- 1CIRAD, UPR AIDA, Montpellier, France
- 2AIDA, Univ Montpellier, CIRAD, Montpellier, France
- 3CIRDES-USPAE, Bobo-Dioulasso, Burkina Faso
- 4UMR Sad-Apt, INRAE, AgroParisTech, Université Paris-Saclay, Thiverval-Grignon, France
Designing innovative cropping systems is an active field of agricultural research challenged by the agroecological transition. One of the challenges is to adapt cropping systems to the diversity of farms and contexts. For instance, in the cotton production zone of Burkina Faso differences between farm resources, agricultural situations and agronomic constraints have resulted in a wide range of farming systems. In this context, to break with the trend toward cotton production, we co-designed eight legume-based innovative cropping systems (ICS) likely to meet the objectives sought and the constraints faced by a wide range of local farmers, thus constituting a “basket of options”. Our approach was to enable each farmer to choose the option they considered best suited to their conditions. To that end, the ICSs were implemented and discussed with farmers in participatory prototyping trials. After one season of co-evaluating the different ICSs, the farmers taking part in the co-evaluation were able to test an ICS on their own farm, by choosing and adapting one of the options. Thirty-nine farmers out of seventy-three chose an ICS to test. They were asked the reasons for their choice. Their selection criteria were analyzed in relation to comments made during collective activities organized in the participatory prototyping trials. To complete this analysis, we built an expert-based farming system typology and a statistical typology based on data collected in a rural household multi-indicator survey (RHoMIS) of 63 farms participating in this study. The two farming system typologies were compared, and the relationships between farming system types and the ICS tested on the farm were analyzed. We found that farmers did not really base their choice on their farming system. Rather, they used a wide range of criteria that varied from farmer to farmer, and they were influenced by what they had learned during the collective activities organized in the participatory prototyping trials.
Introduction
As defined by Altieri (2002), agroecology calls for the design of agricultural systems that (i) can be adapted to social and ecological uncertainties; (ii) are sustainable and resilient; and (iii) are based on the use of local resources and knowledge. It is therefore increasingly necessary to adapt techniques to local problems and to farmers' specific conditions. As each farmer is unique, with their specific means, knowledge, history, and socio-ecological context, there is no “silver bullet” (Meynard et al., 2012). The challenge is particularly acute in West Africa, where most farmers are smallholders with highly variable characteristics, particularly access to land, labor, equipment and knowledge, along with soil and climatic conditions, social rules, and access to cash flow (Tittonell and Giller, 2013).
In response, agronomists have gradually developed approaches that adapt technologies to the diverse needs of farmers based, for example, on farming system types (Landais, 1996; Touré et al., 2021). Farming system typologies provide simplified representations of farming system diversity, by grouping those that share the most uniform characteristics. The choice of characteristics on which the typology is built varies greatly from one typology to another, depending on its objectives (Alvarez et al., 2018; Tittonell et al., 2020). As typologies help in understanding and describing farm diversity, they can be used by development agents or researchers to target solutions for the problems encountered (Tittonell et al., 2010; Kuivanen et al., 2016), and to identify best-fit technologies (Giller et al., 2011). However, typologies involve a delicate trade-off between generic types that enable easy classification of farmers and require few exclusions, but make it difficult to find an option suiting all proponents of one type, and more precise types that exclude many specific cases (Descheemaeker et al., 2019).
Moreover, type-specific technical packages are often prescriptive and not flexible enough to be adopted by small-scale farmers (Tittonell and Giller, 2013; Ronner et al., 2017). An emerging way of overcoming this problem is to propose a variety of flexible technical options, sometimes called a “basket of options”, from which each farmer can choose the system that best fits their own conditions (Descheemaeker et al., 2019; Ronner et al., 2021). This participatory research approach assumes that farmers are in the best position to know what is most suitable for their specific situation, and has the advantage of avoiding the need for agronomists to develop solutions adapted to each possible situation (Ronner et al., 2021).
This was the approach used in our study. In order to support farmers in the agroecological transition, several innovative cropping systems (ICSs) combining different legume species with a variety of local major crops were co-designed through on-farm innovation tracking carried out in the area, and participatory workshops organized in two communities. These various ICSs were then tested and collectively evaluated with a wide range of farmers in each community (Périnelle et al., 2021). After one season of co-evaluation of the different ICSs, each farmer was individually supported to test the option they found most interesting on their own farm.
As highlighted by Ronner et al. (2021), a knowledge gap remains on how to present the various technical options and how to support farmers in their selection of the options that are most relevant to them. The objective of this research was to propose and test an innovative approach to understand farmers' selection process and to help each farmer in selecting a relevant ICS to be tested and adapted on their own farm.
Materials and Methods
Study Area
In this region of Burkina Faso, soil depletion and reduced productivity are issues increasingly being faced by farmers as it is often the case in West African cotton-production zones (Ripoche et al., 2015). This soil depletion is linked with a high land pressure (Jahel et al., 2017), a relatively low crop diversity (mainly maize-cotton rotation), and a limited access to fertilizers (Coulibaly et al., 2012).
The vast majority of farmers in the cotton production zone of Burkina Faso are smallholders. The farming systems there primarily produce cotton (Gossypium hirsutum), but also cereals such as maize (Zea mays) and sorghum (Sorghum bicolor) (Coulibaly et al., 2012). Peanut (Arachis hypogaea) and cowpea (Vigna unguiculata) are the main legumes cropped, but on very limited areas and in very small quantities compared to other regions of Burkina Faso (Dabat et al., 2012). Cropping systems in the cotton production zone are based on short rotations (cotton-maize), animal traction, and the use of fertilizers and herbicides. The Société Burkinabé des Fibres Textiles (SOFITEX) influences the market and plays an important economic role in determining farmers' income. SOFITEX, which contracts with cotton farmers, supplies them with seeds and fertilizer for cotton and maize production through campaign credits repaid with the harvest (Andrieu et al., 2015).
Nomadic Fulani were traditionally the livestock herders in this area, but starting in the 1960s mixed crop-livestock systems using animal traction expanded into the cotton zone encouraged by SOFITEX, to facilitate tillage. Then, from the 1990s, crop farmers gradually invested in livestock (Andrieu et al., 2015), with draft cattle generally kept on the farm and fed with crop residues. Sometimes other livestock is kept by household members, or more often entrusted to Fulani herders, who are now mostly sedentary, but for them livestock raising is now mostly a marginal activity. Many crop farmers who have become wealthy from cotton production are able to invest in livestock to reduce the risks associated with soil degradation and to diversify their activity (Andrieu et al., 2015). Fulani herders raise cattle and grow cereals, mainly for household consumption, using the residues as cattle fodder. They rarely grow cotton and do not use mineral fertilizers, as the organic manure produced by their livestock is largely sufficient to fertilize their staple crops (Vall et al., 2017).
According to several studies conducted in the cotton production zone of Burkina Faso (Vall et al., 2006, 2017; Andrieu et al., 2015), farming systems diversity can be captured through the degree of livestock and cropping activities, respectively reflected in the number of cattle and the cultivated area. They divide farmers into three main types: crop farmers, crop-livestock farmers and livestock farmers. Even though farm structures have evolved since the establishment of these typologies, they are still used by researchers and advisors in the area, by adapting threshold values between types, as these two components (crop and livestock) remain the most discriminant variables of local farming systems.
Following our previous study (Périnelle et al., 2021), two communities in two different municipalities in Tuy Province were selected for further participatory activities: Boni and Founzan, both in Tuy province. These communities were selected mainly because literature detailing their farming systems was already available, and connections with community leaders and farmers had already been established (Coulibaly et al., 2012). Both communities are part of the same agroecosystem in the cotton production zone. However, Boni is located close to the cotton processing plant, so cotton is very important for farmers, while Founzan is located close to a dam, so farmers produce a wider range of products, including fish and irrigated vegetables.
Implementing Participatory Prototyping Trials in Each Community
In the two selected communities, various innovative cropping systems were set up in participatory prototyping trials (PPTs) in 2017, integrating different legume species and different types of integration (rotation, relay, intercropping, intra-annual succession) with sorghum, maize, or cotton. The range of ICSs was chosen to respond to a range of different functions, and to meet the expectations of the main types of farmers in the area (crop, crop-livestock, and livestock farmers). In order to ensure that the systems met farmers' criteria, they were co-designed based on: (i) on-farm innovation tracking conducted throughout Tuy province (systems 1, 2, 3,4, 5, and 7, Table 1) (Périnelle et al., 2021), or (ii) farmers' criteria expressed during participatory workshops organized prior to implementing PPTs in each village (systems 6 and 8, Table 1). All the implemented ICSs were new to the participating farmers.
