- 1Department of Engineering Management and Entrepreneurship, Faculty of Engineering, University of Debrecen, Debrecen, Hungary
- 2College of Business and Economics, University of Johannesburg, Johannesburg, South Africa
- 3Department of Logistics Management, Faculty of Economics and Business, University of Debrecen, Debrecen, Hungary
- 4Ihrig Károly Doctoral School of Management and Business, University of Debrecen, Debrecen, Hungary
- 5School of Management Informatic and Computer Science Primakara, Denpasar, Indonesia
- 6Institute of Accounting and Finance, Department of Accounting, Faculty of Economics and Business, University of Debrecen, Debrecen, Hungary
- 7Department of Economics and Financial Mathematics, Faculty of Economics and Business, University of Debrecen, Debrecen, Hungary
There is growing literature on the concept and objectives of corporate sustainability (CS), but less attention is paid to a comprehensive approach to economic, social and ecological factors and industrial revolution (IR). Specifically, this paper contributes to the academic debate on the relationship between CS and IR in agri-food economics using firm-level data. The study used quantitative pathway models to measure the extent to which technologies affect the development of social, ecological and economic factors in Hungarian food manufacturing companies. The research was conducted using partial least squares path modeling (PLS-PM) and categorical principal component analysis (CATPCA) to calculate the direct and indirect effects of IR technologies on profitability outcomes. This study has shown that the livable and sustainable path hypotheses can be confirmed. Consequently, the food manufacturing businesses whose managers think along the viable and sustainable lines tend to be more profitable. However, the ecological and economic factors strengthened the positive impact of the social dimension on food corporate profitability. Decision-makers should not wait for a pie in the sky for emerging sustainability but consciously embrace the CS issues that only provide a direction for the changes.
Introduction
In recent years, there has been an increasing interest in Industrial Revolutions (IR), for instance, 4IR as a technological revolution aimed at achieving greater efficiency and increased productivity in the global marketplace (Lee and Trimi, 2018), initially as a high-tech strategy of the German government (Cugno et al., 2021). 5IR complements the existing Industry 4.0 paradigm by using research and innovation to drive the transition to, for example, sustainable, human-centered and resilient industries (Breque et al., 2021). From a conceptual perspective, Breque et al. (2021) defined 5IR as something that “… recognizes the power of industry to achieve social goals beyond job creation and growth, and to become a resilient provider of prosperity by respecting the limits of the planet and putting the wellbeing of industrial workers at the heart of the production process.” 5IR technologies serve people and societies, which means that humans are not machined; the technology used in manufacturing adapts to the needs and diversity of industrial workers (Lu et al., 2021).
The Covid-19 pandemic and current geopolitical shifts have highlighted the need to rethink existing types of drivers and barriers, e.g., working conditions, compliance with legislation and adaptation to sustainable strategies to overcome the challenges in the food industries (Kamble et al., 2018). The agri-food sector, more than any other sector, is characterized by a high dependence on natural resources and has a significant impact on the ecosystem, and its companies are highly exposed to the expectations of civil society and policies (Hartmann, 2011). These policies have also amplified the vulnerabilities of food manufacturing enterprises, i.e., fragile strategic supply chains and the need to find innovations to address resilience (Ibn-Mohammed et al., 2021).
Corporate Sustainability (CS) has become a vital component of the complementary causality between business performance, economic, environmental and social dimensions (Tomšič et al., 2015). While the linkage between CS and its driving forces and barriers has been investigated (Bojnec and Tomšič, 2020), there is still a significant research gap when considering the relationship between enterprise performance and sustainability in association with the mediating role of IR. Although there are existing research frameworks and findings on sustainable IR (Młody and Weinert, 2020), most studies explored the concept (Ejsmont et al., 2020). Specifically, this paper contributes to the academic debate on the relationship between CS and IR in agri-food economics using micro- and firm-level data.
The major objective of this study was to investigate to the theoretical framework and modelling of CS and IR interactions by analyzing the impact of sustainable technology implementation on profitability business performance. This study follows a cross-country-specific design in the case of Hungarian food manufacturing companies. It uses an in-depth CATPCA and a PLS-PM approach to analyze the direct and indirect impacts of the IR technologies affect the development of social, ecological and economic factors on corporate performance. This study aims to understand the growing area of research better by exploring the interactions between IR and corporate incomes from a sustainable perspective. Data for this study were collected using 2020-2021 surveys.
The study is structured as follows. This paper begins by reviewing the literature on sustainable concepts and their linkage to IR. It will then go on to designing the data materials. The third section concerns the methodology used for answering the hypotheses described. The fourth section presents the findings of the research. Finally, the conclusion gives a summary and limitations of the findings.
Literature overview
A large and growing body of literature has investigated the concept of Sustainable Development (SD) (Basiago, 1995; Pope et al., 2004; Schoolman et al., 2011; Boyer et al., 2016). Much of the current literature pays particular attention to encompassing economic, social, and ecological (environmental) factors and goals. This threefold approach places sustainability at their intersection (Giddings et al., 2002). According to McKenzie (2004), social development depends on economic and ecological development, and social sustainability is an aspect of development that is on a par with environmental or economic sustainability. However, the three-pillars concept of sustainability is far from being the dominant interpretation in the literature, and its precise meaning has been disputed.
Brown et al. (1987) conducted preliminary work on SD and identified the three contexts in which the concept of sustainability provides a paradigmatic framework. The social perspective focuses on the satisfaction of basic human needs, the ecological on the continued productivity of ecosystems and the protection of scarce resources and biodiversity, and the economic constraints imposed by a sustainable society on economic growth (Eisenmenger et al., 2020). The institutionalizing of SD continued with the “1992 Rio Process”. Central to this was the publication of the ‘Rio Declaration' consisting of 27 principles intended to guide future agendas (Purvis et al., 2019). Following the 2012 World Summit, an Open Working Group was established to develop the UN's Sustainable Development Goals (SDGs) to integrate sustainable development's economic, social and ecological dimensions (United Nations, 2015). More specifically, SDG 9 focuses on promoting sustainable industrialization and encouraging innovation.
IR technologies and their role in sustainability can differ significantly, especially in the context of different industries. In particular, IR is the challenge of moving from conventional technology to smart systems without limiting the sustainability of the industrial economy by addressing all three dimensions and their interconnections in a balanced way (Rosati and Faria, 2019). The sustainable IR transition for small and med-size enterprises (SMEs) can start with digitizing certain operations to support lean manufacturing systems (Ghobakhloo and Fathi, 2020). The green supply chain framework driven by IR technologies directly influences consumer behavior, working processes and transport in sustainable logistics (Sun et al., 2022). IR uses new technologies such as 3D printing, robotics and automated guided vehicles to achieve the optimal solution under different production and sales constraints to maximize corporate profits (Tsai and Lu, 2018). Together, these studies provide insights into the importance of IR and the digitization of the entire value chain (Nhamo et al., 2020).
The evidence presented by Chinese SMEs in the field of precision agriculture confirms that the use of IR technologies has benefits across the three sustainable dimensions (Müller and Voigt, 2018). However, there are different views in the literature on long-term environmental sustainability. The ecological (ECOL) aspect requires agri-food companies to produce quality products and services at the lowest cost and to package them in reusable materials to avoid polluting nature (Coelho et al., 2020). Food companies, logistics providers, retailers and consumers must work together to achieve sustainable solutions to reduce the costs of production, packaging and transport to ensure environmental advances (Bradu et al., 2022). Proper packaging facilitates transportation, and customers will be willing to pay a high price for goods and services that support a green environment, resulting in fewer greenhouse gas emissions (GHGs) and a positive impact on reducing global warming and supporting the expected positive impacts through financial initiatives (Bonilla et al., 2018). The ecological nature of the green technologies, combined with the IR phases, leads to an increase in energy efficiency and a reduction in production waste, but an increase in electrical waste and energy consumption (Yu et al., 2022).
