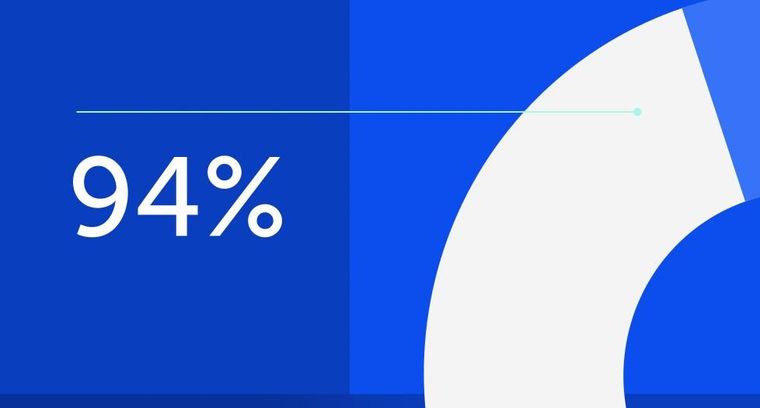
94% of researchers rate our articles as excellent or good
Learn more about the work of our research integrity team to safeguard the quality of each article we publish.
Find out more
ORIGINAL RESEARCH article
Front. Sustain. Food Syst., 28 April 2020
Sec. Agroecology and Ecosystem Services
Volume 4 - 2020 | https://doi.org/10.3389/fsufs.2020.00041
This article is part of the Research TopicMeasuring Interactions among Ecosystem Services in Agricultural LandscapesView all 7 articles
Realizing more sustainable food, feed, and bioenergy systems will require interventions such as increased recycling of nutrients and coordination of biomass flows among farms. Innovative tools to explore the co-benefits and trade-offs of improving flow circularity in agro-food systems at different scales are needed to better understand the efficacy of these sustainability solutions. Here, we applied the FAN (“Flows in Agro-food Networks”) agent-based model to simulate contrasting scenarios of material flows locally in a small farming region of France. These scenarios aim to enhance: (1) best management practices at the farm scale; (2) organic material recycling and biogas production collectively across the agricultural landscape; and (3) system redesign toward complete local circularity through crop and livestock symbiosis, fewer livestock, and elimination of external inputs. Scenario simulation outcomes are assessed in terms of their degree of circularity and food production. We find that best management practices at the farm scale and collective solutions for recycling (organic fertilization and anaerobic digestion) substantially improved the degree of circularity by tightening the local nitrogen (N) cycle without affecting food production. Among other co-benefits, changes in farm rotations to feed livestock locally increased the degree of circularity without appreciably impacting food production. The maximum circularity scenario showed considerable potential to mitigate greenhouse gas (GHG) emissions, however, they involved large trade-offs with food production that were even more pronounced with fewer livestock animals. Although regulating livestock numbers combined with eliminating chemical fertilizers was the most effective at mitigating GHG emissions, when applied simultaneously it substantially impacted food and bioenergy production. Such trade-offs for soil fertility demonstrate the importance of coupling crops and livestock for reaching self-sufficient circular systems. Our study illustrates how the FAN agent-based model can be applied to account for multiple types of interactions involved in transitions toward circularity in local agro-food systems, including the potential for co-benefits, and unintended consequences of interventions.
Current agricultural resource use and its impacts on non-agricultural ecosystems is a major contributor to pushing the Earth system beyond its safe operating space (Tilman and Clark, 2014; Campbell et al., 2017). New strategies and regulations are needed to tackle agriculture's pressure on natural resources including land, water, nutrients, and climate (Foley et al., 2011; Carlson et al., 2016). With a shift toward more intensive agricultural production in some regions, increasingly specialized farms rely on imported synthetic fertilizers that drive global nutrient imbalances and create complex international dependencies (Lassaletta et al., 2014), particularly for feed crop and livestock production (Van Zanten et al., 2018). However, alternatives can be found at the local scale, particularly if agricultural supply chains move toward close-looped systems that reduce dependence on external inputs (Davis et al., 2016) and recycle nutrients locally (Metson et al., 2016). Reducing agricultural pressure on natural resources can be achieved through a better use of local biomass materials (e.g., feed and forage for livestock as well as organic wastes as fertilizers), by applying circular economy and bioeconomy principles (Golembiewski et al., 2015; El-Chichakli et al., 2016). Stronger circularity through local material exchanges among farms is essential to better use and recycle local resources while minimizing losses. Scenarios examining whole farming regions can provide an improved understanding of the positive or negative impacts of applying such circular strategies that can better inform agro-ecological transitions and policies to enhance farming systems sustainability.
To advance scenarios assessment of a farming circular economy, improved estimates of food production and the environmental performance linked to changing material flows in the agro-food system locally are needed. Several studies have analyzed the impacts of farming systems transitions to agroecology at the plot or the farm scale (Pelzer et al., 2012; Marchand et al., 2014; Giuliano et al., 2016; Wery et al., 2018). Yet, fewer studies have tried to go further and evaluate farming systems at larger local scales, such as in small agricultural regions (Le Noë et al., 2017; Billen et al., 2018). On the one hand, some large scale studies focused on a single sector of the agro-food system (e.g., bioenergy) or a specific type of farming system within the region studied. Examples of such approaches include the assessment of sustainable bioenergy production (Toop et al., 2017; Vega-Quezada et al., 2017; Fan et al., 2018; Cobo et al., 2019); the assessment of dairy farming in different European regions (Acosta-Alba et al., 2012; Prado et al., 2013); the exchanges between specialized arable and bovine farms in a region of France (Moraine et al., 2017a,b); and nutrient dynamics of crop-livestock farming systems in Africa (Manlay et al., 2002; Tittonell et al., 2009; Giller et al., 2011). On the other hand, other studies have assessed diverse types of production systems across a single farming region, such as the scenario assessment of the Montérégie region in Canada (Mitchell et al., 2015), the California Nitrogen Assessment (Tomich and Scow, 2016), and a life-cycle assessment (LCA) of a French catchment (Avadí et al., 2016). However, such broader approaches typically did not quantify precisely multiple farm properties for the simulation of alternative farming practices and material exchanges. Although few studies have estimated outputs from different food sectors of the from a complex farming population, including feed and wastes chains in a region, we need to better account for the interactions between farms and with their upstream and downstream partners, to be able to evaluate food systems circularity (de Boer and van Ittersum, 2018; Tseng et al., 2019).
Agent-based models can be a useful tool to simulate different agents' behaviors under alternative scenarios toward sustainability (Alonso-Betanzos et al., 2017). For example, characterizing the agro-food system as a network of interacting agents can help to represent alternative scenarios of biomass materials management. Although there is a large literature on agent-based models applied to land use systems (Matthews et al., 2007), very few studies have applied agent based simulations to study alternative scenarios of materials management in agro-food systems at the local scale (Gaube et al., 2009; Bichraoui et al., 2013; Grillot et al., 2018). To our knowledge, agent-based models have not been used yet to simulate alternative scenarios of material exchanges concerning crops, livestock, wastes, and bioenergy all together. Simulation models combined with alternative scenarios of material exchange can help to assess the circularity of farming systems to better understand the benefits and trade-offs of implementing alternative material flow strategies among farms on the same landscape (Fernandez-Mena et al., 2016).
In this study, we aimed to simulate alternative scenarios of material flows across a single farming region. To do so, we applied the FAN (“Flows in Agro-food Networks”) model to a case study of a small farming region in southwest France. A short description of the FAN agent-based model and its key indicators is provided in Section The FAN Model Brief Description and Indicators Presentation. The Ribéracois is the region used as case study and its characteristics are presented in Section The Ribéracois Case Study. The scenarios examined in the simulations, described in Section Scenario Design, were designed in an additive manner, i.e., including previous step implementations while addressing a new issue at each step: (i) the business as usual (BAU) situation; (ii) best management practices at the farm scale; (iii) optimizing material exchanges; (iv) enhancing bioenergy production; (v) enhancing crop-livestock symbiosis; and (vi) maximizing circularity; scenarios (v) and (vi) also involve reductions in livestock numbers. We also hypothesized about potential effects that these “leverage” factors could present across the scenarios. With the objective of assessing the system performance, we used a series of food production and circularity indicators incorporated in the FAN model, described in Section The FAN Model Brief Description and Indicators Presentation.
