- 1Social, Economic, and Policy Research Team (SEPRT), International Center for Agricultural Research in the Dry Areas (ICARDA), Ariana, Tunisia
- 2Higher School of Agriculture of Mograne, Zaghouan - LR-03AGR02, University of Cartage, Tunis, Tunisia
- 3Laboratory of Agronomy (LA), National Research Agronomic Institute (INRAT), Ariana, Tunisia
- 4Resilient Agro-silvopastoral Systems Program (RASP), International Center for Agricultural Research in the Dry Areas (ICARDA), Ariana, Tunisia
The aim of this paper is twofold. The first objective is to measure the technical efficiency of mixed crop-livestock (CL) smallholder producers operating under conservation agriculture systems in Tunisian rainfed areas. The second objective is to explore complementarities, synergies, and economies of diversification across the different production system components of these crop-livestock producers using the cross-partial derivative framework of output variables in the distance function. A simple random sampling process was employed to select and survey 59 CL smallholders operating under conservation agriculture. The collected data were analyzed using a stochastic input distance function in which synergies were estimated based on the second cross-partial derivative concept of output variables in the distance function. Results show that technical inefficiencies are significant in integrated crop-livestock systems, and there is evidence that economic diversification provides a productivity buffer against climate change threats. As a sustainable intensification strategy, this integrated system also offers a potential advantage. The results further contribute to the debate on crop diversification vs. specialization. Although an enhanced system integration could be a financially and ecologically viable option for mixed crop-livestock systems, more pathways for profitable and viable diversification of cereal-based or orchard-based systems remain to be explored.
Introduction
Intensive farming with limited soil amendments and conservation practices leads to soil erosion and nutrient depletion (IFAD, 2010). Specialization of agricultural systems and the search for economies of scale have guided the evolution of agriculture, with new farming “models” emerging to respond to a growing demand for food. At the same time, it has been suggested that system diversification and integration across production system components would increase household income, reduce vulnerability to shocks, create job opportunities, enhance land productivity, and improve water use efficiency (Moraine et al., 2014). The new paradigm of “sustainable production intensification,” as detailed by (FAO, 2010), recognizes “the need for a productive and remunerative agriculture which at the same time conserves and enhances natural resources and positively contributes to harnessing the environmental services” (Kassam A. et al., 2009). [sic]
Originally, the adoption of conservation agriculture (CA) was mainly driven by severe problems faced by farmers, especially water and wind erosion or drought. Government support has played a significant role in accelerating CA adoption in many countries, leading to relatively increased adoption rates, for example in Kazakhstan and China but also in African countries (Friedrich et al., 2012). The main advantages driving CA adoption can be summarized as follows: (i) better farm economy; (ii) flexible technical possibilities; (iii) yield increase and greater yield stability; (iv) soil protection against water and wind erosion; (v) improved soil health; (vi) better efficiency of nutrients; and (vii) better water economy in dryland areas (Kassam A. H. et al., 2009). Because of these advantages, CA has been widely supported by international donors and development organizations.
Conservation Agriculture (CA) based on direct seeding was initiated in Tunisia between the years 1970 and 1980 through the acquisition of no-till seed drills from the United States (Cheikh M'hamed et al., 2018). In 1999, an integrated agricultural and rural development project (Projet de Développement Rurale Agricole Intégré, PDRAI) was implemented in the Siliana region and was partly focused on some agricultural practices under CA (Baccouri, 2008). From 2001 to 2011, the French Facility for Global Environment (FFEM) funded an R&D project, the Projet d'appui au développement de l'agriculture de conservation (PADAC project), supported by a range of universities, institutions in the public and private sector and international organizations (INRAT, CTC, ESAK, CIRAD, Cotugrain, and more), and intended to contribute to key development challenges (i.e., food security, environmental sustainability, economic development) through the scaling of CA systems (Raunet et al., 2004). The project was aimed primarily at large-scale farmers (exceeding 100 ha) in the north of the country. Since 2006, interest has focused on small farms (<15 ha), with a project on CA for smallholders funded by the Arab Authority for Agricultural Investment and Development (AAAID) and implemented by CTC and ESAK. From 2012 to 2016, the CANA project “Rapid adoption of Conservation Agriculture in North Africa for Smallholders” was carried out in the Fernana region, funded by ACIAR, managed by ICARDA, and implemented by the INRAT and the INGC. In the framework of this project, a new local prototype of NT seed drills (low-cost no-till drills for smallholders) was designed and manufactured.
The selection of smallholder farms (i.e., 0–15 ha) for this study in this farming system was based on the following: the first criterion was the biophysical context and technical characteristics of this cereal-based rainfed agriculture system under semiarid and with low soil fertility. The size of 0–15 ha was considered to be critical for classifying a farm as small. In other words, it was mainly the low output level of being in an arid rainfed farming system that led us to adopt this classification. Indeed, a maximum of 15 ha of cropping area represents the level under which the resilience of this production system is weak, as well as its economic viability (secure livelihood for the farmers' household). The second criterion was based on national literature findings regarding the farms' categorization in Tunisia. According to Bachta (2011), the number of Tunisian farmers who produce cereals amounts to 38,400. The majority of small farmers works on an area of fewer than 20 hectares. In a second study undertaken by the Tunisian Ministry of Agriculture (cited in Khaldi and Saaidia, 2017) considering 47,700 sampling farms, the number of cereal farmers was estimated at 248,458. About 63% of them (157,000) were considered smallholders, working on areas of 10 hectares or less (MARH, 2006). For the last criterion, we considered the project frame given that, for many concerns on spreading CA technologies, we selected this range (i.e., 0–15 ha) as small-size farms to enhance the adoption of these packages, and the project was included in this challenging (climate change, low soil quality, reduction of rainfall, drought, etc.) farming system.
To resolve the conflict between permanent soil cover with residues and stubble grazing, which is considered the major obstacle to CA adoption in the country, the CLCA project (Integrated Crop-Livestock under Conservation Agriculture for Sustainable Intensification of Cereal-based Systems in North Africa and Central Asia), funded by the International Fund for Agricultural Development (IFAD) and managed by ICRADA, was implemented by INRAT and INGC (2013–2016). As the main result of the project, a stubble grazing model of 30:30 was developed (Guesmi et al., 2019) based on the stocking rate of 30 animals ha−1, during a 30-day stubble grazing period. In 2018, the second phase of the CLCA project, “Use of Conservation Agriculture in Crop Livestock Systems (CLCA) in the Drylands for Enhanced Water Efficiency, Soil Fertility and Productivity in NEN and LAC Countries” was launched and aimed to up-scale CLCA technologies in the semiarid regions of the northern part of the country (Rekik et al., 2021).
Recently, three research projects on CA were funded by the EU through the consortium Partnership for Research and Innovation in the Mediterranean Area (PRIMA) program for 4 years (2020–2023), with Tunisia as a partner in all three projects. These projects implemented in Tunisia by INRAT are: (i) the ConServeTerra project “Overcoming the physical and mental barriers for up-scaling Conservation Agriculture in the Mediterranean” (ii) the 4CE-MED project “Camelina: A Cash Cover Crop Enhancing water and soil conservation in Mediterranean dry-farming systems,” and (iii) the CAMA project “Research-based participatory approaches for adopting Conservation Agriculture in the Mediterranean Area”.
The CA area in Tunisia increased rapidly during the first years of adoption. Indeed, the CA area was only 27 ha and 55 ha in 1999 and 2000, respectively. The CA area reached 2,893 ha in 2005, 6,000 ha in 2007, 8,000 ha in 2008, and then 12,000 ha in 2010 (Richard, 2005; Angar, 2010). However, in 2016, the areas recorded a significant decrease (Bahri et al., 2018). This decrease was mainly explained by the unavailability of low-cost direct seed drills and the lack of comprehensive CA adoption studies. The CA area in Tunisia stands at around 14,000 ha and is operated by more than 200 farmers using around 107 NT seed drills (Cheikh M'hamed et al., 2018).
Most of the areas under CA in Tunisia are found in semiarid regions, marked by hot summers, cold winters, and low annual rainfall. The average annual rainfall in these regions is between 200 and 450 millimeters per year. The rainfall in these regions is also characterized by a high degree of inter annual variability and intra annual variability during growing season (Cheikh M'hamed et al., 2022). Indeed, a very limited number of rainy days (fewer than 120 days per year in general) and frequent droughts during the growing season combined with high temperatures are common constraints to plant growth, especially for cereal crops, which are strategic crops for the country.
In addition to water scarcity, soil degradation is the main challenge faced by agriculture production systems in semiarid regions of Tunisia. Indeed, it is reported that >3 million hectares (60 % of total cropland in Tunisia) are being eroded or are at a high-water erosion risk (DGACTA., 2017). The effects of water erosion are expected to be exacerbated by CC. Autumn rains contribute to erosion due to the summer overgrazing of crop residues and the absence of vegetation covering the soil. Furthermore, arable soil in Tunisia is increasingly degraded due to inadequately promoted agricultural practices, especially the dominance of conventional production systems based on intensive tillage. These practices have led to land degradation and the depletion of soil fertility and soil water-storage capability (Cheikh M'hamed et al., 2022).
Production systems in these regions are primarily based on field crops, particularly cereals (wheat, barley, and oats) combined with ruminant livestock. In addition, in these regions, CA farmers usually practice cereals crops integrated with livestock activities. Livestock is the backbone of these mixed farming systems because it serves as a means of reducing the risks associated with crop failure, contributes to food security, and considered as an income diversification strategy for resource-poor small-scale farmers (Cheikh M'hamed et al., 2022; Mrabet et al., 2022). Also, livestock is considered by smallholder farmers as a primary asset that can be easily converted into cash during dry years. This is characteristically interconnected with cropping systems through weedy fallows, residue, stubble grazing, and the use of woodlands and rangelands. Because stubble grazing is usually practiced by farmers, they face major challenges in terms of permanent land cover. Indeed, stubble grazing by livestock, especially during the summer, is a traditional and common practice in the region. As a result, under the CA system, maintaining crop residue on field creates a conflict of interest between mulching the soil surface and stubble grazing, especially during the summer (Tittonell et al., 2015). Thereby, trade-offs between the use of stubbles for livestock feeding or to cover the soil must be resolved, particularly in drylands where fodder potential is low. For better crop-livestock integration under CA, it is necessary to combine diversified crop rotation with controlled and improved managed grazing. This can be effective for preserving or even enhancing soil function and health (Mrabet et al., 2022).
