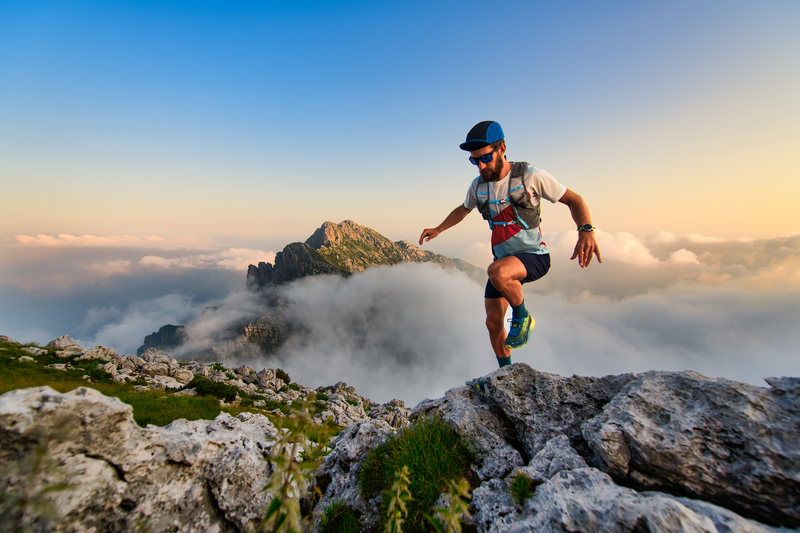
94% of researchers rate our articles as excellent or good
Learn more about the work of our research integrity team to safeguard the quality of each article we publish.
Find out more
ORIGINAL RESEARCH article
Front. Sustain. Food Syst. , 10 January 2023
Sec. Agroecology and Ecosystem Services
Volume 6 - 2022 | https://doi.org/10.3389/fsufs.2022.1058775
Introduction: Cassava production is essential for food security in sub-Saharan Africa and serves as a major calorie-intake source in Nigeria. Estimating the yield gap in Nigeria is essential to indicate the most important limiting factors for production, and identify the yield gap hotspot areas. Secondly, these assessments may help set agendas in policy development and research prioritization where current information is scarce.
Materials and methods: Here, Wwe used a crop model, LINTUL5, calibrated for five different cassava varieties based on field experiments embedded into a modeling framework SIMPLACE to estimate potential, water- and nutrient (current) limited cassava yield gaps (YG) as affected by climate factors and contributing a better understanding of yield gaps and its potentials in 30 states of Nigeria.
Results: Our study shows that cumulative radiation and precipitation were the most significant factors associated with cassava yield variability (p < 0.01). The YG averaged across states was estimated as 18.2 Ton7ha-1, with a maximum of 31.2 Ton7ha-1 35 in Kano state. Across the states, nutrient limitation accounts for 55.3% of the total cassava yield gap, while the remaining 44.7% is attributed to water limitation. The highest untapped water-limited yields were estimated in the northern states, such as Bauchi, Gombe, and Sokoto, characterized by the short rainy season.
Conclusion: Our results showed that most northern states are better equipped to become leading cassava producers in Nigeria under adequate crop management practices involving irrigation and soil fertility enhancement. We reached this conclusion because the northern states usually receive the highest radiation from their characteristic reduced cloud cover, even Therefore, policy and management interventions can be prioritized in these areas. Conclusively, the current cassava yield levels can be increased by a factor of five by emphasizing nutrient and soil health management and irrigation, particularly in areas characterized by a shorter rainy season (Sudan Savanna) in Nigeria.
Cassava (Manihot esculenta Crantz) is a perennial shrub belonging to the dicotyledonous family Euphorbiaceae that is primarily cultivated for starchy roots (Rankine et al., 2021), one of the primary food sources in sub-Saharan Africa (SSA), providing an important source of calories, crucial to food security, and the economy under its diverse applications (Echebiri and Edaba, 2008; Rahman and Awerije, 2016; De Souza et al., 2017). It has become even more critical against the backdrop of a fast-growing population, low crop yields, large yield gaps, and increased fragmentation of farmlands due to increased population pressure (Wahab et al., 2022). Nigeria, the largest cassava root-producing nation globally, harvests more than 59 Mt of fresh cassava roots annually (FAOSTAT, 2021). Most increases in production in the last 26 years (1994–2019) have been attained by improving cassava varieties and expanding the land area for cassava cultivation (Ugwu, 1996; FAOSTAT, 2021). However, the existing literature suggests that the average cassava root yield from 1965 to 2017 (7.0–12.0 Ton·ha−1) was still below its potential productivity (Cock et al., 1979; International Institute of Tropical Agriculture, 2005). Therefore, since the land estimates for cropland expansion in SSA are also contested (Chamberlain et al., 2014), existing cropland must produce substantially more significant quantities than the current yield levels (de Araújo Visses et al., 2018) besides the fact that the finite natural reserves of nutrients in the soils are declining at a fast rate because smallholder farmers generally do not implement measures to replenish nutrient stocks (Nwokoro et al., 2021) leading to a continuous decrease in crop productivity and soil degradation due to nutrient mining (Thierfelder, 2013).
The yield gap (YG) of a crop grown in a particular location and cropping system is defined as the difference between the yield under optimum management and the average observed yield (Yo) achieved by farmers (Lobell et al., 2019). Yield under optimum control is labeled as potential yield (Yp) under irrigated conditions or water-limited yield (Yw) under rainfed conditions (Evans, 1993; Van Ittersum et al., 2013). Yp is location-specific because of the weather, but in theory, it is independent of soil properties, assuming that the water and nutrients required can be added through management. Thus, Yp is the most relevant benchmark for irrigated systems in locations without significant soil constraints, whereas it is Yw for rainfed crops (Van Ittersum et al., 2013). Hence, Yp and Yw are based on optimum planting dates, planting density, and region-specific crop variety. These factors are crucial to determining the feasible growth duration, particularly in tropical climatic conditions where two or three crops are produced yearly and simultaneously. On-station and on-farm research results indicate that in some regions, despite having favorable weather conditions, a sizeable exploitable gap exists between current average yields achieved by farmers and yields that can be achieved under ideal agronomic management conditions (Ayoola and Makinde, 2007; Bamidele et al., 2008; Eke-Okoro and Njoku, 2012). Strong responses have been estimated in the yield dry matter (DM) changes under N, P, and K fertilizers application (2–18, 3–16, and 3–22 Ton·DM·ha−1, respectively) in different agro-ecological zones of Nigeria (Adiele et al., 2020) and elsewhere. For instance, Ezui et al. (2017) reported the positive effect of nitrogen and Potassium on cassava productivity and highlighted the complementary roles of N and K in achieving high radiation and water use efficiency of cassava under rainfed conditions in West Africa. Thus, estimating the YG in Nigeria is essential to indicate the most important limiting factors for production, and identify the YG hotspot areas. Secondly, these assessments may help set agendas in policy development and research prioritization where current information is scarce.
