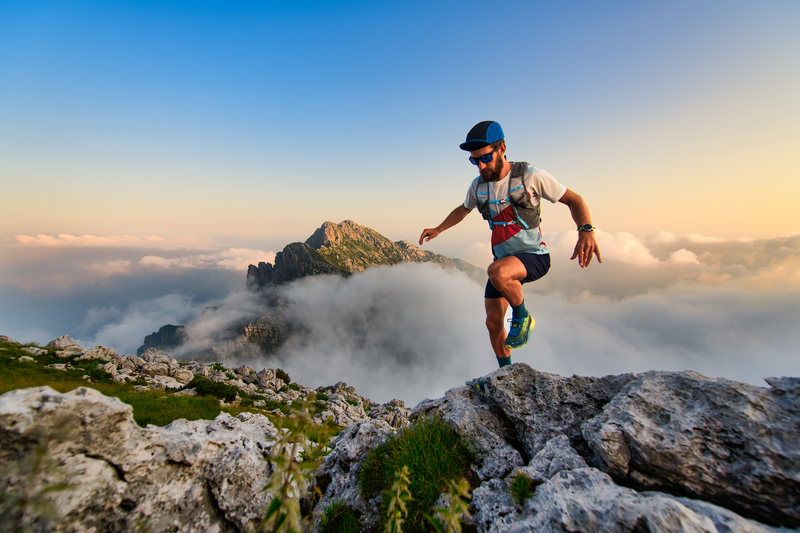
95% of researchers rate our articles as excellent or good
Learn more about the work of our research integrity team to safeguard the quality of each article we publish.
Find out more
ORIGINAL RESEARCH article
Front. Sustain. Food Syst. , 06 July 2021
Sec. Water-Smart Food Production
Volume 5 - 2021 | https://doi.org/10.3389/fsufs.2021.684808
This article is part of the Research Topic Water-Smart Agricultural Production Technologies Under a Changing Climate View all 4 articles
Farmers routinely determine irrigation requirements from visual observations and cultivation experience, but this can lead to under- or over-irrigation. To establish precise irrigation technology for strawberry cultivation, the average daily evapotranspiration and water requirements were estimated according to the environmental data: air temperature and humidity from the center of the greenhouses and solar radiation from outside greenhouses. Makkink FAO24 equations (temperature and cloudiness) were used to estimate the evapotranspiration and water requirements. The temperature equation showed higher correlation coefficients in solar radiation (R2 = 0.60), evapotranspiration (R2 = 0.76), and water requirements (R2 = 0.69) than other tested equations. The daily irrigation, calculated from the estimated evapotranspiration, was 3.8 tons/10a. It is possible to develop a precision irrigation system from estimated evapotranspiration during the winter cultivation of “Seolhyang” strawberries in South Korea.
Agriculture is the biggest global consumer of freshwater, accounting for up to 70% of the total use (FAO, 2016). Freshwater shortages pose a serious threat to sustainable development and food security worldwide. Conservation and efficient use of freshwater in agriculture is necessary to increase crop production while preventing water deficiency (Tsang and Jim, 2016). Rainfall and irrigation are important freshwater sources in agriculture. However, rainfall is unreliable and can cause deleterious effects, both in excess, through surface runoff and erosion, and in deficiency, resulting in drought. In contrast, irrigation is controllable and can be used as required. Thus, if rainfall is not secured and irregular, a well-controlled irrigation system should be used for crop cultivation instead of rainfall.
Irrigation systems are important in agricultural farms. A controlled amount of freshwater required for crop growth and development is artificially provided to the soil (Shibusawa, 2001; Oborkhale et al., 2015). In farms, using conventional irrigation systems, farmers might provide irrigation, without consideration of the exact freshwater needs of individual crops or environmental data. To avoid loss of crop productivity due to water stress (under-irrigation), farmers provide more freshwater than needed (over-irrigation), decreasing productivity, and wasting freshwater and energy. Therefore, conventional irrigation systems may cause undesired water stress on the crops or reduce water-saving capabilities (Kumar et al., 2017; Lakhiar et al., 2018; Say et al., 2018).