One of the fiercest debates has been about the impact of IR technologies on self-employment. IR is closely related to social (SOC) sustainability because firms offer better working conditions for workers, health and safety are prioritized (Adamik and Nowicki, 2018), and workers can even work from home, ensuring flexibility and reducing pollution (Ahn and Kim, 2017). Others argue that it means greater efficiency in energy use, as workers spend less time at the company, resulting in lower cost production (Jankalová and Jankal, 2018). The argument against home working is that it simply means passing on utility costs to workers' households (Barbieri et al., 2021). Moreover, self-employment can reduce worker productivity and motivation (Patanjali and Bhatta, 2022). New technologies have a negative impact on the labor market because they can lead to job losses, thereby reducing demand, and new technologies can completely undermine economic development (Sachs et al., 2015). Conversely, new technologies make it easier to keep jobs, and employment is expected to overgrow among companies in related food industries. Acemoglu and Restrepo (2018) stated that IR tools are not eliminating but redefining the workplace, and machines are taking over basic daily tasks, allowing workers to focus on activities that require creativity. Technological innovation has a positive impact not only on employment but also on efficient production methods. Indeed, if green products are produced at lower costs, there will be greater demand for new green jobs (van Vuuren et al., 2017).
The economic (ECON) context that should be considered for integrating IR with SD is that companies must conserve available resources to maximize profits and ensure adequate returns for stakeholders. The essence of economic sustainability means that companies need to focus not only on short-term but also on long-term goals so that customers are satisfied with their current purchases (Cox and Pezzullo, 2017). Digital technology adopters expect to make scarce material and energy savings, increase capacity utilization and bring new products to market faster to meet changing demand, thereby creating the essential capabilities needed to exploit the potential of 5IR (Burmeister et al., 2016). The use of big data has supported managers in studying internal and external factors and failures to impact productivity, efficiency and competitiveness by improving business processes (Shahid and Sheikh, 2021). While companies are investing in or planning to invest in, for example, big data, computer modeling and simulations are optimistic about their prospects for returns (Anwar et al., 2018).
The paradoxical nature of corporate sustainability (CS) is the need to simultaneously consider social, ecological and commercial aspects (Luo et al., 2020). The essential principle of CS is that it takes a complex approach to economic development, social needs and ecological requirements into account (Hahn et al., 2018). The primary research objective of the analysis is to find explanations for how each sustainable development path can contribute to higher corporate profitability (PROF), either indirectly or directly, through IR technologies in the case of food manufacturing companies. Typical corporate performance indicators include profit margin and return, sales and annual growth ratios (Pache and Santos, 2013). Figure 1 shows the proposed conceptual framework.
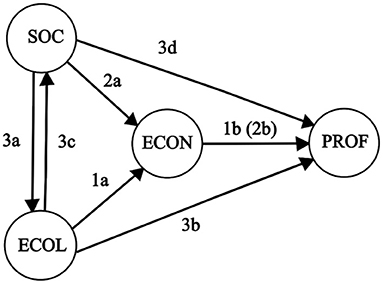
Figure 1. Proposed conceptual framework. ECON: economic, ECOL: ecological, SOC: social and PROF: profitability. The numbers (e.g., 1a, 1b, 2a, 2b, 3a, 3b, 3c and 3d) represent the different SD paths based on McKenzie (2004).
The “livable path” is one in which the ecological footprint, i.e., the environmental burden, is kept low in line with future economic development and environmentally responsible companies tend to be more profitable (Wu et al., 2022). However, traditional economic approaches underestimate the risks from climate change and overestimate the costs of the low-carbon transition, as they do not consider the cumulative gains from path-dependent innovations (Ekins and Zenghelis, 2021).
H1: The sustainable economic factor mediates the impact of the ecological dimension on food corporate profitability (livable path).
The “fair way”, where decent work ensures economic growth, means overconsumption of resources and the depletion of the future. Researchers have generally used synergistic increases in economic and social performance to represent the success of sustainability. However, economic and social aspirations can be detrimental to each other in the short term (Margolis and Walsh, 2003), and impacts on social or environmental performance are assumed to occur only after a long time (Miron-Spektor et al., 2018).
H2: The sustainable economic factor mediates the impact of the social dimension on food corporate profitability (fair path).
The ‘tolerable path' is one in which accessible food and clean water are accompanied by renewable energy affordable for the entire society (McKenzie, 2004). de Amorim et al. (2018) pointed out that water, energy and food are interdependent and essential resources that require sustainable, integrated and intelligent management. The nexus is increasingly seen as a promising approach to address the leadership gaps to the manufacturers in addressing complex and interconnected resource management challenges for enterprises (Pahl-Wostl, 2019).
H3a: The sustainable ecological factor mediates the impact of the social dimension on profitability. Thus, H3b: The social factor mediates the impact of the ecological dimension on corporate profits (tolerable path).
The common area of the three factors (Sustainable path) must be consciously addressed to change the status quo (Hahn et al., 2018). A paradoxical approach to CS explicitly acknowledges the tensions between different desirable but sometimes conflicting sustainability goals (Hahn and Aragón-Correa, 2015). This pragmatic approach allows corporate decision-makers to balance the simultaneous pursuit of competing goals (Schad et al., 2016). Furthermore, it allows for business contributions to sustainable development, as environmental and social considerations are not seen as goals but merely as a means to maximize corporate profits (Schreck, 2011). In this case, firms are making financial revenue and taking environmental and social concerns into account.
H4a: The sustainable ecological and economic factors mediate the impact of the social dimension on food corporate returns. Besides, H4b: The social and economic factors mediate the impact of the ecological dimension on food corporate profitability (sustainable path).
Table 1 summarises the SD pathways associated with IR and corporate profitability, and formulates the following hypotheses based on the indirect (mediation) effects:
Materials and methods
This research was carried out using quantitative pathway models, and a primary questionnaire was used as a research tool to investigate Hungarian food manufacturing businesses following the COVID-19 outbreak. The investigation was conducted typically through online professional events, telephone, and face-to-face inquiries. The surveys were conducted at the beginning of 2020, while the data refer to a two-year period spanning from 2020 to 2021. The composition and structure of the questions were based on the literature collected and on preliminary consultations with the CEOs of the 5 selected food companies and their suggestions. A comprehensive literature review of the variable operationalization was conducted based on ecological (Tang et al., 2016; Bonilla et al., 2018; Gielen et al., 2019), social (Sachs et al., 2015; Jankalová and Jankal, 2018), economic (Westerman et al., 2012; Cox and Pezzullo, 2017) and profitability (Bughin, 2016) dimensions. Some dimensions and items were piloted and feedback, grammatical errors and misunderstandings were corrected. The surveys were then collected with the help of senior and middle managers and IT specialists.
Data design
A Voluntary Questionnaire Was Developed to Measure the Extent to Which IR Technologies Affect the Development of Social, Ecological and Economic Factors in Hungarian Food Manufacturing Companies. A Total of 24 Elements, Each Containing eight Selected Environmental, Economic, and Social Aspects, Are Measured on an Ordinal Scale. Each Item Was Measured on a Five-Point Likert Scale, Where 1 = Strongly Disagree, 2 = Disagree, 3 = Neutral, 4 = Agree, and 5 = Strongly Agree. For the Corporate Return (Profitability) Indicators Were Transformed as Follows: Below 0, 0–5, 5–15, 15–30 and Above 30%. The list and sources of items are shown in Table 2.
