- 1Department of Life Science Systems, School of Life Sciences, Technical University of Munich, Freising, Germany
- 2Institute of Landscape Ecology, University of Münster, Münster, Germany
- 3Berlin-Brandenburg Institute of Advanced Biodiversity Research (BBIB), Berlin, Germany
- 4Department of Ecology, Technische Universität Berlin, Berlin, Germany
The urban heat island (UHI) effect remains a major threat to society as cities densify and sprawl. Urban greening through local to landscape management is a proposed strategy to combat UHI and improve environmental justice in city neighborhoods. For example, urban community gardens are multifunctional green spaces that play an important role for biodiversity and for civic engagement. But the role of urban gardens in urban cooling and relieving UHI remain unclear, specifically how temperatures fluctuate within gardens in relation to garden management factors and city landscape context, and how this relates to urban heat in city neighborhoods. We investigated diurnal and nocturnal temperature ranges, and daily maximum and minimum temperatures in 18 urban gardens over the peak of the summer agricultural growing season. We then analyzed how temperatures were correlated to local land cover factors within the garden, to surrounding landscape imperviousness at various spatial scales, and to environmental justice indicators (stressors) of garden neighborhoods. We found that nocturnal temperature range is negatively correlated to landscape imperviousness, and that the relationship decreases in strength with increasing spatial scale. This result supports the importance of evapotranspiration processes of surrounding green areas for nocturnal cooling. Some local land cover factors were important for temperatures, indicating heating or cooling management mechanisms from within urban gardens. Finally, the mean number of environmental stressors in neighborhoods negatively related to temperature variation. The results of this work can inform resource use and crop selection in urban agriculture, as well as how temperature-related ecosystem services of gardens relate to environmental justice of city neighborhoods.
Introduction
Rapid urbanization continues to pose challenges for urban climate change mitigation, water supply sustainability, and public health (UN-Habitat, 2017). For example, due to urban heat island (UHI) effects, urban temperatures are often higher than in rural surroundings, ranging inside urban areas from 0.5 to 4°C higher during the daytime, and 1 to 3°C higher during the night (Oke, 1973; Taha, 1997; Stewart, 2011). High temperatures may reduce the thermal comfort of populations (Robine et al., 2008; Mohajerani et al., 2017), and increase energy demand; indeed, for each 1°C increase in temperature, the energy demand in cities increases by 2 to 4% (Akbari et al., 1997). As a counter measure to such urban sustainability challenges, the FAO's “Green Cities Initiative” (2020) aimed to improve the urban environment by increasing the availability of green spaces to improve mitigation and adaptation strategies of cities to climate change, to reduce UHI, and also to improve public health (FAO, 2020). Urban greening may be an optimal and cost-effect strategy for urban thermal regulation through evapotranspiration as a cooling process to thereby reduce UHI (Lehmann, 2014; Litardo et al., 2020).
There is a bi-directional relation between urban green and urban climate. On the one hand, urban green space serves as a UHI mitigation strategy due to evapotranspiration rates (Qiu et al., 2013), on the other hand, temperature increases could significantly affect plant survival and nutrient cycles, threatening urban green space functioning and the ecosystem services they could deliver. The higher complexity and biodiversity in a system could make systems more resilient to temperature variation (Lin et al., 2018), highlighting the importance of vegetatively diverse urban greening. Temperature regulation by urban green is a dynamic process and differs temporally and spatially according to differences in land use composition at the local ecosystem scale to the regional landscape scale. For example, during the night there is a strong correlation between the land surface composition and temperature variability with the fraction of built impervious surface around urban green (Quanz et al., 2018).
An urban ecosystem where temperature variation and land cover management may be relevant from an urban cooling perspective and ecosystem service perspective are urban gardens designed for food and flower production. As a horticultural system, urban gardens can support urban cooling and temperature mitigation through vegetation management; this can work to alleviate UHI, while also alleviating food insecurities for city residents (Lin and Egerer, 2020). For example, nocturnal temperature variation in urban allotment gardens can be lower in gardens closer to city center, suggesting that impervious land cover acts as a heating factor (Rost et al., 2020). Furthermore, local impervious cover within gardens can increase local average garden temperatures by acting as a heating factor, while grass cover can decrease average temperatures, acting as a cooling factor; temperatures in turn influenced gardeners water use management (Lin et al., 2018). Such work provides preliminary insight into the role of urban gardens as a potential cooling strategy and for urban ecosystem service provision. Yet it is still necessary to understand how temperatures vary over time and how this may be managed through land cover gradients because temperatures can influence crop selection in urban agriculture and subsequent food production.
Provision of ecosystem services through community gardens may also mitigate effects of environmental injustice across the urban landscape, such as access to green spaces in deprived neighborhoods (Wolch et al., 2014). Environmental justice relates to the equitable distribution and access to green spaces and the services that they provide, from temperature regulation to recreation. Here, gardens may provide cool temperatures and shade to recreate and grow food in respective to their surroundings, which may be especially important for those without private green space and within especially built-up neighborhoods where UHI is most extreme. Some studies show that socio-economic factors of city neighborhoods affect the distribution of community gardens across urban landscape (Smith et al., 2013; Butterfield, 2020). However, few investigations show how other environmental injustices, such as noise or air pollution, relate to community garden locations and their functions and services.
The goal of this study was to investigate temperature variation across the day within urban community gardens and how this may be influenced by land cover at the local to landscape scale, and in addition, how temperature variation within gardens and their distribution may relate to environmental justice factors associated with garden neighborhoods. In 18 gardens in the metropolitan region of Berlin, Germany, we asked: (1) How do temperatures vary over time (diurnal vs. nocturnal time periods) in gardens? (2) Is there an influence of variation in land cover within and surrounding gardens at various spatial scales on temperature variation over time? (3) Is there a relationship between environmental justice factors of the neighborhoods in which gardens are embedded and garden temperature variation and distribution, respectively? The results of the study may inform mechanisms behind urban temperature attenuation at different spatial scales, inform urban food production in the context of the urban climate, and inform how temperature variation may relate to the socioeconomic conditions of city neighborhoods.
