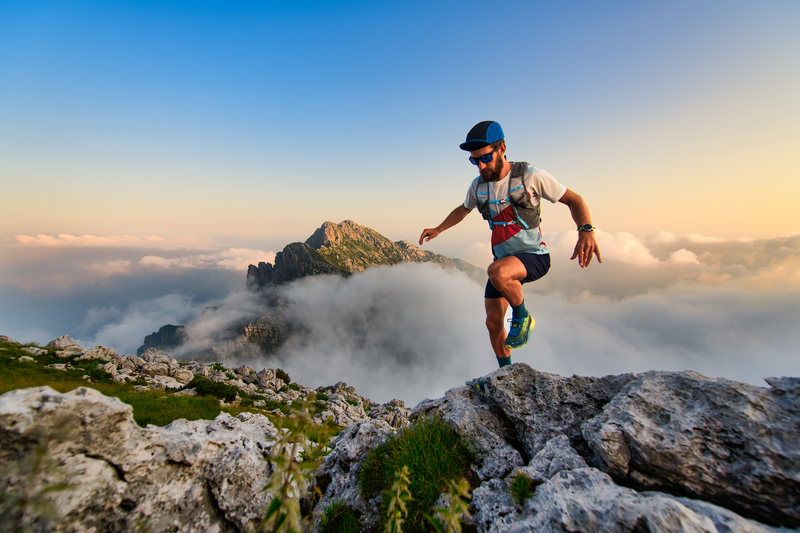
95% of researchers rate our articles as excellent or good
Learn more about the work of our research integrity team to safeguard the quality of each article we publish.
Find out more
ORIGINAL RESEARCH article
Front. Sustain. Food Syst. , 21 September 2022
Sec. Land, Livelihoods and Food Security
Volume 6 - 2022 | https://doi.org/10.3389/fsufs.2022.1009469
This article is part of the Research Topic Sustainable Soil Fertility Practices for Smallholder Farmers View all 11 articles
Low productivity of rice in Uganda is attributed to sub-optimal production practices related to soil nutrient, crop and weed management. Application of improved management practices could enhance productivity. Returning 1 year after a joint experimentation in which different components of recommended agronomic practices (RAP) for rice were tested, we assessed change in management practices and grain yield of participating farmers (participated in joint experimentation) and non-participating farmers (did not participate) with plots in the same irrigation scheme. Participating farmers belonging to the lower-yielding farmers under farmers' practice (FP) during joint experimentation improved their management practices, compared with the middle- and top-yielding farmers. Sixty-one, 24 and 7% of lower-, middle- and top-yielding farmers, respectively, weeded earlier after experimentation compared with weeding time under FP during joint experimentation. Seventy-nine percent of lower-yielding farmers used fertiliser after experimentation compared with 18% during experimentation, with a higher N rate increase than middle- and top-yielding farmers. Overall, participating farmers transplanted and weeded earlier, and applied slightly higher N rates compared with non-participating farmers. Top-yielding farmers had significantly (p = 0.03) higher grain yield, followed by middle- and lower-yielding farmers. However, lower-yielding farmers made significantly (p < 0.001) higher yield gain than middle- and top-yielding farmers. A paired t-test showed that average yield gain was 1,358 (1,027–1,689), 473 (252–695) and −91.7 (−397–213) kg ha−1, respectively, for lower-, middle- and top-yielding farmers. Participating farmers had higher grain yield (4,125 kg ha−1) than non-participating farmers (3,893 kg ha−1). Three farm types were identified that differed in application of RAP, however, with small differences in household characteristics. The farm type with higher fertiliser use in nursery and field, line transplanting, timely weeding and higher N rate had the highest grain yield. We conclude that joint experimentation had a larger effect on raising yield of lower-yielding farmers, bringing farmers closer in their management and outputs. Lack of differences among farm households could indicate that wealth is not crucial in innovation adoption in this production system.
Rice (Oryza sativa L.) in Uganda has become an important food staple and cash crop, especially among smallholder farmers, making it the second most important cereal after maize [KilimoTrust, 2014; Uganda Bureau of Statistics (UBOS), 2021]. Yet, rice yield in Uganda averages only 2,800 kg ha−1 for both irrigated and rainfed rice compared with the global average yield of 4,700 kg ha−1 [Food and Agriculture Organisation of the United Nations (FAO), 2021], and a yield potential in sub-Saharan Africa of 9,200 and 7,000 kg ha−1 for irrigated and rainfed rice, respectively (Global Yield Gap Atlas, 2022). As a result, demand for rice surpasses production, which has resulted in an average net annual milled rice import of around 62,000 tonnes between 2015 and 2020, costing the country about USD 23.2 million each year [Food and Agriculture Organisation of the United Nations (FAO), 2022]. The low yield is attributed to, among other factors, soil-related constraints, poor management of rice fields, and use of low-yielding varieties.
Application of modern agricultural production technologies, such as improved weed management practices, appropriate use of inorganic fertilisers, and modern, high-yielding varieties could enhance rice crop productivity, hence reducing shortage and saving money spent on imports. This is in addition to increasing household food security, reducing poverty directly through increased household incomes and welfare, and indirectly through lower food prices and higher wages (Kassie et al., 2011, 2018; Manda et al., 2019; Wossen et al., 2019). Yet, even with the evident benefits of many of the new agricultural technologies, smallholder farmers either do not adopt them or it takes a long time for such technologies to start being adopted (Mottaleb, 2018). The lack of or slow adoption of improved agricultural technologies is attributed to high costs, uncertainties about proper application and success under local farmers' environmental conditions, and farmers' perceptions and expectations (Mottaleb, 2018; Sinyolo, 2020). Further studies show that variation in adoption of improved management practices that enhance crop yields is related to differences in socio-economic characteristics of farm households, including, for instance, family size, farm size and income, farmers' age and education, labour availability, availability of cash for investment, and risk perception (Danlami et al., 2016; Fosso and Nanfosso, 2016; Hassan et al., 2016; Tadesse et al., 2017; Takahashi et al., 2020; Urfels et al., 2021). Moreover, past participation in on-farm trials, training and awareness about the technology, and contact with extension agents are shown to influence adoption (Danlami et al., 2016, 2019; Hassan et al., 2016; Takahashi et al., 2020). Due to such constraints, improved agricultural technologies may not necessarily result in poverty reduction as some of these constraints make improved technologies inaccessible and less profitable for the poorer farmers (Wossen et al., 2019).
