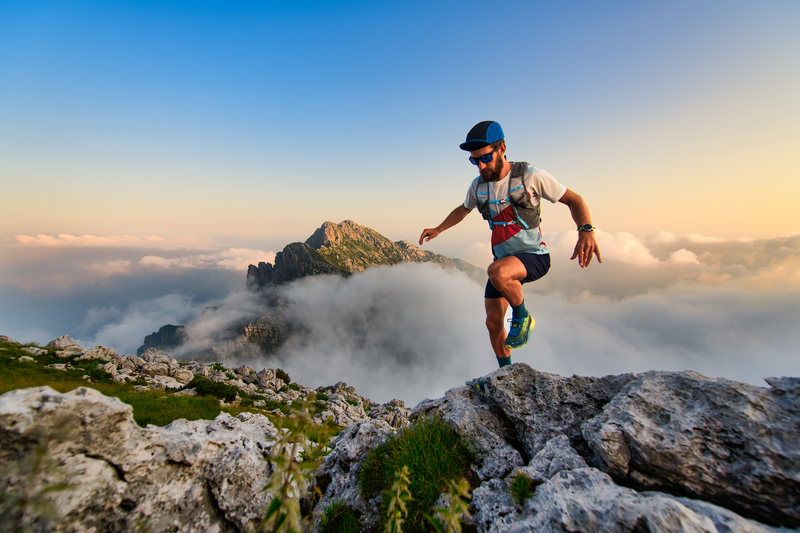
95% of researchers rate our articles as excellent or good
Learn more about the work of our research integrity team to safeguard the quality of each article we publish.
Find out more
SYSTEMATIC REVIEW article
Front. Sustain. Food Syst. , 25 October 2021
Sec. Land, Livelihoods and Food Security
Volume 5 - 2021 | https://doi.org/10.3389/fsufs.2021.727425
This article is part of the Research Topic Agile Data-Oriented Research Tools to Support Smallholder Farm System Transformation View all 17 articles
A systematic review of recent publications was conducted to assess the extent to which contemporary micro-level research on smallholders facilitates data re-use and knowledge synthesis. Following PRISMA standards for systematic review, 1,182 articles were identified (published between 2018 and 2020), and 261 articles were selected for review in full. The themes investigated were: (i) data management, including data source, variables collected, granularity, and availability of the data; (ii) the statistical methods used, including analytical approach and reproducibility; and (iii) the interpretation of results, including the scope and objectives of the study, development issues addressed, scale of recommendations made relative to the scale of the sample, and the audience for recommendations. It was observed that household surveys were the most common data source and tended to be representative at the local (community) level. There was little harmonization of the variables collected between studies. Over three quarters of the studies (77%) drew on data which was not in the public domain, 14% published newly open data, and 9% drew on datasets which were already open. Other than descriptive statistics, linear and logistic regression methods were the most common analytical method used (64% of articles). In the vast majority of those articles, regression was used as an explanatory tool, as opposed to a predictive tool. More than half of the articles (59%) made claims or recommendations which extended beyond the coverage of their datasets. In combination these two common practices may lead to erroneous understanding: the tendency to rely upon simple regressions to explain context-specific and complex associations; and the tendency to generalize beyond the remit of the data collected. We make four key recommendations: (1) increased data sharing and variable harmonization would enable data to be re-used between studies; (2) providing detailed meta-data on sampling frames and study-context would enable more powerful meta-analyses; (3) methodological openness and predictive modeling could help test the transferability of approaches; (4) more precise language in study conclusions could help decision makers understand the relevance of findings for policy planning. Following these practices could leverage greater benefits from the substantial investment already made in data collection on smallholder farms.
Growth in agricultural GDP has been shown to especially benefit the poorest members of society (Ligon and Sadoulet, 2008). Rural areas account for 54% of the world's population, but 79% of the total poor. Agricultural workers accounted for two thirds of the world's extreme poor. Smallholder farms (those of <2 hectares in size) make up 84% of the farms worldwide (Lowder et al., 2016). Agricultural development interventions are an important driver of poverty reduction and smallholders, the targets of these interventions, have even been labeled the “backbone” for implementing the SDGs (Terlau et al., 2019).
Rural development interventions aim to improve the quality of life for smallholders through increases in agricultural productivity, or other dimensions such as improved food security and human well-being. Agricultural research for development is essential for improving the delivery and targeting of development interventions. Traditionally, these interventions were tested in a controlled environment (Wigboldus et al., 2016). Once proven to be effective, they were then made available at a large scale. The uptake of interventions was generally driven by social networks and material incentives and the benefits of scaling exercises were usually assumed rather than measured. This scaling pattern can be thought of as linear, following three key stages: (1) “Find out what works”; (2) “Cross the divide through extension, transfer, diffusion and/or adoption”; (3) “Do more of the same.”
In recent years, there have been important changes to advice on how development interventions are designed, delivered, and studied. Research has shown that some demographic groups benefit more than others from particular interventions, both in terms of adoption and impact (Hammond et al., 2020). Researchers are now investigating how interventions can be tailored to work best for particular demographic groups, and how they can effectively “scale-up.” The PRactice-Oriented Multi-level perspective on Innovation and Scaling (PROMIS) calls for an iterative approach to scaling (Wigboldus et al., 2016). Instead of only testing interventions in a controlled environment, the PROMIS framework states that development practitioners should consider the impact of interventions as they become available to new demographic groups and in new locations. The PROMIS framework also calls for more research into the multiple scales at which we need to consider impact and determinants of adoption. For example, contextual factors, such as climate, local infrastructure, and access to markets can also influence the uptake and impact of an intervention. These multi-level interactions are rarely considered (van Wijk, 2014).