Materials and Methods
Area of Study
This research took place in 18 urban community gardens in Berlin, Germany in June to July of 2020 (Supplementary Table 1; Figures 1A,B). With 891.1 km2 of total area and almost 3,670 million inhabitants as of 2019 (Das Amt für Statistik Berlin-Brandenburg, 2019), Berlin is the biggest city in Germany (Deutsche Welle, 2020). The climate is mainly influenced by high latitude westerlies and has a Cfb climate classification according to the Köppen-Geiger climate classification with warm and humid summers (Kottek et al., 2006).
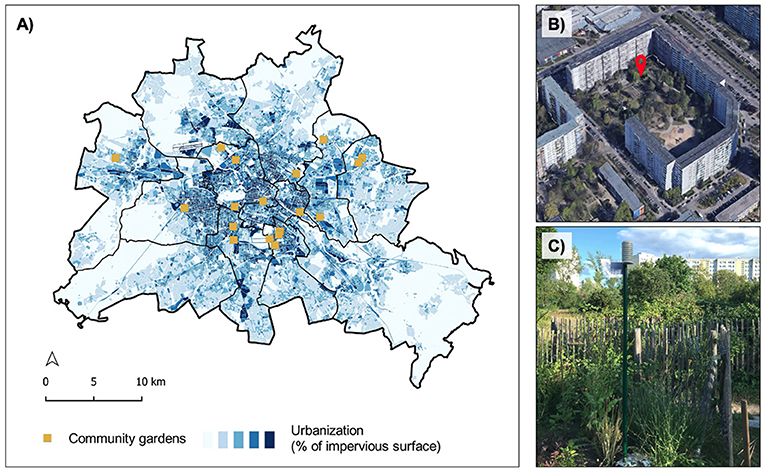
Figure 1. Study system in the metropolitan region of Berlin, Germany where the 18 community gardens examined (yellow squares) and along an urbanization (% impervious surface) gradient (A); an inset of a garden surrounded by residential housing structures [(B); Photo: Google Inc (2021)]. Photo of the temperature station in a garden in Marzahn, Germany [(C); Photo: M Egerer].
Temperature and Land Cover Data
Temperatures were recorded at 30-min intervals from weather stations placed within each garden (Figure 1C). The stations consisted of temperature sensor loggers (EasyLog EL-USB-2 Temperature and Relative Humidity USB Data Logger) placed on 2-m metal poles above ground level following (von der Lippe et al., 2020). The battery-operated sensors can measure > 16,000 readings over a −35 to +80°C and 0 to 100% humidity (RH) range, and data can be downloaded using a PC's USB port and with the EasyLog software. Sensors were also protected by a passively ventilated plastic shield to reduce sensor overheating and potential damage from external factors (e.g., solar radiation, wind, rain). The period of data collection used in this analysis is between 01.06.2020 and 31.07.2020 for all sites, representing an important period in agricultural production in Germany. In some cases, battery life of the temperature loggers led to short-term data gaps in the temperature data. The 18 gardens, their period(s) of data recording and location are presented in Supplementary Table 1.
Land cover within each garden was measured during two field surveys in June and July 2020. In each survey, within a 20 × 20–m plot at the center of each garden, we randomly placed eight 1 × 1-m quadrats across the plot within which to record the percentage of bare soil cover, mulch cover, rock cover, grass cover, herbaceous vegetation cover, and wood cover as our local garden scale land cover variables. Logistical restraints led to missing land cover data for four gardens in June 2020. We averaged land cover data from these quadrats for each survey. Land cover surrounding each garden (landscape scale) was measured at three different radii from the garden center point with publicly available data on the surrounding landscape features of each garden from the Berlin Environmental Atlas (Senatsverwaltung für Stadtentwicklung und Umwelt, 2016). Here we measured the proportion of imperviousness at a 2 × 2-m resolution within 500-m, 1-km and 2-km buffers using the Zonal statistics tool in QGIS software v. 2.18.0 (QGIS Development Team, 2018).
Environmental Justice Data
To investigate relationships between garden locations, temperature, and socioeconomic factors, we collected open access environmental justice data from the Berlin Environmental Atlas, Environmental Justice Berlin (Senatsverwaltung für Stadtentwicklung und Umwelt, 2013). This spatial dataset contains values of five environmental “stressors” that relate to environmental justice: noise pollution, air pollution, green space provision, bioclimatic stress, and social problems. These stressors are defined for 447 structurally connected neighborhoods (henceforth “blocks”) in Berlin. These variables are categorical with either three or four categories from low to high. A sixth variable adds the number of stressors, counting only the highest or lowest category, respectively.
Data Analysis
We calculated the following variables for each garden: daily average temperature, daily maximum temperature, daily minimum temperature, and temperature range (TR = Tmax – Tmin). To determine whether temperature variation varies over time, we then calculated each of the five variables for diurnal (05:00–20:59) and nocturnal (21:00–04.59) periods. We then calculated monthly averages for each garden for the diurnal, nocturnal and full-day periods (DTR = diurnal temperature range; NTR = nocturnal temperature range), which also included a monthly average TR.
We conducted a regression analysis to test for correlations between the temperature variables and the five local land cover variables at each time period and three landscape variables across all sites. Although not the focus of the study, in our preliminary analysis we also tested for relationships between land cover variables and average daily temperatures. We performed the analysis for all sites and all time periods to maximize sample sizes in the analysis. We used Google Earth Pro to qualitatively assess features around the gardens including water bodies and trees. The associations between temperature and the average number of environmental stressors in a 500 m buffer around the gardens were tested with a linear regression model, applying NTR and DTR as response variable and the average number of stressors as predictor. The 500 m radius was set as the maximum catchment area, both for residents but also for any impacts the garden may have on environmental stressors. As the buffer overlaps at all garden locations with more than one block, we calculated the average of the number of stressors across all covered city blocks as proxy. In addition, we used logistic regression to test whether the odds of a garden present in a block is related to the number of or a specific environmental stressor(s) in that block. Multicollinearity was excluded by calculation the variation inflation factors (<2). In addition, a Pearson correlation coefficient was computed to assess the linear relationship between percentage of impervious surface and mean number of environmental stressors of the 500 m buffer radius around the garden locations. All analysis regarding the environmental stressors were conducted in R version 4.1, applying packages rgdal (Bivand et al., 2018), sf (Pebesma, 2018), rgeos (Bivand and Rundel, 2021), maptools (Bivand and Lewin-Koh, 2022), raster (Hijmans, 2015), dplyr (Wickham et al., 2022), car (Fox and Weisberg, 2019) and forcats (Wickham, 2021).