Most commercially available irrigation controllers are programmed for predefined intervals to provide an offline irrigation schedule based on soil and plant characteristics and on empirical knowledge of climate variable dynamics (Lozoya et al., 2014). A high level of precision is needed in irrigation systems to optimize the freshwater input and crop productivity and to minimize adverse environmental impacts. Precision irrigation has been conceptualized as the use of efficient irrigation systems or as the variable control of irrigation systems based on sensor feedback or predefined maps (Raine et al., 2007). The advantages of precision irrigation include improved crop yield and quality, higher water use efficiency, and reduction of energy costs and adverse environmental impacts (Shah and Das, 2012). Precision irrigation can improve agricultural sustainability in terms of improved water use efficiency and environmental quality (Pierce, 2010), by using freshwater more efficiently and effectively, avoiding under- and over-irrigation. Furthermore, variability within a farm may be managed, and a high economic benefit achieved by satisfying the specific irrigation needs of individual crops (Smith and Baillie, 2009; Cambra et al., 2018; El Chami et al., 2019).
However, the establishment of a precision irrigation system depends on accurate evapotranspiration forecast (Yang et al., 2016). And accurate meteorological data are needed. Reference evapotranspiration (ET0) is an expression of the effect of meteorological conditions on the water requirements of crops (Wang et al., 2008). The accurate estimation of ET0 is very important, not only for irrigation guidance and freshwater utilization improving, but also for the environmental effect and global climate change research (Zhang et al., 2012; Fan et al., 2016). An ideal model for ET0 estimation is designed based on minimal data, without affecting the accuracy of the estimation (Shih, 1984; Feng et al., 2017). The Makkink FAO (Food and Agriculture Organization) 24 equation is a simplified empirical model that requires less parameters than other empirical models (Makkink, 1957). Valipour et al. (2020), however, reported that ET0 have been evaluated by data from meteorological offices and thus there may be problems in predicting ET0 for crops within specific conditions containing greenhouses. We used data from environmental sensors and data loggers installed in a greenhouse. The objective of this study is to evaluate and predict the daily average evapotranspiration and water requirements of strawberry with data from a meteorological office and a greenhouse using the Makkink FAO24 equation.
Seolhyang strawberries, a major cultivar in South Korea, were transplanted in a greenhouse (35°42′N, 128°19′E, double-layer plastic houses, PE film, width: 8 m, length: 10 m, height: 3 m) at a density of 10.3 plants/m2 on September 07, 2019 (Figure 1). Eight thousand and five hundred plants were used for evapotranspiration estimation and water requirement analysis. During the experiment period, old leaves were defoliation once a week according to the conventional strawberry management method, and inflorescence and fruit were not removed. Flowers were pollinated by pollinators (honey bees). Water and nutrient solutions were supplied once a week, respectively. Culture solutions of strawberries were made according to the standard recipe, the EC of the supplied nutrient solution was 0.8 ds·m−1 and pH was maintained in the range 5.8–6.0. Environmental data was collected by installing air temperature and humidity sensors (Eyesne5, Nare Trends Inc., Sejong, South Korea) at the central point inside the greenhouse, and solar radiation sensors (WSS 202, Woosung hitech Co. Ltd., Yangsan, South Korea) outside the greenhouse (actual radiation). All data were recorded by data loggers (Nare Trends Inc., Sejong, South Korea). Data were collected from November 17, 2019 to April 28, 2020. Forecast data that was not collected at the site, such as the maximum and minimum air temperature, average atmospheric pressure, average relative humidity, day length, and elevation, but was obtained from a regional meteorological office.
Figure 1. A photograph of the experiment: 8,500 plants of “Seolhyang” strawberry plants were grown for the experiment in Goryeong commercial greenhouse (A) outside and (B) inside.
Daily solar radiation was predicted by estimation equations of temperature and cloudiness methods, according to Hargreaves and Samani (1982) (Equation 1) and Allen et al. (1998) (Equation 2), respectively. The average air temperature data from the past 10 years (2009–2019) from a regional meteorological office were used to estimate the solar radiation and to predict the water requirements.
The modified solar radiation coefficient (Equation 3) and estimated external solar radiation (Equation 4) were calculated from those data, respectively.
* Tmax, Maximum temperature; Tmin, Minimum temperature; τ, Transmittance of plastic film (polyethylene, PE) for the greenhouse (88.2%); z, Altitude above sea level (20 m); n, Number of days in the year.
