- Institute of Geography, University of Bern, Bern, Switzerland
Persistent vulnerability of smallholder farmers to natural hazards and livelihood insecurity call for the identification of measures that enhance the resilience of their agriculture-dependent livelihoods. Without understanding how to secure smallholder livelihoods against adverse social-ecological dynamics, especially related to climate variability and market failures, hunger, poverty, and livelihood collapse are likely to become more entrenched. This study aims for this better understanding by applying the Livelihood Resilience Indicator Framework to investigate the livelihood resilience of smallholder farmers in Makueni County, Kenya, to disease and pest infestations, low yields, and hunger. We analyzed the buffer capacity dimension of resilience among smallholder farmers, using survey data collected in 2016 on 134 households. We conducted principal component analysis to calculate a buffer capacity index at household level, which we then assessed in relation to crop and livestock pests and diseases, yields, and food shortage. We found that there was a significant positive correlation between buffer capacity and maize yields, which could be attributed to diversity in agricultural practices and income. The incidence of pests and diseases correlated significantly and negatively with buffer capacity and specifically with land size, economic status, and social capital. While no significant relationship could be established between buffer capacity and the occurrence of food insecurity, this variable correlated with access to land and livestock, diversity in agricultural practices, and access to infrastructure. The expected positive relation between food security and access to infrastructure and services turned out to be negative, raising questions about the relations between the livelihood resilience construct and rural infrastructure and services. More differentiation is thus needed on the multi-faceted interactions between access to infrastructure and services, including their actual use and benefits to livelihood resilience. In general, most findings supported the Livelihood Resilience Indicator Framework in that households with higher buffer capacity were better equipped to cope with shocks and stressors, hence demonstrating the potential of the framework as an early warning tool.
Introduction
In sub-Saharan Africa, smallholder farmers are increasingly exposed to global shocks such as climate change, economic crises, pandemics, and international political instability (Leichenko and O'Brien, 2002; Boillat et al., 2019). Understanding their capacity to cope with these shocks is crucial for any efforts to mitigate them and contribute to the Sustainable Development Goals (SDGs). The concept of livelihood resilience has been developed to understand how households moderate and overcome disturbances. Livelihood resilience is based on the broader research on the resilience of social-ecological systems (Berkes and Folke, 1998), but specifically focuses on livelihood functions, which refers to the benefit that livelihoods provide, such as food, income, insurance, and poverty reduction (Chambers and Conway, 1992). Livelihood resilience thus focuses on the factors and processes that keep livelihoods functioning despite change and puts people's capabilities to cope with shocks at the center of the analysis (Ifejika Speranza et al., 2014).
To date, a limited number of studies have operationalized farmers' livelihood resilience to shocks and stressors and, in particular, to impacts from extreme climate variability. Though analytical frameworks that address smallholder livelihood resilience in the Global South have been developed (e.g., Department for International Development, 2011; Ifejika Speranza et al., 2014), they have not yet been fully translated into empirically tested, measurable approaches. Developing measuring tools is nevertheless crucial for development practitioners and policy makers in planning and monitoring projects and policies aimed at building resilience to climate change (Ifejika Speranza et al., 2014; Kasie et al., 2018; Quandt, 2018).
Assessing and quantifying livelihood resilience presents several challenges. As a scientific construct, it cannot be directly observed or measured (Obrist et al., 2010), thus its assessment relies on proxy indicators (Ifejika Speranza et al., 2014). These indicators are strongly context-dependent, particularly to the kinds of shocks farmers are at risk of being exposed to (Ifejika Speranza et al., 2014). Furthermore, shocks, stressors, and factors that define buffer capacity change across space and time (Mavhura et al., 2017).
In order to operationalize livelihood resilience in a specific context and choose indicators that capture its multi-dimensionality, researchers have employed various techniques. Most studies use a data-driven or inductive approach to select indicators based exclusively on a review of literature (e.g., Sina et al., 2019) or in combination with stakeholder consultations or household surveys (e.g., Asmamaw et al., 2019; Gong et al., 2020; Campbell, 2021). Focus group discussion and key informant interviews have been used to validate indicators derived from literature review (Gong et al., 2020; Campbell, 2021). A qualitative-quantitative approach is also used to compile the indicators into a framework for measuring resilience (Sina et al., 2019) or a resilience index (Asmamaw et al., 2019; Campbell, 2021). To establish the most relevant indicators for enhancing resilience, Principal Component Analysis (PCA) and multiple regression analysis have been applied (Asmamaw et al., 2019).
In this article, we propose a method to assess buffer capacity, a key component of livelihood resilience. Buffer capacity is measured through proxy indicators based on the Sustainable Livelihoods approach (Scoones, 1998), namely human, financial, social, physical, and natural capital at farming household level as well as livelihood strategy and diversity of capitals and strategy (Ifejika Speranza et al., 2014). We develop a buffer capacity index (BCI) using Principal Component Analysis (PCA) and assess it for farming households in the semi-arid, drought-prone area of South-East Kenya. Following the approach of Cutter et al. (2003) and Mavhura et al. (2017), who constructed a social vulnerability index and Ifejika Speranza (2013), we use PCA as a tool to identify clusters of variables that constitute components underlying buffer capacity (Field, 2013).
We further test our measures of buffer capacity by examining their influence on outcomes of livelihood resilience, namely (a) the ability to maintain farm productivity under adverse climatic conditions, (b) food security, and (c) their relationship with pest outbreaks. Hence, this paper contributes to the growing body of empirical studies on livelihood resilience to climate variability. It contributes to the empirical evidence in identifying key factors underlying livelihood resilience (e.g., Colting-Pulumbarit et al., 2018) and helps to inform interventions and policies aimed at building resilience in farming households.
Conceptual Framework
Livelihood resilience is defined as the “capacity of livelihoods to cushion stressors and disturbances while maintaining or improving essential properties and functions,” including those related to levels of production and well-being (Ifejika Speranza et al., 2014, p. 111). Livelihood resilience bridges the theory of human well-being and capabilities with the theory of social-ecological resilience (Scoones, 1998). A well-being and capability approach focuses on “what people can do or be with their entitlements” (Sen, 1984), thus extending approaches exclusively based on income or food. Resilience theory largely stems from ecology and focuses on the ability of a system to maintain functions after an external shock (Gunderson and Holling, 2002). Livelihood resilience is therefore determined by households' assets and strategies to maintain and increase their asset base, their capacity to self-organize and to learn (Ifejika Speranza et al., 2014). Ifejika Speranza et al. (2014) propose a conceptual framework based on sustained buffer capacity, promotion of self-organization and existence of learning processes to capture the attributes of livelihood resilience. Bahadur et al. (2015) offer a similar framing of livelihood resilience based on absorptive capacity (mobilization of assets to create a buffer), anticipatory capacity (capacity of a social system to plan, share information, and engage in collective action), adaptive capacity (capacity to respond by finding alternative coping strategy, which involves a learning process) and transformation, which brings about leadership and empowerment processes.
Narrower and broader conceptualizations of livelihood resilience have been applied. For instance, Gong et al. (2020) conceptualize resilience as including short-term actions to resist risk (capacity in responding to, absorbing and resisting risk and maintain livelihoods after relocation) but exclude longer-term adaptation, transformation or learning. Kumar et al. (2020) take a yet narrower approach and define resilience as speed of recovery post-shock, measured by variations in crop productivity and crop income. Hence, they follow a narrower definition of resilience compared to Ifejika Speranza et al. (2014) or Bahadur et al. (2015). As assessing livelihood resilience in the context of climate change requires considering multiple factors, their interactions, and processes that are critical for households to maintain their livelihoods across time, we adopt the framework by Ifejika Speranza et al. (2014) in this study.