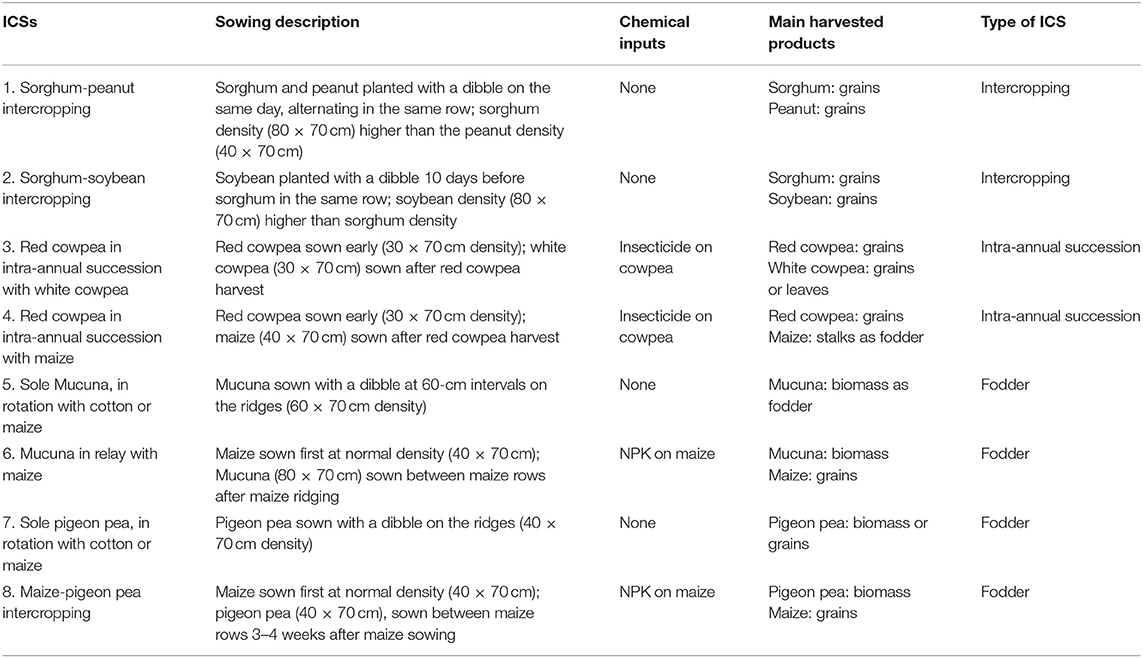
Table 1. Description of the 8 ICSs proposed to farmers as implemented in the PPT after soil preparation by animal traction (ridges spaced 70 cm apart) and application of glyphosate to the whole plot.
One PPT was set up in each community, both managed by the research team, comprising one researcher and one technician. The PPTs were set up to allow farmers to observe and compare the various cropping systems and to be used as a support for debate and co-evaluation with farmers. The layout of the PPTs was the same at the two locations and was organized to display a variety of cropping systems, without randomized replications, but organized to facilitate comparisons. Each PPT was divided into 18 plots (each covering 400 m2) containing either an ICS, or peanut, cowpea, soybean, sorghum, maize, or cotton monocultures. Fertilizers were applied at typical rates for the region (150 kg/ha of NPK 14-23-14 and 50 kg/ha of urea 46% on cotton and maize, none on the other crops). The land was prepared by plowing and ridging with animal traction in compliance with local practices.
During “field days”, farmers were invited to collectively discuss and evaluate each PPT plot. In each community, a contact person (in both cases a literate farmer from the community known by the research team) was tasked with inviting between 20 and 30 farmers representing the different local types of farmers (crop farmers, livestock farmers and mixed crop-livestock farmers) of different ages and gender. After the first field day, a few additional farmers joined the group after having heard of it from other participants. In all, 73 farmers took part in at least one field day. Two field days were organized on the PPT in each community. The first field day was held in August 2017, 3–4 weeks after sowing, and was used to present the trial: each plot was described and the farmers asked questions, but did not evaluate the ICSs. The second field day was held in September 2017, just before harvest, and was used to ascertain the farmers' evaluation of the ICSs. Both field days started with an explanation of the reason for holding the day. Then, each ICS was examined plot by plot. On the second field day, to obtain the farmers' evaluations, the researcher asked the farmers what they liked or disliked about the ICS they saw before them, and what they would do to improve it. The questions were purposely open to avoid influencing the farmers' responses, and the farmers were encouraged to react to each other's comments. The farmers' discussions were recorded and minutes were taken. The recording and the minutes were subsequently discussed between the researcher and the technician and compiled. The compilation was used to describe the criteria used by the farmers to assess the ICSs during the field days.
A Basket of Eight Options
Eight ICSs were proposed to the farmers (Table 1): (i) sorghum-peanut intercropping; (ii) sorghum-soybean intercropping; (iii) red cowpea (Vigna unguiculata) in intra-annual succession with white cowpea; (iv) red cowpea in intra-annual succession with maize; (v) Sole Mucuna (Mucuna pruriens); (vi) Mucuna relayed with maize; (vii) sole pigeon pea (Cajanus Cajan); (viii) maize-pigeon pea intercropping (Table 1). ICSs showing similarities (species involved and organization of the species mixture, use made of production) were grouped in the same “type of ICS” (Table 1, column “type of ICS”) to facilitate statistical comparisons between them.
Organization and Monitoring of Farmer Adaptation Trials
After harvesting the PPT, the farmers were given the opportunity to choose one ICS to test on their farm in “farmer adaptation trial”. The trials, which were conducted in 2018, were individual 0.25 ha plots, set up and managed by each farmer in parallel with their usual systems.
The seeds were provided free of charge, to make it easier to compare “farmer adaptation trial” and to be sure that seed availability would not be an obstacle to the implementation of the trial as some species were not common in the region. The quantities distributed were calculated on the basis of the seeding rates used in the PPTs, but the farmers were not obliged to reproduce the PPT rates and were free to adapt the management of the ICS. For the same reasons, fertilizer (NPK) was distributed for the maize sown with the pigeon pea or the Mucuna at the same rates as those used in the PPTs (i.e., 150 kg/ha of NPK 14-23-14). For the other crops, no fertilizer was distributed because none was used in the PPTs; however, the farmers were free to add fertilizer. No other chemicals were distributed.
In August 2018, seeds for the trial were given to each individual farmer. During the distribution, an open-ended questionnaire was submitted to each farmer to understand their choice of ICS. The main question was “Why did you choose this ICS?” Then, more questions were asked to better understand the reasons for their choice.
The farmers' selection criteria were analyzed based on their responses recorded in the semi-structured interviews. Our final interpretation of farmers' choice criteria was based on (i) the reasons for choosing an ICS given by the farmers during individual open-ended interviews, and (ii) the consistency between those reasons and their first collective assessment of a given ICS in the PPT during the field days.
The following cropping season, the farmers were able to choose an ICS again to test on their farm. They had the option of continuing the same ICS (“carry on with the same ICS”), choosing another ICS from the seven proposed options (the maize-pigeon intercropping was no longer proposed because no one chose it in 2018) (“change ICS”), or to stop doing a trial (“stop trial”). Farmers were individually asked their choice and the reason of their choice about 2 months before sowing the second trial.
Farming System Typology Used to Analyze Farmers' Choices
Farming system typologies were built on information collected through RHoMIS-type guided surveys created by the International Livestock Research Institute (ILRI). This type of survey targets rural households (one survey per household, or farm), and is designed to rapidly characterize a set of standard indicators including farm functioning, agricultural production, and wealth level (Hammond et al., 2017). RHoMIS surveys consist of core modules and additional optional modules. The survey was adapted to the present study by adapting the vocabulary used in the mandatory module, especially units of measure.
In the field, surveys were conducted by two trained interviewers, one in each village. Surveys were conducted with 63 of the 73 farmers who had taken part in the activities, including 37 of the 39 farmers who conducted a “farmer adaptation trial”. Of the 10 farmers missing, six could not be reached and four were excluded from the final analysis due to data inconsistency. All the farmers answering the questionnaire gave oral consent for the use of their data. Sixteen variables were extracted from these surveys to be compared with the farmers' choices (Table 2). The variables corresponded to the standard variables used to build farming system typologies (Berre et al., 2019).
A functional expert-based typology of farming systems was built on the criteria proposed by Vall et al. (2006). The authors classed farms in the cotton production zone of Burkina Faso according to three main types: crop farmers, crop-livestock farmers and livestock farmers, which were broken down into several sub-types. The typology proposed by Vall et al. (2006) used structural features of farming systems to reflect their functioning, especially in terms of crop and livestock system interactions, which is still relevant (Vall et al., 2017). We could therefore adapt the functional typology to the context of our study by updating the threshold numbers for cattle and cultivated areas. This “functional typology” used the number of cattle owned by the household (“cattle” variable) and the number of hectares cultivated (“land cultivated” variable) to class farmers in various types The updated threshold values were part of the results of the analysis and are explained in the results section (see section Links Between Farmers' Choices and Their Farming System Types).
A statistical typology was also built using seven variables from RHoMIS data: number of adult equivalent (AE), cultivated area (land_cultivated), number of adult equivalents according to the cultivated area (AE_per_Land_Cult); number of Tropical Livestock Unit (TLU); number of Tropical Livestock Unit according to the cultivated area (TLU_per_Land_Cult); proportion of cotton crop (land_cotton_prop); proportion of maize crop (land_maize_prop). The multivariate analysis chosen to build this statistical typology is a classic two-step method. First, a principal component analysis (PCA) was implemented on the discriminant variables to synthetize the diversity of the sample into two principal components of the PCA (data- or dimension-reduction process). In a second step, a hierarchical clustering (HC) analysis was carried out on these synthetic principal components. Both the PCA and the HC were implemented under (R Core Team, 2021) using the ade4 package (Thioulouse et al., 2018).