“Flows in Agro-food Networks” (FAN) is an agent-based model that simulates and assesses farming activities based on processing and exchanging agricultural materials. FAN is a theoretical tool to explore and assess opportunities for a circular economy in small farming regions, such as the “Ribéracois”, which we refer to as a “district” herein. At this scale, FAN may help to unravel interactions concerning material exchanges in farming activities by simulating recycling flows and assessing their degree of circularity. The FAN model mechanisms and submodels are described following the ODD (Overview, Design concepts, and Details) protocol for agent-based models in Fernandez-Mena et al. (2019). The model script, the Ribéracois dataset and the scenarios parametrization are available in Fernandez-Mena (2017a).
The FAN model simulates, on an annual time-step, different agro-food system activities in the following order: crop fertilization; crop production; feed exchanges; livestock production; food processing; food waste and by-products exchanges; and, bioenergy production. The materials exchanged include organic and chemical fertilizers, feed, straw, food, by-products, and wastes. The agents involved in the network exchanges are farms and their upstream or downstream partners in small farming regions, such as feed and fertilizer suppliers, food industries, waste processors, and anaerobic digesters. The mechanisms involved in material exchanges selects a probable exchange between two agents based on a stochastic algorithm, after evaluating the whole local combinations of agents willing to exchange a specific material. The combinations are ranked through an equation taking into account: (i) the distance between the agents; (ii) the quantity demanded and supplied; and, (iii) the preference coefficient chosen by the simulator for a specific material use (e.g., use manure for fertilization vs. anaerobic digestion; use grass for animal feeding vs. anaerobic digestion; etc.). A fidelity variable can be used to preserve a percentage of agent exchanges for the following simulated year and accelerate calculations. A radius of action can be set to limit the exchanges of certain biomass materials (e.g., 10 km for fresh manure, 60 km for grass fodder, etc.). In order to create scenarios of exchanges, users can modify a certain number of parameters in FAN, presented in Table S1. Finally, FAN includes a series of indicators, explained in detail in the following sections, which serve to assess alternative scenarios in terms of food production and systems circularity.
In FAN, crop yields vary with nitrogen (N) applications to soils that is consider to be the single limiting factor for crop production. N crop uptake is considered to drive crop yield through a linear relationship until a plateau of maximum yields. Given that N is typically overused at present, maximum yields are assumed to be the current yields observed in this case-study. Initial soil N application were set to the observed fertilizer application in Ribéracois. Fertilized crop rate is adjusted in scenario simulations according to calculated farm N budgets and N availability. N fertilization needs were estimated at the farm scale by summing the N needs of all crop categories within the farm: cereals, oilseeds, fruits and vegetables, silage maize. However, grass, legume forage, and pulse crops were considered not to require fertilization in our simulations due to biological N fixation.
Forage production is estimated in FAN as the addition of grass, silage maize and legume forage production. Livestock production was calculated taking into account the number of adult animals on each farm and their fattening and reproduction ratio according to the standardized feed rations in France (Devun and Guinot, 2012). As we were informed by the extension services in the Southwest of France during informal interviews (see Section The Ribéracois Case Study) that forage intake per ruminant could slightly vary among farms, a threshold is set to −10% of the average forage ratio and a maximum was set to +10% of this value. In FAN, both feed and forage requirements are expected to be satisfied, first, by inner farm production, second, by exchanges with others farms through after local feed stocking and processing, and, third, with global market imports if needed.
Input materials for digestion are chosen in a proportion similar to the average feedstock composition in France that limits food-crop-based materials. This average digestible feedstock contains 68% manure, 17% green biomass (considered to be grass in FAN), and 15% food processing wastes, including cereal, milk, fruit and vegetable wastes (ADEME, 2013). Animal manure is the most important ingredient in the feedstock and was considered to be the limiting factor for maximizing anaerobic digestion without compromising food-crop-based materials, following recommendations in France. Therefore, the anaerobic digester capacity in Ribéracois was calculated according to the total animal manure in the district, estimated as 225,210 tons of feedstock (ADEME, 2013). The bioenergy produced was calculated proportionally to the methane potential of the materials used in the feedstock: 42 m3 of biogas, with 34% of electric yield equivalent to 85.73 kWh of electricity per ton of digested feedstock.
Estimating the number of local flows provides a proxy for the degree of local cooperation in material exchange among farms that is necessary as part of increased network circularity. In the FAN model, materials are assumed to be transported by truck from one farm to another, or between farms and their partners. We proposed “local flows” as a network indicator representing exchanges between two agents within the Ribéracois district. We considered that each local flow stops when the corresponding material is used and transformed to produce either food or energy, while exchanges inflowing from or outflowing to the global market were not included. Local flows were aggregated according to their final uses as follows: (i) local fertilization flows including manure, digestates and sewage sludge applied to soils; (ii) energy flows including manure, grass and food processing wastes allocated to anaerobic digesters; as well as three flows allocated to animal requirements: (iii) feed flows; (iv) forage flows; and (v) straw flows for bedding.
The N balance includes inflows (N from chemical fertilizers, organic fertilizers, digestates, and sewage sludge) and outflows (N in harvested crops, N leaching, as well as losses of N-N2O and N-NH3+NOx). All catch-crops were assumed to reduce 50% N leaching (Valkama et al., 2016). These values were individually calculated for each farm annually and then aggregated to obtain a whole N balance of the district. In addition, since our scenarios were oriented toward maximizing the local circularity of fertilization, two indicators of organic N participating to this circuity are proposed:
“N orgapplied%” accounts for all non-mineral sources in the total N added to soils, whereas “N orgexchanged%” takes into account only the part of organic N that was exchanged between two agents before being used for fertilization. The first indicator accounts for the degree of N circularity in the region, since only organic sources are produced locally; whereas the second accounts for the effort performed to recycle these nutrients by exchanging materials between farms.
The net greenhouse gas (GHG) emissions are estimated including both on- and off-site emissions linked to changes implemented in the scenarios, such as the emissions induced by material truck transportation, chemical fertilizer and feed off-site production as well as avoided emissions induced by bioenergy production. To estimate emissions in FAN, a simple soil mineralization model is applied (Hénin and Dupuis, 1945; I.P.C.C., 2006). Tier 1 default values are used for emissions from farming and the ADEME (2017) database is used for truck, biogas and feed imports emissions. In the net GHG estimation (expressed in CO2-equivalence), emissions are considered to be positive, whereas C stored in soils and CO2 avoided are considered to be negative. Farm machinery and buildings are not included since they are considered to be invariable among scenarios. Grasslands C net sequestration capacity are assumed to be equivalent to those of European grasslands, following Chang et al. (2016). Although emissions induced by producing the food or feed elsewhere to compensate for lower production in the study area are not considered, an efficiency food production ratio in kg of protein or MJ of food energy per CO2-equivalent are calculated for each scenario.
We utilize a multi-criteria assessment to compare the performance of the different scenarios through a relative score obtained for key production and circularity indicators. The relative score is calculated for the three types of production indicators (crop production, livestock production, and bioenergy production) and for the four key system circularity indicators (feed autonomy, bioenergy production, N cycle closing, and GHG mitigation potential). Relative scores range from 0 to 10 and are calculated as an equivalence of the relative scenario performances on each indicator. The percentage of organic N for fertilization and the legumes autonomy are used as a proxy to calculate the relative score for closing the N cycle. A second calculation is made for the cumulative score across the seven indicators presented, assuming that all indicators count equally.
Ribéracois has a total area of around 1,000 km2, with approximately 500 km2 of utilized agricultural area (UAA), a mean altitude of 80 m, an annual rainfall of around 800 mm, and an average annual temperature of 12.3°C. It is located in Dordogne département of the Nouvelle-Aquitaine administrative region, in the southwest of France (Figure 1). For comparison, the surface area of the Ribéracois district is of the same order of magnitude as the average county in the U.S. (1,610 km2).
Figure 1. Geography of the “Ribéracois” district. On the left, location of the district in Dordogne, France. On the right, map of the district and key agents in FAN. Farms are represented by circles colored with their type (green for arable; blue for dairy; red for beef cows; purple for mixed cattle; black for mixed crop-livestock; yellow for horticultural; orange for monogastrics; and gray for ovine and caprine). Squares represent food industries (yellow: feed collectors; blue: milk industries; green: fruits and vegetables industry; red: slaughterhouse).