In semiarid regions, low rainfall limits crop production. Adoption of CA based on its principles can be effective in mitigating yield loss in dry environments (Cheikh M'hamed et al., 2014; Mrabet et al., 2022). Results from research in Tunisia showed that CA can increase yields after a few years of adoption and make crops more resilient to changing climatic conditions (Cheikh M'hamed et al., 2016; Bahri et al., 2019). CA can reduce drought effects through better water storage and availability during the crop-growing season in wheat-based systems (Mrabet et al., 2022). Indeed, CA allows for improving soil infiltration, thus reducing surface runoff and soil erosion, as well as allowing for a greater soil moisture-holding capacity. This is due mainly to the presence of stubble on the soil surface as mulch, which can increase water infiltration and slow moisture losses through evaporation. Furthermore, the adoption of CA gives more flexibility to farmers for the implementation of field crop management that allows timely planting and input application, despite unfavorable field conditions that prevent such operations in conventional agriculture (Mrabet et al., 2022).
The concept of agricultural diversification is key for the implementation of CA. It states the shift from the dominance of monocrops to the production of diversified crops with varied species (including legumes, forages, and cereals) on a farm or in a region (Petit and Barghouti, 1992). Joshi et al. (2004) defined agricultural diversification as connoting crop mix, enterprise mix, and activity mix at a household level aimed at increasing household profit and wealth.
According to Ryan and Spencer (2001) and Joshi et al. (2007, 2006), the determinants of system diversification can largely be classified into two categories: demand and supply. On the demand side, this classification specifically takes into consideration three criteria: per capita income, population growth, and urbanization. These factors are different from those on the supply side, and some of them are farm-level and include household-level factors, biophysical factors, and risk factors. Others are linked to infrastructure or institutions, technology, and resource endowment factors. Household diversification, on the other hand, could be influenced primarily by key socio-economic factors such as age, gender, education, household dependency ratio, crop and livestock capital, and off-farm income resource activities (Asante et al., 2020).
Sichoongwe et al. (2014) concluded that relevant farm-level drivers for crop diversification include the total cultivated land area, the total output value, the types of cultivated crops, and the total input value (external and family labor, fertilizers, and technologies). In addition, diversification is also influenced by other external factors such as farmers' access to markets (proximity and distance, market information), access to extension services and information, access to credit, and networking (e.g., social networks, membership in farmers' associations) (Joshi et al., 2007; Kankwamba et al., 2012). A significant relationship between farm size and diversification was found by Pope and Prescott (1980) when they examined the determinants of farm diversification. Chavas and Aliber (1993) observed a diminishing effect of economies of scale on farm size among farmers in Wisconsin, USA. Weiss and Briglauer (2000) reported that smallholders tend to increase the degree of specialization more rapidly over time than larger farms. A significantly lower degree of diversification (i.e., a higher degree of specialization), as well as a stronger reduction in diversification over time, is also reported for businesses operated by older, less educated, part-time farm operators (Weiss and Briglauer, 2000).
Asante et al. (2018) outlined that in several empirical research studies, the estimation of diversification has been considered a joint decision-making process. Along this process, these studies employ limited dependent-variable models (e.g., the logit, probit, and tobit models and their extensions). The question of explaining the role of diversification or specialization in economic growth and development has been widely explored in economic literature using several techniques. Among them, we can enumerate the following: the index of maximum proportion, the Herfindahl index (HI), the Simpson index, the Ogive index, the Entropy index, and its associated modifications (Ibrahim et al., 2009; Ogundari, 2013). The literature, however, differentiates between the decision to diversify and the decision on how diversification occurs. There is still a need to examine whether both decisions are combined or dispersed.
In Tunisia, small mixed-farming systems hold 75–85% of agricultural land and provide more than 80% of some annual and perennial crops and livestock products (Marzin et al., 2017). Despite the important role of crop-livestock farming systems under CA in reducing the poverty of smallholders and enhancing their food security at the different levels (local, regional, and national) in the dry farming systems, the complementarities between both system components have not been appropriately explored. To our knowledge, no study has examined the impact of crop-livestock diversification under CA farming systems. Therefore, the objectives of this study are to evaluate whether a crop-livestock integrated system under CA is a complementary or rival component of mixed farming systems. This paper contributes to the existing literature on the importance of diversification and what driving factors influence diversification in livestock and integrated crop-livestock production systems under these agroecological farming systems among smallholders in Tunisian semiarid areas. Findings are expected to provide information on the determinants of diversification and whether the system components to be integrated have actual economic synergies and complementarities.
The methodology of the paper is based on a model issued from the stochastic input distance function (Villano et al., 2010; Asante et al., 2020), which explores evidence of economies of diversification and their effect on determining diversification decisions of smallholders in integrated crop-livestock systems. Our hypothesis is based on the fact that “economies of diversification are significant in determining diversification decisions of smallholders in integrated crop–livestock systems under CA”.[sic] We also expect that “economies of scale exist in these systems”, which suggests that there are opportunities to expand crop-livestock outputs without employing additional inputs or improved production technologies. Such economies of diversification are expected to be significant among the potential existing output combinations (cereals and forage crops with other crops and other crops with livestock) in integrated crop-livestock systems under CA farming.
Reasons for crop-livestock integration and diversification under CA farming systems
Complementary relationships between CL farming activities have been documented in many studies (Tilman et al., 1996; Loreau, 1998; Yachi and Loreau, 2007). In fact, the synergy degrees may vary spatially and temporally, even within the same agroecological system. Tarawali et al. (2004) suggested that the diversification of farming systems secures synergies between crop and livestock production, improves productivity, and ensures the resilience of integrated agricultural production systems. Moreover, it has been argued that these mixed-farming systems allow smallholders to expand their sources of foods and staples in household diets (diet diversification) and empower them to actively access local markets for high-value products (FAO, 2010).
Martin et al. (2016) identified the different forms of CL integration, summarized as follows: global coexistence is the transfer of raw materials (e.g., forage and organic manure) among farms through the national or global market; this first form of integration does not include direct coordination between farmers since it is based on a spatial and temporal partition of crop and livestock production; local coexistence happens when a local organization (agricultural cooperative) ensures the coordination among farmers by transferring the raw materials; complementarity is the direct and frequent coordination between farmers, allowing an exchange of raw materials between farms.
Guesmi et al. (2019) claimed that adopting integrated crop-livestock systems under CA is beneficial for two reasons: (i) mulch left on the soil surface can be used as animal fodder; and (ii) permanent vegetative cover with a high level of nutrients improves animal performance. However, competition between crops and livestock may occur. According to Guesmi et al. (2019), in a region where livestock production is the main activity, crop-livestock integration seems inappropriate for CA adoption, although the CL combination offers farmers a more diverse source of food and income-generating options. Adopting a CA system that requires leaving mulch on the soil surface can reduce the quantity of feed for livestock. Some suggestions for successful CL integration refer to either introducing crops with higher biomass production or adapting herd size to forage production capacity or developing alternative feeding options (Ameur et al., 2021). In the literature, much has been written and published on the trade-offs that smallholder farmers face when having to allocate their biomass resources among competing objectives such as feed for animals or mulch (Klapwijk et al., 2014). In natural resource-limited systems (e.g., dry land farming systems) and environments, farmers prioritize the livestock feeding option over soil amendment to improve soil quality (Tittonell et al., 2015). This could be explained by the limited availability of fodder for livestock in these systems which, therefore, often results in constant rivalry for the use of the limited crop residues.
Masmoudi (2012) estimated the effect of crop-livestock integration under direct seeding. Their work shows that grazing integration within the practice of direct seeding is possible considering light and moderate grazing intensity, as long as vegetation cover remains greater than 78% before grazing. Along these lines, Byrnes et al. (2018) argued that grazing management, under no-till, can significantly influence soil quality and health; however, a controlled grazing strategy during the dry season will be a central component of the livestock feed biomass strategy under this mixed farming system. Moujahed et al. (2015) assessed the effect of stocking rate on the variation of stubble biomass and lamb growth. Their results show that the stocking rate decreased in some plots due to animal preferences while grazing. The variation in the chemical composition of stubble is thus suggested to be related to the selective grazing behavior of lambs.
Landers (2007) enumerated the benefits of crop-livestock integration under CA based on no-tillage. The simultaneous implementation of CA principles such as crop diversification allows enhanced forage production for livestock, which is also a source of organic matter and crop fertilization. Other benefits of integrated crop-livestock systems under CA based on no-tillage follow: (i) increased profit through reduced production costs; (ii) reduced disease, pest, and weed pressures on crops; and (iii) maintaining a high stocking rate on rotated pastures (Landers, 2007). The author claimed that the benefit of crop-livestock integration under CA is higher compared to the benefits of crop and livestock systems conducted separately. Landers (2007) also focused on the technical and financial aspects of the integrated crop-livestock system under CA by comparing case studies with and without CA interventions in the Brazilian context. Results showed that adopting integrated crop-livestock systems under CA based on no-tillage improve the situation of winter pastures for cattle, thus leading to a higher income. Other effects of adopting integrated crop-livestock systems under CA are as follows: (i) improved herd performance; (ii) enhanced pasture stocking levels (from 1 to 1.76 AU/ha); (iii) a 10% increase in soybean and maize yields; and (iv) 63% more annual net profit. The adoption of integrated crop-livestock systems under CA also led to a remarkable variation in deforestation, which has been estimated through the proportions of the crop to pasture and variations in cumulative stocking rates. Results of the financial analysis of several case studies indicated a remarkable financial benefit in terms of internal rate of return and net present value. Other similar studies showed that CA-based systems in Zambia generated an increase of up to 33% in grain yields and consequently a greater net benefit (Komarek et al., 2019).
Agriculture diversification includes different stages (Chaplin, 2000; Vyas, 2006). As quoted by Chaplin (2000), “the process of diversification of agriculture may pass through four stages: i) At the first stage the cropping system shifts from monoculture to multiple cropping. This phenomenon generally occurs in the developing countries and most of the third world countries are under this category; ii) At the second stage the farmers start more than one enterprise. For example, crops and animal husbandry, beside the number of crops in a year is more than one; iii) Thirdly, initiation of mixed farming. In the last stage of diversification, the activities which are incorporated are beyond the agricultural domain such as adding the value through the processing, packaging and producing by products”. [sic]
CL diversification refers to the combination of the production of one or more crops and livestock with the available resources (Komarek et al., 2019). Diversification is determined by multiple factors, including per capita income, the number of crops cultivated, livestock structure, and the total value of outputs. Through an assessment of diversification indices, Komarek et al. (2019) argued that the CL diversification index is greater than the separate indices for crop diversification and livestock diversification. Results also revealed that income stability, access to extension services, and market information contribute significantly to increasing crop-livestock diversification and allowing farmers to meet market requirements and increase their profitability from farming (Mesfin et al., 2011). By analyzing the status quo of the crop-livestock farming system practiced in Swaziland, Mhazo et al. (2010) admitted that adopting an integrated crop-livestock farming system is part of a context of food security insurance and increasing productivity. Liniger et al. (2011) confirmed that an integrated CL farming system contributes to productivity enhancement and water use efficiency improvement. Their findings revealed that the adoption of a mixed-farming system generates a 50% improvement in productivity and farm income in Ethiopia.