Results
The ICSs Selected by the Farmers
Of the 73 farmers who had taken part in at least one field day, 39 (53%) conducted a “farmer adaptation trial” (Figure 1). Intra-annual successions were less frequently selected (six farmers) than fodder (14 farmers) and intercropping (19 farmers). The sorghum-peanut intercropping system was by far the most frequently selected ICS (12 farmers). In the fodder type, no farmer selected maize-pigeon pea intercropping. The main reason given by the farmers was fear of competition between pigeon pea and maize. Mucuna as a relay crop with maize was less of a problem, as Mucuna was sown in the maize row, after the maize had been ridged.
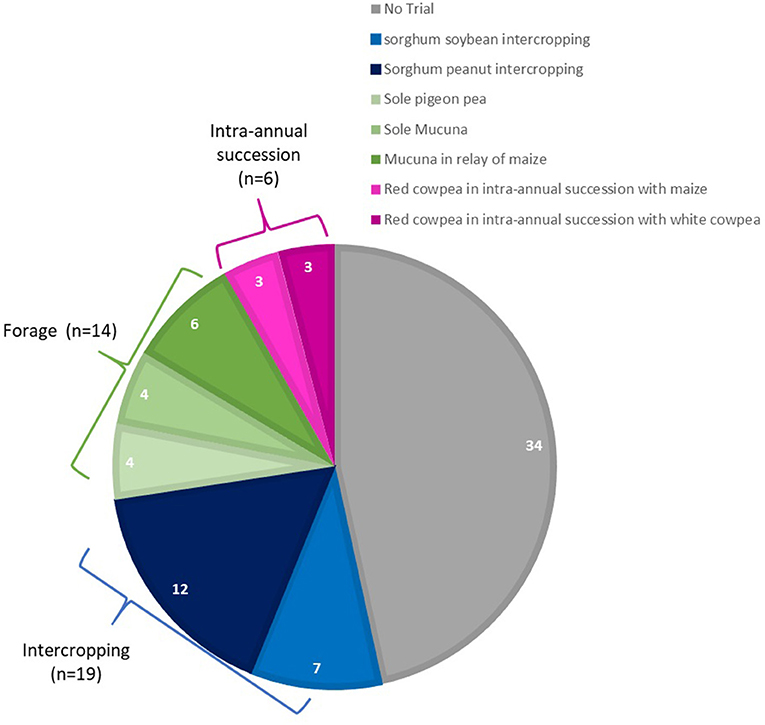
Figure 1. Distribution of the 73 farmers who took part in the field days, according to the choice of ICS implemented in the Farmer Adaptation Trials. The number farmers for each type of ICS (intra-annual successions in purple, fodder crops in green, sorghum-legume intercropping in blue) are indicated in brackets.
Farmers' Selection Criteria
For the ICSs implemented by the farmers, Table 3 details the criteria that they used to select their ICS.
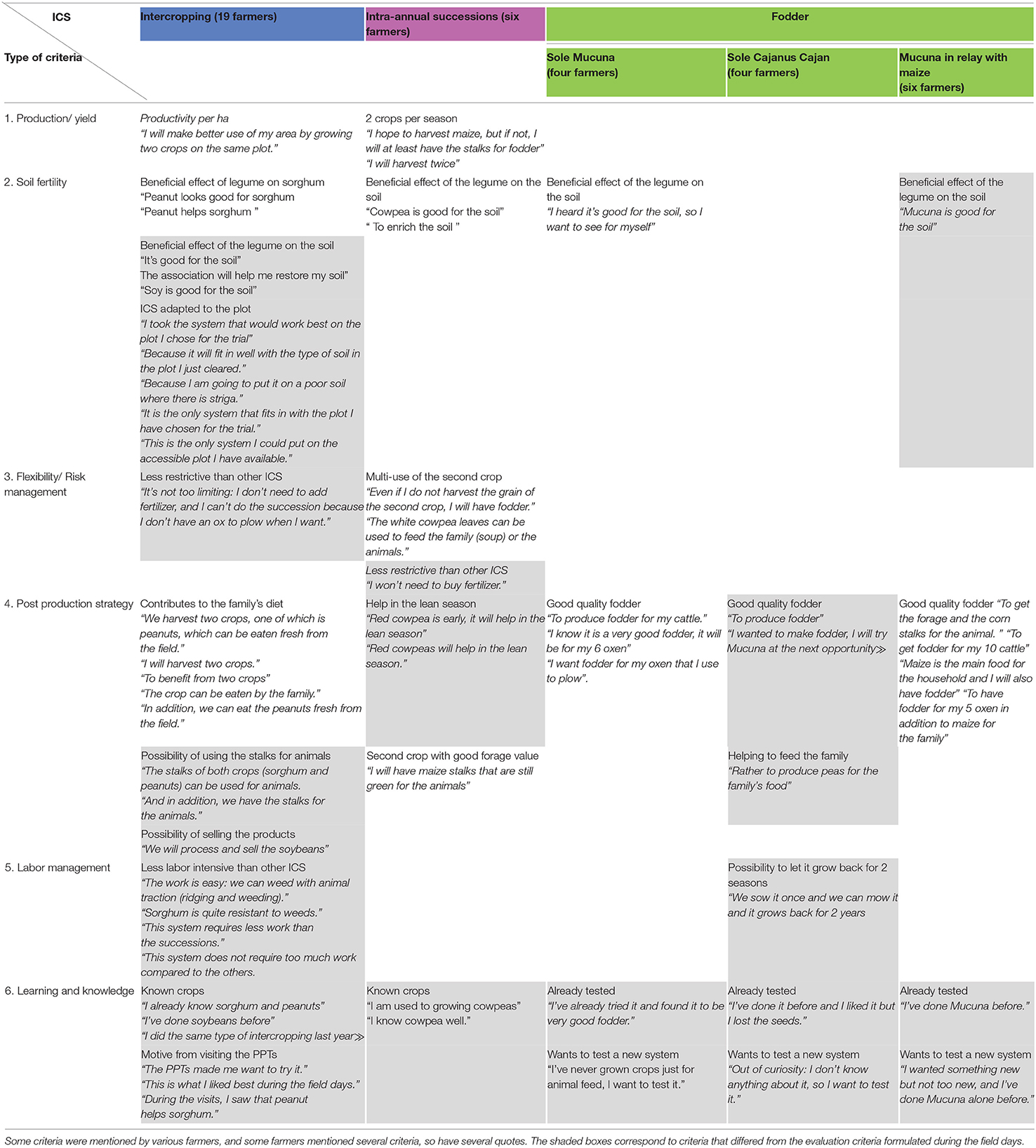
Table 3. For each type of ICS implemented by the farmers (in the columns), the selection criteria they declared in response to the open-ended question “Why did you choose this cropping system?” are presented according to the corresponding type of criteria (in the rows).
The criteria used to select the ICSs were classed in six categories (Table 3): 1. Production/yield; 2. Soil fertility; 3. Flexibility/Risk management; 4. Post-production strategy; 5. Labor management; 6. Learning and knowledge. Criterion types 1, 2, 3, 4, and 5 were the types of criteria used by the farmers during the field days (Périnelle et al., 2021). The new type of criterion, “learning and knowledge”, was related to the farmer's level of knowledge about the ICS, which they may have acquired through personal experience, or during the field days in the PPTs. The choice of an ICS may have been motivated by the fact that the farmer was used to grow the crop species making up the ICS, particularly in the case of associations and intra-annual successions. Conversely, some farmers wanted to test something very new, like Mucuna (fodder).
Differences were observed between the evaluation criteria used during the field days and the criteria used for the implementation of the ICSs (highlighted in the gray boxes in Table 3). Some differences were due to the nature of the criteria: an evaluation criterion may have been assessed positively or negatively by the farmers, whereas all the selection criteria given by the farmers referred to positive aspects of the selected ICS, if only in comparison with the other options. For instance, during the field days, intercropping systems were evaluated negatively in terms of work management, whereas the farmers who selected them said they were easier to manage than intra-annual successions (type 5 criterion): they consider that intra-annual succession requires twice as much work as sole crop, while intercropping would require an intermediate workload. The selection criteria appeared to be more diversified than the evaluation criteria used during the field days. For instance, some farmers selected an ICS because there was no need for fertilizer (type 3 criterion), whereas it was not mentioned during the field days.
The intercropping systems were the only ICSs chosen for all 6 types of criteria, including for criterion 5 “Labor Management”, which was not cited for the intra-annual successions, and by only one farmer for fodder (pigeon pea). Three farmers chose sorghum-peanut intercropping, with “labor management” as their main selection criterion. They considered that sorghum was not very susceptible to weeds, that ridging was possible and that intercropping required less work than intra-annual successions. These three farmers explained that seeing the intercropping systems in the participatory prototyping trials compared with monocrops motivated them (criteria 6): they noticed that sorghum intercropped with peanuts was better developed (taller, greener) than sorghum alone.
Several farmers said they chose their ICS based on the plot they planned to test: they first chose the plot, then chose the ICS that would work best on that plot, taking soil type and location into account.
Intra-annual successions were selected based on all criteria, except “Labor Management,” as the farmers considered that growing two crops doubled the amount of work. Moreover, most of the farmers agreed on the importance of tilling, or at least of scraping the soil between the two crops. Intra-annual successions were mainly chosen because two harvests were possible with a fairly low risk, since the biomass of the second crop could be used, and because the first crop was harvested early, and consequently helped in the lean season.