Data on agricultural land use and livestock units per farm type were collected from the agricultural census of the French Ministry of Agriculture (Agreste, 2013). In addition, a validation process was conducted by contrasting actual farm practices to adapt the FAN model (Garcia, 2016), helping to confirm the main principles of exchange mechanisms simulated in FAN. Farming practices related to animal feed rations, distance of biomass exchanges, and organic fertilization have been further explored based on interviews with agronomists from the local extension services (Chambre d'Agriculture de la Dordogne) and compared with regional statistics (Agreste, 2014; Groupe Régionale d'Expertise Nitrates (GREN), 2016). We consulted with local farming cooperatives, dairy industries, and slaughterhouses on the destination of crop and animal flows and therefore on the production of organic wastes (Garcia, 2016). Finally, the feedstock composition for anaerobic digestion has been contrasted with digesters recently constructed in the areas nearby (based on personal communications with managers of Chambre d'agriculture de Dordogne).
In Ribéracois, the agricultural census registered 835 farms with a diversity of farming activities, with a large population of specialized farms and only some exceptions of mixed crop-livestock farms. Crop production is focused on arable crops, especially cereals, oilseeds, and silage maize for forage. Only a few farms focus on horticultural production (Table 1). Livestock is highly diversified and includes substantial cattle production, specialized in beef or in dairy production, followed by numerous farms breeding small ruminants (ovine and caprine) and some monogastric livestock production of pork and poultry. A number of upstream and downstream partners of farms operate across the district by sourcing raw materials, as well as transforming and trading farming products. These partners include three large companies that collect cereals and process feed, two milk and cheese industries, two slaughterhouses, several small fruits and vegetables industries, four wastewater treatment plants, and some projects of anaerobic digesters construction. Although farming activities in Ribéracois are quite diversified, they are challenged by relatively inefficient nutrient and biomass management. High N fertilization rates for arable crops are applied overall the district (~160 kg N·ha−1·year−1), similar to other regions in France (Agreste, 2014). This high rates lead to high N losses through various pathways. The southern part of the Ribéracois, where arable crops are predominant, has been classified as a Vulnerable Zone by the EU Nitrate Directive since 2015 (DREAL, 2016). Livestock producers are affected by the high volatility of input prices due to overall deficit in soybean (imported mainly from South America) and alfalfa (imported from the north of France) to meet livestock requirements. Green, renewable energy production through anaerobic digestion is strongly pushed by local authorities, both at farm and collective scales.
Table 1. Average farm types characteristics in Ribéracois (Agreste, 2013).
In order to design our scenarios, we considered several circular flows, both at the farm scale through agro-ecological practices and at the local scale via flows among farms and their partners. Therefore, we hypothesized that a series of “leverage” factors could be used to achieve circularity both at the farm and at the district scale with synergies for environmental performance of agriculture. According to the literature, a series of levers were selected for their potential benefits, as developed below. They are: (i) reduction of nutrient losses to the environment by adjusting fertilization rates to crop requirements and by using catch crops (Valkama et al., 2016); (ii) reduction of protein intake in cattle feed rations (Edouard et al., 2016; Pellerin et al., 2017); (iii) exchanging organic fertilizer materials among farms (Nowak et al., 2015); (iv) setting up biogas plants in the district (Mao et al., 2015); (v) enhancing the production of local feed and forage (Bonaudo et al., 2014; Lemaire et al., 2014); (vi) reducing livestock populations (Leip et al., 2015); and (vii) removing chemical fertilizers (Bouwman et al., 2017). Although all these drivers may enhance a component of the system's circularity through better environmental performance, they are also interconnected and may have co-benefits or trade-offs in terms of other indicators and whole system performance (Figure 2).
Figure 2. Hypothesized effects of the leverage factors (boxes) on key indicators of the system performance (circles). Arrows represent interactions between leverage factors and system performances. Green arrows stand for positive effects, red arrows for negative effects and light blue for unknown effects.
Based on the leverage factors, we designed contrasting scenarios to be applied in the Ribéracois region, ranging from relatively simple transition steps to more complex transformations. The scenario design follows an additive manner, i.e., each scenario applied the changes already implemented in the previous one. These are built following the “Efficiency, Substitution and Redesign” framework (ESR), which has already been applied to agricultural systems transition toward an agroecological sustainability (Lamine, 2011; Wezel et al., 2014). “Efficiency-like” solutions are considered in designing the “Best management practices” scenario that implement management recommendations to improve fertilizer input and animal feed use efficiency. “Substitution-like” solutions are used to design scenarios based on local collective solutions through exchanges, without modifying the farm structures. In a complete “Redesign” perspective, external supplies of feed and fertilizer are removed and farm structure (land-use and livestock population) is modified (Figure 3).
Figure 3. Scenarios explored in the Ribéracois case study using the FAN model. The S5b scenario is Crop-Livestock Symbiosis and the S6b Scenario is Maximum Circularity. The scenarios are designed in an additive manner, i.e., each scenario applied the changes already implemented in the previous one.
We hypothesized that greater environmental benefits would accrue in scenarios that require more profound agro-food system changes (i.e., for “Redesign-like” scenarios). The scenarios are built in the FAN model by changing specific parameters and coefficients of the model (Table 2), as detailed in Fernandez-Mena et al. (2019). A brief scenario description is given as follows:
S1. The “Business As Usual” (BAU) situation represents the current farming practices, its production and environmental impacts. It is considered as the baseline situation. Its parameters were set to FAN default values, presented in Table S1. Although there was a project of anaerobic digester construction in the district in 2016 (Garcia, 2016), we did not consider any anaerobic digestion in the baseline and following scenarios.
S2. The adoption of “Best Management Practices” (BMP) at the farm scale. In this scenario, farms aimed to reduce their environmental pressure by applying some basic management interventions that are commonly recommended by agrarian extension agencies (Baumgart-Getz et al., 2012). In particular, the N fertilizer application rate was adjusted to crop requirements plus some unavoidable losses including N volatilization and moderate N leaching (overdose coefficient of 1.3). Farms are also required to use catch crops in order to additionally reduce N losses through leaching. Animal diets are adjusted by slightly reducing protein feed intake according to protein use efficiency recommendations (Edouard et al., 2016; Pellerin et al., 2017), expecting improved nutrient use efficiency.
S3. The fostering of material “exchanges” (EXCH) between farms and their partner network for better closing nutrient cycles, including local forage and organic fertilizers. Here, on top of using best management practices, all farms are predisposed to exchange materials with their partners to meet their fertilizer nutrient demands. In addition, the FAN preference coefficient for using manure and sewage sludge as organic fertilizers are increased to the maximum level.
S4. The implementation of “biogas” (BIOGAS) production plants up to their maximum potential. In this scenario, exchanges are maximized and 10 anaerobic digester units are simulated to ferment biomass materials in order to produce electricity from biogas (CH4 + CO2). Consequently, farms are forced to exchange their manure with the digesters, getting back similar amounts of nitrogen as digestates to fertilize soils. Then, farms may offer their digestates surplus to other farms in the network. FAN coefficients are adapted to facilitate flows with digesters, including manure, grass, and food wastes materials. Note that our approach develops bioenergy scenarios by respecting the important principle of using plant biomass in priority for human food, as described by de Boer and van Ittersum (2018).
S5a. A complete “Crop-Livestock symbiosis” (C-L Symb.) through local feed and forage production is added to S4 (maximized exchanges and biogas). In this scenario, crop production is adjusted to meet the local livestock feed requirement of all the farms. As a consequence, if a forage deficit exists, cereal crops are converted to grasslands in order to compensate such deficit. Similarly, if any concentrate feed deficit exists—in particular in pulses—cereal crop areas are converted to pulses. Cereals were chosen because they are the most common crop in the area.
S6a. A system with maximum circularity is implemented in the “Max. Circularity” scenario, where no external inputs to the system are allowed. Therefore, all the previous circular implementations are retained, for exchanging, producing biogas and locally feeding livestock, and, in addition to this, chemical fertilizers are no longer available, rendering the system fully self-sufficient.
Two alternatives are explored for the last two scenarios:
S5b. Livestock are reduced to half of the initial population for all animal species. In addition, all principles of local feed and forage presented in S5a are also applied (C-L Symb. + L/2). Unused silage maize for forage is reduced and replaced by cereals. Grasslands are not modified.