Understanding the dynamics of agricultural development processes for different demographic groups requires large amounts of household-level data. Synthesizing knowledge from this data is the key challenge we address in this manuscript. Traditionally, narrative reviews were used for knowledge synthesis, however these are often not useful for summarizing complex findings across a large numbers of studies (Gurevitch et al., 2018). Instead, meta-analyses are generally used where large numbers of studies exist across a range of contexts. These analyses come in two main forms: (1) aggregated meta-analyses, which examine results of multiple studies; (2) meta-analysis of individual participant data, which compiles the primary data of multiple studies for combined analysis (Eisenhauer, 2021). In aggregated meta-analysis, the focus is on study findings, the compatibility of the samples, and the methods used to generate these findings. In meta-analysis of individual participant data, considered a gold standard, information on the study design, data-sources, variable selection procedures, and heterogeneity between studies are all required (Riley et al., 2010). For either type of meta-analysis to be reliable, structured information on study design, the data-collected, the methods used to analyze these data, and a clear presentation of study findings, are all essential.
There are several practices in rural development research which make it difficult to conduct this type of collaborative data-driven research at multiple scales: rural development research is highly multi-disciplinary; the interventions evaluated, the metrics used to monitor impact, and the methods used for analysis are incredibly diverse (Carletto et al., 2013); high quality agricultural data, and particularly high-quality smallholder household surveys, are rare due to issues with recall and harmonization (Carletto et al., 2015; Fraval et al., 2019). Studies which took place across different times, locations, and scales require thorough contextual descriptions in order to understand the role of environmental and socio-economic context in influencing outcomes.
In recent years, there have been vast improvements in the availability of data, the efficiency of data-collection tools and the methods used to link and analyze these data. Micro-level research on smallholders, which has been hampered by a lack of data, can now capitalize on these advancements. There is a growing body of literature on data-driven methods to understand smallholder farms in relation to rural development objectives. Concurrently, best practice guidelines for data-intensive research have evolved and gained more prominence. In light of recent advances, we assess the degree to which recent micro-level research on smallholders is aligned to best practice principles, for example, the FAIR principles, the Turing Way, and the STROBE guidelines (von Elm et al., 2007; Wilkinson et al., 2016; The Turing Way Community et al., 2019). Finally, we identify gaps/opportunities where conforming to these best practices could increase the impact of micro-level smallholder research for development impact, and lead to a more collaborative research system centered around data re-use and generation of more useful, scalable insights.
The research question we address in this article is:
1. To what extent do current research practices facilitate the re-use of data and the synthesis of knowledge?
We identify three objectives in relation to this goal:
1. Review and characterize recent quantitative approaches which aim to understand smallholders and inform development practice.
2. Assess the degree to which best practices in data collection, data management, data analysis, and interpretation have been taken up in research on smallholder farmers.
3. Make recommendations for best practice which could be applied to add value to ongoing work in this field.
Our approach followed the PRISMA standards for a systematic review (Moher et al., 2009). The following steps were undertaken: (a) we identified a suitable database which can be used to identify potentially relevant articles; (b) we developed a search string to articles relevant to the research question; (c) we screened the articles based on title and abstract using clearly defined inclusion/exclusion criteria; (d) we used a set of predefined criteria to extract information from reviews of full articles.
We only examined academic research in this review. We considered Scopus, Web of Science, and Google Scholar as search engines to identify relevant articles. Scopus outperformed Web of Science in most disciplines (Martín-Martín et al., 2018), and while Google Scholar had the widest coverage, many of the articles were non-academic and contained few citations. Therefore Scopus was chosen as the most suitable database.
There is no widely accepted heuristic for determining sample size for this type of review. We considered three important points: (i) the review should represent the most contemporary research practice, (ii) the sample of articles should be sufficiently large to identify common practices, and (iii) it should be small enough to conduct the review in a realistic time frame. A similar systematic review, focusing on best practices in the biomedical literature, examined 149 articles published between 2015–2017 (Wallach et al., 2018). Like Wallach et al. (2018), we determined that examining research over the past two years would provide sufficient representation of the field, whilst not overly skewing results with the less up-to-date practices of older research efforts. Guidelines for best practices in data-intensive research have gained traction in recent years, and we wanted to review articles which had been published after some of these guidelines. Examples of such best practice guidelines include: the FAIR principles (Wilkinson et al., 2016); the Turing Way (The Turing Way Community et al., 2019); the OECDs recommendations for access to research data (OECD, 2021); the Transparency and Openness Promotion Guidelines (Nosek et al., 2015).
Based on this approach, a general search of all articles (2018–2020) containing “smallholder,” or any variants of it, was conducted. This resulted in 2,788 articles. To further focus the review, we narrowed the search to articles focused on rural development. To do this, the common keywords which featured in the 2,788 articles were analyzed. We identified the keywords aligning with rural development objectives (e.g., productivity, sustainability, climate change, poverty, and health) and used these to develop the final, and more targeted, search string. The final search string, and the keywords from these articles can be found in the Supplementary Materials.
Once all articles for review had been identified, the most relevant were selected through examination of their titles and abstracts, and comparison against a set of inclusion and exclusion criteria (Table 1). All these inclusion criteria had to be met and none of the exclusion criteria for an article to pass through to the next stage. The criteria were:
1. Only articles which included quantitative data were selected (i.e. purely qualitative studies were excluded, as this type of data is less suitable for re-use, harmonization, and meta-analysis across multiple studies);
2. Articles must use data which considers the heterogeneity of smallholders (i.e. the data should be sufficiently granular to allow identification of differences between smallholders within a single study);
3. At least one data source must be meaningful at the household level (i.e. fit the definition of micro-level research, or linking between the micro-level and other levels).
Table 1. The inclusion and exclusion criteria used to identify relevant articles during the title-abstract review and the review of full articles.
