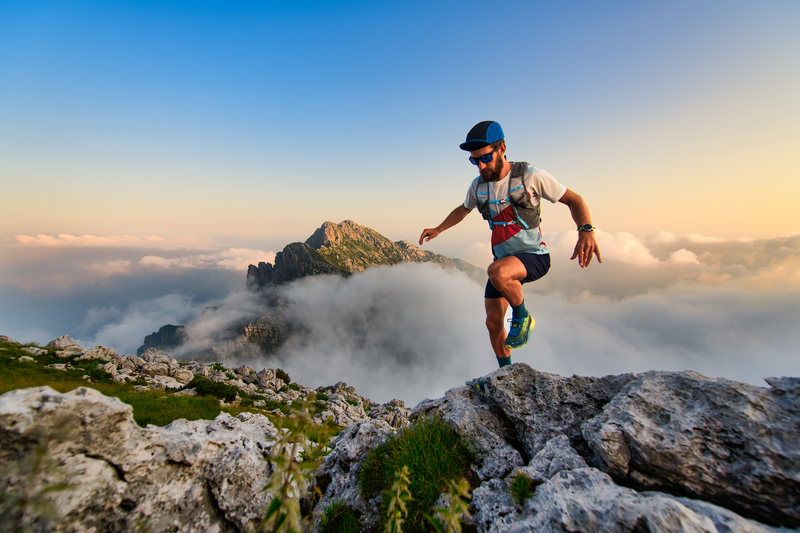
94% of researchers rate our articles as excellent or good
Learn more about the work of our research integrity team to safeguard the quality of each article we publish.
Find out more
ORIGINAL RESEARCH article
Front. Sustain. Food Syst. , 26 August 2021
Sec. Land, Livelihoods and Food Security
Volume 5 - 2021 | https://doi.org/10.3389/fsufs.2021.671419
This article is part of the Research Topic Cross-cutting Issues in the Water, Land, Energy and Food Security Nexus: Perspectives from Sub-Saharan Africa View all 10 articles
Climate change and variability are happening in Tanzania, and their impacts are felt in the socioeconomic sectors, as they have resulted in the decline of agricultural productivity and increased deforestation. This study assessed the actual changes in deforestation levels and improved livelihoods caused by the adoption of climate-smart agriculture (CSA) practices. Data were collected through semi-structured household questionnaires, focus group discussions, key informants, GIS tools, and observations. Quantitative data were analyzed using Arc GIS 10.3 software, SPSS version 16.0, and Excel Spreadsheet, while qualitative data were analyzed through content analysis techniques. The findings show that farmers' livelihoods have improved and also deforestation levels decreased as a result of increased adoption of CSA during the past 30 years. Deforestation levels have been reduced from 64.6 ha per year during 1985–1995 to 11.8 ha per year during 1995–2005 and to 10.4 ha per year during 2005–2017. Further findings showed that the agricultural area had been decreased from 4,534 ha in 1995 to 4,039 ha in 2017, which is evidence that after the scale-up of CSA from the year 1992, agricultural practices were not contributing much to deforestation, while increased crop production and income were observed, which in turn supported farmers in enhancing food security, purchasing production tools, livestock, and payment for medical services, school fees, and construction of modern houses. We found that adoption of CSA systems such as agroforestry (i.e., agrisilviculture) is very crucial for improving farmer's livelihoods and reducing deforestation. Therefore, farmers need close mentoring on climate-resilient agroforestry systems, such as agrisilviculture.
Climate change (CC) is currently at the forefront of debates and discourses on global environmental change (O'Neill et al., 2017). The global nature of causes and consequences of CC implies the need for international collective action for an efficient, effective, and equitable policy response (Harris, 2007). The United Nations Framework Convention on Climate Change (UNFCCC, 2008) identified two policies responsible for addressing CC, which include mitigation of CC by reducing greenhouse gases (GHGs) in the atmosphere and enhancing carbon sinks and adaptation to the impacts of CCs in the world, where climate-smart agriculture (CSA) acts as CC adaptation and mitigation intervention.
CSA refers to an approach that sustainably increases productivity, enhances resilience (adaptation), reduces GHGs (mitigation) where possible, and enhances achievement of food security and development goals (FAO, 2013). It helps people who manage agricultural systems to respond effectively to CC (Lipper et al., 2014). The CSA sustainably increases productivity and incomes without degrading forests, adapting to CC and reducing GHG emissions where possible.
Agriculture provides an important income source to the community in rural areas (Heidhues, 2001). Developing the potential to increase the productivity and incomes from smallholder crop, livestock, fish, and forest production systems will be the key to achieve global food security over the next 20 years (Sage, 2013). CC is expected to hit developing countries the hardest, and its effects include higher temperatures, changes in precipitation patterns, rising sea levels, and more frequent extreme weather events (Kifle, 2008). All of these pose risks for agriculture, forests, and food and water supplies. CSA as a resilience approach is, therefore, a predominant concern.
The CSA plays a potential role in responding to CC and variability (CCV) impacts such as withstanding prolonged and intensive dry seasons as well as in reducing pressure toward natural forests (Nyasimi et al., 2017). For example, trees in agroforestry components can increase the capacity of seasonal crops to tolerate drought and thus support farmer's food security by avoiding total crop failure in the farm (Ekpo and Asuquo, 2012). In Tanzania, CSA systems have shown good performance even in the changing climate (Pye-Smith, 2010; Charles et al., 2013; Uisso, 2015). For example, a study by Pye-Smith (2010), in the Shinyanga Region, reported restoration of the previously deforested shrubs for fodder (Ngitili) from 600 to 500,000 ha in the periods of 1980's and 1990's.