The selection criteria for the fodder crops were more varied than for the others, hence the separate presentation of the three options in Table 3. However, trends appeared for all three fodder options. The choice of the type of fodder was mainly based on the criterion (i) “Post production strategy,” as it was easy to produce good quality fodder (except for one farmer who chose pigeon pea to produce peas for family consumption), and (ii) “learning and knowledge”; Mucuna and pigeon pea are not very common in the region, and the farmers who chose to test them were curious to see how they fared in their plots. The “Soil fertility” criterion was cited by only one farmer, for whom fodder production was not the priority, and who therefore did not mention the “Post production strategy” criterion. This farmer chose Mucuna as a replacement for maize, even though he owned only one ox, as he wanted to plant a new crop that could help restore his soil fertility, while growing maize for family consumption. One farmer who had already tested pigeon pea for fodder decided to test it again because he understood that it is a biennial species that can be mowed 2 years running (“Labor Management” criterion).
One criterion was mentioned only by women: less fertilizer and animal traction required (“Flexibility/ Risk management” criterion) compared to the other options. These women did not have access to farm resources. One woman chose intercropping over intra-annual succession because she was concerned that she would not have access to oxen for plowing early enough for the succession, and the other chose red cowpea in intra-annual succession with white cowpea, mainly because red cowpea is harvested earlier than other food crops, and can consequently help in the lean season.
A comparison of the selection criteria for the three types of ICS showed that each type had specific advantages for the farmers who chose them. According to the farmers, the different types of ICSs allowed them to solve different problems: fodder production for cattle (fodder), or productivity per ha (intercropping and intra-annual succession). In addition, the farmers pointed out that the different types of ICS were suited to different levels of soil fertility: Mucuna and pigeon pea as the sole crop being suitable for poor soils, sorghum-legume intercropping for moderately fertile soils, and Mucuna relayed with maize for richer soils.
Links Between Farmers' Choices and Their Farming System Types
According to the RHoMIS data, the cultivated area and the number of cattle owned varied widely between farms (Figure 2). However, it was possible to define thresholds for these two farming system characteristics that discriminated relatively uniform types and sub-types of farming systems (Figure 2; Table 4). There was no significant difference in the distribution of the sub-types between Boni and Founzan (Chi2 test) (Table 4).
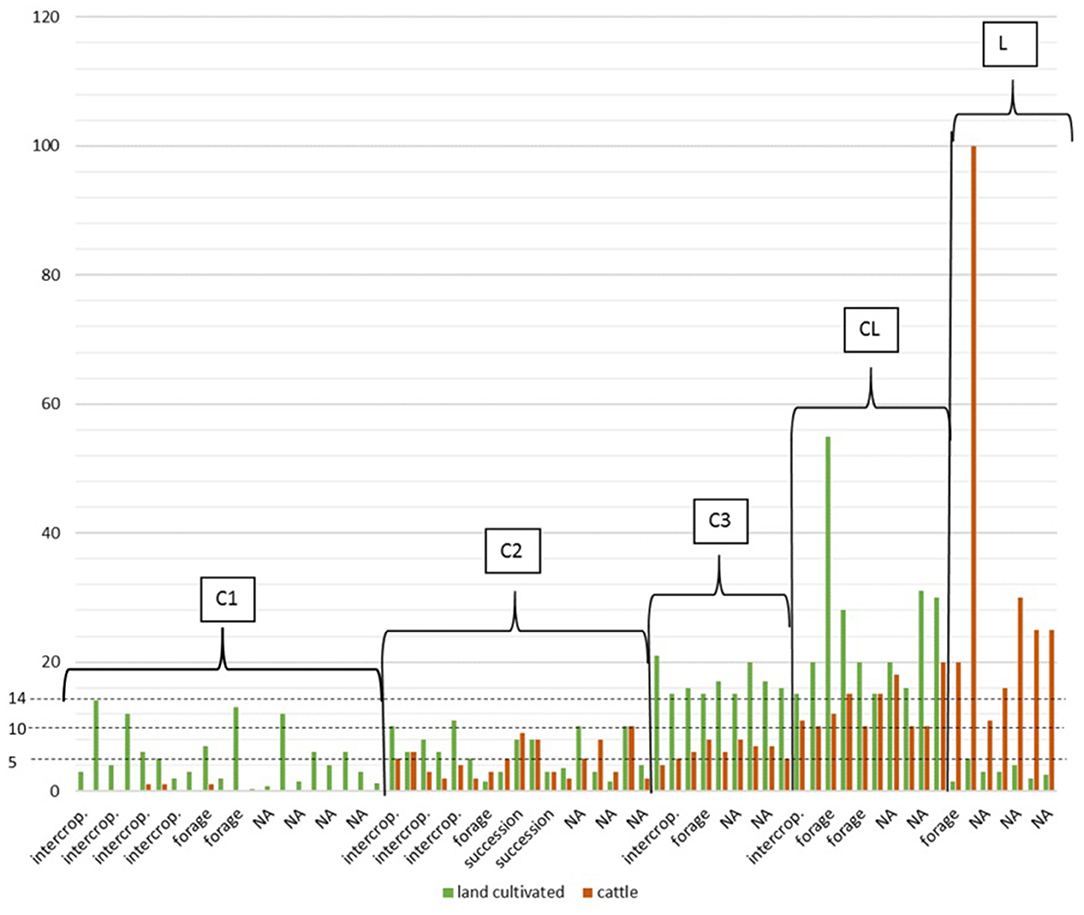
Figure 2. Distribution of the farming system types according to the number of ha cultivated (in green) and the number of cattle (in brown) on the y-axis, and the type of ICS tested in their farmer adaptation trials, or the choice not to take part in the farmer adaptation trials (NA), on the x-axis. The threshold values for cultivated area (≤5ha for livestock farmers, < or ≥ 14 for crop and crop-livestock farmers) and number of cattle (<10 for crop and crop-livestock farmers or ≥10 for livestock farmers) are shown on the y-axis.
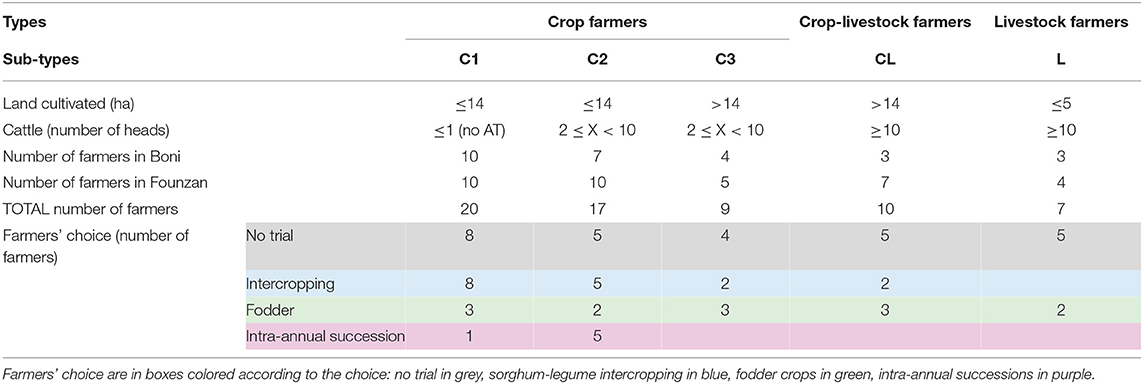
Table 4. Description of the types and sub-types of farmers based on the functional typology proposed by Vall et al. (2006) (no AT: no animal traction), and farmers' choice of ICS according to their type of farming systems.
The “crop farmers” type refers to farmers with fewer than 10 head of cattle, among which we differentiated those who had fewer than two (C1) (Table 4). Consequently, farmers classed as C1 did not have the two oxen required for animal traction, and consequently faced strong constraints for plowing and ridging as they depended on the availability of oxen belonging to other farmers and could not plow when they wanted. None had more than 14 ha of cultivated land. C2 farmers also had <14 ha of cultivated land but owned enough cattle for animal traction, so it was easier for them to plow and ridge their field for weed management. C3 farmers had more than 14 ha of cultivated land, but like the other crop farmers they owned fewer than 10 cattle. Farmers who owned more than 10 cattle were either “Crop-Livestock farmers” (those who cultivated > 14 ha (type CL), or “Livestock farmers” [those who cultivated <5 ha (type L)]. This number of cattle implied that, when they took care of the cattle themselves, a significant share of farm resources (especially labor, crop residues) was used for the cattle, but they had access to manure for their field. For all the crop-livestock farmers, owning animals meant a diversification of their production and therefore a distribution of risks. The livestock herders, who were Fulani herders, had specialized their production into cattle production and had very small cultivated land areas, all used for household consumption.
Table 4 also shows the number of farmers who made each choice (no trial, intercropping, fodder, and succession) according to their expert-based farming system type. There was no statistical difference in the distribution of choices for each farming system type at the 0.05 threshold (p-value = 0.233 with Fisher's exact test). However, a larger proportion of farmers who did not conduct a trial was observed among the farmers who owned the most livestock: half the crop-livestock farmers and over 70% of the livestock farmers did not conduct a farmer adaptation trial. Type A2 farmers were the most likely to conduct a trial. In addition, intra-annual successions were only found among small-scale farmers (A1 and A2), and more often among those who owned at least two head of cattle (A2), hence who had access to animal traction. Three farmers who declared they owned no cattle (A1) chose a fodder crop: among them, one farmer chose Mucuna relayed with maize and two chose pigeon pea, whose seeds could be consumed by the household. The distribution of choices by farmers with more than 14 ha was roughly the same, whether they owned fewer than 10 cattle (A3) or more than 10 (EA), with a major interest in fodder systems.