Conceptual framework and modeling approach
Analytical framework—Theoretical input distance function model
Economic diversification is an essential aspect of sustainable development in dryland regions, which are one of the most sensitive areas to climate change and human activities, as diversification enhances production stability and promotes structural and long-term transformations toward more adaptive farming systems in these regions. According to Villano et al. (2010), these aspects have been investigated using two main approaches: econometric methods and accounting-based profitability measures. The first approach focuses mainly on using the distance function. However, the second approach, the accounting-based profitability approach, has been predominantly employed in investigating economies of diversification between firms and is not appropriate for examining complementarities between and within farm activities (e.g., cereals, legumes, fodder crops, and livestock). Economies of diversification appear when the diversion of the farming system leads to a decrease in costs associated with several outputs produced simultaneously with a set of input combinations (Baumol, 1982). In such a case, smallholder farmers decide to diversify because they expect that synergies obtained from these enterprises could contribute to enhancing their economies of diversification and reduce production risks given the CC threats (Chavas and Aliber, 1993; Paul and Nehring, 2005; Villano et al., 2010). Similarly, there is considerable evidence of the productivity impacts of diversification and climate change linkages as diversification contributes to protecting and improving agricultural and livestock production. This would reinforce and sustain farming productivity and consequently stabilize the volatility of food prices (Coelli and Fleming, 2004; Rahman, 2009; Tibesigwa et al., 2015; Abdulai and Abdulai, 2017). Several studies have explored the economies of diversification in smallholder farming systems and the degree of complementarities between inputs and outputs in the economic performance of diversified farm households (Pope and Prescott, 1980; Weiss and Briglauer, 2000; Coelli and Fleming, 2004; Chavas and Kim, 2007, 2010). The limitation of these empirical studies is that only agricultural diversification in terms of crop production has been examined. The studies dealing with the analysis of livestock diversification or integrated crop-livestock diversification are limited, especially under CA framing systems. Following this premise, it is important to assess crop-livestock integration and diversification and the driving forces contributing to strengthening this integration and diversification and, thus, increasing livestock-based production to meet the demands of the rural communities and consequently the growing Tunisian population. In this study, we applied the distance function approach (Coelli and Fleming, 2004; Villano et al., 2010) to assess diversification economies in mixed crop-livestock production systems operating under CA.
This distance function approach has been frequently used to assess economies of diversification in agricultural farming systems (Chavas and Aliber, 1993; Paul and Nehring, 2005; Rahman, 2009). The distance function approach was first proposed by Shephard (1953). The concept of this function is used to describe and model multiple outputs and inputs in the same production technology framework (Villano et al., 2010). A distance function is characterized by its double possible orientation—output or input orientation (Villano et al., 2010). When an output distance function is considered, a maximal level of outputs for a fixed level of inputs is considered. Whereas a fixed level of outputs for a minimal level of inputs is considered if we select an input distance function, the concept of the theoretical model planned to be used and named as an input distance function is illustrated by considering using a vector of input combinations X to produce a vector combination of outputs Y.
An input distance function is a function of the inputs (X) that produce outputs (Y). An input distance function includes the scaling of an input vector and is defined on the input set, L(Y), as follows:
where
• L(Y) is defined as the set of all input vectors, X, which can produce output vector Y.
• D(X, Y) is a distance function which assumed to be non-decreasing, positively linearly homogeneous and concave in X, and increasing in Y (Coelli and Perelman, 2000).
• D(X, Y) ≥ 1 if the input vector X belongs to the feasible input set L(Y).
• ρ is the scalar “distance” by which the output vector can be deflated.
Empirical model
Model specification
Given that complementarities between crops and livestock (i.e., sheep) occur when additional output (i.e., forages) is generated jointly with other outputs as a combined production system rather than operating the enterprise elements as separate systems, and that inputs are generally fixed by smallholder farmers in dryland areas in general, and in Tunisia in particular, we focused the empirical analysis in this study on the input orientation production technology (Coelli and Perelman, 2000; Coelli and Fleming, 2004; Villano et al., 2010; Asante et al., 2020). Applying an input orientation function allows a description of the specification of the production function, an estimation of technical efficiency, and an assessment of the complementary synergies and/or competition between the generated outputs (both crops and livestock). However, this complementary function does not guarantee the existence of economies of scope. According to Chavas and Kim (2007, 2010), the complementarities conditions are not generally necessary nor sufficient for economies of scope.
To measure complementary synergies and/or competition, derivates from second-order cross-partial analysis of the output variables in an input distance function are used in the empirical analysis. This is suggested by the fact that there are already several production possibilities generated from the nature of this frontier function (i.e., the curvature of the input distance function). This will allow the estimation of the first order and cross-output elasticities. A parametric method is applied using the translog functional form:
where
• In the four considered inputs, D (Xi, Yi) is the input distance function assumed to be non-decreasing, concave, and positively linearly homogeneous;
• X1i, X2i, X3i, and X4i are the inputs used in this function, which are the values of labor (expressed in person-days per year), land (in hectares), crop capital (in value), and livestock capital (in value), respectively;
• Y1i, Y2i, Y3i, and Y4i are the outputs used in this function, which represent the values in Tunisian dinars (TND) of the four outputs of cereals (e.g., wheat), forage crops, legumes, and livestock, respectively;
• i is the ith sample farmer used in the agricultural production function.
Paul and Nehring (2005) argue that the choice of orientation (output or input) depends on two factors: the purpose of the undertaken study and the fixity levels for both outputs and inputs. Within the same theme, we considered the following hypothesis based on the methodological framework of Paul and Nehring (2005):
• –ln D(X, Y) = υ – u;
• Input restrictions must be homogeneous to the degree of +1.
• Consider the labor (X1i) factor (input) to normalize the input vectors;
• All restrictions required for symmetry and homogeneity conditions are:
∘ αij = αji i, j = 1, 2, …, n (n = 4)
∘ βij = βji i, j =1, 2, …, m (m = 4)
The established conditions above led to the empirical model for estimation, specified as follows:
where
• υi by hypothesis is supposed to be an independently and identically distributed normal random error with a mean of zero and a variance of ;
• ui is a nonnegative technical inefficiency effect that is expected to be independently distributed and have a truncated-normal distribution;
• ui is defined following Battese and Coelli (1995) where the truncation (at zero) of the normal distribution with a mean of ui, and a variance of , specified as follows:
where
• Z1i denotes the ith farmer's age, in years;
• Z2i is an education dummy variable that is 1 if the ith farmer has completed at least six years of schooling, and 0 (zero) otherwise;
• Z3i is defined as the dependency ratio value for the ith farmer's household (it is calculated as the number of dependent members divided by the total household size);
• Z4i is defined as the percentage of off-farm income relative to total farm income (the total farm income includes the value of outputs for the ith farmer);
• Z5i is a credit dummy variable for the ith farmer (this value is 1 if the ith farmer obtained credit, and 0 otherwise);
• Z6i is as an extension dummy variable for the ith farmer (this value is 1 if the ith farmer received extension services support or advice, and 0 otherwise);
• Z7i is defined as the HI value of mixed crop-livestock diversification for the ith farmer;
• δ is considered an unknown parameter. The coefficients of these parameters will be estimated to explain the inefficiencies of production of the farm output activities (e.g., cereals, legumes, forage crops, and livestock);
• i: 1, …, N (number of farmers considered in the empirical analysis).
The two models in Equations (4) and (5) are simultaneously estimated using Stata econometric software version 14.
Qualitative assessment of crop-livestock activity diversification: HI
To assess the degree of specialization for the sample of CA smallholder adopters, we use HI defined as follows:
where
• n is the number of farming products (e.g., field crops, cereals, and livestock);
• Sj is defined as the jth farm product share value in the total output of the considered farm;
• In the empirical analysis, it is considered that HI is defined by its minimum value which is 1/n. This suggests that minimizing HI subject to the sum of the shares is to be one. Thus, given Sj = 1/n, for all j = 1, 2, …, n, this indicates a full diversification, with all the farm outputs having the same share (1/n);
The HI ratio ranges from zero to one. The value of one indicates complete specialization (i.e., only one activity). Under the hypothesis of having a high level of diversification, the value of the HI is likely to be small. Based on this hypothesis, the sample farmers were categorized into three groups, namely, diversified, highly specialized, and moderately specialized, based on the value of HI. Thus, if HI ≤ 0.5, this suggests a diversification between crop and livestock products. When the HI of a smallholder is > 0.8 they are considered highly specialized, and moderately specialized if 0.8 > HI > 0.5.
Qualitative evaluation of complementary synergies and/or substitute rivalries in crop-livestock under CA farming systems
From a theoretical perspective, we expect a negative sign for the first derivative of the distance function displayed in Equation 4 for all outputs considered in the empirical analysis. This suggests that producing an additional unit of output while maintaining all the rest of the other variables unchanged will affect the input needs that will be reduced to ensure the efficiency of the production function (Coelli and Fleming, 2004; Rahman, 2009). However, a positive sign is expected in the second derivative of the distance function for all four outputs, suggesting evidence of complementary synergies (Villano et al., 2010). Economic diversification for farmers operating under CA is inextricably linked with the structural transformation of their economies and the achievement of higher levels of productivity resulting from the movement of economic resources within and between selected outputs j and k (j ≠ k; j, k = 1, 2, 3, 4). It exists if the following equation is validated:
Following Villano et al. (2010) and Asante et al. (2020), the concept of complementary synergies has been used in this paper. This suggests that all derivatives (i.e., second order and cross-partial) do not automatically indicate the existence of an economic gain for the smallholder farmer. A positive value indicates the presence of diversification economies, but it is a necessary and not sufficient condition. On the contrary, diversification diseconomies are confirmed by a negative value under a necessary and sufficient condition outlining a high level of competition between farm activities (i.e., livestock and crops). In the empirical application, we considered the following rule: the second partial derivative concerning the logarithms of two involved outputs is equal to the corresponding coefficient of the interaction between these defined outputs.
In this case, we estimated the following values and examined to what extent these values are statistically significant (i.e., testing if they are different from zero) for the considered outputs:
Where:
βjk is defined as a cross-product coefficient.
If the sign of this coefficient is positive, the two outputs show complementary synergies in their production process (Asante et al., 2020).
If the sign is negative, the two outputs are considered substitutes.