Increasing rice production for enhanced food and income security requires understanding the intricacy of smallholder rice farms in Uganda and their use of improved management practices (Giller et al., 2011; Priegnitz et al., 2019). Getting insights into the diverse and specific farm types necessitates evaluating the uptake of improved management practices in the rice production system together with the socio-economic characteristics and the associated variation in yield among rice farmers. Developing farm typologies i.e., collections of farms that are homogeneous in uptake of improved management practices (Priegnitz et al., 2019) is thus the first and crucial step in examining the adoption of improved management practices in smallholder farms. These typologies could help support more strong policy interventions as well as advisory programmes to improve the adoption of production technologies to increase rice yields (Banerjee et al., 2014). Typologies can also be used to help support the development, implementation and monitoring of agricultural development projects; and to develop more suitable agricultural technologies and policies for less-favoured regions and households. This is in addition to being a practical framework for designing differentiated approaches to addressing rural challenges (Kuivanen et al., 2016; Priegnitz et al., 2019).
This study assessed the change in management practices and grain yield of rice farmers 1 year after the end of a 1-year joint experimentation, conducted between January and December 2019 on-farm together with farmers, where different components of recommended agronomic practices (RAP) for lowland rice production were tested (Awio et al., 2022). Farm types were identified and characterised based on packages of RAP applied on-farm. Components of RAP that were used to form clusters of farms based on how these improved management practices were taken up by the farmers included field levelling, use of certified seed, use of fertiliser in the nursery bed, timely transplanting, line transplanting, gap-filling, use of fertiliser in the field, and timely weeding. For these farm clusters, differences in their socio-economic characteristics and additional rice farming practices were evaluated. The overall objective of the study was to evaluate how the joint experimentation with farmers translated 1 year later in changes in farmers' management practices and, hence, grain yield, and to identify factors that were related to uptake of improved management practices. Specific objectives of the study were (i) to assess change in management practices and the related change in grain yield of farmers 1 year after participation in joint experimentation, (ii) to compare management practices, grain yield and yield gap of farmers who participated and those who did not participate in the joint experimentation, and (iii) to identify and characterise farm types based on packages of RAP which farmers have adopted. Examining the impact of farmers' participation in a joint experimentation may be crucial in informing decisions on how yield enhancing technologies for rice could be delivered to farmers to ensure adoption and realisation of expected results.
The study was conducted in the Doho rice irrigation scheme, where components of recommended agronomic practices (RAP) for rice had been previously tested in researcher led on-farm field trials designed and managed together with farmers (Awio et al., 2022). The Doho rice irrigation scheme is located in Eastern Uganda in the Butaleja district1 (34°02′ E, 0°56′ N). It is the largest public rice irrigation scheme in Uganda (Wanyama et al., 2017), covering an area of 1,000 ha, of which 952 ha is cultivated by over 4,000 smallholder farmers. It lies at an altitude of 1,100 m a.s.l. and belongs to the Lake Kyoga basin agroecological zone. It receives irrigation water from River Manafwa that originates from Mt. Elgon. The annual average rainfall in the area is 1,186 mm, distributed over two rainy seasons, from March to May and from August to October. The annual average temperature here is 22.7°C, with daily mean temperatures ranging from 15.4°C to 30.7°C (Namyenya, 2014). The scheme is divided into 11 blocks, each block sub-divided into 5–15 strips, and each strip having 20–30 farmers. Rice varieties commonly grown by farmers within the scheme are K 98 and K 85.
A semi-structured questionnaire was used to collect comprehensive information from smallholder rice farmers in the study area. Field observations were made at the time of field visits after individual farmer interviews to collect information on farmers' crop management practices and grain yield. Pre-testing of the semi-structured questionnaire was done at the beginning of January 2021 with 7 farmers within the scheme. The questionnaire was then refined and revised with closed and open-end questions to improve further discussion with respondents. In total, 146 rice farmers distributed across 6 sub-counties, 20 parishes and 41 villages of the Butaleja district were interviewed face-to-face in the local language (and only for the literate farmers upon indicating preference, English was used) by specifically trained enumerators from mid-January to the start of May 2021. Of these 146 farmers, 86 were part of the 114 farmers who participated in the joint experimentation of 2019 (herein referred to as participating farmers) and 60 had not taken part in the joint experimentation (herein denoted as non-participating farmers). These latter 60 farmers were purposively selected, as a control group to compare with the participating farmers, based on records from block leaders and willingness to take part in the study, to include farmers considered to be from poor, medium and rich socio-economic backgrounds. All farmers who participated in this study were those who were in production and were to harvest their rice within the time frame of the study to make assessment of crop management practices and grain yield in farmers' fields possible. The study was conducted with informed oral consent by all respondents. Confidentiality of all information collected was guaranteed and research protocols ensured that it was impossible to link published, aggregated data to individual respondents. Applicable guidelines and regulations for survey ethics were diligently followed. No ethical approval prior to the study was obtained as this was not required in Uganda.
The collected information (Table 1) included characteristics of the farm household head and farmer (name, gender, age, education), household size, farm size (total household land area, total land area under rice production), herd size (total herd size, number of cattle, small ruminants and poultry), farmer's participation in the joint experimentation, information on family and hired labour for rice production, duration in rice growing, and on rice management practices including adoption of all specified RAP (cf. Table 1), seed source, grain yield, and market price for paddy and milled rice. Cropping area was recorded in acres and converted to hectares for reporting (1 ha being equal to 2.47 acres). All costs were recorded in Ugandan Shillings (UGX) and where it is converted to US Dollar for reporting the exchange rate of May 2021 was used (1 USD = 3,530 UGX). For grain yield estimation, a survey plot of 10 m × 10 m within each farmer's field was marked from the centre of the field during field observations and a net plot of 4 m × 4 m from within the 10 m × 10 m plot was defined for final yield assessment. At harvest all panicles from the net plot were cut using a sickle, threshed, sun-dried, and the grains winnowed to remove empty grains. Grain weight and moisture content were measured using a digital weighing scale (Mini Crane scale model MNCS-M) and moisture metre (SATAKE Moistex Model SS-7). Rice grain yield adjusted to 0% moisture content (dry weight) was expressed in kg ha−1.
