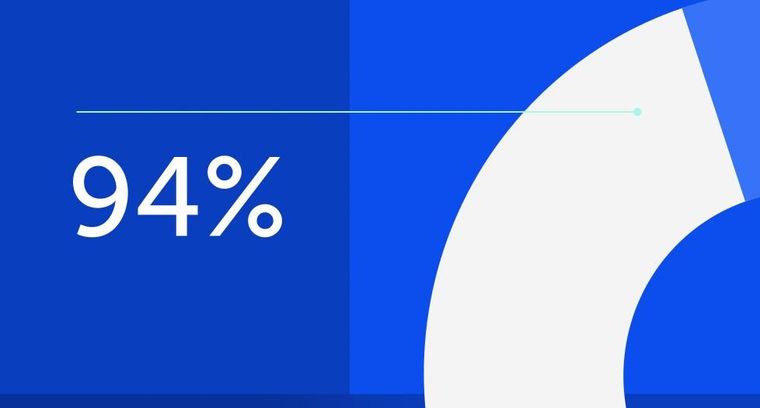
94% of researchers rate our articles as excellent or good
Learn more about the work of our research integrity team to safeguard the quality of each article we publish.
Find out more
ORIGINAL RESEARCH article
Front. Sustain. Food Syst., 12 June 2020
Sec. Agroecology and Ecosystem Services
Volume 4 - 2020 | https://doi.org/10.3389/fsufs.2020.00079
This article is part of the Research TopicCurrent Status and Trends in Urban AgricultureView all 10 articles
Urban agriculture offers the opportunity to provide fresh, local food to urban communities. However, urban agriculture can only be successfully embedded in urban areas if consumers perceive urban farming positively and accept urban farms in their community. Success of urban agriculture is rooted in positive perception of those living close by, and the perception strongly affects acceptance of farming within individuals' direct proximity. This research investigates perception and acceptance of urban agriculture through a qualitative, exploratory field study with N = 19 residents from a major metropolitan area in the southwest U.S. Specifically, in this exploratory research we implement the method of concept mapping testing its use in the field of Agroecology and Ecosystem Services. In the concept mapping procedure, respondents are free to write down all the associations that come to mind when presented with a stimulus, such as, “urban farming.” When applying concept mapping, participants are asked to recall associations and then directly link them to each other displaying their knowledge structure, i.e., perception. Data were analyzed using content analysis and semantic network analysis. Consumers' perception of urban farming is related to the following categories: environment, society, economy, and food and attributes. The number of positive associations is much higher than the number of negative associations signaling that consumers would be likely to accept farming close to where they live. Furthermore, our findings show that individuals' perceptions can differ greatly in terms of what they associate with urban farming and how they evaluate it. While some only think of a few things, others have well-developed knowledge structures. Overall, investigating consumers' perception helps designing strategies for the successful adoption of urban farming.
At present, the number of people living in urban areas worldwide is over three billion, or 55% of the world population, and it is projected that 68% of the world's population will be living in urban areas by 2050 (United Nations, 2018). In the United States alone, 82% of the population currently lives in urban areas (World Bank, 2016). The continued expansion of cities nationwide places a heavy toll on the demand for resources, such as sustainable infrastructure and affordable food retail options, to meet the basic needs of households living within city limits. Within the food sector, the accelerating rate of migration into cities coupled with a growing population imposes the challenge of producing sufficient quantities of food (Satterthwaite et al., 2010). This challenge needs to be addressed to ensure everyone has access to high-quality, nutrient-dense food. Simultaneously, it raises the question of how to provide satisfactory nourishment while consumers are increasingly asking for fresh and local foods (Grebitus et al., 2017).
With urbanization on the rise, one solution to this challenge is the development and expansion of urban agriculture1. Figure 1 below shows the replacement of agricultural areas (yellow) by urban areas (red) in the Phoenix Metropolitan Area. Urban agriculture is a growing sector within the farming industry that aims to increase overall food production in urban and peri-urban areas through the conversion of available land into agricultural farms. As reported in Smith et al. (2017), there are 67,032 vacant parcels (19,592 hectares) potentially suitable for urban agriculture in the Phoenix Metropolitan Area.
Figure 1. Land use map showing the replacement of agricultural areas (yellow) by urban areas (red). Data from the 2006 and 2016 USGS National Land Cover Dataset.
Cities across the United States have already begun to integrate food production, such as commercial urban farms and private or community gardens, into communities (Hughes and Boys, 2015; Printezis and Grebitus, 2018). To predict whether urban farming will be successful and to influence its longevity, it is important to understand consumer perception (Grebitus and Bruhn, 2008). Hence, the objective of this research is to investigate how consumers perceive urban farming and to evaluate whether they would accept this form of commercial agriculture close to their residence.
Food produced in urban and peri-urban communities has various implications. For example, for small- to mid-size farmers, the profitability of urban farmers can be dependent on producing local foods that can be (exclusively) sold through direct channels, such as farmers markets. Urban agriculture also has an effect on societal health. Direct access to local produce through direct-to-consumer marketing channels affects the dietary quality and diversity of food choices of urban consumers. Unlike large agricultural production facilities that occupy 75% of the land in the U.S. and predominantly grow commodity crops used for animal feed, biofuels, and industrial inputs (DeHaan, 2015), outputs from urban agricultural production are largely specialty crops, which require comparatively minimal processing before consumption. Specialty crops, which include most fruits, vegetables, and tree nuts, are rich in nutrients, vitamins, and minerals and are constituents of an optimal diet (WHO, 2018). In this way, both the increased consumption of fruits and vegetables along with the diversity of produce consumed is closely linked with positive human health outcomes and serves as a measure of societal health. Finally, urban agriculture affects environmental quality through changes in urban-vegetation-atmosphere interactions, e.g., the reduction in food miles and the mitigation effects of urban heat islands, as a result of urban agriculture practices. Overall, urban agriculture has the potential to provide a number of benefits, for instance, improving sustainability, and local ecology (Wakefield et al., 2007), assisting with food security (Dimitri et al., 2016; Freedman et al., 2016; Sadler, 2016), and contributing to healthy dietary patterns (Zezza and Tasciotti, 2010; Warren et al., 2015).