The equation displayed in Equation 7 illustrates that the coefficient issued from the cross-partial derivative of this equation is equal to the coefficient of Equation 8 multiplied by the reciprocals of the values of the two involved outputs, Yj and Yk. From the stochastic input distance function, we used the standard errors of the βjk coefficients. This was used to test the null hypothesis (no synergies) against an alternative hypothesis of synergies, taking into consideration the assumption of input homotheticity, which requires the input isoquant to expand or contract radially in the input distance function (i.e., production function). In the empirical model, we expect to have six output combinations associated with the four activities used to assess the complementary synergies or substitution (e.g., specialization) between these activities.
Data sources and specification of the model variables
The data used in this study have been collected from smallholder crop-livestock farmers operating under CA systems who were part of the CL integration under the CA project1 funded by the International Fund for Agricultural Development (IFAD) under agreement number #200116. These farmers were selected randomly from those who benefited from the project programs and innovation packages aimed at crop-livestock integration under CA. The farms in this study are rainfed and located in the arid areas of four governorates in the north of Tunisia: Zaghouan, Beja, Siliana, and Kef. From a total of 100 farmers (who had interventions from the CLCA project), we retained only 59 farmers for two reasons: (i) farmers who had interventions from the project; and (ii) full data completed with these farmers. The data collection process was conducted during the last quarter of 2021, with the country still under lockdown due to COVID-19. The farmers sought to improve their farming systems by enhancing their farming practices through the adoption of a CA component or package (e.g., no-till, residual biomass, forage mixtures, and crop rotation). The data were obtained by using structured questionnaires with pre-identified smallholders. The collected data include socio-demographic and economic information such as technical information on both crop and livestock activities, types of crops and livestock produced, the value of production for both activities, and other household characteristics. Table 1 presents summary statistics (means) for the variables used in the empirical model.
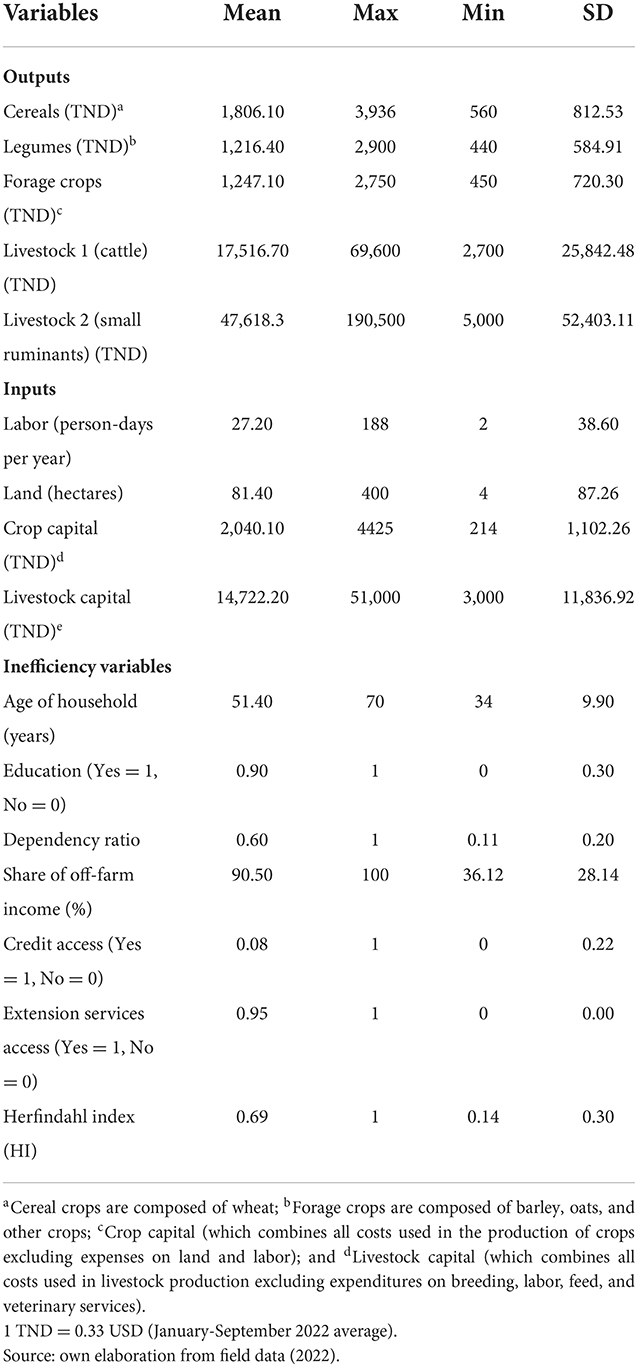
Table 1. Characteristics of sample households–socio-demographic variables used in the empirical model.
The statistical analysis shows that the major crops cultivated by farmers are cereals (especially wheat), legumes, and forages (barley, oats, and other forage crops), with their contributions to the total crop value representing 42, 28.5, and 29%, respectively (Table 1). Major livestock includes small ruminants (73%) and cattle (27%). The average value of cereals (e.g., wheat) produced is 1,806.1 TND/year, and legumes and forage crops have an average of 1,216.4 and 1,247.1 TND/year, respectively. The mean value of small ruminant output is 47,618.3 TND/year. On average, cattle output is 17,516.7 TND/year. Over the course of one cropping season, an average of 27.2 person-days is used for crop-livestock production. The average capital used in crop activities is 2,040.1 TND/year. The average amount of capital used for livestock production is 14,722.2 TND, representing 88% of the total farm costs.
Empirical results and discussion
In our empirical model specification in Equation 4, labor has been used to normalize input X1 so that all other inputs are represented relative to it. Following Singbo et al. (2021), all variables (both inputs and outputs) have been mean-corrected before estimation, where each output and input variable has been divided by its geometric mean. This suggests that the coefficients of the first order terms can be directly interpreted as distance elasticities evaluated at the geometric mean of the data.
Degree of enterprise diversification and/or specialization in integrated crop-livestock systems under CA farming systems
The findings from the HI analysis are shown in Figure 1, which presents farmer distribution according to the degree of enterprise specialization.
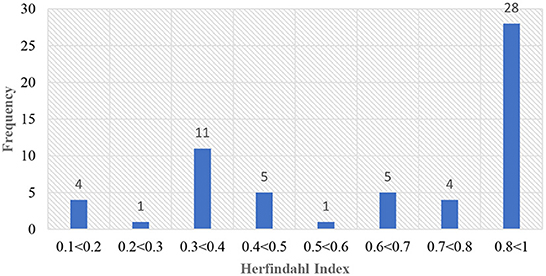
Figure 1. Degree of diversification of crop-livestock activities in integrated crop-livestock under the CA farming system (HI, Herfindahl index). The following classification is used: if HI ≤ 0.5, this suggests diversified crop-livestock smallholders; HI >0.8 indicates highly specialized smallholders, and HI of 0.5–0.8 indicates moderately specialized smallholders. Source: Own elaboration from field data (2022).
A high percentage of the smallholders (37%) are indeed diversified (HI ≤ 0.5) (Figure 1). Around 15% of farmers have a moderate level of specialization (HI of 0.5–0.8) (nine smallholders). Highly specialized smallholders under CA farming systems accounted for around 48% (28 smallholders). In general, there are two major types of farms: 37% are diversified, and 48% are rather specialized. These findings suggest a further examination into the extent to which economies of diversification may be a strategy for increasing farm productivity which has a direct impact on the food security of smallholders in semiarid areas.
Estimated model and empirical results
Given that all variables considered in the analysis (input and output) are estimated in log values and normalized by their respective sample means, the parameters from the input distance function have been interpreted as direct elasticities at the sample mean. The first order estimates of coefficients for these inputs and outputs are presented in Table 2. The sign and magnitude of these coefficients (e.g., input and output elasticities) are expected, and most are statistically significant.
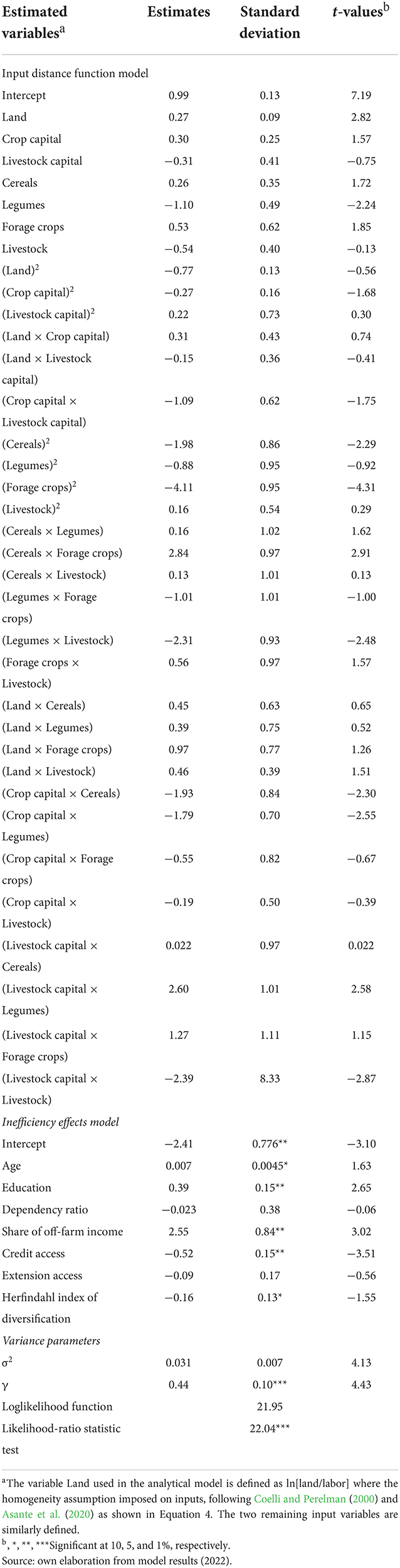
Table 2. Empirical coefficients of the stochastic input distance function (including inefficiency coefficients).
Two input variable coefficients are positive. They are also significant at a 5% level. The highest input elasticity is for labor (0.74) followed by crop capital (0.30), land (0.27), and livestock capital (−0.31). The elasticities of input variables are interpreted as the percentage change in an input variable that is required to support a 1% change in the output variables. For example, an increase of 0.74% in labor is required to generate a 1% increase in all outputs, and a 0.27% increase in land is the minimum required for a 1% increase in all outputs.
In terms of outputs, there are positive and negative estimated coefficients. Given the high importance of forage crops among the farm activities in this CL farming system, forage crop output has the highest significant elasticity of 0.53, followed by cereals (e.g., wheat) with a significant elasticity of 0.26. That is, an increase of 1% in the forage crop output level suggests an increase of 0.53% in all inputs to sustain it. The same trend is evident for wheat, with a 1% increment in this crop requiring an increment of 0.26% in all inputs. These results indicate that the production of forage crops and wheat, on top of legumes and livestock, plays a significant role in integrated CL production under CA farming systems in Tunisian dryland areas.
