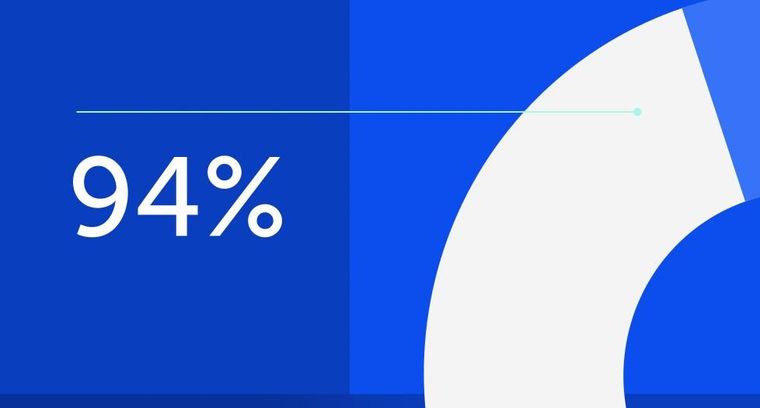
94% of researchers rate our articles as excellent or good
Learn more about the work of our research integrity team to safeguard the quality of each article we publish.
Find out more
ORIGINAL RESEARCH article
Front. Sustain. Food Syst., 29 March 2021
Sec. Climate-Smart Food Systems
Volume 5 - 2021 | https://doi.org/10.3389/fsufs.2021.534616
Surface ozone (O3) pollution poses significant threats to crop production and food security worldwide, but an assessment of present-day and future crop yield losses due to exposure to O3 still abides with great uncertainties, mostly due: (1) to the large spatiotemporal variability and uncertain future projections of O3 concentration itself; (2) different methodological approaches to quantify O3 exposure and impacts; (3) difficulty in accounting for co-varying factors such as CO2 concentration and climatic conditions. In this paper, we explore these issues using a common framework: a consistent set of simulated present-day O3 fields from one chemical transport model, coupled with a terrestrial ecosystem-crop model to derive various O3 exposure metrics and impacts on relative crop yields worldwide, and examine the potential effects of elevated CO2 on O3-induced crop yield losses. Throughout, we review and explain the differences in formulation and parameterization in the various approaches, including the concentration-based metrics, flux-based metrics, and mechanistic biophysical crop modeling. We find that while the spatial pattern of yield losses for a given crop is generally consistent across metrics, the magnitudes can differ substantially. Pooling the concentration-based and flux-based metrics together, we estimate the present-day globally aggregated yield losses to be: 3.6 ± 1.1% for maize, 2.6 ± 0.8% for rice, 6.7 ± 4.1% for soybean, and 7.2 ± 7.3% for wheat; these estimates are generally consistent with previous studies but on the lower end of the uncertainty range covered. We attribute the large combined uncertainty mostly to the differences among methodological approaches, and secondarily to differences in O3 and meteorological inputs. Based on a biophysical crop model that mechanistically simulates photosynthetic and yield responses of crops to stomatal O3 uptake, we further estimate that increasing CO2 concentration from 390 to 600 ppm reduces the globally aggregated O3-induced yield loss by 21–52% for maize and by 27–38% for soybean, reflecting a CO2-induced reduction in stomatal conductance that in turn alleviates stomatal O3 uptake and thus crop damage. Rising CO2 may therefore render the currently used exposure-yield relationships less applicable in a future atmosphere, and we suggest approaches to address such issues.
Future food production is projected to increase by at least 50% by year 2050 in order to fulfill the rapidly growing global food demand, which is a combined result of population growth and a worldwide shift toward a more meat-intensive diet from staple food (Alexandratos and Bruinsma, 2012). However, food production is threatened by various environmental problems, including air pollution. Tropospheric ozone (O3), which is one of the major air pollutants worldwide, has adverse impacts on natural vegetation and crops (e.g., Betzelberger et al., 2012; Feng et al., 2015, 2019; Mills et al., 2018a). It is projected that O3 pollution will become more severe in the future due to a warmer climate and, depending on the region, more anthropogenic emissions of O3 precursors including nitrogen oxides (NOx = NO2 + NO), methane (CH4) and carbon monoxide (CO). For instance, under the Intergovernmental Panel on Climate Change (IPCC) Representative Concentration Pathway 8.5 (RCP8.5), the global tropospheric O3 burden is estimated to increase from 337 Tg in year 2000 to 395 Tg in year 2100 (Young et al., 2013).
To assess the impacts of O3 pollution on crop yields, several metrics have been developed based on results from both field and open-top chamber experiments. The first batch of these metrics are various concentration-based metrics, including daylight-hour growing-season average O3 concentration (M7 or M12), accumulated daylight O3 concentration above certain thresholds (AOT40 and SUM06), and continuously weighted growing-season average O3 concentration (Adams et al., 1989; Lesser et al., 1990; Lee and Hogsett, 1996; Fuhrer et al., 1997; Wang and Mauzerall, 2004; Mills et al., 2007). AOT40 and SUM06 in particular consider the baseline ability of plants to detoxify low levels of O3, whereby crops suffer yield losses only if the hourly O3 concentration exceeds 40 (AOT40) or 60 (SUM06) ppb. W126 considers the non-linear response of yield loss to O3 exposure whereby higher concentrations will result in progressively more severe yield losses. These metrics have been used extensively to evaluate global yield losses due to present-day O3 concentrations, which are estimated to be 2–16% for the four major staple crops (wheat, rice, maize, soybean) (Ainsworth, 2017). These metrics are easy to calculate, but they typically do not consider the combined effects of O3 with other factors that regulate plant O3 uptake, and overlook the active responses of plant ecophysiology to ambient climatic and environmental changes and thus likely inadequate for examining yield losses in a future climate and atmospheric environment (Musselman et al., 2006). The co-varying factors that may modulate O3-crop relationships include ambient CO2 concentration, temperature, drought conditions, soil water stress, etc., all of which may undergo tremendous changes under future climate change conditions (Emberson et al., 2000; Tai et al., 2014).
O3-induced plant damage is mainly caused by the stomatal uptake of O3 into the leaf interior instead of direct plant surface deposition (Amiro et al., 1984; Nouchi, 2002). Stomatal uptake of O3 can be strongly influenced by the extent of stomatal opening, which is usually quantified as stomatal conductance (gs) and strongly dependent on environmental conditions such as soil water availability (e.g., Tingey and Hogsett, 1985), light intensity (e.g., Paoletti and Grulke, 2010), and CO2 concentration (e.g., Onandia et al., 2011). Atmospheric CO2 concentration has been rising, and plants generally respond by reducing stomatal conductance, thereby reducing water loss from transpiration and improving water use efficiency (WUE) (e.g., Franks et al., 2013). The closure of the stomata indirectly alleviates the uptake of O3 and thus the damage inflicted on them by O3. Some studies showed that O3-induced yield loss is alleviated under elevated CO2 concentration (Fiscus et al., 1997; Tao et al., 2017). Meanwhile, higher CO2 itself enhance photosynthesis rates and crop yields via the fertilization effect (e.g., Rosenzweig et al., 2014), which may further complicates crop-O3-CO2 relationships. Likewise, temperature extremes and the often associated drought conditions not only damage crop yields but also modulate crop physiology and thus their uptake of O3. Moreover, temperature and O3 concentration are often highly positively correlated with each other (Jacob and Winner, 2009), which can confound any observed yield-temperature and yield-O3 relationships as found in observational and experimental studies (Tai et al., 2014; Tai and Martin, 2017). For all these reasons, the concentration-based exposure-yield loss metrics described above may not hold in the future as they do not account for how crop physiological responses to the changing atmospheric environment may interfere with stomatal O3 uptake and damage, or how O3 may vary with other environmental conditions that also affect crops.