Table 1. Description of variables, units, and minimum and maximum values of variables used in the principal component analysis (PCA) and cluster analysis (CA), and the subsequent characterisation of farm types.
To assess differences in management practices and grain yield among all farmers, data were subjected to analysis of variance (ANOVA) using an unbalanced treatment structure in Genstat (19th edition) at 5% probability, taking the different farmers' fields as blocks. Where differences were significant, treatment means were separated using Fisher's least significant difference (LSD) test. A paired t-test was done for participating farmers to assess individual farmer's yield gain after participation in the joint experimentation. To quantify the effect of change in management practices of participating farmers after joint experimentation on grain yield, regression analysis using Generalised Linear Model was used. The exploitable yield gap (i.e., the difference between attainable farm yield and actual farm yield) was estimated using the top decile approach (Stuart et al., 2016). Attainable farm yield was defined as the mean yield of the top 10-percentile of yields from all farmers' fields after joint experimentation, and actual farm yield was taken as the mean yield of participating and non-participating farmers. To evaluate the differences in management practices and grain yield of participating farmers, these farmers were grouped based on grain yield in (i) farmers' practice (FP) plot and (ii) recommended agronomic practice (RAP) plot during the joint experimentation. Based on FP plot yield, farmers were categorised as lower-yielding (with grain yields between 1,364 and 3,037 kg ha−1 dry weight, n = 28), middle-yielding (with grain yields varying from 3,048–4,050 kg ha−1 dry weight, n = 29) and top-yielding (with grain yields ranging from 4,065–5,545 kg ha−1 dry weight, n = 29) third during the joint experimentation. Based on RAP plot yield, farmers were grouped as those who had higher yield in RAP plot compared with FP plot (i.e., RAP yield > FP yield: RAP and FP yield ranged from 2,210 to 5,753 and 1,364 to 5,545 kg ha−1 dry weight, respectively, n = 69) and those who had higher FP plot yield compared with RAP plot yield (i.e., FP yield > RAP yield: FP and RAP yield ranged from 3,048 to 4,825 and 2,875 to 4,255 kg ha−1 dry weight, respectively, n = 17) during the joint experimentation.
To construct farm typologies, where combined data for participating and non-participating farm households were used, SPSS (Statistical Package for the Social Sciences), version 25.0 was used to analyse the data following a multivariate method. A principal component analysis (PCA) was first used to reduce the number of variables into a new set of components. Eight variables related to components of RAP (i.e., field levelling, use of certified seed, use of fertiliser in the nursery bed, timely transplanting, line transplanting, gap-filling, use of fertiliser in the field, and timely weeding) were chosen for the PCA. Three principal components exceeding an eigenvalue of 1.00, according to Kaiser's criterion, were retained accounting together for 50.1% of the total variance (Supplementary Table S1). Kaiser-Meyer-Olkin (KMO) measure of sampling adequacy indicated the sample was adequate (value of 0.56) and Bartlett's test of sphericity (p = 0.008) showed that the analysis would be valid. Evaluating the correlations between the variables and the three components, a loading of >0.50 was considered. With the identified components, a hierarchical, agglomerative cluster analysis (CA) was done using Ward's method to minimise the variance within a cluster and squared Euclidean distance to measure the distances. After clustering farms based on the components of RAP applied, one-way ANOVA was used to test for significant differences between clusters for variables in the categories: RAP components adopted, socio-economic characteristics, rice production and farming knowledge, and other sources of income (Table 1). Differences in means between the clusters were separated using Fisher's LSD test. The proportion of participating and non-participating farm households in each identified cluster or farm type was determined. Analysis of household characteristics for participating and non-participating farmers in each cluster was made where results indicated households under a given cluster, whether participating or non-participating farm households, were identical (Supplementary Table S2).
Participating farmers that had lower (lower-yielding third), moderate (middle-yielding third) and higher (top-yielding third) yields under FP during joint experimentation likewise observed significantly different yields after experimentation (p = 0.03, SED = 169). Top-yielding farmers during joint experimentation also had the highest mean yield 1 year later (4,379 kg ha−1), followed by middle (4,039 kg ha−1) and lower (3,951 kg ha−1) yielding farmers who had similar yields (Figure 1A). Average yields during experimentation had been 4,471, 3,566 and 2,593 kg ha−1, respectively, for the top-, middle- and lower-yielding farmers (p < 0.001, SED = 98.5). Median yields after experimentation were 4,458, 4,161 and 3,895 kg ha−1, against 4,323, 3,589 and 2,772 kg ha−1 during experimentation, respectively, for the top-, middle- and lower-yielding farmers (Figure 1A). Despite having lower grain yield, lower-yielding farmers observed a significantly (p < 0.001, SED = 199) higher yield gain compared with the middle- and top-yielding farmers (Figure 1B; Supplementary Table S3). A paired t-test indicated that at individual farmer's field, average yield gain was 1,358 kg ha−1, ranging between 1,027 and 1,689 kg ha−1 for the lower-yielding farmers (p < 0.001). This gain was on average 473 kg ha−1 for the middle-yielding farmers, ranging between 252 and 695 kg ha−1 (p < 0.001). Top-yielding farmers had on average −91.7 kg ha−1 yield gain, ranging from −397 to 213 kg ha−1 (p = 0.54) (Supplementary Table S3).
Figure 1. Distribution of grain yield during and after joint experimentation (A), and yield gain of farmers 1 year after participation in joint experimentation (B). Absolute yield gain was calculated as the difference between grain yield under FP (farmers' practice) 1 year after and during joint experimentation. FP yield 2019: FP yield during joint experimentation in 2019; FP yield 2021: FP yield in 2021, 1 year after joint experimentation; Lower- (n = 28), middle- (n = 29) and top- (n = 29) yielding farmers are grouped based on yield obtained under FP during joint experimentation.