The evapotranspiration of the plants was estimated from the solar radiation, transmittance of the plastic material, air temperature, and relative humidity data. De Bruin (1987) introduced the simplified version of the Makkink model (Makkink, 1957), which provides a reliable estimate of ET0 using only daily solar radiation (RS) and t observations. Following this approach, daily ET0 can be computed from the following (Equation 5):
* ETo, evapotranspiration; b, Adjustment factor (FAO crop water requirement); γ (kPa), Dry and wet constant = r = 0.665 × p×10−3; p(kPa), atmospheric pressure; Δ (kPa/°C), Slope of saturated water vapor pressure curve.
Where ET0 is the reference evapotranspiration (mm/d), RS is solar radiation (MJ m−2 d), λ is the latent heat of vaporization (MJ kg−1) and CMAK is an empirical coefficient depending on climate conditions. De Bruin (1987) suggested a value of 0.65 for the CMAK.
Daily forecasting of crop evapotranspiration (ETc) was determined using the modified FAO56 method (Allen et al., 1998; Equation 6):
* ETc, Crop water requirement; Kc, Crop coefficient (0.15).
The irrigation requirement was determined from the average daily crop water requirement, as follows (Equation 7):
The following parameters were calculated (Willmott, 1982): mean bias error (MBE) and mean absolute error, root mean square error (RMSE), relative error (RE), and the ratio between both average values. The correlation analysis and validation were calculated using R version 4.0.2 (RStudio Inc., USA). The ET and ETC values estimated from the predicted values were verified through the ratio of the predicted value to the measured value, R2, RMSE, RE, and MBE. The values calculated in the estimation formula were expressed as graphs using SigmaPlot (Systat, San Jose, CA, USA).
The air temperature and relative humidity (Figure 2A) and the accumulated radiation and vapor pressure deficit (Figure 2B) in the greenhouse from September 01, 2019 to May 01, 2020 showed opposing trends; the air temperature and the accumulated radiation decreased and then increased, whereas the relative humidity and the vapor pressure deficit increased and then decreased. The air temperature (Figure 2C) and the accumulated radiation (Figure 2D) in a regional meteorological office during the same period displayed the same trend as the data in the greenhouse but the relative humidity (Figure 2C) and the vapor pressure deficit (Figure 2D) did not reveal a trend due to variations in the data. The average air temperature, relative humidity, accumulated radiation, and vapor pressure deficit data from the greenhouse were 15.07°C, 77.76%, 10055.05 W/m2, and 0.77 kPa, respectively. The environment data from a regional meteorological office were 8.23°C, 62.82%, 10055.05 W/m2, and 0.25 kPa, respectively. The results indicated that the average air temperature, relative humidity, and vapor pressure deficit data from the greenhouse were higher than those from a regional meteorological office except accumulated radiation.
Figure 2. Environmental data collected from the experimental greenhouse between September 2019 and May 2020: Daily average air temperature and relative humidity from a greenhouse (A) and a regional meteorological office (C); Accumulated radiation and vapor pressure deficit from a greenhouse (B) and a regional meteorological office (D).
The actual daily solar radiation was 35.42 MJ m−2d.The estimated daily solar radiation from the temperature and cloudiness equations using the collected data were 35.05 (R2 = 0.60) and 40.06 (R2 = 0.42) MJ/m2d, respectively while the estimated daily solar radiation from the temperature and cloudiness equations using the forecasted data were 33.76 (R2 = 0.41) and 40.06 (R2 = 0.42) MJ/m2d, respectively (Figure 3). The RMSE and RE were lowest in the temperature equation using collected data and highest in the cloudiness equation using collected data.
Figure 3. Comparison of solar radiation among predicted values during the experimental period. (A) collected temperature; (B) collected cloudiness; (C) forecasted temperature; (D) forecasted cloudiness.
The daily evapotranspiration, estimated by the Makkink FAO24 equation and the actual daily solar radiation, was 6.36 mm/d. The estimated daily evapotranspiration from the temperature and cloudiness equations using the collected data were 6.32 (R2 = 0.76) and 7.57 mm/d (R2 = 0.33), respectively while the estimated daily evapotranspiration from the temperature and cloudiness equations using the forecasted data were 5.86 (R2 = 0.22) and 7.44 (R2 = 0.35) MJ/m2d, respectively (Figure 4). The RMSE and RE were lowest in the temperature equation using collected data and highest in the cloudiness equation using collected data.