In the recent two decades a newer approach has been developed and popularized to assess the statistical relationships between stomatal O3 uptake and crop yields (Emberson et al., 2000; Pleijel et al., 2007). In this, so called, flux-based approach, the stomatal control of O3 uptake and its environmental dependence (especially in relation to soil water availability) are explicitly taken into account. This approach usually does not require mechanistic simulation of ecophysiological processes (e.g., photosynthesis, carbon allocation) and responses of crops. Typically, stomatal conductance is formulated as a semiempirical function of a baseline, standard-conditioned stomatal conductance modified multiplicatively by a series of “activity factors” accounting for variations in environmental conditions; such kind of a multiplicative function is usually termed “Jarvis-type” formulation due to its origin in the study of Jarvis (1976). Generally, the calculated instantaneous O3 flux, above a certain threshold (e.g., Y nmol O3 m−2 s−1) to account for natural detoxification, is then cumulated over the growing season to calculate a phytotoxic O3 dose (PODY, mmol O3 m−2); the statistical relationships between PODY and crop yields have been determined by several experiments thus far, and are used to determine O3 impacts on crop yields. Such flux-based metrics have been shown to perform better in statistical terms than concentration-based metrics, and better predict the distribution of O3 damage when combined with O3 fields simulated by regional atmospheric chemistry models (Pleijel et al., 2007; Millls et al., 2011). An important reason for their better performance is that they explicitly account for stomatal control of O3 uptake, the variation of which can largely influence crop damage even with the same ambient O3 concentrations (Clifton et al., 2020).
Finally, O3 impacts on crops can also be estimated using mechanistic (“process-based”) models that explicitly consider the effects of O3 flux (either instantaneous or cumulative) on photosynthesis rate and/or stomatal conductance, which ultimately affects crop yields through modifying carbon assimilation and other crop biogeochemical processes. Such an approach has been applied in tandem with land surface and terrestrial ecosystem models that include a crop component, e.g., the Community Land Model (CLM) (Oleson et al., 2013), Joint-UK Land Environment Simulator (JULES) (Osborne et al., 2015), and MOSES-TRIFFID (Sitch et al., 2007). Compared to the metric-based methods, using a mechanistic crop model can more fully simulate different agricultural practices (e.g., irrigation) and crop physiology fully coupled with photosynthesis, which responses to varying environmental conditions including long-term changes in climate and atmospheric chemical composition. However, applications of these process-based models to a large or global scale are challenging due to limited O3 flux data for crops to more comprehensively parameterize the essential model components; most large-scale O3-crop impact studies to date have still relied on concentration-based or flux-based metrics.
The above three approaches to estimate O3 impacts on crops all have different input requirements and have traditionally been used with different modeling frameworks, which makes cross-comparison among them challenging. In this paper, we describe and compare present-day O3-induced yield losses using these different methods including concentration-based metrics (M7/M12, AOT40, W126), flux-based metrics (PODY) and a mechanistic crop model, essentially keeping input requirements and modeling frameworks as consistent as possible so as to reduce inter-model discrepancies that are not due to fundamental methodological differences (e.g., input ozone fields and meteorology).
We use the Terrestrial Ecosystem Model In R (TEMIR) to compute biogeophysical responses of terrestrial ecosystems, including crops, to changes in the atmospheric and terrestrial environment. Equipped with the same driving meteorology as the GEOS-Chem chemical transport model (CTM), TEMIR is designed to be highly compatible with GEOS-Chem so that these two models can be coupled asynchronously to examine atmospheric chemistry-biosphere interactions. TEMIR can simulate up to 24 plant function types (PFTs), with an additional bare land type, which are the same as the Community Land Model version 4.5 (CLM4.5). For these 24 PFTs, default TEMIR can calculate photosynthesis and stomatal conductance based on the Farquhar-Ball-Berry (FBB) model as described by Oleson et al. (2013), which could capture the environmental conditions including radiation, temperature, and soil water availability. Eight of the 24 PFTs are human-managed crops, including maize, soybean, winter cereal and temperate cereal, each being either rainfed or irrigated. As opposed to natural vegetation, human-managed crops have their unique phenology that controls the allocation of the assimilated carbon. They have a separate carbon stock that keeps track of the carbon allocated to the pod, which is turned into a crop yield at the end of the growing season. In addition to the default stomatal conductance scheme that is fully coupled with photosynthesis, we also implement a Jarvis-type stomatal conductance scheme that is consistent with the Deposition of O3 for Stomatal Exchange (DO3SE) model (https://www.sei.org/projects-and-tools/tools/do3se-deposition-ozone-stomatal-exchange/), which has been developed to estimate the risks of O3 damage to European vegetation according to Long-Range Transboundary Air Pollution (LRTAP) methodologies for effect-based risk assessment. Both FBB-based and DO3SE-based stomatal conductances are used to calculate O3 fluxes and PODY. To stay consistent with Mills et al. (2018a), we choose Y = 3 nmol O3 m−2 s−1 in this study.
Distribution of croplands is based on the year-2000 global land surface from the Community Land Model version 5 (CLM5), replacing the cropland distribution used in CLM4.5 whereby crops are missing in the tropical regions. The new input is then regridded to a resolution of 2° latitude by 2.5° longitude consistent with GEOS-Chem, which provides the percentage coverage of major crops including maize, soybean, wheat and rice. In TEMIR, crops are simulated when the land coverage of the crops is >1%. Figure 1 shows global distribution of average production of the four staple crops; data are from the Food and Agriculture Organization (FAO), mapped onto the 2° × 2.5° grid using a data fusion technique following Tai et al. (2014).
Figure 1. Crop production for (A) maize, (B) wheat, (C) soybean, and (D) rice averaged over 2006–2010, shown only when production >500 Mg per grid cell.
Crop calendar is based on the global dataset from Sacks et al. (2010), which provides planting and harvesting dates of major crops including maize, soybean, wheat and rice with a resolution of 0.5° longitude by 0.5° latitude. For crops that have multiple growing season in a year (e.g., maize and rice in the tropical regions), only the primary growing season is considered in this study. With these inputs, planting dates can be prescribed in our simulations and harvesting dates can be either prescribed or estimated using growing degree day (GDD) (section Exposure-Yield Relationships). Supplementary Figure 1 shows the harvesting dates used in our study.
Decidedly, the same O3 concentration and meteorological fields are used throughout to ensure inter-model differences are methodological but not input-driven. The present-day O3 concentration field is simulated by the GEOS-Chem global CTM (http://acmg.seas.harvard.edu/geos/), which simulates fully coupled O3-NOx-VOC-aerosol chemistry together with the emissions, transport and deposition of important atmospheric chemical species. For our simulations of O3, GEOS-Chem is driven by assimilated meteorological data at 2° × 2.5° resolution from the reanalysis product Modern-Era Retrospective analysis for Research and Applications, Version 2 (MERRA-2), which is also used as the input meteorology of TEMIR to ensure internal consistency in the TEMIR-GEOS-Chem framework. The surface O3 concentrations simulated by GEOS-Chem are then used to directly calculate the various concentration-based exposure metrics, and also together with MERRA-2 meteorology as inputs for TEMIR to simulate the corresponding POD3, photosynthetic damage and crop yield losses.
