10.1007/s00248-020-01538-1
Chakrabarti, P., Morré, J. T., Lucas, H. M., Maier, C. S., and Sagili, R. R. (2019). The omics approach to bee nutritional landscape. Metabolomics 15, 127. doi: 10.1007/s11306-019-1590-6
Cheng, T., Xu, C., Lei, L., Li, C., Zhang, Y., and Zhou, S. (2016). Barcoding the kingdom Plantae: new PCR primers for ITS regions of plants with improved universality and specificity. Mol. Ecol. Resour. 16, 138–149. doi: 10.1111/1755-0998.12438
Chittka, L., and Thomson, J. (Eds.). (2001). Cognitive Ecology of Pollination: Animal Behaviour and Floral Evolution. Cambridge: Cambridge University Press. doi: 10.1017/CBO9780511542268
Colgan, T. J., Fletcher, I. K., Arce, A. N., Gill, R. J., Ramos Rodrigues, A., Stolle, E., et al. (2019). Caste- and pesticide-specific effects of neonicotinoid pesticide exposure on gene expression in bumblebees. Mol. Ecol. 28, 1964–1974. doi: 10.1111/mec.15047
Crone, M. K., and Grozinger, C. M. (2021). Pollen protein and lipid content influence resilience to insecticides in honey bees (Apis mellifera). J. Exp. Biol. 224, jeb242040. doi: 10.1242/jeb.242040
Cullen, N., Xia, J., Wei, N., Kaczorowski, R., Arceo-Gómez, G., O'Neill, E., et al. (2021). Diversity and composition of pollen loads carried by pollinators are primarily driven by insect traits, not floral community characteristics. Oecologia 196, 131–143. doi: 10.1007/s00442-021-04911-0
da Silva, J. (2021). Life history and the transitions to eusociality in the hymenoptera. Front. Ecol. Evolut. 9, 727124. doi: 10.3389/fevo.2021.727124
Danforth, B. N. (2019). The Solitary Bees: Biology, Evolution, Conservation. 1st edn. Princeton, NJ: Princeton University Press. doi: 10.2307/j.ctvd1c929
Darby, B., Bryant, R., Keller, A., Jochim, M., Moe, J., Schreiner, Z., et al. (2020). Molecular sequencing and morphological identification reveal similar patterns in native bee communities across public and private grasslands of eastern North Dakota. PLoS ONE 15, e0227918. doi: 10.1371/journal.pone.0227918
Derraik, J. G. B., Early, J. W., Closs, G. P., and Dickinson, K. J. M. (2010). Morphospecies and taxonomic species comparison for hymenoptera. J. Insect Sci. 10, 108. doi: 10.1673/031.010.10801
Dharampal, P. S., Carlson, C. M., Diaz-Garcia, L., and Steffan, S. A. (2018). In vitro rearing of solitary bees: a tool for assessing larval risk factors. J. Vis. Exp. 57876. doi: 10.3791/57876
Donkersley, P. (2019). Trees for bees. Agric. Ecosyst. Environ. 270-271, 79–83. doi: 10.1016/j.agee.2018.10.024
Dorigo, A. S., Rosa-Fontana, A. S., Soares-Lima, H. M., Galaschi-Teixeira, J. S., Nocelli, R. C. F., and Malaspina, O. (2019). In vitro larval rearing protocol for the stingless bee species Melipona scutellaris for toxicological studies. PLoS ONE 14, e0213109. doi: 10.1371/journal.pone.0213109
Dormontt, E. E., van Dijk, K., Bell, K. L., Biffin, E., Breed, M. F., Byrne, M., et al. (2018). Advancing DNA barcoding and metabarcoding applications for plants requires systematic analysis of herbarium collections-an Australian perspective. Front. Ecol. Evolut. 6, 134. doi: 10.3389/fevo.2018.00134
Droege, S., Engler, J. D., Sellers, E., and O'Brien, L. E. (2017). U.S. National Protocol Framework for the Inventory and Monitoring of Bees, Version 2.0. Inventory and Monitoring, National Wildlife Refuge System. Fort Collins, CO: U.S. Fish and Wildlife Service.
Dukas, R., and Real, L. A. (1991). Learning foraging tasks by bees: a comparison between social and solitary species. Anim. Behav. 42, 269–276. doi: 10.1016/S0003-3472(05)80558-5
Eckhardt, M., Haider, M., Dorn, S., and Müller, A. (2014). Pollen mixing in pollen generalist solitary bees: a possible strategy to complement or mitigate unfavourable pollen properties? J. Anim. Ecol. 83, 588–597. doi: 10.1111/1365-2656.12168
Eilers, E. J., Kremen, C., Greenleaf, S. S., Garber, A. K., and Klein, A.-M. (2011). Contribution of pollinator-mediated crops to nutrients in the human food supply. PLoS ONE 6, e21363. doi: 10.1371/journal.pone.0021363
Engel, M. S., Ceríaco, L. M. P., Daniel, G. M., Dellapé, P. M., Löbl, I., Marinov, M., et al. (2021). The taxonomic impediment: a shortage of taxonomists, not the lack of technical approaches. Zool. J. Linn. Soc. 193, 381–387. doi: 10.1093/zoolinnean/zlab072
Erickson, E., Adam, S., Russo, L., Wojcik, V., Patch, H. M., and Grozinger, C. M. (2020). More than meets the eye? The role of annual ornamental flowers in supporting pollinators. Environ. Entomol. 49, 178–188. doi: 10.1093/ee/nvz133
Erickson, E., Junker, R. R., McCartney, N., Ali, J. G., Patch, H. M., and Grozinger, C. M. (2022). Phenotypic variation between cultivars of ornamental flowers shapes pollinator attraction.