Buffer capacity (BC) is a key component of livelihood resilience that specifically focuses on softening and reducing the impact of shocks (Ifejika Speranza, 2013). It is defined as the “capacity to cushion change and use the emerging opportunities to achieve better livelihood outcomes such as reduced poverty” (Ifejika Speranza et al., 2014, p. 112). BC involves securing livelihood assets, maintaining and increasing them. To make the concept operational, it should thus include indicators reflecting endowments and entitlements, strategies, and diversity (Ifejika Speranza et al., 2014).
Endowments are tangible and intangible resources owned by individuals (Ifejika Speranza et al., 2014). Entitlements represent goods and services individuals can gain access to by exchanging their endowments, such as labor power (Sen, 1984). Bourdieu's theory of practice (Bourdieu, 1977, 1986) postulates that people possess different types of “capital” including economic, cultural and social capital and convert a form of capital to another to access resources, goods, and services. Livelihood resilience is based on the assumption that the accumulation of different types of capitals creates buffers against stressors and shocks (Scoones, 1998). The Sustainable Livelihoods approach (Department for International Development, 1999) makes livelihood resilience operational by extending Bourdieu's framing to five livelihood capitals that play an essential role in the building of resilience: human capital (skills, knowledge, health, labor availability), financial capital (savings including livestock, earnings, pensions, or remittances), social capital (networks, group memberships, trust, reciprocity, and informal safety nets), physical capital (energy and communication infrastructure, sanitation, transport) and natural capital (land, forest, water, biodiversity) (Bourdieu, 1986; Department for International Development, 1999). All five assets may be fostered or constrained by transforming structures and processes of the wider society (Obrist et al., 2010).
Nevertheless, the interplay of the different capitals is complex, involving trade-offs at different spatial and temporal scales of analysis (Ifejika Speranza, 2013; Thulstrup, 2015; Quandt, 2018). Hence, farmers may face decisions about satisfying their immediate needs at the cost of future well-being or choosing short-term benefits over long-term gains. This is illustrated by the second determinant of buffer capacity, livelihood strategy (Ifejika Speranza et al., 2014). Inversely, strategies that focus on environmental stewardship, such as soil fertility management, can increase natural capital in the long-term. Buffer capacity can also be increased by adapting farming techniques to a given social-ecological system. This presupposes experience and accumulated knowledge of working in a specific agro-ecosystem that is less likely to prevail among migrant farmers (Ifejika Speranza et al., 2014).
Finally, buffer capacity also depends on aspects of diversity at farm level and in livelihood strategies. Studies have shown that diversification of agricultural systems (such as crop diversification) and livelihood strategies (such as complementing farm income with off-farm income) generally improves resilience by spreading risk (Sallu et al., 2010; Twine, 2013; Kasie et al., 2018). Crop diversification provides partial protection from risks associated with climatic stress or pests, as different crops respond differentially to such adverse events, hence buffering against total crop loss (Ifejika Speranza et al., 2014; Colting-Pulumbarit et al., 2018). Likewise, livelihood diversification can help sustain farmers' income in case of crop failure, hazards, or adverse market conditions (Ifejika Speranza et al., 2014; Mukul et al., 2015; Thulstrup, 2015). Households engaging in livelihood diversification through salaried employment or business activities improve economic and, to a lesser extent, social buffer capacity (Ifejika Speranza, 2013). Off-farm income diversification also positively influences households' food security (Mutea et al., 2019). Diversification as response to risks can therefore help prevent households from falling into a persistent cycle of poverty (Sabates-Wheeler et al., 2010).
Building on the framework developed by Ifejika Speranza et al. (2014), Figure 1 shows how livelihood resilience is embedded into the context of smallholder farming. Livelihood resilience, which includes capacity for learning, self-organization, and buffer capacity, can moderate the impact of climate shocks on crop yields and livestock production, whereby the use of certain agricultural techniques may improve agro-ecological resilience. Shocks include flood, drought, and the incidence of crop and livestock pests and diseases, which may increase as a consequence of climate change (Mbow et al., 2019). On the other hand, livelihood resilience also mediates the impact of low yields on food security. In turn, food security shapes farmers' decision-making for the following season, and therefore functions as a resilience feedback. Subjective perception of climatic risks also constitutes a feedback as farmers adapt their strategies and utilize their newly gained knowledge to build more resilient livelihoods.
In this study, we operationalize the framework using the case of smallholder farmer households in Makueni County, Kenya. Since other studies have their main focus on self-organization and the capacity for learning (e.g., Ifejika Speranza et al., 2014; Bottazzi et al., 2018; Mukhovi et al., 2020), we concentrate our analysis on buffer capacity and examine its interactions with production (yields), livelihood outcomes (food security) and climate-related stressors (pests and diseases).
Methodology
Study Area
Makueni County in South-East Kenya (Figure 2) is a semi-arid area with two rainy seasons: between March and May (120–240 mm) and between October and December (220–410 mm) (Ifejika Speranza et al., 2008). October to December is the main farming season, but rainfall is highly variable and unreliable in both seasons. Households rely mainly on rain-fed agriculture and livestock keeping for consumption and sale. Most households (84%) earned part of their income from crop sales, maize being the dominant crop (Ifejika Speranza et al., 2008). This high reliance on agricultural production makes agro-pastoralists vulnerable to droughts and other production risks, which exacerbate food insecurity (Ifejika Speranza et al., 2008).
Data Collection
Data collection took place from June to August 2016. We interviewed 134 farming households from 38 villages in Makueni County, using structured questionnaires. The respondents were selected among households who participated in previous surveys in the framework of a long-term research programme on rural livelihoods and resilience in the area (e.g., Ifejika Speranza et al., 2008). Fifty-five percent of respondents were female and 45 percent male, with ages ranging from 19 to 100 years. Most interviews were conducted in Kikamba, the local language, and a few in Kiswahili and English.
The questionnaire included 18 main questions with subquestions, focusing on (1) a characterization of households' demographics and income, (2) a description of agricultural techniques used, (3) an estimation of crop yields attained during productive seasons from 2014 to 2016, (4) information on pest and disease incidence and (5) an account of food shortages suffered during the 12 months preceding the date of the survey. The questions covered the period from October 2014 to June 2016 and were specifically designed to elicit responses concerning households' diverse assets and strategies, reflecting their resilience to impacts from adverse climatic events.
Data Analysis
Based on the questionnaire, we obtained 37 variables capturing buffer capacity. The variables were ordered along the different dimensions of buffer capacity, namely the human, financial, social, physical, and natural capitals and their cross-cutting elements of entitlements/endowments, diversity, and livelihood strategy. All numerical variables deviated significantly from normal distribution (Kolmogorov-Smirnov test of normality), with the exception of Number of years working in agriculture [D(131) = 0.071, p = 0.187]. Table 1 summarizes the 37 variables with their main descriptive statistics (For a full descriptive variable analysis, see Supplementary Material 1). Subsequently, we conducted principal component analysis (PCA) using SPSS® Statistics V26 to generate component scores that estimated each respondent's relative buffer capacity. To obtain robust results, we ran an iterative three-step process involving (1) preliminary evaluation, (2) an assessment of PCA outcomes, and (3) reliability analysis (Figure 3) (Field, 2013). We used standardized scores (Z-scores) to run the PCA to avoid biased results caused by the wide range of variances (ranging from 0.04 to 5,291).