Alternatively, urban agriculture may produce negative externalities (Brown and Jameton, 2000; Wortman and Lovell, 2013). For example, a farmer growing food in a city might encounter pushback by the people living next to the farm who might be bothered by dirt and noise from machinery, odors from organic fertilizers, or they might be afraid that pesticides and fertilizers are polluting the air they breathe and the water they drink. A recent study by Wielemaker et al. (2019) showed urban farmers apply fertilizers in excess of crop needs by 450–600%, potentially leading to negative public perceptions. At the same time, urban farms might be preferred due to access to fresh, local, nutrient-dense food which enhance positive perceptions. This suggests that consumers' perception and acceptance of urban farms is vital to ensure that urban agriculture can be successful (Grebitus et al., 2017).
Previous empirical research on urban agriculture has focused on investigating the relationship between urban agriculture and nutrition (variety, food security, and nutrition status), with a particular emphasis on its role in developing countries [see Warren et al. (2015) for a broad review of previous studies]. Mougeot (2005) compiles case studies of development strategies used by developing countries and pays specific attention to the potential that urban agriculture has in meeting development goals (e.g., increased food availability, decreased poverty, increased health status) in each respective country. Studies focused on developed countries highlight the social context of urban agriculture. They assess how community gardens affect communities (Armstrong, 2000; Wakefield et al., 2007; Firth et al., 2011), analyze what urban farmers need when only limited resources are available (Surls et al., 2015), and examine success factors of urban agriculture, such as positive consumer attitudes and increased knowledge regarding local food production (Grebitus et al., 2017).
Recently, Grebitus et al. (2017) found in a quantitative online consumer survey that consumers perceive urban agriculture positively based on food quality characteristics, such as food safety and health. More generally, related to perception, they find the three sustainability pillars (economy, society, and environment) are important with regards to consumer perception. Nevertheless, the authors state that consumers' perception is sometimes conflicting. For example, some consumers perceive produce from urban farms as less expensive while others perceive it as more expensive. Our research builds on the study by Grebitus et al. (2017) by investigating the in-depth perception of urban farming using qualitative, exploratory methods in a face-to-face study. While Grebitus et al. (2017) used a word association test, we employ the method of concept mapping. Concept maps can uncover cognitive structures related to urban farming and show differences between individuals regarding their knowledge structures.
The implications of our findings will offer several insights to those charged with designing and implementing food and agricultural policy. Such policies have the potential to affect new and emerging trends in urban communities, stimulate the growth of direct-to-consumer marketing channels where small- to mid-size farmers sell their products and address the effects of urban agriculture on the environment. Our results will provide insight into how urban farming is perceived by individuals to ensure that incorporating farms in urban areas is accepted by those living there. For example, if our analysis shows that consumers are apprehensive and afraid, e.g., of pesticides or fertilizer run-off, targeted communication can be used to alleviate such tensions.
In the following section, the methodological background is described covering concept mapping, counting, and content analysis. Section three presents the results and section four concludes.
In consumer behavior research, perception is defined as subjective and selective information processing (Kroeber-Riel et al., 2009). Whether something is positively or negatively perceived by consumers is determined by cognitive structures, i.e., semantic networks, which capture a part of the knowledge (associations/concepts) in memory (Martin, 1985; Joiner, 1998). A semantic network is composed of nodes, which represent concepts and units of information, and links, connecting the concepts, which represent the type and the strength of the association between the concepts (Cowley and Mitchell, 2003). To investigate perception toward urban farming we aim to provide insight into consumers' individual cognitive structures, i.e., semantic networks (Kanwar et al., 1981; Jonassen et al., 1993).
Associative elicitation techniques are appropriate to analyze semantic networks (Bonato, 1990). By presenting stimuli, spontaneous reactions and unconscious thoughts are evoked and enable us to analyze individual cognitive structures (Grebitus and Bruhn, 2008). A great variety of associative elicitation techniques exists, ranging from the most qualitative techniques like word association technique (Roininen et al., 2006; Ares et al., 2008) to more structured techniques such as repertory grid (Sampson, 1972; Russell and Cox, 2004) or laddering (Grunert and Bech-Larsen, 2005).
For this study, the qualitative graphing procedure concept mapping was chosen. Concept mapping is a method that produces a schematic representation of the relationships of stored units of information, which are activated by the stimulus (Zsambok, 1993). The interviewees are asked to recall freely their associations concerning a certain stimulus (Olson and Muderrisoglu, 1979). Additionally, they are asked to directly link the associations to each other, which allows the visualization of the semantic networks (Bonato, 1990). The open setting of tasks optimizes the variety of associations of the interviewees (Joiner, 1998). Concept map diagrams are two-dimensional and show relationships between units of information concerning a certain theme. The concepts are understood as terms, i.e., associations, which come to mind regarding the stimulus (Jonassen et al., 1993).
Concept mapping is supported by semantic network theory and can be explained using the spreading activation network model (Rye and Rubba, 1998). Retrieving stored knowledge can be explained by the spreading activation (Collins and Loftus, 1975; Anderson, 1983a,b). When consumers perceive/associate something with a stimulus, information-processing takes place and cognitive structures are activated for interpretation, assessment, and decision-making. The stored knowledge is retrieved by spreading activation from associations (Anderson, 1983b). In this context, existing networks are active cognitive units that can, once activated, influence behavior directly (Olson, 1978). How much and what information is integrated into the information-processing depends on the construction of the semantic network (Cowley and Mitchell, 2003).