Results
Garden Temperatures
Temperatures varied across the gardens and over the season (Table 1). The average monthly temperature recorded for June varied between 19.8°C and 24.6°C across all gardens, with an average of 21.2°C. During July, average temperature among sites were slightly lower than in June and varied from 19.5 and 21.1°C, with an average of 20.3°C. Diurnal temperatures were on average 22.7°C across gardens, and the average minimum and maximum temperatures were 15.3 and 28.7°C, respectively. Nocturnal temperatures were on average 18.2°C across sites with an average minimum of 15.5°C and average maximum of 22.1°C. Diurnal temperatures in July were on average 21.1°C, with an average minimum and maximum of 14.5 and 28°C in July, respectively. The lowest diurnal maximum temperatures were recorded as 26.3°C in July, whereas the highest was recorded in June with 31.3°C. The nocturnal average temperature was 16.8°C with a minimum average of 14.3°C and a maximum average of 20.4°C. The highest DTR during June was 17.9 and 8.1°C for NTR. Whereas the lowest values were 8.5 and 4.6°C for DTR and NTR, respectively. In July, the smallest DTR and NTR were 10.1 and 4.6°C, respectively. Moreover, the highest DTR and NTR during July were 16.2 and 7.8°C. The maximum and minimum temperature in June was recorded at 31.3 and 13°C, respectively, while the maximum and minimum temperatures during July were 29.8 and 12.3°C, respectively.
Local and Landscape Land Cover
Local land cover varied across gardens. Herbaceous vegetation cover ranged from 15 to 65%, whereas grass ranged from 0.4 to 46%. Bare soil cover ranged from 3 to 62%, mulch cover from 0 to 24%, whereas rock cover varied from 0 to 72%. Within the 500-meter radius, the landscape imperviousness across all stations varied from 31 to 86%, with an average of 60%. Within the 1,000- m radius, imperviousness ranged from 34 to 73%, with an average of 57%. Finally, within the 2,000 m radius, imperviousness ranged from 35 to 73%, with an average of 56%.
Relationships Between Temperature Variation and Land Cover
Land cover significantly predicted temperature variations across the gardens (Tables 2, 3). The higher percentage of landscape imperviousness within 500 m of the gardens, the lower the DTR (p < 0.05, Figure 2A). For local land cover, the higher the grass cover, the higher the DTR (p = 0.004), whereas the higher the rock cover the lower the DTR (p = 0.045). Diurnal maximum temperature was negatively correlated with rock cover (p = 0.04) and mulch was positively correlated (p = 0.02). Across all spatial scales, the higher the landscape imperviousness, the lower the NTR (Table 3; Figure 2B). The correlation significance and relationship strength decreased with larger spatial scale; the highest regression coefficient was at 2,000-m, with a coefficient of −0.038, compared to −0.031 and −0.029 for 1,000-m and 500-m. Minimum nocturnal temperatures were significantly correlated with landscape imperviousness at 500 m (p < 0.05; other spatial scales not significant), where minimum temperatures were higher with higher landscape imperviousness. At the local scale, grass cover negatively correlated with nocturnal minimum temperatures (p = 0.008).
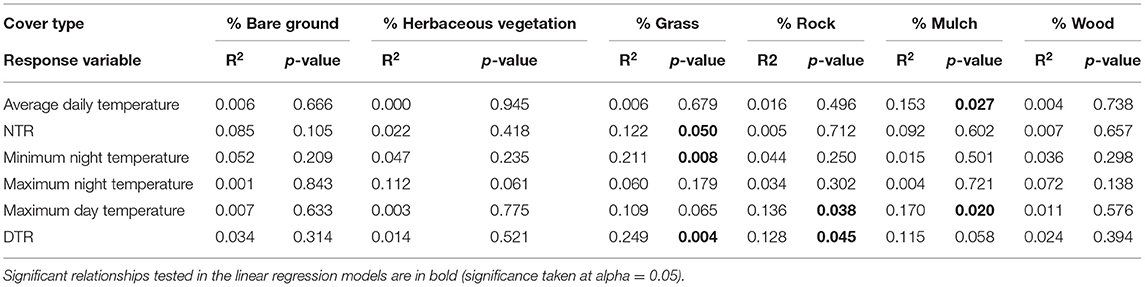
Table 2. Relationships between the temperature parameters (response variables) and the local land use cover variables of the gardens (measured within 8 1 × 1-m plots).
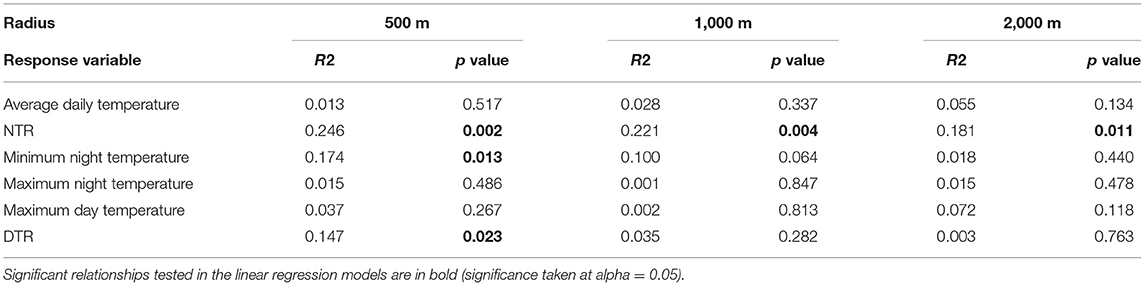
Table 3. Relationships between the temperature parameters (response variables) and impervious cover at the landscape scales measured (500-m, 1,000-m, 2,000-m radius surrounding each site).
