- 1Department of Food Science and Technology, National Institute of Food Technology, Entrepreneurship and Management, Sonipat, India
- 2Department of Agricultural and Food Engineering, Indian Institute of Technology, Kharagpur, India
- 3Department of Electronics and Electrical Communication Engineering, Indian Institute of Technology, Kharagpur, India
- 4Department of Basics and Applied Sciences, National Institute of Food Technology, Entrepreneurship and Management, Sonipat, India
The paper deals with the dynamical behavior of fish volatiles and microbial growth in stored Rohu fish through mathematical modeling. Total volatile basic nitrogen (TVB-N) is formed in stored Rohu (Labeo rohita) fish due to some complicated biochemical activities. It considered the biomass populace of volatiles (TVB-N) and microorganisms in fish stored at two different temperatures, separately. The different models may be used to forecast TVB-N, microbial populace (total viable count; TVC), and various properties change during nourishment stockpiling coordination and diverse preparing tasks. Models might be dynamic, exact, hypothetical, and stochastic in nature. Various parameters are required to build up a model which can be utilized to foresee the freshness and timeframe of realistic usability of storage duration. The ecosystem is represented by algebraic equations involving volatile compounds and microbial populations separately. TVB-N and TVC of stored rohu fish was determined at an interval of 4 days for 24 days. The initial and final biomass of TVB-N was 4.57 (fresh sample), 19.88 (24 days at 5°C), and 7.10 mg/100 g (24th day at 0°C), respectively. The TVC values were found to be 2.29 (fresh sample), 9.5 (24 days at 5°C) and 8.1 log (cfu/g) (24 days at 0°C). Exponential, modified exponential, Howgate, and adapted Howgate models were considered for modeling the TVB-N formation, whereas logistic, modified logistic, Gompertz, and modified Gompertz model were taken forward for modeling the microbial biomass developed in stored rohu fish. The exponential model found be the best fit model fit model for TVB-N prediction in rohu fish stored at 0 and 5°C as it showed the highest R2 (0.9796, 0.9887) the lowest χ2 (0.2782, 0.3976), RMSE (0.52741, 0.6306) AIC (−7.3122, −4.8106), AICc (−0.5122, 1.9894) and BIC (−7.4204, −4.9188), respectively. The Gompertz model was found to be the best fit model for microbial biomass prediction in rohu fish stored at 5°C (R2 = 0.9947, χ2 = 0.0537, AIC = −18.379, AICc = −6.3792 and BIC = −18.542), in contrast, both of the logistic and modified logistic models were the best suited at 0°C storage condition (R2 = 0.9919, χ2 = 0.0823).
Introduction
The increasing demand for fish is directly proportional to its nutritional values. Fish is an excellent food due to its high content of omega 3-fatty acids and proteins. Post-harvest fish losses (PHFL) are a major problem of concern that widely occurs across the world, resulting in less fish availability for the consumer due to which fish is either discarded or fetch a relatively low price due to deterioration in quality. PHFL starts after the death of fish when enzymatic, oxidative, and microbial-assisted physio-functional changes to begin to deteriorate the fish quality. Several factors like time, temperature and handling practices are the major factors that cause PHFL. Generally, raw food is initially contaminated with a wide variety of microorganisms during post-harvest handlings, but few of them can colonize, easily grow in food products, and spoil it. Different types of spoilage depend on the physical and chemical changes in the processing and packaging techniques of fish products (Gram and Huss, 1996). Specific and non-specific factors contribute to complex microbiological reactions and phenomena in seafood, leading to contamination before slaughtering and during product formation. The different intrinsic and extrinsic factors involving temperature, pH, and redox potential exert an electric effect on microbial growth, microbial inhibition, which decides the growth curve of microorganisms (Gram and Huss, 1996). Besides the factors pH, temperature, etc., other factors like the poikilothermic nature of the fish in the aquatic environment also influence the spoilage of the fish that can be dealt with in terms of high pH in the flesh of post-mortem fish and higher content of NPN (non-protein nitrogen). The presence of trimethylamine oxide (TMAO) as part of the NPN fraction after degradation during fish storage is generated as a volatile compound, i.e., trimethylamine (TMA). The volatile formation such as total volatile basic nitrogen (TVB-N) and TMA results from complex biochemical reactions such as microbiological and enzymatic etc. TVB-N, TMA, and other biogenic amines are the major chemical compounds which are used as a freshness index in fish (Tsironi and Petros, 2010; Prabhakar et al., 2019). As the amount of TMA increases, the TVB-N also increases rapidly; TMA is the compound responsible for starting and continuing this spoilage and fishy odor. During the bacterial growth in spoilage, bacteria establish themselves on the outer and inner surfaces of the live fish. Bacteria can easily grow over a wide range of temperatures due to this poikilothermic nature of fish. The main microorganisms present in temperate water where the fish interacts with are psychotropic Gram-negative, rod-shaped bacteria which have genera namely as Pseudomonas, Moraxella, Acinetobacter, Shewan Ella, Flavobacterium, Vibrionaceae and Aeroemonadaceae, but many gram-positive organisms are also found in different proportions such as Bacillus, Micrococcus, Clostridium, Lactobacillus and Corynebacterium. The recent establishment of the specific spoilage organism (SSOs) concept has contributed significantly to understand seafood spoilage. The different SSOs such as Shewanella putrifaciens (Iced marine fish), Pseudomonas spp. (Iced freshwater fish), Phtobacterium phspjoreum (CO2-packed chilled fish) are found in different fish and fisheries products and may be single species. These bacterial activities are also responsible for the breakdown of protein and other components of fish which results in the formation of biogenic amines such as trimethylamine (TMA), Dimethyl Amine (DMA), Ammonia (NH3), and TVB-N. The TMA, DMA, and NH3 are combined, known as TVB-N, which is considered a chemical indicator of further toxicity and spoilage. The amount of volatile formation is a function of fish species and variety (Whittle and Howgate, 2002). Marine species contain TMAO, which is a part of the NPN fraction and is present in all marine. Spoilage is influence by TMAO, especially in anaerobic conditions. Spoilage bacteria utilize TMAO in anaerobic respiration, an electron acceptor at the terminal point, which results in the formation of trimethylamine (TMA) as an end product with off odor and bad flavor (Dalgaard et al., 1993; Broekaert et al., 2012). Bacteria are considered spoilage indicators as they are the sole precursor required for reducing TMAO to TMA. During cold storage and frozen cold chain of fishes, intrinsic enzyme activity induces dimethylamine (DMA) from TMAO with the same molar quantities of formaldehyde equivalents. Many white fishes have their freshness measured through this method. However, most industries and institutes go for direct microbial counts as the source of marking fish freshness and assessing shelf life. The microbial analysis that has been conventionally carried out for decades is time-consuming and quite inconvenient (Peleg, 2016). TMA, DMA, and TVB-N lead to the direct assessment of the freshness of fish. Consequently, here an attempt is made to use rapid and mathematical tools for evaluating fish freshness as a replacement of traditional microbial methods of analysis. The major parameters that affect the formation of volatiles (TMA and TVB-N) are the duration of storage and temperature (Heising et al., 2014a; Peleg et al., 2014; Peleg, 2016). Functional relations can easily be understood in terms of a mathematical model.