Figure 4. Comparison of evapotranspiration among predicted values during the experimental period. (A) collected temperature; (B) collected cloudiness; (C) forecasted temperature; (D) forecasted cloudiness.
When the water requirements of strawberry plants provided by the FAO were applied to the actual and estimated solar radiation using temperature and cloudiness equations, the ratio of the estimated water requirement (1.04) in the temperature equation using the collected data was closest to 1.0 compared to the other equations (the cloudiness equations using the collected data: 1.20; the temperature equation using the forecasted data: 0.94; the cloudiness equation using the forecasted data: 1.18) and the correlation coefficient was highest (R2 = 0.69; Figure 5). Furthermore, the estimated water requirements from the temperature equation using collected data displayed lower errors than other water requirements. Thus, daily irrigation was calculated by the temperature equation using collected data. The requirement for daily irrigation was from 3.8 tons/10a (The daily irrigation = cultivation area × daily average estimated water requirement).
Figure 5. Comparison of water requirement among predicted values during the experimental period. (A) collected temperature; (B) collected cloudiness; (C) forecasted temperature; (D) forecasted cloudiness.
The air temperature and relative humidity and the accumulated radiation and vapor pressure deficit (Figure 2) in a greenhouse and a regional meteorological office is very common in South Korea. The difference between collected and forecasted values may be due to the difference of average air temperature, relative humidity, and vapor pressure deficit.
Most research on the impact of climate change on agriculture has focused on crop productivity (Cammarano et al., 2020, Schmidhubber and Tubiello, 2007, Tubiello et al., 2007). Improving resource efficiency in agricultural systems is crucial for sufficient food production. Remote sensing techniques, data collection, and information technology are key strategies for efficient agricultural water management (Ferrández-Pastor et al., 2018). For example, the efficient use of water, energy, and fertilizers through the Internet of Things improves yield while reducing production costs (Harun et al., 2019). However, climate-induced fluctuations in temperature, solar radiation, and precipitation not only affect crop productivity but also impact water use. The effect of changing weather patterns on the temporal and spatial variation of water requirements, consumption, and deficit has been investigated; however, most studies on strawberry irrigation have been conducted in California and Florida, where open-field cultivation is standard (Clark et al., 1996, Grattan et al., 1998, Hanson and Bendixen, 2004, Trout and Gartung, 2004). In Europe, strawberries are grown in greenhouses (Gavilán et al., 2015), similar to the experimental environment in this study; therefore, an equation was used that did not consider precipitation as a factor.
There are a number of equations that compute evapotranspiration using environmental factors, such as the Penman-Monteith, Priestley-Taylor, Turc, Hargreaves, Makkink, Blaney-Criddle, Abtew, and EnkuTemperature methods. The Makkink FAO24 equation uses solar radiation and air temperature, without precipitation; thus, it is suitable for experiments conducted in greenhouses that are not affected by precipitation. Gavilán et al. (2015) used the Makkink FAO24 equation to estimate the solar radiation and evapotranspiration of strawberries in greenhouses. The collected and forecasted values using the temperature and cloudiness equations had high correlation (R2 > 0.8); however, in this study, high correlations were not obtained due to high errors, although the ratio was ~1. The initial values of predicted solar radiation, evapotranspiration, and water requirements were high, which may increase RMSE and RE, and decrease R2 (Figures 3–5). Irrigation scheduling, based on the crop coefficient (Kc), is simple because sophisticated instruments are not required. Precise Kc values are often difficult to establish due to regional and site-specific crop physiology, soil characteristics, and cultural practices. Recommended Kc values for a regional irrigation scheduling program must be high enough to avoid water stress, yet low enough for efficient water management (Yuan et al., 2004). The Kc for the water requirement is applied differently to the equation depending on the growth stage (initial stage, 0.30; middle stage, 0.80; end stage, 0.70). However, these values correspond to strawberry cultivation in open fields and must be applied differently to greenhouse-cultivated crops. Bhantana et al. (2019) reported Kc values of 0.15, 1.18, and 0.25 in early, middle, and end stage strawberries in greenhouses of Denmark from March to June, respectively. The criterion for classifying growth stage is ambiguous. Therefore, a Kc of 0.15 was applied to the equation in this study regardless of the growth stage considering the lower air temperature than the study of Bhantana et al. (2019).