Erickson, E., Patch, H. M., and Grozinger, C. M. (2021). Herbaceous perennial ornamental plants can support complex pollinator communities. Sci. Rep. 11, 17352. doi: 10.1038/s41598-021-95892-w
Essenberg, C. J., Kalisz, A. E. S., and McPeek, E. M. A. (2012). Explaining variation in the effect of floral density on pollinator visitation. Am. Nat. 180, 153–166. doi: 10.1086/666610
Estravis-Barcala, M. C., Palottini, F., Macri, I., Nery, D., and Farina, W. M. (2021). Managed honeybees and South American bumblebees exhibit complementary foraging patterns in highbush blueberry. Sci. Rep. 11, 8187. doi: 10.1038/s41598-021-87729-3
Field, J., Paxton, R., Soro, A., Craze, P., and Bridge, C. (2012). Body size, demography and foraging in a socially plastic sweat bee: a common garden experiment. Behav. Ecol. Sociobiol. 66, 743–756. doi: 10.1007/s00265-012-1322-7
Filipiak, M., Kuszewska, K., Asselman, M., Denisow, B., Stawiarz, E., Woyciechowski, M., et al. (2017). Ecological stoichiometry of the honeybee: pollen diversity and adequate species composition are needed to mitigate limitations imposed on the growth and development of bees by pollen quality. PLoS ONE 12, e0183236. doi: 10.1371/journal.pone.0183236
Filipiak, Z. M., and Filipiak, M. (2020). The scarcity of specific nutrients in wild bee larval food negatively influences certain life history traits. Biology 9, 462. doi: 10.3390/biology9120462
Fischman, B. J., Pitts-Singer, T. L., and Robinson, G. E. (2017). Nutritional regulation of phenotypic plasticity in a solitary bee (Hymenoptera: Megachilidae). Environ. Entomol. 46, 1070–1079. doi: 10.1093/ee/nvx119
Fortuin, C. C., and Gandhi, K. J. K. (2021). Mason bees (Hymenoptera: Megachilidae) exhibit no avoidance of imidacloprid-treated soils. Environ. Entomol. 50, 1438–1445. doi: 10.1093/ee/nvab083
Fortuin, C. C., McCarty, E., and Gandhi, K. J. K. (2021). Acute contact with imidacloprid in soil affects the nesting and survival success of a solitary wild bee, Osmia lignaria (Hymenoptera: Megachilidae). Chemosphere 264, 128572. doi: 10.1016/j.chemosphere.2020.128572
Fründ, J., Linsenmair, K. E., and Blüthgen, N. (2010). Pollinator diversity and specialization in relation to flower diversity. Oikos 119, 1581–1590. doi: 10.1111/j.1600-0706.2010.18450.x
Fye, R. (1965). Biology of apoidea taken in trap nests in northwestern Ontario (Hymenoptera). Can. Entomol. 97, 863–877. doi: 10.4039/Ent97863-8
Garibaldi, L. A., Sáez, A., Aizen, M. A., Fijen, T., and Bartomeus, I. (2020). Crop pollination management needs flower-visitor monitoring and target values. J. Appl. Ecol. 57, 664–670. doi: 10.1111/1365-2664.13574
Gernat, T., Rao, V. D., Middendorf, M., Dankowicz, H., Goldenfeld, N., and Robinson, G. E. (2018). Automated monitoring of behavior reveals bursty interaction patterns and rapid spreading dynamics in honeybee social networks. Proc. Natl. Acad. Sci. U. S. A. 115, 1433–1438. doi: 10.1073/pnas.1713568115
Ghosh, S., Jeon, H., and Jung, C. (2020). Foraging behaviour and preference of pollen sources by honey bee (Apis mellifera) relative to protein contents. J. Ecol. Environ. 44, 4. doi: 10.1186/s41610-020-0149-9
Gibbs, J., Elle, E., Bobiwash, K., Haapalainen, T., and Isaacs, R. (2016). Contrasting pollinators and pollination in native and non-native regions of highbush blueberry production. PLoS ONE 11, e0158937. doi: 10.1371/journal.pone.0158937
Gibbs, J., Joshi, N. K., Wilson, J. K., Rothwell, N. L., Powers, K., Haas, M., et al. (2017). Does passive sampling accurately reflect the bee (Apoidea: Anthophila) Communities pollinating apple and sour cherry orchards? Environ. Entomol. 46, 579–588. doi: 10.1093/ee/nvx069
Giejdasz, K., and Wilkaniee, Z. (2002). Individual development of the red mason bee (Osmia rufa L., Megachilidae) under natural and laboratory conditions. J. Apicult. Sci. 46, 51–56. Available online at: http://www.jas.org.pl:81/pdf/42?filename=jas6_1_2002.pdf (accessed June 1, 2021).
Giri, S., Giri, B., and Dillon, M. E. (2019). An optimized approach for extraction and quantification of energy reserves in differentially fed bumble bees (Bombus). J. Apic. Res. 58, 531–541. doi: 10.1080/00218839.2019.1614728
Goodwin, E. K., Rader, R., Encinas-Viso, F., and Saunders, M. E. (2021). Weather conditions affect the visitation frequency, richness and detectability of insect flower visitors in the Australian alpine zone. Environ. Entomol. 50, 348–358. doi: 10.1093/ee/nvaa180
Gostel, M. R., Zúñiga, J. D., Kress, W. J., Funk, V. A., and Puente-Lelievre, C. (2020). Microfluidic Enrichment Barcoding (MEBarcoding): a new method for high throughput plant DNA barcoding. Sci. Rep. 10, 8701. doi: 10.1038/s41598-020-64919-z
Gous, A., Swanevelder, D. Z. H., Eardley, C. D., and Willows-Munro, S. (2019). Plant-pollinator interactions over time: pollen metabarcoding from bees in a historic collection. Evol. Appl. 12, 187–197. doi: 10.1111/eva.12707
Grab, H., Blitzer, E. J., Danforth, B., Loeb, G., and Poveda, K. (2017). Temporally dependent pollinator competition and facilitation with mass flowering crops affects yield in co-blooming crops. Sci. Rep. 7, 45296. doi: 10.1038/srep45296
Grab, H., Brokaw, J., Anderson, E., Gedlinske, L., Gibbs, J., Wilson, J., et al. (2019). Habitat enhancements rescue bee body size from the negative effects of landscape simplification. J. Appl. Ecol. 56, 2144–2154. doi: 10.1111/1365-2664.13456
Green, M. R., Sambrook, J., and Sambrook, J. (2012). Molecular Cloning: A Laboratory Manual. 4th ed. Cold Spring Harbor, NY: Cold Spring Harbor Laboratory Press.
Greenleaf, S. S., Williams, N. M., Winfree, R., and Kremen, C. (2007). Bee foraging ranges and their relationship to body size. Oecologia 153, 589–596. doi: 10.1007/s00442-007-0752-9
Greenwald, E., Segre, E., and Feinerman, O. (2015). Ant trophallactic networks: simultaneous measurement of interaction patterns and food dissemination. Sci. Rep. 5, 12496. doi: 10.1038/srep12496
Grozinger, C. M., and Zayed, A. (2020). Improving bee health through genomics. Nat. Rev. Genet. 21, 277–291. doi: 10.1038/s41576-020-0216-1
Halbritter, H., Ulrich, S., Grímsson, F., Weber, M., Zetter, R., Hesse, M., et al. (2018). “Methods in palynology,” in Illustrated Pollen Terminology (Cham: Springer Nature), 97–127. doi: 10.1007/978-3-319-71365-6_6
Hale, H., Gardner, E. M., Viruel, J., Pokorny, L., and Johnson, M. G. (2020). Strategies for reducing per-sample costs in target capture sequencing for phylogenomics and population genomics in plants. Appl. Plant Sci. 8, e11337. doi: 10.1002/aps3.11337
Harmon-Threatt, A. (2020). Influence of nesting characteristics on health of wild bee communities. Annu. Rev. Entomol. 65, 39–56. doi: 10.1146/annurev-ento-011019-024955
Heinrich, B. (1974). Pheromone induced brooding behavior in Bombus vosnesenskii and B. edwardsii (Hymenoptera: Bombidae). J. Kans. Entomol. Soc. 47, 396–404.
Heller, S., Joshi, N. K., Leslie, T., Rajotte, E. G., and Biddinger, D. J. (2019). Diversified floral resource plantings support bee communities after apple bloom in commercial orchards. Sci. Rep. 9, 17232. doi: 10.1038/s41598-019-52601-y
Hendriksma, H. P., Pachow, C. D., and Nieh, J. C. (2019b). Effects of essential amino acid supplementation to promote honey bee gland and muscle development in cages and colonies. J. Insect Physiol. 117, 103906. doi: 10.1016/j.jinsphys.2019.103906
Hendriksma, H. P., and Shafir, S. (2016). Honey bee foragers balance colony nutritional deficiencies. Behav. Ecol. Sociobiol. 70, 509–517. doi: 10.1007/s00265-016-2067-5
Hendriksma, H. P., Toth, A. L., and Shafir, S. (2019a). Individual and colony level foraging decisions of bumble bees and honey bees in relation to balancing of nutrient needs. Front. Ecol. Evolut. 7, 177. doi: 10.3389/fevo.2019.00177
Hoffman, G. D., Lande, C., and Rao, S. (2018). A novel pollen transfer mechanism by honey bee foragers on highbush blueberry (Ericales: Ericaceae). Environ. Entomol. 47, 1465–1470. doi: 10.1093/ee/nvy162
Hogendoorn, K., Gross, C. L., Sedgley, M., and Keller, M. A. (2006). Increased Tomato Yield Through Pollination by Native Australian Amegilla chlorocyanea (Hymenoptera: Anthophoridae). J. Econ Entomol. 99, 828–833. doi: 10.1093/jee/99.3.828.