In this study, we consider three different ozone exposure metrics widely used to quantify ozone exposure and impacts on crops. They include two cumulative exposure metrics (AOT40 and W126) and one mean exposure metrics (M12 or M7). They are defined as:
where [O3]i (ppm) is the hourly mean O3 concentration during the 12 h of local daylight (08:00–19:59); n is the number of hours in the 3-months growing season defined as the 3 months prior to the start of the harvesting period in every country according to the crop calendar; and wi is a weighting function assigning greater weight to higher levels of hourly O3 (Lefohn et al., 1988), defined by:
AOT40 and W126 are in units of ppm-h, and M12 in units of ppb. M7 is defined similarly to M12 but uses a 7-h (09:00–15:59) daytime window instead of 12-hour for summation. To remain consistent with the flux-based metrics, both cumulative exposure metrics and mean exposure metrics are cumulated and averaged, respectively, consistently over the same growing period, from 104 days before the prescribed harvesting date to 14 days before the prescribed harvesting date (Supplementary Figure 1). Supplementary Figures 2–4 show the global distribution of these metrics as calculated from the O3 concentration field simulated by GEOS-Chem for year 2010.
In order to calculate the flux-based metrics, PODY, we simulate stomatal conductance (gs) using both the default TEMIR method of directly coupling gs with net photosynthesis rate (An), and the semiempirical, Jarvis-type formulations as in DO3SE. For both methods, leaf-level PODY (mmol m−2) is calculated as:
where the threshold in this study is Y = 3 nmol O3 m−2 s−1, Δt is the time step (Δt = 3,600 s in TEMIR), and the O3 flux, FO3 (nmol O3 m−2 s−1), minus the threshold is cumulated over a 90-days growing period, from 104 days before the prescribed harvesting date to 14 days before the prescribed harvesting date (Supplementary Figure 1).
The O3 flux is calculated as:
where [O3] (nmol m−3) is the ozone concentration observed or of the lowest atmospheric model layer. Ra (s m−1) is the aerodynamic resistance, and Rb (s m−1) is the quasilaminar (boundary-layer) resistance. We use the same set of equations to be consistent with DO3SE, which are detailed in Supplemental Information. Both Ra and Rb are bulk resistances. In contrast, the stomatal resistance, rs (mmol−1 O3 m2 s) = 1/gs, is at the leaf level, and its unit is converted to s m−1 using a conversion factor α ≈ 42,000 mmol m−3.
The formulation of stomatal conductance for O3, gs (mmol O3 m−2 s−1), is what differs between FBB and DO3SE. In the default TEMIR FBB calculation, gs is coupled with net photosynthesis rate (An, μmol CO2 m−2 s−1) via:
where gs is controlled by the leaf surface CO2 partial pressure cs (Pa), leaf surface water vapor pressure es (Pa) and temperature-dependent saturation vapor pressure esat (Pa); m = 9 and b = 10,000 μmol m−2 s−1 for C3 plants, and m = 4 and b = 40,000 μmol m−2 s−1 for C3 plants; βt (ranging from 0 to 1) is the soil water stress function that accounts for water limitation, and this factor is also applied internally to An during its computation; the factor 1.61 × 103 is the ratio of leaf conductance for water (μmol H2O m−2 s−1) to that for O3 (mmol O3 m−2 s−1). The conductance gs in FBB is calculated separately for sunlit and shaded leaves (i.e., gssun and gssha, respectively) and the canopy-averaged leaf-level gs is:
where LAIsun and LAIsha are the leaf area index (LAI) of sunlit and shaded leaves, respectively.
In contrast to the photosynthesis-based formulation, the stomatal conductance gs (mmol O3 m−2 s−1) in the default DO3SE model is a semi-empirical multiplicative function:
The definitions of different terms in Equation (12) that account for variations in various environmental factors, including plant-type-specific phenology (f phen), light (f light), temperature (fT), vapor pressure deficit (VPD, fD), and soil water potential (SWP, f SW), are listed in Supplementary Table 1.
Figures 2, 3 show the simulated POD3 with the DO3SE multiplicative formulation and default FBB photosynthesis-based formulation, respectively. In general, they show very similar spatial patterns for all four crops, with the DO3SE method generally yielding higher POD3 than FBB, reflecting systematically higher values of gs simulated by DO3SE.
Table 1 lists the statistical relationships of crop yields with the concentration-based exposure metrics (AOT40, M7/M12, W126) and flux-based metrics POD3, summarized from various sources. These metrics have been used extensively to estimate global yield losses (e.g., Mills et al., 2018a). The statistical relationships between the flux-based metrics and crop yields are usually called “dose-response” functions; those between the concentration-based metrics and crop yields are likewise called “concentration-response” functions. Relative yield (RY) is defined to be the ratio of O3-affected yield to unaffected yield at zero O3 exposure. In this study, we find RY for each 2° × 2.5° grid cell i; we then calculate the globally or regionally aggregated yield loss (L, %) from the grid cell-by-grid cell relative yield (RYi) loss following:
where P0i is the theoretical production in each grid cell i without O3 damage, Pi is the actual production as shown in Figure 1, and the summation is over the whole world for globally aggregated estimates, or over the whole region for regionally aggregated estimates. This approach of aggregating regional and global yield (more accurately, production) losses is consistent with previous studies (Ainsworth, 2017; Mills et al., 2018b) with which we will compare our results. We note however that these different metrics were derived from different experiments that might not always have consistent study designs or environmental controls even for the same crop, which may further add to the uncertainties represented merely by the use of different metric definitions.
Finally, we also attempt to estimate crop yield losses based on a biophysical crop model built on the TEMIR model, whereby the instantaneous stomatal O3 uptake directly affects the simulated rate of photosynthesis and/or stomatal conductance, and subsequently impacts on crop yield via modifying carbon fixation and allocation. We may call it the “plant response-based” approach, and this is as opposed to using the cumulative O3 uptake (i.e., PODY) to directly estimate relative yield (i.e., using dose-response functions). To simulate the impacts of O3 on crop growth and yield, here we adopt the O3 damage parameterization developed by Sitch et al. (2007) (Sitch scheme). As with the computation of PODY, the parameterization of the Sitch scheme makes use of the instantaneous stomatal O3 flux (FO3), but instead of computing a cumulative PODY from FO3, it considers a photosynthetic damage factor f (i.e., fractional reduction) that is a function of FO3:
where Fcrit represents a critical threshold accounting for O3 tolerance, below which instantaneous O3 exposure does not affect photosynthesis, and Fcrit = 5 nmol m−2 s−1 for all crops; the O3 sensitivity parameter b (nmol−1 m2 s) is specific to the crop with different photosynthetic pathways. Soybean is treated as a C3 grass and maize is treated as a C4 grass in this study (Supplementary Table 3). Fractional reduction factor f is multiplied directly to the net photosynthesis rate An to represent O3 damage, which then indirectly affects gs via the coupling between An and gs; f, An, and gs are solved together iteratively.