We ran the process until subsequent results showed no further improvement, which was the case in the second round of analysis. This resulted in the exclusion of 15 out of the 37 original variables (Figure 4). Variables eliminated during the first preliminary assessment include a variable with too many missing answers, three redundant variables (with >0.8 correlation with other variables), and eight variables that did not pass the sampling adequacy, namely the likelihood of the data to yield distinct components [Kaiser-Meyer-Olkin (KMO) values below 0.5]. Reliability analysis led to the exclusion of three further variables reflecting human capital (education level, number of languages spoken, and number of years of agricultural experience) using Cronbach's alpha's criterion with a minimum threshold of 0.65. Indeed, the variable measuring agricultural experience correlated negatively with number of languages spoken and educational level (See Supplementary Material 2 for detailed information on the PCA process).
For the remaining 22 variables, we confirmed PCA suitability with sample size adequacy (sample size of 119 respondents and only four of the 22 communalities being below 0.6), and with the confirmation that there was no complete independence between all variables (Bartlett's Test of Sphericity significant with df = 231, p = 0.000). This second round of analysis resulted in an overall KMO of 0.696, which confirmed adequate robustness of the model.
We then ran PCA a second time using varimax rotation to disperse the loadings within the components and get distinct component clusters (Field, 2013). Communalities, which describe the proportion of the variance in each variable that can be explained by the retained underlying components (Field, 2013), were below the 0.5 threshold only for 2 variables. The average communality after extraction was 0.7, which means that a substantial portion of variance could be explained by the retained components (see Table 4 in Supplementary Material 2). We retained seven components that had eigenvalues over Kaiser's criterion of 1 and explained 70% of the cumulative variance after varimax rotation (see Table 5 in Supplementary Material 2).
The components were indicatively labeled as (1) “Infrastructure,” (2) “Land size and livestock,” (3) “Improved agricultural practices,” (4) “Social capital,” (5) “Economic status,” (6) “Economic activities on- and off-farm,” and (7) “Income diversity” (Table 2). Apart from the variable Number of group memberships, which loaded highly both on component 3 (“Improved agricultural practices,” 0.5) and component 4 (“Social capital,” 0.5), each variable loaded exclusively onto one component. All components had Cronbach's alpha above 0.65 and Corrected Item-Total Correlation values above 0.3, which indicates strong internal consistency.
We then calculated the household scores for each PCA component using the Anderson-Rubin method, which produces composite scores that are uncorrelated and standardized (Field, 2013). The sum of respondents' component scores estimates household's buffer-capacity-based livelihood resilience as a whole (Field, 2013). The BC scores were then subdivided into four categories of equal ranges, grouping households into categories of various levels of BC.
We then examined the relationship between BC and three variables: yields, food insecurity and incidence of pests and diseases using Spearmen's rho and Pearson's Correlation analysis. The average yield of maize harvested in the 2014/2015 and 2015/2016 seasons was used as a proxy for yield, since maize is the most important staple crop, being cultivated by 78% of households (Boillat et al., 2019). For food security, respondents reported the number of months in which their households ran out of produced and/or purchased food in the 12 months preceding the survey. The percentage of crop and livestock types affected by pest or disease was calculated based on (1) the number of crops produced or livestock types held in the October to December 2015/2016 season and (2) the number of crop and livestock types affected by diseases/pests during the October to December 2015/2016 season. In order to gain a more in-depth insight into the dynamics of BC, we assessed the relationship between each component representing BC and the three variables, as well as the relationships between the three stressor and livelihood outcome variables.
Results
Livelihood Buffer-Capacity-Based Resilience Index
The aggregated seven component scores provided an index to rank each of the 119 households' buffer capacity (BC). Figure 5 shows the distribution of the BC scores. The measures of this index are relative and ranged from −6, pertaining to the least resilient household, to 8.49 for the household with highest BC scores. Due to the standardization of the scores the mean BC was close to zero. The BC scores follow a normal distribution at p < 0.01 [Kolmogorov-Smirnov D(119) = 0.091, p = 0.018]. Three respondents with scores above 6.32 were outliers.
Figure 6 represents the BC scores subdivided into four categories of equal ranges. Most (54.6%) households fell into the category of low BC (scores ranging from −2.38 to 1.25). About 22% of households had moderate BC with scores from 1.25 to 4.87 and 17.6% were in the very low BC category (scores between −6.0 and −2.38). Only a small percentage, 5.9%, were in category with high BC scores (4.88–8.49).
Relationship Between Livelihood Buffer-Capacity-Based Resilience, Stressors, and Livelihood Outcomes
Spearman's rho was employed to assess significant correlations between the livelihood stressor and outcome variables. As shown in Table 3, food insecurity is negatively related to yield. Furthermore, the number of crops and livestock types affected by diseases and pests is positively related to food insecurity. Finally, no significant correlation was established between maize yield and number of crops and livestock types affected by pests and diseases.
Using the BC scores, we then examined the correlation between BC and indicators of food production, food insecurity, and incidence of crop pests and livestock diseases. For food production, 104 farming households provided valid answers regarding maize yields in our study. Average yields ranged from 0 (complete harvest failure) to 4,667 kg/ha, with a mean of 772 kg/ha and a standard deviation of 827.48. The frequency distribution was positively skewed (skew = 2.438), with most scores clustering around yields between 0 and 1,000 kg/ha. Four scores with yields above 3,000 kg/ha were outliers. The frequency distribution deviated significantly from normality [Kolmogorov-Smirnov Test: D(104) = 0.15, p = 0.000]. BC and maize yields were significantly correlated at the 0.05 level (Spearman's rho rs = 0.235, 95% BCa CI [0.017, 0.424], p = 0.022). This positive relationship is depicted in the scatter plot (Figure 7A) (For further information on the relationship between household resilience and yields, see Supplementary Material 3).
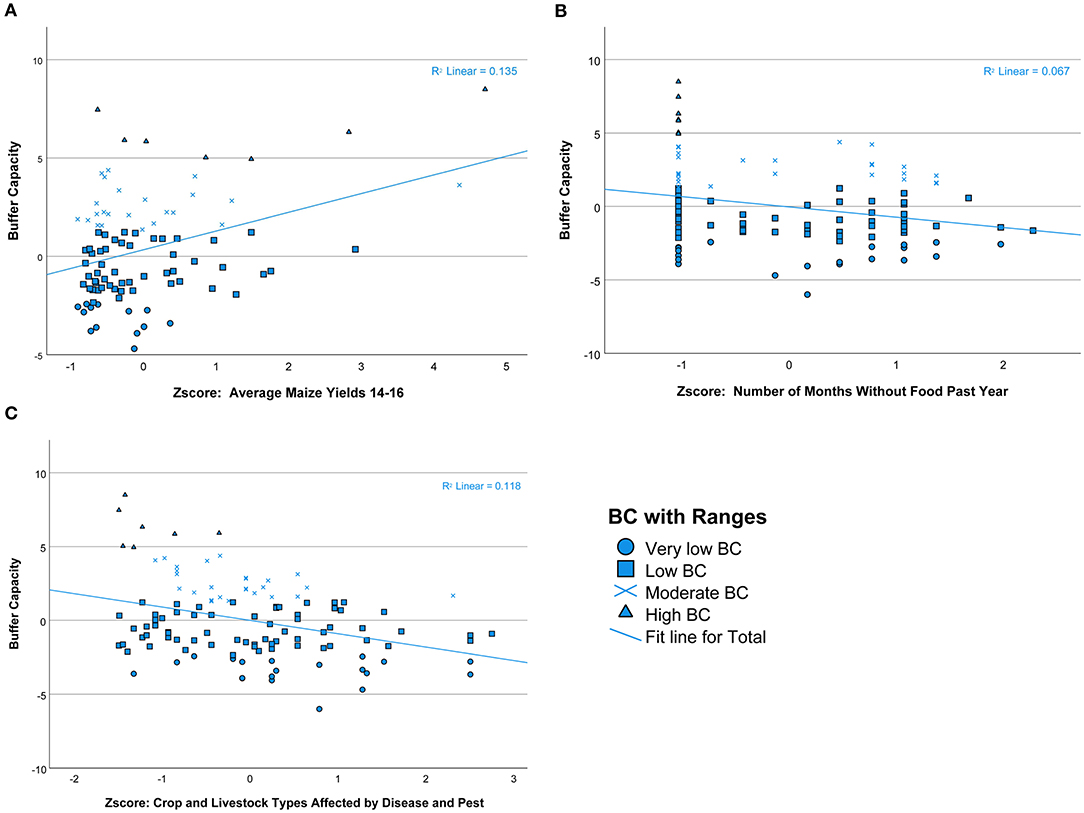
Figure 7. Scatterplots, BC and (A) maize yields 2014–2016, (B) number of months without food past 12 months and (C) crop and livestock types affected by disease and pest.