The estimated coefficients of legume and livestock outputs are negative (−1.1 and −0.54, respectively).
This means that a decrease in labor use led to reducing the production levels of those two outputs (legumes and livestock).
Given that output elasticity (i.e., the elasticity of scale) measures the change in percentage change of output driven by a percent change in the use of all inputs, the total value of all output elasticities, −0.85, is thus considered the “valued elasticity of scale”. The value of this elasticity, in our case, is less than a unit and negative, indicating a strong decreasing return to scale (the efficiency of operation would increase if we increased the land size in our sample). This result seems to corroborate the evidence of economies of scale in integrated CL farming systems in the Tunisian rainfed context, suggesting that opportunities exist to expand crop-livestock outputs with better management of production inputs or improved CA technologies.
Technical inefficiencies in crop-livestock production under the CA farming system
Factors affecting technical inefficiency scores in CL farming systems are displayed in Table 2. Most of the coefficients of these factors are significantly correlated with the technical inefficiency of CL farms. The calculated value of the γ parameter (the technical inefficiency effect), a considerable element of the total variability of crop-livestock production under CA, is positive (0.44) and significant at P < 0.01.
However, before we proceed to discuss the parameters of the z-variables in the inefficiency models, we tested the null hypothesis that these coefficients are all zero and do not contribute to the explanation of the distribution of the inefficiency effects. The calculated test statistic value for this joint significance is 22.04 and it is significant at P < 0.05. The test statistic ratio is about 20.28 for an upper 0.5% point for the χ2 distribution with seven degrees of freedom. This suggests the non-acceptance of the null hypothesis that the coefficients of the z-variables in the inefficiency model are all zero. In this case, we retained the alternative hypothesis that all considered variables will contribute, at different weights, to explaining the inefficiency effects distribution.
The positive significant coefficients of age, education, and share of off-farm income variables are significant at 10, 5, and 1%, respectively. These results suggest that higher farmer age, education level, and share of off-farm income led to higher technical efficiency in CL production under CA (Latruffe et al., 2004; Hadley, 2006; Theodoridis et al., 2014). They provide evidence that age and education are potential factors in efficiency (Karimov, 2014). Hadley (2006) revealed that younger farmers can be more inclined to adopt innovative input-saving technologies. In contrast, older farmers are more efficient in managing farming risks since they can rely on longer practical experience in addressing inefficiency-related risks. A positive correlation also exists between off-farm income and technical efficiency. This suggests that the higher the off-farm income is, the more likely the farm will be technically efficient. This can be explained by the fact that farmers with higher off-farm income have greater investment capacity in terms of mechanization, better and more timely access to necessary farming inputs, and so on (see Frelat et al., 2016). These farmers do have a “buffer capacity”, which allows them to cope with shocks in more effective ways. It is important to note here that farmers' coping capacities are not only determined by their financial situation, and that more social and organizational factors are crucial in this regard. The significant negative coefficient of HI indicates that greater specialization (i.e., lower diversification) is more likely to be associated with higher scores of technical inefficiencies in CL integration under CA. This explains that diversification may lead to tangible benefits by increasing income through the adoption of sustainable intensification and diversification strategies.
The non-significant negative impact of the dependency ratio on technical efficiency reveals that households with a high dependency ratio are more likely to be less efficient. This could be because the number of dependent family members (economically inactive) increases and the household must allocate more financial resources for their basic needs (food, health, education, etc.) and other expenses. A high dependency ratio causes less productivity for labor, which results in a high consumption expense level and less output production. The consequence is that fewer resources might remain for farming (use of good inputs for cropping, livestock management, feeding, etc.). This fact is materialized at the household level, where families cannot afford to use improved agricultural technologies such as fertilizers and improved seed varieties (Asefa, 2011).
The negative effect of access to credit on technical efficiency suggests that farmers with lower access to this service are technically less efficient. This confirms the importance of access to credit services for crop-livestock smallholders operating under CA. Credit can help in acquiring inputs (e.g., machinery and forage seed) and labor at less cost and shorter time (e.g., improving farmers' productivity) and increasing technical efficiency. Regarding access to extension services, although the coefficient is negative, it is not significant. Therefore, the literature confirms the essential role of extension services in technology transfer to smallholders and knowledge sharing and how this service increases technical efficiency (Asante et al., 2020).
The computed average technical efficiency of crop-livestock production under the CA farming system is estimated at 68.40%, with a range of 47.80–98.60%. Taking the present state of technology and the current input-output mix, this suggests that farms in the sample can increase their output by about 31.60% without changing their levels of input use (Table 3). The frequency distribution of technical efficiency across the sample is also presented in Table 3. Estimated efficiency measures reveal that 37.30% of the farms in the sample are relatively more efficient than the sample average efficiency level, with an efficiency score >83.40%, and 37 smallholder farms (62.70% of the total sample) having a mean efficiency of <59.20%.
The findings in Table 3 suggest a strong correlation between land and livestock holding and technical efficiency. The TE increases with livestock capital and decreases with land area. The results for the estimates of technical inefficiency with land holding and livestock capital depict: farmers with an average TE less than average in the sample (class 1) are with an average of 96.70 ha and 14,492.79 TND, and farmers with a TE greater than average in the sample (class 2) are with an average of 55.63 ha and 15,108.08 TND. This suggests the positive impact of livestock capital on TE.
This section focuses on assessing the impact of crop-livestock diversification on farm-level technical efficiency. Summary statistics of diversified and less-diversified farms are reported in Table 4. The farms in the sample are divided into three sub-categories: diversified, moderately specialized, and highly specialized crop-livestock enterprises. These are classified according to what their HI means.
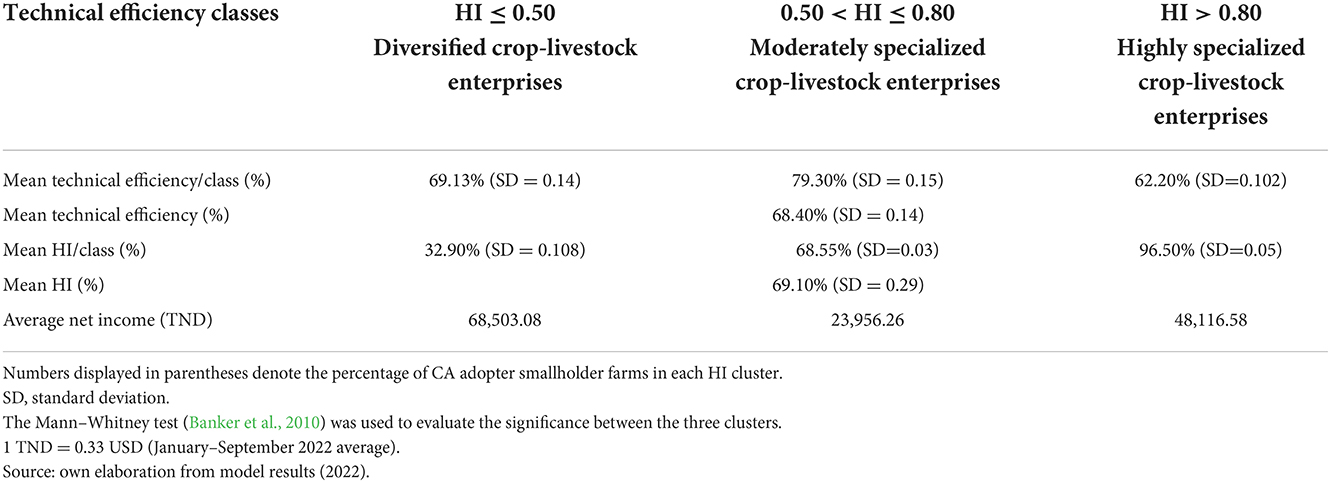
Table 4. Frequency distribution of technical efficiency according to HI clusters for CA adopter smallholders.
Summary analysis shows significantly higher technical efficiency scores for more diversified farms compared to more specialized farms (average technical efficiencies of 69.13 and 62.20%, respectively) (Table 4). The Mann–Whitney test (Banker et al., 2010) shows a z-statistic of 2.062 with P = 0.039, indicating a significant difference between the three technical efficiency clusters (Table 4). In addition, the mean technical efficiency is significantly greater for the moderately specialized (79.30%) compared to the highly specialized crop-livestock smallholders (62.20%), with a z-statistic of 2.81 and P = 0.00496. This can be explained by the fact that diversified enterprises in the study area are usually small in size and are managed by households that try to secure their livelihoods through small-scale diversification primarily devoted to daily income and consumption support. Thus, the scale of operation of these farms and their restricting structural characteristics are potential reasons behind their lower technical efficiency. The highly specialized crop-livestock enterprises are, however, medium-sized farms primarily focusing on either cereals or olive cultivations, in addition to other minor secondary activities. Highly specialized farms are faced with many challenges of market access and reliability and are affected by different types of risks compared to other, more diversified farms. These are all possible reasons for the lower technical efficiency of this group of farms. The empirical findings are consistent with other results in which the variability in technical efficiency is correlated across farming systems (Mariano et al., 2011; Rahman et al., 2011; Asante et al., 2020). Results suggest that diversified smallholders under CA farming systems appear to be operating not only at higher technical efficiencies but also translating into higher income (Table 3) toward promoting diversification (Table 4).
Evidence of synergies and economies of diversification in crop-livestock systems under the CA farming system
The model defined by Equation 8 is used to evaluate to what extent there are complementary synergies between farm activities. Results show evidence of complementary synergies between four of the six output combinations (Table 5). The relative coefficients of the second-order cross-partial derivatives indicate the strength of complementarity between three combinations from these four pairs: cereals with forage crops, cereals with legumes, and forage crops with livestock. The measured parameters for these three combinations are significant at P < 0.05. The fourth positive combination of forage crops with legumes is not significant.
The assessment of the crop-livestock combination reveals a high synergy between forage crops and livestock (with a parameter value of 0.56 significant at P < 0.05). This result implies that livestock production and forage crops are complementary activities, resulting in higher productivity among smallholder farmers operating under CA farming systems in Tunisian semiarid regions. This is not surprising, and strong complementarity indicates how this efficient combination is well integrated by diversified farm smallholders. Under CA, integrated crop-livestock systems are a form of sustainable intensification of agriculture that relies on harmonious relationships between forage and animal feeding system elements to reinforce critical agroecosystem processes, with potential impacts on farm-level productivity and resilience to climate change threats.