Crop yield (Y, gC m−2) can be inferred from the accumulated assimilated carbon (i.e., net primary production or NPP) during the growing season, which is given as:
where R is the plant respiration rate that depends on the total biomass and An; arepr is the biomass partitioning fraction toward plant reproductive organs that depends on growing degree days (GDD) which is calculated by the accumulation of temperature. Similarly, leaf area index (LAI) can be calculated using the above formula by considering the carbon stock of leaf for the whole plant:
where SLA is the specific leaf area (m−2 gC) of the plants and Lsen is the loss of leaf carbon during the later growing stage. The parameterization of Lsen in our model follows the CLM4.5 crop model (Oleson et al., 2013), in which the leaf loss rate is proportional to the leaf carbon stock. Using these two equations, we can infer that O3 can reduce the NPP and thereby crop yield through reducing the leaf-level photosynthetic rate as well as the LAI. The reduction of An due to the O3 damage during the early growing stage can result in loss in leaves as plant prioritizes carbon allocation toward leaf. Whereas, in the later growing stage when plants prioritize the allocation toward pods, it can result in less carbon assimilation for the grain. These two pathways also apply for crops that are grown under elevated CO2 levels, which per se generally increase crop yield by enhancing An. In addition, higher CO2 levels can lead the reduction of gs, which in turn indirectly lowers the uptake of O3 through the stomata and thus reduce O3 damage. These interactive pathways involving CO2 and O3 within crops can only be resolved using a mechanistic model like this one described above. Important parameterization schemes and parameter values are listed in Supplementary Table 2.
We also test another plant response-based approach using cumulative (instead of instantaneous) uptake of O3 (CUO), which is similar to POD, to alter photosynthesis rate and stomatal conductance. The CUO-An and CUO-gs relationships follow the linear statistical relationships consolidated by Lombardozzi et al. (2013) for different plant types. However, the specific statistical relationships for crops only have modest sensitivity of An or gs to CUO beyond a given threshold, and when implemented in TEMIR-crop they result only in minimal damage on crop yield. While theoretically using CUO to construct plant response-based metrics may be preferable to using instantaneous uptake, we resort to use the Sitch scheme only for comparing with the exposure metrics and do not calibrate the Lombardozzi scheme further, as this part of the study aims only to demonstrate how exposure metrics are unable to capture the effects of rising CO2.
To illustrate the effect of elevated CO2 concentration on crop yield and O3 damage, factorial simulations using TEMIR with the biophysical crop module are conducted, which consist of two O3 levels (no O3, present-day O3 concentration), two plant-to-O3 sensitivities in the Sitch scheme (Supplementary Table 3) and two CO2 levels (390 and 600 ppm). These CO2 levels resemble the CO2 concentrations during year 2010 (390 ppm) and the experimental conditions of most of the Free-Air CO2 Enrichment (FACE) experiments (600 ppm).
Figure 4 shows the regional averages of different O3 exposure metrics for different major crop-producing regions for the four staple crops, corresponding to the maps of Supplementary Figures 2–4 and Figures 2, 3. In general, the spatial patterns of the severity of O3 exposure are consistent across the metrics. For a given region, however, flux-based metrics (POD3) generally show similar or higher variability across crops than concentration-based metrics (AOT40, M7/M12, W126), reflecting the dependence of the flux-based metrics on crop-specific phenology and ecophysiology.
Figure 4. Regional average O3 metric values for East Asia (EA), Europe (EU), South America (SA), and United States (US). Units: ppm-h for AOT40, W126; ppb for M7/M12; mmol m−2 for POD3-DO3SE, POD3-FBB.
Figures 5–9 show the maps of relative yield (RY) based on the three concentration-based and two flux-based O3 exposure metrics. While as in the case for the metrics themselves RY for a given crop show generally consistent spatial distribution across different metrics, the magnitudes of RY appear to differ quite substantially. Below we diagnose these differences and compare them with results from previous studies both globally and regionally.
Figure 5. Relative yield based on the AOT40 metric for (A) maize, (B) wheat, (C) soybean, and (D) rice.
Figure 6. Relative yield based on the M7 or M12 metric for (A) maize, (B) wheat, (C) soybean, and (D) rice.
Figure 7. Relative yield based on the W126 metric for (A) maize, (B) wheat, (C) soybean, and (D) rice.
Figure 8. Relative yield based on the flux-based POD3 metric with DO3SE formulation for (A) maize, (B) wheat, (C) soybean, and (D) rice.
Figure 9. Relative yield based on the flux-based POD3 metric with FBB formulation for (A) maize, (B) wheat, (C) soybean, and (D) rice.
Figure 10 shows the aggregated global yield losses from our study for the four crops and different exposure metrics, as well as summarized results from Mills et al. (2018b), which used the flux-based approach similar to POD3-DO3SE to estimate global losses, and Ainsworth, 2017, which presented from AOT40-derived and M7/M12-derived estimates from van Dingenen et al. (2009) and Avnery et al. (2011). Even with a consistent set of O3 concentrations as input into the calculation, our estimates of global yield losses from different metrics differ quite substantially for a given crop. W126 almost always gives the lowest losses, almost appearing to be outlying compared to the other metrics, except for wheat. The concentration-based metrics differ greatly among themselves. In contrast, the two flux-based metrics are generally close to each other, and lie close to the middle of the range covered by all metrics. POD3-DO3SE always gives slightly higher loss estimates than POD3-FBB, mostly reflecting the generally lower stomatal conductance calculated by photosynthetic coupling (as in FBB) than a Jarvis-type multiplicative approach (as in DO3SE). Generally either AOT40 or M7/M12 would give the largest losses for a given crop.
Figure 10. Aggregated global yield loss (%) estimates for four major crops using AOT40, M7/M12, W126, POD3-DO3SE, and POD3-FBB. Shown also are previous findings: Mills et al. (2018b) used flux-based metrics similar to POD3-DO3SE, while Ainsworth (2017) considered AOT40 and M7/M12.
When compared with estimates from previous studies, our estimates of global yield losses using AOT40 and M7/M12 are generally quite consistent with that summarized by Ainsworth (2017), except for wheat, whereby our AOT40 estimate is higher but M7/M12 estimate is lower than theirs. Our flux-based estimates are, however, always quite substantially lower than that found by Mills et al. (2018b). Their estimates are almost always on the high end of the range given by all metrics, except for wheat. The larger differences between our flux-based estimates and theirs may reflect a multitude of factors, but since the methodological approaches are largely similar and we use the same dose-response relationships, we ascribe the differences primarily to the differences in O3 and meteorological fields used as inputs for the calculation.
Figure 11 shows the regionally aggregated yield losses for four major crop-producing regions (our estimates only). As in the case for globally aggregated losses, AOT40 and/or M7/M12 generally lead to the largest yield losses for most regions and crops, W126 leads to the smallest (except for wheat), and the flux-based metrics lead to losses in the middle of the range. What is particularly noteworthy is that for a given crop, the differences in yield losses among the metrics themselves in the same region can be often larger than the inter-regionally differences. This suggests again that the greatest uncertainty behind O3-induced yield losses appears to come from methodological differences, which can be even larger than that from the differences in input data. Comparing across different crops, soybean and wheat appear to suffer greater losses from O3 damage than maize or rice, which is consistent with previous studies (e.g., Ainsworth, 2017; Tai and Martin, 2017; Mills et al., 2018b). However, when we carefully compare across metrics, it appears that very high yield losses for soybean and wheat are mostly driven by a single metric (M7/M12 for soybean, AOT40 for wheat); the average losses over all metrics and the losses derived from the flux-based metrics for soybean and wheat, though still higher than, are generally closer to that for maize or rice.
Figure 11. Regionally aggregated yield losses (%) for maize, rice, soybean, and wheat, using different metrics. Four regions are East Asia (EA), Europe (EU), South America (SA), and United States (US).
