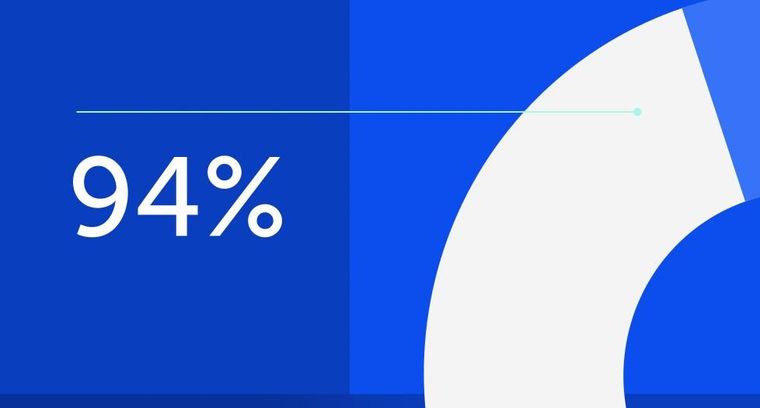
94% of researchers rate our articles as excellent or good
Learn more about the work of our research integrity team to safeguard the quality of each article we publish.
Find out more
ORIGINAL RESEARCH article
Front. Sustain. Food Syst., 27 November 2020
Sec. Agroecology and Ecosystem Services
Volume 4 - 2020 | https://doi.org/10.3389/fsufs.2020.539892
This article is part of the Research TopicMeasuring Interactions among Ecosystem Services in Agricultural LandscapesView all 7 articles
Improving the management of multiple ecosystem services (e.g., food provision, water and air quality regulation, carbon storage, and erosion control) in agricultural landscapes is a critical challenge to improve food system sustainability. However, we currently lack spatially-resolved national-level assessments of the relationships among services in agricultural landscapes over time. This limits our ability to make decisions and predict how environmental changes or agricultural management actions will impact multiple services. How do multiple ecosystem services vary across both space and time, at regional-to-national scales? To address this question, we quantified eight indicators of four ecosystem services across 290 Canadian agricultural landscapes in 1996, 2001, and 2006. We observed consistent correlations between pairs of services across the 290 ecodistricts in each of the 3 years of our study. In particular, ecodistricts with high livestock production had low provision of most regulating services, while ecodistricts with high air quality (ammonia retention) also had high soil and water quality regulation services. However, these ‘snapshot’ correlations poorly predicted how pairs of services changed through time. Ecosystem service change from 1996–2001 to 2001–2006 (as measured by pairwise correlations) showed markedly different patterns than snapshot correlations. In particular, where livestock production increased between years, so did most regulating services. Ecosystem service bundles also showed similar divergent patterns. The distribution of ecosystem service “snapshot” bundles—sets of ecodistricts with similar levels of provision across multiple ecosystem services in a single year—was generally stable between 1996 and 2006; only 15% of ecodistricts changed bundle types in this time period. However, ecosystem service “change” bundles—sets of ecodistricts with similar changes in ecosystem service provision through time—were much more dynamic. Nearly 60% of ecodistricts exhibited a different set of ecosystem service changes from 2001 to 2006 compared to 1996 to 2001. Our results add to the growing evidence that relationships between services across space do not necessarily predict service change through time. Improved understanding of the spatial patterns and temporal dynamics of ecosystem services, and better understanding of underlying processes, is crucial to improve agricultural landscape management for multifunctionality and sustainability.
Due to their global extent and impact on ecosystems and biodiversity (Foley et al., 2011), managing agricultural landscapes for multiple ecosystem services is critical to address current ecological and climate challenges (Bennett, 2017). Agricultural landscapes are multifunctional—they provide multiple ecosystem services to people beyond the food production they are primarily managed for (Jordan and Warner, 2010; Power, 2010). This includes pollination, pest regulation, opportunities for recreation, and aesthetic beauty, among others (Zhang et al., 2007). Managing agricultural systems for these multiple services, and in particular increasing food production while maintaining these other regulating and cultural services, is a critical challenge in food systems sustainability (Foley et al., 2011).
Achieving agricultural landscape multifunctionality requires knowledge of the dynamics, trade-offs, and synergies between ecosystem services (Bennett et al., 2009; Mastrángelo et al., 2019). Agricultural practices influence synergies and trade-offs between ecosystem services in a variety of ways. For example, converting an agricultural landscape in Hawaii to food crops was predicted to impact water quality but improve carbon storage, while conversely, conversion to pasture was predicted to improve water quality but reduce carbon storage through the clearing of currently abandoned fields (Goldstein et al., 2012). Similarly, conversion to perennial energy crops in the US Midwest can decrease farmer income by 75% and increase energy provision by 33%, while simultaneously improving environmental outcomes including phosphorus export to surface waters, carbon sequestration by soils, nitrous oxide emissions, and pollinator abundance (Meehan et al., 2013). In turn, the application of these different agricultural practices depends on environmental and climatic conditions, social capital and dynamics in the landscape, economic conditions, and land management objectives (D'souza et al., 1993; Teklewold et al., 2013). Studies exploring these links between agricultural practices and ecosystem services, while not common, are increasing and can help to inform management practices for agricultural landscapes.
However, to build multifunctional landscapes, we also need to know how environmental changes and different management actions today will impact the bundles of ecosystem services that landscapes provide in the future. In other words, we need to understand how ecosystem service relationships vary through time and space (Renard et al., 2015; Heydinger, 2016). Yet, this temporal knowledge of ecosystem services has been difficult to come by (Nicholson et al., 2009; Bürgi et al., 2014), with most past studies focusing on single snapshots in time (Dick et al., 2014; Crouzat et al., 2015; Jopke et al., 2015), often at limited regional spatial scales (Raudsepp-Hearne et al., 2010; Qiu and Turner, 2013; Bernués et al., 2015). The few studies that have been able to quantify temporal trends in multiple ecosystem services are often limited in their temporal or ecosystem service scope (Lautenbach et al., 2011; Jiang and Bullock, 2013). Though some recent studies have begun to address these shortcomings and represent important advances in ecosystem service science (Renard et al., 2015; Sutherland et al., 2016). These gaps in our understanding around how different ecosystem services vary through time and space across broad spatial scales restricts our ability to manage landscapes for multiple services (Bennett et al., 2009), despite increasing demand for a diversity of services from agricultural landscapes (Robertson and Swinton, 2005; Carpenter et al., 2009).
The benefits of understanding temporal and historical dynamics for sustainably managing natural and socio-ecological systems has been demonstrated in numerous fields, including informing restoration (Higgs et al., 2014) and conservation actions (Foster et al., 2003), understanding global environmental change (Vellend et al., 2013), and identifying drivers of agricultural dynamics (van Wesemael et al., 2010). The ability to identify which system components or drivers have changed historically, at what rates, and what critical thresholds exist is key to developing effective ways to manage socio-ecological systems (Biggs et al., 2009). For example, an historic analysis of European land management over the last 200 years identified three groups of drivers of land-use change and seven discrete land-management regimes, results critical to developing management actions and policies to improve land management sustainability at a continental scale (Jepsen et al., 2015). However, a wide variety of ecosystem service drivers exist, from the local management actions identified by Jepsen et al. to broader-scale climatic or economic conditions, and there is currently little knowledge of which individual variables or combination of these variables drive changes in multiple services (Bennett et al., 2009). Understanding temporal trends in ecosystem service provision is the first step toward identifying drivers for multiple ecosystem services and facilitating further improvements in agricultural sustainability.
While the integration of ecosystem services into agricultural decision-making has the potential to improve the sustainable management of agricultural landscapes, this potential is limited by the current lack of understanding around how static or variable ecosystem service relationships are through time (Renard et al., 2015). A great deal of effort has been put into understanding relationships between services—both synergies (positive relationships between services) and trade-offs (negative relationships between services)—across space at single points in time. Yet, much less effort or attention has focused on the temporal dynamics of these relationships. In agricultural systems, spatial relationships focused on trade-offs between food production and other regulating services have dominated the literature (Chan et al., 2006; Raudsepp-Hearne et al., 2010; Qiu and Turner, 2013), alongside negative relationships between food production and biodiversity conservation (Anderson et al., 2009; Phalan et al., 2011). While these studies often identify trade-offs between provisioning and regulating services (Power, 2010), there is also evidence that alternative agricultural management systems, such as conservation agriculture and agroecological approaches can foster win-win situations between services (Palm et al., 2014; Garbach et al., 2016). However, despite these promising results, it is unclear if the spatial dynamics of multiple services that are widely measured accurately reflect temporal relationships between services. In other words, some have argued that describing spatial patterns of ecosystem service provision and corresponding spatial relationships between services does not automatically mean that we can predict how they will change in the future (Spake et al., 2017), especially under alternative management systems. As human impacts on the environment and agricultural expansion become more widespread and rapid, there is an increasing need to understand how multiple ecosystem services change through time. This is a critical first step toward understanding the underlying processes that determine ecosystem service relationships and, in turn, identifying pathways toward more sustainable agricultural landscapes.