For the context of this study, agroforestry, particularly agrisilviculture, as one of CSA practices has been analyzed in the study area. This system involves the integration of trees and other large woody perennials into crops farming, with the aim of conserving trees and improving soil fertility. Various areas in Tanzania have validated the good performance and contribution of the introduced agroforestry practices (i.e., agrisilviculture) to community livelihoods and land management (e.g., Shalli, 2003; Maduka, 2007, Shilabu, 2008; Namwata et al., 2012; Ruboya, 2013), yet little has been documented and published regarding the extent of reduced deforestation due to the implemented agroforestry system in the study area. Furthermore, few studies that assessed the role of agroforestry in reducing deforestation in Tanzania mostly assumed that due to increased yield of agricultural crops and forest products on farm land, deforestation has decreased (e.g., Ramadhani et al., 2002). Ramadhani et al. (2002), also in the Tabora District, assessed the potential of rotational woodlot in improving household income and reducing deforestation and revealed that the practice supported farmers to generate additional income and had a potential to conserve 1,875 ha of forests per year. However, this has been challenged by Schroth (2004), who argued that the connections between agroforestry and use of surrounding forest areas are complicated functions, and without actually measuring deforestation rates, nothing can be really concluded about change in deforestation. However, in a study conducted in a neighboring Lushoto District, Ilomo (2014) claimed that the existing agroforestry practices were not enough for environmental conservation as whole, as majority of population still used the surrounding natural forests for their firewood needs and cleared farmland in high speed.
A study by Charles et al. (2013) in the Mwanga District assessed agroforestry as a tool in CC adaptation and revealed that the system produces a variety of products that increase farmer's resilience to climate stresses. Also, another study by Uisso (2015) assessed agroforestry as a CC adaptation option and found that agroforestry systems can help withstand the impacts of CC. The study recommended that agroforestry practices that support CC adaptation and mitigation should be given priority. The study further recommended that linking agroforestry with payment for ecosystem services schemes such as carbon credit mechanisms can enhance the adaptive capacity of agroforestry practitioners.
While these studies shed superficial light on the possible contribution of agroforestry in CC adaptation and mitigation as well as sustainable forest management (SFM), there is limited research on the role of CSA in reducing deforestation in the changing climate. Some studies like those of Ramadhani et al. (2002) and Pye-Smith (2010) assumed that due to the increased yield of crops, forest products, and adoption of agroforestry, deforestation has decreased, but the actual measured levels of reduced deforestation were not calculated in these studies. Therefore, this study sought to uncover the potential of CSA (i.e., agroforestry) in addressing deforestation problems and also enhancing crop productivity in the Kilindi District. We examined the change in deforestation levels and improved farmers' livelihoods as a result of CSA practices in the Songe-Bokwa landscape, Kilindi District. Specifically, the study (1) determined farmers' perception on CCV, (2) examined the contribution of CSA to farmers' livelihood in the changing climate, and (3) assessed the potential contribution of CSA in reducing deforestation levels.
This study is based on the conceptual framework (Figure 1) modified from USAID Forestry Programs (2009). The framework explains the role of land use productivity in improving livelihoods and natural resources. It further links failure on sustainability of land use functions due to socioeconomic factors and vulnerability to climate stress, and therefore, interventions to improve land use productivity under climate stress such as agroforestry systems are assumed to enhance sustainable functioning of the landscape ecosystems.
Figure 1. Conceptual framework for integrating climate change in agriculture and forestry landscape.
High population growth, poverty, and limited support from the government are the main factors that drive farmers to practice unsustainable farming practices (Ndaki, 2014; Kideghesho, 2015). Farmers fail to buy agricultural inputs to maintain long-term productivity in their farm. Simultaneously, fallow periods for shifting cultivation have been reduced due to population pressure. As a result, farmers are forced to open new farms in the primary forests to maintain productivity. Moreover, CCV have been reducing crop productivity due to rapid reduction of soil nutrient and soil moisture (Pauline, 2015). This has led to crop failure, thus exacerbating the tendency of clearing of forests for fertile and moist soil as well as overharvesting of forest products to cope with food insecurity (Yanda and Mubaya, 2011; Ndaki, 2014).
To reduce food insecurity, forest degradation, and deforestation, farmers practice agroforestry as well as manage the existing natural forests. According to Ndaki (2014), farmers' willingness to implement sustainable interventions increases if they realize that their unsustainable livelihood activities will continue to affect their own livelihood. The agroforestry practices can result in better yield, as a wider variety of components are integrated as compared with monoculture farming. However, agroforestry will only have an effect on the landscape as a whole if supported by good government policy, such as land use planning and participatory forest management. The integrated and improved agriculture and forest land use will enhance better livelihoods and forest condition. Improved livelihoods and forest condition will enhance biodiversity conservation; reduce poverty and community vulnerability to climate stresses; and, thus, ensure sustainability of both agriculture and forest landscapes.
Trees integrated with crop farming provide farmers with diversity of products including crops, timber, fuelwood, building poles, fruits, fodder, and medicinal plants. These products can directly be consumed for domestic uses or used for additional income generation (Karwani et al., 2016). For example, Joseph (2015) found that in the Morogoro District, crop productivity increased after implementation of agroforestry. Likewise, Ruboya (2013) found that in the Meatu District, the agroforestry practice contributed 44 and 62% of the total household income and food produced, respectively. A study conducted in the Maswa District by Shilabu (2008) found that agroforestry is a more reliable option for sustaining food security and income than monoculture. However, due to low adoption rates of agroforestry practices in the district, the trend in increased food supply was not statistically significant. Furthermore, a study in the Gairo District by Kalineza et al. (1999) concluded that agroforestry practiced in this area was the most popular soil conservation intervention and provided multiple natural resource products to farmers.
The study was conducted in two villages adjacent to Songe-Bokwa Forest in the Kilindi District. The forest is surrounded by four villages, namely, Bokwa, Kwamba, Vilindwa, and Songe, found in Bokwa and Kwamba wards (Figure 2). The district extends between latitudes 5°18′ and 5°48′ S and longitudes 35° and 37°45′ E. The district has an area of 6,443.52 km2, and it is divided into 20 wards). The Kilindi District has a population of 236,833 people based on the last census of 2012 (United Republic of Tanzania, 2012). However, the population provided by village leaders during a household survey was 4,900 and 3,560 for Bokwa and Kwamba villages, respectively. The Kilindi District is one of the 10 districts of the Tanga Region. It is bordered by Handeni and Simanjiro Districts to the north, Mvomero and Kilosa Districts to the south, Handeni District in the eastern side, and Kiteto and Simanjiro Districts to the west.
Figure 2. Map of Kilindi District showing the study villages (source: IRA GIS Lab, University of Dar es Salaam, 2017).
The district is characterized by arid and semiarid climate conditions. It has two rainy seasons, the short and the long, from October to January and from March to June, respectively. The mean annual rainfall is about 750 mm, while the average temperature varies from 27 to 30°C, except for highland areas where temperatures go below 27°C. The cooler season is during June and September, while the hottest one is between December and March.