Finally, we present findings using a mechanistic crop model, which can help examine the direct impacts of O3 on plant physiology whereas using the metrics cannot. Only the results for maize and soybean are presented due to the lack of validation data for wheat or rice. Under the influence of O3, global mean photosynthesis rate under present-day O3 concentrations and ambient CO2 concentration relative to the scenario without O3 is reduced by 18–34% for maize and 33–56% for soybean (Supplementary Figure 5), depending on the sensitivity of crops to O3. Reduction of crop yield couples tightly with the reduction of photosynthesis rate, and yield decreases by 21–38% for maize and 36–59% for soybean (Figure 12). Another impact of O3 on plant physiology is the reduction of LAI (Supplementary Figure 6), whereby our results suggest that maximum growing season LAI is reduced by about 1–2 under low plant-O3 sensitivity. Compared to other major maize-planting region, maize in Europe has higher maximum growing LAI and reduction in maximum LAI caused by O3, which can be attributed to the overestimation of the length of the vegetative stage.
Figure 12. Mean relative yield of maize under (A) low and (B) high plant-to-O3 sensitivity, and mean relative yield of soybean under (C) low and (D) high plant-to-O3 sensitivity, as simulated by TEMIR-crop under a present-day CO2 concentration of 390 ppm.
The Sitch scheme used to estimate O3 damage comes with a range of damage with low and high sensitivity to O3. Based on the relative yield given by the model, we find the globally aggregated yield loss for maize to be 9.5 and 41% with low and high O3 sensitivity, respectively, at preset-day CO2 level. For soybean, the global yield loss is estimated to be 26 and 63%, respectively. These losses are larger than that given by the exposure metrics presented above in Section Comparing Different O3 Exposure Metrics and Yield Losses in which maize loss is around 0–5% and soybean loss is around 2–16%, with the low-sensitivity estimates being closer and more reasonable as compared with the metric-based damage. We further compare our simulation results for soybean with the analysis conducted by Morgen et al. (2003), whereby crop yield decreases only by around 6 ± 5% when O3 level is between 30 and 59 ppb and 24 ± 4% when O3 level is between 60 and 79 ppb. For maize, based on the study by Peng et al. (2019), observed yield changes were +4.1, −2.7, and −16.6% for maize growth under daylight O3 levels of 39, 60, and 76 ppb, respectively. In addition, the effect of O3 on peak LAI is not significant unless plants are exposed to >100 ppb of O3 for a long time (Dermody et al., 2006; Betzelberger et al., 2012). This suggests that even with the low-sensitivity parameters, the Sitch scheme may be overestimating the sensitivities of soybean and maize to O3.
At 600-ppm CO2 level, O3-induced yield losses are found to be always smaller. The globally aggregated yield loss for maize is 3.5 and 5.6% for low and high sensitivity, respectively, and for soybean is 19 and 29% for low and high sensitivity, respectively. Comparing between the O3-induced yield losses at 390 vs. 600 ppm CO2, the higher CO2 concentration can reduce the maize yield loss by 21 and 52% for low and high sensitivity, respectively, and reduce the soybean yield loss by 27 and 38% for low and high sensitivity, respectively. The results from the SoyFACE experiments suggest that at elevated O3 (+25% ambient level), soybean relative yield losses are 10 ± 11% and 5 ± 4% under 370 and 550 ppm of CO2, respectively; i.e., higher CO2 concentration reduces O3-induced yield loss by roughly half (Morgen et al., 2005, 2006). However, the uncertainty of yield losses is still large, which could be attributable to the insufficient numbers and durations of experiments conducted (3 years at elevated CO2; 5 years at ambient CO2 level).
Impacts of higher CO2 concentration level (600 ppm) on plant physiology is reflected on the change of stomatal conductance; global leaf-level stomatal conductance is reduced by 14% for maize and 31–33% for soybean relative to 390 ppm CO2 (Supplementary Figure 7), which indicates that O3-induced yield loss is less severe under higher CO2 concentration (Supplementary Figures 8–11). Compared to the studies by Ainsworth et al. (2002) and Leakey et al. (2004), the reductions in stomatal conductance under elevated CO2 levels are consistent for both maize (−23% at 550 ppm relative to 350 ppm CO2 in their studies) and soybean (−36% at 600 ppm CO2 in their studies), respectively. Supplementary Figures 8–11 show the regression between RY and AOT40 at different crop planting regions. AOT40 is used here only as an indicator of O3 exposure for better comparison with the exposure metrics. It shows that for most of the regions, RY is higher when the CO2 level is higher. It also suggests that the respond of RY to different AOT40 levels varies in different regions, which can be explained by the fact that AOT40 does not take account into the O3 effect during the early growing stage. As mentioned in Section Mechanistic Crop Model and Flux-Based Ozone Damage Parameterization, O3 damage in the early growing stage can lead to foliage loss, limiting carbon assimilation in the later growing stage in which carbon is mainly allocated to the grain. In addition, the threshold in which plants suffer from O3 damage is solely dependent on ambient O3 concentration (40 ppb) for AOT40, whereas in the Sitch scheme and flux-based metrics the thresholds are dependent on meteorological conditions such as radiation and humidity. Hence, it is possible that O3 can inflict damage on plants even the ambient O3 concentration does not exceed 40 ppb. Figure 13 summarizes the RY losses under different CO2 concentrations in four major planting regions. Though the discrepancy in yield loss predictions between using metrics and using a mechanistic crop model is large, it can be minimized by calibrating the O3-plant damage scheme using crop-specific field observations. It is important to note that the impacts of O3 damage and elevated CO2 levels on plants are not solely about photosynthetic rate and stomatal conductance. For instance, O3 damage can lead to early senescence of leaves and plants can adapt to new biomass allocation strategy under higher CO2 concentration by investing more carbon for root development.
Figure 13. Summary of TEMIR-simulated relative yield at four different regions under 390 and 600 ppm CO2 concentration. The error bar represents one standard deviation of the data. Data that is above the 95th percentile or below the 5th percentile is removed.
The exposure to O3, a phytotoxic chemical, brings tremendous harm to crops, but their impacts on global and regional crop yield even for the present day are far from certainty. The large uncertainty may first stem from: (1) large spatiotemporal variability of O3 concentration itself that is not adequately quantified, due to incomplete observations and inter-model differences in O3-relevant process representation in different models; (2) different methodological approaches to quantify O3 exposure and impacts, including the concentration-based approaches, flux-based approaches, and mechanistic crop models; (3) co-varying factors such as CO2 concentration and other environmental conditions (heat stress, water stress, etc.) that are not explicitly accounted for in many of the commonly used approaches. In this study, we therefore attempt to explore these issues using a common methodological framework: a consistent set of simulated present-day O3 fields from one chemical transport model, coupled with a terrestrial ecosystem model (including crops) to derive various O3 exposure metrics and impacts on relative crop yields worldwide. Throughout, we also review and explain in details the differences in formulation and parameterization in different approaches. Finally, we also explore the effects of elevated CO2 on O3 damage to crops.