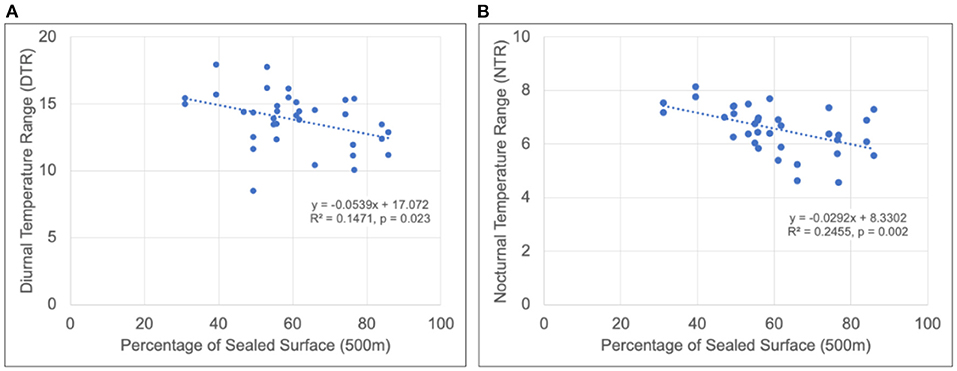
Figure 2. The diurnal temperature ranges [DTR; (A)] and nocturnal temperature ranges [NTR; (B)] were smaller with increased amounts of landscape imperviousness (percentage of sealed surface) surrounding gardens (here, at 500 m).
For supporting information, average temperatures did not vary with landscape imperviousness at any spatial scale, and only mulch cover was positively correlated wth average temperatures did not vary with landscape imperviousness at any spatial scale, and only mulch cover was positively correlated with average temperatures (Table 2).
Relationships Between Environmental Stressors, Temperature Variation and Garden Locations
Environmental stressors significantly negatively associated with both DTR and NTR, and positively associated with landscape imperviousness. The higher the number of environmental stressors within a 500 m radius around the garden locations, the higher the landscape imperviousness (rpearson = 0.740, p < 0.0004) and the lower the temperature ranges (Table 4). To test whether this result suggests that the presence of community gardens is related to the level of environmental justice of a block, we ran a logistic regression with the presence of a garden in a block as the response and environmental stressors as predictors. No significant relationship was found for the summed numbers of stressors. However, for single environmental stressors, we found significant negative correlations between green space provision and bioclimatic stress and garden location. Specifically, at the highest provision of green spaces in a block, the odds of a community garden within that block decrease by 18.6%. In addition, at the highest level of bioclimatic stress, the odds of a community garden within that block decrease by 2.8%. We found no relationship for socioeconomic level alone (represented by the environmental stressor social problems) (Table 5).
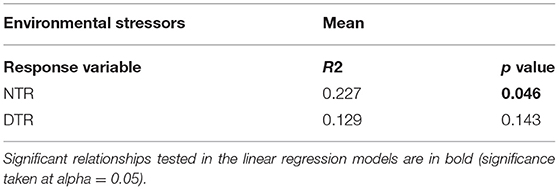
Table 4. Relationships between temperature range parameters (response variables) and mean environmental stressors of the garden within 500 m.
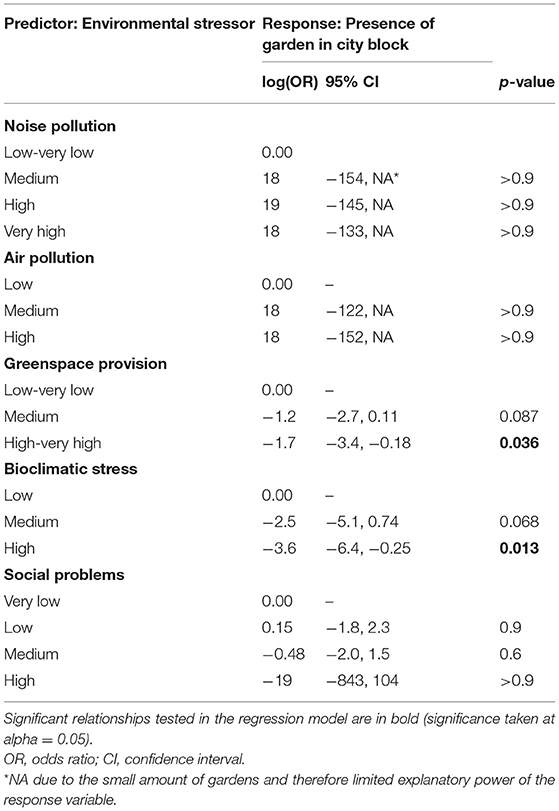
Table 5. Logistic regression summary, investigation correlations of the presence of a garden in a city block and environmental stressors.
Discussion
This study demonstrates a negative relationship between impervious surfaces, environmental stressors related to environmental (in)justice, and temperature variations in different periods of the day in community gardens across Berlin, Germany. In our study, nocturnal temperature range (NTR) was the most strongly affected temperature parameter by local to landscape scale land cover. Furthermore, land cover composition within gardens is not as strong of a temperature regulating factor as the surrounding landscape composition, specifically the amount of impervious sealed surface surrounding gardens, which strongly impacts local temperatures throughout the day. The socio-economic profiles of garden neighborhoods, namely environmental stressors, also negatively correlated with temperature variation, and results suggest that gardens may not be placed where bioclimatic stressors are most dire to offer regulation benefits, but do offer green space in otherwise “gray” highly impervious neighborhoods. Future research may further determine the effects of trees, water bodies, wind and urban morphology within the gardens on temperatures. Our results provide important insight into the temperature dynamics within an urban productive ecosystem, and can inform how these systems could be managed to work with these dynamics to cultivate (potentially new) crops, or placed within city neighborhoods to ameliorate bioclimatic stressors where they are most extreme.