A multiple linear regression showed that change in grain yield after joint experimentation was influenced by change in weeding time and the combined effect of change in fertilisation timing and N rate, accounting for overall 41% of the yield gain observed (Table 2). Weeding and fertilisation by 1 day earlier on average increased grain yield by 40.9 and 11.6 kg ha−1 day−1, respectively. Increasing N amount by 1 kg ha−1 resulted in 16.6 kg ha−1 increase in grain yield (Table 2). There was a significant difference (p < 0.001, SED = 3.20) in change of weeding time among the farmers, explaining the observed differences in yield gain. Lower-yielding farmers improved their weeding time by weeding on average 2 days earlier (ranging from 2 days later to 6 days earlier) after experimentation compared with weeding time in the FP plot during experimentation. Middle- and top-yielding farmers weeded on average 8 (range: 3–12) and 14 (range: 9–19) days later, respectively, after experimentation compared with weeding time in the FP plot during experimentation (Table 3). Overall, the majority (61%) of lower-yielding farmers weeded earlier than their weeding time in the FP plot during joint experimentation, compared with 24 and 7% of middle- and top-yielding farmers, respectively (Figure 2). In all, weeding time delay compared with recommended weeding time, after experimentation, was not different among farmers (p > 0.05, SED = 2.76), with average weeding delay of 14, 15 and 15 days, respectively, for the lower-, middle- and top-yielding farmers (Figure 3). During experimentation, this weeding delay was significantly different among farmers (p < 0.001, SED = 1.76), where lower-, middle- and top-yielding farmers had an average weeding delay of 16, 8 and 3 days, respectively. Lower-yielding farmers also had a slightly larger N rate increase of 19.7 kg ha−1 compared with 16.0 and 12.2 kg ha−1 increase in N rate for the middle- and top-yielding farmers, after experimentation, respectively (Table 3).
Table 2. Slopes of linear regression lines relating change in grain yield on farmers' fields and change in management practices of farmers after participation in joint experimentation, Doho rice irrigation scheme, Butaleja District, Uganda, 2021.
Table 3. Grain yield and management practices during (2019) and after (2021) joint experimentation separately for the same farmers with lower, middle and higher yields during joint experimentation in 2019.
Figure 2. Change in weeding time by farmers after joint experimentation, Doho 2021. Change in weeding time was calculated as the difference between weeding time in FP plot during (2019) and after (2021) joint experimentation, days after transplanting (DAT). Minus (–) value implies that weeding time after experimentation was later compared with weeding time in FP plot during experimentation.
Figure 3. Weeding time delay compared with weeding time under recommended agronomic practices (RAP) during (2019) and after (2021) joint experimentation. Weeding time delay was calculated as the difference in weeding time under FP and RAP. Weeding time delay 2019: weeding delay during joint experimentation in 2019; Weeding time delay 2021: weeding delay after joint experimentation in 2021; Lower- (n = 28), middle- (n = 29) and top- (n = 29) yielding farmers are grouped based on yield obtained under FP during joint experimentation. For the top-yielding farmers in 2019, median was zero delay and negative weeding time delay is not possible, hence a lower whisker is absent.
Analysis of household socio-economic characteristics of lower-, middle- and top-yielding farmers showed no major difference among households that could explain differences in yield or yield gain among farmers. Even though lower-yielding farm households had significantly smaller household size, fewer small ruminants and less income from other crops compared with middle- and top-yielding farm households (Table 4), these differences were small and populations overlapped. Likewise lower-yielding farm households had smaller herd size, land size, area under rice production and a larger income from off-farm activities, however, these differences were not significant among groups. The lack of clear differences among farm households could indicate that all these farmers have the potential to achieve the higher yield levels attained by top producers under their current production system.
Table 4. Household socio-economic characteristics of lower-, middle- and top-yielding farmers, Doho rice irrigation scheme, Butaleja District, Uganda, 2021.
To test if farmers who had, during the joint experimentation, a higher yield in their FP plots than their RAP plots would differ in yield and practices from farmers who had a lower yield in their FP plots than their RAP plots, these two groups were analysed separately. Farmers who had higher yield on their FP plot compared with their RAP plot during joint experimentation also had on average higher yields after experimentation (4,385 kg ha−1, median 4,401 kg ha−1) than farmers who had lower yield under FP plot compared with RAP plot during joint experimentation (4,061 kg ha−1, median 4,153 kg ha−1), (Figure 4A). However, this yield difference was statistically marginal (p = 0.07, SED = 176). During the joint experimentation the average FP yields of these two groups was 3,922 (median 3,854) and 3,464 (median 3,313) kg ha−1, respectively (p = 0.05, SED = 227). Overall, average yield gain by farmers after joint experimentation was 571 kg ha−1, varying between 366 and 776 kg ha−1 (Supplementary Table S3). The yield gains were not different (p = 0.61, SED = 260) between these two groups of farmers (Figure 4B; Supplementary Table S3). At individual farmer's field, a paired t-test showed an average yield gain of 597 (median 630, value ranging between 348 and 846) and 463 (median 420, values ranging 200–727) kg ha−1, respectively, for farmers who had lower and higher yields under FP plot compared with RAP plot during the joint experimentation (Figure 4B; Supplementary Table S3). All management practices a year after the joint experimentation were similar among these two groups except weeding time, where 35% of farmers who had higher yield under FP plot compared with RAP plot weeded within 21 DAT, with an average weeding time of 30 DAT (p < 0.001, SED = 0.08). This was only 4% for farmers who had lower yield under FP plot compared with RAP plot, with an average weeding time of 37 DAT.
Figure 4. Distribution of grain yield during (2019) and after (2021) joint experimentation (A) and yield gain of farmers after participation in joint experimentation (B). Absolute yield gain was calculated as the difference between grain yield under FP (farmer's practice) after and during joint experimentation. FP yield 2019: FP yield during joint experimentation in 2019; FP yield 2021: FP yield after joint experimentation in 2021; RAP yield > FP yield (n = 69): farmers who had lower yield under FP plot compared with RAP plot; and FP yield > RAP yield (n = 17): farmers who had higher yield under FP plot compared with RAP plot, during joint experimentation; RAP, recommended agronomic practices.
Grain yield varied significantly among farmers who participated (participating farmers) and those who did not participate (non-participating farmers) in the joint experimentation (p = 0.05). Participating farmers had a higher grain yield, averaging 4,125 kg ha−1 compared with 3,893 kg ha−1 average grain yield for non-participating farmers (SED = 117). Median yield was 4,184 kg ha−1 for participating farmers, with grain yield ranging between 2,636 and 5,905 kg ha−1. Median yield was 3,971 kg ha−1 for non-participating farmers, and grain yield varied from 1,930 to 5,423 kg ha−1 (Figure 5). The exploitable yield gap was 20.0 and 24.5%, respectively, for participating and non-participating farmers, when the average of top-decile yield from all farmers' fields after joint experimentation (5,158 kg ha−1) was taken as attainable farm yield.