Site-specific simulations of Yp or Yw using crop growth simulation models are suggested as the most reliable tools for estimating Yp or Yw and YG of specific crops within a defined cropping system because these models account for the interactions among weather, soils, and management and therefore, they capture spatial and temporal variations in Yp and Yw, which other empirical methods hardly achieve (van Bussel et al., 2015). The LINTUL5 crop model designed to simulate potential, water-limited and N, P, and K-limited productions (Wolf, 2012) has been used extensively for cropping systems analysis in SSA (Trawally et al., 2015; Faye et al., 2018; Srivastava et al., 2020). To increase food production, identifying the region's untapped production capacity is highly important, and this can be achieved by quantitatively and spatially estimating theYG. This approach requires the consideration of the spatial variation in the climate and the production system. Therefore, this study attempts to (i) evaluate the performance of the LINTUL5 crop model for simulating cassava at the state level across Nigeria, and (ii) identify geospatial gradients of meteorological factors and their effect on Yo and YG spatial variability.
For LINTUL5 crop model calibration at the field scale, cassava storage root yields (Ton·ha−1), above-ground biomass (Ton·ha−1), and fertilizer application (0.3 Ton ha−1, NPK 12:12:17) of five varieties viz.: TME419, Oko-iyawo, TMS91/02324, TMS96/1632, and TMS98/0505 (from International Institute of Tropical Agriculture, IITA, Ibadan, Nigeria) were collected from Ikenne experimental site in 2015. This site is characterized as humid, with rainy and dry seasons spanning April–November and December–March, respectively. The average annual precipitation at Ikenne is 1,517 mm, while the average monthly temperature ranges from 23°C in July to 32°C in February. The soil physicochemical characteristics of the experimental site are summarized in Table 1. The cassava planting and harvesting dates at Ikenne were September 9, 2014, and September 7, 2015, respectively.
The climate data used at the state level consists of a daily time series from 1995 to 2019 which includes the solar radiation (W/m2), precipitation (mm), minimum and maximum temperature (°C). They were obtained from the ERA5-Land offline land surface re-run of ECMWF's (European Center for Medium-Range Weather Forecasts) latest climate product (ECMWF, 2019). The average precipitation, potential evapotranspiration, solar radiation, minimum temperature, maximum temperature, and mean temperature during the cassava growing season of 1995–2019 in 30 states of Nigeria are shown in Figure 1.
Figure 1. Longitudinal and latitudinal gradients of long-term mean values of precipitation, potential evapotranspiration, solar radiation, minimum temperature, maximum temperature, and the mean temperature during the growing season of cassava from 1995 to 2019 in 30 states of Nigeria.
State-level cassava yield data (kg·ha−1) from 1995 to 2014 for 30 states (out of the 36 states) in Nigeria were obtained from the IITA, Ibadan, Nigeria. The remaining six states were excluded due to either lack of data and/or a lack of consistency in the available data. Because no significant trend was observed in the crop yield data (e.g., due to technological developments) from 1995 to 2014, de-trending was not applied. The cassava sowing period was extracted from the crop calendar database (FAO, 2010) and sowing and harvest dates were defined in the simulations accordingly. Net Nitrogen and Phosphorus were applied at the rate of 20 and 5 kg ha−1, respectively across all 30 states as per the recommendations of the Federal Ministry of Agriculture and Rural Development, Nigeria (Chude et al., 2012). Due to the lack of information about the distribution of cassava varieties that farmers grow in the different states of Nigeria, we simulated all five varieties viz: TME419, Oko-iyawo, TMS91/02324, TMS96/1632, and TMS98/0505 across all the 30 states in Nigeria and used the average values for further calculation purposes.
The relevant soil parameters for each soil layer (i.e., sand, silt, clay, gravel content, cation exchange capacity, pH, organic carbon, and bulk density) were extracted from African soil property maps at 1 × 1 km resolution (https://www.isric.org/projects/soil-property-maps-africa-1-km-resolution). Other soil input parameters required in the crop model, such as soil water at field capacity, wilting point, saturation point, and Van-Genuchten parameter, were also estimated according to Rawls et al. (1993). The soil properties for the entire region are depicted in Supplementary Figure 1.
SIMPLACE < LINTUL5-SLIM> solution embedded into the modeling framework SIMPLACE (Scientific Impact Assessment and Modeling Platform for Advanced Crop and Ecosystem Management) (Gaiser et al., 2013) was used in this study to simulate cassava storage roots yield and above-ground biomass. LINTUL5 is designed to simulate potential, water-limited, and nutrient-limited yields. Potential yields were simulated for optimal growing conditions (Van Ittersum et al., 2013), i.e., the crop growth is driven only by solar radiation and temperature, without water stress, nutrient stress, abiotic stress, diseases, and other factors. In addition to these factors, water stress and soil properties are also considered in the simulation of water-limited yield by the model. Water stress occurs when the available soil water is between a defined critical point and wilting point or higher than the field capacity (i.e., waterlogged). The critical point is a crop-specific value (Allen et al., 1998) that depends on crop development, soil water tension, and potential transpiration. Water, nutrients (NPK), temperature, and radiation stresses restrict the daily accumulation of biomass, root growth, and yield in the simulation of nutrient-limited yields by the model. Thus, nutrient-limited yields can by default also be limited by water. Biomass production is based on intercepted radiation according to Lambert-Beer's law and light use effciency. The produced biomass is partitioned among various crop organs (leaves, stems, storage organs, and roots) according to partitioning coeffcients defined as a function of the development stage of the crop. The phenology is simulated by the accumulation of thermal time above a defined base temperature. Photosynthesis and total crop growth rate are calculated by multiplying the intercepted light and radiation use effciency (RUE). The length of the crop growth cycle (i.e., total vegetation days of cassava) in the model is defined by the number of days from emergence to physiological maturity (Wolf, 2012). SLIM is a conceptual soil water balance model subdividing the soil into a variable number of layers, substituting the two-layer approach in LINTUL5 (Addiscott and Whitmore, 1991).
The default cassava crop parameter set for LINTUL5 was used as a starting point to establish a new parameter set for the cassava varieties used for this study. A plausible range over which they vary was obtained from the literature (Ezui et al., 2018). We established a common set of all the model parameters for five varieties (Supplementary Table 1) and evaluated it by comparing the simulated yields and the phenology to the corresponding observations by systematic sampling. Systematic sampling and evaluation were performed for the entire parameter space of all variables before choosing the parameter set with the smallest mean residual error. As a measure of accuracy between the statistical data and simulated values, the mean relative error (MR= ), the mean residual error (ME= ), and root mean square error (RMSE= )) were used, where n is the sample number, Oi is the observed yield and Si is the simulated yield. The ME value of zero indicates no systematic bias between simulated and measured values. The MR shows the mean magnitude of the error related to the observed value; the smaller the value, the smaller the difference between simulated and measured values. Whereas, RMSE is the standard deviation of the residuals (prediction errors).
The crop model was run at 1 km spatial resolution, corresponding to the spatial resolution of the soil input data, for each simulation unit (Figure 2), defined as a combination of soil characteristics, climate, and crop management data. Cassava yields from each state's simulation units were averaged, taking the harvested area of cassava crop into account, sourced from the SPAM (Spatial Production Allocation Model) harvest areas dataset (Yu et al., 2010) to obtain a representative value per state per year. To appraise the spatial variability of cassava in all the states, the coefficient of variation (CV) was calculated for the estimated weather variables (precipitation, potential evapotranspiration, solar radiation, minimum temperature, maximum temperature) within the crop growth period over the simulation period.
Figure 2. Nigeria map highlighting the simulation units of 30 states and the Ikenne experimental site.
