- Egg Safety and Quality Research Unit, United States Department of Agriculture, Agricultural Research Service, U.S. National Poultry Research Center, Athens, GA, United States
The poultry farm environment plays a key role in the microbial colonization of chickens during production, which shapes what enters the processing and final retail environments. The aim of this study was to evaluate the effect of the farm environment on the microbial composition of pasture-raised broilers using a combined cultural and microbiomic farm-to-fork. To achieve this, two nearby pastured poultry farms raised small flocks of Freedom Ranger broilers obtained from the same hatchery flock and fed the same diet throughout live production. The major differences between the two farms were the physical farm environment, the method of feather removal during processing (scalding vs. skinning), and the storage conditions of carcasses before customers received final product (refrigeration vs. freezing). Microbiomes were compared from fecal and soil samples (live production), ceca (processing), and whole carcass rinses (processing, final product) to determine what effect the physical farm environment had on the poultry-related microbiomes. Overall, microbiomes in feces (p < 0.04), soil (p < 0.02), and ceca (p < 0.02) samples from farm 1 harbored a higher taxonomic richness than farm 2. Beta-diversity analysis demonstrated significant differences between the broiler microbiomes of the two farms for samples collected at the live production (p < 0.04) and processing stages (p < 0.01), but not for final product carcass rinses. At the early live production stage (~3 weeks old), fecal microbiomes from farm 1 were positively correlated to aluminum, iron, manganese, silicon and zinc concentrations in feces but not fecal microbiota from farm 2. At the late live production stage (~12 weeks old), fecal microbiomes from both farms were no longer correlated to mineral content of feces but were negatively correlated to fecal pH. Given that the farm environment itself was the major difference, the results show that even when raising the same breed fed the same diet, poultry farms have their own ecology that shape the composition of the poultry-related microbiomes. Therefore, it is vital that future work focuses on elucidating the farm environmental variables that have the greatest influence on these microbiomes, thus allowing for eventual targeted interventions to better manage these microbial populations to benefit animal, environmental, and public health.
Introduction
Microbial communities associated with live poultry are of major importance because they directly impact animal health, food safety and public health (Dupont, 2007; EFSA European Centre for Disease Prevention Control, 2015). Lately, the ecology of the gastrointestinal tract (GIT) microbiome has been specifically studied due to its key role in poultry production performance (Stanley et al., 2014; Apajalahti and Vienola, 2016). The composition of gut microbiota is known to affect many host functions relative to the growth and development of chicken, including nutrient utilization and gut epithelium nourishment (Pan and Yu, 2014) and also to influence the gut immune system and consequently resistance against microbial infections (Schokker et al., 2017).
The GIT of poultry hosts a complex and dynamic bacterial microbiota (Zhu et al., 2002). Considerable variation in poultry GIT microbial community composition has been observed both within and across studies (Stanley et al., 2013; Waite and Taylor, 2014); consequently, it's difficult to define the normal gut microbiota composition and to compare results between the different studies. While a part of this microbial variation may be attributed to technical factors such as sampling procedures, DNA extraction, the choice of PCR primers and corresponding genomic region to be sequenced and the sequencing platforms used (Laukens et al., 2015), the variation in microbial composition may also be explained by different host characteristics and environmental factors (Kers et al., 2018). Poultry-intrinsic host factors identified to influence the composition of intestinal microbiota include birds age (Ballou et al., 2016; Pedroso et al., 2016), sex (Torok et al., 2013; Zhao et al., 2013), type/breed (Videnska et al., 2014; Kim et al., 2015), and GIT regions (Yeoman et al., 2012). Of the external factors that influence the microbiota composition, the diet composition (Pan and Yu, 2014; Walugembe et al., 2015) and the use of feed additives (Videnska et al., 2013; Costa et al., 2017) have been well studied. Environmental factors such as the hygiene levels within the hatcheries (Stanley et al., 2013), the type of housing (Ludvigsen et al., 2016) and production system (Bjerrum et al., 2006; Xu et al., 2016), the litter quality and management (Torok et al., 2009; Dumas et al., 2011) as well as the climate and geographical locations (Videnska et al., 2014; Zhou et al., 2016) are also known to have an effect on the intestinal microbiota.
In the poultry production chain, newly-hatched chicks already have a GIT microbiota at the pre-hatched phase acquired directly from the mother or from the environment surrounding the eggs (Roto et al., 2016). During the first week of chick's life, the diversity of GIT microbiota increases gradually to reach the hundreds to thousands of distinct GIT taxa found in market age adults (Crhanova et al., 2011; Danzeisen et al., 2011; Ballou et al., 2016). In young birds, a high variation is observed in individual microbiota composition compared to older birds from the same flock (Crhanova et al., 2011; Ballou et al., 2016). This observation suggests that chicks might initially be randomly colonized by bacterial species present in their close environment during their early life (from 0 to 20 days), leading to a great individual diversity in microbiota composition which tend to converge in older individuals from the same flocks.
The chicken GIT microbiome commonly contains several taxa capable of causing significant illnesses in humans, most importantly Campylobacter and Salmonella. Pathogen colonization of live poultry can occur at all stages of the production. Newly-hatched chicks are more susceptible to pathogen colonization because they lack mature gut microbiota or feed in the alimentary tract (Nisbet et al., 1993). This colonization occurs by horizontal transfers between the farm environment and chickens (Patriarchi et al., 2011; Hermans et al., 2012) and also among chickens in a flock (Shanker et al., 1990; Byrd et al., 1998).
The surrounding environment of young chickens at the hatchery, during the transport to the farm or at the farm, has a decisive impact on the composition of microbial communities, including pathogenic bacteria, that colonize their intestinal tracts. Data describing the impact of environmental factors on poultry microbiome are scarce and no study to date has investigated the effect of the farm environment as a whole on the microbiota composition of broilers. Therefore, we investigated how the global effect of the farm environment, including management practices, influenced the microbiota of pasture-raised broilers along the production chain. Two all pasture-raised, antibiotic free farms located in the north-central Georgia raised the same breed of broiler with the same diet and a variety of samples were recovered along the entire farm-to-fork continuum. Illumina MiSeq-based 16S rRNA-based sequencing was used to compare the bacterial microbiomes between these two farms from soil and feces samples collected at the live production stage; ceca and carcass rinses samples collected during the processing step; and carcass rinse obtained from final product, to better elucidate the potential environmental and management drivers of the microbial ecology of pastured broilers.
Materials and Methods
Flock Management
This study was conducted in two pasture-raised broiler farms located in the north-central Georgia, United States from March to June 2014. One day old Freedom Ranger type chicks were purchased from the same hatchery flock (Freedom Ranger Hatchery, Reinholds, PA, USA) and were randomly split in two groups of 50 individuals between farm 1 and farm 2. While many similar management practices were used by both farms, the major differences are listed in Table 1. During the brooding period (0–~3.5 weeks of age), chicks at both farms were reared under heat lamps with bedding material composed of wood shavings (with fresh shaving added weekly on top of the existing bedding) and chicks were fed with the DuMOR Chick Starter/Grower 20% (D20). At ~3.5 weeks of age, young broilers were transferred to pasture and were fed with the DuMor 16% Crumbles (D16) under modified Salatin-type chicken tractor housing systems (2 houses per farm with ~25 birds per house). During live production, broilers were provided feed and water ad libitum, and the chicken tractors were moved onto a fresh piece of pasture every day. As broilers increased in size, plastic temporary fencing was placed around the tractors to allow for increased grazing area, and this fencing was moved daily with the houses. At ~13 weeks of age, broilers were processed on-farm for both farms, but farm 1 de-feathered the carcasses by removing the skin (no scalding step used) and air chilled the carcasses, while farm 2 used a traditional scalding step followed by de-feathering using an automated picker (leaving the skin on the carcass) and water chilled the carcasses. Final products as well have a different storage period and temperature (see Table 1).
Sample Collection and Preparation
Soil and feces samples were collected from the pasture where the flock was currently residing at the time of sampling. Samplings occurred three times during grow-out: (i) within a few days of being placed in the pasture (T1), (ii) halfway through their time on pasture (T2), and (iii) on the day the flock was processed (T3). At each sampling time, the pasture area was divided into five separate sections, and five subsamples in each section were pooled into a single sample for each section (a total of five soil samples and five feces samples were collected on each sampling day). Soil samples were collected from the surface (0–7 cm) with sterile scoops, and feces samples were collected from fresh droppings on the soil surface. Gloves and scoops were changed between sample types and between sampling areas.
During the on-farm processing day, ceca and carcass rinse samples were collected. Upon evisceration, cecal sacs from five carcasses were removed and placed into a single sampling bag to create a pooled sample. A total of five pooled samples (n = 5) was created. Gloves and scissors were changed between each pooled sample. Prior to packaging and storage of the carcasses for the consumer, each of the 25 carcasses were placed in sterile plastic bags, rinsed with 100 ml of 10 mM phosphate-buffered saline (PBS) and vigorously shaken for 1 min. Whole carcass rinses (WCR) from five carcasses were pooled together in a filtered stomacher bag creating five pooled samples (n = 25). Carcasses were returned to the farmer to be packed and stored according to the usual procedure applied at the farm. Upon receiving the final product, the procedure described above was repeated to obtain the final product WCR samples.
All fecal, soil, cecal, and WCR samples were transported back to the laboratory on ice and processed within 2 h of collection. To prepare the environmental samples for homogenization, 3 g (feces, soil) or 5 ceca were combined within filtered stomacher bags (Seward Laboratory Systems, Inc., Davie, FL), and diluted 1:3 using 10 mM phosphate-buffered saline (PBS). For the WCR, 100-ml of 10 mM PBS were added to each carcass within the storage bag, and the bags were vigorously shaken for 60 s. Five WCR were pooled into a single filtered stomaching bag, and this was repeated a total of 5 times (N = 25 carcass rinses). No further dilution in 10 mM PBS was required for the WCR samples. All samples were homogenized for 60 s and these homogenates were used for all downstream cultural isolations, and the cecal homogenates and WCR rinsates were used for DNA extraction.
Cultural Isolation Methods
Salmonella spp.