Hungarian food industry
Based on KSH (2020) report, the industry contributed 24.3% of the gross value added to the economy in Hungary. It ranks fourth in terms of manufacturing industry share in the EU behind Slovenia (23%), the Czech Republic (24%), and Ireland (38%), making it one of the most industrialized EU countries. Manufacturing, which accounts for around 96 per cent of total production, experienced a 6.0% decline. However, transportation decreased by 11.2%, but less for food, drink, and tobacco (up 0.8 and 1.0%). In 2020, production was growing steadily for seven years, and the pandemic has only partially impacted certain areas. The most significant shortfall was caused by the disappearance of demand generated by tourism and hospitality. Domestic sales, which account for 59% of the total, remained unchanged from the previous year, while export, which account for 41% of the total, rose by 3.4%. The negative impact of the epidemic was most pronounced in meat processing, preserving and preparations, which accounts for almost a quarter of production, and the beverages, which fall by almost the same amount (5.8 and 5.9%). In the poultry and pig sectors, production was also negatively affected by avian influenza and African swine fever. In addition, the production of bakery products, pasta and other food products (such as confectionery) also fell (by 3.4% and 2.3%).
In 2020, there were only 1157 (TEÁOR-10) food companies. However, most did not respond to the questions, citing confidential company information. After the survey, 276 respondents completed the questionnaire, which was reduced to 259 during the data cleaning process, with no missing data or registry errors. In order to have sufficient data for the analysis, 223 or more measurements are needed to have a confidence level of 95% that the real value is within ±5.9% of the surveyed value (Kirby et al., 2002). The sample size is sufficient for further analysis on partial least squares, as at least 200 individuals are needed (Pérez and Esposito, 2010).
Most responses came from meat processing and canning (97), followed by bakery and pasta (60) and then from fruit and vegetable processing and preservation companies (46). The fewest responses came from companies involved in fish processing and preservation, as well as in the production of vegetable and animal oil, since very few manufacturers in Hungary carry out their main activities in this field. 44% of the food companies are aware of and implement IR tools. While 54% of respondents do not intend to make substantial investments, and they hope to maintain the status quo by adjusting to market demands. Keeping up with new developments and inventions is critical for the group of inventors, which accounts for 38.9% of those active in the Hungarian market for more than 5–10 years. Most laggards are new businesses that lack the resources to grow to the appropriate size (Erdei et al., 2021).
Applied methods
The research was conducted using partial least squares path modeling (PLS-PM) and categorical principal component analysis (CATPCA). CATPCA was used to highlight the hypothetical model structure using the principals' function of the gifi package in R 3.4.4 software (R Core Team, 2022; RStudio Team, 2022). The primary aim of the analysis was to combine all the elements (items) in the same block into a single common principal component and then calculate the correlation between each principal component. CATPCA involves a technique called optimal scaling, whereby numerical values are assigned to each category and applied for further evaluation. Different levels of measurement (e.g., nominal and ordinal scales) can be used in the assessment without restriction, and values are assigned using an iterative method called Alternating Least Squares (Linting et al., 2007). Kaiser Meier Oldkin's (KMO) measure of sampling adequacy was also used to assess the reliability of each principal component by the KMO function of the “psych” package of the R 3.4.4 software (Kaiser and Rice, 1974).
The partial least squares path model (PLS-PM) was used in the next step to fit the hypotheses. In regression models, multicollinearity is often a problem, removing highly correlated variables and losing information (Chin, 1998). Despite multicollinearity, the PLS technique can detect a system of structured mediating effects with multiple manifest variables (Tenenhaus et al., 2005). Ramli et al. (2018) stated that the PLS approach may be a better alternative to clustering methods, especially in the case of many categorical predictors and multicollinearity.
In this research, a reflective modeling approach was used for latent constructs like ecological, economic and social factors and a formative modeling approach was used for the profitability indicators. Reflective modeling means that the latent construct exists independently of the measures, the causality runs from the construct to the indicators, and the elements do not substantially change the content validity of the construct, whereas in formative models the indicators are combinations that form the composite latent variable. (Sarstedt et al., 2016). In order to verify the modeling approach, principal component analysis was used for testing the formative model and factor analysis was used for testing the reflective model. Principal component analysis and factor analysis were performed using the “principals” and “fa” functions of the “psych” package in R 3.4.4 software (R Core Team, 2022; RStudio Team, 2022).
Cronbach's alpha can be used for checking the internal consistency of indicators for each latent variable (Alpha > 0.7) (Cronbach, 1951). The bootstrapping technique proposed by Chin (1998) was used to validate the model with 500 replicates. The model's overall fit was measured using the Goodness of Fit (GoF) test, where a GoF above 0.6 indicates an excellent model fit (Tenenhaus et al., 2005). Dillon Goldstein's rho indexes tested the blocks' composite reliability (CR). The identified dimensions should have a value above the recommended 0.7, and factor loadings should exceed 0.6–0.7 (Hair et al., 2016). R2 values were calculated to assess the quality of the structural model, where values of 0.02, 0.15, and 0.35 are classified as small, medium or large effects (Cohen, 1988). The Fornell and Larcker criterion was used to check the discriminant power of the model. In addition, the average variance extracted (AVE > 0.5) was used to assess discriminant validity in the study (Fornell and Larcker, 1981). Model fitting and estimates were performed using the'plspm' package in R 3.4.4 (Kaiser and Rice, 1974).
Results
As a first step, four blocks were examined, i.e., ecological, social, economic and business profitability performance (Table 3). The Cronbach's Alpha Value of the Scale Items Is High and Acceptable, Especially for the Ecological Factor (0.930). The Descriptive Statistics and the Cross and Factor Loadings Are Examined After the Exclusion. The two Highest Values per Block Were Obtained for ECOL5 and ECOL6; SOC4 and SOC5; ECON4 and ECON7. Only Those With a Value of Around 0.7 or More Were Kept. Therefore, the Final Path Model Excluded ECOL3, SOC1 SOC7 and ROI. After Exclusion, Almost all Other Loadings in the Final Model Improved. The Ecological Dimension (ECOL) Is Best Described by “Recycling” and “Packaging“ Items (0.977). The Latent Social Variable (SOC) Is Mainly Correlated With ”Robots Displace Manual Labor“ (0.833), While the Economic Variable (ECON) Is Best Correlated With ”Producing Quality Products“ (0.871) and ”Competitiveness" (0.878). Cross Loadings Are Lower for all Items Compared to PROF.
In the Subsequent Analysis Stage, a Preliminary CATPCA Analysis Was Carried out on all the Items in the Same Block to Reduce the Dataset and Generate Latent Variables. Table 4 Presents the Reliability Measures and Pearson Correlations Between the Principal Components. The Lower Triangular Matrix Shows the Correlation Coefficients, While the Upper one Shows the Corresponding p-Values in Parentheses. On the one Hand, the Total Measure of Sampling Adequacy (MSA) Values Measured by KMOs Were Higher Than the Required Value of 0.7. On the Other Hand, the Explained Variances (%) Were Above the Required Level of 50 %. The Best Correlation Between ECOL and ECON (0.721) Was Found, but ECOL Was Also Strongly Correlated With the SOC (0.613). Regarding the Correlation With PROF, the Best Relationship Was Found Between ECON and PROF (0.374).
Based on the results of the CATPCA analysis and the literature review, the following path model could be constructed (see Figure 2). Figure 2 shows the parameter estimates of the models.
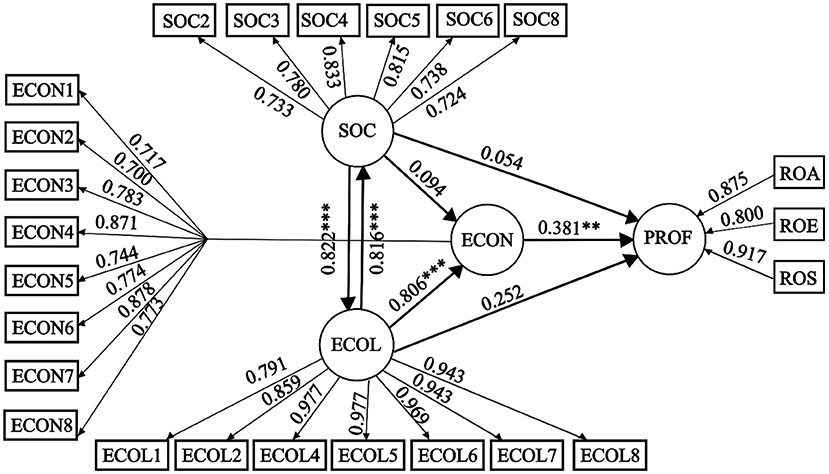
Figure 2. The final path model and coefficient estimates. **: p < 0.05.***: p < 0.001. Source: Authors' compilation.