Here, we use primary and modeled ecosystem services data from 290 ecodistricts across Canadian agricultural landscapes to investigate the temporal and spatial dynamics of four ecosystem services (two indicators each for food production, air quality regulation, water quality regulation, and soil quality regulation) in 1996, 2001, and 2006. We (1) investigate the spatial and temporal relationships between pairs of ecosystem service indicators; (2) identify “snapshot” ecosystem service bundles (Raudsepp-Hearne et al., 2010), or sets of ecodistricts with similar levels of provision across multiple services for a single year; and (3) identify sets of ecodistricts with similar changes in ecosystem service provision (ecosystem service “change” bundles) across the two time steps of our analysis (i.e., 1996–2001 and 2001–2006).
We quantified eight indicators for four ecosystem services (Table 1) at the scale of ecodistricts (n = 290) for five Canadian provinces (Ontario, Manitoba, Saskatchewan, Alberta, and British Columbia) across 10 years (1996–2006) at a 5-year interval. Ecodistricts are part of a hierarchical classification system of ecosystems in Canada and represent the smallest ecological unit currently mapped nationally (Marshall et al., 1999) across which we could consistently estimate agricultural crop production and ecosystem services. Ecodistricts are subdivisions of larger ecoregions and have distinct combinations of relief, landforms, geology, soil, vegetation, waterbodies, and fauna. Only ecodistricts containing cropland in one of the 3 years of analysis (e.g., 1996, 2001, or 2006), as determined by the Canadian Agricultural Census, were included in our study (Supplementary Figure 1). In our analysis, ecodistricts averaged 5,105 km2 in size, with a range of 88–46,025 km2. In total, the ecodistricts in our study cover 1,485,572 km2 or 14.8% of Canada's total land area, although agricultural lands do not cover 100% of each ecodistrict. Provinces including Quebec, the Maritime provinces, and Newfoundland could not be included in our analysis due to a lack of crop yield and seeded-to-harvested crop area data for these regions. However, the provinces that are included in our analysis accounted for ~93% of Canada's croplands, 89% of beef and dairy cows, 69% of pigs and poultry, and 78% of turkeys in 1996 (Statistics Canada, 2008).
Table 1. Ecosystem service indicators for agricultural ecodistricts across Canada from 1996 to 2006.
We used a combination of Canadian Agricultural Census, Agriculture and Agri-Food Canada, and Statistics Canada datasets to estimate ecosystem service provision indicators across the ecodistricts in our study area. While indicators for air quality regulation, soil quality regulation, and water quality regulation came directly from the Canadian Agri-Environmental Indicators (AEI) program (AAFC, 2016), crop and livestock production data were estimated using a variety of national data sources. AEI indicators use a variety of data sources, across soil landscape, ecodistrict, and watershed scales to provide values at the soil landscape scale across Canada (~3,000 polygons in Canada's agricultural regions). AEI indicators have been used in Canada since 1981 to assess the environmental impacts of agriculture, and are a set of science-based indicators that were developed using internationally established methods (AAFC, 2016). Canada submits these agri-environmental indicators to the OECD (Organization for Economic Co-operation and Development) for comparison with other nations. While each indicator does have limitations and sources of uncertainty, most have been validated using on-the-ground data from Canadian agricultural systems. The AEI indicators are also currently the only nationally-consistent data on agri-environmental performance and ecosystem services across Canada. For each indicator, we used area-weighted averaging to calculate AEI values for each ecodistrict. Detailed descriptions of the service indicators are provided below.
For crop production we estimated total calories produced for nine major field crops in Canada: barley, canola, corn (feed and grain), field peas, flaxseed, oats, rye (spring and fall), soybeans, and wheat (Durum, spring, and winter). In 1996, these crops together covered ~72% of the cropland area of Canada (Statistics Canada, 2008). To calculate crop production, we combined data on ecodistrict-specific crop yields from Agriculture and Agri-food Canada, ecodistrict-specific seeded areas from the Canadian Agriculture Census, and seeded-to-harvested crop area losses from the Statistics Canada Field Crop Reporting Series, using the following equation:
where total crop production in calories Ci, j for ecodistrict i in year j equals the sum across all k crops of the product of ecodistrict- and year-specific values of seeded area S in hectares, seeded-to-harvested crop area loss L, crop yield Y in tons ha−1, and crop calorie content E in calories ton−1.
First, we estimated ecodistrict-level seeded-to-harvested crop area losses for the 1996, 2001, and 2006 growing seasons using data from the Canadian Field Crop Survey. This survey, administered five times during each year, collects data from a sample of around 25,000 Canadian farms, stratified across farm size, to gather data on seeded and harvested crop areas, yields, production, and farm stocks for principal field crops in Canada (Statistics Canada, 2018). The survey is administered and reported across 82 Census Agricultural Regions (CAR; 53 in our study area; Supplementary Figure 2), which are generally much larger than ecodistricts (in our analysis, mean: 73,510 km2; range: 47,209–864,027 km2). We calculated proportional seeded-to-harvested crop area changes for each CAR for 1996, 2001, and 2006; converted these shapefiles to 1 km2 resolution raster files; and then downscaled the CAR data to each ecodistrict by calculating area-weighted means at the ecodistrict level. This provided an estimate of the average seeded-to-harvested crop area losses in each ecodistrict for each crop and year of the analysis. For a small number of CAR in some years, the sample of farms that year did not include data on particular crops since they are only grown on a small proportion of farms in that region. In these cases, we used the value from the nearest CAR with data for that crop.
We then used these seeded-to-harvested crop area numbers to estimate harvested crop areas in each ecodistrict. The Canadian Agricultural Census, an in-depth census of farms and agriculture in Canada that is administered every 5 years (e.g., 1996, 2001, 2006) provides ecodistrict-level data on seeded areas for major crops (Statistics Canada, 2019). We combined this data with the seeded-to-harvested crop area changes estimated from the Field Crop Reporting Series to estimate final harvested areas for each ecodistrict for each year of the analysis. In the Field Crop Reporting Series, crop production data for feed corn are not collected. We therefore substituted values for grain corn in this case. Also, up until 2006, the Agricultural Census used aggregated crop categories for some crops. The aggregated categories included grains and cereals (barley, oats, and spring rye), canola and mustard, pulses (chickpeas, dried beans, field peas, and lentils), wheat and rye (fall rye and winter wheat), and spring wheats (Durum wheat and spring wheat). To determine crop-specific seeded areas for these crops, we used estimates from the Field Crop Survey (at the CAR scale) to estimate the proportion of each individual crop within these aggregated categories at the ecodistrict level for each year of analysis.
Next, we used estimated ecodistrict-level harvested area data to estimate crop production using ecodistrict-specific crop yields available from Agriculture and Agri-Food Canada for 1992–2009 (Du et al., 2015). This yield dataset was developed by Du et al. (2015) using farm-scale crop yield data, aggregated to the ecodistrict scale to address privacy concerns. In some situations, where privacy concerns were still present at the ecodistrict level due to the presence of very few farms growing a specific crop, Du et al. aggregated the crop yield data to the ecoregion or ecoprovince level (i.e., all ecodistricts within an ecoprovince or ecoregion have identical yields for a given crop). Some ecodistricts were also missing yield data for certain crops in certain years; in these cases, yields from the nearest ecodistrict were used. For barley, crop yield data in 1996 were missing. In this case, values from the nearest available year (1999) were substituted. All yield data were converted to metric tons per hectare prior to analysis.
Finally, crop production data in tons were converted to calories using crop calorie content data from Cassidy et al. (2013). For flaxseed, where no value was available from Cassidy et al., data from the Canadian Nutrient File database was used (Health Canada, 2018) (Supplementary Table 1). Calories produced provides a better estimate of the actual benefit of crop provision to people within each ecodistrict compared to measures such as cropland area or crop production in tons per hectare because calories are a more direct measure of the potential number of people that can be fed per hectare of cropland.
Similar to crop production, we estimated the total calories produced from agricultural animals per ecodistrict. The total numbers of livestock animals that primarily contribute to human food production present in each ecodistrict were taken from the Canadian Agricultural Census for each year of the analysis. This included the total number of beef cows; grower and finishing hogs; broiler, roaster, and Cornish chickens; turkeys; dairy cows; and laying hens. We were not able to include ducks or geese as these are not provided as separate livestock categories in the Agricultural Census. We then used FAO year-specific data on meat, egg, or milk yields per animal for Canada (FAO, 2019) to estimate the total grams of food produced per animal per year for each livestock type (Supplementary Table 2). Finally, we used calorie content per unit animal weight data from the Canadian Nutrient File to estimate total calories produced per animal for each livestock type and year of the analysis (Supplementary Table 2). These data were then converted into animal calories produced per square kilometer for each ecodistrict.