As a pre-enrichment step, the stomached homogenates remained in the filtered stomacher bags and incubated overnight at 35°C. Two different enrichments broths were used to isolate Salmonella spp. from these environmental samples: Tetrathionate (TT; Becton-Dickinson, Sparks, MD) broth and Rappaport-Vassiliadis (RV; Becton Dickinson) media. After overnight incubation at 42°C in both of these enrichment broths, 1 loopful from each enrichment broth was spread on two different differential media: Brilliant Green Sulfa with novobiocin (BGS; Becton Dickinson) agar and xylose lysine tergitol-4 (XLT-4; Becton Dickinson) agar. These plates were incubated overnight at 35°C, and on each plate, 3 Salmonella–like colonies per subsample were picked and confirmed using triple sugar iron agar (TSI; Becton-Dickinson) and lysine iron agar fermentation (LIA; Becton-Dickinson) using an incubation period of 18–24 h at 35°C. Final confirmation of suspect TSI/LIA isolates was performed using Salmonella polyvalent O antiserum agglutination (Becton-Dickinson), using manufacturer's specifications. Positive salmonellae were serogrouped using individual Salmonella poly O antisera for O groups A through I, following the Kauffman-White scheme (Popoff and Le Minor, 1997).
Campylobacter spp.
Campylobacter spp. were isolated using a selective (Cefex) and non-selective (Campycheck) method. For the selective media method, the recovery of Campylobacter spp. from homogenized samples was performed as previously described (Stern et al., 1992). Initially, 100 μL of homogenized suspension was removed, plated onto Campy-Cefex agar, and subsequently incubated at 42 ± 1°C for 36–48 h in a microaerobic atmosphere (5% O2, 10% CO2, 85% N2). Putative Campylobacter spp. colonies were enumerated, and up to 5 colonies per sample were sub-cultured on Brucella agar supplemented with 10% laked horse blood (BAB plates) for isolation and incubated as previously described. For the Campycheck method (Lastovica and Le Roux, 2001), homogenized samples were applied to non-selective plates using a filtration technique and grown in a hydrogen-enriched atmosphere. Briefly, a 50 mm, 0.6 μm mixed cellulose ester filter (Whatman, Schleicher & Schuell; Dassel, Germany) was aseptically placed in the center of a Brucella agar plate supplemented with Campylobacter growth supplement SR84 (Oxoid/Remel, Lenexa, KS) and 10% laked horse blood (Oxoid/Remel). Four 50 μL aliquots of homogenized fecal sample were applied at distinct locations on the filter and allowed to sit at room temperature for 15 min. The filter was aseptically removed, using sterile tweezers, and the plate placed in a ZipTop bag that was subsequently filled with an atmosphere of 7.5% H2, 2.5% O2, 10% CO2, and 80% N2 and incubated at 37°C for 48 h. Several putative Campylobacter spp. colonies were sub-cultured on Brucella agar supplemented with 10% laked horse blood (BAB plates) and subsequently stored at −80°C in 16% glycerol stocks for further identification and analyses.
Listeria spp.
As a pre-enrichment step, the stomached homogenates remained in the filtered stomacher bags and was incubated overnight at 35°C. This pre-enrichment was followed by two subsequent enrichments in UVM Modified Listeria Enrichment Broth (UVM, Becton-Dickinson) and Fraser Broth (Becton-Dickinson), both requiring an overnight incubation period at 30°C. One loopful of the Fraser's enrichment was streaked for isolation of Listeria-selective agar (Becton-Dickinson). These plates were incubated overnight at 30°C, and on each plate, 3 Listeria–like colonies per positive subsample were picked and confirmed as Listeria using the appropriate BAX PCR assay (DuPont, Wilmington, DE). Listeria species and L. monocytogenes serovars of Listeria-like colonies were determined using multiplex-PCR as described previously (Locatelli et al., 2017b).
Escherichia coli
Recovery of E. coli was performed by spreading 1 mL of the homogenates onto Petrifilm E.coli/Coliform Count Plates (3M, St. Paul, MN) and incubated overnight at 37°C. Blue colonies with associated gas production indicative of E. coli and all blue and red colonies with entrapped gas were counted as coliforms, and up to 5 E.coli colonies per sample were isolated and used for further characterization.
All quantifiable cultural data (E. coli, and Campylobacter isolated on CEFEX) were normalized via log10-transformation prior to any statistical comparisons. A one-way ANOVA followed by Tukey's post hoc test was carried out to compare the E. coli and Campylobacter counts (mean log10 CFU/ml) between the 2 farms for each sample type.
Fecal and Soil Physiochemical Analysis
The moisture content of the fecal and soil samples was determined by drying overnight at 65°C and calculating the difference between the wet and dried weights of the litter. Fecal and soil pH and electrical conductivity (EC) were determined using an Orion Versa Star Advanced Electrochemistry Meter (ThermoScientific) using a 1:5 dilutions in distilled water. Fecal and soil samples were submitted to the University of Georgia Soils Testing Laboratory for Total C, Total N, and elemental (Al, As, B, Ca, Cd, Cr, Cu, Fe, K, Mg, Mn, Mo, Na, Ni, P, Pb, S, Si, Zn) composition.
DNA Extraction and Quantification
DNA extractions were performed on 0.33 g of feces, 0.33 g of soil, and 0.5 ml of cecal homogenate and 0.5 ml of WCR. DNA was extracted from samples according to a semi-automated hybrid DNA extraction protocol previously described (Rothrock et al., 2014). This method was a combination of a mechanical method using the FastDNA Spin Kit for Feces (MP Biomedicals, Solon, OH, USA) and an enzymatic method based on the QIAamp DNA Stool Mini Kit (QIAGEN, Valencia, CA). DNA purification was performed using the DNA Stool—Human Stool—Pathogen Detection Protocol of the QIAcube Robotic Workstation. After purification, the DNA concentration in each sample was determined spectrophotometrically using the Take3 plate in conjunction with the Synergy H4 multimode plate reader (BioTek, Winooski, VT).
Illumina MiSeq Library Construction and Analyses
Library construction and sequencing were performed by the Earth Microbiome Project Laboratory at the U.S. Department of Energy, Argonne National Laboratory (Argonne, IL). In short, the hypervariable V4 domain of bacterial 16S rRNA gene was amplified using the F515 (5′-CACGGTCGKCGGCGCCATT-3′) and R806 (5′-GGACTACHVGGGTWTCT AAT-3′) primer set with each primer containing Illumina adapter regions (Illumina, Inc., San Diego, CA) and the reverse primer containing the Golay barcodes to facilitate multiplexing (Caporaso et al., 2011). Raw reads were obtained by using the Illumina MiSeq platform. A total of 3,297,242 raw sequence reads were generated and processed by the QIIME v1.9.1 (Quantitative Insights Into Microbial Ecology) pipeline (Caporaso et al., 2010b). Quality filtering and library splitting according to the Golay barcode sequences were performed on the R1 read (split_library_fastq.py script, default parameters). Sequences were chimera checked against the gold.fa database (http://drive5.com/uchime/gold.fa) and clustered into Operational Taxonomic Units (OTUs) according to their sequence similarity (97%) using the usearch option (Edgar, 2010) with pick_otus.py script (-m usearch, all other parameters were default). A representative sequence for each OTU was selected with pick_rep_set.py script (using the most_abundant method for picking, all other parameters were default) and used for taxonomic assignment using UCLUST and the Greengenes 13_8 database (Desantis et al., 2006) with assign_taxonomy.py (default parameters). Sequences were aligned (align_seqs.py script, default parameters) using PyNAST (Caporaso et al., 2010a) and filtered (filter_alignment.py, default parameters). A phylogenetic tree was subsequently produced with the make_phylogeny.py script (with default settings and FastTree program). Among the 96 samples analyzed, 5 were removed because they were composed of <100 reads. Finally, a total of 2,557,191 sequences (average of 28,101 sequences/sample) were obtained for further analysis. Overall, among all samples, a total of 1,939 unique OTUs were identified. For all subsequent analyses, sequences were analyzed according to the sample type (feces, soil, ceca, processing carcass rinse and final product carcass rinse).
Alpha diversity was used to describe the microbial richness, evenness and diversity within samples using the Chao1, Equitability and Shannon metrics, respectively. For each sample type, significant differences in alpha diversity parameters were tested between the 2 farms using the compare alpha diversity.py script. To determine β-diversity, the Bray-Curtis distance was used to measure the dissimilarity based on the rarefied OTU table. Data visualization and statistical tests were performed in R (v3.4.3) using the vegan package v2.4-5. Whole bacterial community composition was examined using nonmetric multidimensional scaling (NMDS) of Bray-Curtis dissimilarities with the metaMDS function. The function envfit was used to calculate the regression statistic for fecal and soil physiochemical variables on ordination scores at a p-value ≤ 0.01. Analysis of similarities (ANOSIM) was used to examine the significant differences in community structures between the 3 sampling times for feces and soil samples and between the 2 farms for each sample type. A nonparametric Kruskal-Wallis test with the Dunn's post hoc test was carried out to compare the mean relative abundance of each taxa (phylum and genus) between the 2 farms at each sampling time and for each sample type.
Results and Discussion
Poultry Microbiome at the Live Production Stage
The effect of the farm environment on the pastured poultry microbiome was first evaluated at the live production stage by investigating the bacterial community structure in fecal and pasture soil samples collected at the beginning of their time on pasture (~4 weeks old; T1), at ~8 weeks of age (T2) and on the day of processing (~12 weeks old; T3). Overall, feces samples in farms 1 and 2 were dominated by Firmicutes (70.5 vs. 69.7%), Proteobacteria (15.1 vs. 25.8%), Actinobacteria (6.7 vs. 2.4%), and Bacteroidetes (5.2 vs. 1.9%), which are all phyla that are known to dominate chicken fecal microbiota (Singh et al., 2012; Videnska et al., 2013; Locatelli et al., 2017a). Fecal and soil community composition, based on β-diversity using the Bray-Curtis dissimilarity distances, significantly varied over time for both farms (p < 0.01, Figure 1). As a result, bacterial composition data from the three sampling times were treated independently. The farm environment had a significant effect on fecal microbiome at all three sampling times (Figure 1A), with the farm effect being the strongest during T1 (R = 1, p = 0.02) and T2 (R = 0.916, p = 0.01) and being the weakest (but still significant) at T3 (R = 0.381, p = 0.04) according to ANOSIM analyses. Fecal α-diversity was not as greatly affected by farm environment though, with only one of the three metrics showing significant differences (richness; Table 2) but without a consistent trend between farms (greater for farm 1 at T1 and T2, greater for farm 2 at T3). As expected, soil microbiomes were significantly different between the 2 farms at T1 (R = 0.59, p = 0.012), T2 (R = 1, p = 0.009), and T3 (R = 0.926, p = 0.007) (Figure 1B), and like with the fecal microbiomes, there were some significant differences between farms for α-diversity metrics, but no consistent trends (richness higher for farm 1 at T1, T3, but diversity and evenness greater for farm 2 at T2; Table 2).