Temporal Temperature Variation in Gardens
Our findings on temperature variation in these gardens are in line with other studies that have shown that garden temperatures can vary significantly across an urban gradient (Egerer et al., 2019a). Lower maximum temperatures influence DTR through reduced variation (Pyrgou et al., 2019), and we found that maximum temperatures in the gardens were higher in June than in July (e.g., 29.8°C in a garden; Figure 1), and thus, the DTR in June is also larger compared to July. Our findings are also in line with previous studies that found that DTR are larger than NTR (Ellis et al., 2015), which was the case for every garden. The highest minimum nocturnal temperatures were in June, interestingly in several gardens that are within courtyards (e.g., Figure 1B). Here, confinement at the local scale through built structures could prevent cooling off to increase nighttime temperatures to thereby create “nocturnal heat islands.” Also, shadowing effects of buildings, trees and courtyards during the day may mitigate high temperature extremes leading to lower DTR if temperatures are relatively stable. Causes of the UHI effect (usually perceivable during the night) include canyon radiative geometry, thermal properties of building materials, and sheltering of air masses (Oke et al., 1991). It is important to consider higher temperatures at night and also less pronounced ranges both during the day and night (i.e., a lack of the very cold or hot temperatures) because this can influence ecosystem service and disservice provision—for example, the ability of warm-loving crops to survive colder temperatures (service), but also uninhibited insect activity of mosquitos or other animal pests from colder temperatures (disservice).
Land Cover Influences Variation in Garden Temperatures
Temperature variation across the day in the gardens was negatively related to the amount of impervious surface in the surrounding landscape, and nocturnal temperature range is the most affected temperature variable by land cover. Thus, at the surrounding landscape scale, across all gardens and across all spatial scales, the more impervious the surroundings, the lower the NTR. Other studies in allotment gardens also found that surrounding impervious surface strongly affected nocturnal temperatures (in addition to allotment shape, vegetation complexity, etc.) (Rost et al., 2020). This phenomenon may be explained by the release of heat by building materials during the night (Quanz et al., 2018), which are absorbed and retained during the daytime and more slowly released in the night. Furthermore, urban materials have different thermal properties thus affecting UHI, and though out of the scope of this study, could influence temperature effects of land surface. Another factor to consider is urban morphology, which can encapsulate and limit the movements of wind masses (Oke et al., 1991). Thus, lower percentages of impervious surface cover could be a proxy for greater wind circulation and thereby enhanced cooling effects (Suomi and Käyhkö, 2012). The evapotranspiration process and the urban morphology could also explain the weakened correlation between temperature and impervious surface with increased spatial scale. For example, local air currents within 500 m could have a significantly higher effect than air currents between 1,000 and 2,000 m from the garden (it is possible that in some highly “urbanized” gardens, the effect of the 2,000 m radius is limited due to interactions with other closer elements). Similarly, the cooling effect from evapotranspiration in other green infrastructures (in relation to any of the gardens) could be dampened by surrounding urban infrastructure. For example, the high temperature variation in the garden on Tempelhofer Feld (NTR of 7.19°C)—compared to a garden on a rooftop (NTR of 5.6°C) just about 2 km away—could be due to the influence of more green space in the surroundings of the field.
At the local garden scale, we found that some temperature variables were strongly influenced by the land cover within the gardens. Grass cover related to minimum nocturnal temperatures, which may be explained by evapotranspiration processes that cool down a garden during the night (Lin et al., 2018). In contrast, rock cover negatively related to NTR, suggesting that rock is a heat retainer and “warming factor” in gardens (Lin et al., 2018). Surprisingly, diurnal maximum temperatures were lower with more rock cover, and higher with more mulch cover in the gardens. These mixed findings suggest to further assess and distinguish the actual type of rock cover within these gardens to understand the mechanism driving these relationships (e.g., gravel cover or porous rocks could also have some evaporation). The positive relationship of mulch and temperatures implies that gardeners can use less mulch to prevent raising garden temperatures and to avoid hindering the potential cooling effects of the gardens.
Garden Temperatures and Locations Relate to Environmental Justice Factors
Both diurnal and nocturnal temperature ranges were negatively related to the number of environmental stressors within a 500 m buffer around the garden. In turn, this relates to the previous finding of a negative correlation of environmental stressors with increasing imperviousness; indeed, more dense urban infrastructure (e.g., higher imperviousness, more buildings and roads) is often associated with higher air and noise pollution, reduced green space and negative impacts on human health (Rijnders et al., 2001; Li et al., 2017; Klompmaker et al., 2019). Furthermore, although we did not sample all gardens in Berlin, our results suggest that these gardens may or may not be best placed to ameliorate impacts of urban stressors and provide ecosystem services where needed most, particularly temperature regulation. Specifically, though they are in neighborhoods where green space is scant to thereby provide green space for recreation and to grow food, these gardens are not located in neighborhoods experiencing the highest bioclimatic stress. This suggests that community gardens are missing in neighborhoods where UHI is most extreme and gardens as an urban climate change adaptation strategy is needed most. These findings are in line with recommendations that cities should better optimize garden locations in specific neighborhoods to provision for different ecosystem services (Smith et al., 2021), especially around urban climate regulation (Clarke et al., 2019).
