- 1US Department of Agriculture – Agricultural Research Service, Southeast Watershed Research Laboratory, Tifton, GA, United States
- 2US Department of Agriculture – Agricultural Research Service, Pasture Systems and Watershed Management Research Unit, University Park, PA, United States
- 3US Department of Agriculture – Agricultural Research Service, Cropping Systems and Water Quality Research Unit, Columbia, MO, United States
- 4US Department of Agriculture – Agricultural Research Service, Southwest Watershed Research Center, Tucson, AZ, United States
- 5School of Natural Resources and Environment, University of Arizona, Tucson, AZ, United States
- 6Archbold Biological Station, Venus, FL, United States
- 7US Department of Agriculture – Agricultural Research Service, Water Quality and Ecology Research Unit, Oxford, MS, United States
Introduction
The US Department of Agriculture (USDA)-Agricultural Research Service (ARS) has a long history of monitoring sustainable agriculture across the United States (US). Many of these ARS sites are also part of the USDA Long-Term Agroecosystem Research (LTAR) Network. This partnership of 18 sites in the conterminous US (see Supplementary Figure 1; https://ltar.ars.usda.gov) was established to research the sustainable intensification of agroecosystems while minimizing or reversing adverse environmental impacts and improving rural prosperity (Kleinman et al., 2018). The LTAR Network was built on a shared research strategy to advance four areas of foundational science: agroecosystem productivity, climate variability and change, conservation and environmental quality, and promoting rural opportunity and prosperity (Steiner et al., 2015). Today, LTAR locations comprise diverse agricultural systems and serve as a research platform for regional- to national-scale field assessments and modeling studies of ecosystem goods and services (Goodrich et al., 2016).
For the LTAR Network, sustainable intensification of agriculture describes the increase in production of agricultural systems while lowering environmental impact—a goal aligned with the USDA Strategic Plan and aligning with “Grand Challenges” for agroecology (Nair, 2014; Kleinman et al., 2018; Spiegal et al., 2018; U.S. Department of Agriculture, 2018). Addressing this and other challenging research questions of the twenty-first century requires synthesis across broad geographic areas. The Network is focused on a shared approach of testing sustainable intensification strategies at plot and field experimental scales but also seeks to quantify the uncertainty associated with extrapolating outcomes to larger geographic regions.
A region is “a spatial stereotype for a portion of Earth that has some special signature or characteristic that sets it apart from other regions” (Rowntree et al., 2015). Regional boundaries can describe areas of similarity in collective patterns of biophysical and socioeconomic factors, such as land use (Omernik and Griffith, 2014). Functionally, boundaries can facilitate the comparison and extrapolation of observations and experimental findings. The process of defining regions includes the logic and methods for defining boundaries (de Blij et al., 2014). A valid regional framework is vital for extrapolating observations and model results and representing broad regional- and continental-scale issues and trends. Such a framework can help facilitate long-term agricultural management strategies (Lin et al., 2013) and network resource management. Examples of existing regional frameworks for the US include the Major Land Resource Areas (MLRAs; USDA-NRCS, 2006), the US Ecoregions (Omernik and Griffith, 2014), the Watershed Boundary System (US Geological Survey, 2015), and the Economic Research Service (ERS) Farm Resource Regions (USDA-ERS, 2000; see Supplementary Figure 2). Each of these spatial frameworks were generated in response to specific goals, such as ecological characterization, with methods described in their published resources.
Other continental-scale scientific networks have sought regional frameworks to represent and extrapolate results [e.g., National Ecological Observatory Network (NEON); Keller et al., 2008]. LTAR is a network that requires a multiscale research approach designed to improve our ability to model, test, and forecast outcomes of alternative intensification strategies over short, middle, and long time frames from regional to continental scales. While existing regional frameworks are beneficial for use in interpreting and analyzing data in relatively homogeneous regions, they can be limiting in their precision and do not account for unique variations (Carter and Murwira, 1995). Because agencies tend to operate within their own directive boundaries, this too can create limitations for data sharing and cross-site analyses (McMahon et al., 2001) such as those necessary to address the goals set forth by the LTAR Network.