We used two indicators for air quality regulation: ammonia and particulate matter emissions from agricultural activities. The ammonia (NH3) emissions indicator estimates the amount of NH3 (kg ha−1 yr−1) emitted to the atmosphere from livestock and fertilizer applications (AAFC, 2016). It is calculated using specialized computational models for poultry, swine, dairy cows, beef cattle, and fertilizers. These models are then combined and integrate information on livestock numbers, fertilizer use, farm practices across twelve Canadian ecoregions, the total nitrogen in ammonia excreted by livestock in their manure, Canadian feeding and production practices for each specific livestock type, emissions factors for each Canadian ecoregion, and crop areas (AAFC, 2016). Particulate matter emissions were quantified by estimating emissions (kg ha−1 yr−1) of total particulate matter with a diameter <100 micrometers (combined total suspended particles, PM10, and PM2.5) from agricultural activities. This indicator uses data at the soil landscape scale on wind erosion, land preparation (e.g., tilling), crop harvesting methods and crop area, crop residue burning, grain handling, pollen emissions, fertilizer/chemical applications, animal feeding operations, and livestock carcass burning (AAFC, 2016). We refer to this indicator as dust retention below.
Indicators for soil carbon and soil erosion were used to estimate soil quality regulation services for each ecodistrict. The soil carbon indicator estimates the rate of change in organic C content per year in agricultural soils from land management changes using the Century model (Parton, 1996). Since this indicator measures change and not absolute soil carbon values, it likely better reflects changes in agricultural soil management practices. The indicator combines information on tillage; summer fallow frequency; and changes in the predominance of annual crops, perennial hay, or pasture to estimate soil organic carbon change (kg ha−1 yr−1). Soil erosion was calculated using the SoilERI model to estimate the amount of soil (tons ha−1 yr−1) lost from agricultural lands due to wind, water, and tillage erosion across each soil landscape (AAFC, 2016). This model estimates water erosion risk based on rainfall and runoff, crop type and area, landform, and soil erodibility using the USLE and RUSLE2 models; wind erosion based on soil texture and landform, crop residue levels, and windspeeds and rainfall after seeding; and finally, tillage erosion from tillage erosivity (erosion from tillage activities) and landscape erodibility (the susceptibility of a landscape to erosion due to the soil types and slopes present).
Water quality regulation was quantified using indicators for nitrogen and phosphorus releases to agricultural drainage water at the soil landscape scale. Water contamination from nitrogen (kg N ha−1 yr−1) was estimated using data on residual soil nitrogen, climate conditions, and the agricultural water cycle (water storage and loss) (De Jong et al., 2009). The input of residual soil nitrogen is estimated using the Canadian Agricultural Nitrogen Budget (Yang et al., 2007) that incorporates information on crop yields, fertilizer sales to estimate inputs, crop areas, livestock numbers, fertilizer and manure inputs, estimates of manure N losses from storage and land applications, mineralization from legume crop residues and manure, and climate data (AAFC, 2016). Risk of water contamination by phosphorus was estimated from the annual amount of dissolved P that may potentially be released from agricultural soil annually and the resulting degree of P in surface water (mg P kg water−1 year−1). This indicator incorporates data on surface runoff, drainage (tile drainage, surface drainage, and preferential flow), water erosion, and hydrological connectivity. In this case, the indicator has been calibrated against measured P water quality monitoring data from 88 Canadian agricultural watersheds from 1981 to 2001 (AAFC, 2016).
All of our ecosystem indicators were converted to units per hectare of cropland, units per kilometer ecodistrict area for animal production, or units per liter of water for phosphorus retention. In the case of crop production, we used ecodistrict-level data from the Agricultural Census of the total area of our nine crops to calculate calorie production per hectare. Indicator values were transformed where necessary so that higher values of each indicator corresponded to higher values of ecosystem service provision (e.g., lower levels of soil erosion loss or N loss represent higher values of service provision). For further analyses we standardized each ecosystem service indicator data to unit variance and zero-mean to help deal with the diversity of values and range of values in the data (Renard et al., 2015). However, in some cases we present normalized values where data for each indicator were scaled between their minimum and maximum values (i.e., between 0 and 1) to account for differences in scale.
We analyzed two related sets of ecosystem service data: (1) “snapshot” values for each indicator for each year of analysis, and (2) changes in indicator values through time. For the first, indicator data for each individual year (e.g., 1996, 2001, and 2006) were analyzed to investigate “snapshot” patterns in ecosystem service indicators and how these changed across years. To examine changes in service indicators through time, absolute changes in indicator values across time steps (e.g., between 1996 and 2001 and between 2001 and 2006) were calculated. All data analysis was completed using R 3.6.1.
We analyzed the spatial clustering of each ecosystem service indicator in each year and changes in ecosystem service indicators using Moran's I (Moran, 1950) and the “ape” package in R.
We investigated the temporal trends in each ecosystem service indicator through time using Space-Time Interaction (ST) analysis (Legendre et al., 2010), as used in Renard et al. (2015), to determine if statistically significant space-time interactions, as well as independent temporal differences and spatial patterns were present for each service. This method represents space and time with principal coordinates of neighbor matrices (PCNM) and uses the eigenfunctions from the PCNM analysis in an ANOVA Model 5 to test for a significant STI. If the STI is significant, this is evidence that ecosystem service indicator changes through time are not consistent across all ecodistricts, or that spatial patterns in service provision vary through time. If a significant STI was present, we modeled the spatial structure of each time period separately and used a one-factor nested ANOVA model to test for individual spatial and temporal changes. If the STI was nonsignificant, we used a Model II ANOVA to test for the individual spatial and temporal effects. Significant individual space (S) or time (T) effects indicate that ecosystem service indicator values significantly changed across ecodistricts for each time step or over time for all ecodistricts, respectively. All STI analyses were completed using the “adespatial” package in R.
We calculated two types of spatial correlations: “snapshot” and “change” correlations. For snapshot correlations, we calculated Spearman-rank correlations between each pair of ecosystem service indicators for each year across all 290 ecodistricts. For change correlations, we performed Spearman-rank correlation analysis on the absolute change in indicator values between years 1996–2001 and 2001–2006 for pairs of ecosystem services. This allowed us to examine whether pairs of ecosystem services changed the same way through time. All correlation analyses were performed using the “Hmisc” package in R.
We identified two types of ecosystem service bundles. First, ecosystem service “snapshot” bundles—sets of ecodistricts that provide similar levels of provision across multiple ecosystem services at a single point in time. And second, ecosystem service “change” bundles—sets of ecodistricts with similar ecosystem service changes through time. For both we used affinity propagation clustering (Frey and Dueck, 2007) to identify bundles. Affinity propagation uses measures of similarity between pairs of data points (i.e., ecodistricts) and exchanges messages between these data points until clusters and exemplars (the best representative example of that cluster) emerge. Importantly, affinity propagation does not require a priori specification of cluster number. Instead, an input preference is used to determine how strongly the algorithm considers each data point as a cluster exemplar and the final number of clusters then emerges from the clustering process. Affinity propagation has much lower error rates than other clustering methods and can complete clustering in a fraction of the time of other methods.
Affinity propagation clustering was performed on the entire time series of data for both spatial and change bundles using the “apcluster” package in R. To determine spatial bundle types, we used the normalized values of our eight ecosystem service indicators in each year for each ecodistrict. For change bundle types, we used the normalized absolute changes of the ecosystem service indicators between years for each ecodistrict. For each affinity propagation analysis, we completed an initial clustering exercise and then inspected dendrograms of all possible numbers of clusters to determine the most appropriate cluster number, balancing the amount of variation explained as cluster number increases against the increasingly subtle and small distinctions between individual clusters. We aimed for a small number of distinct clusters that demonstrated the major differences in patterns of ecosystem service provision across ecodistricts. The final number of clusters was six clusters for spatial bundle types and five for the change bundle types. We mapped the spatial distribution of both types of ecosystem service bundles using the “sf” package in R, calculated their spatial clustering using Moran's I and the “ape” package in R, and named each cluster according to its underlying characteristics (e.g., ecosystem service values or changes, crop types, etc.). Performance diagnostics of the clustering results are provided in the supplementary materials (Supplementary Figures 3, 4).
All of our ecosystem service indicators showed significant spatial clustering in each year of analysis (Moran's I 0.10–0.42, all P < 0.01; Supplementary Table 3, Supplementary Figures 5–12). Three of our ecosystem service indicators significantly increased between 1996 and 2006 [main time (T) effect from STI analysis, df = 290, P < 0.03; Figure 1]. This included livestock production (+10%), erosion control (+33%) and soil carbon (+111%). There was also a significant decrease in nitrogen retention (−30%). However, trends in ecosystem service provision through time across ecodistricts were not consistent, with significant space-time interactions for all services (STI interaction effect, df = 487, all P < 0.01; Figure 1). Spatial differences between ecodistricts for all ecosystem services were also significant at each time step [main space (S) effect from STI analysis, df = 594, P < 0.01; Figure 1].