The various physical and chemical changes in the fish are also reflected in many characteristics such as color, texture, tenderness, and flavor. The color and appearance of muscles directly influence the value and acceptance of most fish species (Chouhan et al., 2015). Color is also considered an indicator of freshness, influencing consumers' purchase decisions (Chouhan et al., 2015). Visual assessment is an important parameter affecting the presentation of fish and fish products and a product is generally selected upon its appearance, especially its color. Storage duration at different temperatures causes oxygenation and oxidation of myoglobin which forms oxymyoglobin in fish tissue (Mancini and Hunt, 2005). Metmyoglobin and oxymyoglobin are also associated with changes in the color of the fish filet. The conformational changes in the protein also impact the color change during iced and frozen storage of fish. The solubility of myofibrillar protein and sarcoplasmic protein is influenced by the storage duration and temperature (Mohan et al., 2006), which affects the visibility of Rohu. Degradation of muscle protein is a major problem associated with fish during storage (Mohan et al., 2006). These changes are brought either by enzymatic (endogenous/exogenous) or microbial-induced biochemical reactions during storage. These changes during fish storage play a vital role in the freshness assessment of the fish and fish products. Sensory rejection and sensory assessment based on total viable count (TVC) values of fish being 5.74 and 4.66 log CFU/g for chilling and partial freezing, respectively.
This study considered the biomass population of volatiles coming under TVB-N and microbial load in rohu fish stored at different temperatures. The various models have been used to predict microbial population, enzyme inactivation, and different properties changes during food storage, logistics, and different processing operations. Models may be kinetic, empirical, theoretical, and stochastic in nature. A model to depict the freshness of fishes requires many different parameters under it (Baranyi and Roberts, 1994; Husain et al., 2016). The fish freshness indicators based on kinetic and mathematical modeling can also be applied to design and develop an efficient supply chain for fish and related fish products. There is a difference in the rate of biochemical reactions of frozen and unfrozen fishes. The modeling for this dependence of temperature can be performed in several ways, such as the Arrhenius model, which has wide application in understanding physicochemical and biochemical changes in the food system. The dynamics of food quality also change highly regarding the change in molecular arrangement (Gudmundsson and Kristbergsson, 2009). In case of packed cod filets, a mathematical model has been developed which reports the formation of Trimethylamine (Heising et al., 2014a). Application of trial-and-error method, using approximate and guessed calculations along with other factors is used every time for calculation of the exact value of TMA by using all these equations. This model has enormous potential, which can link the sensor data of TMA in fish to the real status of the freshness of fish (Heising et al., 2014a). Most of the developed models are either to determine microbial biomass or compounds generated in the food matrix. The present study aimed to exercise the multi-model approach for the mathematical analysis of the TVB-N and microbial biomass dynamics.
Experimental Approach and Methods
Fish Handling and Storage Conditions
Live Rohu samples were collected (irrespective of the sex ratio) from a local fish farm pond (near IIT Kharagpur, West Bengal, India) having same cultural practices and rearing environment and then collected sample were transported to the lab in polybag containing farm pond water. Fish were kept free from stress before stunning death. The fish were killed by percussive stunning (involves hitting the fish's head with a wooden) the head for sudden death on 0-day means starting day of storage (Abbas et al., 2005; Jain et al., 2007). Random sampling was done such that three fishes were taken from all the lots, and their average length and weights were further measured and found to be 29.53 ± 2.32 cm and 620 ± 30 g, respectively. Rohu samples were covered by a layer of aluminum foil and kept at different storage temperatures (5 ± 1, 0 ± 1°C) for the study. The fish sample was stored at the required temperature for the study in the refrigerator, which was domestic (Whirlpool, FF2D258C/2016, Gurugram, India), A deep freezer (Voltas, 205 CF Metal Top, Delhi, India), and a quick microprocessor freezer (REMI, RQFVM-205D, Vasai, India) for 24 days. A multi-probe thermocouple (digital thermometer) was used to ensure the consistency of the required set temperature in every case.
Total Volatile Basic Nitrogen (TVB-N)
The volatile compound, TVB-N were estimated by the method executed by Prabhakar et al. (2019) i.e., the Conway micro-diffusion method referred by FSSAI (2015) manual (Chouhan et al., 2015) with partial modification. However, some other routine methods may be used to check the TVB-N limit, such as the direct distillation method described by Conway, Antonacopoulos (1968) and the distillation of an extract deproteinized trichloroacetic acid reported by FAO/WHO through FAO/WHO (1968). As per FSSAI (2015) manual, 5 grams of fish muscle taken from stored fish was homogenized with 45 mL of distilled water. 5 ml of this homogenate were added with an equal volume of 10% trichloroacetic acid (TCA) (w/v) and then filtered through Whatman's filter paper no. 1. 1 ml of this extract and saturated potassium carbonate each was pipetted into the outer ring of the micro-diffusion unit containing 1 ml inner ring solution. Inner ring solution was prepared by mixing 2% boric acid (92 ml), 0.1% alcoholic bromocresol (4 ml), and 0.1% alcoholic methyl red (4 ml). The covered and sealed unit was rotated gently to mix the solutions of the outer ring. The unit was incubated for 180 mins at 37°C and cooled at room temperature. 0.02 N sulfuric acid was used for titration of the inner ring solution until it turned pink. Then, 1 ml of 10% trichloroacetic acid was taken for a blank test instead of sample extract. The same procedure was adopted for TMA-N determination as TVB-N, but 1 ml of formalin was added before adding potassium carbonate to the extract to react with ammonia, allowing only the TMA-N to diffuse over the unit. Each experiment was replicated three times for every storage condition, and the obtained TVB-N and TMA-N results were expressed in mg/100 g of sample. These TVB-N and TMA-N obtained data were used for its kinetic study.
Microbiological Analysis
In general, the number of spoilage bacteria (total bacterial count or specific bacterial) and level of some biochemical indexes (TVB-N) are used to evaluate the freshness of fish. However, as per Chouhan et al. (2015), Fan et al. (2008), and Prabhakar et al. (2020), the total viable count (TVC) was considered to be calculated to explore the microbiological quality of fish and fish products. Therefore, a microbiological quality analysis was done as per Downes and Ito (2001) with minor modification. 10 g of Rohu muscle was weighed aseptically into a sterile sample dish and homogenized with 90 ml of sterile physiological saline (0.854% NaCl) for 3 mins. using a sterile pipette, 1 ml of the supernatant was aseptically transferred into a 9 ml saline tube and mixed well-using vortex mixer. Similarly, further dilutions were prepared for the inoculation. 1 ml each of the appropriate dilutions was made and poured onto agar by spread plate technique. The plates were incubated for 48 h at 37°C in an inverted position. Total viable count (TVC) was enumerated and expressed as log10 cfu/g of the sample. The experiment was conducted at an interval of 4 days.