Past regional frameworks have been almost entirely focused on physical variables, without considering critical drivers and outcomes of agricultural productivity and are of limited use for LTAR. Initial LTAR site selection was based upon criteria that met the call for long-term agricultural research sites with legacies of datasets describing agroecological systems. Since the LTAR Network lacked a coherent spatial framework that could be used for cross-site, cross-scale, network level modeling of production scenarios, the Network sought to develop regional boundaries that integrate domains representing the mission of the LTAR: to provide research enabling sustainable production, environmental quality, and rural prosperity in the US.
To develop a strategy for synthesizing research across the network, LTAR initiated a process in 2017 to quantitatively describe the geographic extent of agricultural landscapes represented by each of the LTAR sites. Goals also included providing a standardized spatial footprint for LTAR cross-site investigations, estimating the confidence with which results from research plots and fields could reasonably be extrapolated to “represented regions,” informing decisions about where additional research sites should be prioritized and facilitating public outreach of the network. This resulted in a new dataset describing regional boundaries for the LTAR Network, “Long-Term Agroecosystem Research Network regions, 2018 version,” archived in the USDA National Agricultural Library's Ag Data Commons repository.
At the inception of LTAR, following a request from USDA in 2011 (and a subsequent one in 2014), research watersheds, farms, and ranches contributed proposals for becoming core experimental sites within the LTAR Network. Site scientists and leaders, who collectively represent a wealth of knowledge about the agronomy, soils, hydrology, and climate in these locations, described their sites, mapping the agricultural regions where long-term research data had been collected (Walbridge and Shafer, 2011). Research at the existing ARS sites crossed geographic and political boundaries (i.e., watersheds and county lines). Many, but not all, site leaders utilized published regional frameworks to describe their boundaries. These included spatial layers that corresponded to environmental boundaries, agricultural commodity zones, and hydrologic boundaries. Thus, LTAR regions were first defined as agricultural landscapes that corresponded to a specific site, were self-determined, and are now referred to as “legacy” boundaries.
For the first years of the LTAR Network, this representation of boundaries was useful for internal identification and for the presentation of site-specific research plans. However, as the Network developed, the fact that this process was not uniform or repeatable came to be recognized as a shortcoming. Their unique determinations with no standard, quantifiable method precluded their use for network-level scientific analyses. As the LTAR Network evolved and increasingly broad-scale questions were being investigated (Spiegal et al., 2018; Baffaut et al., 2020), this lack of a spatial framework was increasingly problematic, and a more cohesive approach was desired.
The LTAR Regionalization Project held its first workshop in March 2018, in Tifton, GA, to address this need. A goal of the workshop was to improve the set of legacy boundaries used to describe the Network. During the workshop, concepts of sustainable intensification related to domains of agricultural production, environmental impacts, and rural prosperity were adopted as an approach for organizing indicators. At the outcome, workshop participants (a) defined new sets of regional boundaries for LTAR sites that were used to (b) map and compare indicators from each domain across the Network. As the boundaries were self-described and expert driven, and did not solve the problem of standardization, the rationale used to define them was documented.
Regional Boundary Delineation
During the 2018 workshop, a task force of geospatial scientists was organized to facilitate the development of a consistent sets of regions (Figure 1) for use by the Network. Since pre-existing regional datasets were used in some combination to derive the legacy regions, a database was created including relevant published regional boundary systems for reference (e.g., MLRAs). Experts from each LTAR site, facilitated by a task force member, identified suitable boundaries for their site corresponding to the three domains of sustainability. The process followed two principles: (a) the regions should enable extrapolation of field and farm results to broader extents, and (b) they should facilitate the cross-network comparison of indicators of production, environmental impact, and rural prosperity. As with the legacy boundaries, the basic idea of the LTAR agro-ecoregions included the contextual landscape, recognizing that non-production components of these areas are part of the land mosaic (Forman, 1995), providing benefits to rural communities and supporting cultural ecosystem services (Millennium Ecosystem Assessment, 2005). Although this new set of boundaries was also driven by expert opinion, the process was facilitated and documented by the task force.