Figure 5. Cumulative distribution of grain yield of farmers after joint experimentation in 2021. Participating farmers are those who participated and non-participating farmers did not participate in the joint experimentation.
We observed differences in some management practices between participating and non-participating farmers. Sixteen percent of participating farmers used certified seed, and 28% transplanted timely, with average transplanting time of 32 DAS, compared with 3 and 13% for non-participating farmers, respectively (Table 5). Differences in weeding time (36 vs. 39 DAT) and N amount (24.3 vs. 19.8 kg ha−1) were statistically marginal (p = 0.07), between participating and non-participating farmers. Analysis of household data showed no difference among participating and non-participating farm households, except for training on rice farming which was significantly different (p < 0.001). More participating farmers (93%) had attended training related to rice production than non-participating farmers (50%) (Table 5). Participating farmers had also spent slightly more years in rice growing than non-participating farmers.
Table 5. Management practices and household socio-economic characteristics of participating and non-participating farmers, Doho rice irrigation scheme, Butaleja District, Uganda, 2021.
Analysis of household socio-economic data indicated that 86% of the farm households were male headed, with 40% of household heads having attained education higher than primary level (Table 6). The average farmer's age was 40 years and 42% of the farmers had attained education higher than primary school. Total household size was on average 10.2 persons. Farmers had on average 1.20 ha of farmland, of which 0.47 ha was under rice production. In terms of labour input in rice production, family and hired labour per rice growing season per ha was on average 22 persons. Regarding application of recommended agronomic practices for rice, 73% of farmers used fertiliser in the nursery bed, 22% transplanted timely (within 28 DAS rice seeds in the nursery), 36% used line transplanting, 76% applied fertiliser in the field, with an average N rate of 22.4 kg ha−1 and 12% weeded timely (within 21 DAT rice seedlings, Table 6). Only 11% of the farmers used certified rice seeds, while 98% did field levelling and 95% incorporated rice straw from the previous season into the soil.
Table 6. Characteristics of farm households and the identified clusters including the p-value of one-way analysis of variance of differences between farm types.
Principal component analysis (PCA) and cluster analysis (CA) resulted in identification of three different clusters defined as farm types with their characteristics (Table 6). Cluster 1 (farms with less application of RAP) constituted the second largest cluster with 28% (n = 41) of the farms. Of the farmers in this group, 32% used fertiliser in the nursery, 15% transplanted timely, 5% transplanted in line, and 27% applied fertiliser in the field, with the lowest N amount of 7.8 kg ha−1. In comparison to the other two clusters, these farmers had moderate land area under rice production (0.45 ha) and expended less cost. Average grain yield was the lowest for them at 3,761 kg ha−1. However, net income from rice growing in these farms was slightly higher at 5,510,000 UGX (ca. 1,560 USD) year−1; in addition to more income from off-farm activities at 1,060,000 UGX (ca. 300 USD). This cluster constituted 26 and 32%, respectively, of participating and non-participating farm households (Supplementary Table S2). Cluster 2 (farms with highest level of application of RAP) was the smallest cluster with 24% (n = 35) of the farms. In this group, 71% of the farmers used fertiliser in the nursery, 63% transplanted timely, 69% transplanted in line, and 94% applied fertiliser in the field, with the highest N application rate of 32.5 kg ha−1. These farmers also had the largest land area under rice production (0.60 ha) and the highest production cost. Average grain yield was the highest at 4,342 kg ha−1, however, with the lowest net income from rice production at 5,030,000 UGX (ca. 1,425 USD) year−1, but the highest income from other crops. The lower income from rice production could be attributed to the higher expenditure on labour. Overall, these farms have more diversified sources of income compared with the other clusters. Thirty percent of participating and 15% of non-participating farm households made up this cluster. Cluster 3 (farms with moderate application of RAP) was the largest cluster with 48% (n = 70) of the total farms studied. Of the farmers in this cluster, 98% used fertiliser in the nursery, 6% transplanted timely, 39% used line transplanting, and 96% applied fertiliser in the field, with average N amount of 26.0 kg ha−1. These farmers had slightly smaller land area under rice production (0.42 ha), with higher production cost. Grain yield was moderate in this cluster at 4,031 kg ha−1, and leading to a net income from rice production of 5,500,000 UGX (ca. 1,560 USD) year−1. Participating and non-participating farm households that made up the cluster were 44 and 53%, respectively (Supplementary Table S2).
This study showed that top-yielding farmers during experimentation still had the highest average yield after experimentation compared with the lower- and middle-yielding farmers during experimentation. Yet, the lower-yielding farmers made the highest yield gains (Figure 1). The higher yield gains by the lower-yielding farmers could be attributed to a significant improvement in management practices after experimentation. Generally lower-yielding farmers improved their weed management, and fertiliser use, amount and timing (Table 3; Figure 2). Even though lower-yielding farmers made larger yield gains, the overall yields recorded by farmers are still low, for the rice variety grown, when compared with yields observed under researcher-managed on-farm trials in the same study area (Awio et al., 2021). Grain yields recorded in this study are, however, higher than yields earlier reported under farmers' practice in the study area (Senthilkumar et al., 2020; Awio et al., 2022). The lack of yield gain by the top-yielding farmers might imply that at their current management level these farmers could not further raise their grain yields beyond the level observed during joint experimentation, probably because the observed current N input could be too low (which was up to a maximum of 68 kg N ha−1 after experimentation from 46 kg N ha−1 maximum rate during experimentation), in combination with lack of P and K application. It may therefore be necessary that farmers in this production system increase N rates, and P and K application be emphasised based on field inherent fertility to further raise grain yields as current farmers' fertilisation strategies do not put into consideration P and K application (Awio et al., 2022). This should be in addition to improved crop management practices, like proper timing of weeding and fertiliser application. Large yield gains have been reported in the same location under researcher management when N, P and K rates were increased from 80-40-40 to 100-50-50 kg ha−1 N, P and K, respectively (Awio et al., 2021). The results of our study are consistent with the findings of Ogada and Nyangena (2015) who observed higher yield gains, due to adoption of improved management practices, by farm households that had lower to medium grain yield than farm households at the upper end of the yield distribution. Shaibu et al. (2021) reported that the highest benefits from scaling up and adoption of improved management practices would be derived by low resource-endowed farm households. Similarly, Ainembabazi et al. (2018) showed that adoption of improved crop varieties would benefit poor farm households more than better-off households. However, in our present study there was no clear difference in resource endowment of lower, middle and top yielding farm households. Farmers who observed higher grain yield in their recommended agronomic practices (RAP) plot compared with their FP plot during experimentation realised larger yield gains than farmers who had lower yield under RAP compared with FP plot, an indication that the former farmers learnt something from the joint experimentation which they were able to apply in their fields and make some gains in grain yields. Franke et al. (2010) in on-farm trials found that farmers copied management practices from experimental treatments, in some cases competing in terms of yield with the researcher-managed plots. This observation could point to the broader influence on-farm experimentation can have on farmer's yield improvements the subsequent seasons, something we observe in the present study.