S6b. In the “Max. Circularity + L/2” scenario, livestock are reduced to half of the initial population and maximum circularity is applied, therefore, implementations of S5b and S6a are applied together.
In total, we launched 30 simulations of each scenario by running each until its 5th year.
In order to initialize the Ribéracois district in FAN, a synthetic farm population is created based on the average features of the different farm types (Table 1). Farms are classified according to their main agricultural production into different farm types. The number of farm types, their total area and the average land use and livestock number of each farm type are used to create a synthetic population of farms. The size of farms is simulated following a normal distribution centered to the mean and standard deviation of the total area. Then, land use and livestock numbers are distributed proportionally to the obtained size, following the real proportion on each farm type. Other farm partners, such as food industries, slaughterhouses and wastewater treatment stations, are located spatially in the FAN map by using their geographical location in the district. Anaerobic digesters are created following a homogeneous spatial distribution of their digestion capacity.
Here, we discuss the FAN simulation results estimated through the various indicators presented above and their relative scores. These indicators covered food and energy production and circularity. The indicators were calculated in annual units as a result of a 5 years' simulation with 30 repetitions for each scenario. We start by presenting the results of each of the indicators in detail and then the relative score multi-criteria assessment that summarize the whole simulation performance.
Overall, the number of total local flows increased from the baseline to the last scenario, especially when implementing anaerobic digestion and crop livestock symbiosis (Figure S1). Local fertilization flows (corresponding to both manure and sewage sludge) increased considerably in the EXCH scenario, up to 450 local flows in the Max. Circularity scenario, where organic manure is the only option for fertilizing soils (Figure 4). However, the number of fertilization flows is reduced when livestock populations are reduced (L/2), since flows for animal requirements are numerous. Local food waste flows (corresponding to both energy and animal requirements) slightly increase when implementing digestion. The number of feed grain flows decrease when crop and livestock integration are applied (C-L Symb.) since this integration occurs mainly at the farm scale. Although straw flows increase with crop livestock integration, local forage does not change as there is enough forage production area. Finally, when reducing livestock population, because there is enough forage within each farm, forage exchanges are no longer needed.
Figure 4. GAMA platform display for local fertilization flows in Ribéracois in two contrasted scenarios: BAU situation on the left and Maximum Circularity on the right. Farm typologies are represented by colored circles: green for arable farms; black for mix farms; red for beef cattle farms; blue for dairy cattle farms; purple for mix cattle farms; gray for ovine and caprine farms; orange for monogastric farms and yellow for horticultural farms. Wastewater treatment station are presented in large blue circles; anaerobic digesters in gray triangles and feed collectors in yellow squares. Material flows are presented in lines joining supplier and demander agents: manure for fertilization flows are in black lines; manure for digestion flows are in brown lines; digestates flows are in orange lines; sewage sludge flows are in blue lines and chemical fertilizer flows in purple lines. Note that manure for digestion returns from digesters to the supplying farm before this farm shares outflows of any surplus digestates to other farms.
Results for N inputs to soils show the highest chemical fertilizer use in the BAU scenario, whereas fertilizer use exhibits a strong decrease when adjusting fertilization to crop needs in the BMP scenario, as well as in the remaining scenarios (Figure 5). After EXCH scenario, N chemical fertilizer remains low, since organic fertilization is privileged through preference coefficients. However, N chemical fertilizer increases when reducing livestock in C-L Symb. + L/2 since the area under crops with strong N demand such as cereals increases. The N from animal excreta (collected in barns) allocated to crop fertilization only satisfied half of crop requirements, however, it was initially reduced with protein intake, and finally proportionally reduced to livestock heads in C-L Symb. + L/2 and Max. Circularity + L/2 scenarios. The N fixed by legumes is particularly remarkable when crop-livestock symbiosis is applied, fostering pulse production. In the BIOGAS scenario, the N added by digested green biomass and food processing wastes behaves as a new input of N to the system not mobilized in the previous scenarios. Finally, sewage sludge inflows do not play an important role in the overall N inputs. Concerning N outputs, N crop uptake varied firstly when changing land-use in C-L Symb, by adding pulses to the system that fixed N from the atmosphere. These pulses are reduced in C-L Symb + L/2 since they are no longer needed. As shown in crop production results, N inputs in scenarios without chemical fertilizers are not enough to satisfy N crop demand for maximum yields. Nitrogen leaching is very high in the BAU scenario, but steps taken in BMP are effective to reduce N losses. In fact, best management practices have a dual effect on N losses: on the one hand, N fertilization rates are adjusted to crop needs and, on the other hand, catch crops are implemented to reduce N leaching. Volatilization losses (as NH3 and NOx produced during manure storage and fertilizers application) increase considerably when anaerobic digestion is applied, due to higher ammonia emissions, approaching one third of total N applied to soils (Ni et al., 2012), but they are reduced according to lower organic inputs when reducing livestock. Finally, although N2O direct and indirect emissions remain a small proportion of N in any scenario, they have a considerable impact on greenhouse gas emissions.
Figure 5. Nitrogen flows from FAN scenarios output, in Mega-grams of N per year. N inputs to the system are on the left and N outputs (crop uptake and losses) on the right. Scenarios names follow those in Figure 3 and Table 2.
Across the progressive implementation of scenarios, the relative importance of organic N in total inputs to soils increases (Figure 6). The fact of adding more N to the system through the digestion of green biomass and food processing wastes after the BIGOAS scenario, and the addition of pulses fixing N in C-L Symb., is important in this process. Still, when mineral fertilizers are an option for farms, its use reaches at least half of the total N inputs to soils. In scenarios where N organic fertilization is the only option (i.e., Max. Circularity), the total N available in the district is inadequate to satisfy total crop N demand plus losses to the environment, therefore limiting crop yields.
Figure 6. N indicators from FAN scenario outputs, expressed as a percentage of total N. “N orgapplied%” accounts for all organic sources of N added to soils, whereas “N orgexchanged%,” takes into account only the part of organic N that is exchanged between two agents before being used for fertilization, see Equation 1, Equation 2. Scenarios names are in Figure 3 and Table 2.
FAN simulations results show that GHG from on- and off-site emissions (in CO2− eq.) decrease substantially from BAU to the final scenario (Figure 7). The BMP and ECH scenario substantially reduce the GHG emissions by lowering chemical fertilizer use and the emissions associated with their synthesis and losses after application (N2O). In BIOGAS, anaerobic digesters help to reduce most of CH4 emissions from manure storage, significantly improving the balance, despite higher CO2 emission from truck transportation. The symbiosis of crops and livestock in C-L Symb through manure recycling for fertilization as well as local feed sources exhibit lower greenhouse gas emissions than previous scenarios, thanks to the elimination of imported feed. The larger GHG emissions sources were from livestock and N management, in particular CH4 produced by both enteric fermentation and manure storage and N2O from fertilizer application. These emissions are substantially reduced when reducing livestock and removing chemical fertilizers at the same time. Eliminating chemical fertilizer is more efficient than reducing livestock, therefore Max. Circularity performs better than C-L Symb+ L/2 where N application to arable land increased. Finally, C storage by grasslands plays an important role in mitigating the CO2 balance by compensating for CH4 enteric fermentation emissions. Surprisingly, the Max. Circularity + L/2 scenario presented a remarkably low net C balance, estimated at about 12.8 Gg of CO2 eq. per year.
Figure 7. Emissions from on- and off-site emissions (positive) and C stored expressed as CO2 avoided (negative), from FAN scenario outputs (in kg of CO2-equivalence per year). Scenarios names are in Figure 3 and Table 2.
When comparing CO2 emissions to food produced in terms of calories and protein, the progressive pattern across the scenarios is maintained (Figure S2). Initially, emissions are reduced in BMP by reducing N losses and some feed imports. Then, anaerobic digestion implementation in EXCH and BIOGAS scenario also presents a difference with previous scenarios in terms of efficiency, even though there is higher truck transportation for transporting materials. Surprisingly, we observed that just removing chemical N fertilizer is more efficient than just reducing livestock on GHG mitigation potential. Finally, the Max. Circularity + L/2 scenario exhibited a considerably lower GHG budget.