The multivariate analysis of the nine chosen variables clearly revealed three statistical farming system types (Figure 3). Type 1, called “subsistence-oriented” grouped small farms with a limited cultivated area (average of 5.6 ha), and limited livestock (average of 1.7 TLU) (Figure 3; Table 5). Type 2, called “cotton based”, had a much larger cultivated area (average of 18.3 ha) and type 3, called “livestock owners” had a much larger number of livestock units (average 29.6) (Figure 3; Table 5).
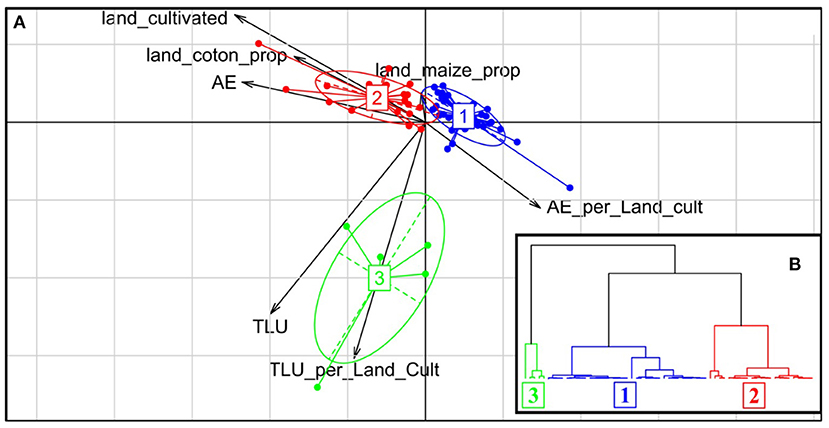
Figure 3. Graphical exploration of the Principal Component Analysis (PCA) and Hierarchical Clustering (HC) for 63 farms: (A) Projection of variables (arrows) and of farmers (colored points) according to their types on the PC1–PC2 plane, (B) Cluster dendogram.
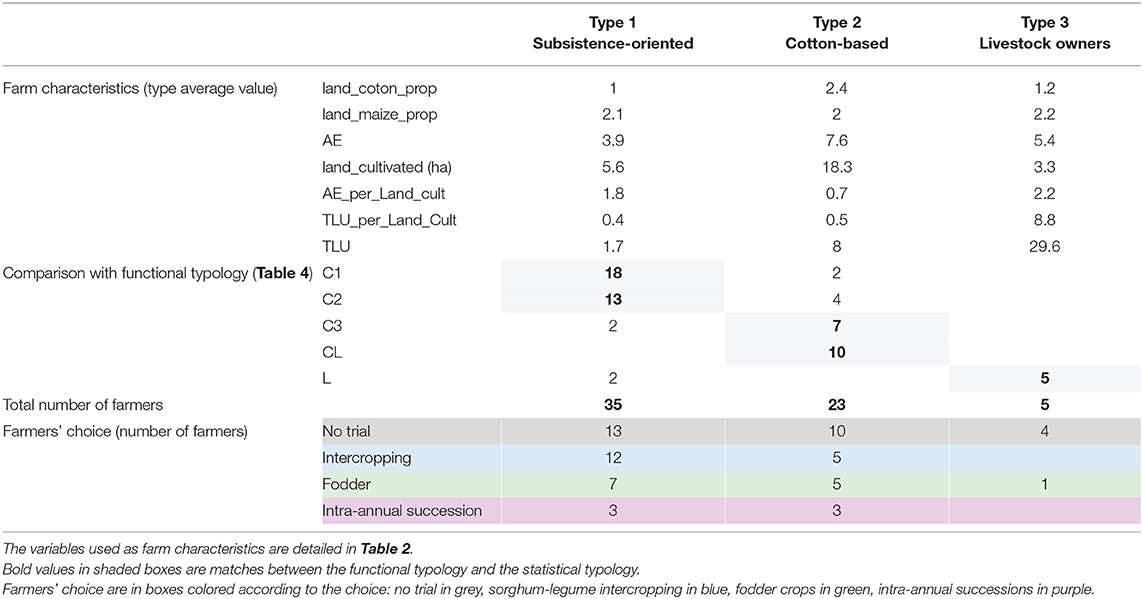
Table 5. Main characteristics of farming system types and type of SCI choices according to the statistical typology.
The statistical typology overlaps fairly well with the expert-based typology: most of the C1 and C2 farming systems belong to type 1, the C3 and CL farming systems belong mostly to type 2, and the L systems correspond more to type 3 (Table 5). In the same way as for the expert typology, a chi-square test did not reveal any significant effect of the farming system type (according to the statistical typology) on the choice of implementing a trial, or not, or on the choice of ICS (Table 5).
Farmers' Evaluation of Their ICS Choice
The following cropping season, out of the 39 farmers having tested an ICS in 2018, 23 continued the same type of ICS in 2019, because they were satisfied enough after having conducted the first trial. Of the 16 farmers who decided to change their type of ICS, six declared to be satisfied with the type of ICS chosen but wanted to try another type (Figure 4).
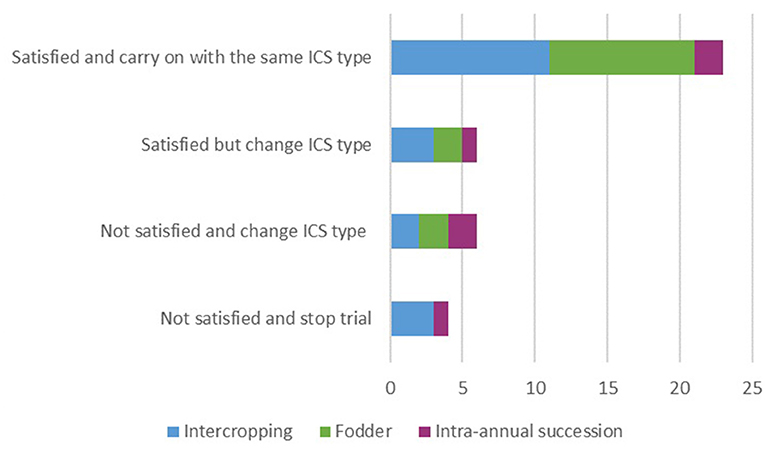
Figure 4. Distribution of the type of ICS tested in 2018 based on the evaluation of the ICS made by each farmer.
Discussion
A Wide Range of Farmers Involved
In this study, we used a participatory action research approach (Faure et al., 2010), seeking to help farmers belonging to a wide variety of farms to change their practices (introducing more legumes in their cropping systems). From a development point of view, we “targeted” and involved as many participants or “beneficiaries” as possible, while remaining able, from a research perspective, to monitor each one for a comprehensive analysis of the process. According to Phillips et al. (2014), who studied farmers' field schools, there are three ways to target farmers for action: (i) open targeting, i.e., open to all farmers, (ii) targeting through a selection process, with established criteria that beneficiaries must meet, or (iii) targeting an identified group, for example farmers belonging to a specific farming system type, as is often the case in development projects. Rather than targeting specific categories of farmers, we chose open targeting, where all activities were open to all interested farmers.
In each community, heterogeneous groups including farmers with different means of production, farmers for whom the main activity was livestock, and women who generally had very limited access to means of production, were called upon. Such a variety of participants was a source of enrichment for the process through the exchanges that took place between the different participants both during and beyond the field day. For instance, a crop farmer who tested a fodder type of ICS may have been influenced by livestock farmers during the collective activities. In general, the discussions that took place during the collective activities in the PPTs were richer when they took place between farmers with different types of farming systems. As shown by Dolinska and d'Aquino (2016), heterogeneous peer networks are conducive to innovation.
Even though all farming system types were targeted, there was still selection based on farmers' motivation and interests in taking part, and arising from the method used to invite them (via the contact person's network). As a result, some farmers did not participate in the process: this was particularly true of farmers who were marginalized, including women and minorities. For instance, relatively few Fulani herders took part in the activities. This may have been because they were less interested in cropping legumes (small cultivated areas, access to organic manure), or because they were often left out of the community. Also, six of the nine women who took part in the collective activities did not have access to a plot to conduct a farmer adaptation trial, and found themselves excluded from the activity. Phillips et al. (2014) pointed out that the poorest or most marginalized individuals, particularly women, are often excluded from rural development activities, except when they are specifically targeted and when activities are tailored to suit them. Even women with equivalent access to productive assets as men are more likely to be excluded than men, one reason being that they do not belong to the same network as their male counterparts (Ragasa et al., 2013). However, with the appropriate support, being part of group working on agricultural innovation may be an opportunity to get less marginalized (Classen et al., 2008). In our case, to be more inclusive, it would have been necessary to work on the obstacles that limited the participation of vulnerable farmers, for example by facilitating their access to the necessary resources (land, animal traction, labor), which was not in the scope of this study.
The sample of farmers in this study was not strictly representative of the general population of farmers in the area, but all types of farmers were represented (Périnelle et al., 2021). In participatory research, researchers often choose the stakeholders they work with in order to ensure a certain representativeness of the study area, with the objective of being able to extrapolate their results to situations with similar characteristics (Faure et al., 2010). This was not the choice made in this study, as farmer motivation was the main condition for their participation. In our approach, it was the co-design process that could be extrapolated, rather than the ICSs.