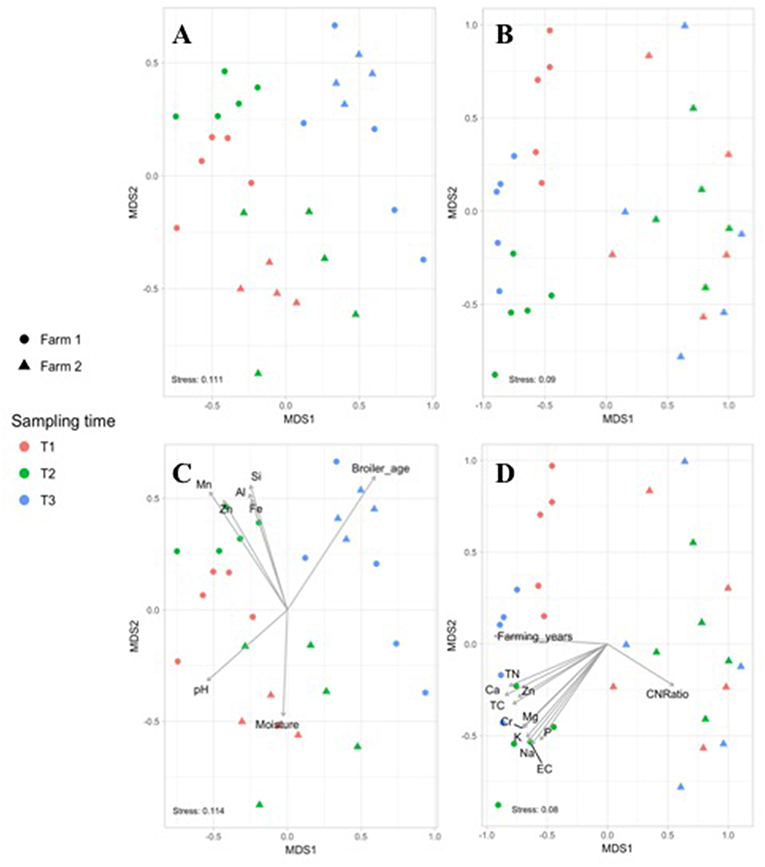
Figure 1. Non-metric multidimensional scaling (NMDS) based on Bray–Curtis dissimilarities of bacterial community composition from fecal (A) and soil (B) samples collected in farm 1 (circle) and 2 (triangle) during the live production stage at T1 (red), T2 (green), and T3 (blue). Significant farm parameters (p < 0.01) were fitted onto the NMDS plot using the envfit function in the VEGAN package for the fecal (C) and soil (D) microbiomes.
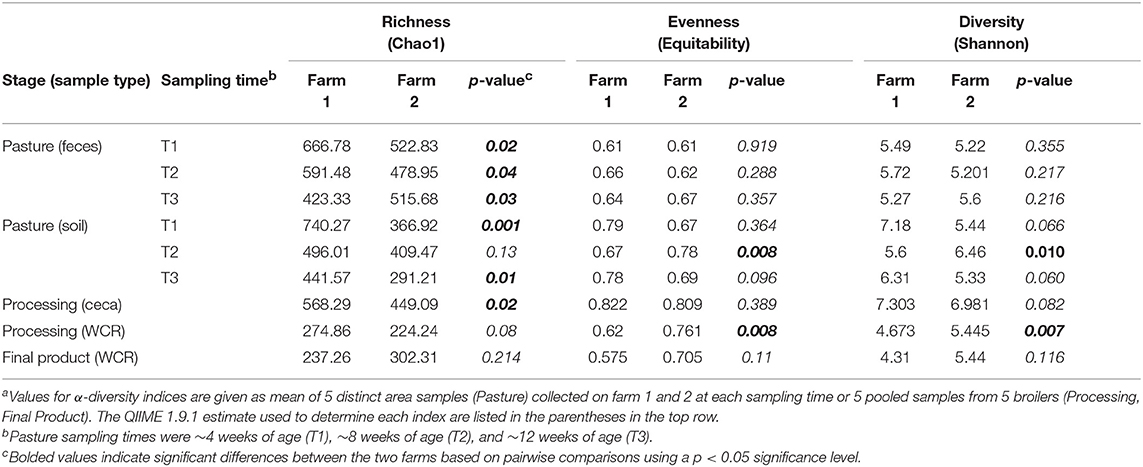
Table 2. Comparison of α-diversity indices of microbiomes throughout the farm-to-fork continuum from two pastured poultry flocksa.
To determine if measured physiochemical or management data could account for these significantly different fecal communities between these farms, non-metric multidimensional scaling (NMDS) analyses were performed. At T1 and T2, fecal microbiomes from farm 1 were significantly positively correlated to aluminum (Al, R2 = 0.337, p = 0.003), iron (Fe, R2 = 0.304, p = 0.003), manganese (Mn, R2 = 0.557, p = 0.001), silicon (Si, R2 = 0.375, p = 0.002) and zinc (Zn, R2 = 0.428, p = 0.002) concentrations, whereas no such significant correlations were observed for farm 2 (Figure 1C). This may be explained by the higher content of these five nutrients in feces from farm 1 as compared to farm 2 (Table 3). Fecal microbiomes of the market age broilers (T3) from farm 1 and farm 2 tended to converge (Figure 1A), confirming the moderate effect of the farm environment for this sampling time according to the ANOSIM analysis. The flock age exhibited the strongest correlation on the bacterial community (R2 = 0.711, p = 0.001), which is consistent with previous reports describing the effect of chicken development stage on the composition of intestinal microbiota (Crhanova et al., 2011). Fecal pH decreased with increasing chicken age and was negatively correlated to bacterial community of both farms (R2 = 0.4, p = 0.001) (Ilhan et al., 2017; Siegerstetter et al., 2018).
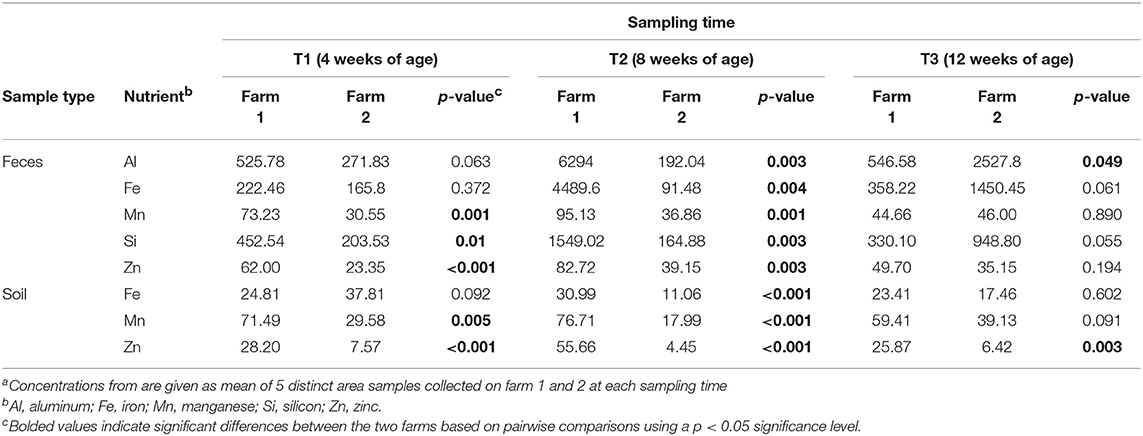
Table 3. Nutrient concentrations (ppm) from fecal and soil samples at three timepoints from two pastured poultry broiler flocksa.
It has been reported that the deficiency or biofortification of micronutrients such as zinc (Reed et al., 2015) or iron (Reed et al., 2017) in chicken diet has led to significant changes in the composition of the gut microbiota. In our study, broilers have been fed with the same diet, so the difference in fecal micronutrient load should be linked to another source. Considering pastured poultry supplement their nutritional needs via foraging, pasture soils could represent a possible source of these micronutrients. In fact, soil samples from farm 1 had higher concentrations of Fe, Mn, and Zn (Al and Si not measured) than farm 2 (Table 3) and may have influenced the concentration of micronutrients absorbed by chickens as they continuously take up elements from the surrounding environment during their growth cycle. This hypothesis may be supported by the fact that soil nutrient concentrations from farm 1 had significant positive correlations to the soil microbiomes, especially at T2, while no such correlations were observed for farm 2 (Figure 1D). The most significant correlations based on the R2 values for farm 1 were calcium (Ca, R2 = 0.797, p = 0.001), total carbon and total nitrogen (TC, TN, R2 = 0.722, p = 0.001), sodium (Na, R2 = 0.716, p = 0.001), and chromium (Cr, R2 = 0.711, p = 0.001). Although different from the nutrients that strongly correlated to fecal microbiomes, these results indicate that higher concentrations of these nutrients in the feces and soil of pastured poultry farms have the potential to shape the β-diversity of those microbial populations.
Not only did the farm environment effect the overall fecal microbiomes throughout live production, but it also significantly affected individual taxa within those microbiomes. Fecal samples from farm 1 exhibited a higher number of OTUs assigned at both the phylum (mean relative abundance 12 vs. 6; Kruskal-Wallis test, p = 0.046) and the genus (mean relative abundance 84 vs. 65; Kruskal-Wallis test, p = 0.043) level compared to farm 2. Significant differences in phyla between farms 1 and 2 were observed at T1 [Firmicutes (84.2 vs. 93.8%), Actinobacteria (3.4 vs. 0.2%)], T2 (Bacteroidetes (10.9 vs. 0.3%) and Actinobacteria (6.1 vs. 366 0.2%)], and at T3 [Bacteroidetes (1.5 vs. 5.5%)]. At the genus level, fecal microbiomes of farm 1 harbored a higher number of total and unique (present only in farm 1 fecal microbiomes) OTUs compared than farm 2 (p = 0.037 and 0.046, respectively), but have an equivalent number of dominant (≥1% relative abundance) OTUs (p = 0.658). The two farms shared 32, 34.6, and 32.5% of their total OTUs and 43.8, 57.1, and 66.7% of their dominant OTUs at T1, T2, and T3, respectively. While Lactobacillus and Escherichia OTUs were dominant for both farms at all three sampling times, significant differences were observed between farms in dominant genera-level OTU distribution throughout live production, although the number of significantly different dominant OTUs decreased as the birds aged (Table 4).