Selected articles were then reviewed in full. After reading the full article, each was re-assessed using the initial inclusion-exclusion criteria from the title abstract review to ensure they were indeed eligible. Then each article was evaluated using a further, predefined set of criteria. These additional, more detailed criteria were designed to evaluate whether the research was conducted in a manner to facilitate data re-use and knowledge synthesis. These criteria were informed by the requirements for an article to be suitable for meta-analysis, meaning an article must include information on: the study design; the data collected; the methods used to analyze these data; and the interpretation of findings. The criteria, and the options used for each criterion are presented in full in Appendix A.1, and summarized in Table 2. All analysis was conducted using the programming language (R Core Team, 2021).
The article selection and screening process is summarized in Figure 1. During the initial broad search 2,788 articles were identified which contained the word smallholder, or any variant of it. The search string was refined, using the steps outlined in Article identification. The refined search string was used to identify 1,182 remaining articles. During the title and abstract screening phase, 884 articles were excluded. Most of the articles which were excluded (60%) were excluded because they did not consider the differences between smallholders within the study (most looked at a single household variable only). A fifth of articles did not meet any of the inclusion criteria. While remote sensing can be used to add detailed information to studies, 72% of the articles which included remote sensing data had to be excluded because they did not combine it with data at the household level. The total number of articles thus selected for review was 298. During full review, a further 37 articles were excluded as on closer examination they did not use any quantitative data.
In total, 261 articles were reviewed in full, 49% of these were open access. A dataset containing the full list of articles reviewed, their associated meta-data, and how they were labeled can be found in the Supplementary Materials. The reviewed articles came from 127 journals. The 10 most common journals were: Sustainability (23 papers), Agricultural Systems (13 papers), World Development (13 papers), Food Security (12 papers), Journal of Rural Studies (12 papers), Land Use Policy (12 papers), PLoS ONE (8 papers), Climate and Development (7 papers), Agriculture, Ecosystems and Environment (6 papers), and Food Policy (6 papers). Many of the papers did not specify their funding sources. The three most frequently cited sources were USAID, the Bill and Melinda Gates Foundation, and the UK Department for International Development (DFID, now part of the Foreign, Commonwealth & Development Office). The articles reviewed studied a wide variety of locations, with the most frequently studied countries being Ethiopia (18%), Kenya (13%), Ghana (10%), Uganda (9%), Tanzania (8%), and Nigeria (5%).
The main development objectives addressed in the articles were climate change and adaptation (25%), agricultural productivity and efficiency (17%), and adoption and scaling of interventions (16%). Other topics which occurred less frequently included perceptions and decision making (13%), gender (12%), livelihood and food sourcing strategy (11%), nutrition (10%), food security (10%), health (10%), farm practices and management (10%), sustainability and environment (10%), vulnerability and resilience (7%), local infrastructure, laws, and services (5%), methodology (5%), and welfare and social issues (5%).
Table 3 summarizes data creation and management findings, showing that structured surveys were the main method of data collection, featuring in 95% of articles reviewed. Other data sources were generally used to supplement the findings from structured surveys. For example, qualitative data, including focus group discussions and open-ended interviews featured in 26% of the articles. Often, quotes from focus-group discussions were used to support statistical findings from the survey data. Other data sources appeared less frequently: remote sensing data was used in 10% of articles; aggregated statistics, such as averages at the county level were included in 7% of articles; crowd-sourced data sources, mobile-phone records and other sources of data appeared in only a few articles.
In addition to collecting household-level information, some studies combined this with data of a different granularity. For example, information such as household size and household income was considered to be at the household level, but assessment of the average age of people within a village could be considered to be at the “local/community” level. It was observed that data relevant at the landscape level was combined with household level data in 11% of articles, this included aggregated information on local physical geography or socioeconomic characteristics. Data which was relevant below the household level, such as information on individual fields, appeared in 9% of articles. Other levels of data, such as sub-national or national data, were rarely included in analyses.
The spatial coverage of the datasets ranged widely. Smaller-scale studies were much more common than larger-scale studies. Datasets with sub-national coverage (i.e., with samples across an entire county) featured in 48% of studies reviewed. Landscape-level studies, which drew on samples from a few clustered villages, featured in 31% of studies. National-level studies, with data covering the majority of subnational units, featured in 11% of the articles reviewed. International-level studies, which either focused on whole regions (e.g., East Africa), or were multi-continental, were extremely rare. In 8% of articles it was not possible to estimate the spatial coverage of the datasets. In most cases, articles were not explicit about the statistical representivity of their datasets. So, while their coverage may have been large, whether the findings were truly representative of the areas investigated was not clear.
None of the reviewed studies used more than one quantitative household-level dataset. The sample size of household-level datasets varied widely, the distribution of sample size is presented in Figure 2. Most studies had a sample size of <500 households. Figure 3 summarizes cumulative sample size in relation to the public availability of the underlying data. Larger-scale studies generally drew upon publicly available datasets. Cumulatively, smaller studies which do not share their data accounted for the majority of household-level data points (104,521 households). New data, which was collected for the study and made publicly available accounted for 26,737 household-level data points. Publicly available datasets (e.g., World Bank LSMS-ISA; Osabohien, 2018) accounted for 64,945 household-level data points in the studies reviewed, although it is doubtful that these are all unique datapoints as public data-sets tended to be re-used.
Figure 2. Histogram showing the distribution of sample size, for household level data, across the articles reviewed. Only articles with a sample size of <2,500 are presented. There were 16 articles with a sample size >2,500, ranging from 2,710 to 8,938.
Figure 3. A barchart showing the cumulative sample size of household-level datasets used by articles, disaggregated by data-sharing practice. Number of articles is represented by “n,” mean sample size represented by “m.s.s,” and percentage of total samples collected represented by “p.o.t”.