We find that while the spatial pattern of yield losses for a given crop is generally consistent across metrics, the magnitudes can differ substantially. Sometimes, the inter-metric differences can be greater than the inter-regional differences. Overall, pulling two concentration-based (AOT40, M7/M12) and two flux-based (POD3-DO3SE, POD3-FBB) together (excluding W126 due to its outlying tendency and lack of comparison with other studies), we estimate the globally aggregated yield losses for the four staple crops to be (± standard deviation): 3.6 ± 1.1% for maize, 2.6 ± 0.8% for rice, 6.7 ± 4.1% for soybean, and 7.2 ± 7.3% for wheat. The average yield losses we find are generally consistent with previous studies (e.g., Ainsworth, 2017; Mills et al., 2018b), but on the lower end of the full uncertainty range previously covered (especially for wheat). We attribute the relative large combined uncertainty we find mostly to the differences among methodological approaches (even within our own study with consistent inputs), and secondarily to differences in inputs (as compared with other studies).
The exposure metrics, either concentration-based or fluxed based, which are almost always derived from well-controlled, single-factor experiments, are generally ill-suited to account for the effects of co-varying and possibly confounding environmental factors, including CO2 concentration, temperature, precipitation, drought frequencies or intensity, etc. We further explore how rising ambient CO2 concentration in the future may affect the impacts of O3 on crop yields using a mechanistic biophysical crop model that explicitly simulates the effects of O3 uptake on photosynthesis rates and thus downstream on crop yields (i.e., plant response-based metrics. Crop ecophysiology embedded within the crop model is able to account for co-varying factors such as CO2, temperature, soil water availability. We find that, first, our mechanistic model as of the current version gives very different, much larger relative yield losses than the exposure metrics; this is expected as the ozone-induced damage parameterization that we have adopted in the simulations is generalized from studies with C3 and C4 grasses, with a lack of data for more refined calibration in different regions for specific crops. We should therefore only focus on the relative differences between two different CO2 levels to infer their impacts. We find that increasing CO2 concentration from 390 ppm (representative of 2010) to 600 ppm could reduce stomatal conductance by 14% for maize and 31–33% for soybean, consistent with the finding from SoyFACE experiments. The globally aggregated O3-induced yield loss is reduced by 21–52% for maize and by 27–38% for soybean. Although the reduction in O3-induced yield loss for soybean is smaller than the field-observed value (54%), it still reflects a CO2-induced reduction in stomatal conductance that in turn quite substantially alleviates stomatal O3 uptake and thus damage to yields. Rising CO2 may therefore render the currently used exposure-yield relationships less applicable in a future atmosphere.
Many modeling studies have used mechanistic crop models to predict future crop yields under rising CO2 concentrations and changes in other co-varying factors such as climatic heat stress (Deryng et al., 2014; Rosenzweig et al., 2014); elevated CO2 is generally found to substantially enhance crop yields via the fertilization effect. However, modeling results for the combined effects of CO2 and O3 pollution on different crops are thus far limited [e.g., Tao et al. (2017) and Guarin et al. (2019) for wheat only; Lombardozzi et al. (2018) for eight crop types], and observations or experiments that may validate the wide applicability of mechanistic crop models for the co-effects of CO2 and O3 are scarce and limited to few crops [e.g., Dermody et al. (2006) for soybean; Rudorff et al. (1996); Cardoso-Vilhena et al. (2004); and Feng et al. (2008) for wheat]. Meanwhile, although the exposure metrics (especially flux-based metrics such as POD3) have found success in assessing current and future impacts of O3 pollution on global crop yields and food security, they are inherently incapable of capturing the co-effects of CO2, which calls into questions their applicability in a future atmosphere enriched with CO2. Ultimately, greater effort should be put into conducting experiments for a basket of globally important crops that explicitly consider co-varying levels of O3 and CO2 as well as co-varying hydrometeorological conditions, and the experimental results can then be used to derive revised exposure-yield relationships that depend on changing CO2 concentration and climatic stress. Before that is possible, a shortcut we suggest is to rely on the mechanistic understanding of CO2-O3 co-effects embedded in either an FBB-like photosynthesis-stomatal conductance model or a biophysical crop model. For instance, since the FBB model explicitly considers the reduction in stomatal conductance and thus O3 uptake at elevated CO2, a framework similar to POD3-FBB can be used directly to evaluate the CO2-O3 co-impacts on crop yields based on existing POD3-yield relationships. This approach, however, can only account for the effect of CO2 via modifying stomatal conductance, but cannot represent the CO2 fertilization effect on crop yields via enhanced photosynthesis. Only a fully mechanistic biophysical crop model (e.g., TEMIR-crop, CLM-crop, JULES-crop, etc.) can incorporate the CO2 fertilization effect. Thus, an alternative approach is to use such a crop model to derive scaling factors that account for the variations in simulated O3-yield relationships at different CO2 levels; these factors can then be used to scale existing exposure-yield relationships. These approaches may be valuable in assessing the global impacts of O3 pollution on crop production and food security over the next coming decades as ambient CO2 concentration is expected to continue to rise, particularly before more of the long-term CO2−O3 covarying experiments for a larger variety of crops can be done.
Data used for generating the figures in this paper can be found online (http://www.cuhk.edu.hk/sci/essc/tgabi/data.html). Full datasets can be made available on request to the corresponding author.
AT coordinated the study, performed data analysis, and wrote the manuscript. MS performed model development and simulations of ozone exposure metrics and yield losses. JP performed model development and simulations of biophysical crop model. DY simulated the ozone concentration fields. ZF performed data analysis and assisted in writing. All authors contributed to the article and approved the submitted version.
This study was supported by the Vice-Chancellor Discretionary Fund (Project ID: 4930744) from The Chinese University of Hong Kong (CUHK) given to the Institute of Environment, Energy and Sustainability, and the funding from the State Key Laboratory of Agrobiotechnology (Project ID: 8300070) at CUHK.
The authors declare that the research was conducted in the absence of any commercial or financial relationships that could be construed as a potential conflict of interest.
The Supplementary Material for this article can be found online at: https://www.frontiersin.org/articles/10.3389/fsufs.2021.534616/full#supplementary-material
Adams, R. M., Glyer, J. D., Johnson, S. L., and McCarl, B. A. (1989). A reassessment of the economic effects of ozone on united states agriculture. J. Air Pollut. Control Assoc. 39, 960–968. doi: 10.1080/08940630.1989.10466583
Ainsworth, E. A. (2017). Understanding and improving global crop response to ozone pollution. Plant J. 90, 886–897. doi: 10.1111/tpj.13298
Ainsworth, E. A., Davey, P. A., Bernacchi, C. J., Dermody, O. C., Heaton, E. A., Moore, D. J., et al. (2002). A meta-analysis of elevated [CO2] effects on soybean (Glycine max) physiology, growth and yield. Glob. Chang. Biol. 8, 695–709. doi: 10.1046/j.1365-2486.2002.00498.x
Alexandratos, N., and Bruinsma, J. (2012). Food and Agriculture Organization, World Agriculture Towards 2030/2050: The 2012 Revision. Rome: FAO.