Holbrook, C. T., Kukuk, P. F., and Fewell, J. H. (2013). Increased group size promotes task specialization in a normally solitary halictine bee. Behaviour 150, 1449–1466. doi: 10.1163/1568539X-00003104
Holzschuh, A., Dormann, C. F., Tscharntke, T., and Steffan-Dewenter, I. (2011). Expansion of mass-flowering crops leads to transient pollinator dilution and reduced wild plant pollination. Proc. R. Soc. B Biol. Sci. 278, 3444–3451. doi: 10.1098/rspb.2011.0268
Hotaling, S., Sproul, J. S., Heckenhauer, J., Powell, A., Larracuente, A. M., Pauls, S. U., et al. (2021). Long reads are revolutionizing 20 years of insect genome sequencing. Genome Biol. Evolut. 13, evab138. doi: 10.1093/gbe/evab138
Howard, S. R., Prendergast, K., Symonds, M. R. E., Shrestha, M., and Dyer, A. G. (2021). Spontaneous choices for insect-pollinated flower shapes by wild non-eusocial halictid bees. J. Exp. Biol. 224, jeb242457. doi: 10.1242/jeb.242457
Høye, T. T., Ärje, J., Bjerge, K., Hansen, O. L. P., Iosifidis, A., Leese, F., et al. (2021). Deep learning and computer vision will transform entomology. Proc. Natl. Acad. Sci. U.S.A. 118, e2002545117. doi: 10.1073/pnas.2002545117
Hubert, N., and Hanner, R. (2015). DNA Barcoding, species delineation and taxonomy: a historical perspective. DNA Barcodes 3, 44–58. doi: 10.1515/dna-2015-0006
Isaacs, R., Williams, N., Ellis, J., Pitts-Singer, T. L., Bommarco, R., and Vaughan, M. (2017). Integrated crop pollination: combining strategies to ensure stable and sustainable yields of pollination-dependent crops. Basic Appl. Ecol. 22, 44–60. doi: 10.1016/j.baae.2017.07.003
Jackson, F. L. C. (1991). Secondary compounds in plants (Allelochemicals) as promoters of human biological variability. Annu. Rev. Anthropol. 20, 505–544. doi: 10.1146/annurev.an.20.100191.002445
Jamieson, M. A., Carper, A. L., Wilson, C. J., Scott, V. L., and Gibbs, J. (2019). Geographic biases in bee research limits understanding of species distribution and response to anthropogenic disturbance. Front. Ecol. Evolut. 7, 194. doi: 10.3389/fevo.2019.00194
Johnson, M. G., Pokorny, L., Dodsworth, S., Botigu,é, L. R., Cowan, R. S., Devault, A., et al. (2019). A universal probe set for targeted sequencing of 353 nuclear genes from any flowering plant designed using k-Medoids clustering. Syst. Biol. 68, 594–606. doi: 10.1093/sysbio/syy086
Jones, B. M., Rao, V. D., Gernat, T., Jagla, T., Cash-Ahmed, A. C., Rubin, B. E., et al. (2020). Individual differences in honey bee behavior enabled by plasticity in brain gene regulatory networks. Elife 9, e62850. doi: 10.7554/eLife.62850.sa2
Jones, G. (2014). Pollen analyses for pollination research, acetolysis. J. Pollinat. Ecol. 13, 203–217. doi: 10.26786/1920-7603(2014)19
Jones, G. D. (2012). Pollen extraction from insects. Paly 36, 86–109. doi: 10.1080/01916122.2011.629523
Jordan, A., Patch, H. M., Grozinger, C. M., and Khanna, V. (2021). Economic dependence and vulnerability of United States agricultural sector on insect-mediated pollination service. Environ. Sci. Technol. 55, 2243–2253. doi: 10.1021/acs.est.0c04786
Joshi, N. K., Leslie, T., Rajotte, E. G., Kammerer, M. A., Otieno, M., and Biddinger, D. J. (2015). Comparative trapping efficiency to characterize bee abundance, diversity, and community composition in apple orchards. Ann. Entomol. Soc. Am. 108, 785–799. doi: 10.1093/aesa/sav057
Kadlec, M., Bellstedt, D. U., Le Maitre, N. C., and Pirie, M. D. (2017). Targeted NGS for species level phylogenomics: “made to measure” or “one size fits all”? PeerJ 5, e3569. doi: 10.7717/peerj.3569
Kammerer, M. A., Biddinger, D. J., Rajotte, E. G., and Mortensen, D. A. (2016). Local plant diversity across multiple habitats supports a diverse wild bee community in pennsylvania apple orchards. Environ. Entomol. 45, 32–38. doi: 10.1093/ee/nvv147
Kapheim, K. M., and Johnson, M. M. (2017). Juvenile hormone, but not nutrition or social cues, affects reproductive maturation in solitary alkali bees (Nomia melanderi). J. Exp. Biol. 220, 3794–3801. doi: 10.1242/jeb.162255
Kapheim, K. M., Johnson, M. M., and Jolley, M. (2021). Composition and acquisition of the microbiome in solitary, ground-nesting alkali bees. Sci. Rep. 11, 2993. doi: 10.1038/s41598-021-82573-x
Kendall, L. K., Evans, L. J., Gee, M., Smith, T. J., Gagic, V., Lobaton, J. D., et al. (2021). The effect of protective covers on pollinator health and pollination service delivery. Agric. Ecosyst. Environ. 319, 107556. doi: 10.1016/j.agee.2021.107556
Khidr, S. K., Hardy, I. C. W., Zaviezo, T., and Mayes, S. (2014). Development of microsatellite markers and detection of genetic variation between Goniozus wasp populations. J. Insect Sci. 14, 43. doi: 10.1093/jis/14.1.43
Kilpatrick, S. K., Gibbs, J., Mikulas, M. M., Spichiger, S.-E., Ostiguy, N., Biddinger, D. J., et al. (2020). An updated checklist of the bees (Hymenoptera, Apoidea, Anthophila) of Pennsylvania, United States of America. J. Hymenopt. Res. 77, 1–86. doi: 10.3897/jhr.77.49622
Kleijn, D., Rundlöf, M., Scheper, J., Smith, H. G., and Tscharntke, T. (2011). Does conservation on farmland contribute to halting the biodiversity decline? Trends Ecol. Evol. 26, 474–481. doi: 10.1016/j.tree.2011.05.009
Klein, A.-M., Vaissière, B. E., Cane, J. H., Steffan-Dewenter, I., Cunningham, S. A., Kremen, C., et al. (2007). Importance of pollinators in changing landscapes for world crops. Proc. R. Soc. B Biol. Sci. 274, 303–313. doi: 10.1098/rspb.2006.3721
Klein, S., Pasquaretta, C., Barron, A. B., Devaud, J.-M., and Lihoreau, M. (2017). Inter-individual variability in the foraging behaviour of traplining bumblebees. Sci. Rep. 7, 4561. doi: 10.1038/s41598-017-04919-8
Klomberg, Y., Tropek, R., Mertens, J. E. J., Kobe, I. N., Hodeček, J., Raška, J., et al. (2022). Spatiotemporal variation in the role of floral traits in shaping tropical plant-pollinator interactions. Ecol. Lett. 1–12. doi: 10.1111/ele.13958