A Variety of Selection Criteria Matured in the PPTs
We noted both convergences and divergences between the farmers' selection criteria for the choice of ICS and evaluation criteria expressed by farmers during the field days. The five criteria used for evaluation during the field days were also mentioned in their choice, and, half of the time, mentioned for the same reasons during field days and for the choice of ICS. On the other hand, some criteria were evaluated differently. For example, during the field days the amount of work was seen as a difficulty involved in intercropping, whereas in the choice leading to the farmer adaptation trials, several farmers asserted that intercropping required less work than intra-annual successions. Moreover, a new evaluation criterion emerged between the field days and the choice of an ICS that was related to the learning process during the collective activities in the PPTs. The collective activities gave the farmers the opportunity to mature their evaluation, by observing the cropping systems, and through exchanges between peers and with agronomists (Cooreman et al., 2018).
Indeed, during the collective activities in the PPTs, the farmers acquired a clearer picture of the relative advantages of each system and enriched their knowledge of the ecosystem services provided by legumes, such as soil fertility improvement or household food diversification (Kerr et al., 2007). Thus, even though farmers did not directly manage and work in the PPTs, several collective evaluation activities allowed them to acquire actionable knowledge on the various options. The acquisition of this knowledge was key, as they may not have chosen the option that best responded to their problem if they had not acquired enough knowledge to make an informed choice (Sumberg et al., 2003), which required a consistent and regular involvement of the farmers in the participatory process (Misiko, 2013). Moreover, thanks to collective debate around several systems, the farmers did not focus on a single technical solution. For instance, some farmers chose to try another ICS the second year, although they were satisfied with the system they chose the first year. While peer-to-peer exchanges are known to have an impact on farmers' perceptions, with learning related to diverse perspectives (Chantre et al., 2015; Cooreman et al., 2018), the exchanges and the learning process enabled by them are difficult to accurately track and evaluate, especially regarding their direct effect in changing practices (Aare et al., 2020).
Some of the farmers chose an ICS based on the knowledge they already had of it; either because they were familiar with the crop species involved, or because they were curious to try something new. In the first case, the farmers tried a new cropping system without requiring a lot of new knowledge and saw it as less risky. This choice may constitute an “antecedent” in the farmer's trajectory and allow a progressive change that can lead to a successful trajectory of change (Lamine, 2011). In the second case, the farmers chose ICSs that were very new to them, and therefore quite risky. In this case, exchanges between farmers helped to demystify the theoretically less known ICSs. While highly participatory approaches are sometimes criticized for promoting the status quo, for various reasons such as farmers' risk aversion (Abadi Ghadim, 1999), in our case the dynamics initiated during the field days helped the farmers to enter a trajectory of change, and may allow continuity of the trajectory beyond the support period (Mawois et al., 2019).
Links Between Farming System Types and Farmers' Choice of ICS
Links between farming system types and the farmers' choices (to conduct a trial or not, and which ICS to choose) were analyzed through two distinct farming system typologies, both RHoMIS survey data: (i) a functional expert-based typology built from a typology established in the same area and recently used (Andrieu et al., 2015; Vall et al., 2017), and (ii) a statistical typology built from classic farm characteristics used to build typologies (Berre et al., 2019). As highlighted by Berre et al. (2019), expert-based and statistical typologies are complementary for understanding farm diversity: expert-based approaches are more explanatory, whereas statistical typologies generally aim to extract types from a large number of data in a process that is intended to be more reproducible and less subjective than expert-based typologies. In our case, the two typologies built were consistent with each other, but no systematic links between farmers' choices and farming system types were found.
Our results showed that farmers with different farming system types may choose the same ICS for different reasons. The different legume species used in the ICSs can provide multiple services (Vanlauwe et al., 2019; Reckling et al., 2020). For instance, pigeon pea has a great carry-over effect on maize, and may be chosen by crop farmers for that, it can be mowed for fodder and be of interest to livestock farmers, or harvested at maturity for the grains, which then contribute to the diversification of the household diet. Mucuna can be grown on very poor soils, where it will help restore fertility, which is a crop farmer's criterion, and is a good quality fodder for livestock farmers.
The lack of a clear link between farmers' choices and the selected RHoMIS variables may be partially due to the fact that these data were based on self-reported interviews, which is a source of uncertainty, and to the limited number of farmers (37 having set up a trial). The number of farmers was restricted to enable the follow-up of each case, but usually RHoMIS surveys are used on larger samples, which makes the data more robust (Fraval et al., 2019). In our study, the use of RHoMIS surveys allowed us to obtain homogeneous indicators on heterogeneous farmers that were suited to our study's objectives. In addition, in the case of women and young people, who are not farm managers and only have the power to decide over their own plot (Gafsi, 2007), analyzing the links between the farming system characteristics and their choices has been probably disrupted by the fact that they made decisions based on their own access to farm resources (plot, labor, animal traction), rather than on their family farming system.
Studies on links between farmer choices and preferences and their farming system characteristics give highly variable results. For the choice of whether or not to conduct a trial, Sumberg et al. (2003) found no clear link between a farmer's socio-economic characteristics and their inclination to conduct a trial, which is consistent with our results. However, many studies show a link between farmers' preference of practices and their farming systems or socio-economic characteristics. For instance, Khatri-Chhetri et al. (2017) found links between farmers' preferences on Climate Smart Agriculture technologies, that are discussed with farmers but not implemented, and socio-economic characteristics; and Zongo et al. (2016) found links between cropping systems implemented by farmers and the farm's level of endowment in Burkina Faso. One difference between those two studies and ours is that there was no medium of exchange (the PPTs in our case) between agronomists and farmers that enabled the different types of actors to share observations and knowledge on innovative systems (Trompette and Vinck, 2009; Klerkx et al., 2010). Our results suggest that collective activities helped the farmers to mature their choice of ICS through collective learning. This learning occurred by seeing, comparing and debating the ICSs, but also by exchanging information with peers and with agronomists. For example, some farmers learned about the existence of a potential market for certain legumes during the collective activities, which influenced their choice, thinking beyond the limits of their farms.
A Co-designed Basket of Options to Meet Each Farmer's Specificities
A basket of options will only be relevant and effective if the options are sufficiently diverse and adapted to the variety of farmers involved (Ronner et al., 2021). We proposed a variety of eight ICSs with the objective of meeting the expectations of the different farmers in the community, and addressing the complexity of their conditions (Descheemaeker et al., 2019). As in the “option by context approach” we considered that the suitability of agronomic innovations depends on many factors (bioclimatic conditions, access to a market and value chain, farming practices, household characteristics, advisory services, etc.) that vary on a fine scale (Sinclair and Coe, 2019), and even from farmer to farmer. In order to increase ICS diversity, we diversified in particular (i) the production objectives to which the systems could respond (e.g., production of forage for cattle for “fodder” crops, productivity per ha for “intercropping” and “intra-annual successions”), (ii) the degree of novelty of the innovations with new species such as Mucuna and pigeon pea, or known species such as cowpea and peanut, and (iii) the design method of the ICS (inspired by on-farm innovation tracking or designed de novo). However, increasing the number of options may increase the proportion of them uninteresting for farmers (Ronner et al., 2021), as was the case of the pigeon pea/maize intercropping system that did not interest any farmer.
Beyond the relevancy of the options, the ability of the farmers to select the right one is crucial. To make a relevant choice, it is important that farmers acquire enough knowledge on the options through their participation. Many approaches other than ours use a central field trial as a medium for experimentation and information flow between agronomists and farmers, such as Farmer Field Schools used as an advisory tool (Duveskog et al., 2011), or mother and baby trials used for participatory varietal selection (Snapp, in Bellon and Reeves, 2002). In both cases, the level of farmer participation in trial design and implementation can vary from consultative to collaborative. Yet, Bakker et al. (2021), who studied the case of farmer field schools, showed that involving farmers in decision-making contributes to enabling a transformative learning process. In our approach, the farmers were involved from the beginning of the ICS co-design process: each ICS was discussed upstream during the participatory workshops and then, during the field days, the farmers actively took part in assessing the options by asking questions, debating between themselves and proposing ways of improvement. Throughout the process, the agronomists took into account suggestions made by the farmers. By involving the farmers from the beginning of the process and by establishing a relationship based on mutual trust, we fostered a transformative learning process (Reed, 2008).
Rather than deciding on the best practices to be implemented, farmers were supported in finding their own solutions, so that the solutions were as relevant as possible to their particular situation, even if they were not optimal from an “agronomist-expert's” point of view (Catalogna et al., 2018). Some farmers' criteria might not seem relevant to agronomists, for instance if they are not directly related to solving the farmer's problem (e.g., making the same choice as his neighbor, choosing a cropping system to obtain expensive seeds for free). However, as farmers have detailed knowledge of their local environment, situations, priorities, and evaluation criteria, they are better placed than an outsider to find innovative solutions that suit them, even if the latter has a detailed and appropriate knowledge of the biological and ecological processes in play (Sumberg et al., 2003). We also considered that there are different valid solutions for each problem and that a farmer may find some solutions more useful than others depending on their priorities, knowledge, means, and history (Darnhofer et al., 2010). In our approach, the agronomist no longer prescribes solutions, but supports the farmers in building their solutions. This change of posture belongs to a change of paradigm where farmers are involved in the design work and provided with design support tools (Salembier et al., 2018). This way of actively involving farmers can help to address the complexity of the issues related to the agroecological transition (Klerkx et al., 2010), but needs substantial institutional innovations in order to be scaled up (Nelson et al., 2019).