Mathematical Modeling: Parameter Estimation and Statistical Analysis
Many biological reactions in fish, such as volatile formations (TVB-N, TMA-N, and other biogenic amines) and vitamins loss during isothermal storage and processing operation, probably follow fixed order of kinetics (Peleg, 2016). The formation of the TVB-N and microbial population in stored Rohu was modeled using different models (Table 1) that all set of algebraic equations (empirical and phenomenological models). In the present study, all analyses (TVB-N and microbial biomass) were done in triplicates, and readings were expresses as average values ± SD. The biomass mass data (TVB-N and microbial population) were fitted to different models (Table 1) using non-linear regression analysis through the ORIGIN 2021 software (Origin Lab Corporation, Northampton, USA). The suitability and fitness of models were examined based on the coefficient of determination (R2), chi-square (χ2), and root mean square error (RMSE). However, secondary modeling, i.e., the Arrhenius model was not for the temperature sensitivity analysis of microbiological and chemical changes in the stored fish because the study has been made at only two temperatures.
Where, n is the number of experiments; Yi,e is the experimental value of the ith experiment; Yi,p is the predicted value of the nth experiment by model; and Ye is the average value of experimentally determined values.
Along with the above statistical analysis, models were also discriminated using statistical analysis of the residuals and Akaike information criterion (AIC) and corrected Akaike information criterion (AICc), which were calculated from Equations 5, 6 (Van Boekel, 2009; Heising et al., 2014a). AICs is AIC with a correction for small sample sizes specially when the sample size is more than the number of model parameters. The lowest values of AIC and AICs are preferred for selecting the best fit model among all models.
Where, SSr is the residual sum of squares, n is the number of observations, and p is the number of parameters.
Bayesian information criterion (BIC) is also a criterion for model selection among a finite set of models. It penalizes the complexity of the model, which refers to the number of parameters in the model. The lowest value is preferred for the selection of the model. BIC can be calculated by using Equation 7.
Mathematical Modeling: Theoretical Status, Experimental Results and Discussion
Theoretical Status of Volatile Formation, Modeling and Parameterization
The results obtained in terms of TVB-N and microbial population from the storage study were used to consider existing empirical models (growth/kinetic) from literature (Table 1) to explore its suitability for TVB-N and Microbial biomass development. Thereafter, parameters were estimated and compared with other validated models from the literature, and the temperature effect was also explored. TVB-N formed in fish during storage is due to some microbial metabolites responsible for off-flavors and off-odors (Cheng et al., 2015; Prabhakar et al., 2019). These metabolites may be TMA-N, ammonia, and DMA. The total volatile base-nitrogen (TVB-N) index combines these amines into a unique indicator used to assess the spoilage of fresh fish. In fact, the content of TVB-N is the only biochemical index presently included in EU regulations to discriminate fit from unfit fish in commerce or an official inspection. Neither TVB-N nor trimethylamine (TMA) content can be used as indexes of quality in the early stages of storage (Dalgaard et al., 1993; Howgate, 2010a). The Codex Alimentarius Committee (CAC) and FAO/WHO (1968) have recommended TVB-N as a regulatory compound for deciding the freshness of different fish species. TVB-N is also associated with the activity of endogenous enzymes (Kyrana et al., 1997; Vareltzis et al., 1997). According to Connell (1990), spoiled fish exhibit a TVB-N level above 35 mg N/100 g. The formation of TVB-N is also the function of fish species. The European Commission (European Union Law 95/149/EC, 1995) has specified TVB-N limits for different species, such as 25 mg/100 g for Sebastes spp. (Helicolenus dactylopterus, Sebastichthys capensis), 30 mg/100 g for species belonging to the Pleuronectidae family (except halibut: Hippoglossus spp.), and 35 mg/100 g for Salmo salar. On the other hand, storage conditions (time and temperature) affect the shelf life and quality of fish (Fan et al., 2008) and can increase the formation of TVB-N, which is a significant cause of spoilage of Rohu fish (Mehta et al., 2014). According to Connell (1990), the permissible limit of the TMA-N level should be lower than 35 mg N/100 g, although this limit varies from species to species. Malle and Poumeyrol (1989) reported TMA-N values of 10–15 mg/100 g in aerobically stored fish and at a level of 30 mg TMA-N/100 g in packed cod. O'zogul et al. (2004) reported that the concentrations of TMA-N in numerous fatty fish never reached the limit of 5 mg TMA-N/100 g. However, the rejection limit in fish flesh is usually higher. Therefore, TMA-N formation in stored fish depends upon species, fish composition, storage temperature, duration, and local environmental composition. However, some studies have found significant correlations between TVB-N, TMA, and storage time (Baixas-Nogueras et al., 2001; Ruiz-Capillas and Moral, 2001). Storage conditions like temperature and duration influence the volatiles formation and other biochemical changes in fish caused by either microbial or enzymatic activities. Spoilage bacteria are selected primarily due to the physical and chemical conditions in the products; however, seafood spoilage involves the growth of the microorganism to a high number (>106-107cfu/g) of antagonisms or symbiosis between the different groups of microorganisms may influence their growth and metabolism (Gram and Dalgaard, 2002).
The majority of quality and shelf-life models in the literature focus on the most probable prediction. There is a huge literature on fish spoilage, technological, economic, and safety implications, and a continuous attempt has been made to monitor the spoilage in quantitative terms. Microbial counts are probably the most direct method, which is generally exercised using a traditional technique that involves incubation. It is very time-consuming and logistically inconvenient (Peleg, 2016). A mathematical tool can be applied to replace this direct method for rapid determination of microbial population, which predicts the shelf life (Heising et al., 2014a; Prabhakar et al., 2019). The various models (for TVB-N and microbial biomass) selected based on literature and the data pattern were analyzed for experimental data obtained in the present study. A variety of mathematical models has described microbial growth. Their properties and how well they fit and predict and predict experimental growth data are discussed by Peleg and Corradini (2011). Most of the empirical models are based on physical and biochemical principles of mass transport in stored fish. Adapted Howgate model uses a mathematical model framework for sensor response of intelligent packaging. Therefore, indicating that the modeling of TVB-N dynamics could be used to predict shelf life. For the volatile formation, a wide range of models starting from complete exponential to asymptotic behavior has been used to explain the dynamics (Van Boekel, 2008; Heising et al., 2014b; Garcia et al., 2017). Furthermore, models having asymptotic nature such as logistic, modified logistic, Gompertz, and modified Gompertz were found to be extensively used in the literature (Baranyi and Roberts, 1994; Giannuzzi et al., 1998). Based on this, these models were also considered for the present study with a hypothesis that asymptote is expected.