Participating farmers had a slightly but significantly higher grain yield and application of some of the improved rice management practices compared with non-participating farmers (Figure 5; Table 5). This shows the potential benefit of exposing farmers to RAP, through participatory learning, on boosting rice yields in Uganda and similar rice production systems in sub-Saharan Africa. The findings further underscore the point that participating farmers learnt something from the joint experimentation and were able to apply that in their fields during the subsequent seasons, resulting in higher grain yields. Similar observation was made by Senthilkumar et al. (2018) who showed improvements in the implementation of RAP for rice by farmers and subsequently increased grain yields after participatory on-farm trials with farmers. Krupnik et al. (2012) and Senthilkumar et al. (2018) noted that farmers learnt by doing to better implement the components of RAP during the course of time the participatory trials were conducted. Kondylis et al. (2017) observed that directly training farmers resulted in a large increase in adoption of sustainable land management practices among farmers. Joint experimentation with farmers can therefore be an interesting way of directly training farmers where learning by doing is facilitated. This farmer training combined with farmers' own experiences with recommended agronomic practices can be used as a tool in rice farming extension efforts to transform rice production, triggering a positive change in the participating farmers' crop management practices, grain yield and livelihoods (Senthilkumar et al., 2018). This, however, requires an enabling environment for rice farmers to increase their production through the adoption of RAP components, for instance, improved access to certified seeds of high-yielding varieties and fertilisers at affordable prices, access to locally adapted simple weeding tools, and fair access to rice markets among others. Joint experimentation can also provide better feedback to research and extension on innovations or innovation components that will not work under local farmers' conditions.
The results of our study indicate that distinguished farm types varied in adoption of improved management practices for rice and grain yield, but not in resource endowment or socio-economic characteristics (Table 6). This may imply that farmers in this production system have the capacity of reaching a higher yield level when improved management practices are applied. The lack of difference in resource endowment among farm types could suggest that wealth is not an important factor in adopting improved management practices for rice in the current production system and rice scheme. Our study finding, however, contrasts with previous studies which reported household wealth and other socio-economic parameters to be key in adoption of innovations. For instance, a study of Urfels et al. (2021) in tropical Asia showed that household resource endowment determined timing of rice planting, in addition to ecosystem and climatic factors. In SSA, Chekene and Chancellor (2015), Nakano et al. (2018) and Nonvide (2021) noted that farmers' education, age, farming experience and training on improved rice production practices were important in the adoption of improved rice production technologies among rice farmers. Similarly, Fosso and Nanfosso (2016), Hassan et al. (2016) and Lulseged et al. (2016) showed household wealth, off-farm employment, farm size, participation in on-farm trials, and farmers' education to be associated with adoption of improved management practices for maize, e.g., improved weed management, improved seeds, and use of fertiliser. Likewise, Dersseh et al. (2016), Tadesse et al. (2017), and Tadesse et al. (2019) observed that adoption of improved potato varieties and production practices was related to household wealth and educational levels. In the present study, however, these variables were not significantly different among the identified farm types, except farmer's gender. Difference among farm types in attending trainings related to rice farming would be significant at 0.10% probability.
This study indicates that joint experimentation had a larger effect on raising yields of originally lower-yielding farmers and narrowed the yield gap between lower- and higher-yielding farmers, thus bringing farmers closer in their management and outputs. Lower-yielding farmers made more gains compared with higher-yielding farmers, an indication that lower-yielding farmers had more room to raise their yields, as it seemed difficult for higher-yielding farmers to further increase their yields. Despite the larger yield gains by lower-yielding farmers, the overall yields observed by farmers in the study area are still rather limited when compared with researcher-managed yields previously reported on-farm in the same rice scheme. No difference in household resource endowment was observed amongst farm types which could imply that wealth is not a crucial element of adoption of available innovation in this production system, unless all households were limited in further innovating. Further studies aimed at understanding the limitations to why some farmers do not apply packages of RAP despite not being socio-economically different from those farmers who apply, may be relevant to identify appropriate solutions to such bottlenecks hence boosting also these farmers' rice productivity.
The data for this study are available upon reasonable request to the corresponding authors and under the condition that the identity of farmers involved can be protected.
Conceptualisation, methodology, and validation: TA and TJS. Data collection, formal analysis, and writing—original draft preparation: TA. Writing—reviewing and editing: TA, PCS, and TJS. All authors contributed to the article and approved the submitted version.
This research was funded by NUFFIC (grant number: NFP - PhD.17/0019) for which we are grateful.
The authors are thankful to the field enumerators Angella Lowra Ajam, Zebrone Gessa, and Joseph Benard Bukuyi for supporting in data collection. Thanks to Joost van Heerwaarden for discussions on the original design, Uta Priegnitz, Phuong Nguyen, and Zhan Xu for providing insights on appropriate data analysis procedures. Farmers are acknowledged for actively participating in the study.
The authors declare that the research was conducted in the absence of any commercial or financial relationships that could be construed as a potential conflict of interest.
All claims expressed in this article are solely those of the authors and do not necessarily represent those of their affiliated organizations, or those of the publisher, the editors and the reviewers. Any product that may be evaluated in this article, or claim that may be made by its manufacturer, is not guaranteed or endorsed by the publisher.
The Supplementary Material for this article can be found online at: https://www.frontiersin.org/articles/10.3389/fsufs.2022.1009469/full#supplementary-material
1. ^A district in Uganda is the local government administrative unit, divided into counties, sub-counties, parishes and villages.