Figure 1. Change in ecosystem service indicators through time for all 290 ecodistricts (mean ± SD). Axes have been flipped where necessary so that in all cases higher values along the y-axis in each plot represent greater levels of ecosystem service provision [e.g., soil, ammonia, total suspended particles (TSP), nitrogen, and phosphorus retention]. Results of a space-time interaction (STI) analysis are also presented for each ecosystem service below each plot (STI, space-time interaction; S, space effect, T, time effect). A significant p-value for the STI interaction indicates that the change in ecosystem service provision through time was not uniform across all ecodistricts or the spatial variation between ecodistricts was not consistent through time, S indicates that values differed across ecodistricts for each time step, and for T indicates values differed through time across all ecodistricts.
Strong and consistent snapshot correlations between ecosystem services were present for all 3 years of our analysis. Averaged across the 3 years of our study, over two-thirds (19 pairs or 68%) of the 28 possible pairs of ecosystem services had strong positive or negative snapshot correlations (−0.2 ≤ r ≥ 0.2; Figure 2). In general, we observed negative correlations between provisioning and regulating services, but positive ones between different regulating services In particular, livestock production had strong negative snapshot correlations with all other services, except for crop production (strong positive correlation) and soil carbon change (no correlation). Contrastingly, ammonia retention had strong positive snapshot correlations with all other services except for crop and livestock production, which were both strongly negative. Many regulating services had strong positive correlations, except for dust retention and soil carbon change. Finally, crop production had strong positive snapshot correlations with livestock production and soil carbon change, but strong negative snapshot correlations with ammonia, dust, and soil retention.
Figure 2. (A) Snapshot correlations between pairs of ecosystem service indicators for each of the 3 years (1996, 2001, and 2006) averaged over all 3 years; and (B) change correlations between pairs of ecosystem service indicators over 1996–2001 and 2001–2006 averaged over the two periods. Spearman-rank correlations are presented: gray indicates neutral or weak correlations (i.e., −0.2 < r < 0.2), green strong positive correlations (i.e., r > 0.2), and orange strong negative correlations (i.e., r < −0.2). In each case, ecosystem service indicator values have been arranged so that higher values indicate greater levels of ecosystem service provision (e.g., lower TSP or runoff P indicate higher provision of air quality or water quality regulation, respectively). Correlations that are significant statistically (P < 0.01) are indicated by “*”.
These snapshot correlations were largely consistent across the individual 3 years of our study (Supplementary Table 4). None of the 28 possible pairs of ecosystem services changed from a strong positive to a strong negative spatial correlation or vice versa between 1996 and 2006. Further, only six pairs changed from a strong positive or strong negative spatial correlation to a weak/nonsignificant spatial correlation or vice versa.
Out of a possible 28 ecosystem services pairs, fourteen (50%) of the change correlations (i.e., positive or negative associations in ecosystem service changes between years) were strongly positive or negative (Figure 2; Supplementary Figures 13–20). Averaged across the two time periods of our analysis, when livestock production increased through time, so did dust retention, soil retention, and soil carbon change. Contrastingly, increases in ammonia retention between years were associated with decreases in livestock production, dust retention, soil carbon change, and soil retention. Changes in crop production between years were not strongly correlated with changes in any other ecosystem service indicators but changes in dust retention, soil carbon change, and soil retention were positively associated.
Change correlations were much more dynamic than the spatial correlations, especially for those involving crop production. Overall, four pairwise change correlations switched from being strongly negative to strongly positive between 1996–2001 and 2001–2006 (Supplementary Table 4). All of these involved crop production and its correlations with livestock production, dust retention, soil carbon change, and soil retention. Additionally, ten change correlations (36%) switched from strong positive or negative to weak/nonsignificant correlations, or vice versa.
Fifteen out of the nineteen (79%) strong snapshot correlations changed direction (five ecosystem service pairs) or changed to weak or nonsignificant correlations (10 pairs) when the corresponding change correlations were considered (Table 2). In other words, only four of the fourteen strong positive or negative change correlations corresponded to snapshot correlations of the same direction and strength. Specifically, livestock production-ammonia retention and livestock production-N retention both had negative snapshot and change correlations, while ammonia retention-N retention and TPS retention-soil retention had positive snapshot and change correlations (Figure 2). Contrastingly, strong positive snapshot correlations between ammonia retention-TSP retention, ammonia retention-soil retention, and TSP retention-soil carbon switched to strong negative change correlations, and strong negative snapshot correlations between livestock production-TSP retention and livestock production-soil retention shifted to strong positive change correlations.
Table 2. Number of agricultural ecodistricts across Canada providing each ecosystem service snapshot bundle type for 1996, 2001, and 2006 and percentage change in these numbers through time.
The direction of the change correlations between ecosystem services was independent of the corresponding snapshot correlations (Chi-square test: Fisher's Exact Test P = 0.937; Supplementary Table 5). Similarly, there was no relationship between the value of the snapshot and change correlations across all of the ecosystem service pairs (linear regression: estimate = 0.120 ± 0.025, P = 0.641).
We identified six ecosystem service snapshot bundle types across the 290 ecodistricts and 3 years of analysis. Overall, the bundle analysis reflected the correlation analysis; bundles demonstrate the same positive and negative pairwise relationships between services but synthesize the paired results into more complex bundles. Two bundle types (S1 and S6) had relatively high levels of crop provision along with lower levels of animal production and a mix of other regulating services (Figure 3). Bundle type S1 (prairie wheat) and S6 (mixed field crops) had similar levels of both provisioning services, but S6 had improved dust and soil retention. Three bundle types (S3, S4, S5) had a mix of crop and animal production but demonstrated trade-offs between provisioning and regulating services. For example, S3 (livestock + corn/soy) had higher levels of animal production relative to crops but lower levels of ammonia retention, dust retention, soil carbon change, and soil retention compared to S4 (field crops + livestock) where animal production was lower. Bundle S5 (intensive agriculture) showed the highest levels of both crop and animal production but also the lowest values for ammonia and phosphorus retention. Finally, bundle type S2 (low input) had low values for both crop and animal production but high values for every regulating service except soil carbon. One bundle type, S5 (intensive agriculture) was represented by a single but unique ecodistrict in the lower Fraser Valley of British Columbia characterized by extremely high crop and livestock production but very low ammonia and phosphorus retention.
Figure 3. Ecosystem service provision within snapshot bundle types across all 3 years of analysis (N = 870). Normalized values are presented; therefore, boxplots present values scaled between the minimum (e.g., 0) and maximum (e.g., 1) values for each ecosystem service indicator across all ecodistricts and years. Where necessary, ecosystem service indicator values have been reversed so that in all cases higher values represent greater levels of ecosystem service provision.
Ecosystem service snapshot bundle types showed strong spatial clustering in all 3 years of the analysis (all Moran's I > 0.13, P < 0.01). Bundle type S1 (prairie wheat) dominated the Canadian prairies in Alberta and Saskatchewan, S3 (livestock + corn/soy) dominated southern Ontario, S2 (low input) more northern and mountain agricultural areas, S4 (field crops + livestock) the eastern slopes of the Rockies and southern Manitoba, and S6 (mixed field crops) northern and eastern prairie areas (Figure 4).
Figure 4. Distribution of snapshot ecosystem service bundle types in Canadian agricultural ecodistricts for (A) 1996, (B) 2001, and (C) 2006.
The spatial distribution of ecosystem service snapshot bundle types shifted across the 3 years of our analysis. The largest shift involved ecodistricts providing S1 (prairie wheat) shifting to S6 (mixed field crops; Table 2). The number of S1 ecodistricts decreased by 54% (50 ecodistricts) between 1996 and 2006, while S6 ecodistricts increased by 58% (42 ecodistricts). Other ecosystem service snapshot bundle types showed much smaller changes; S2 (low input) and S4 (field crops + livestock) increased by 6 and 11% respectively, while S3 (livestock + corn/soy) and S5 (intensive agriculture) did not change.
We identified five different trajectories of ecosystem service change at the ecodistrict level across the two time steps of our analysis (i.e., 1996–2001 and 2001–2006). Two ecosystem service change bundle types were characterized by improvements in most regulating services, especially dust retention, soil carbon change, and soil erosion retention, and either a decrease in crop provision (C1—crop loss) or an increase (C3—increasing crops & soil; Figure 5, Table 3). Two other change bundle types showed more modest improvements in regulating services, along with a small decrease in soil carbon change and small increase in crop production (C2—service stability) or a combination of a large crop production increase, small animal production decrease, and improvement in N retention (C4—animals to crops; Figure 5). One ecodistrict had a unique service change trajectory between 2001 and 2006 that demonstrated a unique improvement in ammonia retention.
Figure 5. Mean change in ecosystem service indicators relative to overall mean change across all ecodistricts, for each ecosystem service change bundle type (N = 580). Standardized values are presented; therefore, values represent ecosystem service indicator change relative to the average levels of change across all ecodistricts and two time steps (1996–2001 and 2001–2006). Bundle type C5 is not shown due to low sample size (N = 1). Where necessary, ecosystem service indicator values have been reversed so that in all cases higher values represent greater levels of ecosystem service provision.
Table 3. Mean 5-year change in ecosystem service indicators (± standard error) for ecosystem service change bundle types across the two time periods (1996–2001 and 2001–2006).