Based on the definitions described in section 1 (Introduction), YG was estimated as a water-limited yield gap (YGw), actual yield gap (YGa), and total yield gap (YGt) (see detailed steps of yield gap estimation in Supplementary Figure 2) as expressed in Equations 1–3 below:
Multiple linear regressions were used to explain the relationship between the cassava yield (dependent variable) and the climate variables (independent variables), namely precipitation, minimum and maximum temperatures, and solar radiation in the crop growing season (Figure 1). As there were only four explanatory variables, the complete statistical model was applied. Random forest with extreme gradient boosting was performed for the state-level yields and yield gaps as the dependent variable and precipitation, temperature, and radiation (during the crop growth period) as independent variables.
Compared to the cassava yields observed in the Ikenne experimental site, the simulated yield under fertilized conditions was overestimated by 1.0–7.4% across the five cassava varieties, except for Oko-Iyawo, with a 0.7% yield underestimation (Table 2). Under unfertilized conditions, the simulated yields were overestimated by 1.2–5% and underestimated by 0.6% for TME419. The above-ground biomass under fertilized conditions was also overestimated by 7.8% except for Oko-Iyawo, with 1.4% under-estimation. Also, under unfertilized conditions, the over-estimation ranged between 0.3 and 5.7%, excluding TME419 where 0.9% under-estimation ensued (Table 2).
Table 2. Observed (in the Ikenne experimental site) and simulated cassava yield dry matter, above-ground biomass (in Ton·ha−1), mean error (ME, in Ton·ha−1), and mean residual error (in %) under control (unfertilized) and fertilized conditions of the five cassava varieties at Ikenne experimental site, Nigeria.
The model performed well in capturing the spatial and temporal yield variability at the state level across Nigeria. The average simulated yield (average over the 20 study years) across the states was overestimated by 6.9% compared to the observed yields with an RMSE of 0.47 Ton·ha−1 and R2 = 0.89 (Figures 3A, B). The simulated yield within the 20 years of simulation (1995–2014) compared to the observed yields was overestimated by 5.5% with an RMSE of 0.40 Ton·ha−1 (Figure 4).
Figure 3. Simulated actual yield (nutrient and water-limited) vs. observed (farmer's yield at the state level in Nigeria) cassava dry matter yield (in Ton.ha−1) averaged per state over 20 years from 1995 to 2014 (A) and regression line across the 30 states (B) in Nigeria.
Figure 4. Annual variation of simulated (nutrient and water-limited) vs. observed (farmer's yield at the state level in Nigeria) cassava yield (in Ton.ha−1) for 20 years (1995–2015) averaged over 30 states in Nigeria.
Simulated cassava's mean potential (Yp) and water-limited (Yw) yields were 22.3 and 14.2 Ton·ha−1, respectively, across the 30 states from 1995 to 2019, with CV values ranging from 2.1 to 4.7% for Yp, and 2.9 to 32.2% for YW. Mean yields ranged from 17.4 to 34.7 Ton·ha−1 for Yp, and 2.9 (Sokoto State) to 19.4 Ton·ha−1 (Imo State) for Yw (Figure 5). However, the observed yields (mean = 4.1 Ton·ha−1) ranged between 0.8 and 6.01 Ton·ha−1 (Figure 3A). The Yp increased with latitude, having the highest values of 34.7, 33.9, and 28.1 Ton·ha−1 in Kano, Bauchi, and Kaduna, respectively, whereas the Yw increased with the lower latitudes, with the highest yields located in the Southeastern states such as Imo (19.4 Ton·ha−1) and Akwa Ibom (18.0 Ton·ha−1).
Figure 5. Spatial analysis of total yield gap, water-limited yield gap, and actual yield gap across the 30 states from 1995 to 2019. (A–C) pertain to the temporal variability in simulated cassava yield under potential, water-limited, and actual conditions, respectively. The Fig at the bottom (last row) illustrates the simulated value average over 20 years of actual yield (Nutrient and water-limited), water-limited (WL) yield, and the potential yield across the 30 states of Nigeria.
Multiple regression analysis (Table 3) shows that the spatial variability in the observed cassava yields across the 30 states correlated significantly with radiation (p < 0.01) and precipitation (p < 0.01) within the crop-growth period. The actual yield (simulated) also correlated significantly with radiation (p < 0.01) and precipitation (p < 0.01) within the same period (R2 = 0.76). The Yw and Yp significantly correlated with radiation (p < 0.05), having an R2 value of 0.84 and 0.91, respectively. Likewise, the simulated estimation of the total yield gap and water-limited yield gap showed a highly significant correlation (p < 0.01) with the precipitation and radiation amount during the crop-growth period with R2 = 0.95 and 0.98, respectively. The maximum temperature within the crop-growth period was also significantly correlated with the water-limited yield gap (Table 3). The actual yield gap was not significantly correlated with any of the dependent variables, i.e., radiation, maximum and minimum temperature, and precipitation. Similar results are shown with the non-linear random forest algorithm based on extreme gradient boosting (Table 4).
Table 3. Regression coefficients of multiple linear regression of simulated potential, water-limited, actual yield (nutrient and water-limited), and observed yield (farmer's yield at the state level in Nigeria) with different climatic variables maximum temperature (Tmax), minimum temperature (Tmin), solar radiation (Rad), and precipitation (Ppt).
Table 4. Feature importance for simulated potential, water-limited, actual yield (nutrient and water-limited), and observed yield (farmer's yield at the state level in Nigeria) with respect to different climatic variables like Maximum temperature (Tmax), Minimum temperature (Tmin), Radiation (Rad), and Precipitation (Ppt).
The difference between the potential yield and the observed yield (farmer's yield at the state level in Nigeria) represents the exploitable yield gap. This gap becomes smaller as farmers improve their agronomic management practices, e.g., increasing fertilizer, irrigation, or use of improved cultivars having higher potential yields. Therefore, the total yield gap can be divided into two parts: yield reduction due to water stress and yield reductions due to other factors such as nutrient stress, diseases, insect pest, and others. On average, for the 30 states, the total yield gap (YGt) was 18.2 Ton·ha−1, about 81.2% of the average simulated potential yield (Yp). Across the states, YGt ranged from 11.9 Ton·ha−1 in Bayelsa to a maximum of 31.2 Ton·ha−1 in the Kano State (Figure 5). The average yield loss due to the suboptimal water availability (YGw) during the growth period was estimated as 8.1 Ton·ha−1, about 36.3% of the average simulated potential yield (Yp) across the states. The yield gaps due to water limitation were prominent at the locations with low cumulative precipitation over the crop growth period, such as in Kano (Figure 1). However, it narrowed down considerably in states with higher rainfall (Figure 1). The average actual yield gap (i.e., YGa) across the 30 states was estimated as 10.0 Ton·ha−1 with the highest and lowest (14.4 and 2.0 Ton·ha−1) in Akwa Ibom and the Sokoto states, respectively.