Knapp, J. L., Shaw, R. F., and Osborne, J. L. (2019). Pollinator visitation to mass-flowering courgette and co-flowering wild flowers: implications for pollination and bee conservation on farms. Basic Appl. Ecol. 34, 85–94. doi: 10.1016/j.baae.2018.09.003
Kocher, S. D., Li, C., Yang, W., Tan, H., Yi, S. V., Yang, X., et al. (2013). The draft genome of a socially polymorphic halictid bee, Lasioglossum albipes. Genome Biol. 14, R142. doi: 10.1186/gb-2013-14-12-r142
Kocher, S. D., Mallarino, R., Rubin, B. E. R., Yu, D. W., Hoekstra, H. E., and Pierce, N. E. (2018). The genetic basis of a social polymorphism in halictid bees. Nat. Commun. 9, 4338. doi: 10.1038/s41467-018-06824-8
Kolter, A., and Gemeinholzer, B. (2021). Plant DNA barcoding necessitates marker-specific efforts to establish more comprehensive reference databases. Genome 64, 265–298. doi: 10.1139/gen-2019-0198
Kostić, A. Ž., Kaluderović, L. M., Dojčinović, B. P., Barać, M. B., Babić, V. B., and Mačukanović-Jocić, M. P. (2017). Preliminary investigation of mineral content of pollen collected from different Serbian maize hybrids - is there any potential nutritional value? J. Sci. Food Agric. 97, 2803–2809. doi: 10.1002/jsfa.8108
Kostić, A. Ž., Pešić, M. B., Mosić, M. D., Dojčinović, B. P., Natić, M. M., and Trifković, J. łD. (2015). Mineral content of bee pollen from Serbia / SadrŽaj minerala u uzorcima pčelinjega peluda iz Srbije. Arch. Indust. Hygiene Toxicol. 66, 251–258. doi: 10.1515/aiht-2015-66-2630
Kraemer, M. E., and Favi, F. D. (2005). Flower phenology and pollen choice of Osmia lignaria (Hymenoptera: Megachilidae) in central Virginia. Environ. Entomol. 34, 1593–1605. doi: 10.1603/0046-225X-34.6.1593
Kraus, S., Gómez-Moracho, T., Pasquaretta, C., Latil, G., Dussutour, A., and Lihoreau, M. (2019). Bumblebees adjust protein and lipid collection rules to the presence of brood. Curr. Zool. 65, 437–446. doi: 10.1093/cz/zoz026
Kuhlman, M. P., Burrows, S., Mummey, D. L., Ramsey, P. W., and Hahn, P. G. (2021). Relative bee abundance varies by collection method and flowering richness: implications for understanding patterns in bee community data. Ecol. Solut. Evidence 2, e12071. doi: 10.1002/2688-8319.12071
Ladurner, E., Maccagnani, B., Tesoriero, D., Nepi, M., and Feliciolo, A. (1999). Laboratory rearing of Osmia cornuta Latreille (Hymenoptera Megachilidae) on artificial diet. Bollettino dell'Istituto di Entomologia della Università di Bologna 53, 133–146.
Larkin, L. L., Neff, J. L., and Simpson, B. B. (2008). The evolution of a pollen diet: host choice and diet breadth of Andrena bees (Hymenoptera: Andrenidae). Apidologie 39:133–145. doi: 10.1051/apido:2007064
Lau, P., Bryant, V., Ellis, J. D., Huang, Z. Y., Sullivan, J., Schmehl, D. R., et al. (2019). Seasonal variation of pollen collected by honey bees (Apis mellifera) in developed areas across four regions in the United States. PLoS ONE 14, e0217294. doi: 10.1371/journal.pone.0217294
Lawson, S. P., Helmreich, S. L., and Rehan, S. M. (2017). Effects of nutritional deprivation on development and behavior in the subsocial bee Ceratina calcarata (Hymenoptera: Xylocopinae). J. Exp. Biol. 220, 4456–4462. doi: 10.1242/jeb.160531
Lawson, S. P., Kennedy, K. B., and Rehan, S. M. (2020). Pollen composition significantly impacts the development and survival of the native small carpenter bee, Ceratina calcarata. Ecol. Entomol. 46, 232–239. doi: 10.1111/een.12955
Leach, M. E., and Drummond, F. (2018). A review of native wild bee nutritional health. Int. J. Ecol. 2018:9607246. doi: 10.1155/2018/9607246
Lee, K. P. (2007). The interactive effects of protein quality and macronutrient imbalance on nutrient balancing in an insect herbivore. J. Exp. Biol. 210, 3236–3244. doi: 10.1242/jeb.008060
Leidenfrost, R. M., Bänsch, S., Prudnikow, L., Brenig, B., Westphal, C., and Wünschiers, R. (2020). Analyzing the dietary diary of bumble bee. Front. Plant Sci. 11, 287. doi: 10.3389/fpls.2020.00287
Leonard, R. J., and Harmon-Threatt, A. N. (2019). Methods for rearing ground-nesting bees under laboratory conditions. Apidologie 50, 689–703. doi: 10.1007/s13592-019-00679-8
Leonhardt, S. D., and Blüthgen, N. (2012). The same, but different: pollen foraging in honeybee and bumblebee colonies. Apidologie 43, 449–464. doi: 10.1007/s13592-011-0112-y
Lewin, H. A., Robinson, G. E., Kress, W. J., Baker, W. J., Coddington, J., Crandall, K. A., et al. (2018). Earth biogenome project: sequencing life for the future of life. Proc. Natl. Acad. Sci. U.S.A. 115, 4325–4333. doi: 10.1073/pnas.1720115115
Lhomme, P., Sramkova, A., Kreuter, K., Lecocq, T., Rasmont, P., and Ayasse, M. (2013). A method for year-round rearing of cuckoo bumblebees (Hymenoptera: Apoidea: Bombus subgenus Psithyrus). Annales de la Société entomologique de France 49, 117–125. doi: 10.1080/00379271.2013.774949
Liang, C., Ding, G., Huang, J., Zhang, X., Miao, C., and An, J. (2020). Characteristics of the two asian bumblebee species Bombus friseanus and Bombus breviceps (Hymenoptera: Apidae). Insects 11, 163. doi: 10.3390/insects11030163
Lihoreau, M., Raine, N. E., Reynolds, A. M., Stelzer, R. J., Lim, K. S., Smith, A. D., et al. (2012). Radar tracking and motion-sensitive cameras on flowers reveal the development of pollinator multi-destination routes over large spatial scales. PLoS Biol. 10, e1001392. doi: 10.1371/journal.pbio.1001392
Lundin, O., Ward, K. L., Artz, D. R., Boyle, N. K., Pitts-Singer, T. L., and Williams, N. M. (2017). Wildflower plantings do not compete with neighboring almond orchards for pollinator visits. Environ. Entomol. 46, 559–564. doi: 10.1093/ee/nvx052
Lybrand, R. A., Fedenko, J., Tfaily, M., and Rao, S. (2020). Soil properties and biochemical composition of ground-dwelling bee nests in agricultural settings. Soil Sci. Soc. Am. J. 84, 1139–1152. doi: 10.1002/saj2.20085
Mackert, M. (2019). Strategies to improve native bee (Hymenoptera: Apoidea) habitat in agroecosystems. Masters thesis, University of Illinois. Available online at: https://dr.lib.iastate.edu/entities/publication/c935336d-9c78-451c-b3f6-594ea263c78f (accessed June 1, 2021).