Similar to the snapshot ecosystem service bundles, the ecosystem service change bundle types demonstrated strong spatial clustering in both time periods (Moran's I > 0.09, P < 0.01). From 1996 to 2001, bundle type C1 (crop loss) was dominant across the Canadian prairies and extreme southern Ontario (Figure 6), while the remainder of ecodistricts in Canada were predominantly C2 (service stability). Contrastingly, in 2001–2006 most of the prairies shifted to C3—increasing crops & soil ecosystem services (Saskatchewan) and C4—animals to crops (Manitoba and Alberta), as did southern Ontario. A few C4 ecodistricts also appeared in British Columbia and northern Alberta. The geographic pattern of ecosystem service bundle types was also similar to that of the snapshot bundle types, especially in 2001–2006, in that the Canadian prairies and southern Ontario regions show distinct bundle types.
Figure 6. Distribution of ecosystem service change bundle types (sets of similar ecosystem service indicator changes through time) in Canadian agricultural ecodistricts between (A) 1996–2001, and (B) 2001–2006. Bundle change type C5 was only present in the 2001–2006 time period.
There was a strong shift in the prevalence and distribution of ecosystem service change bundles between 1996–2001 and 2001–2006 (Table 4). The most obvious change was an almost complete loss of C1 (crop loss) ecodistricts (−99%) in 2001–2006. In 1996–2001, 132 ecodistricts displayed this change bundle, but only one ecodistrict did in 2001–2006. There was also a large loss of C2 (service stability) ecodistricts (−33%) in 2001–2006. These losses were balanced by gains for bundles C3 (increasing crops & soil; +79 ecodistricts) and C4 (animals to crops; +100 ecodistricts). The ecosystem service changes reflected in the change bundle types also reflect the temporal changes in snapshot bundle types, especially in the prairies. For example, the shift from snapshot S1 to S6 bundle types corresponded to increases in dust retention and soil carbon change, decreases in nitrogen retention, and little change in ammonia retention. Similarly, change bundle types C1 (1996–2001) and C3 (2001–2006) showed similar patterns of change in these services.
Table 4. Number of agricultural ecodistricts across Canada exhibiting similar trends in ecosystem service provision change (i.e., ecosystem service change bundles) between years for 1996–2001 and 2001–2006, and percentage change in these numbers through time.
We observed a variety of trends in overall ecosystem service provisioning over the 3 years of our study, some of which likely stem from widespread changes in agricultural practices in Canada. While soil regulating services increased substantially from 1996 to 2006, water regulating services decreased. Much of this improvement in soil regulation likely originates from an increase in conservation tillage and no-till systems across Canada during this time (Friedrich et al., 2012; Awada et al., 2016). Similarly, a reduction in tillage intensity and summer fallow area (cropland that is not cropped for at least 1 year, primarily for conserving soil moisture) is likely drove the increases in soil carbon, especially in the prairies (McConkey, 2003). Between 1981 and 2001, agricultural soils in Canada also switched from a source of CO2 (−1.2 megatonnes CO2 year−1) to a sink (11.9 megatonnes CO2 year−1) (AAFC, 2016). Conversely, for water regulation, increased use of fertilizers and manure, and an increase in livestock production concentration, has increased the risk of N and P loss to agricultural surface water bodies (De Jong et al., 2009; van Bochove et al., 2011). These changes are reflected in the main shift in ecosystem service spatial bundle types between 1996 and 2006. Particularly in the Prairies, S1 ecodistricts (prairie wheat) switched to S6 (mixed field crops) with a corresponding improvement in air quality (dust retention) and soil quality (soil erosion control and soil carbon), but a decline in air quality (ammonia retention) and water quality (N retention).
Similar to past studies of multiple ecosystem services (Chan et al., 2006; Raudsepp-Hearne et al., 2010; Jopke et al., 2015; Renard et al., 2015; Lee and Lautenbach, 2016) we observed strong negative snapshot correlations between crop and animal production and other regulating ecosystem services but strong positive relationships between most regulating services. Intensive agriculture, which is often required to support high density animal production, can generate challenges for soil, fertilizer, and manure management that often lead to loss of water, air, and soil regulating services (Clark and Tilman, 2017). In particular, increasing crop and animal production usually requires agricultural expansion and the fragmentation and loss of natural habitats (Foley et al., 2011). These processes can negatively impact regulating services by impacting biodiversity and ecosystem function (Haddad et al., 2015) and increase flows of water, soil, and pollutants (Mitchell et al., 2015). We assume that these processes are also occurring across our agricultural landscapes, but could not specifically investigate these processes here.
The one exception to the tradeoff between food production and regulating services was a positive relationship between crop production and soil carbon. We expect this is driven both by broad-scale patterns of soil fertility, climatic conditions, and crop production in Canada (i.e., those areas with higher quality soils and carbon content also have the best growing conditions and crop yields) and the fact that improvement in soil management in Canada, especially in the Prairies, has led to improved crop production and soil organic matter. The positive correlations among regulating services most likely reflect the fact that beneficial management practices such as reduced tillage and improved fertilizer management can simultaneously reduce soil erosion and dust emissions while increasing soil carbon, or reduce ammonia emissions to the air along with nitrogen and phosphorus runoff, respectively (Koschke et al., 2013; Syswerda and Robertson, 2014).
Our study also shows that snapshot synergies and trade-offs between ecosystem services do not necessarily correspond to changes in ecosystem services through time. Pairwise correlations in ecosystem service change from 1996–2001 to 2001–2006 contrasted strongly with snapshot correlations, and only a small number were consistent across the two sets. Some of this may stem from the fact that certain services, such as crop production, had contrasting and opposing trends in 1996–2001 vs. 2001–2006. For example, the intense drought of 2001–2002 in the Canadian Prairies meant that crop production declined strongly from 1996 to 2001 but then increased from 2001 to 2006. This likely produced the lack of strong average relationships between crop production changes and changes in other ecosystem services across our two time periods. In other words, we believe that the changes in crop production were more strongly driven by weather conditions, and less connected to the management practices that likely affected other ecosystem services. However, for other pairs of services, such as livestock production with dust retention, soil carbon change, or soil retention, while these services may be negatively related at broad spatial scales, simultaneous growth and broad improvements in the environmental performance of livestock production might result in positive change correlation between livestock production and regulating services at the ecodistrict level. We suspect that improved soil management practices have allowed for increased animal numbers and food production in Canada with a simultaneous improvement in soil conditions. This contrast between snapshot pairwise ecosystem service relationships and how these relationships change through time has important management implications. Specifically, it emphasizes the fact that understanding relationships between management actions and ecosystem service outcomes based on analyzing snapshots of ecosystem service provisioning are unlikely to be sufficient for managing multiple services dynamically through time. Instead, improved temporal datasets and, most importantly, understanding of the processes underlying different sets of services are needed.
For those services that showed consistent snapshot and change correlations, we hypothesize this results from strong shared mechanisms. For example, the strong negative relationships (both snapshot and change) between livestock production and both ammonia and nitrogen retention likely reflect the substantial effect that concentrated livestock production has on air quality and specifically ammonia emissions (65% of total agricultural ammonia emissions in Canada in 2011) and how the application of manure from livestock on croplands leads to nitrogen release to surface waters (AAFC, 2016). This shared driver may also be the source of the strong and consistent positive correlations between ammonia and water quality (nitrogen retention). Similarly, the shared process of wind erosion may be driving the strong positive snapshot and change correlations between dust and soil retention.
Consistent with the ecosystem service relationships we observed, we also saw contrasting changes in ecosystem service snapshot and change bundles. While snapshot bundles changed only minimally over 10 years of our analysis, change bundles showed much more dynamic shifts. We hypothesize that snapshot bundles are primarily determined by broad-scale patterns of soils and climate and how these influence and limit crop production and the biophysical processes that determine soil, air, and water conditions. Therefore, changes in the distribution of snapshot bundles are likely to occur relatively slowly. For example, the distribution of snapshot bundles seems to be to remarkably correlated to ecozones in Canada (Figure 7). This is not surprising, both because the extent and intensity of agricultural activities is largely driven by climate and soil suitability for crops (Leemans and Solomon, 1993; Ramankutty et al., 2002), and the relationships between crop and livestock production and changes in regulating services are strongly affected by the vegetation, soil types, and climatic conditions that are present (Brauman et al., 2007). While change bundles were more dynamic, the patterns of change we observed also reflect regional differences in weather, especially the drought conditions of 2001–2002 on the Canadian Prairies (Bonsal and Regier, 2007). The scale of our analysis, that includes such a broad geographic area of Canada, might in part be influencing this. We suspect that analyses of smaller regions with more similar biophysical conditions or weather through time should facilitate the identification of the local-scale processes and management actions that drive change in multiple ecosystem services.