In terms of food insecurity, all 134 households indicated having run out of food at least once. Forty percent of households reported having run out of food during one of the 12 months, and one respondent (0.7%) had suffered from lack of food every month in the year preceding the survey. On average, households had experienced 4.43 food insecure months. The frequency distribution deviated significantly from the normal curve [D(134) = 0.245, p = 0.000]. The number of months in which households experienced food shortage was negatively correlated to the buffer capacity index; however, this correlation was not significant (Spearman's rho rs = −0.163, 95% BCa CI [−0.332, 0.029], p = 0.077). As can be seen in Figure 7B, the BC scores of households that had suffered hunger in one of the preceding 12 months ranged from very low to high BC, not establishing a clear relationship in the correlation pattern. Nonetheless, those households with high BC scores ran out of food during no more than one of the preceding 12 months. In this sense, it may be implied that very strong buffer capacity (>4.88) could help shield from repeated occurrences of food shortage.
The average number of livestock and crop types affected by pests or diseases was 40.7%, ranging from 15.5 to 87.5%. In terms of distribution normality, the curve conformed to normality [D(133) = 0.078, p = 0.048]. We found a significant but weak negative correlation between pests and diseases and BC at the 0.001 level (Pearson's Correlation, r = −0.343, p = 0.000). This relationship is depicted in Figure 7C.
Finally, we assessed the relationships between each separate BC component named in Table 2 and yields, food insecurity, and pests and disease incidence. Due to standardization, the means of the component scores were zero and the standard deviations and variances were 1. All components deviated significantly from normality according to Kolmogorov-Smirnov test.
For maize yields, significant relationships existed only with two components, namely, improved agricultural practices and income diversity. These correlations were positive, yet weak (see Table 4). This suggests that the more diverse and numerous the sources of household income, and the larger the range of improved agricultural practices employed, the higher the maize yield tended to be.
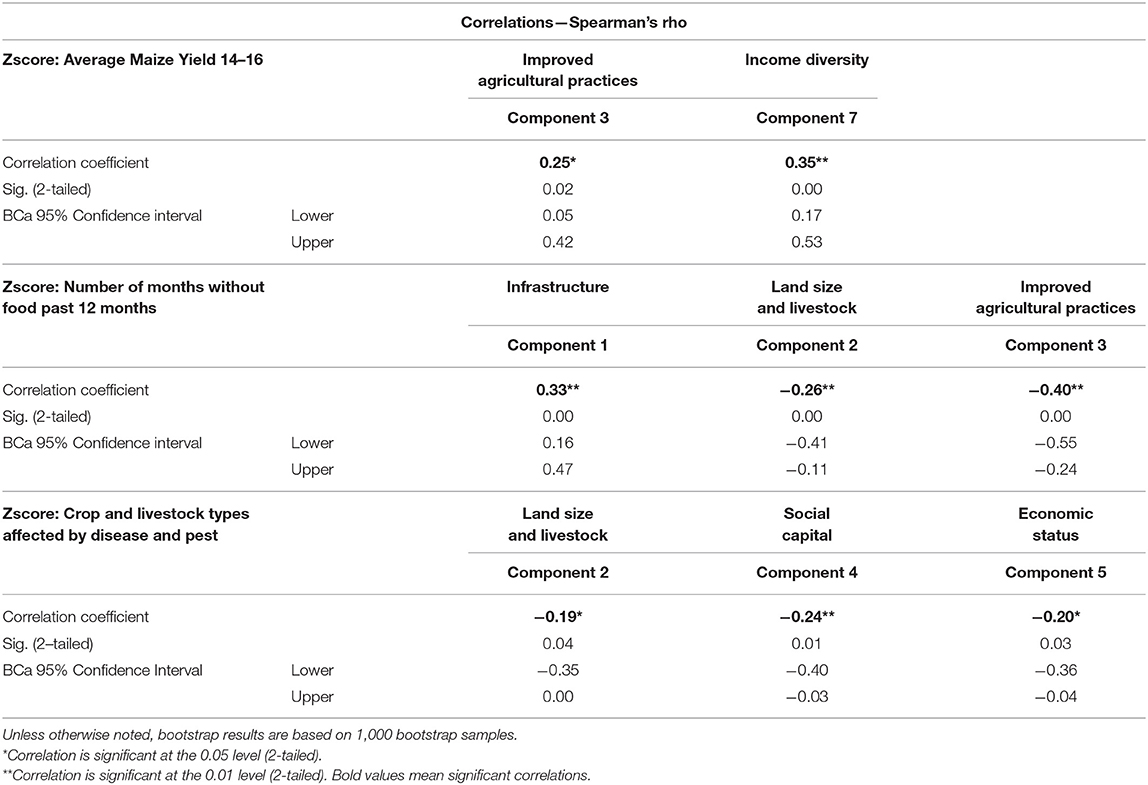
Table 4. Significant correlations between BC components and maize yield, pest/disease incidence and food insecurity (see Table 7 in Supplementary Material 4 for overview of all correlation coefficients).
We found significant negative relationships between food shortage and land size and livestock and between food shortage and improved agricultural practices. Contrary to expectations, infrastructure, which comprises short distance to credit and financial institutions, to livestock extension services, to input and food markets and number of services accessible within 2 h, correlated positively with the number of months in which households ran out of food. This implies that more geographically isolated households with longer travel distances to markets and services experienced fewer food shortages (See Table 8 in Supplementary Material 5 for correlations between food insecurity and original variables depicting the distance to various markets and services).
The incidence of crop pests and livestock diseases correlated negatively and significantly (though weakly) with three components in our study: land size and livestock, social capital, and economic status.
Finally, the component economic activities on- and off-farm (component 6), which includes the number of off-farm activities a household engages in and the overall number of economic activities of a household, did not significantly correlate with any of the stressor/shock variables (yields, pests and disease incidence, and food insecurity).
Figure 8 summarizes the relationships between maize yields, food insecurity, and pest and disease incidence and buffer capacity as a whole, as well as between the three variables and the seven individual component variables making up buffer capacity-based resilience.