We investigated the relationship between climate variability and simulated yield gaps of cassava in Nigeria. Random forest with extreme gradient boosting was performed for the state-level yields and yield gaps as the dependent variable and precipitation, temperature, and radiation (during the crop growth period) as independent variables. Multivariate linear regression with the same framework revealed that precipitation (negative slope) and radiation (negative slope) significantly explained the spatial variability in the simulated actual yield and the observed yield (Table 3). These findings are also corroborated by Akinwumiju et al. (2020), who found that cassava yield-sunshine hour and cassava yield-precipitation relationships are significant, unlike cassava-temperature relationships. However, the negative linear relationship with precipitation could be attributed to the decreasing radiation due to cloud cover changes in the presence of increased rainfall. Additionally, higher precipitation could result in an increased amount of nitrogen leaching from the soil. Water-limited yield and potential yield of cassava were significantly influenced by radiation during the crop growth period.
The observed yield (farmer's yield at the state level in Nigeria) had no significant relation with the temperature values in the crop growing cycle as was revealed by the linear regression. However, linear regression may not be able to disentangle non-linear associations. Random forests, which capture the non-linear relationships as a machine learning methodology highlight that the maximum temperature has the highest importance for observed yield. The linear regression however shows a significant relationship only with the radiation and precipitation amount. From a simplistic mechanistic perspective, we conducted further inference using linear regression. This phenomenon could be ascribed to the higher radiation with ample precipitation within the crop growth period, which could increase the rate of photosynthesis, crop growth rates and eventually promotes higher crop biomass production and yields (through effects on maintenance respiration) (Baron et al., 2005; Sultan et al., 2005; Ramarohetra et al., 2013; Akinwumiju et al., 2020). Past studies have indicated that when incident solar radiation is intercepted almost completely by a cassava canopy, crop growth rate is positively related to the incident solar radiation (Keating et al., 1982; Fukai et al., 1984). Furthermore, studies have shown that the root dry matter production of different growth stages was affected by reduced solar radiation input because assimilates were preferentially distributed to the shoot under reduced light with a resultant low harvest index (Cock and Rosas, 1975). Researchers have also reported that under the non-limiting condition of rainfall, attainable yield depends on solar radiation. Thus, it is a limiting factor for potential crop yield, especially in the humid regions of West Africa, due to the dense cloud covers during the rainy season (Sultan et al., 2005; Folberth et al., 2012; Srivastava et al., 2017).
We have plotted water-limited cassava yield against the cumulated precipitation during the crop growth period (Figure 6). We obtained a biphasic pattern for the water-limited cassava yield, consisting of an exponential relationship between 370 and 1,750 mm of precipitation and a decreasing plateau for the higher cumulative precipitation amounts. The same phenomenon was reported in earlier studies in West African countries for maize and pearl millet (Baron et al., 2005; Srivastava et al., 2017).
Figure 6. Relationship between simulated water-limited yield dry matter (in Ton.ha−1) of cassava, and cumulated precipitation amount (in mm) during the crop growth period.
The model estimated large and variable gaps in cassava yield across the states. The average total yield gap was ~18.2 Ton·ha−1, with a maximum value of 31.2 Ton·ha−1 in Kano State. Also, the average yield gap due to water-limitation was 8.1 Ton·ha−1, with the highest value (24.2 Ton·ha−1) in Kano State, a savanna ecological zone characterized by a short rainy season (Akinwumiju et al., 2020). Similarly, cassava's average actual yield gap for the states was 10.0 Ton·ha−1, with the highest value (14.4 Ton·ha−1) in Akwa-Ibom State, a region characterized by favorable climatic conditions and well-drained conditions soil (Akinwumiju et al., 2020). Therefore, we suggest that improving crop management through nutrient application and soil water-loss reduction could help to minimize cassava yield gaps. The adoption of sustained and controlled release of nutrients from fertilizer sources certainly is the need of the time since it enhances efficient use of nutrients by the crops and, in turn, productivity with lower cost, and less negative environmental impacts. toward a cleaner and more sustainable crop production (Lewu et al., 2020; Versino et al., 2020). As in the current situation, nitrogen is the most limiting nutrient in cassava production in Nigeria, crop management practices that increase the amount and availability of nitrogen in the soil-plant system have to be promoted. In the first place, the integration of leguminous crops that can fix nitrogen into the crop rotation or a mix-crop system with cassava is of prior importance. Cassava can be grown in row intercropping with cowpea or other fast-growing legumes, which may increase the land equivalent ratio by up to 38% (Mutsaers et al., 1993). Integration of cassava into agroforestry systems with leguminous shrubs or trees is another option (Akondé et al., 1996). In addition, organic materials like livestock manure, compost, or cover crops can be applied and combined with adequate amounts of mineral nitrogen or NPK fertilizers. In order to avoid leaching of nitrate or emission of nitrous gases, all fertilizer applications (and in particular mineral N fertilizers) have to be applied in a way that the release of the nitrogen is at the time when N demand by the crop is highest. The combined application of organic manure with judicious amounts of mineral fertilizers could be a viable solution for sustainable crop production since organic manure application not only improves the soil nutrient status but also improves soil porosity, higher aggregate stability in the topsoil and achieves high infiltration rates, which could further lead to lower runoff (loss of water for the crop) and lower risk of soil erosion.
The estimated highest untapped cassava yields (YGt) in states (such as Kano, Bauchi, Gombo, and Kebbi) align clearly with a previous study (Akinwumiju et al., 2020). These states are categorized as “suitable” to “very suitable” cultivable areas. Therefore, cassava cultivation would excel adequately; hence profitable cassava production could be successfully undertaken on at least 60 million hectares of land in Nigeria.
The current study's estimated cassava yield gap, based on water limitation (i.e., with optimal fertilization and water as the major growth-limiting factors), was consistent and within the reported yield gaps range reported elsewhere. For example, Adiele et al. (2020) reported reducing the cassava yield gaps by 230% under optimum fertilizer application in Edo, Cross River, and the Benue states in Nigeria. Another study (Fermont et al., 2009) estimated a water-limited cassava yield gap (i.e., closing the yield gaps) by improving the soil fertility in Kenya and Uganda (East Africa) ranged from an average difference of 6.7 Ton·ha−1 to a maximum of 7.9 Ton·ha−1 concerning the attainable yield.