The study used random and purposive sampling designs to collect both quantitative and qualitative data. The purposive sampling was used to select the two representative villages in the study area, key informants and members of focus group discussions (FGDs). Factors considered in purposive sampling included villages with the most degraded and those with healthy forests, farmers from different farming types (climate-smart and non-climate-smart practices), farming system-knowledgeable farmers, and those living in the village for at least 30 years. A simple random sampling technique was used to select households interviewed through a questionnaire. Pieces of paper with a number representing names of household heads in the village were picked randomly one by one to choose the respondents. After the papers were picked, the selected sample was assessed and confirmed to contain a reasonable representation of CSA and non-CSA farmers and a high percentage of those living in the village for at least 30 years.
The study employed a sample size of 5%. At least 30 respondents resulted from 5% of the total population in each study village. This is a valid representation according to Bailey and Mouton (1998), who recommended that for studies that use statistical data analysis, a minimum of 30 respondents is enough to represent the population.
The primary data were collected through the use of the household survey, key informant interviews, and FDGs. The tested semi-structured questionnaires were administered to household respondents. The questionnaire collected information on demographic characteristics, livelihood activities and status, agricultural productivity, forest utilization, deforestation status, the productivity of CSA practitioners, and community perceptions on CCV. Two FGDs were done in each village whereby each group comprised eight members, one group for each sex. Discussed topics include land use change, farming systems, farm productivity, forest resource availability, farmers' livelihoods for the past 30 years, and drivers for the identified changes. Similarly, necessary measures taken to improve changes in farmers' livelihoods and forest condition were also part of the discussion. Other topics discussed were the trend of CCV variables (e.g., rainfall and temperature), its impacts on farmers' livelihoods and forest conditions, and farmers' responses to impacts of CCV. Furthermore, for triangulation purposes, key informant interviews with selected village leaders and elders, ward executive officers, extension officers, ward and district agricultural officers, and forest and land officers captured detailed information on the same aspects as FGDs.
Four Landsat Thematic Mapper (Landsat TM) images of 1985, 1995, 2005, and 2017 taken during the dry season (Table 1) covering Kilindi District were downloaded from United States Geological Survey (USGS) in the EarthExplorer archive. Dry-season images were preferred to facilitate differentiating the land covers easily, especially the green vegetation and water body cover (Thenya, 2001). Besides, the land use map was collected from Kilindi District Land office and saved as a reference in land use/land cover classification.
The process involved projection of satellite images, satellite image classification, selecting training samples and signature development, supervised image classification, accuracy assessment, and change detection analysis.
• Projection of Satellite Images
The collected satellite images were pre-processed first before being analyzed; the process involved projecting them to Universal Transverse Mercator (UTM) zone 37 S, which corresponds to Tanga Region; then red, green, and blue (RGB) composite color images were created for each year. RGB was created by layer stacking, which involved Bands 2, 3, and 4 for Landsat TM 4-5 and Bands 3, 4, and 5 for Landsat 8. Each image with composite color was extracted to cover the study area by using the study area map.
• Satellite Image Classification Process
Supervised image classification was opted purposely because the whole process is controlled by the user especially on deciding the number of classes to be identified, creation of training samples, and detailed knowledge about the real study area land use and land cover distribution (Coppin and Bauer, 1996).
• Selecting Training Samples and Signature Development
The training samples representing the pixels with particular land covers were created by using polygons with the aid of GPS points, Google Earth image, and land use map of the study area. The land use classes identified were bushland, agriculture, forests, and settlements. The same training samples were stored and used to create signature file for the entire supervised image classification process.
• Supervised Image Classification
Supervised image classification was done after creation of signature file; each composite image was supplied in the so-called maximum likelihood classification algorithm as input together with the associated signature file. After the algorithm was run, the land use and land cover maps with trained classes were produced and ready for the classification accuracy assessment process. All these processes were performed on each image in ArcGIS 10.3 software.
• Accuracy Assessment
The assessment of classification accuracy was performed on each classified map by comparing the land use classes with 25 GPS points showing the current land use (collected during ground truthing) and then creating an error matrix table; the producers, users, and overall accuracy were calculated from the table in Microsoft Excel Spreadsheet, as suggested by Coppin and Bauer (1996). Classification accuracy of min. 70% was considered acceptable. The formula used in accuracy assessment includes that of overall accuracy, user's accuracy, and producer's accuracy, whereby
Overall Accuracy = Sum of correct classified/Number of observation * 100
User's Accuracy = Total correct classified pixels in the row/Total pixels in the row * 100
Producer's Accuracy = Total correct classified pixels in the column/Total pixels in the column * 100
• Change Detection Analysis
The statistics from classified land use and land cover maps of 1985, 1995, 2005, and 2017 were used to detect the changes that occurred in a period of 32 years. Change detection involved finding the quantities of the land use/land cover changed, locations where the changes occurred, and the type of changes that occurred at a certain defined time interval (Kashaigili et al., 2006). In a post-classification process, quantitative changes were detected by comparing the successive pairs of classified maps by subtracting the quantities of the current land use class from the quantities of the past land use class; the differences obtained from each pair were converted to percentage of change by using the following formula.
Percentage on change = Area of observed change/Total area * 100
Through change detection, it is possible to deeply understand the anthropogenic interference in the land use and land cover of an area; hence, this can also help to understand the role of agroforestry in protecting natural forests.
To obtain the trend and the change of rainfall and temperature patterns in the study area from 1985 to 2017, the collection of measured monthly rainfall and temperature for the Kilindi District was necessary. These data were obtained from the Tanzania Meteorological Agency (TMA) head office in Dar es Salaam.
Data in this study on the contribution of CSA to farmers' livelihoods and SFM were subjected to analysis of variance (ANOVA) using the SPSS software package (Copyright SPSS for Windows, Chicago: SPSS Inc.) to compare if there is a statistical difference in productivity between the CSA and non-CSA practitioners. Linear regression and descriptive statistics for temperature and rainfall data were run to obtain the relationship and the means, respectively, using R software. The results were presented in tables, figures, and maps. This includes data on trends of land use size, agricultural productivity, forest resource availability, impacts of CSA in livelihoods and deforestation levels, households' perceptions on CCV, and its trends for the past 32 years.