However, our analysis also indicates that ecosystem service snapshot bundles are not wholly dependent on climatic, biophysical, or soil conditions, but can shift across years, potentially due to changes in agricultural management decisions. An obvious example of this is the large shift of S1 ecodistricts to S6 ecodistricts in the Canadian Prairies between 1996 and 2001. This shift was the result of large improvements to TSP retention, soil retention, and P retention. However, in regions where livestock production is a substantial component (i.e., S3 and S4), similar shifts appear to be much less common and more difficult to achieve. These S3 and S4 landscapes in Canada are dominated by intensive dairy, pig, beef, and chicken production, with consequences for air, soil, and water quality (Tilman et al., 2002). The temporal shifts in service bundles that we observed in some regions of Canada suggest that more multifunctional agricultural landscapes with improved service provision can be developed in the future. However, further identification of the specific local-scale drivers behind these shifts would assist in facilitating these transitions.
Contrastingly, ecosystem service change bundles are likely influenced to a greater degree by shorter timescale weather conditions and agricultural practices and therefore will change to a much greater degree through time. The strong shift in ecosystem service change bundles between 1996–2001 and 2001–2006 is likely mainly driven by drought conditions and crop failures in the Canadian Prairies. The period of 2001–2002 was a period of intense drought and poor growing conditions, especially in Alberta, Saskatchewan, and Manitoba (Bonsal and Regier, 2007). This substantially affected crop production and yields in these areas, and likely led to the low crop production values for bundle C1. These conditions relented in 2003, and crop yields and production rebounded by 2006. Bundles C3 and C4, which dominate the Prairies in 2001 and 2006 reflect this, with crop production values above the mean service provision value across all ecodistricts. However, this increase in crop production was also accompanied by two sets of change for other ecosystem services: larger increases in livestock production, dust retention, soil carbon change, erosion control, and P loss in Saskatchewan (bundle C3); or livestock production losses with more modest increases in regulating services in the rest of the prairies (bundle C4). Some of this may relate to differences in agricultural policy between Saskatchewan and the other prairie provinces, as well as differences in crops grown and agricultural practices that have yet to be identified.
As opposed to past studies of multiple ecosystem services in agricultural landscapes (Renard et al., 2015), our bundles were generally not dominated by only one or two ecosystem services. This contrasting result might be due to the larger units of analysis in our study (e.g., ecodistricts vs. municipalities) compared to previous studies. With a larger area, our ecodistricts likely each contain a variety of ecosystem types (e.g., agriculture, forests, grasslands) and therefore provide a wider diversity of ecosystem services and will be less specialized than smaller agricultural areas. The impact of spatial scale on relationships between ecosystem services has been shown to be strong and important (Anderson et al., 2009), however its impact on both the identity and spatial pattern of ecosystem service bundles is less clear and has not been widely investigated.
Temporal trends in ecosystem service relationships and bundles are only just beginning to be explored (Renard et al., 2015). One of the central assumptions of much of the work on ecosystem service relationships is that snapshot correlations between services across different spatial locations will provide insights into the bundles of services that landscapes can provide through time (i.e., space-for-time assumptions) and help identify actions to build more multifunctional landscapes (Spake et al., 2017). However, this will only be the case if service relationships and bundles identified across space actually reflect service relationships through time. Our analysis is one of the first to investigate this assumption and show that correlations between ecosystem services through time do not necessarily match snapshot relationships. The strong shifts in direction and strength between snapshot and change relationships that we observed suggests that snapshot correlations between services may not be a useful guide for managing services through time. This is because snapshot relationships between ecosystem services may be driven more by broad-scale patterns of climate, soils, and agricultural practices. For example, soil types and climate are largely constant, while overlying socioeconomic conditions may constrain agricultural practices (Khaledi et al., 2010). Contrastingly, changes in ecosystem services through time are more likely to reflect realistic changes in agricultural landscapes that could affect ecosystem services and improve landscape multifunctionality. These include relatively short-scale weather events (e.g., droughts), changes in crops grown that reflect changing market and economic forces, and changes in farming technology and practices. We are just beginning to understand how services change through time and what actions are most important for these changes. Our results continue to raise concerns about the practice of informing long-term management of ecosystem services from analyses of single points of time (Renard et al., 2015; Spake et al., 2017). Increased effort to understand these dynamics, across both temporal and spatial scales, including an understanding of the underlying processes, is likely to lead to important insights about managing multiple services.
Our analysis is a first step to understand how ecosystem services in agricultural landscapes change across space and time at broad scales. Empirical historical data on regulating services is difficult to find, especially at broad regional or national scales, and our study is no exception. The majority of indicators for regulating services were modeled and do not represent primary data. Agri-environmental indicator modeling methods in Canada are rigorous, incorporate multiple data sources, and integrate current understanding of the links between agricultural activities and regulating services. However, how well they represent ecodistrict-specific levels and trends in services is unknown. Even for relatively well-measured services, such as food production, the nine field crops we used represent only a small proportion of the crops grown in certain ecoregions. Improved data collection and methods to quantify regulating services would benefit future analyses of multiple ecosystem services through time. For example, by improving networks for the monitoring of agricultural water quality, creating new monitoring programs that explicitly measure multiple ecosystem services and potential drivers behind them (Bennett et al., 2015), developing new remote sensing approaches to evaluate crop production and other regulating services (Cord et al., 2017), and integrating this information into ecosystem service models (Newlands et al., 2012). At present, a lack of existing or available data on cultural services in agricultural landscapes also prevents a fuller accounting of the trends and interactions between agricultural ecosystem services in Canada. Finally, the impact of spatial or temporal scale (i.e., smaller spatial units of analysis, a more limited spatial extent, or the inclusion of additional years) is currently unknown and limited by available data. Improved efforts to measure and monitor key ecosystem service indicators across the agricultural landscapes of Canada over the long-term are key to understanding these relationships and how to build sustainable and multifunctional landscapes.
Our results add evidence to the growing body of knowledge that ecosystem service patterns and associations are dynamic and vary strongly across space and time. In particular, we provide results at broader regional and national scales than have previously been investigated, and demonstrate that service relationships across space at single points in time may not correspond to patterns of ecosystem service change through time. Our results suggest that the widespread practice of identifying service synergies and trade-offs by measuring service indicators at different places at a single time is unlikely to provide sufficient information needed to manage for multiple services through time. We suggest that increased efforts to understand how the provision of ecosystem services change simultaneously across space and through time, and more importantly better understanding of the processes underlying both spatial and temporal patterns in ecosystem services, are key to developing truly multifunctional agricultural landscapes.
The datasets generated for this study are available on request to the corresponding author.
MM, KC, and NR contributed to the conception and design of the study. NN contributed key data without which the study would have not been possible. MM collected and organized the data and performed the statistical analysis and wrote the first draft of the manuscript. All authors contributed to the manuscript revision, read, and approved the submitted version.
MM was supported by a National Sciences and Engineering Research Council (NSERC) Postdoctoral Fellowship.
The authors declare that the research was conducted in the absence of any commercial or financial relationships that could be construed as a potential conflict of interest.
We would like to acknowledge the Canadian federal agencies (Agriculture and Agri-Food Canada and Statistics Canada) that create and make freely accessible many of the datasets without which this study would not have been possible. The CHANS and LUGE lab groups provided useful feedback and input on this research.
The Supplementary Material for this article can be found online at: https://www.frontiersin.org/articles/10.3389/fsufs.2020.539892/full#supplementary-material
AAFC (2016). “Environmental sustainability of Canadian Agriculture,” in Agri-Environmental Indicator Report Series, eds. R. L. Clearwater, T. Martin, and T. Hoppe (Ottawa, ON: Agriculture and Agri-Food Canada).