Our study across 30 states estimated that nutrient management accounts for 55.3% of the total cassava yield gap, while the remaining 44.7% is attributed to water limitation. The highest untapped water-limited yields were estimated in states, such as Bauchi, Gombe, and Sokoto, characterized by the short rainy season, therefore posing a threat to the widely practiced rainfed agriculture in Nigeria (Mbanasor et al., 2015; Ogunrinde et al., 2019). However, farmers could use abundant renewable water resources to compensate for soil moisture deficits, particularly in regions with a short rainy season. This scenario could be achieved by retaining a large percentage of the annual runoff for irrigation purposes during the dry season (Akinwumiju et al., 2020). Thus, cassava production should be targeted by suitable land allocation where viable irrigation infrastructure is available, particularly in the northern part of Nigeria. Moreover, the nutrient-related constraints accounting for >55% of the untapped cassava potential yield demand for effective on-farm management and soil fertility enhancement (organic and inorganic fertilizer application), already practiced in some SSA countries (Edet et al., 2013; Ezui et al., 2015; Aliyu et al., 2019). Compared to other SSA countries (such as Niger, Ghana, and Cameroon), increased cassava yield has been attributed to effective on-farm management, fertile land allocation, and fertilizer application, where required (Adjei-Nsiah et al., 2007; Kaweewong et al., 2013; Ezui et al., 2017). Whereas, in Nigeria, the reverse is the case. Here, local cassava varieties and nutrient-depleted soils are attributed to their cultivation, and the major cassava production belt lacks the required on-farm management practices. Therefore, in addition to improved high-yielding cassava varieties and adequate fertilizer application, access to sufficient water supply or irrigation facilities (especially in regions with short rainy seasons, and semi-arid regions) is imperative to improve the current cassava yield. There is also a growing concern for ecologically friendly and sustainable production systems. There is increased consumer interest in organic farming and organically produced food (Suja et al., 2012a,b). However, in view of the increasing demand for cassava not only for food but also for feed and industrial purposes makes it necessary to use fertilizer (be it organic or inorganic) to offset soil nutrient depletion and imbalance (Biratu et al., 2022). Foods produced by organic methods are usually believed to be of superior in quality with better taste, biochemical constituents, minerals, and absence of contaminants and toxic substances (Worthington, 2001; Azami et al., 2003; Pérez-Lopez et al., 2007; Bimova and Pokluda, 2009). It is more important in the case of cassava which exports large quantities of nutrients from the soil and there is little understanding of the influence of organic fertilizers on the growth, yield, tuber quality, and soil properties in cassava. Some studies have shown the advantages of using organic fertilizer in cassava in terms of improved leaf and stem growth, transfer of photosynthates to storage roots, and increased yield and starch content in the storage roots of cassava (Zhongyong et al., 2006; Shafeek et al., 2012; Seena Radhakrishnan, 2017). The release of almost all the nutrients and activity of soil enzymes were higher along with an increasing trend in pH and electrical conductivity (Linn and Myint, 2018; Seena Radhakrishnan and Suja, 2019). Nutritional quality was improved by organic fertilization with significant effects on antioxidant activity and phenolic metabolites in cassava (Omar et al., 2012). Additionally and very importantly, organic fertilizers are generally thought to be slow-releasing fertilizers and they contain many trace elements. However, the improper use of organic fertilizers can also lead to overfertilization or nutrient toxicity in the soil. Hence, the controlled release of organic fertilizers is an effective and advanced way to overcome these impacts and maintain sustainable agricultural yield (Shaji et al., 2021). As far as inorganic nitrogen fertilizers are concerned, they can be replaced by other N sources that directly or indirectly increase N input into the soil by fixing Nitrogen from the atmosphere. On the other hand, it will be difficult to add the amount of Phosphorous and Potassium required by the crops through the sole use of manure or other external organic fertilizers. For both nutrients, farmers will have to use inorganic sources containing Phosphorous or potassium, like e.g., ground phosphate rocks, or potassium salts, especially in tropical soils with low P and K availability.
Our results showed that most northern states are better equipped to become leading cassava producers in Nigeria under adequate crop management practices involving irrigation and soil fertility enhancement. We reached this deduction because the northern states usually receive the highest radiation from their characteristic reduced cloud cover, even at the peak of the rainy season. Another reason is that cassava production cost is lower in the north than in the south, because of the availability of extensive, less competitive expanse of lands that could be made suitable for cassava cultivation. Furthermore, considering the ongoing increasing rainfall trend in northern Nigeria (Animashaun et al., 2020), more locations might gain a suitability advantage for rainfed cassava production with time.
There were some limitations of the current study, highlighting the necessary improvements for further research. Usually, large-scale assessments are confronted with the trade-off between the advantages of a large spatial study coverage (useful for policymaking) (Ewert et al., 2015) and the disadvantage of low spatial accuracy at the farmer's field level. The lack of detailed model calibration at a finer scale is a popular challenge for large-scale crop modeling (Müller et al., 2017) because location-specific environmental and management data are usually challenging to obtain consistently for large regions (Angulo et al., 2013). Because we also used the average yield values of five cassava varieties to estimate the yield gaps for Nigeria, a future study can be structured by interacting with local experts so that well-adapted cultivars specific to each state could be simulated. Farmer's fields may have been affected by diseases like the Cassava blight virus, which was not considered in the simulations. However, in general, cassava is less prone to diseases and pests compared to other field crops. The continuous application of pesticides and the use of only inorganic fertilizers have high environmental and health risks. Therefore, the adoption of sustained and controlled release of pesticides and fertilizers certainly is the need of the time since it enhances the rational and adequate use of nutrients, which increases the efficiency in the consumption of each nutritive element and, in turn, the productivity with lower cost and environmental footprint constituting a resource toward a cleaner and more sustainable agriculture practices, as it intends to avoid excessive use of fertilizer and pesticides leading to water pollution (Lewu et al., 2020; Versino et al., 2020). Some studies have shown the positive effect of controlled/slow-release fertilizer on cassava yield and increased net photosynthetic rate (Sheng et al., 2014). Production systems were abstracted at the level of a single crop, ignoring possible interactions within crop rotations. If cropping systems were analyzed instead, crop performance in a given simulation unit would result from its performance in different rotations and under different inputs of resources. We did not consider the effect of certain climatic extremes, such as the impact of intense heat during particular development stages, which may lead to total (cereal) crop failure. To overcome this limitation, small-scale studies that incorporate more detailed information [e.g., on-farming environments, management practices, variety distribution, and the socio-economic context of farmers (especially cassava value chain)] could be used. They would complement our assessment to provide more detailed recommendations for specific local areas cases minimizing the gaps in cassava yield. The current study results have provided vital information on how the cassava yield gap could be closed with many attendant economic benefits and food security in Nigeria. Furthermore, the outcome of this study could better enlighten stakeholders and policymakers in Nigeria on the need to harness renewable resources (such as precipitation) and nutrient management while adopting scientific innovations to optimize the vast expanse of lands currently underutilized, especially in the north.
Quantitative evaluation of yield potentials and yield gaps is necessary for creating strategies to improve crop productivity and secure the food supply. This study contributes to a better understanding of cassava yield gaps (water-limited, and nutrient-limited conditions) and its potential in 30 states of Nigeria. Here, we have calibrated and evaluated the LINTUL5 model by performing field measurements. We showed that our model can be used to simulate cassava's growth and yield and determine the effects of temperature, precipitation, and other variables. The model simulations showed that, across locations, nutrient limitations were more pronounced than the water limitation on cassava yield gaps in 20 out of 30 states. Furthermore, solar radiation and precipitation are the predominant climatic variables that influence cassava yield.
Overall, we conclude that there is a high potential to substantially increase the current cassava yield levels in Nigeria, through farm interventions emphasizing nutrient and soil health management, as well as ensuring an adequate water supply, for example via irrigation schemes in areas characterized by a short rainy season.
The original contributions presented in the study are included in the article/Supplementary material, further inquiries can be directed to the corresponding authors.
AS, TG, and JR conceived and designed the study. MS and AC helped in the post-processing of the data. All authors helped in writing and improving the first draft of the paper.
This research has been supported by the UPSCALERS project (Grant No. AURG II-1-074-2016). We also acknowledge the funding by the German Federal Ministry of Education and Research (BMBF) in the framework of the funding measure Soil as a Sustainable Resource for the Bioeconomy—BonaRes, project BonaRes (Module A): BonaRes Center for Soil Research, subproject Sustainable Subsoil Management—Soil3 (Grant 031B0151A), and partially funded by the Deutsche Forschungsgemeinschaft (DFG, German Research Foundation) under Germany's Excellence Strategy—EXC 2070—390732324.