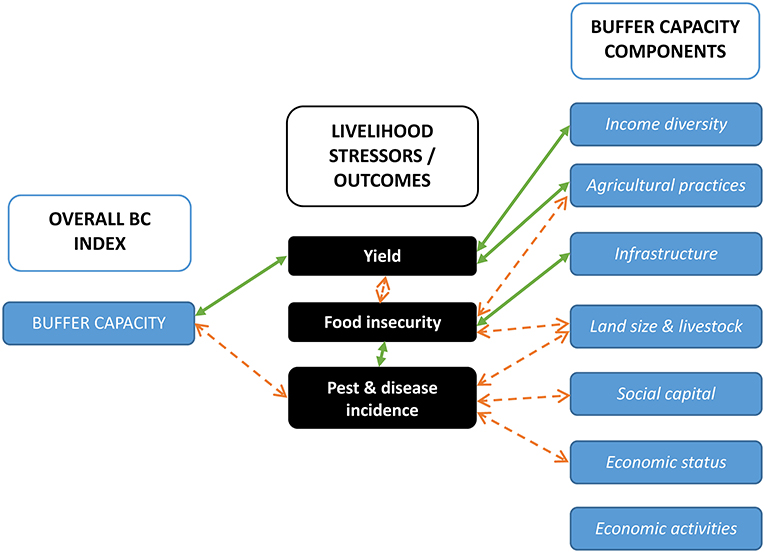
Figure 8. Summary of significant positive (green arrows) and negative (red dashed arrows) correlations (rs) between buffer capacity as well as its seven underlying components and the three variables: Yield, food insecurity, and pest/disease incidence.
Discussion
Understanding Results in the Context of the Livelihoods Resilience Framework
Our findings confirm the main postulates of the livelihoods resilience framework, namely that households with higher buffer capacity cope better with adverse climatic events and recover from shocks; they have higher yields and their crop and livestock were also less affected by pests and diseases. This was true even though in our sample no significant relationship was found between maize yields and pest/disease incidence (Table 3). Higher yields were related to the use of a wider range of improved agricultural practices, such as conservation agricultural practices, management measures (fallowing, growing drought tolerant crops, early planting, and water harvesting) and use of synthetic inputs. This is in line with findings by Boillat et al. (2019), who established that under conditions of erratic rainfall during the 2014 rainy season in Kenya, employing a combination of crop diversification, fertilizer, and herbicide use increased chances of meeting the yield threshold. Employing a diversity of agricultural techniques also improved food security, which is likely an indirect result of enhanced yields. Indeed, higher maize yields correlated with higher food security. Moreover, higher income diversity was also related to improved yields. Households benefitting from a diversified income portfolio are not only more protected against production loss and other risks, they also tend to have more stable incomes (Wan et al., 2016). This, in turn, may allow farmers to re-invest their incomes in higher quality farming inputs and enhance their ability to counter the impacts of weather shocks (Wan et al., 2016).
The assessment of individual components and food insecurity also showed that those households with smaller land plots and with less livestock had higher risk of suffering food insecurity. Boillat et al. (2019) suggested that the availability of larger land plots allows a true crop rotation, which increases the chance of meeting yield thresholds, in particular under dry conditions. Ifejika Speranza et al. (2008) similarly concluded that having access to cropland larger than 2 ha helped reduce vulnerability to food insecurity in seasons with average or below-average rainfall.
The occurrence of pests and diseases was significantly lower for farmers with higher buffer capacity, in particular those with more land, number of livestock and better social capital and economic status. This is likely due to the better options to do crop rotation (Boillat et al., 2019) which reduces crop pest and disease incidence (Zohry and Ouda, 2018). Social capital, which in this study refers to participation and engagement in social groups (as proxy for benefits derived), allows farmers to exchange ideas and resources to address the many challenges they face (Mukhovi et al., 2020), including those related to pests and diseases. These groups can enable access to credit from commercial banks or through group saving schemes (Ulrich et al., 2012), which help smallholder farmers gain access to costly inputs (Mukhovi et al., 2020), including pesticides. Another way in which social groups benefit farmers concerns farm labor participation as self-help groups organize to work on each other's farms, thus addressing labor shortage (Ifejika Speranza et al., 2008). Farm labor shortage can result in delays in threshing and storage of harvested produce, which, in turn, makes harvested produce prone to pest infestation (Ifejika Speranza et al., 2008).
Finally, economic status, which is the component encompassing quality of housing, observers' scoring of households' economic status and household's number of livestock types, was also related to lower pest and disease incidence. If these variables are interpreted to signify purchasing power, it could be concluded that the financial constraints in acquiring chemicals, equipment, and veterinary vaccines to prevent crop and livestock pests and diseases could lead to a higher crop and livestock pest and disease incidence. Indeed, Ifejika Speranza et al. (2008) found that, besides lack of information and technical knowledge on how to combat infestations, lack of financial resources also prevented households in Makueni County from purchasing the required material. However, the confirmation of this relationship should be treated with caution, as the variables underlying this component, in particular number of livestock types households possess, do not necessarily translate into higher economic status or purchasing power.
The above findings confirm the interrelationships of various components of the buffer-capacity-based resilience and in particular, between buffer capacity and the ability to cope with pests and diseases, to maintain yields and, indirectly, to uphold food security.
Nevertheless, a few findings appear to contradict the present model. First, we found no relationship between food insecurity and overall buffer capacity. Breaking down buffer capacity into its components helps to explain this. Infrastructure, which was the component underlying proximity to markets and extension services, correlated positively with the number of months in which households ran out of food, therefore confounding the relationship between food insecurity and overall buffer capacity. This contradicts studies showing that adequate access to markets and services favors resilience (e.g., Colting-Pulumbarit et al., 2018). On the one hand, households that are better integrated in the market might be trapped in a vicious circle forced by cash needs to sell their production at low prices which leads to food deficits and shortage of seeds for the following planting season (Ifejika Speranza et al., 2008). This means that access to markets cannot be assumed to enhance food security, which may also be explained by the finding that the smaller size of land owned by food-insecure households compared to food-secure households are not conducive to successful commercialization (Mutea, 2020). On the other hand, more specific indicators on inclusive access to markets and rural infrastructure and services (Chamberlin and Jayne, 2013) and those that capture the extent of household participation in markets or agricultural services and the benefits they derive, can yield further insights on the importance of infrastructure for food security.
The elevated food insecurity despite proximity to markets and services can moreover be interpreted as a lack of engagement with extension services. Ifejika Speranza et al. (2008) and Muyanga and Jayne (2008) show that extension services have shifted from a public service model to a demand-driven private model. In this context, farmers lack awareness of the procedures to request such services or are unwilling to pay for them (Ifejika Speranza et al., 2008); hence, there is no active contribution of extension services to household food security. Finally, poor adoption rates can explain the positive correlation between access to extension services and food insecurity (D'Alessandro et al., 2015). This may be due to the ineffectiveness of such services, which are marked by poor funding and a lack of coordination (Ifejika Speranza et al., 2008; D'Alessandro et al., 2015; Ministry of Agriculture, 2017). Questions specifically focusing on the contribution of extension services to household food security could help to clarify further this relationship.
A second finding which contradicts the framework involves diversity in economic activities. The role of diversity in promoting resilience has been well-established in the literature (Sallu et al., 2010; Twine, 2013; Kasie et al., 2018). Given the importance of livelihood diversification in its contribution to buffer capacity and, by extension, households' livelihoods outcomes, it was expected that households having various economic activities and earning income both on and off farm would have better access to food (Mutea et al., 2019). However, this relationship could not be confirmed based on this dataset, underlining the fact that food insecurity is shaped by multiple and complex factors (Mutea et al., 2019) and that available livelihood diversification activities offer low poverty reduction potential (Ulrich et al., 2012).