The qualitative data were analyzed using transcribing, describing, classification, and connection techniques. Transcribing involved judgments about what level of detail should be chosen from the collected information to develop specific ideas (transcripts/scripts). The transcripts were then comprehended in a form that can be easily interpreted (describing). The described data were interpreted and grouped into coherent classes (classification). Finally, connection of the data from different classes was done to get meaningful statements. Socioeconomic information helped to give details on the perceived and observed trends in climate (rainfall), agricultural productivity, agroforestry farming practices, land use changes, and their underlying drivers.
The analysis of remote sensing data utilized the supervised image classification. This involved the creation of a signature file (Table 2) where each composite image was supplied in the so-called maximum likelihood classification algorithm as input together with the associated signature file. After the algorithm was run, the land use and land cover maps with trained classes were produced and were ready for classification. All these processes were performed on each image in ArcGIS 10.3 software.
The findings show that a relatively high percentage (60.4%) of source of household's income in the study area comes from climate-sensitive sectors (sale of agricultural crops, forest products, and livestock). The remaining 39.6% come from informal work, sale of bricks, carpentry, and formal work (Figure 3). This concurs with past studies showing similar findings that the majority of households source their income from climate-sensitive economic activities like crop farming (e.g., United Republic of Tanzania, 2002; Paavola, 2008; Rowhani et al., 2011).
Respondents perceived that CC is occurring in the study area as they witnessed repeatedly prolonged dry spells, floods, and erratic rains. The results in Table 3 indicate that CCV had led to a decrease in crop production. Figure 4 shows that 38.7, 18.6, and 12.8% of households perceived that CCV resulted in food shortage, decreased income, increased disease outbreaks, youth emigration, and rise of food price.
Table 3. Estimate of crop harvest per acre in a bag of 90 kg during bad and good climate conditions.
The findings showed that 31.4% of households were still practicing non-CSA practices. About 90% of the non-CSA practices shift cultivation by clearing intact forests, thus decreasing the size of forest land. One key informant from Bokwa village stated that during critical crop failure due to climate stresses (which usually hits the majority of villagers simultaneously), the lives of most of the households depend on the forest resources. Also, as an alternative income channel during the low rainfall period, farmers cultivate tobacco in the forest where enough moisture still prevails in the soil. Unfortunately, tobacco cultivation involves clearing of forests for field and firewood used at the time of curing (drying). Also, nomadic pastoralists have been clearing forests in the area to establish a temporary settlement while looking for pasture.
The practiced CSA practices include agroforestry, conservation agriculture, integrated nutrient management, and agronomic techniques such as cover crops, improved crop varieties, drought-resistant crops, intercropping, and crop rotation.
The findings demonstrated no significant variation in annual mean rainfall (mm/year) [F(1, 31) 0.04, p > 0.05] between 1985 and 2017. However, the highest annual mean rainfall was recorded in 1997 (199 mm) and the lowest annual mean rainfall in 2005 (5.08 mm) (Figure 5).
Also, the variation of annual temperature in the study area was not significant [F(1, 31) 0.04, p > 0.05]. The highest temperature was recorded in 2003, 2007, and 2009 (Figure 6) and the lowest in 2013 and 1989 (Figure 7). The lowest annual mean temperature was observed in 1985–1988, 2000–2010, and 2013–2017. The highest annual mean minimum temperature was recorded in 2003, while the lowest annual mean minimum temperature is observed in 1989. Generally, there was high variability of annual mean maximum and minimum temperature and with many recorded high temperatures from 2000 to 2010 compared with the previous years (1985–1999).
The productivity in farms under the practice of CSA was higher than in non-CSA farms based on t-test (α = 0.05, df = 5, p = 0.0431; Table 4). Also, the production of crops after the introduction of CSA was higher than before the practice (α = 0.05, df = 5, p = 0.028; Table 5). This trend indicated the improvement in the livelihood of agroforestry practitioners, as high crop production enhances food security, purchasing production tools, livestock, and payment for medical services, school fees, and construction of modern houses.
Table 5. Comparison of crop harvest per acre in a bag of 90 kg for climate-smart farmers before and after engaging in CSA interventions.
According to farmers, agroforestry helped them to increase crop productivity. The findings show that there is a significant increase in crop harvest after farmers engaged in agroforestry (Table 4). This has led to increased food security (19%), buying production tools (16%), supporting medical services (15%), improved household income (13%), purchasing livestock (13%), paying school fees (13%), and construction of modern houses (11%) (Figure 8). Through comparison of average crop harvest before and after agroforestry practices from the data provided by interviewed household, the study proved that there is a significant increase in crop harvest after farmers engaged in agroforestry (Table 6). Since crop farming is the main economic activity for 58% of households in the study area, it is logical to assume that most of the income for supporting non-food services came from selling part of the food crop.
The findings from key informants and FGDs revealed that before the intensification of CSA practices in the 1990's, there was a high rate of deforestation. It further affirmed that from the 2000's onwards, the rate of clearing forests for opening new farms has been decreasing due to the adoption and practice of CSA. Key informants also elaborated that the establishment of the Kilindi District resulted in high demand for land for settlements. Settlements occupied much of the agricultural area and forced some farmers to open new farms in the forests to compensate for the lost farming areas. Maps from satellite images also show that deforestation levels during the period of 1985 to 2017 have decreased (Figures 9A–D). In the period from 1985 (Figure 9A) to 1995 (Figure 9B), 646 ha of forest disappeared; from 1995 to 2005 (Figure 9C), only 118 ha disappeared; and from 2005 to 2017 (Figure 9D), deforestation went down to 104 ha. Further findings showed that the agricultural area had been decreased from 4,534 ha in 1995 to 4,039 ha in 2017, which is evidence that after the scale-up of CSA from the year 1992, agricultural practices were not contributing much to deforestation (Table 6). Other factors that might have contributed to a decrease in deforestation include the level of community awareness on SFM, law enforcement, and limited suitable forest landscape for opening farms. Also, findings indicate that during 1985–1995, the size of the agricultural area had increased by 3,721 ha; during 1995–2005, it increased by only 11 ha; and thereafter, the size of agricultural land decreased by 506 ha during 2005–2017. This is an indication that from 1995 to 2005, agricultural activities contributed very little to deforestation, while during 2005 to 2017, agriculture did not have any direct contribution to deforestation. However, key informants reported that during 2005 and 2017, some forests converted into new farms as part of agricultural land were converted to settlements. Also, it had been noted that during 2005–2017, the size of bushland, forest, and agricultural land were decreasing, while the area for settlements increased.