The authors acknowledge the support from anonymous researchers for refining the draft of the manuscript. An unmodified version of the current work as a preprint is available on Research Square.
AAdeb was employed by International Institute of Tropical Agriculture.
The remaining authors declare that the research was conducted in the absence of any commercial or financial relationships that could be construed as a potential conflict of interest.
All claims expressed in this article are solely those of the authors and do not necessarily represent those of their affiliated organizations, or those of the publisher, the editors and the reviewers. Any product that may be evaluated in this article, or claim that may be made by its manufacturer, is not guaranteed or endorsed by the publisher.
The Supplementary Material for this article can be found online at: https://www.frontiersin.org/articles/10.3389/fsufs.2022.1058775/full#supplementary-material
Addiscott, T. M., and Whitmore, A. P. (1991). Simulation of solute leaching in soils with different permeabilities. Soil Use Manage. 7, 94–102. doi: 10.1111/j.1475-2743.1991.tb00856.x
Adiele, J. G., Schut, A. G. T., van den Beuken, R. P. M., Ezui, K. S., Pypers, P., Ano, A. O., et al. (2020). Towards closing cassava yield gap in West Africa: Agronomic efficiency and storage root yield responses to NPK fertilizers. Field Crops Res. 253. doi: 10.1016/j.fcr.2020.107820
Adjei-Nsiah, S., Kuyper, T. W., Leeuwis, C., Abekoe, M. K., and Giller, K. E. (2007). Evaluating sustainable and profitable cropping sequences with cassava and four legume crops: effects on soil fertility and maize yields in the forest/savannah transitional agro-ecological zones of Ghana. Field Crops Res. 103, 87–97. doi: 10.1016/j.fcr.2007.05.001
Akinwumiju, A. S., Adelodun, A. A., and Orimoogunje, O. I. (2020). Agro-climato-edaphic zonation of nigeria for a cassava cultivar using GIS-based analysis of data from 1961 to 2017. Sci. Rep. 10, 1259. doi: 10.1038/s41598-020-58280-4
Akondé, T. P., Leihner, D. E., and Steinmüller, N. (1996). Alley cropping on an Ultisol in subhumid Benin. Part 1: Long-term effect on maize, cassava and tree productivity. Agrofor. Syst. 34, 1–12. doi: 10.1007/BF00129628
Aliyu, I. A., Yusuf, A. A., Uyovbisere, E. O., Masso, C., and Sanders, I. R. (2019). Effect of co-application of phosphorus fertilizer and in vitroproduced mycorrhizal fungal inoculants on yield and leaf nutrient concentration of cassava. PLoS ONE. 14, e0218969. doi: 10.1371/journal.pone.0218969
Allen, R. G., Pereira, L. S., Raes, D., and Smith, M. (1998). Crop evapotranspiration: guidelines for computing crop water requirements. Irrigation and Drainage Paper 56. UN-FAO, Rome, Italy 301.
Angulo, C., Rötter, R., Trnka, N., Pirttioja, N., Gaiser, T., Hlavinka, P., et al. (2013). Characteristic fingerprints of crop model responses to weather input data at different spatial resolutions. Eur. J. Agron. 49, 104–114. doi: 10.1016/j.eja.2013.04.003
Animashaun, I. M., Oguntunde, P. G., Olubanjo, O. O., and Akinwumiju, A. S. (2020). Rainfall Analysis over niger central hydrological area, nigeria: variability, trend and change point detection. Sci. African. 8, e00419. doi: 10.1016/j.sciaf.2020.e00419
Ayoola, O. T., and Makinde, E. A. (2007). Fertilizer treatment effects on performance of cassava under two planting patterns in a cassava-based cropping system in South West Nigeria. Res. J. Agric. Biol. Sci. 3, 13–20.
Azami, D. K., Hong, Y.-J., Barrett, D. M., and Mitchell, A. E. (2003). Comparison of the total phenolic and ascorbic acid content of freeze dried and air-dried marionberry, strawberry and corn grown using conventional, organic and sustainable agricultural practices. J. Agric. Food Chem. 51, 1237–1241. doi: 10.1021/jf020635c
Bamidele, F. S., Babatunde, R. O., and Rasheed, A. (2008). Productivity analysis of cassava-based production systems in the Guinea savannah: case study of Kwara State, Nigeria. Am. Eurasian J. Sci.Res. 3, 33–39.
Baron, C., Sultan, B., Balme, M., Sarr, B., Traore, S., Lebel, T., et al. (2005). From GCM grid cell to agricultural plot: scale issues affecting modelling of climate impact. Phil. Trans. R. Soc. B: Biol. Sci. 360, 2095–2108. doi: 10.1098/rstb.2005.1741
Bimova, P., and Pokluda, R. (2009). Impact of organic fertilizers on total antioxidant capacity of head cabbage. Hort. Sci. 36, 21–25. doi: 10.17221/9/2008-HORTSCI
Biratu, G. K., Elias, E., and Ntawuruhunga, P. (2022). Does the application of mineral and organic fertilizer affect cassava tuber quality? An evidence from Zambia. J. Agric. Food Res. 9, 100339. doi: 10.1016/j.jafr.2022.100339
Chamberlain, J., Jayne, T. S., and Headey, D. (2014). Scarcity amidst abundance? Reassessing the potential for cropland expansion in Africa. Food Policy 48, 51–65. doi: 10.1016/j.foodpol.2014.05.002
Chude, V. O., Olayiwola, S. O., Daudu, C., and Ekeoma, A. (2012). Fertilizer use and Management Practices for Crops in Nigeria, 4th edn. Abhuja: Federal Fertilizer Department, Federal Ministry of Agriculture and Rural Development.
Cock, J. H., Franklin, D., Sandoval, G., and Juri, P. (1979). The ideal cassava plant for maximum yield. Crop Sci. 19, 271–279. doi: 10.2135/cropsci1979.0011183X001900020025x
Cock, J. H., and Rosas, S. C. (1975). “Ecophysiology of cassava,” in Ecophysiology of Tropical Crops. Commun. Div. CEPLAC, Km 22 Rodovia Ilheus/Itabuna, Bahia, Brazil, 1–14. Available online at: https://hdl.handle.net/10568/71783
de Araújo Visses, F., Sentelhas, P. C., and Pereira, A. B. (2018). Yield gap of cassava crop as a measure of food security-an example for the main Brazilian producing regions. Food Secur. 10, 1191–1202. doi: 10.1007/s12571-018-0831-2
De Souza, A. P., Massenburg, L. N., Jaiswal, D., Cheng, S., Shekar, R., and Long, S. P. (2017). Rooting for cassava: insights into photosynthesis and associated physiology as a route to improve yield potential. New Phytol. 213, 50–65. doi: 10.1111/nph.14250
Echebiri, R. N., and Edaba, M. E. I. (2008). Production and utilization of cassava in Nigeria: prospects for food security and infant nutrition. PAT. 4, 38–52. doi: 10.1108/nfs.2008.01738eab.026
ECMWF (2019). ERA5-Land Hourly Data from 1981 to Present, Technical Report, ECMWF. doi: 10.24381/cds.e2161bac
Edet, M., Tijani-Eniola, H., and Okechukwu, R. (2013). Residual effects of fertilizer application on growth and yield of two cassava varieties in Ibadan, South-Western Nigeria. Afr. J. Root Tuber Crops. 10, 33–40.