A bootstrap simulation was used to validate the parameters estimated by the mean and standard errors of the path coefficients. Only path coefficients with a standard error of 50% or less of the mean were considered statistically significant. Not all relationships were significant, as formerly assumed based on the CATPCA results. The PLS-PM model considered the direct relationship between ECON and PROF, and the path coefficients of ECOL, SOC to PROF irrelevant based on the p-values, pseudo t-statistics, and standard errors. The sustainable economic dimension positively impacts on corporate business returns. In other words, the effects of increased IR factors tend to be resulted in more profitable food manufacturing companies. Likewise, the clearest direct relationship is found between SOC and ECOL (B = 0.822; SE = 0.070; t = 11.74; p < 0.001), and reciprocal (B = 0.816; SE = 0.073; t = 11.18; p < 0.001). However, ECOL will also be strongly directly related to ECON (B = 0.806; SE = 0.111; t = 7.26; p < 0.001), which has a positive impact on business profitability (B = 0.381; SE = 0.226; t = 1.69; p = 0.047).
The overall model showed an excellent global fit, and the internal and external model quality was excellent, as the GoF was 0.649. The main diagonal of the numbered columns of the matrix is shown in Table 5. The AVE values are in italics, which express the percentage of the variance of the items explained by a given latent variable (LV). The squared Pearson correlation coefficients are given below the main diagonal. In contrast, the correlation coefficients' significances (i.e., p-values in parentheses) are shown above the main diagonal. It is evident from the AVE values that each LV explained at least 50% of the variance of the items on average. Discriminant validity was also satisfactory, as the correlations for each LV were lower than the AVE values. The proportion of variance explained by the coefficient of determination (R2) in these regressions was significant. R2 values were large, the Dillon-Goldstein rho was above 0.7, and the AVE (Average Variance Extracted) values were also acceptable and more significant than 0.5. The last two columns of Table 5 justified the modeling approach. The reflective model was tested throughout a factor analysis, while a principal component analysis tested the formative one. The explained variances of creating a single factor or component were not significantly different except in the Profitability latent variable case. On the other hand, when the model was run with only formative relationships, the goodness and interpretability were decreased to a large extent.
The main advantage of PLS-PM is the ability to distinguish between direct, indirect and aggregate (total) effects on the outcome variable, i.e., corporate profitability (Table 6). Examining the direct and indirect relationships revealed that ECOL and SOC indirectly affect PROF, and the direct effects were insignificant. The strongest relationship of total effect was found between ECOL and ECON (total = 0.883; SE = 0.075; t = 11.77; p < 0.001). Among the factors influencing PROF, ECOL had the largest total effect (total = 1.007; SE = 0.170; t = 5.92; p < 0.001), followed by SOC (total = 0.610; SE = 0.135; t = 4.52; p < 0.001) and ECON (total = 0.381; SE = 0.226; t = 1.69; p = 0.047). Regarding percentages, ECOL has a 66% effect on profitability, while SOC and ECON have a 24% and 9% influence, respectively.
Table 7 shows that only hypotheses H1 and H4a are supported in order at the 5% significance level. Rucker et al. (1986) showed that the smaller the sample, the more appropriate it is to set a more stringent p-value. Only the livable path (ECOL-ECON) and a sustainable path (SOC-ECOL-ECON) significantly impacted corporate profitability (PROF). Consequently, the food producing businesses whose managers think along the viable and sustainable path tend to be more profitable. The livable path or indirect (mediating) effects of economic (ECON) factors strengthened the positive (0.307*** = 0.806 × 0.381) effect on profitability. Also, the latent economic variable fully mediates (strengthens) the ecological factor's (ECOL) effect on profitability (PROF). It plays an intermediate role in the positive relationship between the independent and dependent variables. H1 accepted. Thus, the sustainable path hypotheses focus on how the independent (sustainable IR specific) factors affect the dependent variable (profitability) through one or more potential intervening variables or mediators. Only the positive impact of social variables (SOC) on corporate profitability is fully mediated and strengthened by the ECOL and ECON IR dimensions (0.252 = 0.822*0.806*0.381). However, the direct effect of social factors on profitability is insignificant, whereas the indirect effect of SOC and PROF was substantial. H4a is accepted, and H4b should be rejected.
Discussion
The primary objective was to identify the direct and indirect impacts of sustainable dimensions, i.e., ecological, economic, and social factors that can contribute to increased corporate profitability through IR technologies.
The study found that the economic IR dimension positively impacts corporate profitability. Another important finding was that ecological factors substantially effect on profitability, while social and economic factors have fewer impacts than environmental ones. Interestingly, in all cases of this study, only the livable path and one sustainable path significantly impacted food corporate profitability. In other words, food manufacturing businesses whose managers think along these lines tend to be more profitable. The economic factor positively mediates the impacts of the environmental dimension on corporate returns. Furthermore, the ecological and economic factors strengthened the positive impact of the social dimension on corporate profitability. In summary, the sustainable IR approach proposed in this analysis is an original and useful tool for the evaluation of food corporate performance in three dimensions: economic, social and environmental. However, with small sample size, caution must be applied, as the findings might not be transferable to entire industries.
There are several possible explanations for these results. The findings further support the idea of the circular economy that ensures an equal distribution of resources so that companies operate efficiently and effectively, the environment is preserved and is able to regenerate for the future (Jørgensen and Pedersen, 2018). Sustainability and ecological impact have become increasingly critical differentiating factors between competing products and services. Consumer Social Responsibility (CSR) initiatives with green, healthy products and services can have an impact on business processes, such as the entire supply chain processes and product life-cycles (Nazzaro et al., 2020). The circular economy aims to increase productivity through more efficient use of natural resources and ecosystems to keep products at their highest value (Pajula et al., 2017).
The findings of the study provide useful implications for theorists, policy makers and practitioners alike, as they contribute to the debate on the role of the IR in considering sustainability in the success of agri-food companies. The CS concerns, which may conflict with corporate profitability objectives, are not ignored. Instead, conflicting sustainability factors are juxtaposed without highlighting one as the 'better solution' (Hahn et al., 2018). Results keeps the door open to the performance paradox and serves as a call for simultaneous action on various economic, social and environmental issues. The paradoxical approach does not mean that firms abandon the profit-driven strategy. Instead, paradox resolution refers to a deliberate iteration between alternatives to gain equal attention over time (Smith and Lewis, 2011). The paradoxical perspective creates scope for meaningful corporate contributions to sustainable development by purposefully balancing and combining instrumental (IR) initiatives where companies addressing environmental and social issues bring business benefits (Hahn et al., 2016).
CS paradox perspective also suggest that society, businesses, logistics providers and consumers must work together to achieve sustainable outcomes that improve overall financial, social, economic and environmental performance (Agyabeng-Mensah et al., 2020). A possible explanation for these results could be that sustainability enhances operating performance, efficiency and effectiveness, minimizes resource use and costs, and benefits society by offering less harmful products and (ICT) services in the most diminutive possible form (Biagi and Falk, 2017). Tsai (2018) has developed a model using 5IR techniques of green production planning and control to maximize profits and reduce carbon emissions, recycling and waste reuse through activity-based costing, which has already been proven in paper, printing and textiles industries, but is also promising in the food sector.