The correlation coefficients of the water requirements, estimated values from temperature and cloudiness equations using the forecasted data, were <0.50. This was presumed to be due to the microclimate and differences between the sensors. Meteorological agency data is convenient for determining the water requirements of agricultural areas at a regional or global level; therefore, strategies to improve this need to be established. For example, an analysis of differences in data according to sensor manufacturer, correction of the equation considering the microclimate, and setting the irrigation schedule considering soil moisture content.
The temperature equation using collected data gave a water requirement estimation of as much as the actual data. When the amount of irrigation is calculated using the estimated water requirement, 3.8 tons/10a strawberry farms is needed; this is similar to the actual water requirements. These results indicate that the evapotranspiration and water requirement values, determined using the Makkink FAO24 equation, will be suitable for developing a precise irrigation system during the cultivation of “Seolhyang” strawberries. This system should be updated to improve the coefficient for more accurate prediction of crop water requirements. Moreover, if environmental sensors and data loggers are not installed in a greenhouse, data from a regional meteorological office should be used for water requirement estimation. However, the results indicated that data from a regional meteorological office are less predictive than those from a greenhouse. To use forecasted data, new equations using data from a regional meteorological office should be designed.
The raw data supporting the conclusions of this article will be made available by the authors, without undue reservation.
WJ, DK, and SK designed the study. HS, SA, and HL did most of the data retrieval. YM and UW did the statistical analyses. WJ and DK wrote the first draft of the manuscript. SK did review and editing supervision. All authors contributed to the final draft.
This research was supported by Kyungpook National University Research Fund, 2019.
The authors declare that the research was conducted in the absence of any commercial or financial relationships that could be construed as a potential conflict of interest.
Allen, R. G., Pereira, L. S., Raes, D., and Smith, M. (1998). Crop Evapotranspiration: Guidelines for Computing Crop Water Requirements. Rome: FAO.
Bhantana, P., Poudel, K., and Petersen, K. K. (2019). Yield, evapotranspiration and quality of greenhouse grown strawberry (Fragaria × Ananassa Duch.) under combined drought and K2SO4 fertigation. Peer Res. Nest. 1, 1–10.
Cambra, C., Sendra, S., Lloret, J., and Lacuesta, R. (2018). Smart system for bicarbonate control in irrigation for hydroponic precision farming. Sensors 18:1333. doi: 10.3390/s18051333
Cammarano, D., Valdivia, R. O., Beletse, Y. G., Durand, W., Crespo, O., Tesfuhuney, W. A., et al. (2020). Integrated assessment of climate change impacts on crop productivity and income of commercial maize farms in northeast South Africa. Food Secur. 12, 659–678. doi: 10.1007/s12571-020-01023-0
Clark, G. A., Albregts, E. E., Stanley, C. D., Smajstrla, A. G., and Zazueta, F. S. (1996). Water requirements and crop coefficients of drip-irrigated strawberry plants. Trans. ASAE 39, 905–913. doi: 10.13031/2013.27575
De Bruin, H. A. R. (1987). “From Penman to Makkink,” in Evaporationand Weather, Proceedings and Informations (The Hague: Committee for Hydrological Research TNO), 5–31.
El Chami, D., Knox, J. W., Daccache, A., and Weatherhead, E. K. (2019). Assessing the financial and environmental impacts of precision irrigation in a humid climate. Hortic. Sci. 46, 43–52. doi: 10.17221/116/2017-HORTSCI
Fan, J., Wu, L., Zhang, F., Xiang, Y., and Zheng, J. (2016). Climate change effects on reference crop evapotranspiration across different climatic zones of China during 1956-2015. J. Hydrol. 542, 923–937. doi: 10.1016/j.jhydrol,.2016.09.060
FAO (2016). AQUA STAT: Water Uses. AQUASTAT - FAO's Global Information System on Water and Agriculture. Available online at: http://www.fao.org/nr/water/aquastat/water_use (accessed January 5, 2019).