Simulation results showed that crop production was unaffected in the first four scenarios (Figure S3), because no changes were applied to land use or fertilizer availability. However, N uptake in crops is reduced in scenarios with no chemical fertilizer (Max. Circularity), especially when reducing livestock (+ L/2), due to a lack of N local inputs, as shown in Figure 5. Therefore, crops with high N needs (i.e., cereals, oilseeds, fruits and vegetables) decreased their production to around half of their initial values. Yet, pulses and grass were not affected since they are not fertilized with chemical sources in FAN, assuming that there is enough N through biological fixation. Cereal production decreased in Crop-Livestock Symbiosis scenarios (C-L Symb) when incorporating more pulses in crop rotations at the expense of cereals, but cereals increased in the scenario reducing livestock (C-L Symb. + L/2) replacing silage maize, which is no longer necessary to feed fewer livestock. Although pulse production increased when integrating crops and livestock (C-L Symb), there are no changes in grass and legume forage areas as there is enough of these products to meet animal forage requirements in the district.
Results showed that livestock production (Figure S4) remains constant until scenarios where livestock populations are halved (C-L Symb. + L/2; Max. Circularity + L/2). In these final two scenarios, pig and poultry production are reduced to half of the value observed in the baseline scenarios. However, livestock reduction does not affect ruminant meat production in the same way because these ruminants benefit from a higher forage availability that, in turn, increases animal productivity for both milk and meat. Therefore, we observed an interesting compensation for meat production from beef in C-L Symb. + L/2 and Max. Circularity + L/2 scenarios. Note that even if crop production is lower in the scenario without chemical fertilizers, FAN assumes that livestock in Ribéracois are fed in priority to crop food transformation, and when needed, by importing external feed and forage to meet animal minimum requirements (Fernandez-Mena et al., 2019).
Reducing local dependency on external feed and forage was possible by better integrating crop and livestock production at the farm scale, by applying changes in the crop rotations to fulfill livestock district requirements and eventually by reducing livestock population at the district scale. Results show that cereals and oilseeds were always in surplus after feeding animals in the district (Figure S5). However, their production decreased in scenarios with crop-livestock symbiosis due to the competition for land with pulses, and also in scenarios without chemical fertilizers due to depression of crop yields with limited N inputs to soils. On the contrary, cereal balance increased in scenarios with reduced livestock population when silage maize was replaced by cereals. Feed by-products exhibited a small deficit when anaerobic digestion was implemented due to the competition induced by biogas plants for these materials. Pulse deficit was reduced through the crop-livestock integration by adding more pulses to the rotations in the C-L Symb. scenario, which increased the circularity of the system and autonomy. Concerning forage, results show that after local exchanges, exchanges inside farms in Ribéracois always satisfied the minimum livestock requirements. In addition, forage was much more available (even without silage maize) in scenarios with reduced livestock population, potentially explaining the higher beef cattle productivity mentioned earlier.
FAN estimates the total capacity of anaerobic digesters at 225,210 tons of substrate for digestion in the BIOGAS scenario (Figure S6). This capacity dropped to half when livestock population is reduced due to lower manure availability, which is the limiting factor of digestible biomass in our simulations. Biogas production was over 9,042,148 m3 in the BIOGAS scenario and decreased to half of that value when reducing livestock population. The biogas produced in the BIOGAS scenario corresponded to 18.4 GWh of electricity, without taking into account the associated heat production. Considering an annual electricity consumption of 4,679 kWh per household in France, bioenergy production in the Ribéracois could satisfy the demands of 3,942 households, which represents almost one third of the Ribéracois' total human population.
We first analyze the relative score of the scenarios across each of the indicators (Figure S7), which is detailed below:
• Crop production: while crop production did not vary across the first four scenarios that did not involve modifying farm land-use, some scenarios strongly impacted crop yields, in particular when chemical fertilizers are eliminated (Max. Circularity), which was exacerbated by livestock reduction (Max. Circularity + L/2).
• Livestock production: animal production remained constant across all the initial scenarios, even without chemical fertilizers, until the scenarios where livestock population was halved on farms.
• Bioenergy production: energy production is maximized in the BIOGAS scenario. Nevertheless, since manure is they main component of the anaerobic digestion feedstock, bioenergy production is substantially reduced when livestock populations are halved.
• Feed and forage autonomy: livestock in the Ribéracois are not particularly dense on the landscape and therefore local forage typically satisfies local livestock demand. However, changes in the crop rotations helped to fulfill livestock requirements in terms of pulses, that are currently imported, as it was implemented in the C-L Symb. scenario and those afterwards.
• Number of local flows (degree of cooperation): these are substantially increased in the EXCH scenario, facilitating material exchanges across the study area. It reaches its maximum in the BIOGAS scenario. However, the number of flows was reduced in scenarios where livestock populations are halved.
• N cycle closing: it increased progressively in the scenarios through fertilization and feeding rations adjustment and recycling by maximizing exchanges, introducing food industry wastes and locally producing legumes for animal feeding. Although it decreased when livestock is reduced C-L Symb. + 1/2, it reached its maximum in both scenarios without chemical fertilizers.
• GHG mitigation: progressively improved from the base-line situation to the last scenario (Max. Circularity + L/2) that presented the best GHG balance (detailed further in this section), except for the C-L Symb. + 1/2.
The cumulated relative scores are a useful approach for comparing the performance across different scenarios (Figure 8). The baseline score in the BAU scenario had a relatively high ranking for both crop and livestock and performed relatively well in feed autonomy based on the relatively low livestock density, and relatively high grass-fed rations currently present in the Ribéracois. Scores increased progressively with the implementation of interventional scenarios, both through farm efficiency changes in BMP and collective solutions through farm network changes in EXCH and BIOGAS scenarios. The improvements of these three scenarios mainly concern nutrient management practices and recycling flows that helped to increase the N cycle coupling and reduce GHG emissions by increasing the number of flows in the network. In addition, BIOGAS produced the maximum bioenergy extractable from farming wastes. The crop-livestock symbiosis implemented in C-L Symb. by locally filling feed deficits (in this case for pulses) helped to increase feed autonomy and also provided GHG mitigation (linked to reduction on external feed). The Max. Circularity scenario obtained a similar score as in C-L Symb., thanks to a strong GHG mitigation potential, even though crop production decreased. Even though GHG mitigation was improved when combined reducing livestock populations and eliminating chemical fertilizers, the redesign scenarios performed better without reducing livestock as animals helped to sustain crop production and bioenergy.
Figure 8. Cumulated relative score in food production and circularity indicators of the scenarios. Scenarios names are presented in Figure 3 and Table 2.
We demonstrate how the FAN model can be applied as a useful tool for simulating exchanges and for anticipating co-benefits or unintended consequences under different scenarios. More precisely, material exchanges in FAN are quantified and have a direct geographic dimension (including truck distance transportation and other spatial components of the farm network). The crop, livestock, and bioenergy submodels are particularly useful to estimate food and energy production. In addition, the GHG balance, the N balance and the number of flows provide relatively straightforward indicators of the potential environmental outcomes of increased circularity at the regional scale.
Although the BMP scenario was very effective at reducing N losses at the farm scale, we observed that scenarios involving more material exchanges (i.e., EXCH, BIOGAS and C-L Symb) proved to be a good compromise for increasing the circularity of the system through the coordination of agents without radically modifying farming systems. Actually, only relatively small land-use changes via pulse crop introductions were necessary to connect crop and livestock in symbiosis (C-L Symb) in Ribéracois. Although local feed rations were approached based on the average in France and assumed to be optimized to meet livestock nutrition requirements, their global impact could be improved by substituting cereals and silage by grass and food wastes in a “low-cost livestock approach” (Van Zanten et al., 2018).
System redesign in the Max. Circularity scenarios illustrate the impact that fundamentally rethinking our agro-food systems could have for enhancing circularity and therefore minimizing the environmental impacts of farming. Nevertheless, some of these scenarios were considerably less productive not only for crop and livestock production but also for bioenergy. Important tradeoffs arose when decreasing livestock and removing chemical fertilizers, highlighting the importance of organic fertilization from livestock manure for the development of a circular agriculture. The trade-offs for organic management would be even more pronounced in farming regions specialized in crop production but may be lower in regions with high livestock densities. In our simulations, the livestock density in Ribéracois measured in Livestock Units (LU) after Eurostat (2013), was, on average, 0.62 LU/ha, slightly lower than the European average of 0.8 LU/ha for 2016 (Eurostat, 2019).