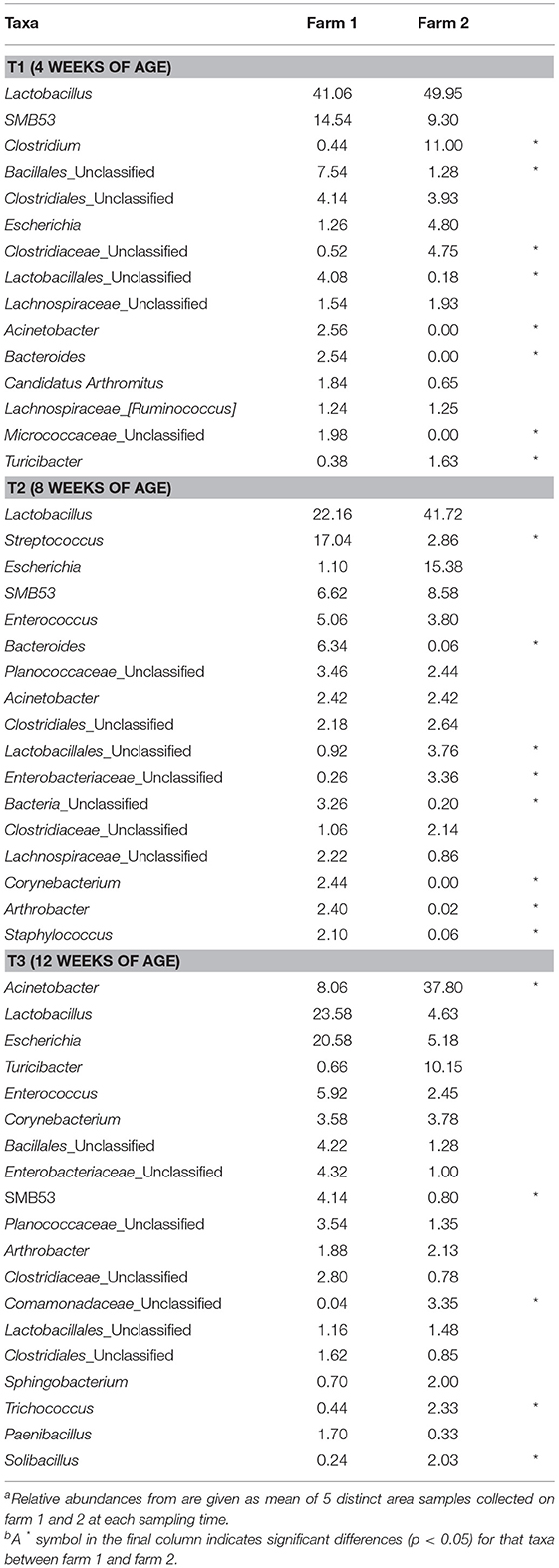
Table 4. Mean relative abundance (%) of dominant genus-level taxa (>1% of total OTUs) of fecal microbiomes at three timepoints from two pastured poultry broiler flocksa,b.
To see if these significant differences in fecal microbiome taxa could be a result of the physical farm environment, fecal OTUs unique to each farm were compared to unique soil OTUs from the same farm (Figure 2A). Shared unique OTUs found only in the feces and soil of farm 1 represented 22.5% (T1), 24.2% (T2), and 28.1% (T3) of the unique farm 1 fecal OTUs, while shared unique farm 2 OTUs represented 25, 33.3, and 47.9% of the unique farm 2 fecal OTUs (for T1, T2, and T3, respectively). When the cross comparisons were made (farm 1 unique feces OTUs vs. farm 2 unique soil OTUs; farm 2 unique feces OTUs vs. farm 1 unique soil OTUs), very few unique OTUs were shared (Figure 2B). This observation indicates that there is a much stronger link in microbiome OTU composition between environmental samples within the same farm compared to samples between farms, further supporting the effects that the farm environment can have on the pastured poultry microbiome during live production. Overall phyla and genus level OTU comparisons for the live production, processing and final product samples can be found in Supplementary Tables 1–3, respectively.
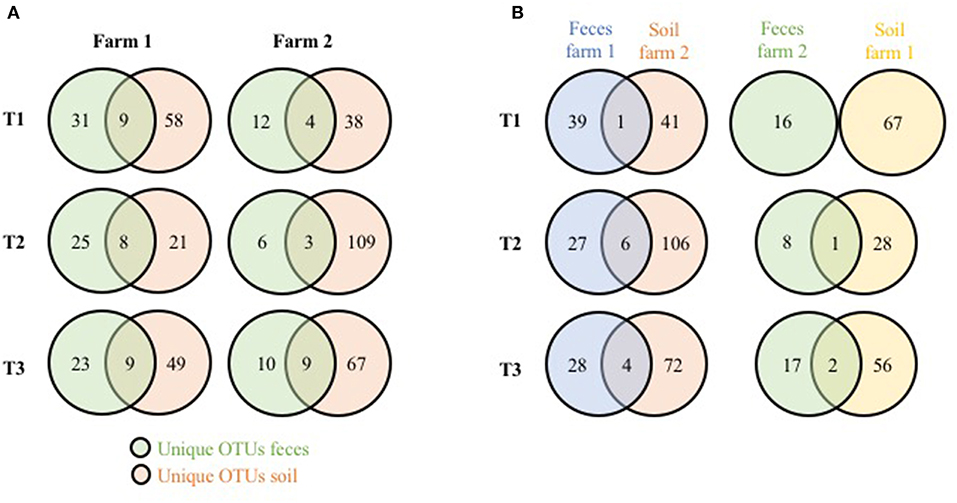
Figure 2. Shared genus-level OTUs between pasture microbiomes (fecal, soil) from three timepoints from two pastured broiler flocks (A) Venn diagram of unique OTUs from the fecal and soil microbiomes on the same farms. (B) Venn diagram of unique OTUs from fecal and soil microbiomes from different farms.
Poultry Microbiome at the Processing Stage
The effect of farm environment was evaluated on the cecal and post-processing, pre-storage whole carcass rinse (WCR) microbiome samples collected during on-farm processing for both farms. Cecal microbiomes were strongly affected by the farm environment (ANOSIM, R = 1, p = 0.01) as shown by the NMDS (Figure 3A; blue symbols) with farm 1 representing a significantly richer populations (chao1, p = 0.02) distributed among significantly more phyla (mean relative abundance 13 vs. 9; Kruskal-Wallis test, p = 0.009) compared to farm 2 (Table 2). In terms of taxa distribution, Firmicutes were the most abundant bacterial phylum in all cecal samples, representing 53.0 and 73.8% of taxa in farms 1 and 2, respectively, followed by Bacteroidetes, Proteobacteria, and Tenericutes. The prevalence and abundance of these phyla have been commonly found within broiler ceca (Danzeisen et al., 2011; Xu et al., 2016; Zhou et al., 2016; Costa et al., 2017). Of the major phyla, only an unassigned OTU group abundance (10 vs. 10.03% for farms 1 and 2, respectively) were not significantly different between farms. At the genus level, 101 total OTUs were identified, including 58 shared between farms 1 and 2. Farm 1 harbored a higher number of dominant (mean relative abundance 20 vs. 14; Kruskal-Wallis test, p < 0.014) OTUs than farm 2. The top-5 genera represented ~50% of the total taxa and was composed of Akkermansia, Clostridiales, Ruminococcaceae, Oscillospira, and Bacteroides for farm 1 and Oscillospira, Ruminococcaceae, Ruminococcus Faecalibacterium, and Clostridiales for farm 2, and significant differences were found between 70% of the dominant cecal OTUs (Table 5). The cecal OTU distributions for these two pastured poultry farms were globally different from other studies focusing on conventional broiler production (Oakley et al., 2014; Oakley and Kogut, 2016; Xu et al., 2016; Zhou et al., 2016); however, some dominant genera identified across farms 1 and 2 such as Ruminococcus, Fecalibacterium, Bacteroides, and Lachnospiraceae have also been reported to be abundant in ceca previously (Danzeisen et al., 2011; Oakley et al., 2014; Sergeant et al., 2014; Oakley and Kogut, 2016; Costa et al., 2017). This indicates that here is a core broiler ceca microbiome, regardless of production management system, although more research will need to be performed to better define this core broiler cecal microbiome.
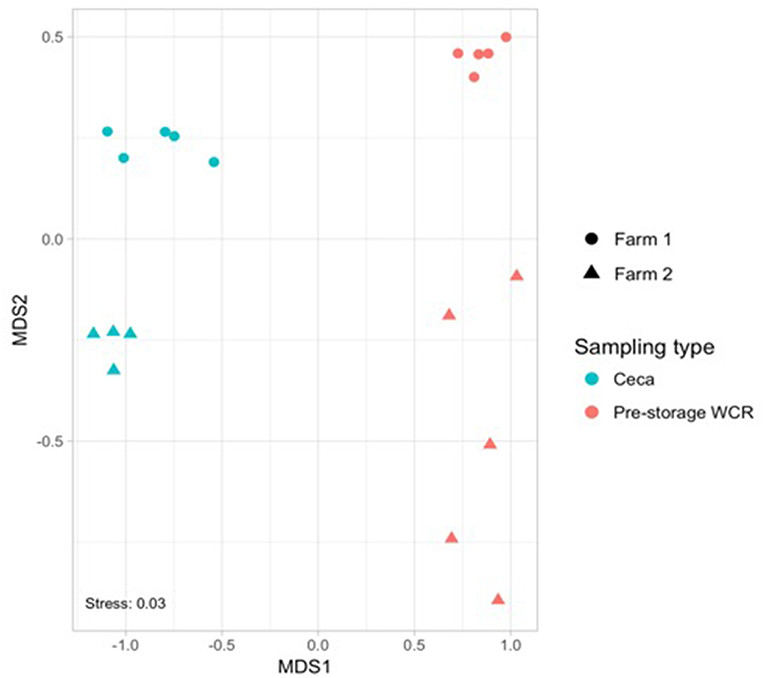
Figure 3. Non-metric multidimensional scaling (NMDS) based on Bray–Curtis dissimilarities of bacterial community composition from cecal (blue) and pre-storage WCR (red) samples collected in farm 1 (circle) and 2 (triangle) during the on-farm processing stage.