Regarding data sharing and documentation, 77% of articles did not provide ways to make the data accessible. Documentation on the data used was included in 15% of articles, this includes the articles which drew on publicly available resources, where documentation was already available. In 8% of cases, it was unclear whether documentation had been provided, this was in cases where the data was available “upon request.” In 2% of cases, articles shared their data and provided no documentation at all. Only 15% of articles explicitly mentioned the use of GPS coordinates to label their data spatially, facilitating linkage to other types of spatial datasets. Longitudinal (multiple time point) datasets were identified in only 14% of studies, even though many more articles were investigating processes which change over time, such as technology adoption or climate change adaptation.
The articles reviewed contained a wide range of household-level measures. Measures often differed in how they were recorded during data collection. For example, in a survey “household size” can be determined through a household roster, or simply by asking for the total number of people in the household. In this review, these would be classed as the same “measure,” recorded in two different ways. We identified 88 measures in total, summarized in Table 4. The most frequent measures used in the reviewed articles were education level of the household head (70%), land size (69%), household size (58%), and the gender of the household head (58%). Many measures did not appear very frequently. Despite differences in measures recorded, many articles collected information on a small number of common themes or topics. These themes are also summarized in Table 4. A total of nine themes were identified: household demographics (89% of articles contain at least one measure in this category), farm characteristics (87%), economic (79%), access to services and infrastructure (77%), farm management (62%), gender (61%), contextual features (33%), perceptions and knowledge (27%), and food security (16%).
The analysis methods used, and how these methods were used and shared, are summarized in Table 5 (further details in Supplementary Material). The analyses of the reviewed articles predominantly relied on descriptive statistics and single-level linear or logistic regression, which appeared in 93% and 64% of articles respectively. More advanced machine-learning methods occurred much less frequently.
Best practices for reproducibility were also investigated. Although scripted analysis software, such as the R programming language, python, or STATA were used in a third of the articles reviewed, analysis scripts or workflows were only shared in 3% of the articles reviewed and only 2% of articles provided documentation for their analysis scripts. Approximately half of the articles reviewed did not specify the software they used to conduct their analyses.
The use of linear and logistic regressions to understand smallholder heterogeneity is widespread, and as such, it warrants special attention. In the articles reviewed, these regression approaches were used to infer relationships between independent and outcome variables. For example, many technology adoption studies used linear regression to understand the relationship between technology adoption and household characteristics (e.g., age and years in education). Simple linear and logistic regressions were used in 64% of studies. More complex types of regression were used much more rarely. These included multi-level modeling, Bayesian regression methods, and partial least squares (PLS) regression. In total, 72% of articles used at least one type of regression method. In 71% of all articles, regression was used for association purposes. In these cases, the regression parameters, along with the appropriate significance tests or uncertainties, were used to infer the strength of association. In 2% of articles, a fitted regression model was used to make predictions. Here, data was split into training data and test data, with accuracy of the model assessed based on how accurately the trained model could predict values in the test set.
Table 6 summarizes how data and results were interpreted in the articles under review. The intended audience for the recommendations was primarily policy makers, who were addressed in 84% of studies. Recommendations for future research were also common, featuring in 54% of articles. Recommendations about specific farming practices featured in 20% of articles.
Table 6. A summary of the types and scale of conclusions drawn, and the intended audience for the recommendations.
Although there was a diverse range of recommendations proposed in these studies, they can broadly be conceptualized in two ways: creating an enabling environment (supra-household); and modification to household or household members' behavior (intra-household). These recommendation types were not mutually exclusive. Supra-household recommendations were made in 70% of articles. This was consistent across the different topics of the articles. These interventions included improvements to financial services, road quality, and information provision. Intra-household interventions were suggested by 36% of articles, which included changes in livelihood strategy, gendered decision making, and farmer-to-farmer information sharing. Other types of interventions, which were typically methodological improvements focused on researchers, were suggested by 15% of articles.
There was often a mismatch between the scale at which the recommendations were pitched vs. the scale at which the data was representative (Table 6). There were 208 articles where it was possible to determine the spatial coverage of the data, and the spatial coverage ascribed to the article's conclusions. Of these 208 articles, 59% drew conclusions with larger spatial coverage than their datasets. Over one third (38%) of articles drew general conclusions about smallholders in general, without reference to any specific locations or demographic groupings. Articles using local and subnational scale data more commonly drew “general” conclusions compared to articles using larger-scale datasets. Only one quarter explicitly confined their conclusions to the area covered by their datasets. In 26% of articles, conclusions were made at the national level, attributing their findings to all smallholders within the country studied. In 21% of articles, conclusions were drawn at the sub-national level (the largest administrative unit below the national level). In 11% of articles conclusions were made at the local level (any area below sub-national). At the larger scale, regional and global conclusions were much less common, occurring in 5 and 2% of articles respectively. Finally, only 3% of articles explicitly stated that their conclusions were relevant to areas with similar physical geography and socioeconomic characteristics. Table 7 compares the coverage of article data to the scale of the conclusions or recommendations made.
Table 7. A cross tabulation of the coverage of the data used in the article, compared to the scale of recommendations made in the articles reviewed.
While all articles used household level data in their analysis, some articles also included contextual information in the analysis, and many reported contextual information even if it was not used in analysis. This includes information on local infrastructure, climate, and markets. We define context to be information which is unique to each farming system due to its physical location. In 96% of articles a description of the study site was included. For 90% of articles, these descriptions were a mixture of text and tables. For 6% of the articles reviewed, contextual information was also included as Supplementary Material that could be easily downloaded and analyzed. Studies generally followed a pattern of describing the climate, physical geography, common farm systems and crops grown, and occasionally details about local infrastructure and markets. As discussed in harmonization of variables collected contextual information was rarely included in the formal analysis. For example, although climate and topography featured in almost every site description, they were only used in 17% and 7% of analyses, respectively. Most (70%) articles discussed the need for interventions which impact farm context (e.g., better infrastructure). Of these articles, only 33% of actually included information about farm context in their analysis.