Ainembabazi, J. H., Abdoulaye, T., Feleke, S., Alene, A., Dontsop-Nguezet, P. M., Ndayisaba, P. C., et al. (2018). Who benefits from which agricultural research-for-development technologies? Evidence from farm household poverty analysis in Central Africa. World Dev. 108, 28–46. doi: 10.1016/j.worlddev.2018.03.013
Awio, T., Senthilkumar, K., Dimkpa, C. O., Otim-Nape, G. W., Kempen, B., Struik, P. C., et al. (2021). Micro-nutrients in East African lowlands: are they needed to intensify rice production? Field Crops Res. 270, 108219. doi: 10.1016/j.fcr.2021.108219
Awio, T., Senthilkumar, K., Dimkpa, C. O., Otim-Nape, G. W., Struik, P. C., and Stomph, T. J. (2022). Yields and yield gaps in lowland rice systems and options to improve smallholder production. Agronomy 12, 552. doi: 10.3390/agronomy12030552
Banerjee, H., Goswami, R., Chakraborty, S., Dutta, S., Majumdar, K., Satyanarayana, T., et al. (2014). Understanding biophysical and socio-economic determinants of maize (Zea mays L.) yield variability in eastern India. NJAS: Wageningen J. Life Sci. 70, 79–93. doi: 10.1016/j.njas.2014.08.001
Chekene, M. B., and Chancellor, T. S. B. (2015). Factors affecting the adoption of improved rice varieties in Borno State, Nigeria. J. Agric. Ext. 19, 21–33. doi: 10.4314/jae.v19i2.2
Danlami A. H. Applanaidu S.-D. and Islam, R. (2019). A microlevel analysis of the adoption and efficiency of modern farm inputs use in rural areas of Kano state, Nigeria. Agric. Res. 8, 392–402. doi: 10.1007/s40003-018-0373-z
Danlami, A. H., Islam, R., Applanaidu, S. D., and Tsauni, A. M. (2016). An empirical analysis of fertiliser use intensity in rural Sub-Saharan Africa. Int. J. Soc. Econ. 43, 1400–1419. doi: 10.1108/IJSE-04-2015-0086
Dersseh, W. M., Gebresilase, Y. T., Schulte, R. P. O., and Struik, P. C. (2016). The analysis of potato farming systems in Chencha, Ethiopia: input, output and constraints. Am. J. Potato Res. 93, 436–447. doi: 10.1007/s12230-016-9521-9
Food Agriculture Organisation of the United Nations (FAO) (2021). Production Data: Crops and Livestock Products. Available online at: http://www.fao.org/faostat/en/#data/QC (accessed September 26, 2021).
Food Agriculture Organisation of the United Nations (FAO) (2022). Trade Data: Crops and Livestock Products. Available online at: https://www.fao.org/faostat/en/#data/TCL (accessed May 19, 2022).
Fosso, P. K., and Nanfosso, R. T. (2016). Adoption of agricultural innovations in risky environment: the case of corn producers in the west of Cameroon. Rev. Agric. Food Environ. Stud. 97, 51–62. doi: 10.1007/s41130-016-0008-3
Franke, A. C., Berkhout, E. D., Iwuafor, E. N. O., Nziguheba, G., Dercon, G., Vandeplas, I., et al. (2010). Does crop-livestock integration lead to improved crop production in the savanna of west Africa? Exp. Agric. 46, 439–455. doi: 10.1017/S0014479710000347
Giller, K. E., Tittonell, P., Rufino, M. C., van Wijk, M. T., Zingore, S., Mapfumo, P., et al. (2011). Communicating complexity: integrated assessment of trade-offs concerning soil fertility management within African farming systems to support innovation and development. Agric. Syst. 104, 191–203. doi: 10.1016/j.agsy.2010.07.002
Global Yield Gap Atlas (2022). Yield Gap for Sub-Saharan Africa. Available online at: https://www.yieldgap.org/gygaviewer/index.html (accessed July 29, 2022).
Hassan, M. B., Baiyegunhi, L. J. S., Ortmann, G. F., and Abdoulaye, T. (2016). Adoption of striga (Striga hermonthica) management technologies in Northern Nigeria. Agrekon 55, 168–188. doi: 10.1080/03031853.2016.1159587
Jahnke, H. E., Tacher, G., Keil, P., and Rojat, D. (1987). Livestock Production in Tropical Africa with Special Reference to the Tsetse-Affected Zone. Livestock Production in Tsetse Affected Areas of Africa. International Livestock Centre for Africa and International Laboratory for Research on Animal Diseases. Kenya: Nairobi, 496.
Kassie, M., Marenya, P., Tessema, Y., Jaleta, M., Zeng, D., Erenstein, O., et al. (2018). Measuring farm and market level economic impacts of improved maize production technologies in Ethiopia: evidence from panel data. J. Agric. Econ. 69, 76–95. doi: 10.1111/1477-9552.12221
Kassie, M., Shiferaw, B., and Muricho, G. (2011). Agricultural technology, crop income, and poverty alleviation in Uganda. World Dev. 39, 1784–1795. doi: 10.1016/j.worlddev.2011.04.023
KilimoTrust (2014). Expanding Rice Markets in the East African Community. Kampala: KilimoTrustEA, 64.
Kondylis, F., Mueller, V., and Zhu, J. (2017). Seeing is believing? Evidence from an extension network experiment. J. Dev. Econ. 125, 1–20. doi: 10.1596/27680
Krupnik, T. J., Shennan, C., Settle, W. H., Demont, M., Ndiaye, A. B., and Rodenburg, J. (2012). Improving irrigated rice production in the Senegal River Valley through experiential learning and innovation. Agric. Syst. 109, 101–112. doi: 10.1016/j.agsy.2012.01.008
Kuivanen, K. S., Alvarez, S., Michalscheck, M., Adjei-Nsiah, S., Descheemaeker, K., Mellon-Bedi, S., et al. (2016). Characterising the diversity of smallholder farming systems and their constraints and opportunities for innovation: a case study from the Northern Region, Ghana. NJAS - Wageningen J. Life Sci. 78, 153–166. doi: 10.1016/j.njas.2016.04.003
Lulseged, T., Powell, M., Gift, N., and Kihara, J. (2016). Assessment of maize yield gap and major determinant factors between smallholder farmers in the Dedza district of Malawi. Nutr. Cycling Agroecosyst. 105, 291–308. doi: 10.1007/s10705-015-9692-7
Manda, J., Alene, A. D., Tufa, A. H., Abdoulaye, T., Wossen, T., Chikoye, D., et al. (2019). The poverty impacts of improved cowpea varieties in Nigeria: a counterfactual analysis. World Dev. 122, 261–271. doi: 10.1016/j.worlddev.2019.05.027
Mottaleb, K. A. (2018). Perception and adoption of a new agricultural technology: evidence from a developing country. Technol. Soc. 55, 126–135. doi: 10.1016/j.techsoc.2018.07.007
Nakano, Y., Tanaka, Y., and Otsuka, K. (2018). Impact of training on the intensification of rice farming: evidence from rainfed areas in Tanzania. Agric. Econ. 49, 193–202. doi: 10.1111/agec.12408
Namyenya, A. (2014). Farmers' willingness to pay for irrigation water: the case of Doho rice irrigation scheme in eastern Uganda (MSc. Thesis), Makerere University, 90.