Conclusion
Our approach, consisting in setting up participatory prototyping trials and organizing collective activities, was relevant for helping farmers to make the best choice of an adapted innovative cropping system from a basket of options. Interactive learning, enabled by collective activities, helped each farmer to make an informed choice. By presenting a variety of ICSs, it was possible to work with a variety of farmers, with contrasting interests in potential alternative agricultural practices. Farmers' criteria and choices depend on many complex factors, including social norms, means of production, agronomic conditions, but also their personality, preferences, and skills, and these cannot all be diagnosed by agronomists. However, farmers can be supported in making their choices through collective activities and participatory trials.
Our approach, should now be applied to produce knowledge on participatory approaches, and for development purposes. In addition to furthering the participatory co-design process initiated in our study, it would be interesting to support farmers in adapting the management of the selected cropping system to their own conditions via a step-by-step design process, thus putting farmers in a position to design their own systems through an empowerment process.
Data Availability Statement
The raw data supporting the conclusions of this article will be made available by the authors upon request.
Ethics Statement
Ethical review and approval was not required for the study on human participants in accordance with the local legislation and institutional requirements. The patients/participants provided their written informed consent to participate in this study.
Author Contributions
AP did the field work (implementation of the various activities, data collection, and analysis) and was the main writer of the article. DB contributed to the data analysis and the realization of the figures. ES and J-MM supervised the whole study and contributed to the writing of the article. All authors contributed to the article and approved the submitted version.
Funding
This work was conducted with financial support from the SANTE (Sécurité Alimentaire et Nutritionnelle et Transition agro-écologique) project, part of the Inra-Cirad GloFoodS meta-program. The publication fees were granted by Cirad. The funders were not involved in the study design, collection, analysis, interpretation of data, the writing of this article or the decision to submit it for publication.
Conflict of Interest
AP, ES, and DB were employed by company Cirad.
The remaining author declares that the research was conducted in the absence of any commercial or financial relationships that could be construed as a potential conflict of interest.
Publisher's Note
All claims expressed in this article are solely those of the authors and do not necessarily represent those of their affiliated organizations, or those of the publisher, the editors and the reviewers. Any product that may be evaluated in this article, or claim that may be made by its manufacturer, is not guaranteed or endorsed by the publisher.
References
Aare, A. K., Cooreman, H., Garayoa, C. V., Arrieta, E. S., Bellostas, N., Marchand, F., et al. (2020). Methodological reflections on monitoring interactive knowledge creation during farming demonstrations by means of surveys and observations. Sustainability 12, 5739. doi: 10.3390/su12145739
Abadi Ghadim, A. (1999). A conceptual framework of adoption of an agricultural innovation. Agric. Econ. 21, 145–154. doi: 10.1016/S0169-5150(99)00023-7
Altieri, M. A. (2002). Agroecology: the science of natural resource management for poor farmers in marginal environments. Agric. Ecosyst. Environ. 93, 1–24. doi: 10.1016/S0167-8809(02)00085-3
Alvarez, S., Timler, C. J., Michalscheck, M., Paas, W., Descheemaeker, K., Tittonell, P., et al. (2018). Capturing farm diversity with hypothesis-based typologies: An innovative methodological framework for farming system typology development. PLoS ONE 13, e0194757. doi: 10.1371/journal.pone.0194757
Andrieu, N., Descheemaeker, K., Sanou, T., and Chia, E. (2015). Effects of technical interventions on flexibility of farming systems in Burkina Faso: Lessons for the design of innovations in West Africa. Agric. Syst. 136, 125–137. doi: 10.1016/j.agsy.2015.02.010
Bakker, T., Blundo Canto, G., Dugué, P., and de Tourdonnet, S. (2021). To what extent is the diversity of Farmer Field Schools reflected in their assessment? A literature review. J. Agric. Educ. Extens. 27, 381–401. doi: 10.1080/1389224X.2020.1858890
Bellon, M., and Reeves, J. (2002). Quantitative Analysis of Data from Participatory Methods in Plant Breeding. Available online at: http://citeseerx.ist.psu.edu/viewdoc/download?doi=10.1.1.119.3173&rep=rep1&type=pdf
Berre, D., Baudron, F., Kassie, M., Craufurd, P., and Lopez-Ridaura, S. (2019). Different ways to cut a cake: comparing expert-based and statistical typologies to target sustainable intensification technologies, a case-study in Southern Ethiopia. Exerc. Agric. 55, 191–207. doi: 10.1017/S0014479716000727
Catalogna, M., Dubois, M., and Navarrete, M. (2018). Diversity of experimentation by farmers engaged in agroecology. Agron. Sustain. Dev. 38:50. doi: 10.1007/s13593-018-0526-2
Chantre, E., Cerf, M., and Le Bail, M. (2015). Transitional pathways towards input reduction on French field crop farms. Int. J. Agric. Sustain. 13, 69–86. doi: 10.1080/14735903.2014.945316
Classen, L., Humphries, S., FitzSimons, J., Kaaria, S., Jiménez, J., Sierra, F., et al. (2008). Opening participatory spaces for the most marginal: learning from collective action in the Honduran Hillsides. World Dev. 36, 2402–2420. doi: 10.1016/j.worlddev.2008.04.007
Cooreman, H., Vandenabeele, J., Debruyne, L., Ingram, J., Chiswell, H., Koutsouris, A., et al. (2018). A conceptual framework to investigate the role of peer learning processes at on-farm demonstrations in the light of sustainable agriculture. Int. J. Agric. Extens. 6, 91–103.
Coulibaly, K., Vall, E., Autfray, P., Nacro, H., and Sedogo, M. (2012). Effets de la culture permanente coton-maïs sur l'évolution d'indicateurs de fertilité des sols de l'Ouest du Burkina Faso. Int. J. Biol. Chem. Sci. 6, 1069–1080. doi: 10.4314/ijbcs.v6i3.13
Dabat, M.-H., Lahmar, R., and Guissou, R. (2012). La culture du niébé au Burkina Faso : une voie d'adaptation de la petite agriculture à son environnement?, Growing cowpea in Burkina Faso: a pathway for small-scale farming contextual adaptation? Autrepart 62, 95–114. doi: 10.3917/autr.062.0095
Darnhofer, I., Bellon, S., Dedieu, B., and Milestad, R. (2010). Adaptiveness to enhance the sustainability of farming systems. A review. Agron. Sustain. Dev. 30, 545–555. doi: 10.1051/agro/2009053
Descheemaeker, K., Ronner, E., Ollenburger, M., Franke, A. C., Klapwijk, C. J., Falconnier, G. N., et al. (2019). Which options fit best? Operationalizing the socio-ecological niche concept. Exerc. Agric. 55, 169–190. doi: 10.1017/S001447971600048X
Dolinska, A., and d'Aquino, P. (2016). Farmers as agents in innovation systems. Empowering farmers for innovation through communities of practice. Agric. Syst. 142, 122–130. doi: 10.1016/j.agsy.2015.11.009
Duveskog, D., Friis-Hansen, E., and Taylor, E. W. (2011). Farmer field schools in Rural Kenya: a transformative learning experience. J. Dev. Stud. 47, 1529–1544. doi: 10.1080/00220388.2011.561328
Faure, G., Gasselin, P., Triomphe, B., Temple, L., and Hocdé, H. (2010). Innover avec les Acteurs du Monde Rural: La Recherche-Action en Partenariat. Versailles: Editions Quae.
Fraval, S., Hammond, J., Wichern, J., Oosting, S. J., De Boer, I. J.M., Teufel, N., et al. (2019). Making the most of imperfect data: a critical evaluation of standard information collected in farm household surveys. Exerc. Agric. 55, 230–250. doi: 10.1017/S0014479718000388
Gafsi, M. (2007). Exploitations Agricoles Familiales en Afrique de l'Ouest et du Centre: Enjeux, caractéristiques et éléments de gestion. Versailles: Editions Quae.