The present results are significant in at least two major respects. Firstly, ecological factors may play a more crucial role in the profitability of the food industry than in other industries, as the sector is highly vulnerable to scarcity of natural and food resources due to climate change (Leisner, 2020). Involving farmers in sustainable agricultural practices and applying food innovations can contribute to resilient economic and employment recovery, avoiding land abandonment and environmental degradation (Pancino et al., 2019). Further factors, e.g., sustainable, innovative approaches, can be integrated to allow food plants to use waste to feed the energy system, such as coal-fired power plants, instead of further deforestation (Chen et al., 2020). On the other hand, the focus shift from technology-driven advancement to a human-centric approach is one of the most substantial paradigmatic transitions defining 5IR (Breque et al., 2021). It implies that the food manufacturing sector must consider societal restrictions relating to a healthy and safe working environment, respect for human rights, and the qualifications needed for employees. The evolving position of the industrial worker and the narrative surrounding them suggest that the employer is interested in investing in their workforce's talents, skills, and general wellbeing to meet their goals for profitability. Measures of the social dimension of the path models focus on human-machine interactions such as employment, poverty and gender equality and do not take into account the importance of food security issues. However, social IR dimension has crucial impact on profitability by providing affordable access to certain foods and services to achieve competitiveness by making production processes more efficient and improving economic performance and market position (Stanco et al., 2020). Consequently, sustainable IR will bring positive results, both in terms of consumer reactions and of producers, who will pay more attention to working conditions and the quality, wholeness and origin of food (Vermeir et al., 2020). Some of other findings relate specifically to the different accounting standards and legal regimes that significantly impact companies' investment opportunities. For instance, corporate taxation incentives, preferential loans and subsidies contribute even more to encouraging food producing companies and accomplishing higher revenues (Kostakis et al., 2016).
Further research is needed to examine how should managers make decisions to balance the different dimensions of sustainability in IR investments to stay profitable. This empirical framework provides guidance for research on how sustainable venture capital can help startups and nascent entrepreneurs succeed. Sustainable entrepreneurs seek to address economic prosperity, social justice and ecological resilience through entrepreneurial behavior (Bocken, 2015). Sustainable entrepreneurship is about preserving nature, ecosystems and communities while creating benefits for individuals, the economy and society (Rosário et al., 2022).
Conclusions
The present study was designed to determine the impacts of sustainable IR factors from economic, social and ecological perspectives in the food production sector, where appropriate supply utilization and minimal adverse environmental impacts are crucial for the future. The main objective of the study was to propose explanations for analyzing how each sustainability pathways can contribute to higher corporate profitability.
This research was conducted with quantitative models using partial least squares path modeling (PLS-PM) and categorical principal component analysis (CATPCA). The advantage of CATPCA implies an optimal scaling technique, whereby numerical values are assigned to each category and applied for further evaluation. PLS-PM is mainly used to develop theories for exploratory research (Henseler et al., 2016). The method is recommended for cases such as lower sample size requirements, easier testing of direct and mediating (indirect) relationships and built-in ability to handle formative indicators (Sarstedt et al., 2016).
The present study makes several noteworthy contributions to the CS literature. This study has shown that the livable and sustainable path hypotheses can be confirmed. However, the economic dimension of sustainable IR impacts positively and significantly on corporate profitability. The ecological and economic factors mediate and strengthen the positive impact of the social dimension on corporate profitability. Contrary to previous approaches (Yolles, 2018), it is not only the natural environment that determines the structure and functioning of society, and the economy must respond to it. The results suggest that this sustainable path is one where the environment and the economy determine the functioning of societies. Together, these results suggest that sustainability through IR plays a crucial role in improving food manufacturing companies' profitability. However, decision-makers should not wait for sustainability to emerge but consciously embrace it. The transition into sustainability is a long journey, full of questions, but it provides a direction for the necessary changes (Kot et al., 2019).
The results support the idea that sustainable development requires responsible financial management for changes in the global food systems, affecting farmers, food supply chains, production companies, different food production systems and local ecologies, and the diversity of food traditions and cultures (Bhat and Jõudu, 2019). Another crucial practical implication confirms that adopting IR tools support improved business performance (Kovacs and Kot, 2016; Rajnoha et al., 2017). Internet of Things (IoT), e.g., Big Data, RFID, helps managers study internal and external factors that significantly impact sales and profits (Nagy et al., 2018). For similar efforts (see SOC, ECOL linkage), by extending paths from multiple directions and path models, the techniques used in this paper can be applied to other indirect links in any field of the social sciences.
Finally, several limitations need to be considered. First, the study is limited by the lack of information on the Hungarian sectoral case, which restricts the generalizability of the results. The omitted variables bias is a major limitation of this study, as the variables included in the models reflect only subjective selections and do not provide a complete picture of IR sustainability factors. We urge researchers to evaluate additional interactions in the context of agri-food industry, such as hunger reduction, water pollution, and soil degradation, as vital social and ecological factors related to food security and climate change. In the future, researchers should look at organizations from different industries to better understand the dimensions of sustainability and the impact of technological changes. The data collection method used in this study was an opinion-based survey, which suffers from the potential problem of common method bias, which describes measurement error exacerbated by the conscious perceptions of respondents who choose to provide only positive responses (Chang et al., 2010). The most crucial concern is that the study used a cross-sectional approach, which limits the conclusions that can be drawn. The effects of food and energy crises caused by the current war conflicts are not reflected. The current study has only examined the profitability performance and expected returns of food manufacturing companies. More research is required to determine the usefulness of such vital financial indicators as liquidity, efficiency and leverage.
Data availability statement
The original contributions presented in the study are included in the article/Supplementary material, further inquiries can be directed to the corresponding author.
Ethics statement
Written informed consent from the participants was not required to participate in this study in accordance with the national legislation and the institutional requirements.
Author contributions
Conceptualisation: DM. Methodology and software: SK. Validation: EE. Resources: ZB. Writing—review and editing: NE. Visualization and funding acquisition: JO. All authors contributed to the article and approved the submitted version.
Funding
The research was funded by the ÚNKP-22-5-DE-406 New National Excellence Program of the Ministry for Innovation and Technology from the source of the National Research, Development and Innovation Fund, Hungary.
Acknowledgments
This work was supported by the János Bolyai Research Scholarship of the Hungarian Academy of Sciences. This research was supported by the ÚNKP-22-5-DE-406 New National Excellence Program of the Ministry for Innovation and Technology from the source of the National Research, Development and Innovation Fund.
Conflict of interest
The authors declare that the research was conducted without any commercial or financial relationships construed as a potential conflict of interest.
Publisher's note
All claims expressed in this article are solely those of the authors and do not necessarily represent those of their affiliated organizations, or those of the publisher, the editors and the reviewers. Any product that may be evaluated in this article, or claim that may be made by its manufacturer, is not guaranteed or endorsed by the publisher.