Maebe, K., Meeus, I., Ganne, M., Meulemeester, T. D., Biesmeijer, K., and Smagghe, G. (2015). Microsatellite analysis of museum specimens reveals historical differences in genetic diversity between declining and more stable bombus species. PLoS ONE 10, e0127870. doi: 10.1371/journal.pone.0127870
Manjon, C., Troczka, B. J., Zaworra, M., Beadle, K., Randall, E., Hertlein, G., et al. (2018). Unravelling the molecular determinants of bee sensitivity to neonicotinoid insecticides. Curr. Biol. 28, 1137–1143.e5. doi: 10.1016/j.cub.2018.02.045
Mărgăoan, R., Mărghita,ş, L. A., Dezmirean, D. S., Dulf, F. V., Bunea, A., Socaci, S. A., et al. (2014). Predominant and secondary pollen botanical origins influence the carotenoid and fatty acid profile in fresh honeybee-collected pollen. J. Agric. Food Chem. 62, 6306–6316. doi: 10.1021/jf5020318
Marten-Rodriguez, S., and Fenster, C. B. (2008). Pollination ecology and breeding systems of five gesneria species from puerto rico. Ann. Bot. 102, 23–30. doi: 10.1093/aob/mcn056
Martin, A. C., and Harvey, W. J. (2017). The Global Pollen Project: a new tool for pollen identification and the dissemination of physical reference collections. Methods Ecol. Evolut. 8, 892–897. doi: 10.1111/2041-210X.12752
Martins, C. F., Ferreira, R. P., and Carneiro, L. T. (2012). Influence of the orientation of nest entrance shading, and substrate on sampling trap-nesting bees and wasps. Neotrop. Entomol. 41, 105–111. doi: 10.1007/s13744-012-0020-5
McLaughlin, R., Keller, J., Wagner, E., Biddinger, D., and Grozinger, C, Hoover, K. (2021). Insect visitors of black cherry (Prunus serotina) (Rosales: Rosaceae) and factors affecting viable seed production. Environ. Entomol. nvab141. doi: 10.1093/ee/nvab141
M'Gonigle, L. K., Williams, N. M., Lonsdorf, E., and Kremen, C. (2016). A tool for selecting plants when restoring habitat for pollinators. Conserv. Lett. 10, 105–111. doi: 10.1111/conl.12261
Michener, C. D. (2007). The Bees of the World. 2nd ed. Baltimore, MD: Johns Hopkins University Press.
Milet-Pinheiro, P., Ayasse, M., Schlindwein, C., Dobson, H. E. M., and Dötterl, S. (2012). Host location by visual and olfactory floral cues in an oligolectic bee: innate and learned behavior. Behav. Ecol. 23, 531–538. doi: 10.1093/beheco/arr219
Milla, L., Sniderman, K., Lines, R., Mousavi-Derazmahalleh, M., and Encinas-Viso, F. (2021). Pollen DNA metabarcoding identifies regional provenance and high plant diversity in Australian honey. Ecol. Evol. 11, 8683–8698. doi: 10.1002/ece3.7679
Montgomery, G. A., Belitz, M. W., Guralnick, R. P., and Tingley, M. W. (2021). Standards and best practices for monitoring and benchmarking insects. Front. Ecol. Evolut. 8, 579193. doi: 10.3389/fevo.2020.579193
Mora, B., Shin, E., Cara Donna, P. J., and Stouffer, D. B. (2020). Untangling the seasonal dynamics of plant-pollinator communities. Nat. Commun. 11, 4086. doi: 10.1038/s41467-020-17894-y
Müller, A., Diener, S., Schnyder, S., Stutz, K., Sedivy, C., and Dorn, S. (2006). Quantitative pollen requirements of solitary bees: Implications for bee conservation and the evolution of bee-flower relationships. Biol. Conserv. 130, 604–615. doi: 10.1016/j.biocon.2006.01.023
Muth, F., Francis, J. S., and Leonard, A. S. (2016). Bees use the taste of pollen to determine which flowers to visit. Biol. Lett. 12, 20160356. doi: 10.1098/rsbl.2016.0356
Ne'eman, G., Shavit, O., Shaltiel, L., and Shmida, A. (2006). Foraging by male and female solitary bees with implications for pollination. J. Insect Behav. 19, 383. doi: 10.1007/s10905-006-9030-7
Neumüller, U., Burger, H., Schwenninger, H. R., Hopfenmüller, S., Krausch, S., Wei,ß, K., et al. (2021). Prolonged blooming season of flower plantings increases wild bee abundance and richness in agricultural landscapes. Biodivers. Conserv. 30, 3003–3021. doi: 10.1007/s10531-021-02233-4
Nicholls, E., and Hempel de Ibarra, N. (2017). Assessment of pollen rewards by foraging bees. Funct. Ecol. 31, 76–87. doi: 10.1111/1365-2435.12778
Nicolson, S. W. (2011). Bee food: the chemistry and nutritional value of nectar, pollen and mixtures of the two. Af. Zool. 46, 197–204. doi: 10.1080/15627020.2011.11407495
Nicolson, S. W., and Human, H. (2013). Chemical composition of the 'low quality' pollen of sunflower (Helianthus annuus, Asteraceae). Apidologie 44, 144–152. doi: 10.1007/s13592-012-0166-5
Ohashi, K., and Thomson, J. D. (2009). Trapline foraging by pollinators: its ontogeny, economics and possible consequences for plants. Ann. Bot. 103, 1365–1378. doi: 10.1093/aob/mcp088
O'Neill, R., and O'Neill, K. (2011). Pollen load composition and size in the leafcutting bee Megachile rotundata (Hymenoptera: Megachilidae). Apidologie 42, 223–233. doi: 10.1051/apido/2010057
Palmer, W. J., and Jiggins, F. M. (2015). Comparative genomics reveals the origins and diversity of arthropod immune systems. Mol. Biol. Evol. 32, 2111–2129. doi: 10.1093/molbev/msv093
Pegoraro, L., Hidalgo, O., Leitch, I. J., Pellicer, J., and Barlow, S. E. (2020). Automated video monitoring of insect pollinators in the field. Emerg. Topics Life Sci. 4, 87–97. doi: 10.1042/ETLS20190074
Phan, N. T., Joshi, N. K., Rajotte, E. G., López-Uribe, M. M., Zhu, F., and Biddinger, D. J. (2020). A new ingestion bioassay protocol for assessing pesticide toxicity to the adult Japanese orchard bee (Osmia cornifrons). Sci. Rep. 10, 9517. doi: 10.1038/s41598-020-66118-2
Pimm, S. L., and Joppa, L. N. (2015). How many plant species are there, where are they, and at what rate are they going extinct? mobt 100, 170–176. doi: 10.3417/2012018
Pinilla-Gallego, M. S., Crum, J., Schaetzl, R., and Issacs, R. (2018). Soil textures of nest partitions made by the mason bees Osmia lignaria and O. cornifrons (Hymenoptera: Megachilidae). Apidologie 49, 464–472. doi: 10.1007/s13592-018-0574-2
Pinilla-Gallego, M. S., and Isaacs, R. (2018). Pollen use by Osmia lignaria (Hymenoptera: Megachilidae) in highbush blueberry fields. Ann. Entomol. Soc. Am. 111, 335–340. doi: 10.1093/aesa/say028
Plateaux-Quénu, C., Plateaux, L., and Packer, L. (2000). Population-typical behaviours are retained when eusocial and non-eusocial forms of Evylaeus albipes (F.) (Hymenoptera, Halictidae) are reared simultaneously in the laboratory. Insectes Soc. 47, 263–270. doi: 10.1007/PL00001713
Pollinator Partnership (2022). Planting Guides Pollinator.org (2022). Available online at: https://www.pollinator.org/guides (accessed February 22, 2022)
Pornon, A., Andalo, C., Burrus, M., and Escaravage, N. (2017). DNA metabarcoding data unveils invisible pollination networks. Sci. Rep. 7, 16828. doi: 10.1038/s41598-017-16785-5
Potter, C., Vere, N., de, Jones, L. E., Ford, C. R., Hegarty, M. J., Hodder, K. H., et al. (2019). Pollen metabarcoding reveals broad and species-specific resource use by urban bees. PeerJ 7, e5999. doi: 10.7717/peerj.5999