Giller, K. E., Tittonell, P., Rufino, M. C., van Wijk, M. T., Zingore, S., Mapfumo, P., et al. (2011). Communicating complexity: integrated assessment of trade-offs concerning soil fertility management within African farming systems to support innovation and development. Agric. Syst. 104, 191–203. doi: 10.1016/j.agsy.2010.07.002
Hammond, J., Fraval, S., van Etten, J., Suchini, J. G., Mercado, L., Pagella, T., et al. (2017). The Rural Household Multi-Indicator Survey (RHoMIS) for rapid characterisation of households to inform climate smart agriculture interventions: description and applications in East Africa and Central America. Agric. Syst. 151, 225–233. doi: 10.1016/j.agsy.2016.05.003
Jahel, C., Baron, C., Vall, E., Karambiri, M., Castets, M., Coulibaly, K., et al. (2017). Spatial modelling of agro-ecosystem dynamics across scales: A case in the cotton region of West-Burkina Faso. Agri. Syst. 157, 303–315. doi: 10.1016/j.agsy.2016.05.016
Kerr, R. B., Snapp, S., CHIRWA (deceased), M., Shumba, L., and Msachi, R. (2007). Participatory research on legume diversification with Malawian smallholder farmers for improved human nutrition and soil fertility. Exp. Agric. 43, 437–453. doi: 10.1017/S0014479707005339
Khatri-Chhetri, A., Aggarwal, P. K., Joshi, P. K., and Vyas, S. (2017). Farmers' prioritization of climate-smart agriculture (CSA) technologies. Agric. Syst. 151, 184–191. doi: 10.1016/j.agsy.2016.10.005
Klerkx, L., Aarts, N., and Leeuwis, C. (2010). Adaptive management in agricultural innovation systems: the interactions between innovation networks and their environment. Agric. Syst. 103, 390–400. doi: 10.1016/j.agsy.2010.03.012
Kuivanen, K. S., Alvarez, S., Michalscheck, M., Adjei-Nsiah, S., Descheemaeker, K., Mellon-Bedi, S., et al. (2016). Characterising the diversity of smallholder farming systems and their constraints and opportunities for innovation: a case study from the Northern Region, Ghana. NJAS Wageningen J. Life Sci. 78, 153–166. doi: 10.1016/j.njas.2016.04.003
Lamine, C. (2011). Transition pathways towards a robust ecologization of agriculture and the need for system redesign. Cases from organic farming and IPM. J. Rural Stud. 27, 209–219. doi: 10.1016/j.jrurstud.2011.02.001
Landais, E. (1996). Typologies d'exploitations agricoles. Nouvelles questions, nouvelles méthodes. Écon. Rurale 236, 3–15. doi: 10.3406/ecoru.1996.4819
Mawois, M., Vidal, A., Revoyron, E., Casagrande, M., Jeuffroy, M.-H., and Le Bail, M. (2019). Transition to legume-based farming systems requires stable outlets, learning, and peer-networking. Agron. Sustain. Dev. 39, 14. doi: 10.1007/s13593-019-0559-1
Meynard, J.-M., Dedieu, B., and Bos, A. P. (Bram) (2012). “Re-design and co-design of farming systems. An overview of methods and practices,” in Farming Systems Research into the 21st Century: The New Dynamic, eds I. Darnhofer, D. Gibbon, D., and B. Dedieu (Heidelberg: Springer Netherlands), 405–429.
Misiko, M. (2013). Dilemma in participatory selection of varieties. Agric. Syst. 119, 35–42. doi: 10.1016/j.agsy.2013.04.004
Nelson, R., Coe, R., and Haussmann, B. I.G. (2019). Farmer research networks as a strategy for matching diverse options and contexts in smallholder agriculture. Exp. Agric. 55, 125–144. doi: 10.1017/S0014479716000454
Périnelle, A., Meynard, J.-M., and Scopel, E. (2021). Combining on-farm innovation tracking and participatory prototyping trials to develop legume-based cropping systems in West Africa. Agric. Syst. 187, 102978. doi: 10.1016/j.agsy.2020.102978
Phillips, D., Waddington, H., and White, H. (2014). Better targeting of farmers as a channel for poverty reduction: a systematic review of Farmer Field Schools targeting. Dev. Stud. Res. 1, 113–136. doi: 10.1080/21665095.2014.924841
R Core Team (2021). R: A Language and Environment for Statistical Computing. Vienna: R Foundation for Statistical Computing.
Ragasa, C., Berhane, G., Tadesse, F., and Taffesse, A. S. (2013). Gender differences in access to extension services and agricultural productivity. J. Agric. Educ. Exten. 19, 437–468. doi: 10.1080/1389224X.2013.817343
Reckling, M., Bergkvist, G., Watson, C. A., Stoddard, F. L., and Bachinger, J. (2020). Re-designing organic grain legume cropping systems using systems agronomy. Eur. J. Agron. 112, 125951. doi: 10.1016/j.eja.2019.125951
Reed, M. S. (2008). Stakeholder participation for environmental management: a literature review. Biol. Conserv. 141, 2417–2431. doi: 10.1016/j.biocon.2008.07.014
Ripoche, A., Crétenet, M., Corbeels, M., Affholder, F., Naudin, K., Sissoko, F., et al. (2015). Cotton as an entry point for soil fertility maintenance and food crop productivity in savannah agroecosystems–Evidence from a long-term experiment in southern Mali. Field Crops Res. 177, 37–48. doi: 10.1016/j.fcr.2015.02.013
Ronner, E., Descheemaeker, K., Almekinders, C. J. M., Ebanyat, P., and Giller, K. E. (2017). Farmers' use and adaptation of improved climbing bean production practices in the highlands of Uganda. Agric. Ecosyst. Environ. (2017) 261, 186–200. doi: 10.1016/j.agee.2017.09.004
Ronner, E., Sumberg, J., Glover, D., Descheemaeker, K., Almekinders, C., Kuyper, T., et al. (2021). Basket of options: unpacking the concept. Outlook Agric. 50, 116–124. doi: 10.1177/00307270211019427
Salembier, C., Segrestin, B., Berthet, E., Weil, B., and Meynard, J.-M. (2018). Genealogy of design reasoning in agronomy: lessons for supporting the design of agricultural systems. Agric. Syst. 164, 277–290. doi: 10.1016/j.agsy.2018.05.005
Sinclair, F., and Coe, R. (2019). The options by context approach: a paradigm shift in agronomy. Exp. Agric. 55, 1–13. doi: 10.1017/S0014479719000139
Sumberg, J., Okali, C., and Reece, D. (2003). Agricultural research in the face of diversity, local knowledge and the participation imperative: theoretical considerations. Agric. Syst. 76, 739–753. doi: 10.1016/S0308-521X(02)00153-1
Thioulouse, J., Dray, S., Dufour, A.-B., Siberchicot, A., Jombart, T., and Pavoine, S. (2018). “Useful R functions and data structures,” in Multivariate Analysis of Ecological Data with Ade4, eds J. Thioulouse, S. Dray, A.-B. Dufour, A. Siberchicot, T. Jombart, and S. Pavoine (New York, NY: Springer), 13–28.
Tittonell, P., Bruzzone, O., Solano-Hernández, A., López-Ridaura, S., and Easdale, M. H. (2020). Functional farm household typologies through archetypal responses to disturbances. Agric. Syst. 178, 102714. doi: 10.1016/j.agsy.2019.102714
Tittonell, P., and Giller, K. E. (2013). When yield gaps are poverty traps: the paradigm of ecological intensification in African smallholder agriculture. Field Crops Res. 143, 76–90. doi: 10.1016/j.fcr.2012.10.007
Tittonell, P., Muriuki, A., Shepherd, K. D., Mugendi, D., Kaizzi, K. C., Okeyo, J., et al. (2010). The diversity of rural livelihoods and their influence on soil fertility in agricultural systems of East Africa – A typology of smallholder farms. Agric. Syst. 103, 83–97. doi: 10.1016/j.agsy.2009.10.001
Touré, A., Huat, J., and Rodenburg, J. (2021). Identifying farm-type specific entry points for innovations in weed management in smallholder inland-valley rice-based systems in West Africa. Int. J. Pest Manag. 0, 1–15. doi: 10.1080/09670874.2021.1959083
Trompette, P., and Vinck, D. (2009). Retour sur la notion d'objet-frontière. Rev. d'Anthropol. Connaissances 3, 5–27. doi: 10.3917/rac.006.0005
Vall, E., Marre-Cast, L., and Kamgang, H. J. (2017). Chemins d'intensification et durabilité des exploitations de polyculture-élevage en Afrique subsaharienne : contribution de l'association agriculture-élevage. Cahiers Agric. 26, 25006. doi: 10.1051/cagri/2017011
Vall, É., Dugué, P., and Blanchard, M. (2006). Le tissage des relations agriculture-élevage au fil du coton. EDP Sciences. 8.
Vanlauwe, B., Hungria, M., Kanampiu, F., and Giller, K. E. (2019). The role of legumes in the sustainable intensification of African smallholder agriculture: lessons learnt and challenges for the future. Agric. Ecosyst. Environ. 284, 106583. doi: 10.1016/j.agee.2019.106583
Zongo, K. F., Hien, E., Drevon, J.-J., Blavet, D., Masse, D., and Clermont-Dauphin, C. (2016). Typologie et logique socio-économique des systèmes de culture associant céréales et légumineuses dans les agro-écosystèmes soudano-sahéliens du Burkina Faso. Int. J. Biol. Chem. Sci. 10, 290. doi: 10.4314/ijbcs.v10i1.23
Keywords: on-farm experiment, collective learning, participatory research, basket of options, farmers' criteria, farming system typology, legumes, West Africa
Citation: Périnelle A, Scopel E, Berre D and Meynard J-M (2022) Which Innovative Cropping System for Which Farmer? Supporting Farmers' Choices Through Collective Activities. Front. Sustain. Food Syst. 6:753310. doi: 10.3389/fsufs.2022.753310
Received: 04 August 2021; Accepted: 15 March 2022;
Published: 27 April 2022.
Edited by:
Mark Van Wijk, International Livestock Research Institute (ILRI), KenyaReviewed by:
Jonathan Steinke, Humboldt University of Berlin, GermanyLeonard Rusinamhodzi, International Institute of Tropical Agriculture (IITA), Nigeria
Copyright © 2022 Périnelle, Scopel, Berre and Meynard. This is an open-access article distributed under the terms of the Creative Commons Attribution License (CC BY). The use, distribution or reproduction in other forums is permitted, provided the original author(s) and the copyright owner(s) are credited and that the original publication in this journal is cited, in accordance with accepted academic practice. No use, distribution or reproduction is permitted which does not comply with these terms.
*Correspondence: Anne Périnelle, YW5uZS5wZXJpbmVsbGVAY2lyYWQuZnI=