Repeatedly prolonged dry spells, floods, and erratic rains confirmed respondents' perception that CC is happening in the study area. This perception was congruent with observed rainfall records from the Kilindi District as reported by Mjata (2015). The study revealed that there was rainfall variability, a shift in rainfall patterns, and an increase in temperature since the 1980's. Also, the study of Magreth and Bushesha (2017) on the potential of forest resources on adaptation to CCV in the Kilindi District revealed that farmers perceived that CC has prolonged droughts and shifts in rainfall patterns. Similar perceptions have been given by farmers in the Great Ruaha sub-basin: rainfall pattern is fluctuating and temperature increases (Pauline et al., 2017, Pauline and Grab, 2018). Likewise, farmers perceived that CC affects agriculture, forestry components, other environment components, and household livelihoods. The findings show that CCV led to a decrease in crop productivity. The study by Yanda et al. (2005) observed a similar trend that in Tanzania, CCV has caused a general decline in crop productivity.
The climatic conditions leading to a decrease in crop harvesting includes decreased rainy days, prolonged dry spell, floods, and unpredictable (early and late) rainfall onset. The findings from key informants and FGDs revealed that low rainfall triggers pest and disease outbreaks. During field observations, it was confirmed that crops had been attacked by pests and affected by floods. It was also highlighted that despite CCV, loss in soil fertility and moisture in the farms was attributed to low knowledge and limited capital for practicing sustainable farming methods. The perceived CCV increased the rate of soil moisture and nutrients loss and thus exacerbated the decrease in crop productivity. Other studies including Yanda and Mubaya (2011) and Ndaki (2014) also concluded that climatic stress intensifies the loss in soil moisture and nutrients leading to food insecurity and degradation of the natural resources. One key informant from Kwamba village stated that “we used to abandon a piece of land for about 8 years to restore its fertility, but presently we can only leave it on fallow for not more than 4 years.” This agrees with the findings of Kilawe (2016) that in Kilosa traditional shifting cultivation in Tanzania is mainly transformed into short fallow and permanent monoculture.
Furthermore, findings show that CCV affect some agricultural components. The annual crops and livestock are major victims than the trees. In one of the farms in Bokwa village, it was observed that sugarcane had been eroded by floods while the trees in the same field withstand the impacts. This finding is supported by Thorlakson and Neufeldt (2012), who also found that trees had been more resilient to extreme weather events such as floods and drought and thus helps to reduce the risk of crop losses.
Farmers perceived that CC resulted in increased pest outbreaks, a rise in temperature, deforestation, decreased water table, and a decline in rainfall. The findings from FGDs affirmed that during critical crop failure, some farmers have been relying on charcoal making, which involved clearing the forests, and most of the water wells around the cleared forests dried. Similarly, Ndaki (2014) reported the same incidence that during the periods of crop failure, the rate of cutting of trees for charcoal making increased to provide an alternative source of income.
Also, households perceived that CC affects household livelihoods. According to them, it resulted in a shortage of food, a decrease in income, youth emigration, increased disease outbreaks, and increased cost of food. A similar situation was also noted in the Great Ruaha River sub-basin that the shift in rainfall onset affected the normal growth of crops leading to reduced crop yield to the extent that farmers faced food insecurity (Pauline et al., 2017; Pauline and Grab, 2018). Likewise, Ndaki (2014) in his study of CC adaptation strategies at Mkomazi sub-catchment found similar results regarding out-migration. An increased number of men temporarily moved to the nearby towns in search of casual work to sustain their households during prolonged dry seasons. Liwenga (2003) in a study on rainfall-induced crop failure, food insecurity, and out-migration in Same-Kilimanjaro found that when farmers faced food insecurity, they migrated outside the village to find alternative livelihoods.
In response to the decline in crop productivity and deforestation, farmers practiced CSA practices such as agroforestry (i.e., agrisilviculture), conservation agriculture, integrated nutrient management, and agronomic techniques such as cover crops, improved crop varieties, drought-resistant crops, intercropping, and crop rotation. The findings from household interviews showed that farmers engaged in CSA to solve the problem of food insecurity, reduce dependency on forest resources, and increase household income. Farmers in both Bokwa and Kwamba villages commented that CSA was beneficial, as it withstands the negative impacts brought by CCV. One non-climate-smart farmer witnessed that during prolonged dry spells, most of those who were not practicing CSA have faced total crop failures, while those practicing agrisilviculture harvested at least limited crops and fruit from their trees. During field visits, it was observed that trees were less affected by climatic stress than were other crops. These findings were also affirmed by Charles et al. (2013) and Mngumi (2016) that practicing monoculture is seen as a risk in bad climatic conditions, which results in crop failure or damage.
A notable increase in crop harvest after farmers engaged in CSA was recorded in the study area. Farmers were food secured and gained more income through sales of their crops. They used part of their income for paying school fees, buying production tools, supporting medical services, purchasing livestock, and paying house construction. This made CSA farmers more resilient to negative climatic effects. The results are similar to findings from other studies (Shalli, 2003; Maduka, 2007; Shilabu, 2008; Namwata et al., 2012, Ruboya, 2013) that found that CSA practices contributed additional household food and income to normal agricultural practices. Mahenge (2014) compared the productivity of conservation agriculture and conventional farming in Southern Uluguru mountains and found that the marginal productivity of land for conservation agriculture farmers was 366 kg/ha while that of conventional agriculture farmers was 248 kg/ha. Maize species resistant or tolerant to maize streak virus in Central Tanzania proved to have yielded potential ranging from 2.5 (Kito) to 6.25 t/ha, while the local breed is almost yielding nothing when attacked by maize streak virus (Kaliba et al., 1998). Another study by Ilomo (2014) in the Lushoto District revealed that 17.7% of the respondents' annual income has increased since they started CSA. The result lines with that of Charles et al. (2013) who revealed that CSA practices in the Mwanga District gave farmers benefits such as food, fodder, and additional income from sales of livestock, fruits, and timber. Likewise, Joseph (2015), in a study on the effectiveness of CC mitigation interventions in Morogoro District, revealed that the average crop production per acre has increased after farmers started practicing CSA. A related study by Ekboir et al. (2002) in Ghana found that no-tillage farming (conservation agriculture) supported 62% of farmers to increase crop yield in maize, cassava, rice, sorghum, and related crops. Intercropping and crop rotation in Kenya resulted in a 71% maize yield increase. Verchot et al. (2007) reported that in Malawi, agroforestry intervention resulted to increase in maize yield from 0.7 to 1.5 t/ha. Another related study by Nguyen et al. (2013) in Vietnam found that while rice and other rain-fed crops suffered over 40% yield losses in years of extreme drought or flood, tree-based systems and cattle were less affected. The trees provided income, food, feed, and other environmental benefits; thus, agroforestry systems, with high resilience and multiple benefits, made farmers food secure during extreme climatic conditions. Moreover, Lasco et al. (2014) studied the role of trees and agroforestry in reducing smallholder farmer's exposure to climatic risks in Philippines and found that agroforestry resulted in improved crop productivity, diversification of food sources, and increase in income. Also, Rahman (2017) studied the impacts of incorporating trees in smallholder farms in Bangladesh and Indonesia and found that 73% of the 176 tons of fuelwood used annually were sourced from agroforestry tree components established through short rotation coppice technology.