The spread of activation constantly expands through the links to all connected nodes (associations) in the network, starting with the first activated concept. At first, it expands to all the nodes directly linked to the first node, and then to all the nodes linked to each of those nodes. This way, the activation is spreading through all nodes of the network, even through those nodes that are only indirectly associated with the “stimulus node” (Collins and Loftus, 1975). The stronger the link between two nodes, the easier and faster the activation passes to the connected nodes (Cowley and Mitchell, 2003). How far the activation spreads also depends on the distance from the stimulus node. Concepts that are closely related and directly linked will be activated faster and with higher intensity (Henderson et al., 1998). See Figure 2 for an illustration of nodes and links in a semantic network.
The concept mapping technique elicits respondents to recall knowledge from long-term memory and to write down what they know, which stimulates the spread of activation in memory (Rye and Rubba, 1998). The more linkages a semantic network contains, the higher is the dimensionality and complexity of cognitive structures. The higher the dimensionality of the cognitive structures, the larger the number of concepts that can be activated and the more differentiated and complex the networks (Kanwar et al., 1981). Depending on personal relevance and involvement, consumers' semantic networks are more or less extensively structured (Peter and Olson, 2008).
To conduct the concept mapping procedure, we adapted the instructions used by Grebitus (2008). Respondents received an instructions page. At the top of the page, the respondents read the following passage:
Researchers believe that our knowledge is stored in memory. The knowledge we have can be described through central concepts and the relationship between them. These concepts depict our belief of different knowledge domains such as food or vacation. These beliefs can also be related to each other. For example, when you think of a car, you may spontaneously think of “tires”, “white”, or “traffic”. If you then think further, “gas” and “expensive” may come to mind. These can also be related to each other and thus are indirectly related with a car. People have a lot of such associations. To find out yours is one objective of this study.
Respondents were then given a blank piece of paper and started by writing the term “Urban Farming” in the center of the paper. They were then instructed to start thinking of anything that comes to mind, related to the key concept and write it down. After writing down the concepts, the interviewees had to construct the concept map by connecting all the words that they believe, in their minds, are related to each other and belong to each other (i.e., drawing links). Then, they had to add a plus or minus to associations they thought to be positive or negative.
To investigate how many associations and what kind of information is stored in memory concerning urban farming, the items were counted and aggregated (Kanwar et al., 1981; Martin, 1985; Grebitus, 2008). Next, the individual associations were evaluated using qualitative content analysis following Mayring (2002). This allowed us to make assumptions, and investigate intent and motivation regarding the topic in a formal way (Stempel, 1981; Hsia, 1988). Content analysis is an objective and systematic way to apply quantitative measures to qualitative data (Stempel, 1981; Wimmer and Dominick, 1983; Hsia, 1988, p. 320).
The aim of this study is to provide meaning to the participants' associations. Hence, we classified the content according to categories. This offers a framework to assess the perception of urban farming. The associations written down by the respondents in the concept maps regarding the key stimulus, urban farming, were organized and categorized, then they were added up into frequencies (Bonato, 1990; Lamnek, 1995). The categories are the core of the perception analysis. They are used to investigate the topic further (Wimmer and Dominick, 1983). Therefore, the categories should be closely related to the research topic. They have to be practical, reliable, comprehensive (each word fits into one of the categories) and mutually exclusive (each word fits only one category) (Stempel, 1981; Wimmer and Dominick, 1983). In this research, we used the categories provided by Grebitus et al. (2017) who used a word association test for the key concept: urban agriculture, a close proxy for the one used in our study “urban farming.” Accordingly, we used the three sustainability pillars Economy, Society, and Environment, as well as, Food and Attributes, and Others as categories to group the data for urban farming in a meaningful way.
To investigate consumer perception of urban farming, exploratory, face-to-face interviews were conducted. The qualitative graphing procedure concept mapping was used to reveal consumers' associations regarding urban farming. In addition to concept mapping, participants filled out a survey to collect socio-demographic information. For detailed information on the data collected, refer to Table S1 in the Supplementary Material.
We collected data in Phoenix, AZ. We chose this location because the Phoenix metropolitan area is ideal for a case study as it is home to a large and growing urban population. Phoenix provides context that has many similar natural and social complexities and barriers (e.g., climate challenges, a lack of food access, rapidly growing, diverse, multi-cultural population), with a large variance in educational and economic levels of residents compared to other urban areas in the U.S. The Phoenix metropolitan area (i.e., Maricopa and Pinal Counties) is the eleventh largest metro area in the U.S. with Maricopa County identified as the fastest-growing county in the U.S. (U.S. Census Bureau, 2019). This rapid population growth demonstrates an important need for sustainable urban farming practices, given the benefits of food security, economic stability, and environmental conservation. Phoenix has a climate where food can be grown all year round, with multiple growing seasons. The extended growing season allows harvest year-round and may affect consumer purchasing patterns and related dietary quality differently than when food is grown only during certain seasons. Meanwhile, Phoenix experiences unique climatic extremes: from being an urban heat island, experiencing short and long-term drought, while simultaneously dealing with seasonal monsoons that can bring rapid and devastating flooding. Hence, urban farming might have different environmental impacts compared to cities where this is not the case. Also, within urban planning and development, Phoenix has begun to recognize urban agriculture as an attractive fixture in revitalizing communities, especially since urban expansion has replaced nearby agriculture at a large rate (Shrestha et al., 2012). Also, Phoenix has vacant land available that can potentially be used for urban farming (Aragon et al., 2019).