Modeling of TVB-N and Effect of Temperature on Model Parameters
The experimental TVB-N values during storage of Rohu fish at an interval of 4 days were modeled to find the best empirical models. The most common TMA-N or TVB-N formation model is usually an exponential model, i.e., Equation 8 (Tsironi et al., 2009; Tsironi and Petros, 2010; Yao et al., 2011; Garcia et al., 2017).
Where, C stands for TVB-N content, k is the velocity of the reaction, and t describes the time elapsed since the fish arrived for the market (days). C0 is the TVB-N content at t = 0, i.e., when the fish arrives to the storage. The experimental data of TVB-N were fitted to an exponential model (Equation 8). Model and statistical parameters were represented in Table 2. As per the fitted exponential model, the predicted initial concentration (C0) of TVB-N was found to be closer to the sample stored at 5°C (5.69 ± 0.3102 mg/100 g) than 0°C (5.29 ± 0.2825 mg/100 g), which showed that it was independent of temperature. Figure 1 showed the exponential model curve having coefficient of determination (R2 = 0.9887 and 0.9796), Chi-square (χ2 = 0.3976 and 0.2782). The values of R2 are quite high, and χ2 are low, indicating its goodness of fit for the dynamical understanding of TVB-N under experimental conditions of the present study. The reaction rate, i.e., k-values, were 0.053 ± 0.003 and 0.042 ± 0.003 day−1 for the TVB-N formation of the Rohu sample stored at 5°C and 0°C, respectively. k values indicated that lower storage temperature would have arrested the reaction, slowing the reaction velocity. Figure 1 showed that there was a continuous increasing pattern of TVB-N formation throughout the storage period, irrespective of the demarcation of slow and advanced spoilage, without reaching and explaining a maximum asymptote. Howgate (2010a); Heising et al. (2014b) critically pointed out that the critical drawback of the conventional model of TVB-N content is their inability to produce the initial dwell and the exponential behavior.
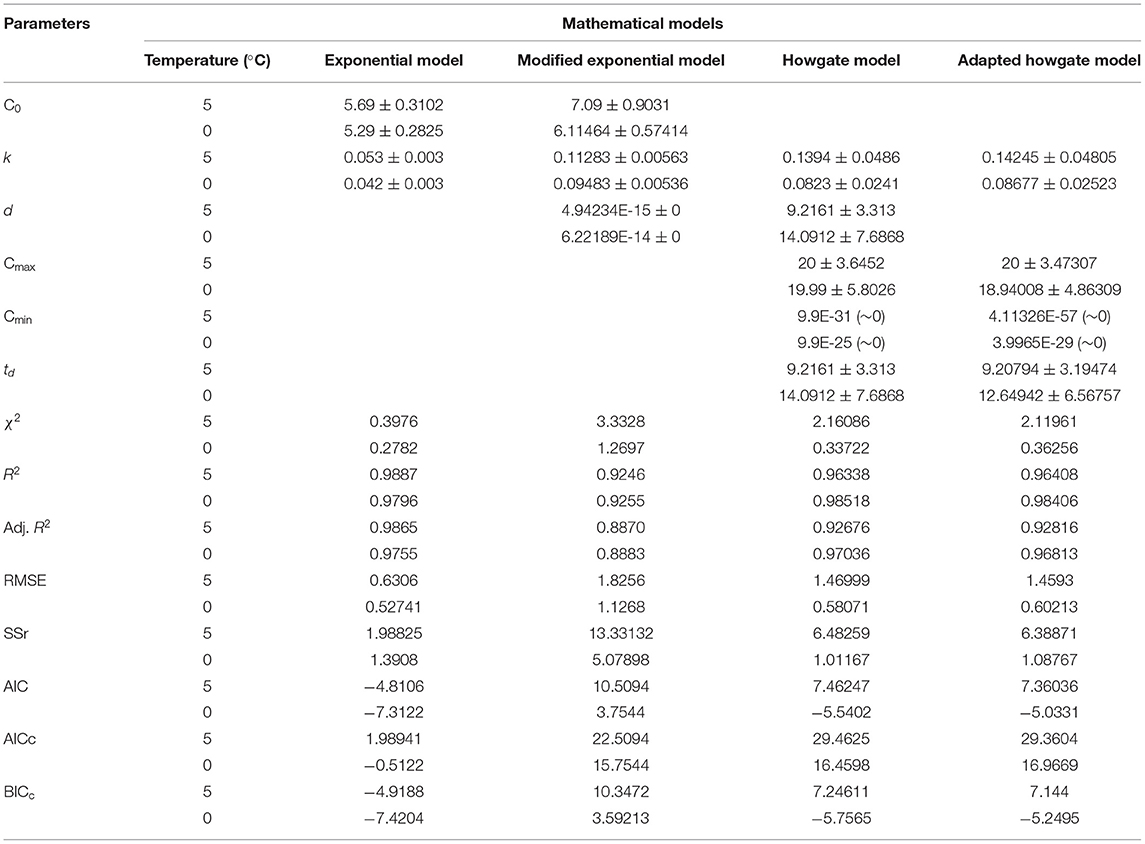
Table 2. Model and statistical parameters of different empirical models for TVB-N formation in stored Rohu fish.
Heising et al. (2014b) postulated that the volatile formation in fish and fish products stored in ice or refrigerated conditions from the harvesting/catch often starts with a period in which the volatiles like TMA-N was not increased. It was followed by another phase with a rapid increase in the TMA-N concentration, followed by a third phase in which the volatile formation rate diminishes. The TVB-N is comprised of TMA-N, DMA-N, and NH3 gas, and its formation is a function of enzymatic and bacterial actions influenced by storage conditions (Huss, 1995; Prabhakar et al., 2020). Nevertheless, the exponential model is sufficient enough to simulate the TVB-N formation in all phases and stages. Though the fitting of exponential mode is good, as attributed by R2 and χ2 values. Keeping these facts, another model, such as the modified exponential model (Equation 9), was considered for modeling the dynamics of TVB-N formation.
Moreover, this model was suggested by Howgate (2010b) for the TMA-N formation in fish stored on ice. The parameter “d” represents the dwell (days), and C0 describes the TVB-N concentration at time t = 0. A logistic model, which is an advanced form of the exponential model, was found to have a good fit with the present TVB-N experiment values as (R2 = 0.9246 and 0.9255), and chi-square (χ2 = 3.3328 and 1.2697) these statistical parameters showed less fit in comparison to exponential model (Equation 8).