Anderson, B. J., Armsworth, P. R., Eigenbrod, F., Thomas, C. D., Gillings, S., Heinemeyer, A., et al. (2009). Spatial covariance between biodiversity and other ecosystem service priorities. J. Appl. Ecol. 46, 888–896. doi: 10.1111/j.1365-2664.2009.01666.x
Awada, L., Gray, R., and Nagy, C. (2016). The benefits and costs of zero tillage RD&E on the Canadian Prairies. Canad. J. Agr. Econ. 64, 417–438. doi: 10.1111/cjag.12080
Bennett, E. M. (2017). Changing the agriculture and environment conversation. Nat. Ecol. Evol. 1:0018. doi: 10.1038/s41559-016-0018
Bennett, E. M., Cramer, W., Begossi, A., Cundill, G., Diaz, S., Egoh, B. N., et al. (2015). Linking biodiversity, ecosystem services, and human well-being: three challenges for designing research for sustainability. Curr. Opin. Environ. Sustain. 14, 76–85. doi: 10.1016/j.cosust.2015.03.007
Bennett, E. M., Peterson, G. D., and Gordon, L. J. (2009). Understanding relationships among multiple ecosystem services. Ecol. Lett. 12, 1394–1404. doi: 10.1111/j.1461-0248.2009.01387.x
Bernués, A., Rodríguez-Ortega, T., Alfnes, F., Clemetsen, M., and Eik, L. O. (2015). Quantifying the multifunctionality of fjord and mountain agriculture by means of sociocultural and economic valuation of ecosystem services. Land Use Policy 48, 170–178. doi: 10.1016/j.landusepol.2015.05.022
Biggs, R., Carpenter, S. R., and Brock, W. A. (2009). Turning back from the brink: Detecting an impending regime shift in time to avert it. Proc. Natl. Acad. Sci. U.S.A. 106, 826–831. doi: 10.1073/pnas.0811729106
Bonsal, B., and Regier, M. (2007). Historical comparison of the 2001/2002 drought in the Canadian Prairies. Clim. Res. 33, 229–242. doi: 10.3354/cr033229
Brauman, K. A., Daily, G. C., Duarte, T. K., and Mooney, H. A. (2007). The nature and value of ecosystem services: an overview highlighting hydrologic services. Ann. Rev. Environ. Resour. 32, 67–98. doi: 10.1146/annurev.energy.32.031306.102758
Bürgi, M., Silbernagel, J., Wu, J., and Kienast, F. (2014). Linking ecosystem services with landscape history. Landscape Ecol. 30, 11–20. doi: 10.1007/s10980-014-0102-3
Carpenter, S. R., Mooney, H. A., Agard, J., Capistrano, D., DeFries, R. S., Díaz, S., et al. (2009). Science for managing ecosystem services: beyond the millennium ecosystem assessment. Proc. Natl. Acad. Sci. U.S.A. 106, 1305–1312. doi: 10.1073/pnas.0808772106
Cassidy, E. S., West, P. C., Gerber, J. S., and Foley, J. A. (2013). Redefining agricultural yields: from tonnes to people nourished per hectare. Environ. Res. Lett. 8, 034015–034019. doi: 10.1088/1748-9326/8/3/034015
Chan, K. M. A., Shaw, M. R., Cameron, D. R., Underwood, E. C., and Daily, G. C. (2006). Conservation planning for ecosystem services. PLoS Biol. 4, 2138–2152. doi: 10.1371/journal.pbio.0040379
Clark, M., and Tilman, D. (2017). Comparative analysis of environmental impacts of agricultural production systems, agricultural input efficiency, and food choice. Environ. Res. Lett. 12, 064016. doi: 10.1088/1748-9326/aa6cd5
Cord, A. F., Brauman, K. A., Chaplin-Kramer, R., Huth, A., Ziv, G., and Seppelt, R. (2017). Priorities to advance monitoring of ecosystem services using earth observation. Trends Ecol. Evol. 32, 416–428. doi: 10.1016/j.tree.2017.03.003
Crouzat, E., Mouchet, M., Turkelboom, F., Byczek, C., Meersmans, J., Berger, F., et al. (2015). Assessing bundles of ecosystem services from regional to landscape scale: insights from the French Alps. J. Appl. Ecol. 52, 1145–1155. doi: 10.1111/1365-2664.12502
De Jong, R., Drury, C. F., Yang, J. Y., and Campbell, C. A. (2009). Risk of water contamination by nitrogen in Canada as estimated by the IROWC-N model. J. Environ. Manag. 90, 3169–3181. doi: 10.1016/j.jenvman.2009.05.034
Dick, J., Maes, J., Smith, R. I., Paracchini, M. L., and Zulian, G. (2014). Cross-scale analysis of ecosystem services identified and assessed at local and European level. Ecol. Indic. 38, 20–30. doi: 10.1016/j.ecolind.2013.10.023
D'souza, G., Cyphers, D., and Phipps, T. (1993). Factors affecting the adoption of sustainable agricultural practices. Agric. Resour. Econ. Rev. 22, 159–165. doi: 10.1017/S1068280500004743
Du, Y., Huffman, T., Daneshfar, B., Green, M., Feng, F., Liu, J., et al. (2015). Improving the spatial resolution and ecostratification of crop yield estimates in Canada. Can. J. Soil Sci. 95, 287–297. doi: 10.4141/cjss-2014-017
FAO (2019). FAOSTAT - Livestock Primary Database. Food and Agriculture Organization of the United Nations. Available online at: http://www.fao.org/faostat/en/#data/QL (accessed Febraury 28, 2020).
Foley, J. A., Ramankutty, N., Brauman, K. A., Cassidy, E. S., Gerber, J. S., Johnston, M., et al. (2011). Solutions for a cultivated planet. Nature 478, 337–342. doi: 10.1038/nature10452
Foster, D., Swanson, F., Aber, J., Burke, I., and Brokaw, N. (2003). The importance of land-use legacies to ecology and conservation. BioScience 52, 77–88. doi: 10.1641/0006-3568(2003)0530077:TIOLUL2.0.CO;2
Frey, B. J., and Dueck, D. (2007). Clustering by passing messages between data points. Science 315, 972–976. doi: 10.1126/science.1136800
Friedrich, T., Derpsch, R., and Kassam, A. (2012). Overview of the global spread of conservation agriculture. Field Actions Sci. Rep. 6, 1–7. doi: 10.1080/00207233.2018.1494927
Garbach, K., Milder, J. C., Declerck, F., de Wit, M. M., Driscoll, L., and Gemmill-Herren, B. (2016). Examining multi-functionality for crop yield and ecosystem services in five systems of agroecological intensification. Int. J. Agric. Sustain. 15, 11–28. doi: 10.1080/14735903.2016.1174810
Goldstein, J. H., Caldarone, G., Kaeo Duarte, T., Ennaanay, D., Hannahs, N., Mendoza, G., et al. (2012). Integrating ecosystem-service tradeoffs into land-use decisions. Proc. Natl. Acad. Sci. U.S.A. 109, 7565–7570. doi: 10.1073/pnas.1201040109
Haddad, N. M., Brudvig, L. A., Clobert, J., Davies, K. F., Gonzalez, A., Holt, R. D., et al. (2015). Habitat fragmentation and its lasting impact on Earth's ecosystems. Sci. Adv. 1:e1500052. doi: 10.1126/sciadv.1500052
Health Canada (2018). Canadian Nutrient File. Available online at: https://food-nutrition.canada.ca/cnf-fce/index-eng.jsp (accessed Febraury 28, 2020).
Heydinger, J. M. (2016). Reinforcing the ecosystem services perspective: the temporal component. Ecosystems 19, 661–673. doi: 10.1007/s10021-016-9959-0
Higgs, E., Falk, D. A., Guerrini, A., Hall, M., Harris, J., Hobbs, R. J., et al. (2014). The changing role of history in restoration ecology. Front. Ecol. Environ. 12, 499–506. doi: 10.1890/110267
Jepsen, M. R., Kuemmerle, T., Müller, D., Erb, K., Verburg, P. H., Haberl, H., et al. (2015). Transitions in European land-management regimes between 1800 and 2010. Land Use Policy 49, 53–64. doi: 10.1016/j.landusepol.2015.07.003
Jiang, M., and Bullock, J. M. (2013). Mapping ecosystem service and biodiversity changes over 70 years in a rural English county. J. Appl. Ecol. 50, 841–50. doi: 10.1111/1365-2664.12093
Jopke, C., Kreyling, J., Maes, J., and Koellner, T. (2015). Interactions among ecosystem services across Europe: Bagplots and cumulative correlation coefficients reveal synergies, trade-offs, and regional patterns. Ecol. Indic. 49, 46–52. doi: 10.1016/j.ecolind.2014.09.037
Jordan, N., and Warner, K. D. (2010). Enhancing the multifunctionality of US agriculture. BioScience 60, 60–66. doi: 10.1525/bio.2010.60.1.10
Khaledi, M., Weseen, S., Sawyer, E., Ferguson, S., and Gray, R. (2010). Factors influencing partial and complete adoption of organic farming practices in Saskatchewan, Canada. Can. J. Agric. Econ. Rev. Can. D'agroecon. 58, 37–56. doi: 10.1111/j.1744-7976.2009.01172.x
Koschke, L., Fürst, C., Lorenz, M., Witt, A., Frank, S., and Makeschin, F. (2013). The integration of crop rotation and tillage practices in the assessment of ecosystem services provision at the regional scale. Ecol. Indicat. 32, 157–171. doi: 10.1016/j.ecolind.2013.03.008
Lautenbach, S., Kugel, C., Lausch, A., and Seppelt, R. (2011). Analysis of historic changes in regional ecosystem service provisioning using land use data. Ecol. Indic. 11, 676–687. doi: 10.1016/j.ecolind.2010.09.007
Lee, H., and Lautenbach, S. (2016). A quantitative review of relationships between ecosystem services. Ecol. Indicat. 66, 340–351. doi: 10.1016/j.ecolind.2016.02.004
Leemans, R., and Solomon, A. M. (1993). Modeling the potential change in yield and distribution of the earth's crops under a warmed climate. Clim. Res. 3, 79–86. doi: 10.3354/cr003079
Legendre, P., De Cáceres, M., and Borcard, D. (2010). Community surveys through space and time: testing the space–time interaction in the absence of replication. Ecology 91, 262–272. doi: 10.1890/09-0199.1
Marshall, I. B., Schut, P. H., and Ballard, M. (1999). A National Ecological Framework for Canada: Attribute Data. Ottawa/Hull, QC: Government of Canada. Available online at: http://sis.agr.gc.ca/cansis/nsdb/ecostrat/1999report/index.html (accessed Febraury 28, 2020).