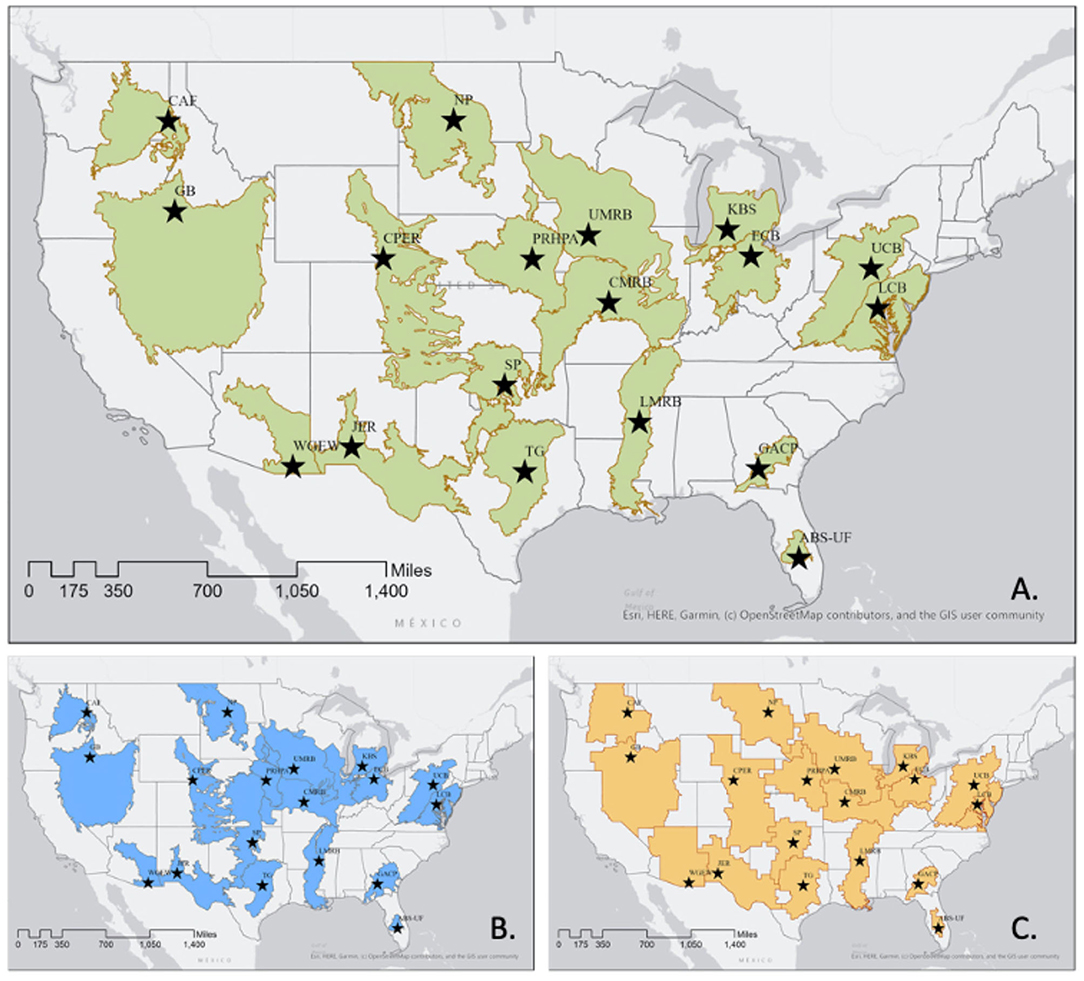
Figure 1. Maps of current Long-Term Agroecosystem Research (LTAR) agroecoregions. (A) Production Boundaries; (B) Environment Boundaries; and (C) Rural Prosperity Boundaries. LTAR sites from west to east: CAF, R.J. Cook Agronomy Farm in Pullman, WA; GB, Great Basin in Boise, ID; WGEW, Walnut Gulch Experimental Watershed in Tucson, AZ; JER, Jornada Experimental Range in Las Cruces, NM; CPER, Central Plains Experimental Range in Nunn, CO; NP, Northern Plains in Mandan, ND; SP, Southern Plains in El Reno, OK; TG, Texas Gulf in Temple, TX; PRHPA, Platte River/High Plains Aquifer in Lincoln, NE; UMRB, Upper Mississippi River Basin in Ames, IA; CMRB, Central Mississippi River Basin in Columbia, MO; LMRB, Lower Mississippi River Basin in Oxford, MS; KBS, Kellogg Biological Station in Hickory Corners, MI; GACP, Gulf Atlantic Coastal Plain in Tifton, GA; ECB, Eastern Corn Belt in Columbus, OH; ABS-UF, Archbold-University of Florida in Venus, FL; UCB, Upper Chesapeake Bay in University Park, PA; LCB, Lower Chesapeake Bay in Beltsville, MD.