Modified exponential model (Equation 9) attributed a dwell period of 4.94 × 10−15 days at 5°C, and a 6.22 × 10−14 day at 0°C, which was negligible, which can be understood through the logistic model curve (Figure 2), the corresponding k values were found to be 0.11283 ± 0.006 and 0.09483 ± 0.005 day−1. The present findings are in line with the trend of fishy odor formation in packed codfish filets at varied storage temperatures (0, 5, 10, 15°C) which were reported by Heising et al. (2014a).
It was inferred that this modified exponential model (Equation 9) doesn't include and describe a parameter for maximum concentration of TVB-N. Thus, the model increases exponentially even under the advanced spoilage phase (Howgate, 2010b; Heising et al., 2014b). This equation only uses for the initial phase of volatile formation. For minimum and maximum asymptotic concepts, other models such as the Howgate (Equation 10) and adapted Howgate model (Equation 11) were considered for fitting analysis under present experimental conditions. The parameters Cmax represents upper asymptote concentration (mg TVB-N per 100 g fish muscle), Cmin lower asymptote concentration (mg TVB-N per 100 g fish). This equation gives the asymptote value of Cmax-Cmin for a higher value of t. It will give a lower value of CTVB−N at the same time t and Cmax since the value of the numerator decreases with increasing Cmin. The data were modeled using Equations 10, 11 considering Cmin > 0, k > 0, td > 0, and t > 0; otherwise, it will make an unrealistic situation. The data of TVB-N under both storage conditions and the Cmax were parameterized for Howgate and adapted Howgate model as 20 ± 3.6452 and 20 ± 3.4707 mg/100 at 5 °C which are very close to each other and, for 0°C, Cmax values were estimated to be 19.99 ± 5.8026 and 18.94 ± 4.8631 mg/100 g for the respective model.
The points of inflection (td) ranged between 9.2161 ± 3.313 to 14.0912 ± 7.6868 days in the Howgate model (Equation 10), whereas it was ranged from 9.2079 ± 3.1947 to 12.6494 ± 6.5675 days for the adapted Howgate model (Equation 11). The estimated statistical parameters like R2 ranged from 0.96338 to 0.98518 for the Howgate model. For the adapted Howgate model, R2 values were found to be 0.9640 and 0.9840. The k-values of the Howgate model were 0.1394 ± 0.0486 and 0.0823 ± 0.0241 day−1 whereas, for the adapted Howgate model, it was found 0.14245 ± 0.0450 and 0.08677 ± 0.0252 day−1. The graphical pattern of TVB-N formation in the model curve of Howgate and adapted Howgate model was present in Figures 3, 4. As a result, the exponential model was found to be the best fit model for predicting TVB-N biomass in rohu fish stored at 0°C and 5°C. The exponential model fitting showed R2 (0.9796, 0.98887) Adj. R2 (0.9755; 0.9865), the lowest χ2 value (0.3976, 0.2782), RMSE (0.52741, 0.6306), AIC (−7.3122, −4.8106), AICc (−0.5122, 1.9894) and BIC (−7.4204, −4.9188) and thus this can be selected as the best fit model for TVB-N prediction in rohu fish stored at 0 and 5°C, respectively. After a complete interpretation, it was found that the TVB-N curve was not captured fully the whole storage phenomena, where it was hypothesized that an asymptote is expected. The asymptote model could be a better fit for this study, although no asymptote is observed.
Modeling of Microbial Growth and Effect of Temperature on Model Parameters
Predictive microbiology is now a possible alternative to traditional microbiological assessment of food safety and quality. Various environmental factors on the growth of microorganisms have since been quantified in laboratory media, and kinetic models have been developed (Dalgaard and Huss, 2020). However, for most fish products, the microorganisms responsible for spoilage are not known. Furthermore, the spoilage microflora is also likely to change when storage conditions (e.g., temperature and atmosphere) are changed over wide ranges. This dynamic nature of the microbial spoilage process indicates that specific spoilage organisms' spoilage domains must be determined before kinetic models can be developed. Sometimes, interpreting growth curves and growth parameters of non-specific microbial groups is always a complex undertaking since they represent the sum of growth curves of a mix of different microorganisms. Nevertheless, in the present study, specific spoilage bacteria were not determined; instead, total viable count (TVC) was calculated for dynamical analysis. At the first stage, three-parameter models (logistic, modified logistic, and Gompertz) and after that, four-parameter models such as modified Gompertz were fitted to microbial experimental data of stored Rohu. Figures 5–8 represented the logistic, modified logistic, Gompertz, and modified Gompertz model curve. All the model equations are appended below:
The logistic model (Equation 12), initially proposed by Verhulst in the 18th centuries, is been a widely used model of organisms' growth dynamics in a finite resource habitat. It has opened the framework of more elaborative models like Baranyi and Roberts (1994) and others (Peleg et al., 2007). It represents the momentary growth rate of a microbial population in a virgin environment. There is a lot to explore about the habitat and resources. Thus, this study considered this model as a comparative mode and further exploitation of multi-parametric models as deemed fit to explore the microbial growth dynamics in stored rohu fish.
Parameter “a” represents the difference of microbial biomass at lower and higher asymptote of Equation 12. The k is for growth rate, and c describes the carrying capacity of the biomass dynamics, and M is the microbial population after time t. Another modification like the modified logistic model is conceptualized by introducing another parameter like “b” (b > 0) depends upon temperature and other environmental factors; c without carrying capacity.
A general Gompertz model expression (Equation 14) of three parameters was used to understand the microbial biomass during rohu fish storage as the Gompertz model and Baranyi and Roberts (1994) have been extensively used in the literature. The further extension of the Gompertz model in the form of modified Gompertz equation, as reported previously (Zwietering et al., 1991). Equation 15 represents the modified Gompertz model having some response factors such as lag phase duration (tl) and maximal growth rate (μmax). This model has four parameters that can explain the storage conditions having an influence on the rohu fish.