Similarly, the synergies between cereals (e.g., wheat) and legume crops (which are largely dual-purpose) in the cereal–livestock system indicate the synergetic way the diversified farmers have integrated these crop combinations in CA farming systems. This result reveals the benefit of this synergy toward improving soil health, including increasing soil organic matter, due to the adoption of conservation practices such as CA (Laroca et al., 2018; Souissi et al., 2020). Evidence suggests that crop diversification strategies applied by diversified smallholders under CA, such as combining legumes with wheat production in an efficient agronomic and management way, will provide a beneficial effect in improving soil fertility and, thus, enhancing productivity among all produced crops. The highest significant synergy is between cereals and forage crops (coefficient value of 2.84). The capacity of wheat crop activity to strengthen the complementarity with the forage crop activity is still strong, reflecting the complementarity advantages gained from the traditional small-ruminant (i.e., sheep)-cereal (i.e., wheat/barley) farming system under CA. The evidence of only slight and non-significant synergies between cereals and livestock activity is surprising, given the high levels of labor and farming management skills that both activities could share within the crop-livestock farming system. The existing competition between the two activities for the same inputs (e.g., land and labor) provides evidence of the low level of synergy. Chavas and Di Falco (2012) discussed the existing synergies in crop diversification in Ethiopian crop-farming systems. Similar synergies were also found in several global contexts, such as mixed farms in Australian wheat–sheep farming systems (Villano et al., 2010), Ghana crop-livestock farming systems (Asante et al., 2020), and Brazilian mixed crop-livestock systems (Carvalho et al., 2018; Peterson et al., 2020).
In this research paper, Carvalho et al. (2018) examined cover crop grazing as a management strategy for land-use diversification and sustainable intensification of agriculture in Brazil. The authors suggested that the use of animals to graze cover crops in rotation with cash crops while using no-till farming techniques added a level of system complexity uncommon in current food production systems. In such systems, emergent features result from complex interactions between the soil compartment, plants, and animals, which act as a storehouse of markers of higher-level ecosystem functioning. The management tool in ICLS that influences system performance in terms of positive or negative looping feedback is grazing intensity. A moderate grazing intensity is required to encourage positive feedbacks that balance productivity and sustainability. This has consequences for increased system resilience and economic profitability. Schuster et al. (2018) argued that grazing management and its relationship with crop rotation determine the severity of weed infestation in an ICLS, and the most important element influencing weed outcomes is forage allowance, together with the conventional management of no-tillage ICLS.
Such findings suggest that diversifying output combinations would lead to additional synergies for farm households that branch out into these activities under crop-mixed farming systems.
There are significant and non-complementary synergies between legumes and livestock (coefficient of −2.31). The fact that we found no evidence of significant dis-synergies between these two activities means that smallholder farmers operating under CA would not benefit from more specialized production processes in the landscape context considered in this research study. Legumes cultivated in the study area are usually rainfed food legumes (fava beans, chickpeas, lentils), which are either cultivated in open fields or between the lines of olive trees. As shown in the table, legumes in the study area are also negatively interacting with forage crops, which is indeed the case in the production systems investigated. For a farmer with small to medium livestock breeders, a preferred rotation will be to cultivate forage crops, which are essential to minimizing their feeding production costs. Farmers without livestock tend to cultivate food legumes. This suggests a benefit of diversification under CA farming systems, in which smallholder farmers integrate crops and livestock simultaneously to ensure the sustainability of their farming systems. The t-statistics and magnitude of the coefficient linking forage and legumes suggest a weak and non-significant complementary synergy between these two crops. This could be because legume crop production competes with the production of other crops (e.g., wheat and forage crops) on the limited existing resources, and so enhancing the production of legumes means sacrificing the limited resources to produce forage crops.
From the above, we can argue that the concept of integrated crop-livestock systems under CA has not been adequately adopted by smallholder farmers, and the agricultural system in the studied region continues to exhibit a low level of productivity and resource use efficiency. From another perspective, food insecurity indicators remained high among smallholder farming communities producing crops and livestock despite the availability of arable land, technologies, and an abundance of other natural resources. This suggests the need to better understand and address such complex problems as food security for these communities, as managing such diversified production systems could lead to a positive impact on reducing poverty and improving food security. This evidence was supported by Szymczak et al. (2020) in a study focusing on Brazilian smallholder farmers. They argue that in addition to diversifying the system, combining cropping with livestock was a good way to increase economic resilience. In the same sense, Danso-Abbeam et al. (2021), by applying a network model, investigate the input and output flow in a Latino Caribbean farm's integrated crop-livestock system and its relation to food security. They conclude that, with a significance level of 5%, the integrated crop-livestock diversity index was found to have a positive and significant impact on food security.
Conclusions and policy implications
This paper provides an empirical evaluation of diversification economies in crop-livestock systems, with a special focus on measuring technical efficiency and assessing complementary synergies at the smallholder farming system level operating under CA farming systems in the Tunisian dryland areas.
The objective of this study was to assess whether diversification or specialization in crop-livestock systems, conducted under CA, can be accredited to the exploration for better economic performance. Examining the economies of diversification under this system helps understand if smallholders can benefit from synergies through cost savings by choosing the optimal combination of outputs in crop-livestock production under a CA farming system. Additionally, this study also examined evidence concerning economies of scale in integrated CL systems among smallholder farmers. A stochastic input distance function was used, and a measure of synergies was undertaken based on an empirical framework of the second-order cross-partial derivative of output variables in the distance function model.
Empirical findings revealed that technical inefficiencies are significant in CL systems, suggesting that enhancing CL diversification will lead to improvements in technical efficiency. The key driving forces that significantly improved technical efficiency were farmers' education level, the share of off-farm income, and access to credit. This finding has important implications because it suggests that actions on these factors would lead to higher technical efficiency in crop-livestock production under CA. This result implies that policies in drylands:
• Consider the improvement in demographic characteristics (e.g., education, extension services, and knowledge of CA technology management) and institutional factors (e.g., Agricultural Development Group- GDA, Mutual Agricultural Service Company SMSA, and cooperatives).
• Provide financial support (i.e., credits and loans) with low-interest rates to smallholder farmers is considered a good way to overcome some of the financial barriers associated with technology adoption by these dryland farmers.
• While extension policy is expected to play an important role in enhancing the diversification and consequently the evolution of agricultural production, specifically crop-livestock production under CA, our findings emphasize the need to use the most effective extension measures. This could be through a participatory method of training including all key actors and at all levels (research, private, and extension) to both improve the access of farmers to various pieces of training and raise their awareness, particularly those who practice agricultural diversification under CA farming systems.
• Design appropriate strategies for enhancing the production of specific output combinations in crop-livestock diversified systems under CA among smallholders in rainfed areas.
• Promote the production and integration of crops such as legumes with other crops and livestock with other crops in diversified farming systems to enhance overall farm productivity. This will reduce food insecurity and poverty among rural farm households and the entire rural population.
These actions will certainly contribute to helping increase farmers' adaptive capacity and their acceptance of the adoption of agricultural diversification practices and ensure they generate concrete benefits by increasing income through sustainable agricultural operations and farming practices.
The contribution of diversification in output combinations to technical efficiency was very high and significant, indicating that specialization in integrated crop-livestock under CA farming systems leads to greater technical inefficiency. The evidence of strong synergies from complementarity between sheep and crop activities (e.g., forage crops) under CA farming systems implies that crop-livestock diversification is a desirable strategy for improving overall farm productivity. However, to achieve this, policymakers should include strategies for enhancing the production of specific output combinations in crop-livestock diversification systems among smallholders in Tunisia's arid areas. Such policies should promote the production and integration of crops such as wheat with forage crops and livestock with crop forages in diversified farming systems to enhance overall farm productivity.
The empirical findings presented in this paper were an attempt to help assess and understand the role that agricultural diversification can play in improving the livelihoods of CL farming households by generating high levels of income, especially under CA farming systems. By incorporating input distance functions into the productivity-based approach used in this research we were also able to assess the economic benefits of diversification to comprehend the economics of CL diversification.
Data availability statement
The raw data supporting the conclusions of this article will be made available by the authors, without undue reservation.
Author contributions
Conceptualization, validation, and writing—review and editing: BD, AFo, AFr, MA, HM'h, HO, and MR. Formal analysis: BD, AFo, AFr, MA, and HO. Investigation: BD, AFo, AFr, MA, HM'h, and MR. Software: BD, AFo, AFr, and MA. Writing—original draft: BD, AFo, AFr, MA, and HM'h. All authors contributed to manuscript revision, read, and approved the submitted version.
Funding
This research was conducted as part of the “Use of Conservation Agriculture in Crop–Livestock Systems (CLCA) in the Drylands for Enhanced Water Use Efficiency, Soil Fertility and Productivity in NEN and LAC Countries” project (https://mel.cgiar.org/projects/clca2) funded by IFAD (https://www.ifad.org/en/), under a grant agreement with the International Center for Agricultural Research in the Dry Areas (ICARDA—http://www.icarda.org – Agreement #20016) within the framework of the CGIAR Research Program on Livestock (https://livestock.cgiar.org/). This research was also partially funded by the CGIAR Research Initiative on Transformational Agroecology Across Food, Land, and Water Systems (Agreement #200302).
Conflict of interest
The authors declare that the research was conducted in the absence of any commercial or financial relationships that could be construed as a potential conflict of interest.
Publisher's note
All claims expressed in this article are solely those of the authors and do not necessarily represent those of their affiliated organizations, or those of the publisher, the editors and the reviewers. Any product that may be evaluated in this article, or claim that may be made by its manufacturer, is not guaranteed or endorsed by the publisher.
Author disclaimer
The opinions expressed here belong to the authors and do not necessarily reflect those of ICARDA, CGIAR, or IFAD.
Footnotes
1. ^See https://mel.cgiar.org/projects/clca2 for more information about the project.
References
Abdulai, A., and Abdulai, A. (2017). Examining the impact of conservation agriculture on environmental efficiency among maize farmers in Zambia. Environ. Dev. Econ. 22, 177–201. doi: 10.1017/S1355770X16000309
Ameur, W., Frija, A., Abdeladhim, M.A., and Thabet, C. (2021). Patterns of use of residue biomass in cereal–sheep production systems of North Africa: case of Tunisia. Agronomy. 11, 1–18. doi: 10.3390/agriculture11070612
Angar, H. (2010). Le développement de l'agriculture de conservation en Tunisie: Séminaire National: Dix ans d'Agriculture de Conservation en Tunisie: Bilan et Perspectives. Institut national des grandes cultures (INGC) - Tunisie.