To create a set of boundaries describing agricultural production, or “production regions” (Figure 1A), some sites used MLRA regions, while others used watershed basins to delineate polygons or groups of polygons corresponding to the dominant agricultural commodities at each LTAR location. These boundaries could be used to map and compare indicators of agricultural production. Site experts also considered the “area of inference” or a reasonable spatial extent to which LTAR research results could be extrapolated. Facilitators recorded a brief rationale for selecting the specific production regions, attached to the dataset (Table 1). In a few cases, notably the Cook Agronomy Farm (CAF), and the Gulf Atlantic Coastal Plain (GACP), careful thought and planning had already gone into the description of the boundaries, so the steps involved documenting a process that had already occurred.
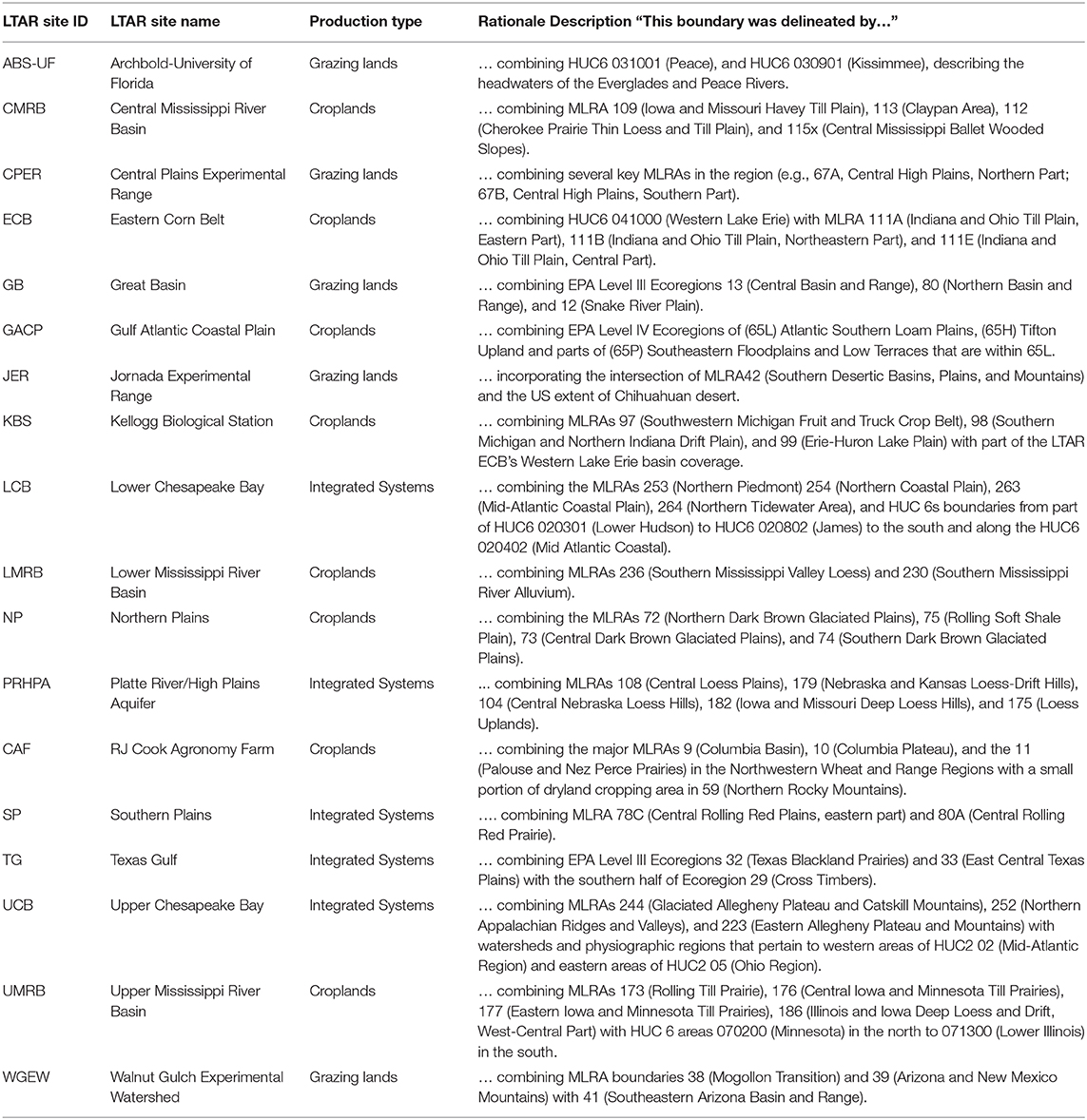
Table 1. Rationale for “production boundary” delineation of the 18 Long-Term Agroecosystem Research (LTAR) sites.