The data presented in Table 3 imply the model and statistical parameters estimated after data fitting to different models (Equations 12, 13, 14, and 15). In light of this literature, discussion, and criticisms, all these models were considered curve fitting analysis to understand the dynamics of microbiological assisted volatile formation to find the best fit model for predicting the freshness of stored Rohu fish under limited controlled refrigerated storage. The values range varied from 9.5031 ± 0.3533 to 5.8469 ± 0.4564, 9.5031 ± 0.3534 to 8.5469 ± 0.4564, 10.0799 ± 0.4297 to 9.4356 ± 0.9688 and 5 ± 3.190 to 5 ± 2.455 for logistic and Gompertz and modified Gompertz model. The Gompertz model's carrying capacity (c) was found to be ranged from 3.3349 ± 0.4799 and 4.4769 ± 1.2541. The maximal growth rate (μmax) values were 0.4602 ± 0.41115 and 0.3631 ± 0.1772 day−1 for the rohu samples stored at 5 and 0°C. The statistical parameters of model fitting ranged from 0.0823 to 0.0769, 0.0823–0.0769, 0.0537–0.1071 and 2.0169–0.6329 (χ2) and 0.9919–0.9899, 0.2291–0.9899, 0.9947–0.9859 and 0.8518–0.9379 (R2). From the analysis of model parameters, the Gompertz model was found to be the best fit model for microbial biomass prediction in rohu fish stored at 5°C (R2 = 0.9947, χ2 = 0.0537), having lowest AIC (−18.379), AICc (−6.3792), and BIC (−18.542). In contrast, both of logistic and modified logistic models were best suited at 0°C storage condition (R2 = 0.9919, χ2 = 0.0823, RMSE = 0.28683) as it showed the lowest AIC (−16.342), AICc (−3.4013) and BIC (−14.123) values. It has been evident from the literature that most researchers have modeled the microbial growth of specific species and strains. Antunes-Rohling et al. (2019) studied modeling of microbial growth in terms of different microbial groups such as Aerobic Mesophiles, Anaerobic Mesophiles, Aerobic Psychrotrophes, Anaerobic Psychrotrophes, Pseudomonas, Shewanella, Lactic Acid Bacteria, Enterobacteriaceae, Molds, and Yeasts. He examined these analyses in modified-atmosphere-packed hake (Merluccius merluccius) filets stored at different temperatures ranging from 1–10°C. The present study's model curve and statistical parameters were found in line with Antunes-Rohling et al. (2019). Moreover, it is recommended to explore further growth modeling of a specific group of microorganisms under more range of storage temperatures. Overall, as a future study, it is recommended to go for higher no of data points (days of storage) to make the modeling process more robust and highly significant.
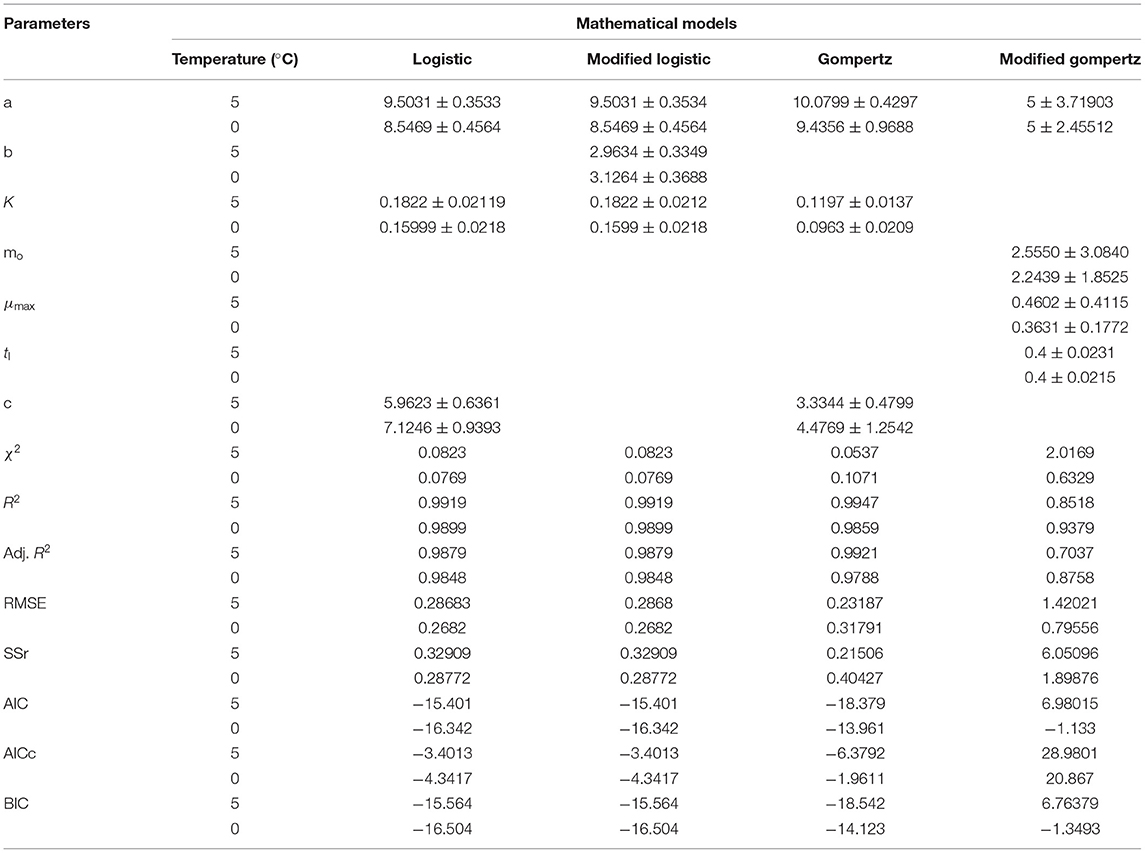
Table 3. Model and statistical parameters of different empirical models for microbial growth in stored Rohu fish.
Concluding Remarks
In this study, mathematical models for the formation of TVB-N and microbial biomass were studied in order to predict the freshness of rohu fish stored under 0 and 5°C. The model fitting and its analysis was done along with the estimation of statistical and model parameters. Based on curve fitting statistical parameters, an exponential model was found the best fit model for predicting TVB-N biomass in rohu fish stored at 0 and 5°C. The exponential model fitting showed the highest R2, the lowest χ2, AIC, AICc, and BIC. The Gompertz model was found to be the best fit model for microbial biomass prediction in rohu fish stored at 5°C. Whereas, logistic and modified models were best suited for 0°C storage conditions. The finding of this study can be helpful in the prediction of the freshness of rohu fish. In addition, it may help in designing and understanding cold chain logistics. It is to ponder that new research and technological development can make such measurement and mathematical analysis more accessible, faster, and non-destructive. These analyzed models under the present experimental conditions would produce a reliable statistic of the preliminary and initial conditions. However, TVB-N formation is the primary function of microbial and enzymatic actions. In the future, assuming no enzymatic conditions, a separate mechanistic model may be developed, which would predict TVB-N as a function of the microbial population.
Data Availability Statement
The raw data supporting the conclusions of this article will be made available by the authors, without undue reservation.
Ethics Statement
Ethical review and approval was not required for this animal study, in accordance with the local legislation and institutional requirements.
Author Contributions
PP: conceptualization, experimentation, data curation, analysis, and writing original draft. PS and SP: supervision, data analysis, and editing. KD: editing and analysis. All authors contributed to the article and approved the submitted version.
Conflict of Interest
The authors declare that the research was conducted in the absence of any commercial or financial relationships that could be construed as a potential conflict of interest.
Publisher's Note
All claims expressed in this article are solely those of the authors and do not necessarily represent those of their affiliated organizations, or those of the publisher, the editors and the reviewers. Any product that may be evaluated in this article, or claim that may be made by its manufacturer, is not guaranteed or endorsed by the publisher.