The application of our scenarios in Ribéracois may be discussed in terms of feasibility and facilitation. Despite the insights provided by our analysis, a key limitation is that no economic cost calculations are implemented, in part due to the fact that monetary values could change depending on public subsidies, such as those existing for biogas production in many countries (Börjesson and Ahlgren, 2012; Sun et al., 2014; Appel et al., 2016). In FAN simulations, however, economic data is implicit in the mechanisms underlying the exchanges even though there is no actual cost analysis, as discussed in more detail by Fernandez-Mena et al. (2019). We assumed that for implementing these practices and innovations famers will need support by public and engagement of private institutions.
We proposed several system circularity indicators that are easy to calculate through the application of a N balance, a C balance, a feed and forage balance and a count of number of flows. Indicators present limitations since several assumptions were made, in particular for N and C cycle. For instance, once losses were subtracted, all N was assumed to be available for crops and all catch-crops were assumed to performed identically. In the C balance, some emissions factors could be more precise to soils and materials, in particular for digestates volatilization. Avoided emissions by biogas production in France were quite low since nuclear power is the main source of electricity and would be around four times greater if references for Europe were considered instead (European Environment Agency, 2018). In addition, the average of C net sequestration in European grasslands is a discussed value that may vary among grasslands (Chang et al., 2017; Conant et al., 2017) and was assumed to be performed identically in scenarios where livestock were reduced. Finally, we calculated a relative score from these indicators that facilitated the comparison of scenarios and the understanding of their trade-offs. Nonetheless, this score was relative to the performance among the current scenarios and was cumulated considering that all scores counted the same, although production indicators may be considered to be more important.
A first step toward circularity will involve efforts on farmer individual behavior toward a more efficient nutrient management at the farm scale by choosing local materials, reasoning fertilization and feeding ratios and using catch crops. For that, farmers may be supported by independent agronomists and monitored by regulations penalizing nutrients overuse. Recycling for fertilization, bioenergy and local animal feeding need an important collective coordination. This coordination could be supported by the private farming sector either through collective engagements in groups, or by individuals connected through new digital technologies. Open source and private initiatives could focus on geo-locating farms on the landscape, informing their material needs and facilitating better communication among other farmers seeking to meet different types of material requirements. On top of that, biogas production through anaerobic digestion requires specific knowledge and important capital investment in equipment that may be supported by public subsidies and technical assistance. The leverages that substantially increased circularity were also those requiring the most fundamental farm transformations, i.e., livestock reduction and chemical fertilizers elimination. Nevertheless, both scenarios together present important tradeoffs for overall agro-food system productivity. Paradoxically, current food consumption tendencies in western countries may encourage these two opposed levers (Seconda et al., 2017; Christensen and Denver, 2018), either by eliminating animal proteins in diets or by promoting organic farm management. Larger-scale simulations may take into account human diets to more fully explore the compromises involved between changes in livestock populations and availability of organic fertility management in croplands.
In this study, we applied a network approach using FAN to examine system-wide outcomes of multiple types of scenarios with varying degrees of system transformation. Overall, we verified our hypotheses that circularity and therefore environmental performance can be improved along a gradient of scenarios requiring progressive management interventions. Our analysis provides a “proof of concept” for the implementation of circular scenarios in a French agro-food system, giving an insight based on multiple system components and indicators regarding how different sustainability solutions across a farming region could result in very different outcomes. Simulations from agent-based models like FAN could in turn help to inform the design of agricultural and environmental policies and regulations, particularly those that seek to bring about more fundamental changes in circular agro-food systems.
The FAN model and the Ribéracois datasets used in this study are available on Fernandez-Mena (2017a). Complementary data are available in request to the corresponding author.
SP, TN, and GM were co-supervisors of HF-M Ph.D. project. They were listed according to their participation on this article.
The authors declare that the research was conducted in the absence of any commercial or financial relationships that could be construed as a potential conflict of interest.
This study was part of HF-M doctoral dissertation at University of Bordeaux (Fernandez-Mena, 2017b). The work was funded by Bordeaux Sciences Agro (University of Bordeaux) and INRA (currently INRAE), Division of Environment and Agronomy. HF-M received financial support from Labex COTE (University of Bordeaux, France) and Agreenium consortium. The data about farming systems were collected thanks to the French National Research Agency (ANR) as part of the Investissements d'avenir program (reference: ANR-10-EQPX-17—Center d'accès sécurisé aux données—CASD).
The Supplementary Material for this article can be found online at: https://www.frontiersin.org/articles/10.3389/fsufs.2020.00041/full#supplementary-material
Acosta-Alba, I., Lopéz-Ridaura, S., van der Werf, H. M. G., Leterme, P., and Corson, M. S. (2012). Exploring sustainable farming scenarios at a regional scale: an application to dairy farms in Brittany. Work. More Sustain. Agri-Food Ind. Main Find. Food LCA 2010 Conf. Bari Italy 28, 160–167. doi: 10.1016/j.jclepro.2011.11.061
ADEME (2013). Bilan National des Projets Biogaz. Réalisé par J. Thual. Consulted on 1/03/2017. Available online at: https://www.ademe.fr/sites/default/files/assets/documents/bilan__national__methani~sation.pdf
ADEME (2017). Base Carbone ADEME. Version 2.17.0. Available online at: http://www.bilans-ges.ademe.fr/
Agreste (2013). Recensement Agricole 2010. Available online at: http://agreste.agriculture.gouv.fr/page-d-accueil/article/agreste-donnees-en-ligne (accessed April 28, 2019).
Agreste (2014). Analyse de Résultats de l'enquête Pratiques Culturales Grandes Cultures et Prairies 2011. Available online at: http://46.29.123.56/IMG/pdf/dossier21_fertilisation.pdf (accessed May 05, 2017 ).
Alonso-Betanzos, A., Sánchez-Maroño, N., Fontenla-Romero, O., Polhill, J. G., Craig, T., Pérez, J. B., et al. (2017). Agent-Based Modeling of Sustainable Behaviors. Cham: Springer. doi: 10.1007/978-3-319-46331-5
Appel, F., Ostermeyer-Wiethaup, A., and Balmann, A. (2016). Effects of the german renewable energy act on structural change in agriculture–the case of biogas. Util. Policy 41, 172–182. doi: 10.1016/j.jup.2016.02.013
Avadí, A., Nitschelm, L., Corson, M., and Vertès, F. (2016). Data strategy for environmental assessment of agricultural regions via LCA: case study of a French catchment. Int. J. Life Cycle Assess. 21, 476–491. doi: 10.1007/s11367-016-1036-6
Baumgart-Getz, A., Prokopy, L. S., and Floress, K. (2012). Why farmers adopt best management practice in the United States: a meta-analysis of the adoption literature. J. Environ. Manage. 96, 17–25. doi: 10.1016/j.jenvman.2011.10.006
Bichraoui, N., Guillaume, B., and Halog, A. (2013). Agent-based modelling simulation for the development of an industrial symbiosis-preliminary results. Procedia Environ. Sci. 17, 195–204. doi: 10.1016/j.proenv.2013.02.029
Billen, G., Le Noë, J., and Garnier, J. (2018). Two contrasted future scenarios for the French agro-food system. Sci. Total Environ. 637, 695–705. doi: 10.1016/j.scitotenv.2018.05.043
Bonaudo, T., Bendahan, A. B., Sabatier, R., Ryschawy, J., Bellon, S., Leger, F., et al. (2014). Agroecological principles for the redesign of integrated crop–livestock systems. Eur. J. Agron. 57, 43–51. doi: 10.1016/j.eja.2013.09.010
Börjesson, M., and Ahlgren, E. O. (2012). Cost-effective biogas utilisation–A modelling assessment of gas infrastructural options in a regional energy system. Energy 48, 212–226. doi: 10.1016/j.energy.2012.06.058
Bouwman, A., Beusen, A., Lassaletta, L., Van Apeldoorn, D., Van Grinsven, H., and Zhang, J. (2017). Lessons from temporal and spatial patterns in global use of N and P fertilizer on cropland. Sci. Rep. 7:40366. doi: 10.1038/srep40366
Campbell, B. M., Beare, D. J., Bennett, E. M., Hall-Spencer, J. M., Ingram, J. S., Jaramillo, F., et al. (2017). Agriculture production as a major driver of the Earth system exceeding planetary boundaries. Ecol. Soc. 22. doi: 10.5751/ES-09595-220408
Carlson, K. M., Gerber, J. S., Mueller, N. D., Herrero, M., MacDonald, G. K., Brauman, K. A., et al. (2016). Greenhouse gas emissions intensity of global croplands. Nat. Clim. Change. 7, 63–68. doi: 10.1038/nclimate3158
Chang, J., Ciais, P., Viovy, N., Soussana, J.-F., Klumpp, K., and Sultan, B. (2017). Future productivity and phenology changes in European grasslands for different warming levels: implications for grassland management and carbon balance. Carbon Balance Manag. 12, 11. doi: 10.1186/s13021-017-0079-8
Chang, J., Ciais, P., Viovy, N., Vuichard, N., Herrero, M., Havlík, P., et al. (2016). Effect of climate change, CO2 trends, nitrogen addition, and land-cover and management intensity changes on the carbon balance of European grasslands. Glob. Change Biol. 22, 338–350. doi: 10.1111/gcb.13050
Christensen, T., and Denver, S. (2018). “Do Danish consumers prefer an organic vegetarian meal or a non-organic meaty alternative? Evidence from a choice experiment,” in Paper at: International Food Marketing Research Symposium (Bournemouth).