Supplementary material
The Supplementary Material for this article can be found online at: https://www.frontiersin.org/articles/10.3389/fsufs.2022.1034010/full#supplementary-material
References
Acemoglu, D., and Restrepo, P. (2018). The race between man and machine: implications of technology for growth, factor shares, and employment. Am. Econ. Rev. 108, 1488–1542. doi: 10.1257/AER.20160696
Adamik, A., and Nowicki, M. (2018). Preparedness of companies for digital transformation and creating a competitive advantage in the age of Industry 4.0. Proceed. Int. Conf. Business Excel. 12, 10–24. doi: 10.2478/PICBE-2018-0003
Agyabeng-Mensah, Y., Afum, E., and Ahenkorah, E. (2020). Exploring financial performance and green logistics management practices: Examining the mediating influences of market, environmental and social performances. J. Clean. Prod. 258, 120613. doi: 10.1016/j.jclepro.2020.120613
Ahn, S.-Y., and Kim, S.-H. (2017). What makes firms innovative? The role of social capital in corporate innovation. Sustainability 9, 1564. doi: 10.3390/su9091564
Anwar, M., Khan, S. Z., and Shah, S. Z. A. (2018). Big data capabilities and firm's performance: a mediating role of competitive advantage. J. Inf. Knowl. Manag. 17, 1850045. doi: 10.1142/S0219649218500454
Barbieri, B., Balia, S., Sulis, I., Cois, E., Cabras, C., Atzara, S., et al. (2021). Don't call it smart: working from home during the pandemic crisis. Front. Psychol. 12, 4133. doi: 10.3389/FPSYG.2021.741585/BIBTEX
Basiago, A. D. (1995). Methods of defining ‘sustainability.' Sustain. Dev. 3, 109–119. doi: 10.1002/SD.3460030302
Bhat, R., and Jõudu, I. (2019). “Emerging issues and challenges in agri-food supply chain,” in Sustainable Food Supply Chains (Amsterdam: Elsevier), 23–37. doi: 10.1016/B978-0-12-813411-5.00002-8
Biagi, F., and Falk, M. (2017). The impact of ICT and e-commerce on employment in Europe. J. Policy Model 39, 1–18. doi: 10.1016/J.JPOLMOD.2016.12.004
Bocken, N. M. P. (2015). Sustainable venture capital – catalyst for sustainable start-up success? J. Clean. Prod. 108, 647–658. doi: 10.1016/J.JCLEPRO.2015.05.079
Bojnec, Š., and Tomšič, N. (2020). Corporate sustainability and enterprise performance. Int. J. Prod. Perf. Manag. 70, 21–39. doi: 10.1108/IJPPM-05-2019-0226
Bonilla, S., Silva, H., Terra da Silva, M., Franco Gonçalves, R., and Sacomano, J. (2018). Industry 4.0 and sustainability implications: a scenario-based analysis of the impacts and challenges. Sustainability 10, 3740. doi: 10.3390/su10103740
Boyer, R., Peterson, N., Arora, P., and Caldwell, K. (2016). Five approaches to social sustainability and an integrated way forward. Sustainability 8, 878. doi: 10.3390/su8090878
Bradu, P., Biswas, A., Nair, C., Sreevalsakumar, S., Patil, M., Kannampuzha, S., et al. (2022). Recent advances in green technology and Industrial Revolution 4.0 for a sustainable future. Environ. Sci. Pollut. Res. 1, 1–32. doi: 10.1007/s11356-022-20024-4
Breque, M., de Nul, L., and Petridis, A. (2021). Industry 5.0—Publications Office of the EU. Brussels, European Commission. Available online at: https://op.europa.eu/en/publication-detail/-/publication/468a892a-5097-11eb-b59f-01aa75ed71a1/ (accessed August 6, 2022).
Brown, B. J., Hanson, M. E., Liverman, D. M., and Merideth, R. W. (1987). Global sustainability: toward definition. Environ. Manag. 11, 713–719. doi: 10.1007/BF01867238
Burmeister, C., Luettgens, D., and Piller, F. T. (2016). Business model innovation for industrie 4.0: why the “Industrial Internet” mandates a new perspective on innovation. Die Unternehmung. 70, 124–152. doi: 10.5771/0042-059X-2016-2-124
Chang, S. J., van Witteloostuijn, A., and Eden, L. (2010). From the editors: common method variance in international business research. J. Int. Bus Stud. 41, 178–184. doi: 10.1057/JIBS.2009.88/FIGURES/1
Chen, H., Zhang, M., Xue, K., Xu, G., Yang, Y., Wang, Z., et al. (2020). An innovative waste-to-energy system integrated with a coal-fired power plant. Energy 194, 116893. doi: 10.1016/J.ENERGY.2019.116893
Chin, W. W. (1998). “The partial least squares approach for structural equation modeling,” in Modern Methods for Business Research, ed. G. A. Marcoulides (Mahwah: Lawrence Erlbaum Associates Publishers), 295–336. Available online at: https://psycnet.apa.org/record/1998-07269-010 (accessed July 18, 2022).
Coelho, P. M., Corona, B., ten Klooster, R., and Worrell, E. (2020). Sustainability of reusable packaging–Current situation and trends. Resourc. Conserv. Recycl. 6, 100037. doi: 10.1016/j.rcrx.2020.100037
Cohen, J. (1988). Statistical Power Analysis for the Behavioral Sciences, 2nd edn. Hillside, USA: Lawrence Earlbaum Association.
Cox, J. R., and Pezzullo, P. C. (2017). Environmental Communication and the Public Sphere. London, UK: SAGE Publications.
Cronbach, L. J. (1951). Coefficient alpha and the internal structure of tests. Psychometrika 16, 297–334. doi: 10.1007/BF02310555
Cugno, M., Castagnoli, R., and Büchi, G. (2021). Openness to industry 4.0 and performance: the impact of barriers and incentives. Technol. Forecast. Soc. Chang. 168, 120756. doi: 10.1016/J.TECHFORE.2021.120756
de Amorim, W. S., Valduga, I. B., Ribeiro, J. M. P., Williamson, V. G., Krauser, G. E., Magtoto, M. K., et al. (2018). The nexus between water, energy, and food in the context of the global risks: An analysis of the interactions between food, water, and energy security. Environ. Impact Assess Rev 72, 1–11. doi: 10.1016/j.eiar.2018.05.002
Eisenmenger, N., Pichler, M., Krenmayr, N., Noll, D., Plank, B., Schalmann, E., et al. (2020). The sustainable development goals prioritize economic growth over sustainable resource use: a critical reflection on the SDGs from a socio-ecological perspective. Sustain. Sci. 15, 1101–1110. doi: 10.1007/s11625-020-00813-x
Ejsmont, K., Gladysz, B., and Kluczek, A. (2020). Impact of industry 4.0 on sustainability—bibliometric literature review. Sustainability 12, 5650. doi: 10.3390/su12145650
Ekins, P., and Zenghelis, D. (2021). The costs and benefits of environmental sustainability. Sustain. Sci. 16, 949–965. doi: 10.1007/S11625-021-00910-5/FIGURES/5
Erdei, E., Popp, J., Neményi, M., and Oláh, J. (2021). Az Ipar 4.0 technológiák szerepe az élelmiszergyártó vállalatok innovációs tevékenységében. Statisztikai Szemle 99, 978–996. doi: 10.20311/stat2021.10.hu0978
Fornell, C., and Larcker, D. F. (1981). Evaluating structural equation models with unobservable variables and measurement error. J. Marketing Res. 18, 1–39. doi: 10.2307/3151312
Ghobakhloo, M., and Fathi, M. (2020). Corporate survival in Industry 4.0 era: the enabling role of lean-digitized manufacturing. J. Manuf. Technol. Manag. 31, 1–30. doi: 10.1108/JMTM-11-2018-0417/FULL/PDF
Giddings, B., Hopwood, B., and O'Brien, G. (2002). Environment, economy and society: fitting them together into sustainable development. Sustain. Dev. 10, 187–196. doi: 10.1002/SD.199
Gielen, D., Boshell, F., Saygin, D., Bazilian, M. D., Wagner, N., and Gorini, R. (2019). The role of renewable energy in the global energy transformation. Energy Strat. Rev. 24, 38–50. doi: 10.1016/J.ESR.2019.01.006
Hahn, T., and Aragón-Correa, J. A. (2015). Toward cognitive plurality on corporate sustainability in organizations: the role of organizational factors. Organ. Environ. 28, 255–263. doi: 10.1177/1086026615604446/ASSET/1086026615604446.FP.PNG_V03
Hahn, T., Figge, F., Pinkse, J., and Preuss, L. (2018). A paradox perspective on corporate sustainability: descriptive, instrumental, and normative aspects. J. Business Ethic. 148, 235–248. doi: 10.1007/S10551-017-3587-2/FIGURES/2
Hahn, T., Pinkse, J., Preuss, L., and Figge, F. (2016). Ambidexterity for corporate social performance. Organ. Stud. 37, 213–235. doi: 10.1177/0170840615604506
Hair, J. F., Hult, G. T. M., Ringle, C. M., and Sarstedt, M. (2016). A Primer on Partial Least Squares Structural Equation Modeling (PLS-SEM). Second. SAGE Publications, Inc.