Table 8 summarizes the main findings, showing that more articles tend to be located at the finer spatial scales, drawing on data which has local or subnational coverage. These smaller-scale datasets are rarely made open access. Descriptive statistics are used in almost all the smaller-scale articles, and single-level linear or logistic regressions are used in the majority. Smaller-scale studies in general make claims which extend beyond the coverage of their datasets in most cases. National and international-level studies which draw on household-level data are much rarer. For these larger-scale studies, publicly available datasets are used much more frequently. Where larger-scale new data is collected, it is more often shared. In the majority of cases, large-scale studies make claims which match the scale of their datasets. Across all scales, contextual information is used in less than half of the articles reviewed.
This review revealed that micro-level research on smallholders tends to be local in scope, data tends to be inaccessible for re-use, there is a narrow focus on specific analytical methodologies, and findings are difficult to generalize and re-use. These factors contribute to a rather fragmented body of knowledge. Admittedly, it is difficult to synthesize knowledge for policy use from the findings of many micro-level studies (Laborde et al., 2020). However, improvements could be made by improving data handling practices, broadening the suite of analytical approaches commonly used, and exploring more systematically the multi-level relationships between smallholders and their environmental and socio-economic context (van Wijk, 2014). We discuss below how the evidence from this review, the evidence from the literature, and the broader best practice guidelines can help inform the design of a more coherent research landscape, which facilitates continuous knowledge synthesis and systematic investigation of smallholder contexts. We explore the potential of meta-analysis (Gurevitch et al., 2018); and also discuss levers of behavioral change in this field of research. Finally, we discuss the limitations of this systematic review and how it limits the claims which we can make on these topics.
This review has shown that, in many instances, data in micro-level research on smallholders was not findable, accessible, interoperable, or re-useable (FAIR) (Wilkinson et al., 2016). Data was often not shared, and where it was shared it was scattered across a range of repositories. Data that had been shared was rarely documented. Datasets often did not collect GPS information, and there was poor harmonization of measures collected for each study, limiting the ability to link newly collected data with other datasets. All these findings limit the ability to re-use data for meta-analysis and knowledge synthesis. Three particular issues hamper the creation of FAIR data in micro-level research on smallholders: (1) the lack of standardization in household surveys, (2) non-standardized meta-data, and (3) variation in approaches to sampling.
The review has shown that household-level data often covered a similar range of topics (such as farm characteristics and access to resources), but variables or indicators were rarely harmonized between studies, making them incomparable. As 95% of the household-level datasets reviewed drew on structured surveys, we suggest that survey harmonization should be a top priority. Other domains facing similar challenges have pursued a modular approach to survey design. In a modular survey, a core set of questions is used to collect information common to many studies and optional modules are added to the survey to answer specific questions. This approach has been used by the UK's Office of National Statistics (ONS) to standardize household surveys, and the World Health Organization (WHO) to standardize health interview surveys (de Bruin et al., 1996; Smith, 2009).
For agricultural research, survey modules could be designed by the agricultural research community using community standards and ontologies. The CGIAR's working group on ontologies provide ontologies on a range of topics, including socioeconomics and agronomy (Arnaud et al., 2020). Digital data-collection tools, which can draw on a bank of standardized modules have an important role to play. There are several initiatives working toward standardized surveys on smallholders. The World Bank's LSMS-ISA (Osabohien, 2018), the Rural Household Multi-Indicator Survey (Hammond et al., 2017) and the CGIAR's 100Q initiative (van Wijk et al., 2019). The LSMS-ISA is a detailed survey, consisting of multiple rounds of data collection. The Rural Household Multi-Indicator Survey (RHoMIS) is a rapid survey, covering a range of topics using a lean-data approach. The 100Q initiative is a set of 100 questions, designed to accompany any smallholder household-level survey. This review has demonstrated that small-scale studies, with smaller sample sizes, make up the bulk of academic research on smallholders. As such, more agile tools which are less resource-intensive, like RHoMIS and the 100Q initiative, may be more equipped to deal with the challenges of small-scale research.
To enable meta-analysis and multi-level analysis, data standardization also needs to take place above the household level. A clear example of the importance of study context for meta-analysis is provided by Sibhatu and Qaim (2018). This meta-analysis examined the relationships between production diversity, diets, and nutrition for smallholders. It identified positive associations between production diversity and dietary diversity in some locations, and negative associations in others. The study used location and sample characteristics as variables in their meta-regression and found that these data were able to account for some of the differences between study findings.
This review showed that few studies provided meta-data in a standardized way. Meta-data generally came in the form of a site description, which included information on local climate, common crops grown, and common farming systems. The variables provided in site descriptions were rarely harmonized, and information was provided in various locations throughout the manuscript. It was observed that sampling procedures and statistical representativeness were not clearly documented in many of the studies reviewed. The common practices identified in this review, regarding meta-data and sampling, would not enable such meta-analysis to be conducted for most of the micro-level research on smallholders.
We recommend that researchers draw upon guidelines from other fields. The STROBE statement is particularly relevant for the reporting of observational studies (Field et al., 2014). This statement outlines what information on “setting” should be reported, including requirements for contextual information and sampling procedures. However, this checklist is generic, and the agricultural research community must still develop an approach which properly defines the context of a smallholder farm.