The change in forest size observed in the study area was linked to the settlement expansion, farms, and charcoal making. Opening farms in the intact forests was partly preferred as an adaptation strategy to the loss in soil moisture and nutrients due to extreme temperature. To reduce the rate of deforestation caused by the opening of new farms, farmers engaged in CSA practices. The findings from key informants and FGDs revealed that before the scale-up of CSA practices from the 1990's, there was a high rate of deforestation due to regular expansion of the farming areas. But from the 2000's onwards, the rate of opening new farms by cutting forest has been decreasing, as a high percentage of the farmers are no longer practicing shifting cultivation. This is because CSA components retain soil moisture and nutrients that help in the growth of the annual crops.
The findings show that forest size has not stopped decreasing, but deforestation levels have decreased. Land use/cover maps for 1985, 1995, 2005, and 2017 for the study area show significant changes in forest size. The findings indicate that the deforestation levels from 1985 to 1995, 1995 to 2005, and 2005 to 2017 were 646, 118, and 104 ha, respectively. Information from key informants and FGDs shows that from 1992, there was great awareness creation on CSA in the area, and households in the study area started to implement CSA interventions. Therefore, the decrease in deforestation levels from 1995 onwards is linked to the scaling up of CSA activities in the area. During 1985–1995, the size of the agricultural area increased by 3,721 ha; during 1995–2005, it increased by only 11 ha; and thereafter, the size of agricultural land decreased by 506 ha during 2005–2017. This is an indication that from 1995 to 2005, agricultural activities contributed very little to deforestation, while from 2005 to 2017, agriculture did not have any direct contribution to deforestation. Also, it had been noted that during 2005–2017, the size of bushland, forests, and agricultural land was decreasing, while the area for settlements has been increasing. Generally, from 1985 to 2017, the size of forest area and bushland decreased, while agriculture and settlements increased. This is linked to the fact that the study area became the headquarters for the Kilindi District since 2002, and therefore there was an increase in population, which led to high demand for expansion of settlements and increasing crop productivity by improving farming methods but also compensating agricultural areas occupied by settlements.
The study revealed that there was rainfall variability, shift in rainfall patterns, and increase in temperature in the study area since 1980's. These changes negatively affected agricultural productivity. This study provides empirical evidence on reduced deforestation and improved farmers' livelihoods due to implemented CSA. It revealed that CSA practices help farmers to withstand climatic stresses while improving their livelihoods by increasing crop productivity and income. This, in turn, improves food security and decreases deforestation. An increase in crop productivity influenced by agroforestry has contributed to an increased income and key livelihoods. The findings also revealed that CSA practices were mostly emphasized from 1992, and its implementation has contributed to the reduction of deforestation levels. Deforestation levels have been reduced from 64.6 ha per year during 1985–1995 to 11.8 ha per year during 1995–2005 and 10.4 ha per year during 2005–2017. Further findings showed that the agricultural area had been decreased from 4,534 ha in 1995 to 4,039 ha in 2017, which is evidence that after the scale-up of CSA from the year 1992, agricultural practices were not contributing much to deforestation. Therefore, agroforestry helped to reduce deforestation levels, especially that caused by farming. We found that adoption of CSA systems such as agroforestry (i.e., agrisilviculture) is very crucial for improving farmer's livelihoods and reducing deforestation. Therefore, farmers need close mentoring on climate-resilient agroforestry systems.
The raw data supporting the conclusions of this article will be made available by the authors, without undue reservation.
Ethical review and approval was not required for the study on human participants in accordance with the local legislation and institutional requirements. Written informed consent for participation was not required for this study in accordance with the national legislation and the institutional requirements.
All authors listed have made a substantial, direct and intellectual contribution to the work, and approved it for publication.
The authors declare that the research was conducted in the absence of any commercial or financial relationships that could be construed as a potential conflict of interest.
All claims expressed in this article are solely those of the authors and do not necessarily represent those of their affiliated organizations, or those of the publisher, the editors and the reviewers. Any product that may be evaluated in this article, or claim that may be made by its manufacturer, is not guaranteed or endorsed by the publisher.
Bailey, D., and Mouton, J. (1998). Methods of Social Science Research. London: The free press Collier Macmillan Publisher, 25.
Charles, R. L., Munishi, P. K. T., and Nzunda, E. F. (2013). Agroforestry as adaptation strategy under climate change in Mwanga District, Kilimanjaro, Tanzania. Int. J. Environ. 3, 29–38.
Coppin, P., and Bauer, M. (1996). Digital change detection in forest ecosystems with remote sensing imagery. Rem. Sens. 207–234.
Ekboir, J., Boa, K., and Dankyi, A. A. (2002). Impact of No-Till Technologies in Ghana. Mexico: CIMMYT.
Ekpo, F. E., and Asuquo, M. E. (2012). Agroforestry practice as adaptation tools to climate change hazards in ItuLga, Akwa Ibom State, Nigeria. Glob. J. Hum. Soc. Sci. Geogr. Environ. Geosci. 12, 27–36.
Harris, P. G. (2007). Collective action on climate change: the logic of regime failure. Nat. Resources J. 47:195.