Eke-Okoro, O., and Njoku, D. (2012). A review of cassava development in Nigeria from 1940-2010. J. Agric. Bio. Sci. 7, 59–65.
Ewert, F., Rötter, R. P., Bindi, M., Webber, H., Trnka, M., Kersebaum, K. C., et al. (2015). Crop modelling for integrated assessment of risk to food production from climate change. Environ. Model. Softw. 72, 287–303. doi: 10.1016/j.envsoft.2014.12.003
Ezui, K. S., Franke, A. C., Ahiabor, B. D. K., Tetteh, F. M., Sogbedgi, J., Janssen, B. H., et al. (2017). Understanding cassava yield response to soil and fertilizer nutrient supply in West Africa. Plant Soil. 420, 331–347. doi: 10.1007/s11104-017-3387-6
Ezui, K. S., Franke, A. C., Mando, A., Ahiabor, B. D. K., Tetteh, F. M., Sogbedji, J., et al. (2015). Fertilizer requirements for balanced nutrition of cassava across eight locations in West Africa. Field Crops Res. 185, 69–78. doi: 10.1016/j.fcr.2015.10.005
Ezui, K. S., Leffelaar, P. A., Franke, A. C., Mando, A., and Giller, K. E. (2018). Simulating drought impact and mitigation in cassava using the LINTUL model. Field Crops Res. 219, 256–272. doi: 10.1016/j.fcr.2018.01.033
FAO (2010). FAOSTAT Statistical Database. Food and Agricultural Organization of the UN, Rome. Available online at: http://faostat.fao.org (accessed April 10, 2022).
FAOSTAT (2021). Food and Agriculture Organization of the United Nations. Available online at: http://www.fao.org/faostat/en/#data/QA (accessed October 30, 2021).
Faye, B., Webber, H., Diop, M., Mbaye, M., Owusu-Sekyere, J. D., Naab, J. B., et al. (2018). Potential impact of climate change on peanut yield in Senegal, West Africa. Field Crop. Res. 219, 148–159. doi: 10.1016/j.fcr.2018.01.034
Fermont, A. M., van Asten, P. J. A., Tittonell, P., van Wijk, M. T., and Giller, K. E. (2009). Closing the cassava yield gap: an analysis from smallholder farms in East Africa. Field Crops Res. 112, 24–36. doi: 10.1016/j.fcr.2009.01.009
Folberth, C., Gaiser, T., Abbaspour, K. C., Schulin, R., and Yang, H. (2012). Regionalization of a large-scale crop growth model for sub-Saharan Africa: model setup, evaluation, and estimation of maize yields. Agric. Ecosyst. Environ. 151, 21–33. doi: 10.1016/j.agee.2012.01.026
Fukai, S., Alcoy, A. B., Llamelo, A. B., and Patterson, R. D. (1984). Effects of solar radiation on growth of cassava (Manihot esculenta Crantz.). I. Canopy development and dry matter growth. Field Crops Res. 9, 347–360. doi: 10.1016/0378-4290(84)90038-8
Gaiser, T., Perkons, U., Kupper, P. M., Kautz, T., Puschmann, D. U., Ewert, F., et al. (2013). Modeling biopore effects on root growth and biomass production on soils with pronounced sub-soil clay accumulation. Ecol. Model. 256, 6–15. doi: 10.1016/j.ecolmodel.2013.02.016
International Institute of Tropical Agriculture (2005). The Nigerian Cassava Industry: Statistical Handbook. Available online at: IITA. https://www.iita.org/ (accessed 2014).
Kaweewong, J., Kongkeaw, T., Tawornprek, S., Yampracha, S., and Yost, R. (2013). Nitrogen requirements of cassava in selected soils in Thailand. J. Agr. Rural Dev. Trop. Subtrop. 114, 13–19.
Keating, B. A., Evenson, J. P., and Fukai, S. (1982). Environmental effects on growth and development of cassava (Manihot esculenta Crantz.). 2. Crop growth rate and biomass yield. Field Crops Res. 5, 283–292. doi: 10.1016/0378-4290(82)90031-4
Lewu, F. B., Volova, T., Thomas, S., and Rakhimol, K. R. (2020). Controlled release fertilizers for sustainable agriculture. doi: 10.1016/C2018-0-04238-3
Linn, K. Z., and Myint, P. P. (2018). Study on the effectiveness of natural organic fertilizers on cassava (Manihot esculenta Crantz) cultivation. Asian J. Soil Sci. Plant Nutr.3, 1–10. doi: 10.9734/AJSSPN/2018/42912
Lobell, D. B., Cassman, K. G., and Field, C. B. (2019). Crop yield gaps: their importance, magnitudes, and causes. Annu. Rev. Environ. Resour. 34, 179–204. doi: 10.1146/annurev.environ.041008.093740
Mbanasor, J. A., Nwachukwu, I. N., Agwu, N. M., and Onwusiribe, N. C. (2015). Impact of climate change on the productivity of cassava in Nigeria. J. Agric. Environ. Sci. 4, 138–147.
Müller, C., Elliott, J., Chryssanthacopoulos, J., Arneth, A., Balkovic, J., Ciais, P., et al. (2017). Global gridded crop model evaluation: benchmarking, skills, deficiencies and implications. Geosci. Model Dev. 10, 1403–1422. doi: 10.5194/gmd-10-1403-2017
Mutsaers, H. J. W., Ezumah, H. C., and Osiru, D. S. O. (1993). Cassava-based intercropping: a review. Field Crops Res. 34, 431–457. doi: 10.1016/0378-4290(93)90125-7
Nwokoro, C. C., Kreye, C., Necpalova, M., Adeyemi, O., Busari, M., Tariku, M., et al. (2021). Developing recommendations for increased productivity in cassava-maize intercropping systems in Southern Nigeria. Field Crops Res. 272, 108283. doi: 10.1016/j.fcr.2021.108283
Ogunrinde, A. T., Oguntunde, P. G., Akinwumiju, A. S., and Fasinmirin, J. T. (2019). Analysis of recent changes in rainfall and drought indices in Nigeria, 1981–2015. Hydrol. Sci. J. 64, 1755–1768. doi: 10.1080/02626667.2019.1673396
Omar, N. F., Hassan, S. A., Yusoff, U. K., Abdullah, N. A. P., Wahab, P. E. M., and Sinniah, U. R. (2012). Phenolics, flavonoids, antioxidant activity and cyanogenic glycosides of organic and mineral-base fertilized cassava tubers. Molecules 17, 2378–2387. doi: 10.3390/molecules17032378
Pérez-Lopez, A. J., López-Nicolas, J. M., and Carbonell-Barrachina, A. A. (2007). Effects of organic farming on mineral contents andaroma composition of Clemenules mandarin juice. Eur. FoodRes. Technol. 225, 255–260. doi: 10.1007/s00217-006-0412-z
Rahman, S., and Awerije, B. O. (2016). Exploring the potential of cassava in promoting agricultural growth in Nigeria. J. Agric. Rural Dev. Trop. 117, 149–163.