Feng, Y., Jia, Y., Cui, N. B., Zhao, L., Li, C., and Gong, D. Z. (2017). Calibration of Hargreaves model for reference evapotranspiration estimation in Sichuan basin of southwest China. Agric. Water Manage. 181, 1–9. doi: 10.1016/j.agwat.2016.11.010
Ferrández-Pastor, F. J., García-Chamizo, J. M., Nieto-Hidalgo, M., and Mora-Martínez, J. (2018). Precision agriculture design method using a distributed computing architecture on Internet of Things context. Sensors 18:1731. doi: 10.3390/s18061731
Gavilán, P., Ruiz, N., and Lozano, D. (2015). Daily forecasting of reference and strawberry crop evapotranspiration in greenhouses in a Mediterranean climate based on solar radiation estimates. Agric. Water Manage. 159, 307–317. doi: 10.1016/j.agwat.2015.06.012
Grattan, S. R., Bowers, W., Dong, A., Snyder, R. L., Carrol, J. J., and George, W. (1998). New crop coefficients estimate water use of vegetables, row crops. Cal. Agric. 52, 16–21. doi: 10.3733/ca.v052n01p16
Hanson, B., and Bendixen, W. (2004). Drip irrigation evaluated in Santa Maria Valley strawberries. Cal. Agric. 58, 48–53. doi: 10.3733/ca.v058n01p48
Hargreaves, G. H., and Samani, Z. A. (1982). Estimating potential evapotranspiration. J. Irrig. Drain. Div. 108, 225–230. doi: 10.1061/JRCEA4.0001390
Harun, A. N., Mohamed, N., Ahmad, R., Rahim, A. R. A., and Ani, N. N. (2019). Improved Internet of Things (IoT) monitoring system for growth optimization of Brassica chinensis. Comput. Electron. Agric. 164:104836. doi: 10.1016/j.compag.2019.05.045
Kumar, V. V., Ramasamy, R., Janarthanan, S., and Babu, M. V. (2017). Implementation of IoT in smart irrigation system using arduino processor. Int. J. Civil Eng. Technol. 8, 1304–1314. Available online at: https://www.iaeme.com/ijciet/issues.asp
Lakhiar, I. A., Jianmin, G., Syed, T. N., Chandio, F. A., Buttar, N. A., and Qureshi, W. A. (2018). Monitoring and control systems in agriculture using intelligent sensor techniques: a review of the aeroponic system. J. Sens. 2018:8672769. doi: 10.1155/2018/8672769
Lozoya, C., Mendoza, C., Mej, L., Mendoza, G., Bustillos, M., Arras, O., et al. (2014). “Model predictive control for closed-loop irrigation,” in Preprints of the 19th World Congress The International Federation of Automatic Control (Cape Town), 4429–4434. doi: 10.3182/20140824-6-ZA-1003.02067
Makkink, G. F. (1957). Testing the Penman formula by means of lysimeters. J. Inst. Water Eng. 11, 277–288.
Oborkhale, L., Abioye, A. E., Egonwa, B. O., and Olalekan, T. A. (2015). Design and implementation of automatic irrigation control system. IOSR J. Comput. Eng. 17, 99–111.
Pierce, F. J. (2010). Precision irrigation. Landbauforsch SH 340, 45–56. Available online at: https://literatur.thuenen.de/digbib_extern/dn046667
Raine, S. R., Meyer, W. S., Rassam, D. W., Hutson, J. L., and Cook, F. J. (2007). Soil-water and solute movement under precision irrigation: knowledge gaps for managing sustainable root zones. Irrig. Sci. 26, 91–100. doi: 10.1007/s00271-007-0075-y
Say, S. M., Keskin, M., Sehri, M., Sekerli, Y. E., and Engineering, T. (2018). “Adoption of precision agriculture technologies in developed and developing countries,” in International Science and Technology Conference (Berlin), 7–15.
Schmidhubber, J., and Tubiello, F. N. (2007). Global food security. Proc. Natl. Acad. Sci. U.S.A. 104, 19703–19708. doi: 10.1073/pnas.0701976104
Shah, N. G., and Das, I. (2012). “Precision irrigation: sensor network based irrigation,” in Problems, Perspectives and Challenges of Agricultural Water Management (Rijeka: InTech), 217–232.
Shibusawa, S. (2001). Precision farming approaches to small-farm agriculture. IFAC Proc. 34, 22–27. doi: 10.1016/S1474-6670(17)34099-5
Shih, S. F. (1984). Data requirement for evapotranspiration estimation. J. Irrig. Drain Eng. 110, 263–274. doi: 10.1061/(ASCE)0733-9437(1984)110:3(263)
Smith, R., and Baillie, J. (2009). “Defining precision irrigation: a new approach to irrigation management,” in Irrigation and Drainage Conference 2009 (Swan Hill, VIC: Irrigation Australia Ltd.), 18–21.