Prasifka, J. R., Mallinger, R. E., Portlas, Z. M., Hulke, B. S., Fugate, K. K., Paradis, T., et al. (2018). Using nectar-related traits to enhance crop-pollinator interactions. Front. Plant Sci. 9, 821. doi: 10.3389/fpls.2018.00812
Praz, C. J., Müller, A., and Dorn, S. (2008). Host recognition in a pollen-specialist bee: evidence for a genetic basis. Apidologie 39, 547–557. doi: 10.1051/apido:2008034
Prendergast, K. S., Menz, M. H. M., Dixon, K. W., and Bateman, P. W. (2020). The relative performance of sampling methods for native bees: an empirical test and review of the literature. Ecosphere 11, e03076. doi: 10.1002/ecs2.3076
Preti, M., Verheggen, F., and Angeli, S. (2021). Insect pest monitoring with camera-equipped traps: strengths and limitations. J. Pest Sci. 94, 203–217. doi: 10.1007/s10340-020-01309-4
Ptáček, V., Votavová, A., and Komzáková, O. (2015). Experience in rearing common carder bees (Bombus pascuorum Scop.), with Some notes on three similar species: shrill carder bee (B. sylvarum L.), red-shanked carder bee (B. ruderarius Müll.), and Brown-banded Carder Bee (B. humilis Ill.) (Hymenoptera: Apidae). Acta Univ. Agric. Silviculturae Mendelianae Brunensis 63, 1535–1542. doi: 10.11118/actaun201563051535
Pufal, G., Steffan-Dewenter, I., and Klein, A.-M. (2017). Crop pollination services at the landscape scale. Curr. Opinion Insect Sci. 21, 91–97. doi: 10.1016/j.cois.2017.05.021
Rader, R., Bartomeus, I., Garibaldi, L. A., Garratt, M. P. D., Howlett, B. G., Winfree, R., et al. (2016). Non-bee insects are important contributors to global crop pollination. Proc. Natl. Acad. Sci. U.S.A. 113, 146–151. doi: 10.1073/pnas.1517092112
Raine, N. E., and Chittka, L. (2007). Pollen foraging: learning a complex motor skill by bumblebees (Bombus terrestris). Naturwissenschaften 94, 459–464. doi: 10.1007/s00114-006-0184-0
Raitoharju, J., Riabchenko, E., Ahmad, I., Iosifidis, A., Gabbouj, M., Kiranyaz, S., et al. (2018). Benchmark database for fine-grained image classification of benthic macroinvertebrates. Image Vis. Comput. 78, 73–83. doi: 10.1016/j.imavis.2018.06.005
Ratnayake, M. N., Dyer, A. G., and Dorin, A. (2021). Tracking individual honeybees among wildflower clusters with computer vision-facilitated pollinator monitoring. PLoS ONE 16, e0239504. doi: 10.1371/journal.pone.0239504
Rehan, S. M. (2020). “Small carpenter bees (Ceratina),” in Encyclopedia of Social Insects, ed C. Starr (Cham: Springer). doi: 10.1007/978-3-319-90306-4_106-1
Rehan, S. M., and Richards, M. H. (2010a). Nesting biology and subsociality in Ceratina calcarata (Hymenoptera: Apidae). Can. Entomol. 142, 65–74. doi: 10.4039/n09-056
Rehan, S. M., and Richards, M. H. (2010b). The influence of maternal quality on brood sex allocation in the small carpenter bee, Ceratina calcarata. Ethology 116, 876–887. doi: 10.1111/j.1439-0310.2010.01804.x
Rhoades, P., Griswold, T., Waits, L., Bosque-Pérez, N. A., Kennedy, C. M., and Eigenbrode, S. D. (2017). Sampling technique affects detection of habitat factors influencing wild bee communities. J. Insect Conserv. 21, 703–714. doi: 10.1007/s10841-017-0013-0
Richardson, R. T., Curtis, H. R., Matcham, E. G., Lin, C.-H., Suresh, S., Sponsler, D. B., et al. (2019). Quantitative multi-locus metabarcoding and waggle dance interpretation reveal honey bee spring foraging patterns in Midwest agroecosystems. Mol. Ecol. 28, 686–697. doi: 10.1111/mec.14975
Richardson, R. T., Lin, C.-H., Sponsler, D. B., Quijia, J. O., Goodell, K., and Johnson, R. M. (2015). Application of ITS2 metabarcoding to determine the provenance of pollen collected by honey bees in an agroecosystem. Appl. Plant Sci. 3, 1400066. doi: 10.3732/apps.1400066
Rodger, J. G., Bennett, J. M., Razanajatovo, M., Knight, T. M., van Kleunen, M., Ashman, T.-L., et al. (2021). Widespread vulnerability of flowering plant seed production to pollinator declines. Sci. Adv. 7, eabd3524. doi: 10.1126/sciadv.abd3524
Roulston, T. H., and Cane, J. H. (2002). The effect of pollen protein concentration on body size in the sweat bee Lasioglossum zephyrum (Hymenoptera: Apiformes). Evol. Ecol. 16, 49–65. doi: 10.1023/A:1016048526475
Ruedenauer, F. A., Raubenheimer, D., Kessner-Beierlein, D., Grund-Mueller, N., Noack, L., Spaethe, J., et al. (2020). Best be(e) on low fat: linking nutrient perception, regulation and fitness. Ecol. Lett. 23, 545–554. doi: 10.1111/ele.13454
Ruedenauer, F. A., Spaethe, J., van der Kooi, C. J., and Leonhardt, S. D. (2019). Pollinator or pedigree: which factors determine the evolution of pollen nutrients? Oecologia 191, 349–358. doi: 10.1007/s00442-019-04494-x
Russell, A. L., Golden, R. E., Leonard, A. S., and Papaj, D. R. (2016). Bees learn preferences for plant species that offer only pollen as a reward. Behav. Ecol. 27, 731–740. doi: 10.1093/beheco/arv213
Russell, A. L., Morrison, S. J., Moschonas, E. H., and Papaj, D. R. (2017). Patterns of pollen and nectar foraging specialization by bumblebees over multiple timescales using RFID. Sci. Rep. 7, 42448. doi: 10.1038/srep42448
Saleh, N., and Chittka, L. (2007). Traplining in bumblebees (Bombus impatiens): a foraging strategy's ontogeny and the importance of spatial reference memory in short-range foraging. Oecologia 151, 719–730. doi: 10.1007/s00442-006-0607-9
Saleh, N., Ohashi, K., Thomson, J. D., and Chittka, L. (2006). Facultative use of the repellent scent mark in foraging bumblebees: complex versus simple flowers. Anim. Behav. 71, 847–854. doi: 10.1016/j.anbehav.2005.06.014
Sampson, B. J., Danka, R. G., and Stringer, S. J. (2004). Nectar robbery by bees xylocopa virginica and apis mellifera contributes to the pollination of rabbiteye blueberry. Apic. Soc. Insects 97, 735–740. doi: 10.1093/jee/97.3.735
Sardiñas, H. S., and Kremen, C. (2015). Pollination services from field-scale agricultural diversification may be context-dependent. Agric. Ecosyst. Environ. 207, 17–25. doi: 10.1016/j.agee.2015.03.020
Schäffler, I., and Dötterl, S. (2011). A day in the life of an oil bee: phenology, nesting, and foraging behavior. Apidologie 42, 409–424. doi: 10.1007/s13592-011-0010-3
Serrana, J. M., Miyake, Y., Gamboa, M., and Watanabe, K. (2019). Comparison of DNA metabarcoding and morphological identification for stream macroinvertebrate biodiversity assessment and monitoring. Ecol. Indic. 101, 963–972. doi: 10.1016/j.ecolind.2019.02.008
Sevillano, V., Holt, K., and Aznarte, J. L. (2020). Precise automatic classification of 46 different pollen types with convolutional neural networks. PLoS ONE 15, e0229751. doi: 10.1371/journal.pone.0229751
Sidhu, S. (2013). Farmscape and Landscape-Level Effects on Cucurbit Pollinators on Small Farms in A Diversified Agroecosystem. Available online at: https://etda.libraries.psu.edu/files/final_submissions/9121 (accessed July 24, 2021).