Hartmann, M. (2011). Corporate social responsibility in the food sector. Eur. Rev. Agric. Econ. 38, 297–324. doi: 10.1093/erae/jbr031
Henseler, J., Hubona, G., and Ray, P. A. (2016). Using PLS path modeling in new technology research: updated guidelines. Ind. Manag. Data Syst. 116, 2–20. doi: 10.1108/IMDS-09-2015-0382/FULL/PDF
Ibn-Mohammed, T., Mustapha, K. B., Godsell, J., Adamu, Z., Babatunde, K. A., Akintade, D. D., et al. (2021). A critical analysis of the impacts of COVID-19 on the global economy and ecosystems and opportunities for circular economy strategies. Resour. Conserv. Recycl. 164, 105169. doi: 10.1016/J.RESCONREC.2020.105169
Jankalová, M., and Jankal, R. (2018). Sustainability assessment according to the selected business excellence models. Sustainability 10, 3784. doi: 10.3390/su10103784
Jørgensen, S., and Pedersen, L. J. T. (2018). The Circular Rather than the Linear Economy. (Palgrave Macmillan, Cham), 103–120. doi: 10.1007/978-3-319-91971-3_8
Kaiser, H. F., and Rice, J. (1974). Little Jiffy, Mark Iv. Educ. Psychol. Meas. 34, 111–117. doi: 10.1177/001316447403400115
Kamble, S. S., Gunasekaran, A., and Sharma, R. (2018). Analysis of the driving and dependence power of barriers to adopt industry 4.0 in Indian manufacturing industry. Comput. Ind. 101, 107–119. doi: 10.1016/J.COMPIND.2018.06.004
Kirby, A., Gebski, V., and Keech, A. C. (2002). Determining the sample size in a clinical trial. Med. J. Austr. 177, 256–257. doi: 10.5694/J.1326-5377.2002.TB04759.X
Kostakis, V., Roos, A., and Bauwens, M. (2016). Towards a political ecology of the digital economy: Socio-environmental implications of two competing value models. Environ. Innov. Soc. Transit. 18, 82–100. doi: 10.1016/J.EIST.2015.08.002
Kot, S., Haque, A., and Kozlovski, E. (2019). Strategic SCM's mediating effect on the sustainable operations: multinational perspective. Organizacija 52, 219–235. doi: 10.2478/orga-2019-0014
Kovacs, G., and Kot, S. (2016). New logistics and production trends as the effect of global economy changes. Polish J. Manag. Stud. 14, 115–126. doi: 10.17512/pjms.2016.14.2.11
KSH (2020). Helyzetkép az Iparról 2020. Available online at: https://www.ksh.hu/docs/hun/xftp/idoszaki/jelipar/2020/index.html (accessed October 1, 2022).
Lee, S. M., and Trimi, S. (2018). Innovation for creating a smart future. J. Innov. Knowl. 3, 1–8. doi: 10.1016/J.JIK.2016.11.001
Leisner, C. P. (2020). Review: climate change impacts on food security—focus on perennial cropping systems and nutritional value. Plant Sci. 293, 110412. doi: 10.1016/j.plantsci.2020.110412
Linting, M., Meulman, J. J., Groenen, P. J. F., and van der Koojj, A. J. (2007). Nonlinear principal components analysis: introduction and application. Psychol. Methods 12, 336–358. doi: 10.1037/1082-989X.12.3.336
Lu, Y., Adrados, J. S., Chand, S. S., and Wang, L. (2021). Humans are not machines—anthropocentric human–machine symbiosis for ultra-flexible smart manufacturing. Engineering 7, 734–737. doi: 10.1016/J.ENG.2020.09.018
Luo, B. N., Tang, Y., Chen, E. W., Li, S., and Luo, D. (2020). Corporate sustainability paradox management: a systematic review and future Agenda. Front Psychol 11, 3149. doi: 10.3389/FPSYG.2020.579272/BIBTEX
Margolis, J. D., and Walsh, J. P. (2003). Misery loves companies: rethinking social initiatives by business. Adm. Sci. Q. 48, 268–305. doi: 10.2307/3556659
McKenzie, S. (2004). Social Sustainability: Towards Some Definitions. University of South Australia Magill, South Australia. Available online at: http://www.hawkecentre.unisa.edu.au/institute/ (accessed August 6, 2022).
Miron-Spektor, E., Ingram, A., Keller, J., Smith, W. K., and Lewis, M. W. (2018). Microfoundations of organizational paradox: the problem is how we think about the problem. Acad. Manag. J. 61, 26–45. doi: 10.5465/amj.2016.0594
Młody, M., and Weinert, A. (2020). Industry 4.0 in Poland: A Systematic Literature Review and Future Research Directions. Springer Proceedings in Business and Economics, 43–71. doi: 10.1007/978-3-030-30549-9_2/TABLES/8
Müller, J. M., and Voigt, K.-I. (2018). Sustainable industrial value creation in SMEs: a comparison between industry 4.0 and made in China 2025. Int. J. Precis. Eng. Manuf. Green Technol. 5, 659–670. doi: 10.1007/s40684-018-0056-z
Nagy, J., Oláh, J., Erdei, E., Máté, D., and Popp, J. (2018). The role and impact of industry 4.0 and the internet of things on the business strategy of the value Chain—the case of hungary. Sustainability 10, 3491. doi: 10.3390/su10103491
Nazzaro, C., Stanco, M., and Marotta, G. (2020). The life cycle of corporate social responsibility in agri-food: value creation models. Sustainability 12, 1287. doi: 10.3390/su12041287
Nhamo, G., Nhemachena, C., and Nhamo, S. (2020). Using ICT indicators to measure readiness of countries to implement industry 4.0 and the SDGs. Environ. Econ. Policy Stud. 22, 315–337. doi: 10.1007/s10018-019-00259-1
Pache, A.-C., and Santos, F. (2013). Inside the hybrid organization: selective coupling as a response to competing institutional logics. Acad. Manag. J. 56, 972–1001. doi: 10.5465/amj.2011.0405
Pahl-Wostl, C. (2019). Governance of the water-energy-food security nexus: a multi-level coordination challenge. Environ. Sci. Policy 92, 356–367. doi: 10.1016/j.envsci.2017.07.017
Pajula, T., Behm, K., Vatanen, S., and Saarivuori, E. (2017). “Managing the life cycle to reduce environmental impacts,” in Dynamics of Long-Life Assets (Cham: Springer International Publishing), 93–113. doi: 10.1007/978-3-319-45438-2_6
Pancino, B., Blasi, E., Rappoldt, A., Pascucci, S., Ruini, L., and Ronchi, C. (2019). Partnering for sustainability in agri-food supply chains: the case of Barilla sustainable farming in the Po Valley. Agric. Food Econ. 7, 1–10. doi: 10.1186/S40100-019-0133-9/FIGURES/1
Patanjali, S., and Bhatta, N. M. K. (2022). Work from home during the pandemic: the impact of organizational factors on the productivity of employees in the IT industry. Vis. J. Business Perspect. 097226292210741. doi: 10.1177/09722629221074137
Pérez, F., and Esposito, L. (2010). The global addiction and human rights: insatiable consumerism, neoliberalism, and harm reduction. Perspect. Global Dev. Technol. 9, 84–100. doi: 10.1163/156914910X487933
Pope, J., Annandale, D., and Morrison-Saunders, A. (2004). Conceptualising sustainability assessment. Environ. Impact Assess. Rev. 24, 595–616. doi: 10.1016/J.EIAR.2004.03.001
Purvis, B., Mao, Y., and Robinson, D. (2019). Three pillars of sustainability: in search of conceptual origins. Sustain. Sci. 14, 681–695. doi: 10.1007/S11625-018-0627-5/FIGURES/1
R Core Team, R. (2022). A Language and Environment for Statistical Computing (R Foundation for Statistical Computing. Available online at: https://www.eea.europa.eu/data-and-maps/indicators/oxygen-consuming-substances-in-rivers/r-development-core-team-2006 (accessed January 17, 2022).