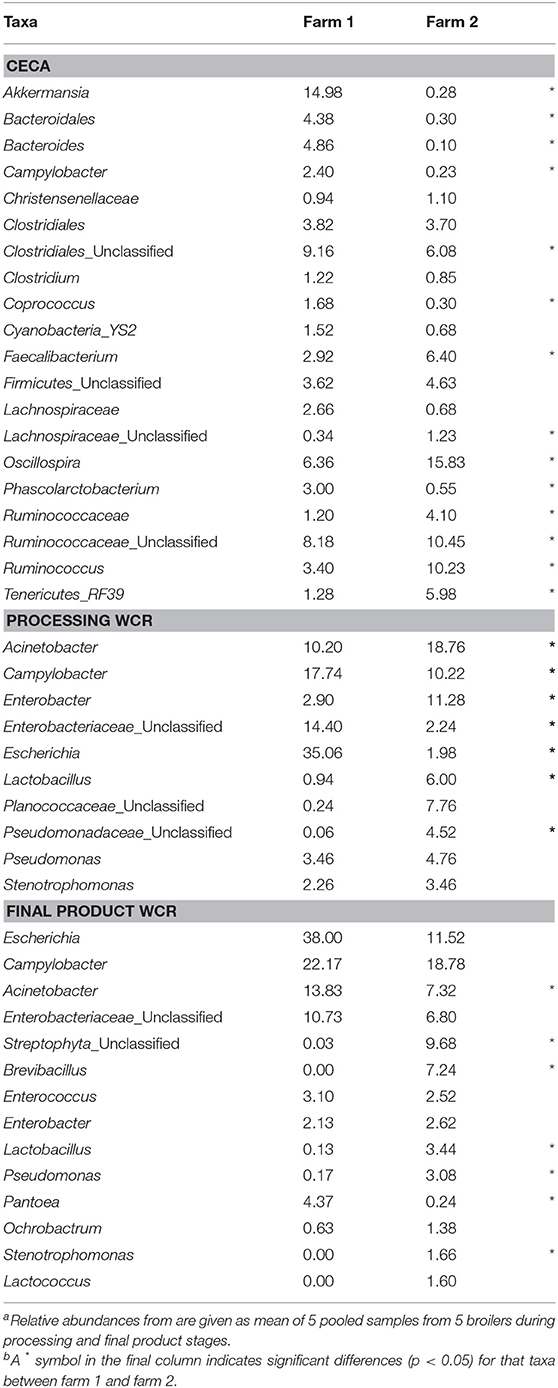
Table 5. Mean relative abundance (%) of dominant genus-level taxa (>1% of total OTUs) of processing (ceca, WCR) and final product (WCR) microbiomes from two pastured poultry broiler flocksa,b.
Thirty-four and Nine OTUs were unique to cecal samples from farm 1 and farm 2, respectively. In farm 1, 79.4 and 52.9% of the unique cecal OTUs were also identified in fecal and soil samples, respectively; only 14.7% of the unique cecal OTUs were not found before the processing stage and were likely acquired during processing. In farm 2, 55.5% of the unique OTUs in ceca were found for both the fecal and soil samples, while 11% of these OTUs were absent in the pre-harvest/live production samples. Although broiler cecal and fecal samples are known to have different phylogenetic distributions of sequences (Oakley and Kogut, 2016), our study suggests a conservation of certain OTUs across the live production and processing environments that could potentially constitute a unique biosignature for the farm environment.
Microbiomes from post-processing, pre-storage WCR samples significantly differed between farms (ANOSIM, R = 0.842, p = 0.008; Figure 3, red symbols). A greater number of dominant OTUs was observed in processing WCR samples from farm 2 along with an overall higher microbial equitability (p = 0.008) and diversity (p = 0.007) compared to farm 1 (Table 2). The processing WCR microbiomes from both farms shared the same dominant phyla, with Proteobacteria (89.2 vs. 63.7%), Firmicutes (5.4 vs. 28.4%), Cyanobacteria (2.1 vs. 2.4%), Bacteroidetes (1.7 vs. 2.0%), and Actinobacteria (1.2 vs. 2.0%) accounting for more than 98% of all sequences. The qualitative and quantitative composition of the dominant phyla associated with the processing WCR microbiomes agreed with previous findings (Kim et al., 2017; de Cesare et al., 2018). Among the 15 phyla identified across all processing WCR samples, only the relative abundance of Proteobacteria and Firmicutes significantly differed between the two farms. A total of 153 OTUs were assigned at the genus level, including 60 OTUs shared by processing WCR microbiomes from both farms. Among the 11 dominant OTUs shared between farms, the distribution and relative abundances significantly differed based on the farm (Table 5), although it should be noted that two of the top-3 genera within the processing WCR microbiomes for both farms are emerging or known foodborne pathogens (Acinetobacter and Campylobacter).
While these results indicate that the farm environment significantly influences processing microbiomes, it must be noted that the on-farm processing strategies differed in two main ways: (1) defeathering process and (2) chilling method. Farm 1 defeathered carcasses via removal of the skin and chilled the carcasses via air chilling, while farm 2 defeathered carcasses via scalding followed by automated picking and chilled the carcasses via immersion chilling. We expect that the defeathering method has a greater effect on the WCR samples compared to the cecal samples (due to the quick scalding process), and the chilling method to only effect the WCR samples (since the ceca are removed prior to chilling). Indeed, bacterial populations recovered from broiler carcass rinse samples with or without skin differ according to the chicken parts (Berrang et al., 2001). Additionally, the comparison of chilling methods used in commercial poultry facilities can lead to significant differences in pathogenic bacterial incidence and specific population concentrations within carcass rinse samples (Sanchez et al., 2002; Berrang et al., 2008). It should be noted that in the current study, for both farms, more OTUs were shared exclusively between the processing WCR microbiomes and soil microbiomes (17 and 19 OTUs for farm 1 and 2, respectively) than between the processing WCR and fecal microbiomes (1 and 6 OTUs for farm 1 and 2, respectively) (Figure 4). This suggests that the pastured poultry farm environment farm environment, specifically the pasture soil, has a lasting effect on the processing WCR microbiomes, regardless of processing management strategies.
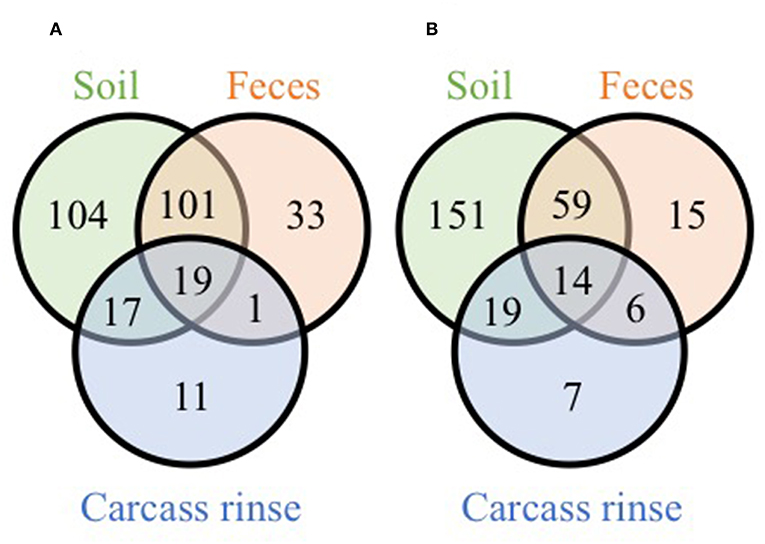
Figure 4. Venn diagrams of shared genus-level OTUs from soil, fecal and pre-storage WCR microbiomes from farm 1 (A) and farm 2 (B).
Poultry Microbiome in Final Product
While there were significant differences in the microbiomes between the farms from the live production and processing stages, final product WCR microbiomes were more uniform between farms. Although the number of OTUs assigned at the phyla (Kruskal-Wallis test, p = 0.021) and genera (p = 0.024) level were higher on farm 2, the species richness (p = 0.214), evenness (p = 0.11), and diversity (p = 0.116) did not significantly vary between the two farms (Table 2). Additionally, there were no significant differences in the β-diversity of the final product WCR microbiomes between farms (ANOSIM, R = 0.415, p = 0.093). Among the 16 phyla identified among all final product WCR samples, no significant differences were observed between farms 1 and 2. The final product WCR microbiomes were dominated by three genera, Escherichia, Campylobacter and Acinetobacter, representing 74.0 and 37.6% of the total OTUs recovered for farms 1 and 2, respectively (Table 5). These three genera were also the major genera in the processing WCR microbiomes. Unlike the processing WCR microbiomes where significant differences between farms were found for ~73% of the dominant genus-level taxa, only 50% of the dominant genus-level taxa from the final product WCR microbiomes were significantly different between farms. This finding was unexpected, not only given the differences between the microbiomes from these two farms at all stages up to this point, but the fact that the final products from both farms were stored under different conditions based on each farm's typical practices: Farm 1 stored the carcasses for 1 day at 4°C while farm 2 stored the carcasses at −20°C for 2 weeks. The storage temperature (refrigeration vs. freezing) has been previously shown to have a variable effect on bacterial populations (Bailey et al., 2000). Generally, bacterial populations on carcasses increased significantly during a storage of 7 days at 4°C (Bailey et al., 2000; Hinton et al., 2004; Pradhan et al., 2012), while remaining unchanged or declined for a storage at freezing temperatures below −18°C (Bailey et al., 2000; Georgsson et al., 2006). Regardless of all the diverse live production, processing, and final product handling conditions, very few differences were observed between farms for the final product WCR microbiomes at either the gross community (α and β-diversity estimates) or individual taxa levels, potentially indicating that there is a stable microbiome for pastured broiler products that needs to be further investigated in future studies.
Occurrence of Putative Pathogens
Due to the increased access to the environment and other farm animals in the pastured poultry management system (Siemon et al., 2007; Park et al., 2013), there is a hypothesis that this exposure would increase the prevalence of foodborne pathogens within pasture-raised flocks. But does the farm environment itself have any effect on the presence of known and emerging pathogens, or indicators? To address this question, taxa related to putative foodborne pathogens (Salmonella, Campylobacter, Listeria) and an indicator organism (Escherichia coli) were specifically analyzed and compared with cultural data (enumeration and/or enrichment) targeting these same four organisms. OTUs associated to the genus Escherichia were found in all samples of both farms (Table 6), with relative abundances being higher in the farm 2 preharvest samples (feces, soil), but significantly higher for farm 1 postharvest (ceca, processing WCR, final product WCR) samples. Escherichia has been commonly reported genera for poultry-associated samples (Oakley et al., 2014; de Cesare et al., 2018). The ubiquitous nature of E. coli throughout the farm-to-fork continuum was confirmed culturally, although cell counts were higher for all farm 1 samples other than the ceca, unlike what was observed in the non-quantitative microbiome relative abundance data. While there is no direct correlation between the microbiome and cell count data, it should be noted that a similar trend was observed in the final product WCR samples, where the estimate for farm 1 was approximately 3 times higher than farm 2.