Amiro, B. D., Gillespie, T. J., and Thurtell, G. W. (1984). Injury response of Phaseolus Vulgaris to ozone flux density. Atmos. Environ. 18, 1207–1215. doi: 10.1016/0004-6981(84)90152-5
Avnery, S., Mauzerall, D. L., Liu, J. F., and Horowitz, L. W. (2011). Global crop yield reductions due to surface ozone exposure: 1. Year 2000 crop production losses and economic damage. Atmosp. Environ. 45, 2284–2296. doi: 10.1016/j.atmosenv.2010.11.045
Betzelberger, A. M., Yendrek, C. R., Sun, J., Leisner, C. P., Nelson, R. L., Ort, D. R., et al. (2012). Ozone exposure response for U.S. soybean cultivars: linear reductions in photosynthetic potential, biomass and yield. Plant Physiol. 160, 1827–1839. doi: 10.1104/pp.112.205591
Cardoso-Vilhena, J., Balaguer, L., Eamus, D., Ollerenshaw, J., and Barnes, J. (2004). Mechanisms underlying the amelioration of O3-induced damage by elevated atmospheric concentrations of CO2. J. Exp. Botany 55, 771–781. doi: 10.1093/jxb/erh080
Clifton, O. E., Fiore, A. M., Massman, W. J., Baublitz, C. B., Coyle, M., Emberson, L., et al. (2020). Dry deposition of ozone over land: Processes, measurement, and modeling. Rev. Geophys. 58:e2019RG000670. doi: 10.1029/2019RG000670
Dermody, O., Long, S. P., and DeLucia, E. H. (2006). How does elevated CO2 or ozone affect the leaf-area index of soybean when applied independently. N Phytol. 169, 145–155. doi: 10.1111/j.1469-8137.2005.01565.x
Deryng, D., Conway, D., Ramankutty, N., Price, J., and Warren, R. (2014). Global crop yield response to extreme heat stress under multiple climate change futures. Environ. Res. Lett. 9:034011. doi: 10.1088/1748-9326/9/3/03401
Emberson, L. D., Ashmore, M. R., Cambridge, H. M., Simpson, D., and Tuovinen, J. P. (2000). Modelling stomatal ozone flux across Europe. Environ. Pollut. 109, 403–413. doi: 10.1016/S0269-7491(00)00043-9
Feng, Z. Z., De Marco, A., Anav, A., Gualtieri, M., Sicard, P., Tian, H. Q., et al. (2019). Economic losses due to ozone impacts on human health, forest productivity and crop yield across China. Environ. Int. 131:104966. doi: 10.1016/j.envint.2019.104966
Feng, Z. Z., Hu, E. Z., Wang, X. K., Jiang, L. J., and Liu, X. J. (2015). Ground-level O-3 pollution and its impacts on food crops in China: a review. Environ. Pollut. 199, 42–48. doi: 10.1016/j.envpol.2015.01.016
Feng, Z. Z., Kobayashi, K., and Ainsworth, E. A. (2008). Impact of elevated ozone concentration on growth, physiology, and yield of wheat (Triticum aestivum L.): a meta-analysis. Global Change Biol. 14, 2696–2708. doi: 10.1111/j.1365-2486.2008.01673.x
Fiscus, E. L., Reid, C. D., Miller, J. E., and Heagle, A. S. (1997). Elevated CO2 reduces O3 flux and O3-induced yield losses in soybean: possible implications for elevated CO2 studies. J. Exp. Botany 48, 307–313. doi: 10.1093/jxb/48.2.307
Franks, P. J., Adams, M. A., Amthor, J. S., Barbour, M. M., Berry, J. A., Ellsworth, D. S., et al. (2013). Sensitivity of plants to changing atmospheric CO2 concentration: from the geological past to the next century. New Phytol. 197, 1077–1094. doi: 10.1111/nph.12104
Fuhrer, J., Skarby, L., and Ashmore, M. R. (1997). Critical levels for ozone effects on vegetation in Europe. Environ. Pollut. 97, 91–106. doi: 10.1016/S0269-7491(97)00067-5
Guarin, J. R., Kassie, B., Mashaheet, A. M., Burkey, K., and Asseng, S. (2019). Modelling the effects of tropospheric ozone on wheat growth and yield. Europ. J. Agronomy. 105, 13–23. doi: 10.1016/j.eja.2019.02.004
Jacob, D. J., and Winner, D. A. (2009). Effect of climate change on air quality. Atmos. Environ. 43, 51–63. doi: 10.1016/j.atmosenv.2008.09.051
Jarvis, P. G. (1976). The interpretation of the variations in leaf water potential and stomatal conductance found in canopies in the field. Phil. Trans. R. Soc. Land. B. 273, 593–610. doi: 10.1098/rstb.1976.0035
Leakey, A. D. B., Bernacchi, C. J., Dohleman, F. G., Ort, D. R., and Long, S. P. (2004). Will photosynthesis of maize (Zea mays) in the US Corn Belt increase in future [CO2] rich atmospheres? An analysis of diurnal courses of CO2 uptake under free-air concentration enrichment (FACE). Glob. Chang. Biol. 10, 951–962. doi: 10.1111/j.1529-8817.2003.00767.x
Lee, E. H., and Hogsett, W. E. (1996). Methodology for Calculating Inputs for Ozone Secondary Standard Benefits Analysis: Part II. Report Prepared for Office of Air Quality Planning and Standards. Research Triangle Park, NC: U.S. Environmental Protection Agency.
Lefohn, A. S., Laurence, J. A., and Kohut, R. J. (1988). A comparison of indices that describe the relationship between exposure to ozone and reduction in the yield of agricultural crops. Atmosph. Environ. 22, 1229–1240. doi: 10.1016/0004-6981(88)90353-8
Lesser, V. M., Rawlings, J. O., Spruill, S. E., and Somerville, M. C. (1990). Ozone effects on agricultural crops: statistical methodologies and estimated dose-response relationships. Crop Sci. 30, 148–155. doi: 10.2135/cropsci1990.0011183X003000010033x
Lombardozzi, D., Sparks, J. P., and Bonan, G. (2013). Integrating O3 influences on terrestrial processes: photosynthetic and stomatal response data available for regional and global modeling. Biogeosciences. 10, 6815–6831. doi: 10.5194/bg-10-6815-2013
Lombardozzi, D. L., Bonan, G. B., Levis, S., and Lawrence, D. M. (2018). Changes in wood biomass and crop yields in response to projected CO2, O3, nitrogen deposition, and climate. J. Geophys. Res. Biogeosci. 123, 3262–82. doi: 10.1029/2018JG004680
Millls, G., Hayes, F., Simpson, D., Emberson, L., Norris, D., Harmens, H., and Buker, P. (2011). Evidence of widespread effects of ozone on crops and (semi-)natural vegetation in Europe (1990–2006) in relation to AOT40- and flux-based risk maps. Glob. Change Biol. 17, 592–613. doi: 10.1111/j.1365-2486.2010.02217.x
Mills, G., Buse, A., Gimeno, B., Bermejo, V., Holland, M., Emberson, L., et al. (2007). A synthesis of AOT40-based response functions and critical levels of ozone for agricultural and horticultural crops. Atmosph. Environ. 40, 2630–2643. doi: 10.1016/j.atmosenv.2006.11.016
Mills, G., Sharps, K., Simpson, D., Pleijel, H., Broberg, M., Uddling, J., et al. (2018a). Ozone pollution will compromise efforts to increase global wheat production. Glob. Chang. Biol. 24, 3560–3574. doi: 10.1111/gcb.14157
Mills, G., Sharps, K., Simpson, D., Pleijel, H., Frei, M., Burkey, K., et al. (2018b). Closing the global ozone yield gap: quantification and cobenefits for multistress tolerance. Glob. Chang. Biol. 24, 4869–4893. doi: 10.1111/gcb.14381
Morgen, P. B., Ainsworth, E. A., and Long, S. P. (2003). How does elevated ozone impact soybean? A meta-analysis of photosynthesis, growth and yield. Plant Cell Environ. 26, 1317–1328. doi: 10.1046/j.0016-8025.2003.01056.x
Morgen, P. B., Bollero, G. A., Nelson, R. L., Dohleman, F. G., and Long, S. P. (2005). Smaller than predicted increase in aboveground net primary production and yield of field-grown soybean under fully open-air [CO2] elevation. Glob. Chang. Biol. 11, 1856–1865. doi: 10.1111/j.1365-2486.2005.001017.x
Morgen, P. B., Mies, T. A., Bollero, G. A., Nelson, R. L., and Long, S. P. (2006). Season-long elevation of ozone concentration to projected 2050 levels under fully open-air conditions substantially decreases the growth and production of soybean. New Phytol. 170, 333–343. doi: 10.1111/j.1469-8137.2006.01679.x
Musselman, R. C., Lefohn, A. S., Massman, W. J., and Heath, R. L. (2006). A critical review and analysis of the use of exposure- and flux-based ozone indices for predicting vegetation effects. Atmosph. Environ. 40, 1869–1888. doi: 10.1016/j.atmosenv.2005.10.064
Nouchi, I. (2002). “Responses of whole plants to air pollutants,” in Air Pollution and Plant Biotechnology–Prospects for Phytomonitoring and Phytoremediation, eds K. Omasa, H. Saji, S. Youssefian and N. Kondo (Tokyo: Springer), 3–39.