We interviewed a total of 19 participants in the summer of 2019 at two locations. A total of 14 participants were interviewed at a large public farmers' market. Another five participants were interviewed at a second location near an open green space2. All interviews were carried out by one interviewer. The sample is a convenience sample. Participants were reimbursed for their time with $10 each.
In terms of sample characteristics, 47% of the sample were female, the average age was 38 years old. Household size was on average three persons in the household, with 26% having children in the household. 21% were graduate students and 21% were undergraduate students. In terms of the level of education, 26% had some college education, 32% a Bachelor's degree, and 42% a graduate degree.
This paper aims to analyze consumers' perception of urban farming. This objective is based on the notion that for urban farming to be more fully and successfully integrated into urban and peri-urban communities, consumers need to perceive it positively.
Table 1 depicts the descriptive findings for the counting of the concepts of the two groups and the total over both samples. The results show a total of 333 associations were written down when considering all participants. The mean is 17.5 concepts with a standard deviation of 13.5. The lowest number of concepts associated with urban farming is eight, the highest 68. The farmers' market sample had a higher mean (18.4) than the second location (M = 15). The standard deviation, however, was considerably smaller at the second location (SD = 5.1) compared to the farmers' market sample (SD = 15.5).
Among the 333 concepts were single terms (e.g., community, convenience, microclimate) and whole phrases [e.g., “Creates ‘villages' (people work together)”]. Following Grebitus et al. (2017), the concepts were grouped into five categories: Economy, Society, Environment, Food and Attributes, and Other shown in Table 2. Note, Grebitus et al. (2017) had a sixth category, Point of Sale, but this did not apply to our data. Findings show that participants primarily think of environment-related associations (36%) followed by specific foods and attributes associated with urban farming (25%), and society (20%). The category economy ranks fourth with 11%.
Table 3 shows the associations that were organized in the categories. To reduce the large number of associations, concepts were merged based on similarity using content analysis. For example, “community,” “community centered,” and “community experience” were aggregated up to “community” (see the complete list of associations in Appendix A included in the Supplementary Material). The strongest category, “environment” is dominated by associations related to production (33% of category associations) and conservation (14% of category associations), as well as agriculture (8% of category associations) and waste (8% of category associations). “Sustainability,” “environment,” “beautification,” and “pollution” are also included in this category. The category “food and attributes,” is dominated by associations related to health (14% of category associations) and fresh (11% of category associations), as well as convenience (10% of category associations) and food security (10% of category associations). “Local,” “plant,” and “produce” are also mentioned, as well as, “location” and “organic.” The category “society” is dominated by associations related to community building (39% of category associations), education (27% of category associations), family (9% of category associations) and municipality (8%). “Advocacy,” “research,” “migration trends,” and “youth” also fit this category. The category “economy” is dominated by associations related to cost (35% of category associations) and labor (16% of category associations), as well as economics (14% of category associations) and externalities (14% of category associations). “Policy,” “benefits,” and “vocation” are the remaining associations in this category. The category “other” entails associations, such as “positive feelings” and “gratitude,” that did not fit in the other established categories. Out of all associations, community and sustainability are among those associated most with urban farms. The result that these two concepts are the most prevalent among our responses suggests the importance of environmentally sustainable farms in urban communities.
Overall, findings show that consumers mainly associate production and environmentally related concepts with urban farming. Many food attribute associations can be considered as generally positive, such as “fresh,” “healthy,” “convenient,” “organic,” and “local.” Participants also associate sustainability and conservation with urban farming. They think of social aspects, such as “flourishing neighborhood,” “friend development,” and “meet other gardeners,” when asked about urban farming. Furthermore, urban farming evokes thoughts of “the economy,” “saving money,” “reducing grocery cost,” and “cost effectiveness.” In this regard, we find some differing opinions with some participants believing that they can save money while others consider urban farming is expensive. This is an indicator that urban farming most likely will not be perceived positively by everyone. Some citizens will be in favor of urban farming and others not. This could be resolved using educational measures given that previous studies have shown that individuals do not feel very knowledgeable with regards to urban agriculture (Grebitus et al., 2017).
To get a better understanding of consumer acceptance of urban farming and whether they perceive urban farming as predominantly positive or negative, they were asked to indicate with a plus (+) those associations they think are positive, and with a minus (–) those they consider to be negative. Table 4 summarizes the number of positive and negative evaluations that were given. Appendix A provides a complete list of all associations including the evaluations. As shown in Table 4, urban farming is mainly perceived as positive. Seventy-three percent (73%) of all associations are evaluated positively while only 15% are evaluated as negative. Less than two percent (1.5%) of the associations fall in the category where individuals felt it could go either way. Except for ID 7, all participants that evaluated their associations have a larger share of positively perceived characteristics. ID 7 has 20% positive and 80% negative associations. Only a small share of associations was left unevaluated. Examples of positive associations are “community,” “environment,” “fresh,” “local,” “green,” “farmer's market,” “healthy,” “organic,” and “sustainability.” Meanwhile, “cost,” “expensive,” “pollution,” “smell,” “possible bacteria,” “disease,” and “pesticides” are examples of negative associations.
After considering what associations are stored in memory regarding urban farming, this section aims to give insight into how the information is stored and what relationships exist between the stated concepts described in the section Perception of urban farming: Results from content analysis. In this regard, figures 3 through 7 show five different concept maps as examples of semantic networks from five different participants, illustrated by the use of the software UCInet (Borgatti et al., 2002). The concept maps differ in shape and complexity.
Figure 3 is a star-shaped semantic network (Wasserman and Faust, 1994). Based on the spreading activation network theory, this pattern means that when “urban farming” is activated, i.e., the individual thinks about it all related associations will be activated and included in thoughts, evaluations and decision making. In this case, sustainability, jobs, information, livestock, possible pesticides, aesthetic and food. These associations can then lead to further associations if the activation is strong enough. For example, possible pesticides can lead to thoughts about runoff in public areas.