Šigut, M., Kostovčík, M., Šigutov,á, H., Hulcr, J., Drozd, P., and Hrček, J. (2017). Performance of DNA metabarcoding, standard barcoding, and morphological approach in the identification of host-parasitoid interactions. PLoS ONE 12, e0187803. doi: 10.1371/journal.pone.0187803
Sihag, R. C. (2018). Some unresolved issues of measuring the efficiency of pollinators: experimentally testing and assessing the predictive power of different methods. Int. J. Ecol. 2018, e3904973. doi: 10.1155/2018/3904973
Simpson, S. J., and Raubenheimer, D. (2012). The Nature of Nutrition: A Unifying Framework From Animal Adaptation to Human Obesity. Princeton University Press. doi: 10.1515/9781400842803
Smart, M. D., Otto, C. R. V., and Lundgren, J. G. (2019). Nutritional status of honey bee (Apis mellifera L.) workers across an agricultural land-use gradient. Sci. Rep. 9, 16252. doi: 10.1038/s41598-019-52485-y
Smith-Ramirez, C., Rendón-Funes, A., Barahona-Segovia, R., and Moya, W. (2021). Consequences of the high abundance of Bombus terrestris on the pollination of Vicia faba. J. Pollinat. Ecol. 29, 258–272. doi: 10.26786/1920-7603(2021)630
Spiesman, B. J., Gratton, C., Hatfield, R. G., Hsu, W. H., Jepsen, S., McCornack, B., et al. (2021). Assessing the potential for deep learning and computer vision to identify bumble bee species from images. Sci. Rep. 11, 7580. doi: 10.1038/s41598-021-87210-1
Sponsler, D. B., Shump, D., Richardson, R. T., and Grozinger, C. M. (2020). Characterizing the floral resources of a North American metropolis using a honey bee foraging assay. Ecosphere 11, e03102. doi: 10.1002/ecs2.3102
Stabler, D., Al-Esawy, M., Chennells, J. A., Perri, G., Robinson, A., and Wright, G. A. (2021). Regulation of dietary intake of protein and lipid by nurse-age adult worker honeybees. J. Exp. Biol. 224, jeb230615. doi: 10.1242/jeb.230615
Stabler, D., Paoli, P. P., Nicolson, S. W., and Wright, G. A. (2015). Nutrient balancing of the adult worker bumblebee (Bombus terrestris) depends on the dietary source of essential amino acids. J. Exp. Biol. 218, 793–802. doi: 10.1242/jeb.114249
Steen, R. (2017). Diel activity, frequency and visit duration of pollinators in focal plants: in situ automatic camera monitoring and data processing. Methods Ecol. Evolut. 8, 203–213. doi: 10.1111/2041-210X.12654
Steitz, I., and Ayasse, M. (2020). Macrocyclic lactones act as a queen pheromone in a primitively eusocial sweat bee. Curr. Biol. 30, 1136–1141.e3. doi: 10.1016/j.cub.2020.01.026
Strange, J. P. (2010). Nest initiation in three North American bumble bees (Bombus): gyne number and presence of honey bee workers influence establishment success and colony size. J. Insect Sci. 10, 130. doi: 10.1673/031.010.13001
Sutherland, W. J., Roy, D. B., and Amano, T. (2015). An agenda for the future of biological recording for ecological monitoring and citizen science. Biol. J. Linn. Soc. Lond. 115, 779–784. doi: 10.1111/bij.12576
Theodorou, P., Radzevičiute, R., Lentendu, G., Kahnt, B., Husemann, M., Bleidorn, C., et al. (2020). Urban areas as hotspots for bees and pollination but not a panacea for all insects. Nat. Commun. 11, 576. doi: 10.1038/s41467-020-14496-6
Tian, L., Rahman, S. R., Ezray, B. D., Franzini, L., Strange, J. P., Lhomme, P., et al. (2019). A homeotic shift late in development drives mimetic color variation in a bumble bee. Proc. Natl. Acad. Sci. U.S.A. 116, 11857–11865. doi: 10.1073/pnas.1900365116
Treanore, E. D., Vaudo, A. D., Grozinger, C. M., and Fleischer, S. J. (2019). Examining the nutritional value and effects of different floral resources in pumpkin agroecosystems on Bombus impatiens worker physiology. Apidologie 50, 542–552. doi: 10.1007/s13592-019-00668-x
Trinkl, M., Kaluza, B. F., Wallace, H., Heard, T. A., Keller, A., and Leonhardt, S. D. (2020). Floral species richness correlates with changes in the nutritional quality of larval diets in a stingless bee. Insects 11, 125. doi: 10.3390/insects11020125
Tritschler, M., Vollmann, J. J., Yañez, O., Chejanovsky, N., Crailsheim, K., and Neumann, P. (2017). Protein nutrition governs within-host race of honey bee pathogens. Sci. Rep. 7, 14988. doi: 10.1038/s41598-017-15358-w
Tuell, J. K., Ascher, J. S., and Isaacs, R. (2009). Wild bees (Hymenoptera: Apoidea: Anthophila) of the michigan highbush blueberry agroecosystem. Ann. Entomol. Soc. Am. 102, 275–287. doi: 10.1603/008.102.0209
Turunen, J., Mykr,ä, H., Elbrecht, V., Steinke, D., Braukmann, T., and Aroviita, J. (2021). The power of metabarcoding: can we improve bioassessment and biodiversity surveys of stream macroinvertebrate communities? Metabarcod. Metagenom. 5, e68938. doi: 10.3897/mbmg.5.68938
Ullman, K., Shih, T., Vaughan, M., and Kremen, C. (2008). Pennsylvania Native Bee Survey Citizen Science Pollinator Monitoring Guide. Xerces Society.
Ullmann, K. S., Meisner, M. H., and Williams, N. M. (2016). Impact of tillage on the crop pollinating, ground-nesting bee, Peponapis pruinosa in California. Agric. Ecosyst. Environ. 232, 240–246. doi: 10.1016/j.agee.2016.08.002
USDA NRCS Maine New Hampshire Vermont, Connecticut, Massachusetts, Rhode Island State Offices, the Xerces Society for Invertebrate Conservation's Pollinator Conservation Program, the University of Maine Cooperative Extension. (2012). New England Pollinator Handbook. Available online at: https://www.nrcs.usda.gov/Internet/FSE_DOCUMENTS/stelprdb1075524.pdf (accessed November 18, 2021)
Valido, A., Rodríguez-Rodríguez, M. C., and Jordano, P. (2019). Honeybees disrupt the structure and functionality of plant-pollinator networks. Sci. Rep. 9, 4711. doi: 10.1038/s41598-019-41271-5
van den Eijnde, J., de Ruijter, A., and van der Steen, J. (1991). Method for rearing bombus terrestris continuously and the production of bumblebee colonies for pollination purposes. Acta Hortic. 154–158. doi: 10.17660/ActaHortic.1991.288.20
Van Handel, E., and Day, J. F. (1988). Assay of lipids, glycogen and sugars in individual mosquitoes: correlations with wing length in field-collected Aedes vexans. J. Am. Mosq. Control Assoc. 4, 549–550.