Cobo, S., Levis, J. W., Dominguez-Ramos, A., and Irabien, A. (2019). Economics of enhancing nutrient circularity in an organic waste valorization system. Environ. Sci. Technol. 53, 6123–32. doi: 10.1021/acs.est.8b06035
Conant, R. T., Cerri, C. E., Osborne, B. B., and Paustian, K. (2017). Grassland management impacts on soil carbon stocks: a new synthesis. Ecol. Appl. 27, 662–668. doi: 10.1002/eap.1473
Davis, S. C., Kauneckis, D., Kruse, N. A., Miller, K. E., Zimmer, M., and Dabelko, G. D. (2016). Closing the loop: integrative systems management of waste in food, energy, and water systems. J. Environ. Stud. Sci. 6, 11–24. doi: 10.1007/s13412-016-0370-0
de Boer, I. J., and van Ittersum, M. K. (2018). Circularity in Agricultural Production. Wageningen: Wageningen University & Research.
Devun, J., and Guinot, C. (2012). Alimentation des bovins: rations Moyennes et Autonomie Alimentaire. Collection Résultats. CR 0, 005.
DREAL (2016). Quelles sont les zones vulnérables en Nouvelle-Aquitaine? Available online at: http://www.nouvelle-aquitaine.developpement-durable.gouv.fr/quelles-sont-les-zones-vulnerables-en-nouvelle-a1766.html (accessed October 10, 2017).
Edouard, N., Hassouna, M., Robin, P., and Faverdin, P. (2016). Low degradable protein supply to increase nitrogen efficiency in lactating dairy cows and reduce environmental impacts at barn level. Animal 10, 212–220. doi: 10.1017/S1751731115002050
El-Chichakli, B., von Braun, J., Lang, C., Barben, D., and Philp, J. (2016). Five cornerstones of a global bioeconomy. Nature 535, 221–223. doi: 10.1038/535221a
Fan, W., Dong, X., Wei, H., Weng, B., Liang, L., Xu, Z., et al. (2018). Is it true that the longer the extended industrial chain, the better the circular agriculture? A case study of circular agriculture industry company in Fuqing, Fujian. J. Clean. Prod. 189, 718–728. doi: 10.1016/j.jclepro.2018.04.119
Fernandez-Mena, H. (2017a). “FAN Model Dataset”, Mendeley Data, v1. Available online at: http://dx.doi.org/10.17632/sytdc5yscj.1
Fernandez-Mena, H. (2017b). Modelling biomass and nutrient flows in agro-food systems at the local scale: scenario simulation and assessment in a French case-study (Ph.D. thesis). University of Bordeaux, Bordeaux, France.
Fernandez-Mena, H., Gaudou, B., Pellerin, S., MacDonald, G. K., and Nesme, T. (2019). Flows in Agro-food Networks (FAN): An agent-based model to simulate local agricultural material flows. Agric. Syst. 102718. doi: 10.1016/j.agsy.2019.102718
Fernandez-Mena, H., Nesme, T., and Pellerin, S. (2016). Towards an Agro-Industrial Ecology: a review of nutrient flow modelling and assessment tools in agro-food systems at the local scale. Sci. Total Environ. 543, 467–479. doi: 10.1016/j.scitotenv.2015.11.032
Foley, J. A., Ramankutty, N., Brauman, K. A., Cassidy, E. S., Gerber, J. S., Johnston, M., et al. (2011). Solutions for a cultivated planet. Nature 478, 337–342. doi: 10.1038/nature10452
Garcia, M. (2016). Analyse de flux de matières et de nutriments entre les fermes et ses partenaires dans deux cas d'études en France: le Ribéracois et les Vosges. Mémoire fin d'études (ENSAT Toulouse), réalisé à l'INRA de Bordeaux-Aquitaine.
Gaube, V., Kaiser, C., Wildenberg, M., Adensam, H., Fleissner, P., Kobler, J., et al. (2009). Combining agent-based and stock-flow modelling approaches in a participative analysis of the integrated land system in Reichraming, Austria. Landsc. Ecol. 24, 1149–1165. doi: 10.1007/s10980-009-9356-6
Giller, K. E., Tittonell, P., Rufino, M. C., van Wijk, M. T., Zingore, S., Mapfumo, P., et al. (2011). Communicating complexity: Integrated assessment of trade-offs concerning soil fertility management within African farming systems to support innovation and development. Methods Tools Integr. Assess. Sustain. Agric. Syst. Land Use 104, 191–203. doi: 10.1016/j.agsy.2010.07.002
Giuliano, S., Ryan, M. R., Véricel, G., Rametti, G., Perdrieux, F., Justes, E., et al. (2016). Low-input cropping systems to reduce input dependency and environmental impacts in maize production: a multi-criteria assessment. Eur. J. Agron. 76, 160–175. doi: 10.1016/j.eja.2015.12.016
Golembiewski, B., Sick, N., and Bröring, S. (2015). The emerging research landscape on bioeconomy: What has been done so far and what is essential from a technology and innovation management perspective? Innov. Food Sci. Emerg. Technol. 29, 308–317. doi: 10.1016/j.ifset.2015.03.006
Grillot, M., Vayssières, J., and Masse, D. (2018). Agent-based modelling as a time machine to assess nutrient cycling reorganization during past agrarian transitions in West Africa. Agric. Syst. 164, 133–151. doi: 10.1016/j.agsy.2018.04.008
Groupe Régionale d'Expertise Nitrates (GREN) (2016). de la Nouvelle-Aquitaine. Available online at: http://www.nouvelle-aquitaine.developpement-durable.gouv.fr/le-groupe-regional-d-expertise-nitrates-gren-a1768.html (accessed April 10, 2018).
I.P.C.C. (2006). IPCC Guidelines for National Greenhouse Gas Inventories, Prepared by the National Greenhouse Gas Inventories Programme, Eggleston. Hayama: IGES.