Figure 4 depicts a graph that contains three cycles but is also mainly in a star-shaped composition (Wasserman and Faust, 1994). Here, urban farming is seen as family-oriented, providing fresh food with less pollution and less space, e.g., when using hydroponics.
Figure 5 depicts a graph in a tree-shaped composition (Wasserman and Faust, 1994). In this case, more activation is needed to reach associations that are further away from the key stimulus. For example, self-sufficient adults might not be activated, and hence not be included in decisions unless the activation is strong. That said this individual has a semantic network that is more developed in terms of linking associations further. For example, the individual thinks that urban farming is a community experience that can lead to youth interaction, which then should ultimately lead to self-sufficient adults.
Figure 6 displays a more complex semantic network as displayed by the larger number of associations that are more connected to each other. This individual thinks urban farming can save money, land, and resources in general. The individual also associates organic and easy access, i.e., convenience with urban farming. Community is linked to urban farming and then has links to togetherness and beneficial. Togetherness, in turn, is linked to family and neighbors which are both connected to understanding. This suggests that urban farming could play a role in the communication of people living together, the family and the neighbors.
Figure 7 displays the most complex semantic network of the participants with over 60 associations. In this case, a lot of activation would be needed so that the individual would access all stored information regarding urban farming. For example, between intermittent fasting and urban farming, six other associations need to be activated and processed before intermittent fasting is accessed. This individual points out less favorable associations, such as “neighbor complaints,” which are related to “smell” and “noise.” Overall, this concept map is highly differentiated and complex.
These examples are by no means exhaustive. There is a wide variety of different network structures among the 19 individuals. However, there are few visual differences observed between the concept maps of the two groups in terms of shapes and structures. Each group varies in complexity. Some participants have complex cognitive structures using a great number of associations, while others hold simple cognitive structures, i.e., semantic networks, which can be explained by the use of key information. In this case, urban farming is related to several key associations, so that the activation of a lower amount of stored information is sufficient for its perception. The rather simple network structures can also result from low familiarity with urban farming or a potential lack of interest by some individuals.
Urban agriculture offers a promising opportunity to provide direct access to fresh produce close to urban residents. This may enhance dietary quality and food diversity while addressing consumers' preference for local food. However, urban agriculture will only be successful if it is accepted and perceived positively by those living in close proximity. Therefore, one must account for consumer perception. Hence, our research provides an exploratory analysis of consumer perception regarding urban farming catering to the success of urban agriculture.
To better evaluate consumers' perception, we employ the method of concept mapping in an exploratory and qualitative study of 19 participants from the Phoenix Metropolitan Area. This analysis provided 333 associations with urban farming. Using content analysis, five categories—Environment, Food and Attributes, Society, Economy and Other—were distinguished to group the concepts/associations in a meaningful way. Participants offered a great variety of perceptions, such as organic, local, community, family, agriculture, and sustainability. One of the overarching themes that emerged from our study was the myriad positive perceptions, e.g., fresh, local, and green. Though negative associations exist, e.g., expensive, possible disease, and pollution, these were fewer in comparison. From a marketing standpoint, highlighting those positive aspects of urban agriculture could incite a more favorable perception and willingness to accept urban agriculture. This could also present opportunities for cities to offer incentives to households who do perceive urban farming negatively. The negative associations also deserve further research as they have the potential to deter the further development of urban agriculture.
In terms of individual semantic networks concerning urban farming, we found that there are vast differences regarding how many associations individuals hold and how connected the associations are. Generally, the more associations and the more links in a network the greater the expertise and involvement. Investigating this more deeply could be used to infer educational strategies.
The use of concept mapping offers detailed insight into participants' semantic networks. It serves as an important, theoretically motivated tool to demonstrate what individuals think and how different concepts are related to each other. Individuals' evaluations of positive and negative associations enables the researcher to determine if the researched area (e.g., urban farming) is perceived favorably or not. That said, knowledge structures are complex, and, with increasing sample sizes, analysis on topics that induce many associations – both positive and negative – can quickly become computationally intensive.
This research is not without limitations. While our findings are encouraging toward acceptance of farming in the city, it should be kept in mind that this is an exploratory study. The present study analyzes stored information, i.e., semantic networks regarding urban farming using qualitative methods for a small sample size from only two study locations, so the results might be dependent on the study area. A more robust approach would be sampling from different regions in the U.S. Future research should include a larger number of participants and expand to more study sites. In doing so, recommendations to stakeholders can be made for the successful integration of sustainable urban agriculture. Garnering an understanding of regional perceptions is of importance, as minimizing the length of the supply chain is associated with a number of benefits, especially in resource-limited environments like the Southwest, and improved well-being at the individual level. Future research could examine the multi-scalar dynamics of urban agriculture, shedding light on market opportunities for agricultural producers and regulators, while simultaneously identifying those factors that could lead to market rejection, e.g., consumer reactance, or practices that may reduce the long-term environmental sustainability of the urban farm. Ultimately, there is a need for interdisciplinary research, for instance, between social scientists, economists, and agroecologists to provide insight into different perspectives that underscore the future success and adoption of urban agriculture.
All datasets generated for this study are included in the article/Supplementary Material.
The studies involving human participants were reviewed and approved by Arizona State University IRB, Study Number STUDY00010463. Written informed consent for participation was not required for this study in accordance with the national legislation and the institutional requirements.
CG contributed to conception and design of the study, organized the database, performed the analysis, and wrote the first draft of the manuscript. LC served as secondary writer of the manuscript and contributed to study design. LC and RM contributed to initial discussions of methods and the review of the concept categorization. RM reviewed and revised the draft for important intellectual content and created Figure 1. AM contributed to conception and design of the study. All authors contributed to manuscript revision, read, and approved the submitted version.