A similar process was used to create “environment regions” (Figure 1B), which could be used to map and compare indicators of agro-environmental impacts. For most LTAR sites, this process made use of the Environmental Protection Agency (EPA) level III or IV Ecoregions, the NEON and United States Geological Survey (USGS) hydrologic unit code (HUC) boundaries to derive the environmental area of inference. In 13 of the 18 cases, the environment and production regions were very similar, if not identical (e.g., Great Basin, GB, or Upper Chesapeake Bay, UCB), and in those cases, the rationale underlying the environment regions was the same.
Finally, boundaries associated with indicators of “rural prosperity” were developed that could make use of the abundant data available through the Census of Agriculture or the National Agricultural Statistical Service (NASS). Because most of these data are available only at the county level, county boundaries were used to derive these regions even though other indicators of rural prosperity may not conform to this spatial framework. In all cases, “rural prosperity boundaries” consisted of the intersection of production regions with county boundaries (Figure 1C), so the rationale describing production regions also underlaid the rural prosperity regions.
As an example, the Central Mississippi River Basin (CMRB) LTAR site, in Columbus, MO, used existing regional frameworks and expert knowledge to derive their site-specific regional boundary (Supplementary Figure 3). The core experiment of the CMRB site lies in MLRA113 (Claypan Area), which covers the northeast part of Missouri and southern part of Illinois. The area is characterized by poor natural soil drainage due to a clay layer that impedes infiltration, thus affecting production and environmental impacts. On the western edge of Missouri and Eastern edge of Kansas, MLRA112 (Cherokee Prairies) has soils with hydrologically restrictive layers that act as system drivers similar to soils in MLRA113. In order to link these two regions spatially and contiguously, they included MLRA114B (Southern Illinois and Indiana Thin Loess and Till Plain, Western Part) and MLRA115x (Central Mississippi Valley Wooded Slopes) since the river bottoms are so similar. Finally, CMRB had cooperators in MLRA109 (Iowa and Missouri Heavy Till Plain), which is characterized by soils with strong contrast in terms of soils and topography to those in MLRA113, so MLRA109 was included in the CMRB production region. For the CMRB environment region, the NEON Domain 6 (Prairie Peninsula) was selected. For the rural prosperity boundary, CMRB used the county-based ERS Farm Resources Region 1 (Heartland), following suit with the overall LTAR decision to use county boundaries for rural prosperity indicators.
Characterization of LTAR Network Regions
In addition to describing three sets of boundaries, the task force identified three indicators that could characterize the LTAR Network across the domains of sustainable intensification. A range of potential national-scale indicators were considered and included recent data on land use, crop and forage yields, and variables that quantify animal products (for production); variables that describe soil health, water quantity and quality, air quality, and biodiversity (for environmental impact); and farm income, costs of production, labor, and profits (for rural prosperity). One indicator from each domain was selected, and the boundaries were used to summarize them for comparison. While it was recognized that these indicators alone were not fully representative of each domain, they were selected as examples to demonstrate a basic characterization of the Network using publicly available datasets.
Agricultural land use is an indication of the potential for production or the potential for provisioning services from working lands. There is no true national-scale “land use” map of the US; however, the USDA-NASS Cropland Data Layer (CDL; Boryan et al., 2011) provides annual land cover data from which many land uses can be inferred. The 2017 CDL was reclassified into land cover classes that estimated land use and used for this purpose (U.S. Department of Agriculture-National Agricultural Statistics Service, 2018). To characterize environmental impact, a model-estimated indicator of agricultural nitrogen runoff (N-runoff) was used, which is provided in the Environmental Protection Agency's EnviroAtlas (www.epa.gov/enviroatlas; US Environmental Protection Agency, 2016). To characterize rural prosperity, data on county-level farm income derived from NASS Census of Agriculture data (NASS, 2012) were used.