Acknowledgments
The authors acknowledge the Agricultural and Food Engineering Dept., IIT Kharagpur, and Food Science and Technology Dept., NIFTEM, Kundli, India, for providing necessary assistance for accomplishing the work.
References
Abbas, K. A., Mokhtar, A. S., Sapuan, S. M., and Mawlood, M. K. (2005). Quality deterioration of Malaysian patin fish during cold storage. J. Food Agric. Environ. 3, 88–91.
Antonacopoulos, N. (1968). “Handbuch der Lebensmittelchemie,” in Bd III/2, ed I. Acker (Berlin: Springer), 1493–1494.
Antunes-Rohling, A., Artaiz, Á., Calero, S., Halaihel, N., Guillén, S., Raso, J., et al. (2019). Modelling microbial growth in modified-atmosphere-packed hake (Merluccius merluccius) fillets stored at different temperatures. Food Res. Int. 122, 506–516. doi: 10.1016/j.foodres.2019.05.018
Baixas-Nogueras, S., Bover-Cid, S., Vidal-Carou, M. C., and Veciana-Nogues, M. T. (2001). Volatile and non-volatile amines in Mediterranean hake as function of their storage temperature. J. Food Sci. 66, 83–88. doi: 10.1111/j.1365-2621.2001.tb15586.x
Baranyi, J., and Roberts, T. A. (1994). A dynamic approach to predicting bacterial growth in food. Int. J. Food Microbiol. 23, 277–294. doi: 10.1016/0168-1605(94)90157-0
Broekaert, K., Noseda, B., Heyndrickx, M., Vlaemynck, G., and Devlieghere, F. (2012). “The spoilage microbiota of ray (Raja sp.) during ice storage under different conditions: molecular identification and characterization of the spoilage potential,” in Molecular Identification of the Dominant Microbiota and Their Spoilage Potential of Crangon Crangon and Raja Sp. (Ghent: Ghent University), 93–118.
Cheng, J. H., Sun, D. W., Zeng, X. A., and Liu, D. (2015). Recent advances in methods and techniques for freshness quality determination and evaluation of fish and fish fillets: a review. Crit. Rev. Food Sci. Nutr. 55, 1012–1225. doi: 10.1080/10408398.2013.769934
Chouhan, A., Kaur, B. P., and Rao, P. S. (2015). Effect of high-pressure processing and thermal treatment on quality of hilsa (Tenualosailisha) fillets during refrigerated storage. Innov. Food Sci. Emerg. Tech. 29, 151–160. doi: 10.1016/j.ifset.2015.03.016
Connell, J. J. (1990). “Methods of assessing and selecting for quality,” in Control of Fish Quality, 3rd Edn, ed J. J. Connell (Oxford: Fishing News Books), 122–150.
Dalgaard, P., Gram, L., and Huss, H. H. (1993). Spoilage and shelf-life of cod fillets packed in vacuum or modified atmospheres. Int. J. Food Microbiol. 19, 283–294. doi: 10.1016/0168-1605(93)90020-H
Dalgaard, P., and Huss, H. H. (2020). “Mathematical modeling used for evaluation and prediction of microbial fish spoilage,” in Seafood Safety, Processing, and Biotechnology, eds F. Shahidi, Y. Jones, and D. D. Kitts (Boca Raton, FL: CRC Press), 73–89. doi: 10.1201/9781003075899-10
Downes, F. P., and Ito, K. (2001). Compendium of Methods for the Microbiological Examination of Foods, 4th Edn. Washington, DC: American Public Health Association. doi: 10.2105/9780875531755
European Union Law 95/149/EC. (1995). Available online at: http://eur-lex.europa.eu/LexUriServ/LexUriServ.do?uri=CELEX:31995D0149:~en:NOT (accessed January 24, 2021).
Fan, W., Chi, Y., and Zhang, S. (2008). The use of a tea polyphenol dip to extend the shelf life of silver carp (Hypophthalmicthys molitrix) during storage in ice. Food Chem. 108, 148–153. doi: 10.1016/j.foodchem0.2007.10.057
FAO/WHO (1968). “Method for the determination of total volatile basic nitrogen (TVB) in fish muscle,” Proceedings of the Presented to the Codex Committee on Fish and Fishery Products, 3rd session (Bergen). doi: 10.1055/s-0028-1105114
FSSAI (2015). Manual of Methods of Analysis of Foods: Meat and Meat Products and Fish and Fish Products. Lab Manual 6. New Delhi: Food Safety and Standards Authority of India.
Garcia, M. R., Cabo, M. L., Herrera, J. R., Ramilo-Fernández, G., Alonso, A. A., and Balsa-Canto, E. (2017). Smart sensor to predict retail fresh fish quality under ice storage. J. Food Eng. 197, 87–97. doi: 10.1016/j.jfoodeng.2016.11.006
Giannuzzi, L., Pinotti, A., and Zaritzky, N. (1998). Mathematical modelling of microbial growth in packaged refrigerated beef stored at different temperatures. Int. J. Food Microbiol. 39, 101–110. doi: 10.1016/S0168-1605(97)00127-X
Gram, L., and Dalgaard, P. (2002). Fish spoilage bacteria–problems and solutions. Curr. Opin. Biotech. 13, 262–266. doi: 10.1016/S0958-1669(02)00309-9
Gram, L., and Huss, H. H. (1996). Microbiological spoilage of fish and fish products. Int. J. Food Microbiol. 33, 121–137. doi: 10.1016/0168-1605(96)01134-8
Gudmundsson, G., and Kristbergsson, K. (2009). Shelf-life prediction of chilled foods. Pred. Model. Risk Assess. 4, 101–119. doi: 10.1007/978-0-387-68776-6_6
Heising, J. K., Bartels, P., Van Boekel, M. A. J. S., and Dekker, M. (2014b). Non-destructive sensing of the freshness of packed cod fish using conductivity and pH electrodes. J. Food Eng. 124:80e85. doi: 10.1016/j.jfoodeng.2013.10.008
Heising, J. K., Van Boekel, M. A. J. S., and Dekker, M. (2014a). Mathematical models for the trimethylamine (TMA) formation on packed cod fish fillets at different temperatures. Food Res. Int. 56, 272–278. doi: 10.1016/j.foodres.2014.01.011
Howgate, P. (2010a). A critical review of total volatile bases and trimethylamine as indices of freshness of fish. Formation of the bases, and application in quality assurance. Elec. J. Env. Agricult. Food Chem. 9(Pt 2), 29–57.