Vanderplanck, M., Leroy, B., Wathelet, B., Wattiez, R., and Michez, D. (2014). Standardized protocol to evaluate pollen polypeptides as bee food source. Apidologie 45, 192–204. doi: 10.1007/s13592-013-0239-0
Vanderplanck, M., Vereecken, N. J., Grumiau, L., Esposito, F., Lognay, G., Wattiez, R., et al. (2017). The importance of pollen chemistry in evolutionary host shifts of bees. Sci. Rep. 7, 43058. doi: 10.1038/srep43058
Vaudo, A. D., Biddinger, D. J., Sickel, W., Keller, A., and López-Uribe, M. M. (2020b). Introduced bees (Osmia cornifrons) collect pollen from both coevolved and novel host-plant species within their family-level phylogenetic preferences. R. Soc. Open Sci. 7, 200225. doi: 10.1098/rsos.200225
Vaudo, A. D., Patch, H. M., Mortensen, D. A., Grozinger, C. M., and Tooker, J. F. (2014). Bumble bees exhibit daily behavioral patterns in pollen foraging. Arthropod. Plant. Interact. 8, 273–283. doi: 10.1007/s11829-014-9312-5
Vaudo, A. D., Patch, H. M., Mortensen, D. A., Tooker, J. F., and Grozinger, C. M. (2016). Macronutrient ratios in pollen shape bumble bee (Bombus impatiens) foraging strategies and floral preferences. Proc. Natl. Acad. Sci. U.S.A. 113, E4035–E4042. doi: 10.1073/pnas.1606101113
Vaudo, A. D., Tooker, J. F., Grozinger, C. M., and Patch, H. M. (2015). Bee nutrition and floral resource restoration. Curr. Opinion Insect Sci. 10, 133–141. doi: 10.1016/j.cois.2015.05.008
Vaudo, A., and Leonard, A. (2021). “Pollen nutrition structures bee-wildflower community interactions in the Eastern Sierra,” in Pollinator Nutritional Research: From Collecting and Characterizing Floral Resource Provisions to the Inference of Ecological and Evolutionary Consequences (Denver, CO). Available online at: https://www.eventscribe.net/2021/entomology2021/index.asp?presTarget=1717881
Vaudo, A. D., Tooker, J. F., Patch, H. M., Biddinger, D. J., Coccia, M., Crone, M. K., et al. (2020a). Pollen protein: lipid macronutrient ratios may guide broad patterns of bee species floral preferences. Insects 11, 132. doi: 10.3390/insects11020132
Velthuis, H. H. W., and van Doorn, A. (2006). A century of advances in bumblebee domestication and the economic and environmental aspects of its commercialization for pollination. Apidologie 37, 421–451. doi: 10.1051/apido:2006019
Venturini, E. M., Drummond, F. A., Hoshide, A. K., Dibble, A. C., and Stack, L. B. (2017). Pollination reservoirs for wild bee habitat enhancement in cropping systems: a review. Agroecol. Sustain. Food Syst. 41, 101–142. doi: 10.1080/21683565.2016.1258377
Wang, R., Hu, C., Fu, X., Long, T., and Zeng, T. (2017). Micro-Doppler measurement of insect wing-beat frequencies with W-band coherent radar. Sci. Rep. 7, 1396. doi: 10.1038/s41598-017-01616-4
Waters, N. D. (1971). A Technique for laboratory rearing immature alfalfa leafcutter bees (Hymenoptera: Megachilidae)1,2. Ann. Entomol. Soc. Am. 64, 1183–1183. doi: 10.1093/aesa/64.5.1183
Weiner, C. N., Hilpert, A., Werner, M., Linsenmair, K. E., and Blüthgen, N. (2010). Pollen amino acids and flower specialisation in solitary bees. Apidologie 41, 476–487. doi: 10.1051/apido/2009083
Whittington, R., Winston, M. L., Tucker, C., and Parachnowitsch, A. L. (2004). Plant-species identity of pollen collected by bumblebees placed in greenhouses for tomato pollination. Can. J. Plant Sci. 84, 599–602. doi: 10.4141/P02-192
Chan, D. S., and Raine, N. E. (2021). Population decline in a ground-nesting solitary squash bee (Eucera pruinosa) following exposure to a neonicotinoid insecticide treated crop (Cucurbita pepo). Sci. Rep. 11, 4241. doi: 10.1038/s41598-021-83341-7
Wilson, J. S., Griswold, T., and Messinger, O. J. (2008). Sampling bee communities (Hymenoptera: Apiformes) in a desert landscape: are pan traps sufficient? J. Kans. Entomol. Soc. 81, 288–300. doi: 10.2317/JKES-802.06.1
Wood, T. J., Gibbs, J., Graham, K. K., and Isaacs, R. (2019). Narrow pollen diets are associated with declining Midwestern bumble bee species. Ecology 100, e02697. doi: 10.1002/ecy.2697
Wood, T. J., Holland, J. M., and Goulson, D. (2016). Diet characterisation of solitary bees on farmland: dietary specialisation predicts rarity. Biodivers. Conserv. 25, 2655–2671. doi: 10.1007/s10531-016-1191-x
Wood, T. J., Holland, J. M., Hughes, W. O. H., and Goulson, D. (2015). Targeted agri-environment schemes significantly improve the population size of common farmland bumblebee species. Mol. Ecol. 24, 1668–1680. doi: 10.1111/mec.13144
Woodard, S. H., Duennes, M. A., Watrous, K. M., and Jha, S. (2020). Diet and nutritional status during early adult life have immediate and persistent effects on queen bumble bees. Conserv. Physiol. 7, coaa007, doi: 10.1093/conphys/coz048
Woodcock, B. A., Isaac, N. J. B., Bullock, J. M., Roy, D. B., Garthwaite, D. G., Crowe, A., et al. (2016). Impacts of neonicotinoid use on long-term population changes in wild bees in England. Nat. Commun. 7, 12459. doi: 10.1038/ncomms12459
Wratten, S. D., Gillespie, M., Decourtye, A., Mader, E., and Desneux, N. (2012). Pollinator habitat enhancement: Benefits to other ecosystem services. Agric. Ecosyst. Environ. 159, 112–122. doi: 10.1016/j.agee.2012.06.020
Wright, G. A., Nicolson, S. W., and Shafir, S. (2018). Nutritional physiology and ecology of honey bees. Annu. Rev. Entomol. 63, 327–344. doi: 10.1146/annurev-ento-020117-043423
Zhang, H., Zhou, Z., Huang, J., Yuan, X., Ding, G., and An, J. (2018). Queen traits and colony size of four bumblebee species of China. Insect. Soc. 65, 537–547. doi: 10.1007/s00040-018-0639-2
Keywords: nutrition, pollen, wild bees, native bees, nutritional ecology of insects, experimental methods and procedure
Citation: Crone MK, Biddinger DJ and Grozinger CM (2022) Wild Bee Nutritional Ecology: Integrative Strategies to Assess Foraging Preferences and Nutritional Requirements. Front. Sustain. Food Syst. 6:847003. doi: 10.3389/fsufs.2022.847003
Received: 31 December 2021; Accepted: 07 March 2022;
Published: 14 April 2022.
Edited by:
Harmen P. Hendriksma, Julius Kühn-Institut - Braunschweig, GermanyReviewed by:
Mauricio Gonzalez-Chang, Universidad Austral de Chile, ChileT'Ai Roulston, University of Virginia, United States
Copyright © 2022 Crone, Biddinger and Grozinger. This is an open-access article distributed under the terms of the Creative Commons Attribution License (CC BY). The use, distribution or reproduction in other forums is permitted, provided the original author(s) and the copyright owner(s) are credited and that the original publication in this journal is cited, in accordance with accepted academic practice. No use, distribution or reproduction is permitted which does not comply with these terms.
*Correspondence: Makaylee K. Crone, bWtjMjA2JiN4MDAwNDA7cHN1LmVkdQ==