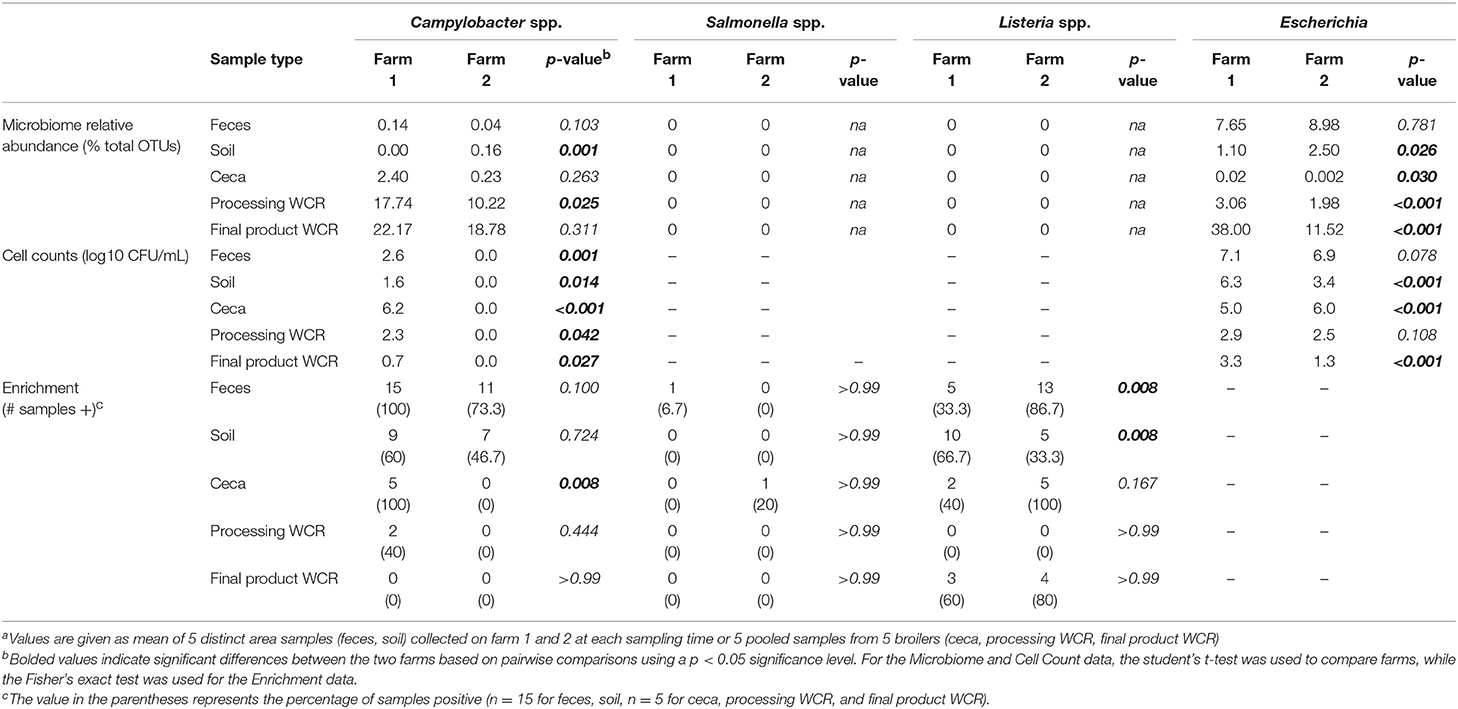
Table 6. Putative foodborne pathogen (Campylobacter, Salmonella, Listeria) and indicator (Escherichia) levels in different sample types throughout the farm-to-fork continuum from two pastured broiler flocks, measured by microbiome, direct cell counts, and enrichmentsa.
Of the three targeted foodborne pathogens, only OTUs related to Campylobacter were found within the pastured poultry-related microbiomes (Table 6). Like Escherichia, Campylobacter OTUs were found in all sample types for both farms, with the highest values found in the final product WCR samples. In general, relative abundances of Campylobacter were higher in farm 1, although it was only significantly higher in the processing WCR samples. Campylobacter spp. (mostly C. jejuni and C. coli) are present in nearly all birds at up to 107 CFU g−1 in the chicken intestine (Stern et al., 1995) and were evidenced in sequencing results from samples collected along the poultry production chain (Oakley et al., 2014; Park et al., 2016). Unlike the microbiome data, Campylobacter was only recovered from farm 1 samples culturally using selective CEFEX agar, although enrichment using a non-selective media allowed for the recovery for Campylobacter from the fecal and soil farm 2 samples but with a significantly lower prevalence that recovery from farm 1 (p = 0.011). These disparate results indicate a complex Campylobacter ecology throughout the pastured poultry farm-to-fork continuum but given the consistently higher prevalence on farm 1 for all microbiome and cultural estimates, farm environment appears to influence Campylobacter colonization and prevalence within pastured poultry.
No sequences were assigned to the Salmonella or Listeria genera in this study (Table 6). The low prevalence of Salmonella was confirmed culturally, with only a single Salmonella isolate recovered for both farms. Generally, Salmonella is a minor component of the poultry-associated microbiota (Oakley et al., 2014; de Cesare et al., 2018). However, the occurrence of Salmonella in samples collected from pasture farms to retail carcasses in this study is low compared to others (Melendez et al., 2010; Álvarez-Fernández et al., 2012; Lee et al., 2016). Unlike Salmonella, Listeria was recovered via enrichments from numerous samples, and while there were some significant differences in prevalence in the preharvest samples (Table 6; farm 1 soil, farm 2 feces), overall farm environment did not have a significant effect on Listeria prevalence (p = 0.205). The overall prevalence of Listeria spp. along the different production stages is consistent with previous reports (Sakaridis et al., 2011; Rothrock et al., 2017). While prevalence was unaffected, farm environment did majorly affect the species recovered, since all isolates from farm 1 were L. innocua while all isolates from farm 2 were L. monocytogenes serogroup 1/2a-3a. It has been recently reported that Listeria species recovery from pastured poultry farms can be potentially affected by culture conditions (Locatelli et al., 2017b), so it is possible that the different environmental conditions on both farms (such as the fertilization of pasture with organic fertilizer for organic vegetable production on farm 2) could equally influence the Listeria species on these farms.
Conclusion
Although both farms in this study raised the same chicken breed fed the same diet, each pastured poultry farm possessed their own ecology that shapes the structure and composition of the poultry-related microbiomes throughout the farm-to-fork continuum. Significant differences were observed in terms of not only α- (richness, diversity, evenness) and β- (Bray Curtis PCoA) diversity estimates between the two farms for the different sample types, but also in the taxa distribution within those samples. Farm environmental also significantly affected the presence of foodborne pathogens within these microbiomes, both quantitatively (Campylobacter) and in terms of recovered species (Listeria). The pasture soils from both farms significantly affected the OTU composition and nutrient composition of the live production fecal samples, highlighting the need for a better understanding of farm-level ecological dynamics inherent within pastured poultry management systems and its effect on poultry-related microbiomes. Future studies focusing on these farm-level environmental drivers of overall and foodborne pathogen microbial ecology will allow us to better manage these microbial populations to benefit animal, environmental and public health.
Ethics Statement
We did not handle any live birds or sample anything from live birds. We sampled soil and fecal droppings during live production, and only sampled ceca once the birds were butchered/processed by the farmers themselves. The farmers were responsible for the management and care of the birds during live production and they processed/butchered the birds on their own farms. We did not sample until the birds were already butchered. Therefore, no IACUC was required
Author Contributions
MR: designed the study. MR and AL: analyzed the data and wrote the manuscript.
Conflict of Interest Statement
The authors declare that the research was conducted in the absence of any commercial or financial relationships that could be construed as a potential conflict of interest.
Acknowledgments
The authors thank Kelli Hiett for initial conception of the overall parent study that this study was contained within. We would also like to thank Katelyn Griffin, Ariella Perry, Hellen Noh, Sarah Khorramzadeh, and Manju Amin for assistance in sample acquisition and pathogen isolation, Laura Lee Rutherford, Cheryl Gresham-Pearson, and Latoya Wiggins for performing the NARMS panels, and Sarah Owens at the US-DOE Argonne National Laboratory for assistance with sample preparation guidance for Illumina MiSeq sequencing. These investigations were supported by the Agricultural Research Service, USDA CRIS Projects Genetic Analysis of Poultry-Associated Salmonella enterica to Identify and Characterize Properties and Markers Associated with Egg-Borne Transmission of Illness #6040-32000-007-00 and Molecular Approaches for the Characterization of Foodborne Pathogens in Poultry #6612-32000-059-00.