Oleson, K. W., Lawrence, D. M., Bonan, G. B., Drewniak, B., Huang, M., Koven, C. D., et al. (2013). Technical Description of version 4.5 of the Community Land Model (CLM). Boulder, CO: National Center for Atmospheric Research.
Onandia, G., Olsson, A., Barth, S., King, J. S., and Uddling, J. (2011). Exposure to moderate concentration of tropospheric ozone impairs tree stomatal response to carbon dioxide. Environ. Pollut. 159, 2350–2354. doi: 10.1016/j.envpol.2011.06.001
Osborne, T., Gornall, J., Hooker, J., Williams, K., Wiltshire, A., Betts, R., et al. (2015). JULES-crop: a parameterisation of crops in the Joint UK Land Environment Simulator. Geoscient. Model Dev. 8, 1139–1155. doi: 10.5194/gmd-8-1139-2015
Paoletti, E., and Grulke, N. E. (2010). Ozone exposure and stomatal sluggishness in different plant physiognomic classes. Environ. Pollut. 158, 2664–2671. doi: 10.1016/j.envpol.2010.04.024
Peng, J., Shang, B., Xu, Y., Feng, Z., Pleijel, H., and Calatayud, V. (2019). Ozone exposure- and flux-yield response relationship for maize. Environ. Pollut. 252, 1–7. doi: 10.1016/j.envpol.2019.05.088
Pleijel, H., Danielsson, H., Emberson, L., Ashmore, M. R., and Mills, G. (2007). Ozone risk assessment for agricultural crops in Europe: further development of stomatal flux and flux. Response relationships for European wheat and potato. Atmos. Environ. 41, 3022–3040. doi: 10.1016/j.atmosenv.2006.12.002
Rosenzweig, C., Elliott, J., Deryng, D., Ruane, A. C., Muller, C., Arneth, A., et al. (2014). Assessing agricultural risks of climate change in the 21st century in a global gridded crop model intercomparison. Proc. Natl. Acad. Sci. U.S.A. 111, 3268–3273. doi: 10.1073/pnas.1222463110
Rudorff, B. F. T., Mulchi, C. L., Lee, E., Rowland, R., and Pausch, R. (1996). Photosynthetic characteristics in wheat exposed to elevated O3 and CO2. Crop Sci. 36, 1247–1251. doi: 10.2135/cropsci1996.0011183X003600050029x
Sacks, W. J., Deryng, D., Foley, J. A., and Ramankutty, N. (2010). Crop planting dates: an analysis of global patterns. Global Ecol. Biogeogr. 19, 607–620. doi: 10.1111/j.1466-8238.2010.00551.x
Sitch, S., Cox, P. M., Collins, W. J., and Huntingford, C. (2007). Indirect radiative forcing of climate change through ozone effects on the land-carbon sink. Nature. 448, 791–794. doi: 10.1038/nature06059
Tai, A. P. K., and Martin, M. V. (2017). Impacts of ozone air pollution and temperature extremes on crop yields: spatial variability, adaptation and implications for future food security. Atmos. Environ. 169, 11–21. doi: 10.1016/j.atmosenv.2017.09.002
Tai, A. P. K., Martin, M. V., and Heald, C. L. (2014). Threat to future global food security from climate change and ozone air pollution. Nat. Clim. Change. 4, 817–821. doi: 10.1038/nclimate2317
Tao, F., Feng, Z., Tang, H., Chen, Y., and Kobayashi, K. (2017). Effects of climate change, CO2 and O3 on wheat productivity in Eastern China, singly and in combination. Atmos. Environ. 157, 182–193. doi: 10.1016/j.atmosenv.2017.01.032
Tingey, D. T., and Hogsett, W. E. (1985). Water stress reduces ozone injury via a stomatal mechanism. Plant Physiol. 77, 944–947. doi: 10.1104/pp.77.4.944
van Dingenen, R., Dentener, F. J., Raes, F., Krol, M. C., Emberson, L., and Cofala, J. (2009). The global impact of ozone on agricultural crop yields under current and future air quality legislation. Atmos. Environ. 43, 604–618. doi: 10.1016/j.atmosenv.2008.10.033
Wang, X., and Mauzerall, D. L. (2004). Characterizing distributions of surface ozone and its impact on grain production in China, Japan and South Korea: 1990 and 2020. Atmosph. Environ. 38, 4383–4402. doi: 10.1016/j.atmosenv.2004.03.067
Young, P. J., Archibald, A. T., Bowman, K. W., Lamarque, J. F., Naik, V., Stevenson, D. S., et al. (2013). Pre-industrial to end 21st century of projections of tropospheric ozone from the Atmospheric Chemistry and Climate Model Intercomparsion Project (ACCMIP). Atmosph. Chem. Phys. 13, 2063–2090. doi: 10.5194/acp-13-2063-2013
Keywords: ozone pollution, ozone exposure metrics, biophysical crop model, crop yields, global food security
Citation: Tai APK, Sadiq M, Pang JYS, Yung DHY and Feng Z (2021) Impacts of Surface Ozone Pollution on Global Crop Yields: Comparing Different Ozone Exposure Metrics and Incorporating Co-effects of CO2. Front. Sustain. Food Syst. 5:534616. doi: 10.3389/fsufs.2021.534616
Received: 13 February 2020; Accepted: 15 February 2021;
Published: 29 March 2021.
Edited by:
Steve Arnold, University of Leeds, United KingdomReviewed by:
David Kanter, New York University, United StatesCopyright © 2021 Tai, Sadiq, Pang, Yung and Feng. This is an open-access article distributed under the terms of the Creative Commons Attribution License (CC BY). The use, distribution or reproduction in other forums is permitted, provided the original author(s) and the copyright owner(s) are credited and that the original publication in this journal is cited, in accordance with accepted academic practice. No use, distribution or reproduction is permitted which does not comply with these terms.
*Correspondence: Amos P. K. Tai, YW1vc3RhaUBjdWhrLmVkdS5oaw==
Disclaimer: All claims expressed in this article are solely those of the authors and do not necessarily represent those of their affiliated organizations, or those of the publisher, the editors and the reviewers. Any product that may be evaluated in this article or claim that may be made by its manufacturer is not guaranteed or endorsed by the publisher.
Research integrity at Frontiers
Learn more about the work of our research integrity team to safeguard the quality of each article we publish.