Nonvide, G. M. A. (2021). Adoption of agricultural technologies among rice farmers in Benin. Rev. Dev. Econ. 25, 2372–2390. doi: 10.1111/rode.12802
Ogada, M. J., and Nyangena, W. (2015). Impact of Improved Farm Technologies on Yields: The Case of Improved Maize Varieties and Inorganic Fertilizers in Kenya. International Conference of Agricultural Economists, Italy, 31.
Priegnitz, U., Lommen, W. J. M., Onakuse, S., and Struik, P. C. (2019). A Farm typology for adoption of innovations in potato production in Southwestern Uganda. Front. Sustain. Food Sys. 3, 68. doi: 10.3389/fsufs.2019.00068
Senthilkumar, K., Jonne, R., Ibnou, D., Elke, V., Fitta, S. S., Jean-Martial, J., et al. (2020). Quantifying rice yield gaps and their causes in Eastern and Southern Africa. J. Agron. Crop Sci. Special Issue 13, 1–13. doi: 10.1111/jac.12417
Senthilkumar, K., Tesha, B. J., Mghase, J., and Rodenburg, J. (2018). Increasing paddy yields and improving farm management: results from participatory experiments with good agricultural practices (GAP) in Tanzania. Paddy Water Environ. 16, 749–766. doi: 10.1007/s10333-018-0666-7
Shaibu, M. B., Azzarri, C., Bekele, H. K., Kornher, L., and von Braun, J. (2021). Scaling-up agricultural technologies: who should be targeted? Eur. Rev. Agric. Econ. 49, 857–875. doi: 10.1093/erae/jbab054
Sinyolo, S. (2020). Technology adoption and household food security among rural households in South Africa: the role of improved maize varieties. Technol. Soc. 60, 101214. doi: 10.1016/j.techsoc.2019.101214
Stuart, A. M., Pame, A. R. P., Silva, J. V., Dikitanan, R. C., Rutsaert, P., Malabayabas, A. J. B., et al. (2016). Yield gaps in rice-based farming systems: insights from local studies and prospects for future analysis. Field Crops Res. 194, 43–56. doi: 10.1016/j.fcr.2016.04.039
Tadesse, Y., Almekinders, C. J. M., Schulte, R. P. O., and Struik, P. C. (2017). Understanding farmers' potato production practices and use of improved varieties in Chencha, Ethiopia. J. Crop Improv. 31, 673–688. doi: 10.1080/15427528.2017.1345817
Tadesse, Y., Almekinders, C. J. M., Schulte, R. P. O., and Struik, P. C. (2019). Potatoes and livelihoods in Chencha, southern Ethiopia. NJAS: Wageningen J. Life Sci. 88, 105–111. doi: 10.1016/j.njas.2018.05.005
Takahashi, K., Muraoka, R., and Otsuka, K. (2020). Technology adoption, impact, and extension in developing countries' agriculture: a review of the recent literature. Agric. Econ. 51, 31–45. doi: 10.1111/agec.12539
Uganda Bureau of Statistics (UBOS) (2021). Statistical Abstract: 350. Available online at: http://www.ubos.org (accessed May 19, 2022).
Urfels, A., McDonald, A. J., van Halsema, G., Struik, P. C., Kumar, P., Malik, R. K., et al. (2021). Social-ecological analysis of timely rice planting in Eastern India. Agron. Sustain. Dev. 41, 14. doi: 10.1007/s13593-021-00668-1
Wanyama, J., Ssegane, H., Kisekka, I., Komakech, A. J., Banadda, N., Zziwa, A., et al. (2017). Irrigation development in Uganda: constraints, lessons learned, and future perspectives. J. Irrig. Drain Eng. 143, 10. doi: 10.1061/(ASCE)IR.1943-4774.0001159
Keywords: joint experimentation, participating farmers, non-participating farmers, recommended agronomic practices, Oryza sativa
Citation: Awio T, Struik PC and Stomph TJ (2022) Management practices and rice grain yield of farmers after participation in a joint experimentation. Front. Sustain. Food Syst. 6:1009469. doi: 10.3389/fsufs.2022.1009469
Received: 01 August 2022; Accepted: 25 August 2022;
Published: 21 September 2022.
Edited by:
Cosmas Parwada, Zimbabwe Open University, ZimbabweCopyright © 2022 Awio, Struik and Stomph. This is an open-access article distributed under the terms of the Creative Commons Attribution License (CC BY). The use, distribution or reproduction in other forums is permitted, provided the original author(s) and the copyright owner(s) are credited and that the original publication in this journal is cited, in accordance with accepted academic practice. No use, distribution or reproduction is permitted which does not comply with these terms.
*Correspondence: Thomas Awio, dGhvbWFzLmF3aW9Ad3VyLm5s; dGhvbWFzYXdpb0BnbWFpbC5jb20=; Tjeerd Jan Stomph, dGplZXJkamFuLnN0b21waEB3dXIubmw=
Disclaimer: All claims expressed in this article are solely those of the authors and do not necessarily represent those of their affiliated organizations, or those of the publisher, the editors and the reviewers. Any product that may be evaluated in this article or claim that may be made by its manufacturer is not guaranteed or endorsed by the publisher.
Research integrity at Frontiers
Learn more about the work of our research integrity team to safeguard the quality of each article we publish.