Supplementary Material
The Supplementary Material for this article can be found online at: https://www.frontiersin.org/articles/10.3389/fsufs.2019.00048/full#supplementary-material
References
Álvarez-Fernández, E., Alonso-Calleja, C., García-Fernández, C., and Capita, R. (2012). Prevalence and antimicrobial resistance of Salmonella serotypes isolated from poultry in Spain: comparison between 1993 and 2006. Int. J. Food Microbiol. 153, 281–287. doi: 10.1016/j.ijfoodmicro.2011.11.011
Apajalahti, J., and Vienola, K. (2016). Interaction between chicken intestinal microbiota and protein digestion. Animal Feed Sci. Technol. 221, 323–330. doi: 10.1016/j.anifeedsci.2016.05.004
Bailey, J., Lyon, B., Lyon, C., and Windham, W. (2000). The microbiological profile of chilled and frozen chicken. J. Food Protect. 63, 1228–1230. doi: 10.4315/0362-028X-63.9.1228
Ballou, A. L., Ali, R. A., Mendoza, M. A., Ellis, J., Hassan, H. M., Croom, W., et al. (2016). Development of the chick microbiome: how early exposure influences future microbial diversity. Front. Vet. Sci. 3:2. doi: 10.3389/fvets.2016.00002
Berrang, M., Ladely, S., and Buhr, R. (2001). Presence and level of Campylobacter, coliforms, Escherichia coli, and total aerobic bacteria recovered from broiler parts with and without skin. J. Food Protect. 64, 184–188. doi: 10.4315/0362-028X-64.2.184
Berrang, M. E., Meinersmann, R. J., Smith, D. P., and Zhuang, H. (2008). The effect of chilling in cold air or ice water on the microbiological quality of broiler carcasses and the population of Campylobacter. Poult. Sci. 87, 992–998. doi: 10.3382/ps.2007-00406
Bjerrum, L., Engberg, R., Leser, T. D., Jensen, B. B., Finster, K., and Pedersen, K. (2006). Microbial community composition of the ileum and cecum of broiler chickens as revealed by molecular and culture-based techniques. Poult. Sci. 85, 1151–1164. doi: 10.1093/ps/85.7.1151
Byrd, J., Corrier, D., Deloach, J., Nisbet, D., and Stanker, L. (1998). Horizontal transmission of Salmonella typhimurium in broiler chicks. J. Appl. Poult. Res. 7, 75–80. doi: 10.1093/japr/7.1.75
Caporaso, J. G., Bittinger, K., Bushman, F. D., Desantis, T. Z., Andersen, G. L., and Knight, R. (2010a). PyNAST: a flexible tool for aligning sequences to a template alignment. Bioinformatics 26, 266–267. doi: 10.1093/bioinformatics/btp636
Caporaso, J. G., Kuczynski, J., Stombaugh, J., Bittinger, K., Bushman, F. D., Costello, E. K., et al. (2010b). QIIME allows analysis of high-throughput community sequencing data. Nat. Methods 7, 335–336. doi: 10.1038/nmeth.f.303
Caporaso, J. G., Lauber, C. L., Walters, W. A., Berg-Lyons, D., Lozupone, C. A., Turnbaugh, P. J., et al. (2011). Global patterns of 16S rRNA diversity at a depth of millions of sequences per sample. Proc. Natl. Acad. Sci. U.S.A. 108, 4516–4522. doi: 10.1073/pnas.1000080107
Costa, M. C., Bessegatto, J. A., Alfieri, A. A., Weese, J. S., João Filho, A., and Oba, A. (2017). Different antibiotic growth promoters induce specific changes in the cecal microbiota membership of broiler chicken. PLoS ONE 12:e0171642. doi: 10.1371/journal.pone.0171642
Crhanova, M., Hradecka, H., Faldynova, M., Matulova, M., Havlickova, H., Sisak, F., et al. (2011). Immune response of chicken gut to natural colonization by gut microflora and to Salmonella enterica serovar enteritidis infection. Infect. Immunity 79, 2755–2763. doi: 10.1128/IAI.01375-10
Danzeisen, J. L., Kim, H. B., Isaacson, R. E., Tu, Z. J., and Johnson, T. J. (2011). Modulations of the chicken cecal microbiome and metagenome in response to anticoccidial and growth promoter treatment. PLoS ONE 6:e27949. doi: 10.1371/journal.pone.0027949
de Cesare, A., Palma, F., Lucchi, A., Pasquali, F., and Manfreda, G. (2018). Microbiological profile of chicken carcasses: a comparative analysis using shotgun metagenomic sequencing. Ital. J. Food Saf. 7:6923. doi: 10.4081/ijfs.2018.6923
Desantis, T. Z., Hugenholtz, P., Larsen, N., Rojas, M., Brodie, E. L., Keller, K., et al. (2006). Greengenes, a chimera-checked 16S rRNA gene database and workbench compatible with ARB. Appl. Environ. Microbiol. 72, 5069–5072. doi: 10.1128/AEM.03006-05
Dumas, M. D., Polson, S. W., Ritter, D., Ravel, J., Gelb, J Jr, Morgan, R., et al. (2011). Impacts of poultry house environment on poultry litter bacterial community composition. PLoS ONE 6:e24785. doi: 10.1371/journal.pone.0024785
Dupont, H. L. (2007). The growing threat of foodborne bacterial enteropathogens of animal origin. Clin. Infect. Dis. 45, 1353–1361. doi: 10.1086/522662
Edgar, R. C. (2010). Search and clustering orders of magnitude faster than BLAST. Bioinformatics 26, 2460–2461. doi: 10.1093/bioinformatics/btq461
EFSA and European Centre for Disease Prevention and Control (2015). The European Union summary report on trends and sources of zoonoses, zoonotic agents and food-borne outbreaks in 2013. EFSA J. 13:3991.
Georgsson, F., Þorkelsson, Á. E., Geirsdóttir, M., Reiersen, J., and Stern, N. J. (2006). The influence of freezing and duration of storage on Campylobacter and indicator bacteria in broiler carcasses. Food Microbiol. 23, 677–683. doi: 10.1016/j.fm.2005.10.003
Hermans, D., Pasmans, F., Messens, W., Martel, A., Van Immerseel, F., Rasschaert, G., et al. (2012). Poultry as a host for the zoonotic pathogen Campylobacter jejuni. Vector-Borne Zoonotic Dis. 12, 89–98. doi: 10.1089/vbz.2011.0676
Hinton, A., Cason, J., and Ingram, K. D. (2004). Tracking spoilage bacteria in commercial poultry processing and refrigerated storage of poultry carcasses. Int J Food Microbiol. 91, 155–165. doi: 10.1016/S0168-1605(03)00377-5
Ilhan, Z. E., Marcus, A. K., Kang, D.-W., Rittmann, B. E., and Krajmalnik-Brown, R. (2017). pH-Mediated microbial and metabolic interactions in fecal enrichment cultures. mSphere 2, e00047–e00017. doi: 10.1128/mSphere.00047-17
Kers, J. G., Velkers, F. C., Fischer, E. A., Hermes, G. D., Stegeman, J. A., and Smidt, H. (2018). Host and environmental factors affecting the intestinal microbiota in chickens. Front. Microbiol. 9:235. doi: 10.3389/fmicb.2018.00235
Kim, J. E., Lillehoj, H. S., Hong, Y. H., Kim, G. B., Lee, S. H., Lillehoj, E. P., et al. (2015). Dietary capsicum and Curcuma longa oleoresins increase intestinal microbiome and necrotic enteritis in three commercial broiler breeds. Res. Vet. Sci. 102, 150–158. doi: 10.1016/j.rvsc.2015.07.022
Kim, S., Park, S. H., Lee, S. I., Owens, C. M., and Ricke, S. C. (2017). Assessment of chicken carcass microbiome responses during processing in the presence of commercial antimicrobials using a next generation sequencing approach. Sci. Rep. 7:43354. doi: 10.1038/srep43354
Lastovica, A. J., and Le Roux, E. (2001). Efficient isolation of Campylobacter upsaliensis from stools. J. Clin. Microbiol. 39, 4222–4223. doi: 10.1128/JCM.39.11.4222-4223.2001
Laukens, D., Brinkman, B. M., Raes, J., De Vos, M., and Vandenabeele, P. (2015). Heterogeneity of the gut microbiome in mice: guidelines for optimizing experimental design. FEMS Microbiol. Rev. 40, 117–132. doi: 10.1093/femsre/fuv036
Lee, S. I., Park, S. H., and Ricke, S. C. (2016). Assessment of cecal microbiota, integron occurrence, fermentation responses, and Salmonella frequency in conventionally raised broilers fed a commercial yeast-based prebiotic compound. Poult. Sci. 95, 144–153. doi: 10.3382/ps/pev322
Locatelli, A., Hiett, K. L., Caudill, A. C., and Rothrock, M. J. Jr. (2017a). Do fecal and litter microbiomes vary within the major areas of a commercial poultry house, and does this affect sampling strategies for whole-house microbiomic studies? J. Appl. Poult. Res. 26, 325–336. doi: 10.3382/japr/pfw076
Locatelli, A., Lewis, M. A., and Rothrock, M. J. (2017b). The distribution of Listeria in pasture-raised broiler farm soils is potentially related to University of Vermont medium enrichment bias toward Listeria innocua over Listeria monocytogenes. Front. Vet. Sci. 4:227. doi: 10.3389/fvets.2017.00227
Ludvigsen, J., Svihus, B., and Rudi, K. (2016). Rearing room affects the non-dominant chicken cecum microbiota, while diet affects the dominant microbiota. Front. Vet. Sci. 3:16. doi: 10.3389/fvets.2016.00016
Melendez, S. N., Hanning, I., Han, J., Nayak, R., Clement, A., Wooming, A., et al. (2010). Salmonella enterica isolates from pasture-raised poultry exhibit antimicrobial resistance and class I integrons. J. Appl. Microbiol. 109, 1957–1966. doi: 10.1111/j.1365-2672.2010.04825.x
Nisbet, D. J., Corrier, D. E., and Deloach, J. R. (1993). Effect of mixed cecal microflora maintained in continuous culture and of dietary lactose on Salmonella typhimurium colonization in broiler chicks. Avian Dis. 37, 528–535. doi: 10.2307/1591682
Oakley, B. B., Buhr, R. J., Ritz, C. W., Kiepper, B. H., Berrang, M. E., Seal, B. S., et al. (2014). Successional changes in the chicken cecal microbiome during 42 days of growth are independent of organic acid feed additives. BMC Vet. Res. 10:1. doi: 10.1186/s12917-014-0282-8
Oakley, B. B., and Kogut, M. H. (2016). Spatial and temporal changes in the broiler chicken cecal and fecal microbiomes and correlations of bacterial taxa with cytokine gene expression. Front. Vet. Sci. 3, 1–12. doi: 10.3389/fvets.2016.00011
Pan, D., and Yu, Z. (2014). Intestinal microbiome of poultry and its interaction with host and diet. Gut Microbes 5, 108–119. doi: 10.4161/gmic.26945
Park, S. H., Hanning, I., Perrota, A., Bench, B. J., Alm, E., and Ricke, S. C. (2013). Modifying the gastrointestinal ecology in alternatively raised poultry and the potential for molecular and metabolomic assessment. Poult. Sci. 92, 546–561. doi: 10.3382/ps.2012-02734
Park, S. H., Lee, S. I., and Ricke, S. C. (2016). Microbial populations in naked neck chicken ceca raised on pasture flock fed with commercial yeast cell wall prebiotics via an Illumina MiSeq platform. PLoS ONE 11:e0151944. doi: 10.1371/journal.pone.0151944
Patriarchi, A., Fox, Á., Maunsell, B., Fanning, S., and Bolton, D. (2011). Molecular characterization and environmental mapping of Campylobacter isolates in a subset of intensive poultry flocks in Ireland. Foodborne Pathog. Dis. 8, 99–108. doi: 10.1089/fpd.2010.0637
Pedroso, A. A., Batal, A. B., and Lee, M. D. (2016). Effect of in ovo administration of an adult-derived microbiota on establishment of the intestinal microbiome in chickens. Am. J. Vet. Res. 77, 514–526. doi: 10.2460/ajvr.77.5.514
Popoff, M., and Le Minor, L. (1997). Antigenic Formulas of the Salmonella serovars 7th revision: World Health Organization Collaborating Centre for Reference and Research on Salmonella. Paris: Pasteur Institute.