Heidhues, F. (2001). The Future of World, National and Household Food Security. Crop Science: Progress and Prospects. Wallingford: CABI Publishing, 15–31. doi: 10.1079/9780851995304.0015
Ilomo, F. M. (2014). Assessment of Agroforestry Potential in Environmental Conservation and Poverty Reduction in Rural Tanzania: A Case of Lushoto District (Ph.D. Dissertation). University of Dar es Salaam, Dar es Salaam, Tanzania.
Joseph, J. P. (2015). Effectiveness of Climate Change Mitigation Interventions on Crop Productivity in Morogoro District, Tanzania (Ph.D. dissertation). Department of Development Studies, Sokoine University of Agriculture, Morogoro, Tanzania.
Kaliba, A. R. M., Verkuijl, H., Mwangi, W., Mwilawa, A. J. T., Anandajayasekeram, P., and Moshi, A. J. (1998). Adoption of Maize Production Technologies in Central Tanzania. Mexico: International Maize and Wheat Improvement Center (CIMMYT), the United Republic of Tanzania, and the Southern Africa Centre for Cooperation in Agricultural Research (SACCAR).
Kalineza, H. M. M., Mdoe, M. S. Y., and Mlozi, M. R. S. (1999). “Factors influencing the adoption of soil conservation technologies in Tanzania: a case study in Gairo,” in Fourth Annual Scientific Conference of the Faculty of Agriculture, 17–19.
Karwani, G. M., Lulandala, L. L. L., Kimaro, A., and Msigwa, Z. P. (2016). The role of short rotation coppice technology in fuel wood supply in Rungwe district, Tanzania. Int. J. Agri. Res. Innov. Technol. 6, 41–46. doi: 10.3329/ijarit.v6i1.29211
Kashaigili, J. J., Mbilinyi, B. P., McCartney, M., and Mwanukuzi, F. L. (2006). Dynamics of Usangu Plains Wetlands: use of remote sensing and GIS as management decision tools. Phys. Chem. Earth 31:969975. doi: 10.1016/j.pce.2006.08.007
Kideghesho, J. R. (2015). Realities on Deforestation in Tanzania Trends, Drivers, Implications and the Way Forward. Precious Forests—precious Earth. Rijeka: Intech Open Science/Open Minds, 21–47. doi: 10.5772/61002
Kifle, T. (2008) Africa hit hardest by Global Warming despite its low Greenhouse Gas Emissions. Bremen: Institutfür Weltwirt schaft und Internationales Management, Universität Bremen.
Kilawe, C. J. (2016). Intensification of Shifting Cultivation in Tanzania: Degree, Drivers and Effects on Vegetation and Soils (Ph.D. dissertation). Department of Geosciences and Natural Resource Management, Faculty of Science, University of Copenhagen, Copenhagen, Denmark.
Lasco, R. D., Delfino, R. J. P., Catacutan, D. C., Simelton, E. S., and Wilson, D. M. (2014). Climate risk adaptation by smallholder farmers: the roles of trees and agroforestry. Curr. Opin. Environ. Sustain. 6, 83-88. doi: 10.1016/j.cosust.2013.11.013
Lipper, L., Thornton, P., Campbell, B. M., Baedeker, T., Braimoh, A., Bwalya, M., et al. (2014). Climate-smart agriculture for food security. Nat. Climate Change 4:1068. doi: 10.1038/nclimate2437
Liwenga, E. T. (2003). Food Insecurity and Coping Strategies in Semiarid Areas: the Case of Mvumi in Central Tanzania (Ph.D dissertation). Almqvist and Wiksell International, Stockholm, Sweden.
Maduka, S. M. (2007). Role of Agroforestry Products in Household Income and Poverty Reduction in Semi-Arid Areas of Misungwi District, Mwanza Tanzania (Ph.D. dissertation). Department of Ecosystems and Conservation, Sokoine Universitry of Agriculture, Morogoro, Tanzania.
Magreth, S., and Bushesha, P. M. (2017). Potentials of the forest resources in adaptation to climate variability and change in the North Nguu Mountain Block. Int. J. Environ. Protect. Policy 4, 178–186. doi: 10.11648/j.ijepp.20160406.14
Mahenge, J. (2014). Comparative Economic Analysis of Conservation and Conventional Agricultural Practices in Southern Uluguru Mountains, Morogoro, Tanzania (Ph.D. dissertation). Sokoine University of Agriculture, Morogoro, Tanzania.
Mjata, P. J. (2015). The Impact of Climate Change on Rural Livelihoods in the North Nguu Mountains in Kilindi District, Tanzania (Ph.D. dissertation). The Open University of Tanzania, Dar es Salaam, Tanzania.
Mngumi, J. W. (2016). Perceptions of Climate Change, Environmental Variability and the Role of Agricultural Adaptation Strategies by Small-Scale Farmers in Africa: The Case of Mwanga District in Northern Tanzania (Ph.D. dissertation). University of Glasgow, Glasgow, Scotland.
Namwata, B. M., Masanyiwa, Z. S., and Mzirai, O. B. (2012). Productivity of the agroforestry systems and its contribution to household income among farmers in Lushoto District, Tanzania. Int. J. Phys. Soc. Sci. 2, 369–392.
Ndaki, P. M. (2014). Climate Change Adaptation for Smallholder Farmers in Rural Communities: The Case of Mkomazi Sub-Catchment, Tanzania (Ph.D. dissertation). Oldenburg-Carl von Ossietzky University of Oldenburg, Oldenburg, Germany.
Nguyen, Q., Hoang, M. H., Öborn, I., and van Noordwijk, M. (2013). Multipurpose agroforestry as a climate change resiliency option for farmers: an example of local adaptation in Vietnam. Climatic Change 117, 241–257. doi: 10.1007/s10584-012-0550-1
Nyasimi, M., Kimeli, P., Sayula, G., Radeny, M., Kinyangi, J., and Mungai, C. (2017). Adoption and dissemination pathways for climate-smart agriculture technologies and practices for climate-resilient livelihoods in Lushoto, Northeast Tanzania. Climate 5:63. doi: 10.3390/cli5030063
O'Neill, B. C., Oppenheimer, M., Warren, R., Hallegatte, S., Kopp, R. E., Pörtner, H. O., et al. (2017). IPCC reasons for concern regarding climate change risks. Nat. Climate Change 7, 28–37. doi: 10.1038/nclimate3179
Paavola, J. (2008). Livelihoods, vulnerability and adaptation to climate change in Morogoro, Tanzania. Environ. Sci. Policy 11, 642–654. doi: 10.1016/j.envsci.2008.06.002
Pauline, N., and Grab, S. (2018). Whose knowledge matters in climate change adaptation? Perceived and measured rainfall trends during the last half century in south-western Tanzania. Singapore J. Trop. Geogr. 39, 266–280. doi: 10.1111/sjtg.12232
Pauline, N. M. (2015). Living With Climate Variability and Change: Lessons From Tanzania (Ph.D. dissertation). Johannesburg: Faculty of Science, University of the Witwatersrand.