Production: Agricultural Land Use
Land use was inferred from the 2017 Cropland Data Layer by combining land cover classes (Supplementary Table 1) into six classes (cropping, grazing/hay, wetlands, non-Ag/Forest, developed, and open water). The data were summarized as the land use proportions at each of the LTAR locations and the conterminous US (Supplementary Figure 4). The analysis showed that LTAR regions are distributed across agricultural land uses with nine sites predominantly including grazing land or hay (ABS-UF, CPER, NP, CAF, SP, GB, JER, WGEW, and TG), five sites with large proportions of cropland (LMRB, PRHPA, ECB, UMRB, and KBS), and four sites with a nearly even mix of grazing land/hay or cropland, as well as other land uses, such as forest or wetlands (CMRB, GACP, UCB, and LCB). This analysis highlights the diversity of land uses across the regions and the need to account for multiple dominant land use types within a region. It was noted that land use activities in the wetlands and non-Ag/forest classes also potentially included grazing, hay production (e.g., salt marsh hay), aquaculture, and non-timber forest product harvesting. However, this map does not include the potential for multiple uses for the same piece of land (e.g., grazing on crop residue); this temporal complexity should be considered in future analyses.
Environmental Impact: Model-Estimated Agricultural Nitrogen Runoff (N-Runoff)
Given the diversity of land uses, there is a great deal of variation in the estimated N-runoff between the various LTAR regions. A model-estimated indicator of agricultural nitrogen runoff (N-runoff) was used from the Environmental Protection Agency's EnviroAtlas (www.epa.gov/enviroatlas; US Environmental Protection Agency, 2016). The data were summarized as box plots indicating the median and spread of data values for each of the LTAR sites (Supplementary Figure 5). Results showed that, in general, western LTAR regions that are predominantly grazing lands have lower N-runoff, while LTAR regions in the Great Plains, Upper Midwest, Southeast, and Northeast all have moderate to high estimated N-runoff. The greatest hotspots in N-runoff occur in the Mississippi River Basin and Florida. Such results help to group LTAR sites into Network projects studying nutrient management, water quality, and related topics. One can imagine many iterations of this map for other environmental indicators that would aid the Network in cross-site evaluation of topics such as soil erosion, biodiversity conservation, and groundwater stress.
Rural Prosperity: Farm Income
Farm income does not follow a simple geographic pattern, but instead, there are both high and low values in most regions. The data on farm income were summarized in series of box plots displaying the median and variability (Supplementary Figure 6). The Midwest regions (i.e., NP, PRHPA, and UMRB) with concentrated row cropland have the highest median farm incomes in the Network, while several of the grazing land sites in the Southern US (i.e., TG and JER) have the lowest median farm income. The Southeast and Great Plains regions (especially the LMRB) have large inequalities in farm income, with some counties falling into the highest category (over $200,000; dark green) and others falling into the lowest category (0–$24,000). The spread in income is greatest in the LMRB and CPER, while the variability is lowest in the TG and UCB regions, which both have fairly low farm income overall. This straightforward analysis can lead to questions about drivers of farm income and subsequently agriculturally related income inequality. For example: why does the Eastern Corn Belt (ECB) region have lower median farm income than other Midwest regions; is there an overall pattern with drivers of farm income or are they region specific? These questions are stimulated through regional analyses and may spur further research into strategies for improving economic conditions for rural communities.
Conclusions
The maps presented here provide information that can be useful to LTAR researchers and external stakeholders as they begin the complex task of synthesis, integration, and uncertainty analysis using LTAR Network data across the domains of production, environmental impacts, and rural prosperity. While the set of boundaries describing production regions are currently used for the Network, we anticipate that regions generated for the three domains will be regularly updated to incorporate new data and changing conditions as the LTAR Regionalization Project advances. These will become increasingly relevant to the scientific community, as LTAR datasets become publicly available in USDA data repositories.