This review also identified several key issues relating to the analysis methodologies used in micro-level research on smallholders. Unclear and unpublished analysis methods made it difficult to replicate findings, or to apply the same methodology to a new location. Regression findings were generally presented in a tabular format with an R-squared and a p-value for each covariate. While useful for interpretability, this approach makes it difficult to test the power of a model in another location. This is particularly problematic considering that many articles were local in scale.
There are multiple ways researchers could improve the transferability of findings. By focusing on reproducibility, researchers can allow their methods to be tested in new locations. To begin with, researchers need to specify the software they are using to analyze their data. Where possible, scripted languages, such as R, Python, or Stata should be used to conduct analyses, and these scripts should be shared and usable. If using a graphical user interface (GUI) software, such as Excel, tools are available to facilitate reproducibility (The Turing Way Community et al., 2019). Publishing regression findings in a standardized way, and sharing these findings in a data repository, could facilitate meta-analysis. In the health sciences, there is a catalog of guidelines on reporting findings (Simera et al., 2010). For example, reporting only p-values limits how findings can be subsequently incorporated into a meta-analysis, where at least confidence intervals should be reported for analytical power. The STROBE guidelines provide information on how to present statistical findings using a range of methods (Field et al., 2014). The TRIPOD guidelines outline procedures to present prediction findings, including guidance on variable selection methods (Collins et al., 2015). Finally, publishing data sources used in the analysis would allow users to replicate previous findings, and test models on new datasets.
The review identified a clear preference for descriptive statistics and linear or logistic regression. A narrow focus on any particular analysis methods can limit study design and has an effect on the type of research questions which are asked (Carletto et al., 2015). A more diverse research landscape, with balanced use of methods can facilitate innovation (Petrescu and Krishen, 2019). For example, multi-level correlations between covariates should be modeled using random effects through multi-level modeling techniques, while repeated measurements could be handled through random effects, curve fitting or time series modeling as appropriate. The CERES2030 reviews demonstrate that rural development research must consider a diverse range of outcome variables, and the complex interactions between household level determinants (Laborde et al., 2020). The use of models which can balance multiple objectives is essential. Few of the studies examined in this review compared the utility of different modeling approaches. The STROBE checklist also requires information on model selection criteria.
Encouraging researchers to adhere to best practices will require some reorientation of research culture. Researchers are career driven, and often have high demands on their time. Research output is often measured using publication metrics, although this is changing. The data collected for a study and the methods used in analysis are also valuable contributions to science. Data in Brief, and Scientific Data are journals which provide mechanisms for research data to be cited when it is used. MethodsX is a journal which provides the opportunity for analysis methods to be cited also. Individual researchers aiming to capitalize on these initiatives should harness harmonization and standardization tools to ensure their data and methods are easy to re-use.
There are key levers of change which can be used to encourage adherence to best practices. Funding bodies, journals, and research organizations all have the power to influence research practices and encourage or enforce open research principles. The majority of peer-reviewed publications examined in this study did not share data or methods, indicating significant room for improvement. Reviewers should also consider how they evaluate a study's impact. A significant proportion of the articles reviewed in this study made claims beyond the scope of their data. Researchers are often required to argue for the impact or wide-ranging interest of their work, which is often linked to the spatial scale of their findings. We suggest that reviewers should consider whether claims of impact are supported by the data. Knowing exactly where findings apply, where they do not, and how research findings can be transferred to other contexts should be a key point of evaluation for reviewers.
This review highlighted key challenges in micro-level research on smallholder farmers. However, systematic reviews are inherently narrow in scope, focusing on specific research questions. This review examined only the most recent research to understand adherence to recent best practice guidelines. As such, research conducted prior to January 2018 has not been examined. Given that data-sharing and best-practices have grown in recent times, it is likely that the problems identified in this review are more prevalent in articles published prior to those reviewed.
This review also only examined academic research. This likely explains why common public datasets (such as the World Bank's LSMS-ISA) appeared relatively infrequently. Despite this, arguments for adherence to best practices still stand. While the World Bank and other sources of gray literature have shared their data, a significant proportion of rural development research takes place in smaller organizations which do not have the same requirements for data sharing and standardization. It is likely that the problems identified in this review also go far beyond academic research. Finally, this review aimed to examine broad issues in research, covering data acquisition, analysis methodologies, and interpretation of findings. Each of the issues covered in this broad review could benefit from further examination. In particular, investigation of the exact variables collected, and how variables differed between studies, would support the development of more useful ontologies. Further examination of how regression was used, how models were selected, and how findings were presented could support the design of specific reporting guidelines for micro-level smallholder research. Finally, a more specific focus on sample descriptions could help develop procedures and ontologies that describe sample context.
This review pointed to several issues which limit the potential for micro-level research on smallholders to generate coherent and widely applicable findings. The lack of harmonized metadata makes it difficult to compare the findings of two or more studies, for example through meta-analysis. The lack of harmonized microdata makes it difficult to conduct multi-level studies, comparing the impact of household level determinants and contextual determinants on key outcome metrics such as poverty and food security.
We propose that solutions to this entail following best practices in regards to: (a) data sharing, harmonization, and interoperability; (b) generation of more transferable findings by systematic description of study contexts and a more considered application of analysis methodologies; (c) a re-orientation in the culture of manuscript writing, whereby claiming unjustifiably wide spatial relevance is not valued, but instead contributing to knowledge synthesis is more highly valued. Particularly relevant are the FAIR principles. Central to the FAIR principles is the concept of actionability. Researchers must consider how their assets and findings are presented, prioritizing actionability. Parallel efforts in other research domains such as the health sciences could be of use. Building on such initiatives, like the STROBE and TRIPOD statements, the agricultural research community need to consider how their work can be presented in a way which can contribute to knowledge synthesis efforts. These steps would help leverage greater impact from the substantial investments already made in household level data-collection on smallholder farmers.