Limitations and Future Directions
Our research presents some limitations to explaining temperature variation in the gardens, for example the potential impact of nearby water bodies and the urban forest. Water bodies can influence garden minimum temperatures (Suomi and Käyhkö, 2012), because during the night they have a limiting effect on temperature drop due to its thermal capacity (Qiu et al., 2013). Interestingly, the highest values of minimum nocturnal temperature were recorded in gardens relatively close to a water body (e.g., in proximity to Malchower Lake, Rummelburger Lake, and the Spree River). Water bodies within the city help as a cooling mechanism during the day but during the night water could have a warming effect due to thermal inertia (Manteghi et al., 2015). This effect could describe the NTR in sites close to water. In addition, although we did not assess gardeners' irrigation behavior, irrigation is influenced by mean and maximum temperatures within gardens (Lin et al., 2018) and perceived higher temperatures in gardens (Egerer et al., 2019b), and some studies conducted in USA and China have demonstrated the cooling effect of irrigation mainly reflected on maximum temperatures (Lobell et al., 2008; Zhu et al., 2012).
In addition, trees generally reduce local urban diurnal air temperature (Potchter et al., 2006; Bowler et al., 2010), and may have cooling effects not captured here. During the summer days, denser tree canopies have a high influence in the maximum temperature, cooling down urban areas by shadowing (Ellis et al., 2015), while during the night, tree canopies can retain heat (Bowler et al., 2010). Previous studies have established the relationship between trees and heat storage under the canopies during the night (Quanz et al., 2018; Rost et al., 2020). For example, Rost et al. (2020) found that allotment gardens and urban parks' temperature variation differs, with allotment gardens having higher variation potentially due to the prohibition of trees in the gardens. This could explain temperature variations in gardens surrounded by trees, which, despite having relatively high impervious landscape surroundings, had relatively variable temperatures.
Conclusion
This study provides insight into the relationship between urban land use and temperature variations in different periods of the day in various community gardens across Berlin. We show that nocturnal temperature range is strongly affected by urban surroundings, and the inner composition of gardens is not as strong in regulating temperature as the surrounding landscape composition. These results suggest the potential to use urban gardens as green infrastructure to create cooling islands within the impervious landscape, especially for vulnerable populations where bioclimatic stressors are most extreme. With growing concerns of climate change, urban planning interventions should leverage spatially-explicit temperature data, land cover data, and environmental justice data to optimize where community gardens could be implemented to alleviate both inequitable green space provision and UHI impact. In sum, our results have important implications for the understanding of inner-city temperature dynamics to mitigate climate change, alleviate environmental stressors, and also to better produce food within city regions. Information on temperature-land use relationships can help to create micro-climatic zones within urban gardens and determine crop types to grow according to the temperature parameters. Furthermore, as gardeners begin to experiment with new crops to grow under changing climate conditions, our results could inform which plants can grow where in the city, and under what scenarios gardeners could utilize urban heat to grow previously uncultivated crops. Indeed, gardeners in these gardeners have already taken recommendations as to whether they can, for example, grow citrus and Mediterranean crops due to fewer frost days and in relation to cold temperature ranges at night. Our results can provide a view into crop plant selection and the potential to grow diverse, heat-loving crops in the city in a changing world.
Data Availability Statement
The raw data supporting the conclusions of this article will be made available by the authors, without undue reservation.
Author Contributions
ME and SB led the conception of the data collection. ME carried out the field data collection and edited the manuscript with input from AN, NP, and SB. AN led the data analysis and first draft of the manuscript. NP contributed to the data analysis and manuscript editing. All authors contributed to the article and approved the submitted version.
Funding
This research was funded by the International Postdoc Initiative of the TU Berlin (to ME) and the German Federal Ministry of Education and Research BMBF within the Collaborative Project Bridging in Biodiversity Science—BIBS (Funding Number 01LC1501A-H; to SB).
Conflict of Interest
The authors declare that the research was conducted in the absence of any commercial or financial relationships that could be construed as a potential conflict of interest.
Publisher's Note
All claims expressed in this article are solely those of the authors and do not necessarily represent those of their affiliated organizations, or those of the publisher, the editors and the reviewers. Any product that may be evaluated in this article, or claim that may be made by its manufacturer, is not guaranteed or endorsed by the publisher.
Acknowledgments
We thank the land owners and the community gardeners for supporting this work in their gardens: Allmende-Kontor, Gemeinschaftsgarten am Burbacher Weg, Himmelbeet, Inselgarten Schoeneberg, Kiezgarten Fischerstrasse, KlunkerGarten in der Horstwirtschaft e.V., Nachbarschaftsgarten Wiecker Strasse, Garten der Begegnung, Peace of Land, Pflanz Was Vattenfall—Gemeinschaftsgarten Neue Gruenstrasse, prinzessinnengarten kollektiv berlin, Rote Beete, Schalottengarten, Spiel/Feld Marzahn, Vollguter Gemeinschaftsgarten, Gemeinschaftsgarten Wachsenlassen, Gartenarbeitsschule Tempelhof-Schoeneberg, Gartenarbeitsschule Friedrichshain-Kreuzberg. Thank to M. Penzel for support establishing and maintaining temperature stations in the gardens. Thank to B. Seitz, J. Herrmann, L. Neuerburg, J. Wedler, and L. Winker for collecting the vegetation data. Thank to A. Hiller and J. Herrmann for collecting and visualizing the spatial data. Thank to U. Sturm and the Museum für Naturkunde Berlin for project support. Thank to I. Kowarik, M. von der Lippe, C. Achilles, J. Felderhoff, and A.K. Gathof for supporting field work. Finally, we would like to thank the TUM Nexus Group for support and feedback throughout the project.
Supplementary Material
The Supplementary Material for this article can be found online at: https://www.frontiersin.org/articles/10.3389/fsufs.2022.826437/full#supplementary-material
References
Akbari, H., Kurn, D. M., Bretz, S. E., and Hanford, J. W. (1997). Peak power and cooling energy savings of shade trees. Energy Build. 25, 139–148. doi: 10.1016/S0378-7788(96)01003-1
Bivand, R., Keitt, T., and Rowlingson, B. (2018). Bindings for the “Geospatial” Data Abstraction Library. R package rgdal version 1.4-3.
Bivand, R., and Lewin-Koh, N. (2022). maptools: Tools for Handling Spatial Objects. CRAN R Proj. Available online at: https://cloud.r-project.org/web/packages/maptools/index.html (accessed March 22, 2022).