Pauline, N. M., Vogel, C., Grab, S., and Liwenga, E. T. (2017). Smallholder farmers in the Great Ruaha River sub-Basin of Tanzania: coping or adapting to rainfall variability? Climate Dev. 9, 217–230. doi: 10.1080/17565529.2016.1184607
Pye-Smith, C. (2010). A Rural Revival in Tanzania: How Agroforestry Is Helping Farmers to Restore the Woodlands in Shinyanga Region. Nairobi: World Agroforestry Centre.
Rahman, S. A. (2017). Incorporation of Trees in Smallholder Land Use Systems: Farm Characteristics, Rates of Return and Policy Issues Influencing Farmer Adoption (Ph.D. dissertation). Department of Food and Resource Economics, Faculty of Science, University of Copenhagen, Copenhagen, Denmark.
Ramadhani, T., Otsyina, R., and Franzel, S. (2002). Improving household incomes and reducing deforestation using rotational woodlots in Tabora district, Tanzania. Agri. Ecosyst. Environ. 89, 229–239. doi: 10.1016/S0167-8809(01)00165-7
Rowhani, P., Lobell, D. B., Linderman, M., and Ramankutty, N. (2011). Climate variability and crop production in Tanzania. Agric. Forest Meteorol. 151, 449–460. doi: 10.1016/j.agrformet.2010.12.002
Ruboya, A. A. (2013). Effects of Rotational Woodlots on Households' Livelihood in Meatu District, Tanzania (Doctoral dissertation). Sokoine university of Agriculure, Morogoro, Tanzania.
Sage, C. (2013). The interconnected challenges for food security from a food regimes perspective: energy, climate and malconsumption. J. Rural Stud. 29, 71–80. doi: 10.1016/j.jrurstud.2012.02.005
Schroth, G., (ed.). (2004). Agroforestry and Biodiversity Conservation in Tropical Landscapes. Island Press.
Shalli, M. S. (2003). Contribution of Agroforestry against Rural Household Food Security in Kibaha District, Coast Region (Ph.D. dissertation). Sokoine University of Agriculture, Morogoro, Tanzania.
Shilabu, M. D. T. (2008). The Contribution of Agroforestry to Household Food Security and Income Generation in Maswa District, Shinyanga Region (Ph.D. dissertation). Sokoine University of Agriculture, Morogoro, Tanzania.
Thenya, T. (2001). Challenges of Conservation of Dryland Shallow Waters. Laikipia: Ewaso Narok Swamp.
Thorlakson, T., and Neufeldt, H. (2012). Reducing subsistence farmers? vulnerability to climate change: evaluating the potential contributions of agroforestry in western Kenya. Agric Food Secur. 1:15. doi: 10.1186/2048-7010-1-15
Uisso, A. J. (2015). Agroforestry practices, an option for climate change adaptation: a review. Octa J. Environ. Res. 3, 219–225.
UNFCCC (2008). Investment and Financial Flows to Address Climate Change: An Update, Vol. 13. United Nations Framework Convention on Climate Change (UNFCCC), 1–111.
United Republic of Tanzania (2002). Population and Housing census by Sex and Number of Households. Tanzania. Available online at: http://www.nbs.go.tz/sense/popu2.php (accessed August 12, 2017).
United Republic of Tanzania (2012). Population and Housing Census by Sex and Number of Households. Tanzania. Available online at: http://www.nbs.go.tz/sense/popu2.php (accessed August 12, 2017).
Verchot, L. V., Van Noordwijk, M., Kandji, S., Tomich, T., Ong, C., Albrecht, A., et al. (2007). Climate Change: linking adaptation and mitigation through agroforestry. Mitigation Adaptat. Strateg. Glob. Change 12, 901–918. doi: 10.1007/s11027-007-9105-6
Yanda, P. Z., Kangalawe, R. Y., and Sigalla, R. J. (2005). Climatic and Socioeconomic Influences on Malaria and Cholera Risks in the Lake Victoria Region of Tanzania. AIACC Working Papers.
Keywords: climate change, climate-smart agriculture, livelihood, forest management, Tanzania
Citation: Nkumulwa HO and Pauline NM (2021) Role of Climate-Smart Agriculture in Enhancing Farmers' Livelihoods and Sustainable Forest Management: A Case of Villages Around Songe-Bokwa Forest, Kilindi District, Tanzania. Front. Sustain. Food Syst. 5:671419. doi: 10.3389/fsufs.2021.671419
Received: 23 February 2021; Accepted: 20 April 2021;
Published: 26 August 2021.
Edited by:
Mulala Danny Simatele, University of the Witwatersrand, South AfricaReviewed by:
Kazi Kamrul Islam, Bangladesh Agricultural University, BangladeshCopyright © 2021 Nkumulwa and Pauline. This is an open-access article distributed under the terms of the Creative Commons Attribution License (CC BY). The use, distribution or reproduction in other forums is permitted, provided the original author(s) and the copyright owner(s) are credited and that the original publication in this journal is cited, in accordance with accepted academic practice. No use, distribution or reproduction is permitted which does not comply with these terms.
*Correspondence: Noah Makula Pauline, cGF1bGluZS5ub2FoQHVkc20uYWMudHo=; orcid.org/0000-0001-6560-2932
Disclaimer: All claims expressed in this article are solely those of the authors and do not necessarily represent those of their affiliated organizations, or those of the publisher, the editors and the reviewers. Any product that may be evaluated in this article or claim that may be made by its manufacturer is not guaranteed or endorsed by the publisher.
Research integrity at Frontiers
Learn more about the work of our research integrity team to safeguard the quality of each article we publish.