Research at LTAR locations provides highly detailed information regarding responses of production systems to experimental manipulations. However, research to test the geographic extent of spatial and temporal model limits is needed for LTAR to inform agricultural science at the continental scale. Such a regional framework will allow researchers to spatially extrapolate and detect when key drivers, such as climatic variables or demographic shifts, affect processes like plant water and nutrient use efficiencies or market flows. Delineated regions will also help agricultural research directors to determine how well the LTAR Network represents US agricultural concerns and learn where gaps may exist. A strategic value provided by the LTAR Regionalization Project is to highlight the need to prioritize a set of indicators across the Network that contribute to regional impact assessments, cost–benefit analyses, risk management/mitigation opportunities, marketing strategies, and policy development.
Data Availability Statement
The data described in this paper are available in the Ag Data Commons repository of the USDA National Agricultural Library, doi: 10.15482/USDA.ADC/1520632. Original contributions presented in this paper are included in the Supplementary Materials. Further inquiries can be directed to the corresponding author.
Author Contributions
AB, AC, SG, CB, CH, VS, and LY contributed to the conceptualization of this report. AC, DA, CH, VS, and LY created the datasets. AC, VS, and CH analyzed the data. AB, AC, SG, CB, GP-C, DA, and LY wrote the manuscript. AC, GP-C, and LY led the overall project. SG led project methods development. DA served as the project data manager. All authors contributed to the article and approved the submitted version.
Funding
This research was a contribution from the Long-Term Agroecosystem Research (LTAR) Network. LTAR is supported by the United States Department of Agriculture.
Conflict of Interest
The authors declare that the research was conducted in the absence of any commercial or financial relationships that could be construed as a potential conflict of interest.
Acknowledgments
The authors would like to acknowledge the contributions of Brandon Bestelmeyer, Dawn Browning, Mike Holly, David Huggins, Pete Kleinman, Sheri Spiegal, Tim Strickland, and participants from the 2017 Tri-Societies Meeting in Tampa, FL, where the kernel of the Regionalization Project was first discussed. We are also grateful to participants from the 2018 LTAR Tifton Workshop and members of the resulting geospatial analysis task force for aiding in this effort.
Supplementary Material
The Supplementary Material for this article can be found online at: https://www.frontiersin.org/articles/10.3389/fsufs.2020.612785/full#supplementary-material
Presentation 1 includes the supplementary table and all supplementary figures along with captions, including: Table 1; Figures 1–5.
References
Baffaut, C., Baker, J. M., Biederman, J. A., Bosch, D. D., Brooks, E. S., Buda, A. R., et al. (2020). Comparative analysis of water budgets across the US Long-Term Agroecosystem Research network. J. Hydrol. 588:125021. doi: 10.1016/j.jhydrol.2020.125021
Boryan, C., Yang, Z., Mueller, R., and Craig, M. (2011). Monitoring US agriculture: the US Department of Agriculture, National Agricultural Statistics Service, Cropland Data Layer Program. Geocarto Int. 26. 341–358. doi: 10.1080/10106049.2011.562309
Carter, S. E., and Murwira, H. K. (1995). Spatial variability in soil fertility management and crop response in Mutoko Communal Area, Zimbabwe. Ambio 24, 77–84.
de Blij, H. J., Muller, P. O., and Nijman, J. (2014). Geography: Realms, Regions, and Concepts. 16th Edn. New York, NY: Wiley.
Forman, R. T. T. (1995). Land Mosaics: The Ecology of Landscapes and Regions. Cambridge MA: Cambridge University Press.
Goodrich, D. C., Walthall, C. L., Campbell, J. D., Derner, J. D., Huggins, D. R., Kleinman, P. J., et al. (2016). The USDA Long-Term Agro-ecosystems Research (LTAR) Network. San Francisco, CA: AGUFM 2016:H34C-03.
Keller, M., Schimel, D. S., Hargrove, W. W., and Hoffman, F. M. (2008). A continental strategy for the National Ecological Observatory Network. Ecol. Soc. Am. 6, 282–284. doi: 10.1890/1540-9295(2008)6[282:ACSFTN]2.0.CO;2
Kleinman, P. J. A., Spiegal, S., Rigby, J. R., Goslee, S. C., Baker, J. M., Bestelmeyer, B. T., et al. (2018). Advancing the sustainability of US agriculture through long-term research. J. Environ. Q. 47, 1412–1425. doi: 10.2134/jeq2018.05.0171
Lin, Y., Liu, A., Ma, E., and Zhang, F. (2013). Impacts of future climate changes on shifting patterns of the agro-ecological zones in China. Adv. Meteorol. 2013:163248. doi: 10.1155/2013/163248
McMahon, G., Gregonis, S. M., Waltman, S. W., Omernik, J. M., Thorson, T. D., Freeouf, J. A., et al. (2001). Developing a spatial framework of common ecological regions for the conterminous United States. Environ. Manage. 28, 293–316. doi: 10.1007/s0026702429
Millennium Ecosystem Assessment (2005). Ecosystems and Human Well-being: A Framework for Assessment. Washington, DC: World Resources Institute.