Pradhan, A., Li, M., Li, Y., Kelso, L., Costello, T., and Johnson, M. (2012). A modified Weibull model for growth and survival of Listeria innocua and Salmonella Typhimurium in chicken breasts during refrigerated and frozen storage. Poult. Sci. 91, 1482–1488. doi: 10.3382/ps.2011-01851
Reed, S., Neuman, H., Glahn, R. P., Koren, O., and Tako, E. (2017). Characterizing the gut (Gallus gallus) microbiota following the consumption of an iron biofortified Rwandan cream seeded carioca (Phaseolus Vulgaris L.) bean-based diet. PLoS ONE 12:e0182431. doi: 10.1371/journal.pone.0182431
Reed, S., Neuman, H., Moscovich, S., Glahn, R. P., Koren, O., and Tako, E. (2015). Chronic zinc deficiency alters chick gut microbiota composition and function. Nutrients 7, 9768–9784. doi: 10.3390/nu7125497
Rothrock, M. J., Davis, M. L., Locatelli, A., Bodie, A., Mcintosh, T. G., Donaldson, J. R., et al. (2017). Listeria occurrence in poultry flocks: detection and potential implications. Front. Vet. Sci. 4:125. doi: 10.3389/fvets.2017.00125
Rothrock, M. J. Jr., Hiett, K. L., Gamble, J., Caudill, A. C., Cicconi-Hogan, K. M., and Caporaso, J. G. (2014). A hybrid DNA extraction method for the qualitative and quantitative assessment of bacterial communities from poultry production samples. J. Visual. Exp. 94:e52161. doi: 10.3791/52161
Roto, S. M., Kwon, Y. M., and Ricke, S. C. (2016). Applications of in ovo technique for the optimal development of the gastrointestinal tract and the potential influence on the establishment of its microbiome in poultry. Front. Vet. Sci. 3:63. doi: 10.3389/fvets.2016.00063
Sakaridis, I., Soultos, N., Iossifidou, E., Papa, A., Ambrosiadis, I., and Koidis, P. (2011). Prevalence and antimicrobial resistance of Listeria monocytogenes isolated in chicken slaughterhouses in northern Greece. J. Food Prot. 74, 1017–1021. doi: 10.4315/0362-028X.JFP-10-545
Sanchez, M. X. S., Fluckey, W. M., Brashears, M. M., and Mckee, S. R. (2002). Microbial profile and antibiotic susceptibility of Campylobacter spp. and Salmonella spp. in broilers processed in air-chilled and immersion-chilled environments. J. Food Protect. 65, 948–956. doi: 10.4315/0362-028X-65.6.948
Schokker, D., Jansman, A. J., Veninga, G., De Bruin, N., Vastenhouw, S. A., De Bree, F. M., et al. (2017). Perturbation of microbiota in one-day old broiler chickens with antibiotic for 24 hours negatively affects intestinal immune development. BMC Genom. 18:241. doi: 10.1186/s12864-017-3625-6
Sergeant, M. J., Constantinidou, C., Cogan, T. A., Bedford, M. R., Penn, C. W., and Pallen, M. J. (2014). Extensive microbial and functional diversity within the chicken cecal microbiome. PLoS ONE 9:e91941. doi: 10.1371/journal.pone.0091941
Shanker, S., Lee, A., and Sorrell, T. (1990). Horizontal transmission of Campylobacter jejuni amongst broiler chicks: experimental studies. Epidemiol. Infect. 104, 101–110. doi: 10.1017/S0950268800054571
Siegerstetter, S.-C., Petri, R. M., Magowan, E., Lawlor, P. G., Zebeli, Q., O'connell, N. E., et al. (2018). Fecal microbiota transplant from highly feed efficient donors shows little effects on age-related changes in feed efficiency-associated fecal microbiota in chickens. Appl. Environ. Microbiol. 84, 2330–2317. doi: 10.1128/AEM.02330-17
Siemon, C. E., Bahnson, P. B., and Gebreyes, W. A. (2007). Comparative investigation of prevalence and antimicrobial resistance of Salmonella between pasture and conventionally reared poultry. Avian Dis. 51, 112–117. doi: 10.1637/0005-2086(2007)051[0112:CIOPAA]2.0.CO;2
Singh, K., Shah, T., Deshpande, S., Jakhesara, S., Koringa, P., Rank, D., et al. (2012). High through put 16S rRNA gene-based pyrosequencing analysis of the fecal microbiota of high FCR and low FCR broiler growers. Mol. Biol. Rep. 39, 10595–10602. doi: 10.1007/s11033-012-1947-7
Stanley, D., Geier, M. S., Hughes, R. J., Denman, S. E., and Moore, R. J. (2013). Highly variable microbiota development in the chicken gastrointestinal tract. PLoS ONE 8:e84290. doi: 10.1371/journal.pone.0084290
Stanley, D., Hughes, R. J., and Moore, R. J. (2014). Microbiota of the chicken gastrointestinal tract: influence on health, productivity and disease. Appl. Microbiol. Biotechnol. 98, 4301–4310. doi: 10.1007/s00253-014-5646-2
Stern, N. J., Clavero, M. R., Bailey, J. S., Cox, N. A., and Robach, M. C. (1995). Campylobacter spp. in broilers on the farm and after transport. Poult. Sci. 74, 937–941. doi: 10.3382/ps.0740937
Stern, N. J., Wojton, B., and Kwiatek, K. (1992). A differential-selective medium and dry ice-generated atmosphere for recovery of Campylobacter jejuni. J. Food Protect. 55, 514–517. doi: 10.4315/0362-028X-55.7.514
Torok, V., Dyson, C., Mckay, A., and Ophel-Keller, K. (2013). Quantitative molecular assays for evaluating changes in broiler gut microbiota linked with diet and performance. Anim. Prod. Sci. 53, 1260–1268. doi: 10.1071/AN12272
Torok, V. A., Hughes, R. J., Ophel-Keller, K., Ali, M., and Macalpine, R. (2009). Influence of different litter materials on cecal microbiota colonization in broiler chickens. Poult. Sci. 88, 2474–2481. doi: 10.3382/ps.2008-00381
Videnska, P., Faldynova, M., Juricova, H., Babak, V., Sisak, F., Havlickova, H., et al. (2013). Chicken faecal microbiota and disturbances induced by single or repeated therapy with tetracycline and streptomycin. BMC Vet. Res. 9:30. doi: 10.1186/1746-6148-9-30
Videnska, P., Rahman, M. M., Faldynova, M., Babak, V., Matulova, M. E., Prukner-Radovcic, E., et al. (2014). Characterization of egg laying hen and broiler fecal microbiota in poultry farms in Croatia, Czech Republic, Hungary and Slovenia. PLoS ONE 9:e110076. doi: 10.1371/journal.pone.0110076
Waite, D. W., and Taylor, M. W. (2014). Characterizing the avian gut microbiota: membership, driving influences, and potential function. Front. Microbiol. 5:223. doi: 10.3389/fmicb.2014.00223
Walugembe, M., Hsieh, J., Koszewski, N., Lamont, S., Persia, M., and Rothschild, M. (2015). Effects of dietary fiber on cecal short-chain fatty acid and cecal microbiota of broiler and laying-hen chicks. Poult. Sci. 94, 2351–2359. doi: 10.3382/ps/pev242
Xu, Y., Yang, H., Zhang, L., Su, Y., Shi, D., Xiao, H., et al. (2016). High-throughput sequencing technology to reveal the composition and function of cecal microbiota in Dagu chicken. BMC Microbiol. 16:259. doi: 10.1186/s12866-016-0877-2
Yeoman, C. J., Chia, N., Jeraldo, P., Sipos, M., Goldenfeld, N. D., and White, B. A. (2012). The microbiome of the chicken gastrointestinal tract. Anim. Health Res. Rev. 13, 89–99. doi: 10.1017/S1466252312000138
Zhao, L., Wang, G., Siegel, P., He, C., Wang, H., Zhao, W., et al. (2013). Quantitative genetic background of the host influences gut microbiomes in chickens. Sci. Rep. 3:1163. doi: 10.1038/srep01163
Zhou, X., Jiang, X., Yang, C., Ma, B., Lei, C., Xu, C., et al. (2016). Cecal microbiota of Tibetan chickens from five geographic regions were determined by 16S rRNA sequencing. MicrobiologyOpen 5, 753–762. doi: 10.1002/mbo3.367
Keywords: pastured poultry, farm-to-fork, microbiome, ecology, Campylobacter
Citation: Rothrock MJ Jr and Locatelli A (2019) Importance of Farm Environment to Shape Poultry-Related Microbiomes Throughout the Farm-to-Fork Continuum of Pasture-Raised Broiler Flocks. Front. Sustain. Food Syst. 3:48. doi: 10.3389/fsufs.2019.00048
Received: 08 November 2018; Accepted: 11 June 2019;
Published: 26 June 2019.
Edited by:
Katri Johanna Björkroth, University of Helsinki, FinlandReviewed by:
Séamus Fanning, University College Dublin, IrelandIan F. Connerton, University of Nottingham, United Kingdom
Copyright © 2019 Rothrock and Locatelli. This is an open-access article distributed under the terms of the Creative Commons Attribution License (CC BY). The use, distribution or reproduction in other forums is permitted, provided the original author(s) and the copyright owner(s) are credited and that the original publication in this journal is cited, in accordance with accepted academic practice. No use, distribution or reproduction is permitted which does not comply with these terms.
*Correspondence: Michael J. Rothrock Jr., TWljaGFlbC5yb3Rocm9ja0BhcnMudXNkYS5nb3Y=
†These authors have contributed equally to this work