Conceptually, the elimination of variables related to human capital, which lacked internal consistency, should be further explored. Consistent with the literature, skills, and knowledge characterize human capital (e.g., Department for International Development, 1999; Colting-Pulumbarit et al., 2018). Based on the assessment framework in analyzing buffer capacity proposed by Ifejika Speranza et al. (2014), factors such as literacy level and knowledge form an integral part of buffer capacity. Education, in particular, facilitates the access to information and therefore should play a critical role in building buffer capacity. However, the observed negative correlation between agricultural experience and the education level suggests that more educated people tend to reduce farming in favor of other economic opportunities. Gong et al. (2020) indicate that extensive experience in agricultural work could inhibit flexibility in adjusting modes of production. Based on the above, further studies on livelihood resilience could benefit from the inclusion of a human capital component not related to education considering the overall low levels of education in the study area.
The mixed results related to human capital show the importance to build context-specific resilience assessments. The determinants of livelihood resilience of farming household to climate-related stressors and shocks are highly context dependent (e.g., Quandt, 2018). This hampers the development of widely applicable tools for resilience assessment. We suggest that looking at the underlying components—such as social capital, economic activity off-farm, economic status, access to infrastructure, land and livestock, agricultural practices, and income diversification—instead of specific variables in assessing resilience can help overcome this problem. Tools could capture the concept at the component level and adapt variables pertaining to each component to the local context.
Implications for Policy and Practice
This study has shown that with use of PCA and by quantitatively assessing livelihood resilience at the household level, households with greatest needs of building resilience can be identified. The buffer capacity index can be employed as a tool for targeting agricultural extension (Colting-Pulumbarit et al., 2018) and social development policies to ensure that they reach vulnerable populations (Ayeb-Karlsson et al., 2015), and also to monitor the effectiveness of these interventions in the context of extreme climate variability (Ifejika Speranza and Bockel, 2015; Bockel et al., 2018). Considering the steep decline in maize yields in several counties—including Makueni County—between 1993 and 2009, which can be partially attributed to climate change, and the implications on food security in that region (Omoyo et al., 2015), the design and implementation of targeted social policies is particularly critical.
Although cautious about drawing inferences about the larger population, diverse aspects were identified as entry points to enable surveyed households to build livelihood resilience. These include improved access to land and livestock, and creating opportunities to diversify household income into higher-income yielding activities. Diversified livelihoods were also identified by Gong et al. (2020) as a critical aspect for quickly adapting livelihood strategies following shocks. The authors suggest that local governments offer skills training to enhance access to diversified income-generating activities. Local governments should moreover disseminate information about the benefit of group memberships in fostering social capital and promote and support activities carried out by community groups. Finally, the effectiveness, accessibility and appropriateness of extension services must be improved to widen the range of agricultural practices employed by the farmers, with the aim of improving yields and reducing food insecurity. Coincidentally, there was a high local demand for agricultural extension, judging from the 40 study participants who specifically asked for training. These considerations stress the need to strengthen capabilities and responsibilities of local governments and collectives.
Besides the assessed internal capacities of households, other external socio-economic constraints can affect their buffer capacity. For example, in the study area, several families felled trees and abandoned agroforestry due to the prospects of being displaced by the construction of the Thwake dam. Considering the importance of natural capital, including access to land, in building buffer capacity, it is critical that relocated households are adequately compensated for their loss (Gong et al., 2020). Inequalities in voice and power matter when smallholders face competition for land and water resources, which also impacts livelihood resilience (Ayeb-Karlsson et al., 2015; Boillat and Bottazzi, 2020).
Limitations and Recommendations
This study has focused on the buffer-capacity dimension of resilience to climate extremes. Further research could expand the scope to also include variables related to self-organization and learning dimensions of the resilience concept. In doing so, attention has to be paid to not duplicate variables, since indicators in different dimensions overlap (for instance, those pertaining to social capital and self-organization). As a way of control, the validity of the index could be confirmed by comparing it to participants' subjective assessment of resilience, as done by Quandt (2018) and Gong et al. (2020).
While PCA has proven an adequate way to reduce dimensions and a tool to construct the resilience index, the method also brought about loss of data, such as that related to the human capital dimension and other variables that were eliminated to ensure internal consistency. Future studies could set out with a broader set of variables, such as health-related data in measuring human capital.
In employing a dimension-reducing technique such as principal component analysis, the breadth of indicators used to assess livelihood resilience is narrowed down by retaining only components that adequately measure the concept in a given context. This facilitates periodic measurement of resilience and longitudinal studies, which is particularly important due to the dynamics inherent in resilience (Ifejika Speranza et al., 2014), livelihoods and their social-ecological contexts. By conducting a follow-up study involving the same households and including data from earlier (2013) surveys, the dynamics of resilience could be further explored. Moreover, future studies can deepen the understanding of the concept by comparing inequalities in buffer capacity to other types of inequality. Future research can expand on this study, which only analyzed buffer capacity at household level, and explore buffer capacity at higher organizational levels such as community cohesion, collective action, and interrelations with powerful actors, which can be key drivers of buffer capacity at household level.
Conclusion
This study has deepened the understanding of buffer capacity-based resilience and its interaction with stressors related to agricultural production at household level. Methodologically, we developed a quantitative approach for constructing a buffer capacity index derived through principal component analysis, a technique aimed at reducing dimensionality while also ensuring internal data consistency. The developed index provides a tool for monitoring livelihood resilience over time and across households and can help identify livelihood dimensions that need to be improved and the approaches to do so. One key insight is thus that such a BCI needs to be stratified into a generalizable part that can be used to compare across different farms and households but also a context specific part that enables capturing variations that are not generalizable but locally critical.
The components identified in the analysis reflect the broader literature and demonstrate the range of underlying determinants of buffer capacity. The BC index, which constitutes the sum of the seven component scores, correlated positively with yields and negatively with crop and livestock pests and disease incidence, which was expected based on the literature. However, a significant correlation between BC and food insecurity was not established. This may imply that the BC index could benefit from further refinements by replicating this research across time and in different geographic regions. As a further step, the BC index could be employed to track resilience over time and to identify least resilient households. Hence, it has the potential to positively contribute to better understanding the factors underpinning livelihood resilience and indicate levers for improving livelihood outcomes.
Data Availability Statement
Upon request, the raw data supporting the conclusions of this article can be made available by the authors.
Ethics Statement
Ethical review and approval was not required for the study on human participants in accordance with the local legislation and institutional requirements. Written informed consent for participation was not required for this study in accordance with the national legislation and the institutional requirements.
Author Contributions
CIS: conceptualization, methodology, supervision and project coordination. CIS and SB: organization of data collection in collaboration with Kenyan research partner. SM: data organization, formal analysis, and writing of first manuscript. SM, CIS, and SB: interpretation of results, review and editing. All authors have read and approved the submitted version of the manuscript.
Funding
This paper builds on the framework, data and insights gained in the research project Resilient agriculture-based livelihoods and resilient agricultural landscapes? Adaptation to climate change in African agriculture funded by the Swiss National Science Foundation (SNSF) under its Ambizione grant [PZ00P1_137068]. Follow-up research was funded by the by NERC DFID El Nino Programme 2016 (Ref. NE/P004091/1) through project ACRES—Agricultural Climate Resilience to El-Nino in sub-Saharan Africa. SB's work is currently funded by Swiss National Foundation for Scientific Research (SNSF), AgroWork project grant number 176736.