Nair, R. (2014). Grand challenges in agroecology and land use systems. Front. Environ. Sci. 2:1. doi: 10.3389/fenvs.2014.00001
Omernik, J. M., and Griffith, G. E. (2014). Ecoregions of the conterminous United States: evolution of a hierarchical spatial framework. Environ. Manage. 54, 1249–1266. doi: 10.1007/s00267-014-0364-1
Rowntree, L., Lewis, M., Price, M., and Wyckoff, W. (2015). Diversity Amid Globalization. Upper Saddle River, NJ: Pearson Education UK.
Spiegal, S., Bestelmeyer, B. T., Archer, D. W., Augustine, D. J., Boughton, E. H., Boughton, R. K., et al. (2018). Evaluating strategies for sustainable intensification of US agriculture through the Long-Term Agroecosystem Research network. Environ. Res. Lett. 13:034031. doi: 10.1088/1748-9326/aaa779
Steiner, J. L., Strickland, T., Kleinman, P. J. A., Havstad, K., Moorman, T. B., Moran, M. S., et al. (2015). “The long term agroecosystem research network – shared research strategy,” in 5th Interagency Conference on Research in the Watersheds (North Charleston, SC: U.S. Department of Agriculture Forest Service, Southern Research Station).
U.S. Department of Agriculture (2018). USDA Strategic Plan, FY 2018 – 2022. in U.S. Department of Agriculture. Washington, DC: U.S. Department of Agriculture.
U.S. Department of Agriculture-National Agricultural Statistics Service (2018). 2017 Cropland Data Layer. Washington, DC: USDA-NASS.
US Environmental Protection Agency (2016). EnviroAtlas - 2002 Edge-of-Field Simulated Nitrogen, Phosphorous and Water Quantity Loss by 12-digit HUC for the Conterminous United States. Research Triangle Park, NC: US EPA.
US Geological Survey USDA-Natural Resources Conservation Service, and US Environmental Protection Agency. (2015). National Watershed Boundary Dataset (WBD).
USDA-NRCS (2006). Land Resource Regions and Major Land Resource Areas of the United States, the Caribbean, and the Pacific Basin. U.S. Department of Agriculture Handbook, 296.
Keywords: Long-Term Agroecosystem Research Network, LTAR, regionalization, agroecoregions, boundary analysis, agriculture, United States of America
Citation: Bean AR, Coffin AW, Arthur DK, Baffaut C, Holifield Collins C, Goslee SC, Ponce-Campos GE, Sclater VL, Strickland TC and Yasarer LM (2021) Regional Frameworks for the USDA Long-Term Agroecosystem Research Network. Front. Sustain. Food Syst. 4:612785. doi: 10.3389/fsufs.2020.612785
Received: 30 September 2020; Accepted: 31 December 2020;
Published: 15 February 2021.
Edited by:
Carla Pinheiro, New University of Lisbon, PortugalReviewed by:
Hanna J. Poffenbarger, University of Kentucky, United StatesAndreas Meyer-Aurich, Leibniz Institut für Agrartechnik und Bioökonomie, Germany
Copyright © 2021 Bean, Coffin, Arthur, Baffaut, Holifield Collins, Goslee, Ponce-Campos, Sclater, Strickland and Yasarer. This is an open-access article distributed under the terms of the Creative Commons Attribution License (CC BY). The use, distribution or reproduction in other forums is permitted, provided the original author(s) and the copyright owner(s) are credited and that the original publication in this journal is cited, in accordance with accepted academic practice. No use, distribution or reproduction is permitted which does not comply with these terms.
*Correspondence: Alisa W. Coffin, YWxpc2EuY29mZmluJiN4MDAwNDA7dXNkYS5nb3Y=