Bivand, R., and Rundel, C. (2021). rgeo: Interface to Geometry Engine—Open Source (‘GEOS'). R package version 0.5-5. Available online at: https://CRAN.R-project.org/package=rgeos
Bowler, D. E., Buyung-ali, L., Knight, T. M., and Pullin, A. S. (2010). Urban greening to cool towns and cities: a systematic review of the empirical evidence. Landsc. Urban Plan. 97, 147–155. doi: 10.1016/j.landurbplan.2010.05.006
Butterfield, K. L.. (2020). Neighborhood composition and community garden locations: the effect of thnicity, income, and education. Sociol. Perspect. 63, 738–763. doi: 10.1177/0731121420908902
Clarke, M., Davidson, M., Egerer, M., Anderson, E., and Fouch, N. (2019). The underutilized role of community gardens in improving cities' adaptation to climate change: a review. People Place Policy 12, 241–251. doi: 10.3351/ppp.2019.3396732665
Das Amt für Statistik Berlin-Brandenburg (2019). Statistiken. Bevölkerung. Available online at: https://www.statistik-berlin-brandenburg.de/ (accessed November 25, 2021).
Deutsche Welle (2020). Top 10: Germany′s Largest Cities. Available online at: https://www.dw.com/en/top-10-germanys-largest-cities/g-52632011 (accessed November 25, 2021).
Egerer, M. H., Lin, B. B., and Kendal, D. (2019a). Temperature variability differs in urban agroecosystems across two metropolitan regions. Climate 7, 1–18. doi: 10.3390/cli7040050
Egerer, M. H., Lin, B. B., Threlfall, C. G., and Kendal, D. (2019b). Temperature variability influences urban garden plant richness and gardener water use behavior, but not planting decisions. Sci. Total Environ. 646, 111–120. doi: 10.1016/j.scitotenv.2018.07.270
Ellis, K. N., Hathaway, J. M., Mason, L. R., Howe, D. A., Epps, T. H., and Brown, V. M. (2015). Summer temperature variability across four urban neighborhoods in Knoxville, Tennessee, USA. Theor. Appl. Climatol. 2015 127, 701–710. doi: 10.1007/S00704-015-1659-8
Fox, J., and Weisberg, S. (2019). An R Companion to Applied Regression, Third Edition. London: Sage.
Google Inc. (2021). Google Earth. Available online at: www.earth.google.com (accessed December 01, 2020).
Klompmaker, J. O., Janssen, N. A. H., Bloemsma, L. D., Gehring, U., Wijga, A. H., van den Brink, C., Lebret, E., Brunekreef, B., and Hoek, G. (2019). Residential surrounding green, air pollution, traffic noise and self- perceived general health. Environ. Res. 179, 108751. doi: 10.1016/J.ENVRES.2019.108751
Kottek, M., Grieser, J., Beck, C., Rudolf, B., and Rubel, F. (2006). World Map of the Köppen-Geiger climate classification updated. Meteorol. Zeitschrift 15, 259–263. doi: 10.1127/0941-2948/2006/0130
Lehmann, S.. (2014). Low carbon districts: Mitigating the urban heat island with green roof infrastructure. City Cult. Soc. 5, 1–8. doi: 10.1016/J.CCS.2014.02.002
Li, W., Cao, Q., Lang, K., and Wu, J. (2017). Linking potential heat source and sink to urban heat island: Heterogeneous effects of landscape pattern on land surface temperature. Sci. Total Environ. 586, 457–465. doi: 10.1016/J.SCITOTENV.2017.01.191
Lin, B. B., and Egerer, M. H. (2020). Global social and environmental change drives the management and delivery of ecosystem services from urban gardens: a case study from Central Coast, California. Glob. Environ. Chang. 60, 1–10. doi: 10.1016/j.gloenvcha.2019.102006
Lin, B. B., Egerer, M. H., Liere, H., Jha, S., Bichier, p., and Philpott, S. M. (2018). Local- and landscape-scale land cover affects microclimate and water use in urban gardens. Sci. Total Environ. 610–611, 570–575. doi: 10.1016/j.scitotenv.2017.08.091
Litardo, J., Palme, M., Borbor-Cordova, M., Caiza, R., Macias, J., Hidalgo-Leon, R., and Soriano, G. (2020). Urban Heat Island intensity and buildings' energy needs in Duran, Ecuador: simulation studies and proposal of mitigation strategies. Sustain. Cities Soc. 62, 102387. doi: 10.1016/J.SCS.2020.102387
Lobell, D. B., Bonfils, C. J., Kueppers, L. M., and Snyder, M. A. (2008). Irrigation cooling effect on temperature and heat index extremes. Geophys. Res. Lett. 35. (9705). doi: 10.1029/2008GL034145
Manteghi, G., Bin Limit, H., and Remaz, D. (2015). Water bodies an urban microclimate: a review. Mod. Appl. Sci. 9, 1–12. doi: 10.5539/MAS.V9N6P1
Mohajerani, A., Bakaric, J., and Jeffrey-Bailey, T. (2017). The urban heat island effect, its causes, and mitigation, with reference to the thermal properties of asphalt concrete. J. Environ. Manage. 197, 522–538. doi: 10.1016/J.JENVMAN.2017.03.095
Oke, T. R.. (1973). City size and the urban heat island. Atmos. Environ. 7, 769–779. doi: 10.1016/00046981(73)90140-6
Oke, T. R., Johnson, G. T., Steyn, D. G., and Watson, I. D. (1991). Simulation of surface urban heat islands under ‘ideal' conditions at night part 2: diagnosis of causation. Boundary-Layer Meteorol. 56, 339–358. doi: 10.1007/BF00119211
Pebesma, E.. (2018). Simple features for R: standardized support for spatial vector data. R J. 10, 439–446. Available online at: http://pebesma.staff.ifgi.de/RJwrapper.pdf
Potchter, O., Cohen, p., and Bitan, A. (2006). Climatic behavior of various urban parks during hot and humid summer in the mediterranean city of Tel Aviv, Israel. Int. J. Climatol. 26, 1695–1711. doi: 10.1002/JOC.1330
Pyrgou, A., Santamouris, M., and Livada, I. (2019). Spatiotemporal analysis of diurnal temperature range: effect of urbanization, cloud cover, solar radiation, and precipitation. Climate 7, 89. doi: 10.3390/cli7070089
QGIS Development Team (2018). QGIS Geographic Information System: Open Source Geospatial Foundation. Available online at: http://qgis.osgeo.org (accessed December 27, 2019).