This work was supported by EASM-3: Collaborative Research: Physics-Based Predictive Modeling for Integrated Agricultural and Urban Applications, USDA-NIFA (Grant Number: 2015-67003-23508), NSF-MPS-DMS (Award Number: 1419593), and by the Swette Center for Sustainable Food Systems, Arizona State University.
The authors declare that the research was conducted in the absence of any commercial or financial relationships that could be construed as a potential conflict of interest.
The Supplementary Material for this article can be found online at: https://www.frontiersin.org/articles/10.3389/fsufs.2020.00079/full#supplementary-material
1. ^The FAO defines urban agriculture as “a dynamic concept that comprises a variety of livelihood systems ranging from subsistence production and processing at the household level to more commercialized agriculture. It takes place in different locations and under varying socioeconomic conditions and political regimes” (FAO, 2007, p. 5).
2. ^Note the relatively small sample size in this study. While this would be a drawback for a quantitative study targeting to be representative, our objective is to provide an exploratory study on the perception of urban farming. The aim is not to uncover the perception of the whole population. In that case, a method such as concept mapping would not be well-suited, rather one would use free elicitation technique. That said, free elicitation technique does not allow for a depiction of cognitive structures. This could be tackled by future research. In this research, we set out to conduct qualitative research. The sample size for qualitative studies often ranges from 5 to 50 participants, as pointed out by Dworkin (2012): “An extremely large number of articles, book chapters, and books recommend guidance and suggest anywhere from 5 to 50 participants as adequate.” Participant numbers are similarly small, for example in studies by Sonneville et al. (2009); Lachal et al. (2012); Bennett et al. (2013); Van Gilder and Abdi (2014); Takahashi et al. (2016); Hunold et al. (2017), and Mitter et al. (2019) ranging from 12 to 21.
Anderson, J. R. (1983a). Retrieval of information from long-term memory. Science 220, 25–30. doi: 10.1126/science.6828877
Anderson, J. R. (1983b). A spreading activation theory of memory. J. Verb. Learn. Verb. Behav. 22, 261–295. doi: 10.1016/S0022-5371(83)90201-3
Aragon, N. U., Stuhlmacher, M., Smith, J. P., Clinton, N., and Georgescu, M. (2019). Urban agriculture's bounty: contributions to phoenix's sustainability goals. Environ. Res. Lett. 14:105001. doi: 10.1088/1748-9326/ab428f
Ares, G., Gámbaro, A., and Giménez, A. (2008). Understanding consumers' perception of conventional and functional yoghurts using word association and hard laddering. Food Qual. Prefer. 19, 636–643. doi: 10.1016/j.foodqual.2008.05.005
Armstrong, D. (2000). A survey of community gardens in upstate New York: implications for health promotion and community development. Health Place 6, 319–327. doi: 10.1016/S1353-8292(00)00013-7
Bennett, J., Greene, G., and Schwartz-Barcott, D. (2013). Perceptions of emotional eating behavior. A qualitative study of college students. Appetite 60, 187–192. doi: 10.1016/j.appet.2012.09.023
Bonato, M. (1990). Wissensstrukturierung mittels struktur-lege-techniken, eine graphentheoretische analyse von wissensnetzen, europäische hochschulschriften. Psychologie 6:297.
Borgatti, S. P., Everett, M. G., and Freeman, L. C. (2002). Ucinet for Windows: Software for Social Network Analysis. Harvard, MA: Analytic Technologies.
Brown, K. H., and Jameton, A. L. (2000). Public health implications of urban agriculture. J. Public Health Policy 21, 20–39. doi: 10.2307/3343472
Collins, A. M., and Loftus, E. (1975). A spreading-activation theory of semantic processing. Psychol. Rev. 82, 407–428. doi: 10.1037/0033-295X.82.6.407
Cowley, E., and Mitchell, A. (2003). The moderating effect of product knowledge on the learning and organization of product information. J. Consum. Res. 30, 443–454. doi: 10.1086/378620
DeHaan, L. R. (2015). “Perennial crops are a key to sustainably productive agriculture,” in Food Security: Production and Sustainability. Institute on Science for Global Policy, ISGP Academic Partnership with Eckerd College. Available online at: https://www.scienceforglobalpolicy.org (accessed May, 2020).
Dimitri, C., Oberholtzer, L., and Pressman, A. (2016). Urban agriculture: connecting producers with consumers. Br. Food J. 118, 603–617. doi: 10.1108/BFJ-06-2015-0200
Dworkin, S. L. (2012). Sample size policy for qualitative studies using in-depth interviews. Arch. Sex. Behav. 41, 1319–1320. doi: 10.1007/s10508-012-0016-6
FAO (2007). Profitability and Sustainability of Urban and Peri-urban Agriculture. Agricultural Management, Marketing and Finance Occasional Paper 19.
Firth, C., Maye, D., and Pearson, D. (2011). Developing “community” in community gardens. Local Environ. 16, 555–568. doi: 10.1080/13549839.2011.586025
Freedman, D. A., Vaudrin, N., Schneider, C., Trapl, E., Ohri-Vachaspati, P., and Taggart, M‘. (2016). Systematic review of factors influencing farmers' market use overall and among low-income populations. J. Acad. Nutr. Dietetics 116, 1136–1155. doi: 10.1016/j.jand.2016.02.010
Grebitus, C. (2008): Food Quality from the Consumer's Perspective - An Empirical Analysis of Perceived Pork Quality. Göttingen: Cuvillier Publisher.