Howgate, P. (2010b). A critical review of total volatile bases and trimethylamine as indices of freshness of fish. Determination. Elec. J. Env. Agricult. Food Chem. 9(Pt 1), 29–57
Husain, R., Suparmo, H. E., and Hidayat, C. (2016). Kinetic oxidation of protein and fat in snapper (Lutjanus sp) fillet during storage. AIP Conf. Proc. 1755:050006. doi: 10.1063/1.4958489
Jain, D., Pathare, P. B., and Manikantan, M. R. (2007). Evaluation of texture parameters of Rohu fish (Labeo rohita) during iced storage. J. Food Eng. 81, 336–340. doi: 10.1016/j.jfoodeng.2006.11.006
Kyrana, V. R., Lougovois, V. P., and Valsamis, D. S. (1997). Assessment of shelf life of maricultured gilthead sea bream (Sparus aurata) stored in ice. Int. J. Food. Sci. Tech. 32, 339–347. doi: 10.1046/j.1365-2621.1997.00408.x
Malle, P., and Poumeyrol, M. (1989). A new chemical criterion for the quality control of fish: trimethylamine/total volatile basic nitrogen (%). J. Food Prot. 52, 419–423. doi: 10.4315/0362-028X-52.6.419
Mancini, R. A., and Hunt, M. (2005). Current research in meat color. Meat Sci. 71, 100–121. doi: 10.1016/j.meatsci.2005.03.003
Mehta, N. K., Elavarasan, K., Reddy, A. M., and Shamasundar, B. A. (2014). Effect of ice storage on the functional properties of proteins from a few species of fresh water fish (Indian major carps) with special emphasis on gel forming ability. J. Food Sci. Tech. 51, 655–663. doi: 10.1007/s13197-011-0558-y
Mohan, M., Ramachandran, D., and Sankar, T. V. (2006). Functional properties of Rohu (Labeo rohita) proteins during iced storage. Food Res. Int. 39, 847–854. doi: 10.1016/j.foodres.2006.04.003
O'zogul, F., Polat, A., and O'zogul, Y. (2004). The effects of modified atmosphere packaging and vacuum packaging on chemical, sensory and microbiological changes of sardines (Sardina pilchardus). Food Chem. 85, 49–57. doi: 10.1016/j.foodchem.2003.05.006
Peleg, M. (2016). A kinetic model and endpoints method for volatiles formation in stored fresh fish. Food Res. Int. 86, 156–161. doi: 10.1016/j.foodres.2016.06.004
Peleg, M., and Corradini, M. G. (2011). Microbial growth curves: what the models tell us and what they cannot. Crit. Rev. Food Sci. Nutr. 51, 917–945. doi: 10.1080/10408398.2011.570463
Peleg, M., Corradini, M. G., and Normand, M. D. (2007). The logistic (Verhulst) model for sigmoid microbial growth curves revisited. Food Res. Int. 40, 808–818. doi: 10.1016/j.foodres.2007.01.012
Peleg, M., Mark, D. N., and Amy, D. K. (2014). Estimating thermal degradation kinetics parameters from the endpoints of non-isothermal heat processes or storage. Food Res. Int. 66, 313–324. doi: 10.1016/j.foodres.2014.10.003
Prabhakar, P. K., Srivastav, P. P., and Pathak, S. S. (2019). Kinetics of total volatile basic nitrogen and trimethylamine formation in stored Rohu (Labeo rohita) fish. J. Aquat. Food Prod. Technol. 28, 452–464, doi: 10.1080/10498850.2019.1604598
Prabhakar, P. K., Vatsa, S., Srivastav, P. P., and Pathak, S. S. (2020). A comprehensive review on freshness of fish and assessment: analytical methods and recent innovations. Food Res. Int. 133:109157. doi: 10.1016/j.foodres.2020.109157
Ruiz-Capillas, C., and Moral, A. (2001). Correlation between biochemical and sensory quality indices in hake stored in ice. Food Res. Int. 34, 441–447. doi: 10.1016/S0963-9969(00)00189-7
Tsironi, T., Dermesonlouoglou, E., Giannakourou, M., and Taoukis, P. (2009). Shelf-life modelling of frozen shrimp at variable temperature conditions. LWT Food Sci. Technol. 42:664e671. doi: 10.1016/j.lwt.2008.07.010
Tsironi, T. N., and Petros, S. T. (2010). Modeling microbial spoilage and quality of gilthead seabream fillets: combined effect of osmotic pretreatment, modified atmosphere packaging, and nisin on shelf life. J. Food Sci. 75, 243–251. doi: 10.1111/j.1750-3841.2010.01574.x
Van Boekel, M. A. J. S. (2008). Kinetic modeling of food quality: a critical review. Comp. Rev. Food Sci. Tech. 7, 144–158. doi: 10.1111/j.1541-4337.2007.00036.x
Van Boekel, M. A. J. S. (2009). Kinetic Modeling of Reactions in Foods. Boca Raton, FL: CRC Press. doi: 10.1201/9781420017410
Vareltzis, K., Koufidis, D., Gavriilidou, E., Papavergou, E., and Vasiliadou, S. (1997). Effectiveness of a natural Rosemary (Rosmarinus officinalis) extract on the stability of filleted and minced fish during frozen storage. Z. Lebensm. Unters. Forsch. 205, 93–96. doi: 10.1007/s002170050131
Whittle, K. J., and Howgate, P. (2002). Glossary of Fish Technology Terms. Rome: Food and Agriculture Organization of the United Nations.
Yao, L., Luo, Y., Sun, Y., and Shen, H. (2011). Establishment of kinetic models based on electrical conductivity and freshness indictors for the forecasting of crucian carp (Carassius carassius) freshness. J. Food Eng. 107:147e151. doi: 10.1016/j.jfoodeng.2011.06.034
Keywords: empirical model, growth dynamics, microbial population, rohu (Labeo rohita), Total volatile basic nitrogen (TVBN)
Citation: Prabhakar PK, Srivastav PP, Pathak SS and Das K (2021) Mathematical Modeling of Total Volatile Basic Nitrogen and Microbial Biomass in Stored Rohu (Labeo rohita) Fish. Front. Sustain. Food Syst. 5:669473. doi: 10.3389/fsufs.2021.669473
Received: 18 February 2021; Accepted: 16 September 2021;
Published: 20 October 2021.
Edited by:
Shalini Gaur Rudra, Indian Agricultural Research Institute, IndiaReviewed by:
Theofania Tsironi, Agricultural University of Athens, GreeceEldho Varghese, Central Marine Fisheries Research Institute, India
Copyright © 2021 Prabhakar, Srivastav, Pathak and Das. This is an open-access article distributed under the terms of the Creative Commons Attribution License (CC BY). The use, distribution or reproduction in other forums is permitted, provided the original author(s) and the copyright owner(s) are credited and that the original publication in this journal is cited, in accordance with accepted academic practice. No use, distribution or reproduction is permitted which does not comply with these terms.
*Correspondence: Pramod K. Prabhakar, pramodkp@niftem.ac.in; Prem P. Srivastav, pps@agfe.iitkgp.ac.in; Kalyan Das, daskalyan27@gmail.com