Ramarohetra, J., Sultan, B., Baron, C., Gaiser, T., and Gosset, M. (2013). How satellite rainfall estimates errors may impact rain-fed cereal yield simulation in West Africa? Agric. For. Meteorol. 180, 118–131. doi: 10.1016/j.agrformet.2013.05.010
Rankine, D., Cohen, J., Murray, F., Moreno-Cadena, P., Hoogenboom, G., Campbell, J., et al. (2021). Evaluation of DSSAT-MANIHOT-Cassava model to determine potential irrigation benefits for cassava in Jamaica. Agron. J. 113, 5317–5334. doi: 10.1002/agj2.20876
Rawls, W. J., Ahuja, L. R., and Maidment, D. R. (1993). Handbook of Hydrology. (McGraw-Hill). 1442. Available online at: http://dl.watereng.ir/HANDBOOK_OF_HYDROLOGY.PDF.
Seena Radhakrishnan, A. R. (2017). Evaluation of Agronomic, Nutritional and Socio-Economic Impacts of Organic Production of Cassava (Manihot esculenta Crantz) (Ph.D. Thesis). Submitted to University of Kerela, Thiruvananthapuram, Kerela, India.
Seena Radhakrishnan, A. R., and Suja, G. (2019). Nutrient release pattern of organic and inorganic resources used in cassava production (Manihot esculenta Crantz). J. Plant Nutr. 42, 1301–1315. doi: 10.1080/01904167.2019.1617305
Shafeek, M. R., Omar, N. M., Mahmad, R. A., and Abd EI-Baky, M. M. H. (2012). Effect of bio-organic fertilization on growth and yield of cassava plants in newly cultivated land. Middle East J. Agric. Res. 1, 40–46.
Shaji, H., Chandran, V., and Mathew, L. (2021). Organic fertilizers as a route to controlled release of nutrients. Control. Release Ferti. Sustan. Agri. 231–245. doi: 10.1016/B978-0-12-819555-0.00013-3
Sheng, C., WeiYan, X., HuiXian, C., XingWei, W., Xin, Q., and Dou, F. (2014). Effects of controlled/slow-release fertilizer on growth and yield of cassava. J. Southern Agric. 45, 790–795.
Srivastava, A. K., Ceglar, A., Zeng, W., Gaiser, T., Mboh, C. M., and Ewert, F. (2020). The Implication of different sets of climate variables on regional maize yield simulations. Atmosphere 11, 180. doi: 10.3390/atmos11020180
Srivastava, A. K., Mboh, C. M., Gaiser, T., and Ewert, F. (2017). Impact of climatic variables on the spatial and temporal variability of crop yield and biomass gap in Sub-Saharan Africa-a case study in Central Ghana. Field Crop. Res. 203, 33–46. doi: 10.1016/j.fcr.2016.11.010
Suja, G., Sreekumar, J., Susan John, K., and Sundaresan, S. (2012a). Organic production of tuberous vegetables: agronomic, nutritional and economic benefits. J. Root Crops 38, 135–141.
Suja, G., Sundaresan, S., Susan John, K., Sreekumar, J., and Misra, R. S. (2012b). Higher yield, profit and soil quality from organic farming of elephant foot yam. Agron. Sustain. Dev. 32, 755–764. doi: 10.1007/s13593-011-0058-5
Sultan, B., Baron, C., Dingkuhn, M., and Janicot, S. (2005). Agricultural impacts of large-scale variability of the west African monsoon. Agric. For. Meteorol. 128, 93–110. doi: 10.1016/j.agrformet.2004.08.005
Thierfelder, C. (2013). Integration of conservation agriculture in smallholder farming systems of southern Africa: identification of key entry points. Int. J. Agric. Sustain. 11, 317–330. doi: 10.1080/14735903.2013.764222
Trawally, D., Webber, H., Agyare, W. A., Fosu, M., Naab, J., and Gaiser, T. (2015). Effect of heat stress on two maize varieties under irrigation in Northern Region of Ghana. Int. J. Biol. Chem. Sci. 9, 1571–1587.
Ugwu, B. (1996). Increasing cassava production in Nigeria and prospects for sustaining the trend. Outlook Agric. 25, 179–185. doi: 10.1177/003072709602500307
van Bussel, L. G. J., Grassini, P., Van Wart, J., Wolf, J., Claessens, L., Yang, H., et al. (2015). From field to atlas: upscaling of location-specific yield gap estimates. Field Crop. Res. 177, 98–108. doi: 10.1016/j.fcr.2015.03.005
Van Ittersum, M. K., Cassman, K. G., Grassini, P., Wolf, J., Tittonell, P., and Hochman, Z. (2013). Yield gap analysis with local to global relevance—A review. Field Crop. Res. 143, 4–17. doi: 10.1016/j.fcr.2012.09.009
Versino, F., Urriza, M., and Garcia, M. A. (2020). Cassava-based biocomposites as fertilizer controlled-release systems for plant growth improvement. Indust. Crops Product. 144, 112062. doi: 10.1016/j.indcrop.2019.112062
Wahab, I., Hall, O., and Jirstrom, M. (2022). The maize is the cost of the farming, and the cassava is our profit: smallholders' perceptions and attitudes to poor crop patches in the eastern region of Ghana. Agric. Food Security. 11, 1–17. doi: 10.1186/s40066-022-00361-w
Wolf, J. (2012). User Guide for LINTUL5: Simple Generic Model for Simulation of Crop Growth Under Potential, Water Limited and Nitrogen, Phosphorus and Potassium Limited Conditions. Wageningen, 1–63. Available online at: https://www.wur.nl/en/Publication-details.htm?publicationId=publication-way-343434373139
Worthington, V. (2001). Nutritional quality of organic vs conventional fruits, vegetables and grains. J. Altern. Complement. Med. 7, 161–173. doi: 10.1089/107555301750164244
Keywords: nitrogen, water, climate, potential yield, water-limited yield, actual yield
Citation: Srivastava AK, Ewert F, Akinwumiju AS, Zeng W, Ceglar A, Ezui KS, Adelodun A, Adebayo A, Sobamowo J, Singh M, Rahimi J and Gaiser T (2023) Cassava yield gap—A model-based assessment in Nigeria. Front. Sustain. Food Syst. 6:1058775. doi: 10.3389/fsufs.2022.1058775
Received: 30 September 2022; Accepted: 08 December 2022;
Published: 10 January 2023.
Edited by:
Somasundaram Jayaraman, Indian Institute of Soil Science (ICAR), IndiaReviewed by:
Ana I. F. Ribeiro-Barros, University of Lisbon, PortugalCopyright © 2023 Srivastava, Ewert, Akinwumiju, Zeng, Ceglar, Ezui, Adelodun, Adebayo, Sobamowo, Singh, Rahimi and Gaiser. This is an open-access article distributed under the terms of the Creative Commons Attribution License (CC BY). The use, distribution or reproduction in other forums is permitted, provided the original author(s) and the copyright owner(s) are credited and that the original publication in this journal is cited, in accordance with accepted academic practice. No use, distribution or reproduction is permitted which does not comply with these terms.
*Correspondence: Amit Kumar Srivastava, YW1pdEB1bmktYm9ubi5kZQ==
Disclaimer: All claims expressed in this article are solely those of the authors and do not necessarily represent those of their affiliated organizations, or those of the publisher, the editors and the reviewers. Any product that may be evaluated in this article or claim that may be made by its manufacturer is not guaranteed or endorsed by the publisher.
Research integrity at Frontiers
Learn more about the work of our research integrity team to safeguard the quality of each article we publish.