Grebitus, C., and Bruhn, M. (2008). Analysing semantic networks of pork quality by means of concept mapping. Food Qual. Prefer. 19, 86–96. doi: 10.1016/j.foodqual.2007.07.007
Grebitus, C., Printezis, I., and Printezis, A. (2017). Relationship between consumer behavior and success of urban agriculture. Ecol. Econ. 136, 189–200. doi: 10.1016/j.ecolecon.2017.02.010
Grunert, K. G., and Bech-Larsen, T. (2005). Explaining choice option attractiveness by beliefs elicited by the laddering method. J. Econ. Psychol. 26, 223–241. doi: 10.1016/j.joep.2004.04.002
Henderson, G. R., Iacobucci, D., and Calder, B. J. (1998). Brand diagnostics: mapping branding effects using consumer associative networks. Eur. J. Operat. Res. 111, 306–327. doi: 10.1016/S0377-2217(98)00151-9
Hsia, H. J. (1988). “Mass communications research methods: a step-by-step approach,” in Communication Textbook Series. Mass Communication, eds J. Bryant and A. M. Rubin (Hillsdale, NJ: Lawrence Erlbaum Associates, Inc.).
Hughes, D.W., and Boys, K. A. (2015). What we know and don't know about the economic development benefits of local food systems. Choices 1–6. Available online at: http://choicesmagazine.org/choices-magazine/theme-articles/community-economics-of-local-foods/what-we-know-and-dont-know-about-the-economic-development-benefits-of-local-food-systems (accessed May, 2020).
Hunold, C., Sorunmu, Y., Lindy, R., Spatari, S., and Gurian, P. (2017). Is urban agriculture financially sustainable? An exploratory study of small-scale market farming in Philadelphia, Pennsylvania. J. Agric. Food Syst. Commun. Dev. 7, 51–67. doi: 10.5304/jafscd.2017.072.012
Joiner, C. (1998). Concept mapping in marketing: a research tool for uncovering consumers' knowledge structure associations. Adv. Consum. Res. 25, 311–317.
Jonassen, D. H., Beissner, K., and Yacci, M. (1993). Structural Knowledge: Techniques for Representing, Conveying and Acquiring Structural Knowledge. Hillsdale, NJ: Routledge.
Kanwar, R., Olson, J. C., and Sims, L. S. (1981). Toward conceptualizing and measuring cognitive structures. Adv. Consum. Res. 8, 122–127.
Kroeber-Riel, W., Weinberg, P., and Gröppel-Klein, A. (2009). Konsumentenverhalten, 9th Edn. München: Vahlen.
Lachal, J., Speranza, M., Taïeb, O., Falissard, B., Lefèvre, H., Moro, M. R., and Revah-Levy, A. Q. (2012). Qualitative research using photo-elicitation to explore the role of food in family relationships among obese adolescents. Appetite 58, 1099–1105. doi: 10.1016/j.appet.2012.02.045
Lamnek, S. (1995). Qualitative Sozialforschung. Band 2: Methoden und Techniken, 3rd Edn. Weinheim Verlag.
Martin, J. (1985). Measuring clients' cognitive competence in research on counseling. J. Couns. Dev. 63, 556–560. doi: 10.1002/j.1556-6676.1985.tb00680.x
Mayring, P. (2002). Einführung in die Qualitative Sozialforschung. Eine Anleitung zu Qualitativem Denken, 5th Edn. Weinheim: Beltz Studium.
Mitter, H., Larcher, M., Schönhart, M., Stöttinger, M., and Schmid, E. (2019). Exploring farmers' climate change perceptions and adaptation intentions: empirical evidence from Austria. Environ. Manag. 63, 804–821. doi: 10.1007/s00267-019-01158-7
Mougeot, L. J. (Ed.). (2005). Agropolis: The Social, Political, and Environmental Dimensions of Urban Agriculture. London: IDRC.
Olson, J. C. (1978). Inferential belief formation in the cue utilization process. Adv. Consum. Res. 5, 706–713.
Olson, J. C., and Muderrisoglu, A. (1979). The stability of responses obtained by free elicitation: implications for measuring attribute salience and memory structures. Adv. Consum. Res. 6, 269–275.
Peter, J. P., and Olson, J. C. (2008). Consumer Behavior and Marketing Strategy, 8th Edn. New York, NY: Mcgraw-Hill/Irwin Series in Marketing.
Printezis, I., and Grebitus, C. (2018). Marketing channels for local food. Ecol. Econ. 152, 161–171. doi: 10.1016/j.ecolecon.2018.05.021
Roininen, K., Arvola, A., and Lähteenmäki, L. (2006). Exploring consumers' perceptions of local food with two different qualitative techniques: laddering and word association. Food Qual. Prefer. 17, 20–30. doi: 10.1016/j.foodqual.2005.04.012
Russell, C., and Cox, D. (2004). Understanding middle-aged consumers' perception of meat using repertory grid methodology. Food Qual. Prefer. 15, 317–329. doi: 10.1016/S0950-3293(03)00073-9
Rye, J. A., and Rubba, P. A. (1998). An exploration of the concept map as an interview tool to facilitate the externalization of students' understandings about global atmospheric change. J. Res. Sci. Teach. 35, 521–546. doi: 10.1002/(SICI)1098-2736(199805)35:5<521::AID-TEA4>3.0.CO2-R
Sadler, R. C. (2016). Strengthening the core, improving access: bringing healthy food downtown via a farmers' market move. Appl. Geogr. 67, 119–128. doi: 10.1016/j.apgeog.2015.12.010
Sampson, P. (1972). Using the repertory grid test. J. Market. Res. 9, 78–81. doi: 10.1177/002224377200900117
Satterthwaite, D., McGranahan, G., and Tacoli, C. (2010). Urbanization and its implications for food and farming. Philos. Trans. R. Soc. Lond. Series B Biol. Sci. 365, 2809–2820. doi: 10.1098/rstb.2010.0136
Shrestha, M. K., York, A. M., Boone, C. G., and Zhang, S. (2012). Land fragmentation due to rapid urbanization in the phoenix metropolitan area: analyzing the spatiotemporal patterns and drivers. Appl. Geogr. 32, 522–531. doi: 10.1016/j.apgeog.2011.04.004
Smith, J. P., Li, X., and Turner, B. L. (2017). Lots for greening: identification of metropolitan vacant land and its potential use for cooling and agriculture in phoenix, AZ, USA. Appl. Geogr. 85, 139–151. doi: 10.1016/j.apgeog.2017.06.005
Sonneville, K. R., La Pelle, N., Taveras, E. M., Gillman, M. W., and Prosser, L. A. (2009). Economic and other barriers to adopting recommendations to prevent childhood obesity: results of a focus group study with parents. BMC Pediatr 9:81. doi: 10.1186/1471-2431-9-81
Stempel, G. H. III. (1981). “Content analysis,” in Research Methods in Mass Communication, eds G. H. Stempel III and B. H. Westley (New Jersey, NY: Prentice-Hall, Inc.).