Lamine, C. (2011). Transition pathways towards a robust ecologization of agriculture and the need for system redesign. Cases from organic farming and IPM. J. Rural Stud. 27, 209–219. doi: 10.1016/j.jrurstud.2011.02.001
Lassaletta, L., Billen, G., Grizzetti, B., Garnier, J., Leach, A. M., and Galloway, J. N. (2014). Food and feed trade as a driver in the global nitrogen cycle: 50-year trends. Biogeochemistry 118, 225–241. doi: 10.1007/s10533-013-9923-4
Le Noë, J., Billen, G., and Garnier, J. (2017). How the structure of agro-food systems shapes nitrogen, phosphorus, and carbon fluxes: The generalized representation of agro-food system applied at the regional scale in France. Sci. Total Environ. 586, 42–55. doi: 10.1016/j.scitotenv.2017.02.040
Leip, A., Billen, G., Garnier, J., Grizzetti, B., Lassaletta, L., Reis, S., et al. (2015). Impacts of European livestock production: nitrogen, sulphur, phosphorus and greenhouse gas emissions, land-use, water eutrophication and biodiversity. Environ. Res. Lett. 10:115004. doi: 10.1088/1748-9326/10/11/115004
Lemaire, G., Franzluebbers, A., de Faccio Carvalho, P. C., and Dedieu, B. (2014). Integrated crop–livestock systems: strategies to achieve synergy between agricultural production and environmental quality. Agric. Ecosyst. Environ. 190, 4–8. doi: 10.1016/j.agee.2013.08.009
Manlay, R. J., Chotte, J.-L., Masse, D., Laurent, J.-Y., and Feller, C. (2002). Carbon, nitrogen and phosphorus allocation in agro-ecosystems of a West African savanna: III. Plant and soil components under continuous cultivation. Agric. Ecosyst. Environ. 88, 249–269. doi: 10.1016/S0167-8809(01)00220-1
Mao, C., Feng, Y., Wang, X., and Ren, G. (2015). Review on research achievements of biogas from anaerobic digestion. Renew. Sustain. Energy Rev. 45, 540–555. doi: 10.1016/j.rser.2015.02.032
Marchand, F., Debruyne, L., Triste, L., Gerrard, C., Padel, S., and Lauwers, L. (2014). Key characteristics for tool choice in indicator-based sustainability assessment at farm level. Ecol. Soc. 19:46. doi: 10.5751/ES-06876-190346
Matthews, R. B., Gilbert, N. G., Roach, A., Polhill, J. G., and Gotts, N. M. (2007). Agent-based land-use models: a review of applications. Landsc. Ecol. 22, 1447–1459. doi: 10.1007/s10980-007-9135-1
Metson, G. S., MacDonald, G. K., Haberman, D., Nesme, T., and Bennett, E. M. (2016). Feeding the corn belt: opportunities for phosphorus recycling in US agriculture. Sci Total Env. 542, 1117–1126. doi: 10.1016/j.scitotenv.2015.08.047
Mitchell, M., Bennett, E., Gonzalez, A., Lechowicz, M., Rhemtulla, J., Cardille, J., et al. (2015). The Montérégie Connection: linking landscapes, biodiversity, and ecosystem services to improve decision making. Ecol. Soc. 20:15. doi: 10.5751/ES-07927-200415
Moraine, M., Duru, M., and Therond, O. (2017a). A social-ecological framework for analyzing and designing integrated crop–livestock systems from farm to territory levels. Renew. Agric. Food Syst. 32, 43–56. doi: 10.1017/S1742170515000526
Moraine, M., Mélac, P., Ryschawy, J., Duru, M., and Therond, O. (2017b). A participatory method for the design and integrated assessment of crop-livestock systems in farmers' groups. Ecol. Indic. 72, 340–351. doi: 10.1016/j.ecolind.2016.08.012
Ni, K., Pacholski, A., Gericke, D., and Kage, H. (2012). Analysis of ammonia losses after field application of biogas slurries by an empirical model. J. Plant Nutr. Soil Sci. 175, 253–264. doi: 10.1002/jpln.201000358
Nowak, B., Nesme, T., David, C., and Pellerin, S. (2015). Nutrient recycling in organic farming is related to diversity in farm types at the local level. Agric. Ecosyst. Environ. 204, 17–26. doi: 10.1016/j.agee.2015.02.010
Pellerin, S., Bamière, L., Angers, D., Béline, F., Benoit, M., Butault, J.-P., et al. (2017). Identifying cost-competitive greenhouse gas mitigation potential of French agriculture. Environ. Sci. Policy 77, 130–139.
Pelzer, E., Fortino, G., Bockstaller, C., Angevin, F., Lamine, C., Moonen, C., et al. (2012). Assessing innovative cropping systems with DEXiPM, a qualitative multi-criteria assessment tool derived from DEXi. Ecol. Indic. 18, 171–182. doi: 10.1016/j.ecolind.2011.11.019
Prado, A. D., Mas, K., Pardo, G., and Gallejones, P. (2013). Modelling the interactions between C and N farm balances and GHG emissions from confinement dairy farms in northern Spain. Sci. Total Environ. 465, 156–165. doi: 10.1016/j.scitotenv.2013.03.064
Seconda, L., Baudry, J., Allès, B., Hamza, O., Boizot-Szantai, C., Soler, L.-G., et al. (2017). Assessment of the sustainability of the Mediterranean diet combined with organic food consumption: an individual behaviour approach. Nutrients 9:61. doi: 10.3390/nu9010061
Sun, D., Bai, J., Qiu, H., and Cai, Y. (2014). Impact of government subsidies on household biogas use in rural China. Energy Policy 73, 748–756. doi: 10.1016/j.enpol.2014.06.009
Tilman, D., and Clark, M. (2014). Global diets link environmental sustainability and human health. Nature 515, 518–522. doi: 10.1038/nature13959
Tittonell, P., Van Wijk, M., Herrero, M., Rufino, M., de Ridder, N., and Giller, K. (2009). Beyond resource constraints–Exploring the biophysical feasibility of options for the intensification of smallholder crop-livestock systems in Vihiga district, Kenya. Agric. Syst. 101, 1–19. doi: 10.1016/j.agsy.2009.02.003
Tomich, T. P., and Scow, K. M. (2016). The California Nitrogen Assessment: Challenges and Solutions for People, Agriculture, and the Environment. Oakland, CA: University of California Press.
Toop, T. A., Ward, S., Oldfield, T., Hull, M., Kirby, M. E., and Theodorou, M. K. (2017). AgroCycle–developing a circular economy in agriculture. Energy Procedia 123, 76–80. doi: 10.1016/j.egypro.2017.07.269
Tseng, M.-L., Chiu, A. S., Chien, C.-F., and Tan, R. R. (2019). Pathways and barriers to circularity in food systems. Resour. Conserv. Recycl. 143, 236–237. doi: 10.1016/j.resconrec.2019.01.015
Valkama, E., Lemola, R., Känkänen, H., and Turtola, E. (2016). “Catch crops as universal and effective method for reducing nitrogen leaching loss in spring cereal production: a meta-analysis,” in Presented at the EGU General Assembly Conference Abstracts, 1934.
Van Zanten, H. H., Herrero, M., Van Hal, O., Röös, E., Muller, A., Garnett, T., et al. (2018). Defining a land boundary for sustainable livestock consumption. Glob. Change Biol. 24, 4185–4194. doi: 10.1111/gcb.14321
Vega-Quezada, C., Blanco, M., and Romero, H. (2017). Synergies between agriculture and bioenergy in Latin American countries: a circular economy strategy for bioenergy production in Ecuador. New Biotechnol. 39, 81–89. doi: 10.1016/j.nbt.2016.06.730
Wery, J., Affholder, F., Belhouchette, H., Bergez, J.-E., Blazy, J. M., and Thérond, O. (2018). Farm-Centered Integrated Modelling for the Design of Sustainable Agricultural Systems. Pl-I-01. Agroscope.
Keywords: material flows, circular economy, recycling, bioeconomy, agroecology
Citation: Fernandez-Mena H, MacDonald GK, Pellerin S and Nesme T (2020) Co-benefits and Trade-Offs From Agro-Food System Redesign for Circularity: A Case Study With the FAN Agent-Based Model. Front. Sustain. Food Syst. 4:41. doi: 10.3389/fsufs.2020.00041
Received: 31 January 2020; Accepted: 26 March 2020;
Published: 28 April 2020.
Edited by:
Agustin Del Prado, Basque Centre for Climate Change, SpainReviewed by:
Le Noë Julia, University of Natural Resources and Life Sciences Vienna, AustriaCopyright © 2020 Fernandez-Mena, MacDonald, Pellerin and Nesme. This is an open-access article distributed under the terms of the Creative Commons Attribution License (CC BY). The use, distribution or reproduction in other forums is permitted, provided the original author(s) and the copyright owner(s) are credited and that the original publication in this journal is cited, in accordance with accepted academic practice. No use, distribution or reproduction is permitted which does not comply with these terms.
*Correspondence: Hugo Fernandez-Mena, aHVnby5mZXJuYW5kZXotbWVuYUBzdXBhZ3JvLmZy
Disclaimer: All claims expressed in this article are solely those of the authors and do not necessarily represent those of their affiliated organizations, or those of the publisher, the editors and the reviewers. Any product that may be evaluated in this article or claim that may be made by its manufacturer is not guaranteed or endorsed by the publisher.
Research integrity at Frontiers
Learn more about the work of our research integrity team to safeguard the quality of each article we publish.