Asante, B. O., Villano, R. A., and Battese, G. E. (2020). Evaluating complementary synergies in integrated crop-livestock systems in Ghana. Int. J. Soc. Econ. 47, 72–85. doi: 10.1108/IJSE-04-2019-0274
Asante, B. O., Villano, R. A., Patrick, I. W., and Battese, G. E. (2018). Determinants of farm diversification in integrated crop–livestock farming systems in Ghana. Renew. Agric. Food. Syst. 33, 131–149. doi: 10.1017/S1742170516000545
Asefa, S. (2011). Analysis of technical efficiency of crop producing smallholder farmers in Tigray, Ethiopia. Available online at: https://mpra.ub.uni-muenchen.de/40461/ (accessed December 2021).
Baccouri, S. (2008). Conservation Agriculture in Tunisia. Conservation Ag. Carbon Offset Consultation. West Lafayette/USA: FAO-CTIC.
Bachta, M. S. (2011). La céréaliculture en Tunisie Une politique de régulation à repenser. Les notes d'analyse du CIHEAM. 64, 19.
Bahri, H., Annabi, M., Cheikh M'hamed, H., Chibani, R., Chtourou, M., Riahi, N., et al. (2018). Evaluation de l'impact agro-environnemental et de la durabilité de l'adoption du semis direct au Nord de la Tunisie. Ann INRAT. 91, 98–111.
Bahri, H., Annabi, M., Cheikh M'hamed, H., and Frija, A. (2019). Assessing the long-term impact of conservation agriculture on wheat-based systems in Tunisia using APSIM simulations under a climate change context. Sci.Tot.Env. 1223–1233. doi: 10.1016/j.scitotenv.2019.07.307
Banker, R. D., Zheng, Z. E., and Natarajan, R. (2010). DEA-based hypothesis tests for comparing two groups of decision-making units. Eur. J. Oper. Res. 206, 231–238. doi: 10.1016/j.ejor.2010.01.027
Battese, G. E., and Coelli, T. J. (1995). A model for technical inefficiency effects in a stochastic frontier production function for panel data. Empir. Econ. 20, 325–332. doi: 10.1007/BF01205442
Baumol, W. (1982). Contestable markets: an uprising in the theory of industry structure. Am. Econ. Rev. 72, 1–15. doi: 10.2307/134928
Byrnes, R. C., Eastburn, D. J., Tate, K. W., and Roche, L. M. (2018). A global meta-analysis of grazing impacts on soil health indicators. J. Environ. Qual. 47, 758–765. doi: 10.2134/jeq2017.08.0313
Carvalho, P. C., de, F., Peterson, C. A., Nunes, P. A. de, A., Martins, A. P., de Souza Filho, W., et al. (2018). Animal production and soil characteristics from integrated crop-livestock systems: toward sustainable intensification. J.Ani.Sci. 96, 3513–3525. doi: 10.1093/jas/sky085
Chaplin, H. (2000). Agricultural diversification: a review of methodological approaches and empirical evidence. Available online at: http://www.ilr.uni-bonn.de/agpo/rsrch/Idara/Farm/wyewp2.doc (accessed December 2021).
Chavas, J. P., and Aliber, M. (1993). An analysis of economic efficiency in agriculture: a nonparametric approach. J. Agric. Resour. Econ. 18, 1–16.
Chavas, J. P., and Di Falco, S. (2012). On the role of risk vs. economies of scope in farm diversification with an application to Ethiopian farms. J. Agric. Econ. 63, 25–55. doi: 10.1111/j.1477-9552.2011.00319.x
Chavas, J. P., and Kim, K. (2007). Measurement and sources of economies of scope: a primal approach. J. Inst. Theor. Econ. 163, 411–427. doi: 10.1628/093245607781871354
Chavas, J. P., and Kim, K. (2010). Economies of diversification: a generalization and decomposition of economies of scope. Int. J. Prod. Econ. 26, 229–235. doi: 10.1016/j.ijpe.2010.03.010
Cheikh M'hamed, H., Bahri, H., and Annabi, M. (2018). Conservation agriculture in Tunisia: historical, current status and future perspectives for rapid adoption by smallholder farmers. Johannesburg, South Africa: Second African Congress on Conservation Agriculture. p. 57–60.
Cheikh M'hamed, H., Bahri, H., Annabi, M., Frija, A., and Idoudi, Z. (2022). “Historical Review and Future Opportunities for Wider Scaling of Conservation Agriculture in Tunisia,” Conservation agriculture in Africa: climate smart agricultural development, Mkomwa, S., Kassam, A., (ed.). p. 475. doi: 10.1079/9781789245745.0007
Coelli, T. J., and Fleming, E. (2004). Diversification economies and specialisation efficiencies in a mixed food and coffee smallholder farming system in Papua New Guinea. Agric. Econ. 31, 229–239. doi: 10.1111/j.1574-0862.2004.tb00260.x
Coelli, T. J., and Perelman, S. (2000). Technical efficiency of European railways: a distance function approach. Appl. Econ. 32, 1967–1976. doi: 10.1080/00036840050155896
Danso-Abbeam, G., Dagunga, G., Ehiakpor, D. S., Ogundeji, A. A., Setsoafia, E. D., and Awuni, J. A. (2021). Crop–livestock diversification in the mixed farming systems: implication on food security in Northern Ghana. Agri. Food Secur. 10, 1–14. doi: 10.1186/s40066-021-00319-4
DGACTA. (2017). Direction Générale de l'Aménagement et de la Conservation des Terres Agricoles: Elaboration de la Stratégie de Conservation des Eaux et des Sols de la Tunisie, Ministère de l'Agriculture, des Ressources Hydrauliques et de la Pèche, Tunisie, p. 202.
FAO (2010). An international consultation on integrated crop-livestock systems for development: The way forward for sustainable production intensification. Rome: Integrated Crop Management. Available online at: https://www.fao.org/publications/card/en/c/1ffb177e-64c9-5e77-8614-1342b8643e9d/
Frelat, R., Lopez-Ridaura, S., Giller, K. E., Herrero, M., Douxchamps, S., Djurfeldt, A. A., et al. (2016). Drivers of household food availability in sub-Saharan Africa based on big data from small farms. Proc. Natl. Acad. Sci. U.S.A. 113, 458–463. doi: 10.1073/pnas.1518384112
Friedrich, T., Derpsch, R., and Kassam, A. (2012). Overview of the global spread of conservation agriculture. Field Actions Sci. Rep. 6, 9.
Guesmi, H., Salem, H., and Moujahed, N. (2019). Integration crop-livestock under conservation agriculture system. J. New. Sci. 65, 4061–4065.
Hadley, D. (2006). Patterns in technical efficiency and technical change at the farm-level in England and Wales, 1982–2002. J. Agric. Econ. 57, 81–100. doi: 10.1111/j.1477-9552.2006.00033.x
Ibrahim, H., Rahman, S., Envulus, E., and Oyewole, S. (2009). Income and crop diversification among farming households in a rural area of north central Nigeria. Agro. Sci. 8, 84–89. doi: 10.4314/as.v8i2.51102
IFAD (2010). Integrated Crop–Livestock Farming Systems. Livestock Thematic Papers. Tools for Project Design. Rome: IFAD
Joshi, P. K., Gulati, A., Birthal, P. S., and Tewari, L. (2004). Agriculture diversification in South Asia: patterns, determinants and policy implications. Econ. Polit. Wkly. 39, 2457–2467.
Joshi, P. K., Gulati, A., and Cummings, R. W. (2007). Agricultural Diversification and Smallholders in South Asia. New Delhi, India: Academic Foundation.
Joshi, P. K., Joshi, L., and Birthal, P. S. (2006). Diversification and its impact on small holders: Evidence from a study on vegetable production. Agric. Econ. 19, 219–236.
Kankwamba, H., Mapila, M., and Pauw, K. (2012). Determinants and Spatio-Temporal Dimensions of Crop Diversification in Malawi. Project Report – No 2. Malawi: IFPRI.
Karimov, A. A. (2014). Factors affecting efficiency of cotton producers in rural Khorezm, Uzbekistan: Re-examining the role of knowledge indicators in technical efficiency improvement. Agric. Econ. 2, 1–16. doi: 10.1186/s40100-014-0007-0
Kassam, A., Kueneman, E., Kebe, B., Ouedraogo, S., and Youdeowei, A. (2009). Enhancing crop-livestock systems in conservation agriculture for sustainable production intensification: a farmer discovery process going to scale in Burkina. Integrated Crop Management. Rome: FAO.
Kassam, A. H., Friedrich, T., Shaxson, F., and Pretty, J. (2009). The spread of conservation agriculture: justification, sustainability and uptake. Int. J. Agric. Sust. 7, 292–320 doi: 10.3763/ijas.2009.0477
Khaldi, R., and Saaidia, B. (2017). Analyse de la Filière Céréalière en Tunisie et Identification des Principaux Points de Dysfonctionnement à L'origine des Pertes. Rapport de projet FAO (GCP/RNE/004/ITA). p. 102. Available online at: http://www.onagri.nat.tn/uploads/Etudes/RapportIVF.pdf (accessed November 2022).
Klapwijk, C. J., van Wijk, M. T., Rosenstock, T. S., van Asten, P. J. A., Thornton, P. K., and Giller, K. E. (2014). Analysis of trade-offs in agricultural systems: current status and way forward. Cur Opi Env Sust. 6, 110–115. doi: 10.1016/j.cosust.2013.11.012
Komarek, A. M., Kwon, H., Haile, B., Thierfelder, C., Mutenje, M. J., and Azzarri, C. (2019). From plot to scale: ex-ante assessment of conservation agriculture in Zambia. Agric. Syst. 173, 504–518. doi: 10.1016/j.agsy.2019.04.001
Landers, J. N. (2007). Tropical Crop-Livestock Systems in Conservation Agriculture: The Brazilian Experience. Rome: FAO: Integrated Crop Management.