Mastrángelo, M. E., Pérez-Harguindeguy, N., Enrico, L., Bennett, E. M., Lavorel, S., Cumming, G. S., et al. (2019). Key knowledge gaps to achieve global sustainability goals. Nat. Sustain. 2, 1115–1121. doi: 10.1038/s41893-019-0412-1
McConkey, B. (2003). Crop rotation and tillage impact on carbon sequestration in Canadian prairie soils. Soil Till. Res. 74, 81–90. doi: 10.1016/S0167-1987(03)00121-1
Meehan, T. D., Gratton, C., Dieh, E., Hunt, N. D., Mooney, D. F., Ventura, S. J., et al. (2013). Ecosystem-service tradeoffs associated with switching from annual to perennial energy crops in riparian zones of the US Midwest. PLoS ONE 8:e80093. doi: 10.1371/journal.pone.0080093
Mitchell, M. G. E., Suarez-Castro, A. F., Martinez-Harms, M., Maron, M., McAlpine, C., Gaston, K. J., et al. (2015). Reframing landscape fragmentation's effects on ecosystem services. Trends Ecol. Evol. 30:190–8. doi: 10.1016/j.tree.2015.01.011
Moran, P. A. P. (1950). Notes on continuous stochastic phenomena. Biometrika 37:17. doi: 10.1093/biomet/37.1-2.17
Newlands, N. K., Zamar, D. S., McConkey, B., Townley-Smith, L., and Clark, O. G. (2012). “An integrated assessment model for exploring potential impacts of global change scenarios on the canadian agricultural system,” in 46th Hawaii International Conference on System Sciences (Maui, HI: IEEE), 915–924. doi: 10.1109/HICSS.2013.88
Nicholson, E., Mace, G. M., Armsworth, P. R., Atkinson, G., Buckle, S., Clements, T., et al. (2009). Priority research areas for ecosystem services in a changing world. J. Appl. Ecol. 46, 1139–1144. doi: 10.1111/j.1365-2664.2009.01716.x
Palm, C., Blanco-Canqui, H., Declerck, F., Gatere, L., and Grace, P. (2014). Agriculture, ecosystems and environment. Agric. Ecosyst. Environ. 187, 87–105. doi: 10.1016/j.agee.2013.10.010
Parton, W. J. (1996). “The CENTURY model,” in Evaluation of Soil Organic Matter Models, eds D. S. Powlson, P. Smith, and J. U. Smith (Berlin, Heidelberg: Springer), 283–291.
Phalan, B., Onial, M., Balmford, A., and Green, R. E. (2011). Reconciling food production and biodiversity conservation: land sharing and land sparing compared. Science 333, 1289–1291. doi: 10.1126/science.1208742
Power, A. G. G. (2010). Ecosystem services and agriculture: tradeoffs and synergies. Philos. T. R. Soc. B 365, 2959–2971. doi: 10.1098/rstb.2010.0143
Qiu, J., and Turner, M. G. (2013). Spatial interactions among ecosystem services in an urbanizing agricultural watershed. Proc. Natl. Acad. Sci. U.S.A. 110, 12149–12154. doi: 10.1073/pnas.1310539110
Ramankutty, N., Foley, J. A., Norman, J., and McSweeney, K. (2002). The global distribution of cultivable lands: current patterns and sensitivity to possible climate change. Global Ecol. Biogeogr. 11, 377–392. doi: 10.1046/j.1466-822x.2002.00294.x
Raudsepp-Hearne, C., Peterson, G. D., and Bennett, E. M. (2010). Ecosystem service bundles for analyzing tradeoffs in diverse landscapes. Proc. Natl. Acad. Sci. U.S.A. 107, 5242–5247. doi: 10.1073/pnas.0907284107
Renard, D., Rhemtulla, J. M., and Bennett, E. M. (2015). Historical dynamics in ecosystem service bundles. Proc. Natl. Acad. Sci. U.S.A. 112, 13411–13416. doi: 10.1073/pnas.1502565112
Robertson, G. P., and Swinton, S. M. (2005). Reconciling agricultural productivity and environmental integrity: a grand challenge for agriculture. Front. Ecol. Environ. 3, 38–46. doi: 10.1890/1540-9295(2005)003[0038:RAPAEI]2.0.CO;2
Spake, R., Lasseur, R., Crouzat, E., Bullock, J. M., Lavorel, S., Parks, K. E., et al. (2017). Unpacking ecosystem service bundles_ Towards predictive mapping of synergies and trade-offs between ecosystem services. Global Environ. Chang. 47, 37–50. doi: 10.1016/j.gloenvcha.2017.08.004
Statistics Canada (2018). Field Crop Reporting Series. Available online at: https://www.statcan.gc.ca/eng/dai/btd/fcrs (accessed September 30, 2020).
Statistics Canada (2019). 2016 Census of Agriculture. Available online at: https://www.statcan.gc.ca/eng/ca2016 (accessed September 30, 2020).
Statistics Canada. (2008). Selected Historical Data from the Census of Agriculture - Tables 95-632-X. Available online at: https://www150.statcan.gc.ca/n1/en/catalogue/95-632-X
Sutherland, I. J., Bennett, E. M., and Gergel, S. E. (2016). Recovery trends for multiple ecosystem services reveal non-linear responses and long-term tradeoffs from temperate forest harvesting. For. Ecol. Manage. 374, 61–70. doi: 10.1016/j.foreco.2016.04.037
Syswerda, S. P., and Robertson, G. P. (2014). Ecosystem services along a management gradient in Michigan (USA) cropping systems. Agric. Ecosyst. Environ. 189, 28–35. doi: 10.1016/j.agee.2014.03.006
Teklewold, H., Kassie, M., and Shiferaw, B. (2013). Adoption of multiple sustainable agricultural practices in rural Ethiopia. J. Agric. Econ. 64:597–623. doi: 10.1111/1477-9552.12011
Tilman, D., Cassman, K. G., Matson, P. A., Naylor, R., and Polasky, S. (2002). Agricultural sustainability and intensive production practices. Nature 418, 671–677. doi: 10.1038/nature01014
van Bochove, E., Denault, J.-T., Leclerc, M.-L., Theriault, G., Dechmi, F., Allaire, S. E., et al. (2011). Temporal trends of risk of water contamination by phosphorus from agricultural land in the Great Lakes Watersheds of Canada. Can. J. Soil Sci. 91, 443–453. doi: 10.4141/cjss09122
van Wesemael, B., Paustian, K., Meersmans, J., Goidts, E., Barancikova, G., and Easter, M. (2010). Agricultural management explains historic changes in regional soil carbon stocks. Proc. Natl. Acad. Sci. U.S.A. 107:14926–14930. doi: 10.1073/pnas.1002592107
Vellend, M., Brown, C. D., Kharouba, H. M., McCune, J. L., and Smith, I. H. M. (2013). Historical ecology: Using unconventional data sources to test for effects of global environmental change. Am. J. Bot. 100, 1294–1305. doi: 10.3732/ajb.1200503
Yang, J. Y., De Jong, R., Drury, C. F., Huffman, E. C., Kirkwood, V., and Yang, X. M. (2007). Development of a Canadian Agricultural Nitrogen Budget (CANB v2.0) model and the evaluation of various policy scenarios. Can. J. Soil Sci. 87, 153–165. doi: 10.4141/S06-063
Keywords: ecosystem services, Canada, temporal correlations, ecosystem service bundles, agricultural landscapes, soil quality regulation, water quality regulation, air quality regulation
Citation: Mitchell MGE, Chan KMA, Newlands NK and Ramankutty N (2020) Spatial Correlations Don't Predict Changes in Agricultural Ecosystem Services: A Canada-Wide Case Study. Front. Sustain. Food Syst. 4:539892. doi: 10.3389/fsufs.2020.539892
Received: 02 March 2020; Accepted: 26 October 2020;
Published: 27 November 2020.
Edited by:
Lisa Schulte Moore, Iowa State University, United StatesReviewed by:
Fei Wang, Chinese Academy of Sciences, ChinaCopyright © 2020 Mitchell, Chan, Ramankutty, and Her Majesty the Queen in Right of Canada, as represented by the Minister of Agriculture and Agri-Food Canada. This is an open-access article distributed under the terms of the Creative Commons Attribution License (CC BY). The use, distribution or reproduction in other forums is permitted, provided the original author(s) and the copyright owner(s) are credited and that the original publication in this journal is cited, in accordance with accepted academic practice. No use, distribution or reproduction is permitted which does not comply with these terms.
*Correspondence: Matthew G. E. Mitchell, bWF0dGhldy5taXRjaGVsbEB1YmMuY2E=
Disclaimer: All claims expressed in this article are solely those of the authors and do not necessarily represent those of their affiliated organizations, or those of the publisher, the editors and the reviewers. Any product that may be evaluated in this article or claim that may be made by its manufacturer is not guaranteed or endorsed by the publisher.
Research integrity at Frontiers
Learn more about the work of our research integrity team to safeguard the quality of each article we publish.