Conflict of Interest
The authors declare that the research was conducted in the absence of any commercial or financial relationships that could be construed as a potential conflict of interest.
Acknowledgments
We would like to thank the households in Makueni County who readily and patiently answered the survey questions. We acknowledge the Center for Training and Integrated Research in Arid and Semi-arid Lands Development (CETRAD) for their collaboration on the questionnaire. Moreover, we thank the field assistants for their hard work during data collection. We thank the reviewers for their constructive comments. This study contributes to the Programme on Ecosystem Change and Society (www.pecs-science.org) and the Global Land Programme (www.glp.earth).
Supplementary Material
The Supplementary Material for this article can be found online at: https://www.frontiersin.org/articles/10.3389/fsufs.2021.645046/full#supplementary-material
References
Asmamaw, M., Mereta, S. T., and Ambelu, A. (2019). Exploring households' resilience to climate change-induced shocks using Climate Resilience Index in Dinki watershed, central highlands of Ethiopia. PLoS ONE 14:e0219393. doi: 10.1371/journal.pone.0219393
Ayeb-Karlsson, S., Tanner, T., van der Geest, K., and Warner, K. (2015). Livelihood Resilience in a Changing World - 6 Global Policy Recommendations for a More Sustainable Future. United Nations University, Institute for Environment and Human Security (UNU-EHS). United Nations University. Retrieved from: https://www.munichre-foundation.org/de/dms/MRS/Documents/Resilience-Academy/2014_resilience_academy_wp_policy_recommendations/20160510_Livelihood_resilience_2015_WP22.pdf (accessed January 30, 2020).
Bahadur, A., Peters, K., Wilkinson, E., Pichon, F., Gray, K., and Tanner, T. (2015). The 3As: Tracking Resilience Across BRACED. London: Overseas Development Institute.
Berkes, F., and Folke, C. (1998). “Linking social and ecological systems for resilience and sustainability,” in Linking Social and Ecological Systems, eds F. Berkes and C. Folke (Cambridge: Cambridge University Press), 414–436.
Bockel, L., Vian, L., and Torre, C. (2018). Ex-Ante Carbon-balance Tool for Measurement, Reporting and Verification (EX-ACT MRV). Rome: Food and Agriculture Organisation of the United Nations (FAO). Retrieved from: http://www.fao.org/fileadmin/templates/ex_act/EX-ACT_MRV/EX-ACT_MRV_guidelines.pdf (accessed April 24, 2020).
Boillat, S., and Bottazzi, P. (2020). Agroecology as a pathway to resilience justice: peasant movements and collective action in the Niayes coastal region of Senegal. Int. J. Sustain. Dev. World Ecol. 27, 662–677. doi: 10.1080/13504509.2020.1758972
Boillat, S., Jew, E. K., Steward, P. R., Ifejika Speranza, C., Whitfield, S., Mkwambisi, D., et al. (2019). Can smallholder farmers buffer rainfall variability through conservation agriculture? On-farm practices and maize yields in Kenya and Malawi. Environ. Res. Lett. 14:115007. doi: 10.1088/1748-9326/ab45ad
Bottazzi, P., Winkler, M. S., Boillat, S., Diagne, A., Chabi Sika, M., Kpangon, A., et al. (2018). Measuring subjective flood resilience in suburban dakar: a before–after evaluation of the “Live with Water” project. Sustainability 10, 21–35. doi: 10.3390/su10072135
Bourdieu, P. (1977). Outline of a Theory of Practice. Cambridge: Cambridge University Press. doi: 10.1017/CBO9780511812507
Bourdieu, P. (1986). “The forms of capital,” in Handbook of Theory and Research for the Sociology of Education, ed J. E. Richardson (New York, NY: Greenwood Press), 241–258.
Campbell, D. (2021). Environmental change and the livelihood resilience of coffee farmers in Jamaica: a case study of the Cedar Valley farming region. J. Rural Stud. 81, 220–234. doi: 10.1016/j.jrurstud.2020.10.027
Chamberlin, J., and Jayne, T. S. (2013). Unpacking the meaning of 'Market Access': evidence from Rural Kenya. World Dev. 41, 245–264. doi: 10.1016/j.worlddev.2012.06.004
Chambers, R., and Conway, G. (1992). Sustainable Rural Livelihoods: Practical Concepts for the 21st Century. IDS Discussion Paper 296. Retrieved from: https://opendocs.ids.ac.uk/opendocs/bitstream/handle/20.500.12413/775/Dp296.pdf?sequence=1 (accessed July 27, 2020).
Colting-Pulumbarit, C., Lasco, R. D., Rebancos, C. M., and Coladilla, J. O. (2018). Sustainable livelihoods-based assessment of adaptive capacity to climate change: the case of organic and conventional vegetable farmers in La Trinidad, Benguet, Philippines. J. Environ. Sci. Manage. 21, 57–69.
Cutter, S. L., Boruff, B. J., and Shirley, W. (2003). Social vulnerability to environmental hazards. Soc. Sci. Q. 84, 242–261. doi: 10.1111/1540-6237.8402002
D'Alessandro, S. P., Caballero, J., Lichte, J., and Simpkin, S. (2015). Kenya: Agricultural Sector Risk Assessment. Agricultural Global Practice Technical Assistance Paper. Washington, DC: World Bank Group. Retrieved from: http://documents.worldbank.org/curated/en/294711467992513646/pdf/97887-REVISED-WP-P148139-PUBLIC-Box393257B-Kenya-Agricultural-Sector-Risk-Assessment.pdf (accessed May 20, 2020).
Department for International Development (1999). Sustainable Livelihoods Guidance Sheets. London: DFID. Retrieved from: http://www.livelihoodscentre.org/documents/20720/100145/Sustainable+livelihoods+guidance+sheets/8f35b59f-8207-43fc-8b99-df75d3000e86 (accessed January 9, 2020).
Department for International Development (2011). Defining Disaster Resilience: A DFID Approach Paper. London: Department for International Development. Retrieved from: https://www.gov.uk/government/publications/defining-disaster-resilience-a-dfid-approach-paper (accessed December 31, 2019).
Gong, Y., Zhang, R., Yao, K., Liu, B., and Wang, F. (2020). A livelihood resilience measurement framework for dam-induced displacement and resettlement. Water 12, 1–23. doi: 10.3390/w12113191
Gunderson, L. H., and Holling, C. S. (Eds.) (2002). Panarchy: Understanding Transformations in Human and Natural Systems. Washington, DC: Island Press.
Ifejika Speranza, C. (2013). Buffer capacity: capturing a dimension of resilience to climate change in African smallholder agriculture. Regional Environ. Change 13, 521–535. doi: 10.1007/s10113-012-0391-5
Ifejika Speranza, C., and Bockel, L. (2015). Climate Resilience Assessment of Agriculture and Forestry Projects and Programmes (CRAAF) -An Expert Assessment Tool. Bern; Rome: Centre for Development and Environment, University of Bern, Food and Agriculture Organisation of the United Nations (FAO).