Laroca, J. V., dos, S., Souza, J. M. A., de Pires, G. C., Pires, G. J. C., Pacheco, L. P., Silva, F. D., et al. (2018). Soil quality and soybean productivity in crop-livestock integrated system in no-tillage. Pesquisa Agropecuária Brasileira 53, 1248–1258. doi: 10.1590/s0100-204x2018001100007
Latruffe, L., Balcombe, K., Davidova, S., and Zawalinska, K. (2004). Determinants of technical efficiency of crop and livestock farms in Poland. Appl. Econ. 36, 1255–1263 doi: 10.1080/0003684042000176793
Liniger, H., Mekdaschi, S. R., Hauert, C., and Gurtner, M. (2011). Sustainable Land Management in Practice: Guidelines and Best Practices for Sub-Saharan Africa: Field Application. Rome: FAO
Loreau, M. (1998). Biodiversity and ecosystem functioning: a mechanistic model. Proc. Natl. Acad. Sci. U. S. A. 95, 5632–5636. doi: 10.1073/pnas.95.10.5632
Mariano, M. J., Villano, R., and Fleming, E. (2011). Technical efficiency of rice farms in different agroclimatic zones in the Philippines: an application of a stochastic metafrontier model. Asian. Econ. J. 25, 245–269. doi: 10.1111/j.1467-8381.2011.02060.x
Martin, G., Moraine, M., Ryschawy, J., Magne, M. A., Asai, M., Sarthou, J. P., et al. (2016). Crop–livestock integration beyond the farm level: a review. Agron. Sustain. Dev. 36, 1–21. doi: 10.1007/s13593-016-0390-x
Marzin, J., Bonnet, P., Bessaoud, O., and Ton-Nu, C. (2017). Study on Small-Scale Family Farming in the Near-East and North Africa Region: Synthesis. Rome: FAO
Masmoudi, A. (2012). Etude de certains paramètres de durabilité des systèmes de production céréaliculture-élevage dans le contexte de l'intégration des techniques de l'agriculture de conservation. [Doctoral dissertation]. Sétif: Université de Sétif 1-Ferhat Abbas
Mesfin, W., Fufa, B., and Haji, J. (2011). Pattern, trend and determinants of crop diversification: Empirical evidence from smallholders in Eastern Ethiopia. J. Econ. Sust. Dev. 2, 78–89.
Mhazo, N., Manyatsi, A. M., Masarirambi, M. T., and Mhazo, M. L. (2010). Conservation agriculture in an integrated crop and livestock farming system Challenges and opportunities in Swaziland. Swaziland. J. Sust. Dev. 1, 1–69.
Moraine, M., Duru, M., Nicholas, P., Leterme, P., and Therond, O. (2014). Farming system design for innovative crop–livestock integration in Europe. Animal 8, 1204–1217. doi: 10.1017/S1751731114001189
Moujahed, N., Abidi, S., Youssef, S. B., Darej, C., Chakroun, M., and Salem, H. B. (2015). Effect of stocking rate on biomass variation and lamb performances for barley stubble in Tunisian semi arid region and under conservation agriculture conditions. African J. Agric. Res. 10, 4584–4590. doi: 10.5897/AJAR2015.10396
Mrabet, R., Bahri, H., Zaghouane, O., Cheikh M'Hamed, H., Mohamed El-Areed, S.-R., and Abou El-Enin, M.-M. (2022). “Adoption and spread of Conservation Agriculture in North Africa,” in Advances in Conservation Agriculture Volume 3 Kassam, A. (ed.). Cambridge, UK: Adoption and Spread, Burleigh Dodds Science Publishing. p. 185–230 doi: 10.19103/AS.2021.0088.06
Ogundari, K. (2013). Crop diversification and technical efficiency in food crop production: a study of peasant farmers in Nigeria. Int. J. Soc. Econ. 40, 267–287 doi: 10.1108/03068291311291536
Paul, C. J. M., and Nehring, R. (2005). Product diversification, production systems, and economic performance in US agricultural production. J. Econ. 126, 525–548 doi: 10.1016/j.jeconom.2004.05.012
Peterson, C. A., Bell, L. W., Carvalho, P. C. D. F., and Gaudin, A. C. (2020). Resilience of an integrated crop–livestock system to climate change: a simulation analysis of cover crop grazing in southern Brazil. Front. Sust. Food Syst. 4, 604099 doi: 10.3389/fsufs.2020.604099
Petit, M., and Barghouti, S. (1992). “Diversification: challenges and opportunities,” in Trends in Agricultural Diversification, Barghouti, S., Garbus, L., and Umali, D. (Washington DC: World Bank) p. 1–13.
Pope, R. D., and Prescott, R. (1980). Diversification in relation to farm size and other socioeconomic characteristics. Am. J. Agric. Econ. 62, 554–559. doi: 10.2307/1240214
Rahman, S. (2009). Whether crop diversification is a desired strategy for agricultural growth in Bangladesh? Food Policy. 34, 340–349. doi: 10.1016/j.foodpol.2009.02.004
Rahman, S., Barmon, B. K., and Ahmed, N. (2011). Diversification economies and efficiencies in a ‘BlueGreen Revolution' combination: a case study of prawn-carp-rice farming in the ‘Gher' system in Bangladesh. Aquac. Int. 19, 665–682. doi: 10.1007/s10499-010-9382-z
Raunet, M., Richard, J. F., and Rojat, D. (2004). Premiers résultats d'introduction du semis direct sous couvert et lutte antiérosive en Tunisie, in Gestion de la biomasse, érosion et séquestration du carbone. Séquestration du carbone et érosion des sols, Roose, E., De Noni, G., Prat, C., Ganry, F. and Bourgeon, G. (Eds). Paris, France: institut de recherche pour le développement. p. 388–404.
Rekik, M., Frija, A., Idoudi, Z., López Ridaura, S., Louahdi, N., Dhehibi, B., et al. (2021). Use of Conservation Agriculture in Crop-Livestock Systems (CLCA) in the Drylands for Enhanced Water Use Efficiency, Soil Fertility and Productivity in NEN and LAC Countries – Progress Highlights: Year (3) - April 2020 to March 2021. Lebanon: International Center for Agricultural Research in the Dry Areas (ICARDA).
Richard, F. (2005). Le semis direct en Tunisie, La, Gazette des SCV au Cirad. Groupe Agence Française de Développement (AFD-France), 68 p. Available online at: http://open-library.cirad.fr/files/2/230__1162774426.pdf
Ryan, J. G., and Spencer, D. (2001). Future Challenges and Opportunities for Agricultural RandD in the Semi-arid Tropics. Patancheru, India: International Crops Research Institute for the Semi-Arid Tropics.
Schuster, M. Z., Harrison, S. K., de Moraes, A., Sulc, R. M., Carvalho, P. C. F., Lang, C. R., et al. (2018). Effects of crop rotation and sheep grazing management on the seedbank and emerged weed flora under a no-tillage integrated crop-livestock system. J. Agri. Sci. 1–11. doi: 10.1017/S0021859618000813
Sichoongwe, K., Mapemba, L., Ng'ong'ola, D., and Tembo, G. (2014). The Determinants and Extent of Crop Diversification Among Smallholder Farmers: A Case Study of Southern Province, Zambia. Washington, DC: IFPRI. doi: 10.5539/jas.v6n11p150
Singbo, A. G., Emvalomatis, G., and Oude Lansink, A. (2021). The effect of crop specialization on farms' performance: a Bayesian non-neutral stochastic frontier approach. Front. Sustain. Food Syst. 5, 711530. doi: 10.3389/fsufs.2021.711530
Souissi, A., Bahri, H., Cheikh M'hamed, H., Chakroun, M., Ben Youssef, S., Frija, A., et al. (2020). Effect of Tillage, Previous Crop, and N Fertilization on Agronomic and Economic Performances of Durum Wheat (Triticum durum) under Rainfed Semi-arid Environment. Agronomy. 10, 1–16. doi: 10.3390/agronomy10081161
Szymczak, L. S., de Faccio, P., Lurette, C. A., De Moraes, A., de Albuquerque Nunes, P. A., et al. (2020). System Diversification and Grazing Management as Resilience-Enhancing Agricultural Practices: The Case of Crop-Livestock Integration. Agricultural Systems, Elsevier Masson. p. 184.
Tarawali, S., Keating, J., Powell, J., Hiernaux, P., Lyasse, O., and Sanginga, N. (2004). “Integrated natural resource management in West African crop–livestock systems,” in Sustainable Crop–livestock Production for Improved Livelihoods and Natural Resource Management in West Africa, Williams, T. P., Tarawali, S. A., Hiernaux, P., and Fernandez-Rivera, S. (Ibadan: IITA) p. 349–370.
Theodoridis, A., Ragkos, A., Roustemis, D., Arsenos, G., Abas, Z., and Sinapis, E. (2014). Technical indicators of economic performance in dairy sheep farming. Animal. 8, 133–140. doi: 10.1017/S1751731113001845
Tibesigwa, B., Visser, M., and Turpie, J. (2015). The impact of climate change on net revenue and food adequacy of subsistence farming households in South Africa. Environ. Dev. Econ. 20, 327–353. doi: 10.1017/S1355770X14000540
Tilman, D., Wedin, D., and Knops, J. (1996). Productivity and sustainability influenced by biodiversity in grassland ecosystems. Nature. 379, 718–720 doi: 10.1038/379718a0
Tittonell, P., Gérard, B., and Erenstein, O. (2015). Tradeoffs around crop residue biomass in smallholder crop-livestock systems – What's next?, Agr Sys. 134, 119-128. doi: 10.1016/j.agsy.2015.02.003
Villano, R., Fleming, E., and Fleming, P. (2010). Evidence of farm-level synergies in mixed-farming systems in the Australian wheat-sheep zone. Agric. Syst. 103, 146–152. doi: 10.1016/j.agsy.2009.11.005
Vyas, V. (2006). “Diversification in agriculture: concept, rationale and approaches,” in Indian Agriculture in the New Millennium: Changing Perceptions and Development Policy, Mujumdar, N. A., and Kapila, U. (New Delhi: Academic Foundation) p. 245–256.
Weiss, C. R., and Briglauer, W. (2000). Determinants and Dynamics of Farm Diversification. FE Working Paper-No. 2. Kiel: Universität Kiel, Department of Food Economics and Consumption Studies.
Keywords: conservation agriculture, economies of diversification, input distance function, integrated crop-livestock farming, technical efficiency
Citation: Dhehibi B, Fouzai A, Frija A, Adhim MA, M'hamed HC, Ouerghemmi H and Rekik M (2023) Assessing complementary synergies for integrated crop–livestock systems under conservation agriculture in Tunisian dryland farming systems. Front. Sustain. Food Syst. 6:1022213. doi: 10.3389/fsufs.2022.1022213
Received: 18 August 2022; Accepted: 11 November 2022;
Published: 04 January 2023.
Edited by:
Subhasis Mandal, Central Soil Salinity Research Institute (ICAR), IndiaReviewed by:
Suresh Kumar, Central Soil Salinity Research Institute (ICAR), IndiaDwaipayan Bardhan, Indian Veterinary Research Institute (IVRI), India
Copyright © 2023 Dhehibi, Fouzai, Frija, Adhim, M'hamed, Ouerghemmi and Rekik. This is an open-access article distributed under the terms of the Creative Commons Attribution License (CC BY). The use, distribution or reproduction in other forums is permitted, provided the original author(s) and the copyright owner(s) are credited and that the original publication in this journal is cited, in accordance with accepted academic practice. No use, distribution or reproduction is permitted which does not comply with these terms.
*Correspondence: Boubaker Dhehibi, Yi5kaGVoaWJpQGNnaWFyLm9yZw==
†These authors have contributed equally to this work and share first authorship
‡These authors have contributed equally to this work and share last authorship