Surls, R., Feenstra, G., Golden, S., Galt, R., Hardesty, S., Napawan, C., et al. (2015). Gearing up to support urban farming in California: preliminary results of a needs assessment. Renew. Agric. Food Syst. 30, 33–42. doi: 10.1017/S1742170514000052
Takahashi, B., Burnham, M., Terracina-Hartman, C., Sopchak, A. R., and Selfa, T. (2016). Climate change perceptions of NY state farmers: the role of risk perceptions and adaptive capacity. Environ. Manag. 58, 946–957. doi: 10.1007/s00267-016-0742-y
U.S. Census Bureau (2019). Annual Estimates of the Resident Population. American FactFinder. Available online at: https://www.factfinder.census.gov/faces/tableservices/jsf/pages/productview.%20xhtml?src=CF (accessed February 10, 2019).
United Nations (2018). 68% of the World Population Projected to live in Urban Areas by 2050, Says UN. Available online at: https://www.un.org/development/desa/en/news/population/2018-revision-of-world-urbanization-prospects.html (accessed April 12, 2019).
Van Gilder, B., and Abdi, S. (2014). Identity management and the fostering of network ignorance: accounts of queer Iranian women in the United States. J. Intercult. Commun. Res. 43, 151–170. doi: 10.1080/17475759.2014.892895
Wakefield, S., Yeudall, F., Taron, C., Reynolds, J., and Skinner, A. (2007). Growing urban health: community gardening in South-East Toronto. Health Promot. Int. 22, 92–101. doi: 10.1093/heapro/dam001
Warren, E., Hawkesworth, S., and Knai, C. (2015). Investigating the association between urban agriculture and food security, dietary diversity, and nutritional status: a systematic literature review. Food Policy 53, 54–66. doi: 10.1016/j.foodpol.2015.03.004
Wasserman, S., and Faust, K. (1994). Social Network Analysis: Methods and Applications. Cambridge: Cambridge University Press. doi: 10.1017/CBO9780511815478
WHO (2018). WHO. Available online at: http://www.who.int/elena/titles/fruit_vegetables_ncds/en/ (accessed August 23, 2018).
Wielemaker, R., Oenema, O., Zeeman, G., and Weijma, J. (2019). Fertile cities: nutrient management practices in urban agriculture. Sci. Total Environ. 668, 1277–1288. doi: 10.1016/j.scitotenv.2019.02.424
Wimmer, R. D., and Dominick, J. R. (1983). “Mass media research. An introduction,” in Wadsworth Series in Mass Communication, ed R. Hayden (Belmont, CA: Wadsworth Publishing Company).
World Bank (2016). Urban Population (% of total). Available online at: https://www.data.worldbank.org/indicator/SP.URB.TOTL.IN.ZS (accessed January 15, 2017).
Wortman, S. E., and Lovell, S. T. (2013). Environmental challenges threatening the growth of urban agriculture in the United States. J. Environ. Qual. 42, 1283–1294. doi: 10.2134/jeq2013.01.0031
Zezza, A., and Tasciotti, L. (2010). Urban agriculture, poverty, and food security: empirical evidence from a sample of developing countries. Food Policy 35, 265–273. doi: 10.1016/j.foodpol.2010.04.007
Keywords: cognitive structures, concept mapping, exploratory, semantic network, urban agriculture
Citation: Grebitus C, Chenarides L, Muenich R and Mahalov A (2020) Consumers' Perception of Urban Farming—An Exploratory Study. Front. Sustain. Food Syst. 4:79. doi: 10.3389/fsufs.2020.00079
Received: 22 December 2019; Accepted: 01 May 2020;
Published: 12 June 2020.
Edited by:
Helda Morales, The South Border College (ECOSUR), MexicoReviewed by:
Indira Devi Puthussery, Kerala Agricultural University, IndiaCopyright © 2020 Grebitus, Chenarides, Muenich and Mahalov. This is an open-access article distributed under the terms of the Creative Commons Attribution License (CC BY). The use, distribution or reproduction in other forums is permitted, provided the original author(s) and the copyright owner(s) are credited and that the original publication in this journal is cited, in accordance with accepted academic practice. No use, distribution or reproduction is permitted which does not comply with these terms.
*Correspondence: Carola Grebitus, Y2Fyb2xhLmdyZWJpdHVzQGFzdS5lZHU=
Disclaimer: All claims expressed in this article are solely those of the authors and do not necessarily represent those of their affiliated organizations, or those of the publisher, the editors and the reviewers. Any product that may be evaluated in this article or claim that may be made by its manufacturer is not guaranteed or endorsed by the publisher.
Research integrity at Frontiers
Learn more about the work of our research integrity team to safeguard the quality of each article we publish.