Ifejika Speranza, C., Kiteme, B., and Wiesmann, U. (2008). Droughts and famines: the underlying factors and the causal links among agro-pastoral households in semi-arid Makueni district, Kenya. Glob. Environ. Change 18, 220–233. doi: 10.1016/j.gloenvcha.2007.05.001
Ifejika Speranza, C., Wiesmann, U., and Rist, S. (2014). An indicator framework for assessing livelihood resilience in the context of social–ecological dynamics. Glob. Environ. Change 28, 109–119. doi: 10.1016/j.gloenvcha.2014.06.005
Kasie, T. A., Tsegaye, E. A., Grandío-Botella, A., and Giménez-Garcia, I. (2018). Measuring resilience properties of household livelihoods and food security outcomes in the risky environments of Ethiopia. Iberoam. J. Dev. Stud. 7, 52–80. doi: 10.26754/ojs_ried/ijds.252
Kumar, S., Mishra, A. K., Pramanik, S., Mamidanna, S., and Whitbread, A. (2020). Climate risk, vulnerability and resilience: supporting livelihood of smallholders in semiarid India. Land Use Policy 97, 1–12. doi: 10.1016/j.landusepol.2020.104729
Leichenko, R. M., and O'Brien, K. (2002). The dynamics of vulnerability to rural change. mitigation and adaptation strategies for global change. Mitigat. Adapt. Strateg. Glob. Change 7, 1–18. doi: 10.1023/A:1015860421954
Mavhura, E., Manyena, B., and Collins, A. E. (2017). An approach for measuring social vulnerability in context: The case of flood hazards in Muzarabani district, Zimbabwe. Geoforum 86, 103–117. doi: 10.1016/j.geoforum.2017.09.008
Mbow, C., Rosenzweig, C., Barioni, L. G., Benton, T. G., Herrero, M., Krishnapillai, M., et al. (2019). Food Security in Climate Change and Land. An IPCC Special Report on Climate Change, Desertification, Land Degradation, Sustainable Land Management, Food Security, and Greenhouse Gas Fluxes in Terrestrial Ecosystems. IPCC. Retrieved from: https://www.ipcc.ch/site/assets/uploads/sites/4/2019/11/08_Chapter-5.pdf (accessed January 21, 2020).
Ministry of Agriculture Livestock Fisheries Republic of Kenya. (2017). Guidelines and Standards for Agricultural Extension and Advisory Services. Retrieved from: http://www.kilimo.go.ke/wp-content/uploads/2018/04/Approved-Guidelines-and-Standards-for-Agricultural-Extension-Advisory-Services-27th-November-2017.pdf (accessed May 20, 2020).
Mukhovi, S. M., Jacobi, J., Llanque, A., Rist, S., Delgado, F., Kiteme, B., et al. (2020). Social self-organization and social-ecological resilience in food systems. lessons from smallholder agriculture in Kenya and Indigenous Guaraní Communities in Bolivia. Food Stud. 10, 19–42. doi: 10.18848/2160-1933/CGP/v10i01/19-42
Mukul, S. A., Manzoor Rashid, A., Uddin, M. B., and Khan, N. A. (2015). Role of non-timber forest products in sustaining forest-based livelihoods and rural households' resilience capacity in and around protected area: a Bangladesh study. J. Environ. Plann. Manage. 59, 628–642. doi: 10.1080/09640568.2015.1035774
Mutea, E., Bottazzi, P., Jacobi, J., Kiteme, B., Ifejika Speranza, C., and Rist, S. (2019). Livelihoods and food security among rural households in the north-western Mount Kenya region. Front. Sustain. Food Syst. 3:98. doi: 10.3389/fsufs.2019.00098
Mutea, E. N. (2020). Access to productive resources, and smallholder farms' commercialization in the achievement of household food security in Northwest Mount Kenya region. dissertation. University of Bern, Bern, Switzerland.
Muyanga, M., and Jayne, T. S. (2008). Private agricultural extension system in Kenya: practice and policy lessons. J. Agric. Educ. Exten. 14, 111–124. doi: 10.1080/13892240802019063
Obrist, B., Pfeiffer, C., and Henley, R. (2010). Multi-layered social resilience: a new approach in mitigation research. Prog. Dev. Stud. 10, 283–293. doi: 10.1177/146499340901000402
Omoyo, N., Wakhungu, J., and Oteng'i, S. (2015). Effects of climate variability on maize yield in the arid and semi arid lands of lower eastern Kenya. Agric. Food Secu. 4, 1–13. doi: 10.1186/s40066-015-0028-2
Quandt, A. (2018). Measuring livelihood resilience: the Household Livelihood Resilience Approach (HLRA). World Dev. 107, 253–263. doi: 10.1016/j.worlddev.2018.02.024
Sabates-Wheeler, R., Butters, S., and Greeley, M. (2010). Context-specific and project-induced risk: designing projects for promoting resilient livelihoods. Inst. Dev. Stud. Bull. 41, 96–104. doi: 10.1111/j.1759-5436.2010.00187.x
Sallu, S. M., Twyman, C., and Stringer, L. C. (2010). Resilient or vulnerable livelihoods? Assessing livelihood dynamics and trajectories in rural Botswana. Ecol. Soc. 15, 1–24. doi: 10.5751/ES-03505-150403
Scoones, I. (1998). Sustainable Rural Livelihoods: A Framework for Analysis. IDS working paper 72. Retrieved from: https://www.staff.ncl.ac.uk/david.harvey/AEF806/Sconnes1998.pdf (accessed December 31, 2019).
Sina, D., Chang-Richards, A. Y., Wilkinson, S., and Potangaroa, R. (2019). A conceptual framework for measuring livelihood resilience: relocation experience from Aceh, Indonesia. World Dev. 117, 253–265. doi: 10.1016/j.worlddev.2019.01.003
Thulstrup, A. W. (2015). Livelihood resilience and adaptive capacity: tracing changes in household access to capital in Central Vietnam. World Dev. 74, 352–362. doi: 10.1016/j.worlddev.2015.05.019
Twine, W. (2013). Multiple strategies for resilient livelihoods in communal areas of South Africa. Afr. J. Range Forage Sci. 30, 39–43. doi: 10.2989/10220119.2013.768703
Ulrich, A., Ifejika Speranza, C., Roden, P., Kiteme, B., Wiesmann, U., and Nüsser, M. (2012). Small-scale farming in semi-arid areas: livelihood dynamics between 1997 and 2010 in Laikipia, Kenya. J. Rural Stud. 28, 241–251. doi: 10.1016/j.jrurstud.2012.02.003
Wan, J., Li, R., Wang, W., Liu, Z., and Chen, B. (2016). Income diversification: a strategy for rural region risk management. Sustainability 8, 1064–1076. doi: 10.3390/su8101064
Keywords: buffer capacity index, Kenya, food security, agricultural production, early warning, smallholder farmers, livelihood resilience
Citation: Matter S, Boillat S and Ifejika Speranza C (2021) Buffer-Capacity-Based Livelihood Resilience to Stressors—An Early Warning Tool and Its Application in Makueni County, Kenya. Front. Sustain. Food Syst. 5:645046. doi: 10.3389/fsufs.2021.645046
Received: 22 December 2020; Accepted: 28 May 2021;
Published: 05 July 2021.
Edited by:
Ademola Braimoh, World Bank Group, United StatesReviewed by:
Mohammed Jashimuddin, University of Chittagong, BangladeshRuilian Zhang, The University of Queensland, Australia
Copyright © 2021 Matter, Boillat and Ifejika Speranza. This is an open-access article distributed under the terms of the Creative Commons Attribution License (CC BY). The use, distribution or reproduction in other forums is permitted, provided the original author(s) and the copyright owner(s) are credited and that the original publication in this journal is cited, in accordance with accepted academic practice. No use, distribution or reproduction is permitted which does not comply with these terms.
*Correspondence: Selina Matter, c2VsaW5hLm1hdHRlckBnbWFpbC5jb20=