The original contributions presented in the study are included in the article/Supplementary Material, further inquiries can be directed to the corresponding author/s.
LG conducted the review and analyzed the results. LG designed the study with support from JH. LG wrote the manuscript with support from JH, AWD, and WJB. All authors discussed the results and contributed to the final manuscript.
This work was supported by an Alan Turing Institute PhD Studentship funded under EPSRC grant EP/N510129/1, and a University of Bristol Enhancing Knowledge Partnerships grant funded by the Quality-Related Global Challenges Research Fund allocation. JH and MW acknowledge the support of the CGIAR Research Program on Livestock and its donors. CW acknowledges funding via a EPSRC RSE Fellowship (EP/N018591/1).
The authors declare that the research was conducted in the absence of any commercial or financial relationships that could be construed as a potential conflict of interest.
All claims expressed in this article are solely those of the authors and do not necessarily represent those of their affiliated organizations, or those of the publisher, the editors and the reviewers. Any product that may be evaluated in this article, or claim that may be made by its manufacturer, is not guaranteed or endorsed by the publisher.
The authors would like to thank Kate Robson-Brown and the University of Bristol Jean Golding Institute for their support, and Levi J. Wolf and Maria Paula Escobar Tello for their comments on early drafts of this review.
The Supplementary Material for this article can be found online at: https://www.frontiersin.org/articles/10.3389/fsufs.2021.727425/full#supplementary-material
Arnaud, E., Laporte, M.-A., Kim, S., Aubert, C., Leonelli, S., Miro, B., et al. (2020). The ontologies community of practice: a CGIAR initiative for big data in agrifood systems. Patterns 1:100105. doi: 10.1016/j.patter.2020.100105
Carletto, C., Jolliffe, D., and Banerjee, R. (2015). From tragedy to renaissance: improving agricultural data for better policies. J. Dev. Stud. 51, 133–148. doi: 10.1080/00220388.2014.968140
Carletto, C., Zezza, A., and Banerjee, R. (2013). Towards better measurement of household food security: harmonizing indicators and the role of household surveys. Global Food Secur. 2, 30–40. doi: 10.1016/j.gfs.2012.11.006
Collins, G. S., Reitsma, J. B., Altman, D. G., and Moons, K. G. (2015). Transparent reporting of a multivariable prediction model for individual prognosis or diagnosis (TRIPOD): the TRIPOD Statement. BMC Med. 13:1. doi: 10.1186/s12916-014-0241-z
de Bruin, A., Picavet, H. S. J., and Nossikov, A. (1996). Health Interview Surveys: Towards International Harmonization of Methods and Instruments. WHO Regional Publications, European Series, No. 58. Copenhagen: Office of Publications, WHO Regional Office for Europe. Available online at: https://eric.ed.gov/?id=ED394961 (accessed June 3, 2021).
Eisenhauer, J. G. (2021). Meta-analysis and mega-analysis: a simple introduction. Teach. Stat. 43, 21–27. doi: 10.1111/test.12242
Field, N., Cohen, T., Struelens, M. J., Palm, D., Cookson, B., Glynn, J. R., et al. (2014). Strengthening the Reporting of Molecular Epidemiology for Infectious Diseases (STROME-ID): an extension of the STROBE statement. Lancet Infect. Dis. 14, 341–352. doi: 10.1016/S1473-3099(13)70324-4
Fraval, S., Hammond, J., Wichern, J., Oosting, S. J., De Boer, I. J. M., Teufel, N., et al. (2019). Making the most of imperfect data: a critical evaluation of standard information collected in farm household surveys. Exp. Agric. 55, 230–250. doi: 10.1017/S0014479718000388
Gurevitch, J., Koricheva, J., Nakagawa, S., and Stewart, G. (2018). Meta-analysis and the science of research synthesis. Nature 555, 175–182. doi: 10.1038/nature25753
Hammond, J., Fraval, S., van Etten, J., Suchini, J. G., Mercado, L., Pagella, T., et al. (2017). The Rural Household Multi-Indicator Survey (RHoMIS) for rapid characterisation of households to inform climate smart agriculture interventions: description and applications in East Africa and Central America. Agric. Syst. 151, 225–233. doi: 10.1016/j.agsy.2016.05.003
Hammond, J., Rosenblum, N., Breseman, D., Gorman, L., Manners, R., van Wijk, M. T., et al. (2020). Towards actionable farm typologies: scaling adoption of agricultural inputs in Rwanda. Agric. Syst. 183:102857. doi: 10.1016/j.agsy.2020.102857
Laborde, D., Murphy, S., Parent, M., Porciello, J., and Smaller, C. (2020). Ceres2030: Sustainable Solutions to End Hunger - Summary Report. Cornell University, IFPRI, and IISD. Available online at: https://ceres2030.org/wp-content/uploads/2021/03/ceres2030_en-summary-report.pdf (accessed June 2, 2021).
Ligon, E. A., and Sadoulet, E. (2008). Estimating the Effects of Aggregate Agricultural Growth on the Distribution of Expenditures. Washington, DC: World Bank Available online at: http://www.ssrn.com/abstract=1769944 (accessed April 21, 2021).