Trout, T. J., and Gartung, J. (2004). Irrigation water requirements of strawberries. Act. Hort. 664, 665–671. doi: 10.17660/ActaHortic.2004.664.84
Tsang, S. W., and Jim, C. Y. (2016). Applying artificial intelligence modeling to optimize green roof irrigation. Energy Build. 127, 360–369. doi: 10.1016/j.enbuild.2016.06.005
Tubiello, F. N., Soussana, J. F., and Howden, S. M. (2007). Crop and pasture response to climate change. Proc. Natl. Acad. Sci. U.S.A. 104, 19686–19690. doi: 10.1073/pnas.0701728104
Valipour, M., Bateni, S. M., Gholami Sefidkouhi, M. A., Raeini-Sarjaz, M., and Singh, V. P. (2020). Complexity of forces driving trend of reference evapotranspiration and signals of climate change. Atmosphere 11:1081. doi: 10.3390/atmos11101081
Wang, S. F., Duan, A. W., and Zhang, Z. Y. (2008). Comparison and analysis of Hargreaves equation and Penman-Monteith equation during the different hydrological years in the semi-arid region. Trans. Chin. Soc. Agric. Eng. 24, 29–33. Available online at: https://en.cnki.com.cn/Article_en/CJFDTotal-NYGU200807008.htm
Willmott, C. J. (1982). Some comments on the evaluation of model performance. Bull. Amer. Meteor. Soc. 63, 1309–1317. doi: 10.1175/1520-0477(1982)063<1309:SCOTEO>2.0.CO;2
Yang, Y., Cui, Y. L., Luo, Y. F., Lyu, X. W., Traore, S., Khan, S., et al. (2016). Shortterm forecasting of daily reference evapotranspiration using the Penman-Monteith model and public weather forecasts. Agric. Water Manage. 177, 329–339. doi: 10.1016/j.agwat.2016.08.020
Yuan, B. Z., Sun, J., and Nishiyama, S. (2004). Effect of drip irrigation on strawberry growth and yield inside a plastic greenhouse. Biosyst. Eng. 87, 237–245. doi: 10.1016/j.biosystemseng.2003.10.014
Zhang, Y. H., Wang, X. L., and Hu, G. G. (2012). Evapotranspiration estimation of Hailiu river basin based on MODIS data. Earth Sci. J. Chin. Univ. Geosci. 37, 375–380. Available online at: http://caod.oriprobe.com/order.htm?id=29974408&ftext=base
Keywords: solar radiation, temperature, cloudiness, Makkink, Seolhyang
Citation: Jo WJ, Kim DS, Sim HS, Ahn SR, Lee HJ, Moon YH, Woo UJ and Kim SK (2021) Estimation of Evapotranspiration and Water Requirements of Strawberry Plants in Greenhouses Using Environmental Data. Front. Sustain. Food Syst. 5:684808. doi: 10.3389/fsufs.2021.684808
Received: 24 March 2021; Accepted: 10 June 2021;
Published: 06 July 2021.
Edited by:
Yanjun Shen, University of Chinese Academy of Sciences, ChinaReviewed by:
Zhang Yucui, Chinese Academy of Sciences (CAS), ChinaCopyright © 2021 Jo, Kim, Sim, Ahn, Lee, Moon, Woo and Kim. This is an open-access article distributed under the terms of the Creative Commons Attribution License (CC BY). The use, distribution or reproduction in other forums is permitted, provided the original author(s) and the copyright owner(s) are credited and that the original publication in this journal is cited, in accordance with accepted academic practice. No use, distribution or reproduction is permitted which does not comply with these terms.
*Correspondence: Sung Kyeom Kim, c2traW03NkBrbnUuYWMua3I=
†These authors have contributed equally to this work
Disclaimer: All claims expressed in this article are solely those of the authors and do not necessarily represent those of their affiliated organizations, or those of the publisher, the editors and the reviewers. Any product that may be evaluated in this article or claim that may be made by its manufacturer is not guaranteed or endorsed by the publisher.
Research integrity at Frontiers
Learn more about the work of our research integrity team to safeguard the quality of each article we publish.