Qiu, G., Yu, Li, H., Yong, Zhang, Q., Tao, Chen, W., Liang, X., and Li, X. (2013). Effects of evapotranspiration on mitigation of urban temperature by vegetation and urban agriculture. J. Integr. Agric. 12, 1307–1315. doi: 10.1016/S2095-3119(13)60543-2
Quanz, J. A., Ulrich, S., Fenner, D., Holtmann, A., and Eimermacher, J. (2018). Micro-scale variability of air temperature within a local climate zone in Berlin, Germany, during summer. Climate 6, 5. doi: 10.3390/cli6010005
Rijnders, E., Janssen, N. A. H., van Vliet, p. H. N., and Brunekreef, B. (2001). Personal and outdoor nitrogen dioxide concentrations in relation to degree of urbanization and traffic density. Environ. Health Perspect. 109, 411–417. doi: 10.1289/EHP.01109S3411
Robine, J. M., Cheung, S. L. K., Le Roy, S., Van Oyen, H., Griffiths, C., Michel, J. p., and Herrmann, F. R. (2008). Death toll exceeded 70,000 in Europe during the summer of 2003. C. R. Biol. 331, 171–178. doi: 10.1016/J.CRVI.2007.12.001
Rost, A. T., Liste, V., Seidel, C., Matscheroth, L., Otto, M., Meier, F., and Fenner, D. (2020). How cool are allotment gardens? A case study of nocturnal air temperature differences in Berlin, Germany. Atmosphere. 11, 500. doi: 10.3390/atmos11050500
Senatsverwaltung für Stadtentwicklung und Umwelt (2013). Umweltgerechtigkeit Berlin. Umweltgerechtigkeit Berlin. Available online at: https://www.berlin.de/umweltatlas/mensch/umweltgerechtigkeit/ (accessed March 16, 2022).
Senatsverwaltung für Stadtentwicklung und Umwelt (2016). Versorgung mit öffentlichen, wohnungsnahen Grünanlagen. Umweltatlas. Available online at: https://www.stadtentwicklung.berlin.de/umwelt/umweltatlas/index.shtml (accessed December 11, 2019).
Smith, J. p., Meerow, S., and Turner, B. L. (2021). Planning urban community gardens strategically through multicriteria decision analysis. Urban For. Urban Green. 58, 126897. doi: 10.1016/J.UFUG.2020.126897
Smith, V. M., Greene, R. B., and Silbernagel, J. (2013). The social and spatial dynamics of community food production: a landscape approach to policy and program development. Landsc. Ecol. 28, 1415–1426. doi: 10.1007/S10980-013-9891-Z
Stewart, I. D.. (2011). A systematic review and scientific critique of methodology in modern urban heat island literature. Int. J. Climatol. 31, 200–217. doi: 10.1002/joc.2141
Suomi, J., and Käyhkö, J. (2012). The impact of environmental factors on urban temperature variability in the coastal city of Turku, SW Finland. Int. J. Climatol. 32, 451–463. doi: 10.1002/JOC.2277
Taha, H.. (1997). Urban climates and heat islands: albedo, evapotranspiration, and anthropogenic heat. Energy Build. 25, 99–103. doi,:10.1016/S.0378-7788(96)00999-1
von der Lippe, M., Buchholz, S., Hiller, A., Seitz, B., and Kowarik, I. (2020). CityScapeLab Berlin: a research platform for untangling urbanization effects on biodiversity. Sustainability 12, 2565. doi: 10.3390/su12062565
Wickham, H.. (2021). forcats: Tools for Working with Categorical Variables (Factors). CRAN R Proj. Available online at: https://cran.r-project.org/web/packages/forcats/index.html (accessed March 22, 2022).
Wickham, H., Girlich, M., and Ruiz, E. (2022). dbplyr: A “dplyr” Back End for Databases. Comprehensive R Archive Network (CRAN). Available online at: https://CRAN.R-project.org/package=dbplyr.
Wolch, J. R., Byrne, J., and Newell, J. p. (2014). Urban green space, public health, and environmental justice: the challenge of making cities “just green enough. Landsc. Urban Plan. 125, 234–244. doi: 10.1016/j.landurbplan.2014.01.017
Keywords: urban green infrastructure, urban agriculture, temperature variability, evapotranspiration, microclimate
Citation: Nolte AC, Buchholz S, Pernat N and Egerer M (2022) Temporal Temperature Variation in Urban Gardens Is Mediated by Local and Landscape Land Cover and Is Linked to Environmental Justice. Front. Sustain. Food Syst. 6:826437. doi: 10.3389/fsufs.2022.826437
Received: 30 November 2021; Accepted: 24 March 2022;
Published: 29 April 2022.
Edited by:
Sören Köpke, University of Kassel, GermanyReviewed by:
Elena Lioubimtseva, Grand Valley State University, United StatesJaroslav Hofierka, Pavol Jozef Šafárik University in Košice, Slovakia
Copyright © 2022 Nolte, Buchholz, Pernat and Egerer. This is an open-access article distributed under the terms of the Creative Commons Attribution License (CC BY). The use, distribution or reproduction in other forums is permitted, provided the original author(s) and the copyright owner(s) are credited and that the original publication in this journal is cited, in accordance with accepted academic practice. No use, distribution or reproduction is permitted which does not comply with these terms.
*Correspondence: Monika Egerer, monika.egerer@tum.de