Lowder, S. K., Skoet, J., and Raney, T. (2016). The number, size, and distribution of farms, smallholder farms, and family farms worldwide. World Dev. 87, 16–29. doi: 10.1016/j.worlddev.2015.10.041
Martín-Martín, A., Orduna-Malea, E., Thelwall, M., and Delgado López-Cózar, E. (2018). Google scholar, web of science, and scopus: a systematic comparison of citations in 252 subject categories. J. Informetr. 12, 1160–1177. doi: 10.1016/j.joi.2018.09.002
Moher, D., Liberati, A., Tetzlaff, J., and Altman, D. G. (2009). Preferred reporting items for systematic reviews and meta-analyses: the PRISMA statement. BMJ 339:b2535. doi: 10.1136/bmj.b2535
Nosek, B. A., Alter, G., Banks, G. C., Borsboom, D., Bowman, S. D., Breckler, S. J., et al. (2015). Promoting an open research culture. Science 348, 1422–1425. doi: 10.1126/science.aab2374
OECD (2021). Recommendation of the Council concerning Access to Research Data from Public Funding. Available online at: https://legalinstruments.oecd.org/en/instruments/OECD-LEGAL-0347 (accessed August 12, 2021).
Osabohien, R. (2018). Contributing to agricultural mix:analysis of the living standard measurement study – Integrated survey on agriculture data set. Data Brief 20, 96–100. doi: 10.1016/j.dib.2018.07.057
Petrescu, M., and Krishen, A. S. (2019). Strength in diversity: methods and analytics. J. Market. Anal. 7, 203–204. doi: 10.1057/s41270-019-00064-5
R Core Team (2021). R: A Language and Environment for Statistical Computing. Vienna: R Foundation for Statistical Computing. Available online at: https://www.R-project.org/
Riley, R. D., Lambert, P. C., and Abo-Zaid, G. (2010). Meta-analysis of individual participant data: rationale, conduct, and reporting. BMJ 340:c221. doi: 10.1136/bmj.c221
Sibhatu, K. T., and Qaim, M. (2018). Review: meta-analysis of the association between production diversity, diets, and nutrition in smallholder farm households. Food Policy 77, 1–18. doi: 10.1016/j.foodpol.2018.04.013
Simera, I., Moher, D., Hoey, J., Schulz, K. F., and Altman, D. G. (2010). A catalogue of reporting guidelines for health research. Eur. J. Clin. Invest. 40, 35–53. doi: 10.1111/j.1365-2362.2009.02234.x
Smith, P. (2009). Survey Harmonisation in Official Household Surveys in the United Kingdom. Newport: National Statistics.
Terlau, W., Hirsch, D., and Blanke, M. (2019). Smallholder farmers as a backbone for the implementation of the sustainable development goals. Sustain. Dev. 27, 523–529. doi: 10.1002/sd.1907
The Turing Way Community Arnold, B, Bowler, L., Gibson, S., Herterich, P., and Higman, R. (2019). The Turing Way: A Handbook for Reproducible Data Science. Zenodo
van Wijk, M. T. (2014). From global economic modelling to household level analyses of food security and sustainability: how big is the gap and can we bridge it? Food Policy 49, 378–388. doi: 10.1016/j.foodpol.2014.10.003
van Wijk, M. T., van Álvarez, C., Anupama, G., Arnaud, E., Azzarri, C., Burra, D. D., et al. (2019). Towards a Core Approach for Cross-Sectional Farm Household Survey Data Collection: A Tiered Setup for Quantifying Key Farm and Livelihood Indicators. CGIAR Platform for Big Data in Agriculture. Available online at: https://cgspace.cgiar.org/handle/10568/105714 (accessed November 23, 2020).
von Elm, E., Altman, D. G., Egger, M., Pocock, S. J., Gøtzsche, P. C., Vandenbroucke, J. P., et al. (2007). The Strengthening the Reporting of Observational Studies in Epidemiology (STROBE) statement: guidelines for reporting observational studies. PLoS Med. 4:e296. doi: 10.1136/bmj.39335.541782.AD
Wallach, J. D., Boyack, K. W., and Ioannidis, J. P. A. (2018). Reproducible research practices, transparency, and open access data in the biomedical literature, 2015–2017. PLOS Biol. 16:e2006930. doi: 10.1371/journal.pbio.2006930
Wigboldus, S., Klerkx, L., Leeuwis, C., Schut, M., Muilerman, S., and Jochemsen, H. (2016). Systemic perspectives on scaling agricultural innovations. A review. Agron. Sustain. Dev. 36:46. doi: 10.1007/s13593-016-0380-z
Keywords: agricultural research, best practices, systematic review, quantitative research, smallholder agriculture
Citation: Gorman L, Browne WJ, Woods CJ, Eisler MC, van Wijk MT, Dowsey AW and Hammond J (2021) What's Stopping Knowledge Synthesis? A Systematic Review of Recent Practices in Research on Smallholder Diversity. Front. Sustain. Food Syst. 5:727425. doi: 10.3389/fsufs.2021.727425
Received: 18 June 2021; Accepted: 28 September 2021;
Published: 25 October 2021.
Edited by:
Christina Marie Kennedy, The Nature Conservancy, United StatesReviewed by:
Viswanathan Pozhamkandath Karthiayani, Amrita Vishwa Vidyapeetham, Kochi Campus, IndiaCopyright © 2021 Gorman, Browne, Woods, Eisler, van Wijk, Dowsey and Hammond. This is an open-access article distributed under the terms of the Creative Commons Attribution License (CC BY). The use, distribution or reproduction in other forums is permitted, provided the original author(s) and the copyright owner(s) are credited and that the original publication in this journal is cited, in accordance with accepted academic practice. No use, distribution or reproduction is permitted which does not comply with these terms.
*Correspondence: Léo Gorman, bGdvcm1hbkB0dXJpbmcuYWMudWs=
†These authors have contributed equally to this work and share last authorship
Disclaimer: All claims expressed in this article are solely those of the authors and do not necessarily represent those of their affiliated organizations, or those of the publisher, the editors and the reviewers. Any product that may be evaluated in this article or claim that may be made by its manufacturer is not guaranteed or endorsed by the publisher.
Research integrity at Frontiers
Learn more about the work of our research integrity team to safeguard the quality of each article we publish.