Rajnoha, R., Lesníková, P., and Krajčík, V. (2017). Influence of business performance measurement systems and corporate sustainability concept to overal business performance: “save the planet and keep your performance.” E+M Ekonomie a Management 20, 111–128. doi: 10.15240/tul/001/2017-1-008
Ramli, N. A., Latan, H., and Nartea, G. v. (2018). Partial Least Squares Structural Equation Modeling, eds. N. K. Avkiran and C. M. Ringle (Cham: Springer International Publishing). doi: 10.1007/978-3-319-71691-6
Rosário, A., Raimundo, R., and Cruz, S. (2022). Sustainable entrepreneurship: a literature review. Sustainability 14, 5556. doi: 10.3390/su14095556
Rosati, F., and Faria, L. G. D. (2019). Addressing the SDGs in sustainability reports: the relationship with institutional factors. J. Clean. Prod. 215, 1312–1326. doi: 10.1016/j.jclepro.2018.12.107
RStudio Team (2022). RStudio | Open Source and Professional Software for Data Science Teams. Available online at: https://www.rstudio.com/ (accessed July 18, 2022).
Rucker, D. D., Preacher, K. J., Tormala, Z. L., and Petty, R. E. (1986). Mediation analysis in social psychology: current practices and new recommendations. Soc. Person. Psychol. Compass 5, 359–371. doi: 10.1111/j.1751-9004.2011.00355.x
Sachs, J. D., Benzell, S. G., and LaGarda, G. (2015). Robots: curse or blessing? Basic Framework. 1–29. doi: 10.3386/W21091
Sarstedt, M., Hair, J. F., Ringle, C. M., Thiele, K. O., and Gudergan, S. P. (2016). Estimation issues with PLS and CBSEM: Where the bias lies! J. Bus. Res. 69, 3998–4010. doi: 10.1016/J.JBUSRES.2016.06.007
Schad, J., Lewis, M. W., Raisch, S., and Smith, W. K. (2016). Paradox research in management science: looking back to move forward. Acad. Manag. Ann. 10, 5–64. doi: 10.5465/19416520.2016.1162422
Schoolman, E. D., Guest, J. S., Bush, K. F., and Bell, A. R. (2011). How interdisciplinary is sustainability research? Analyzing the structure of an emerging scientific field. Sustain. Sci. 7, 67–80. doi: 10.1007/S11625-011-0139-Z
Schreck, P. (2011). Reviewing the business case for corporate social responsibility: new evidence and analysis. J. Bus. Ethic. 103, 167–188. doi: 10.1007/s10551-011-0867-0
Shahid, N. U., and Sheikh, N. J. (2021). Impact of big data on innovation, competitive advantage, productivity, and decision making: literature review. Open J. Bus. Manag. 9, 586–617. doi: 10.4236/ojbm.2021.92032
Smith, W. K., and Lewis, M. W. (2011). Toward a theory of paradox: a dynamic equilibrium model of organizing. Acad. Manag. Rev. 36, 381–403. doi: 10.5465/amr.2009.0223
Stanco, M., Nazzaro, C., Lerro, M., and Marotta, G. (2020). Sustainable collective innovation in the agri-food value Chain: the case of the “Aureo” wheat supply Chain. Sustainability 12, 5642. doi: 10.3390/su12145642
Sun, X., Yu, H., Solvang, W. D., Wang, Y., and Wang, K. (2022). The application of industry 4.0 technologies in sustainable logistics: a systematic literature review (2012–2020) to explore future research opportunities. Enviro. Sci. Pollut. Res. 29, 9560–9591. doi: 10.1007/S11356-021-17693-Y/TABLES/8
Tang, A. K. Y., Lai, K. H., and Cheng, T. C. E. (2016). A multi-research-method approach to studying environmental sustainability in retail operations. Int. J. Prod. Econ. 171, 394–404. doi: 10.1016/J.IJPE.2015.09.042
Tenenhaus, M., Vinzi, V. E., Chatelin, Y.-M., and Lauro, C. (2005). PLS path modeling. Comput. Stat. Data Ana.l 48, 159–205. doi: 10.1016/j.csda.2004.03.005
Tomšič, N., Bojnec, Š., and Simčič, B. (2015). Corporate sustainability and economic performance in small and medium sized enterprises. J. Clean. Prod. 108, 603–612. doi: 10.1016/j.jclepro.2015.08.106
Tsai, W.-H. (2018). Green production planning and control for the textile industry by using mathematical programming and industry 4.0 techniques. Energies. 11, 2072. doi: 10.3390/en11082072
Tsai, W.-H., and Lu, Y.-H. (2018). A framework of production planning and control with carbon tax under industry 4.0. Sustainability 10, 3221. doi: 10.3390/su10093221
United Nations (2015). Transforming our world: the 2030 Agenda for Sustainable Development Preamble—A/RES/70/1. Available online at: https://www.un.org/en/development/desa/population/migration/generalassembly/docs/globalcompact/A_RES_70_1_E.pdf (accessed August 3, 2022).
van Vuuren, D. P., Stehfest, E., Gernaat, D. E. H. J., Doelman, J. C., van den Berg, M., Harmsen, M., et al. (2017). Energy, land-use and greenhouse gas emissions trajectories under a green growth paradigm. Global Environ. Chang. 42, 237–250. doi: 10.1016/j.gloenvcha.2016.05.008
Vermeir, I., Weijters, B., de Houwer, J., Geuens, M., Slabbinck, H., Spruyt, A., et al. (2020). Environmentally sustainable food consumption: a review and research agenda from a goal-directed perspective. Front. Psychol. 11, 1603. doi: 10.3389/FPSYG.2020.01603/BIBTEX
Westerman, G., Tannou, M., Bonnet, D., Ferraris, P., McAfee, A., and McAfee, A. (2012). The Digital Advantage: How Digital Leaders Outperform their Peers in Every Industry. MIT Sloan Management and Capgemini Consulting.
Wu, L., Qing, C., and Jin, S. (2022). Environmental protection and sustainable development of enterprises in China: the moderating role of media attention. Front. Environ. Sci.10, 1781. doi: 10.3389/fenvs.2022.966479
Yolles, M. (2018). Sustainability development: part 2—exploring the dimensions of sustainability development. Int. J. Markets Bus. Syst. 3, 257–275. doi: 10.1504/IJMABS.2018.093310
Keywords: sustainable development, industrial revolution (IR), path models (PLS-PM), agri-food analysis, financial performance
Citation: Máté D, Oláh J, Erdei E, Estiyanti NM, Bács Z and Kovács S (2022) The impacts of sustainable industrial revolution (IR) on the profitability of Hungarian food companies. Front. Sustain. Food Syst. 6:1034010. doi: 10.3389/fsufs.2022.1034010
Received: 01 September 2022; Accepted: 04 October 2022;
Published: 20 October 2022.
Edited by:
Roberta Selvaggi, University of Catania, ItalyReviewed by:
Štefan Bojnec, University of Primorska, SloveniaConcetta Nazzaro, University of Sannio, Italy
Copyright © 2022 Máté, Oláh, Erdei, Estiyanti, Bács and Kovács. This is an open-access article distributed under the terms of the Creative Commons Attribution License (CC BY). The use, distribution or reproduction in other forums is permitted, provided the original author(s) and the copyright owner(s) are credited and that the original publication in this journal is cited, in accordance with accepted academic practice. No use, distribution or reproduction is permitted which does not comply with these terms.
*Correspondence: Judit Oláh, b2xhaC5qdWRpdEBlY29uLnVuaWRlYi5odQ==