- 1Institute for Resources, Environment and Sustainability, University of British Columbia, Vancouver, BC, Canada
- 2Gund Institute for Environment and Rubenstein School of Environment and Natural Resources, University of Vermont, Burlington, VT, United States
- 3Department of Community Sustainability, Michigan State University, East Lansing, MI, United States
- 4School of Planning, Design, and Construction, and the Center for Global Change and Earth Observations, Michigan State University, East Lansing, MI, United States
- 5Institute of Energy and Environment, University of São Paulo, São Paulo, Brazil
- 6Environmental Change Institute, University of Oxford, Oxford, United Kingdom
- 7Department of Nutrition and Food Sciences, Food Systems Program, University of Vermont, Burlington, VT, United States
Farmers in the Indo-Gangetic Plains produce much of the wheat and rice grown in India. However, food production and millions of farm-based livelihoods in this region will continue to be adversely affected by hydro-climatic change and variation, reduced land productivity, and declining groundwater levels. Thus, agricultural adaptations are essential for protecting and improving upon intersecting goals of food security, poverty alleviation, and wellbeing. Household “capital” (e.g., natural, human, financial, physical, and social) is commonly cited as an indicator of livelihood adaptability and innovation. We develop a series of mediated structural equation models to empirically evaluate the validity of capital as a suitable indicator for adaptation and adaptive capacity. These models assess the extent to which household capital mediates the relationship between over 1,000 socio-economically differentiated and randomly selected farm households, and their crop, livestock, and land management adaptations in the states of Haryana and Bihar. Central to our models is a single household capital variable, constructed by aggregating nearly 80 different measurements of households' (i) physical/infrastructural capital, (ii) owned or accessed assets, (iii) livelihood diversity, (iv) ability to reach market or commercialize, (v) access to weather information, and (vi) social capital. We find household capital is a significant predictor in adopting crop, land management, and livestock-related adaptation strategies across both states. Second, and in certain cases, lower castes and less educated households engaged in fewer agricultural adaptations—an outcome mediated by their lower composition of capital. Further, and across almost all contexts, household capital mediated the effect of owning a greater proportion of land, and the higher uptake of agricultural adaptation activities. While improvements in any capital category can improve adoption, we recommend programs that improve (i) access to public and private agricultural infrastructure for lower castes; (ii) education and shared knowledge spaces for less-educated households; and (iii) the availability of low-interest loans and the more efficient legal transfer of land for agriculturalists owning a smaller proportion of their land. Through this novel and large-scale analysis of household data, we provide short-term and immediate recommendations for more equitable agricultural adaptation in this breadbasket region of northern India.
Introduction
Across India, and much of the global South, millions of agricultural households and livelihoods are considered vulnerable to hydro-climatological change and variation (Dasgupta et al., 2014; Shukla et al., 2017). Dry-spells, extreme temperatures, and precipitation variability are key effects of global climate change that will be felt by Indian farmers in their everyday agricultural activities (Kelkar et al., 2008; Immerzeel et al., 2010; Meshram et al., 2016). These risks will manifest in the Indo-Gangetic Plains (IGP) of India—the fertile regions of Punjab, Haryana, Uttar Pradesh, and Bihar that produce between 70–74% and 40–45% of national wheat and rice, respectively (Sekar and Pal, 2012; Khatri-Chhetri et al., 2016). In Haryana for example, it is projected that agriculture and livestock will be impacted by shifts in the range of temperature, unpredictable moisture deficits, and heat exposure (Government of Haryana, 2011). In Bihar, heat stress in the winter season combined with more intense precipitation and lengthier dry spells in the monsoon season present risks and opportunities for agricultural systems (Tesfaye et al., 2017). Importantly, such climate-driven risks are situated within a broader agrarian context where declining groundwater levels, land-use productivity, shifting labor dynamics, and structural policy changes continue to affect the foundation of agriculture (Chand and Haque, 1998; Reddy and Mishra, 2009; Rodell et al., 2009; Sekar and Pal, 2012).
In order to adapt with such climatic and non-climatic risks, it is essential that farm households are able to engage in a variety of crop, land, and livestock adaptation strategies (Aggarwal et al., 2004; Lipper et al., 2014; Sapkota et al., 2015). In this sense, adaptability or adaptive capacity refers to the capacity of a household to “prepare for stresses and changes in advance or adjust and respond to the effects caused by the stresses” (Engle, 2011, p. 647; Smit and Wandel, 2006; Agard et al., 2014). Some examples of agricultural adaptation strategies in the IGP include the adoption of land and water management strategies (e.g., improved drainage, irrigation, and altered planting dates), conservation agriculture practices, and climate-tolerant and diseases resistant varieties (Ojha et al., 2014; Bhatta and Aggarwal, 2015; Khatri-Chhetri et al., 2016; Bhatta et al., 2017; but cf. Bastakoti et al., 2017). Few studies have, however, extensively examined who is adapting and the reasons why certain households may not be able to invest in, or avail agricultural services and technologies in this breadbasket region (but see Ojha et al., 2014; Sugden et al., 2014; Bhatta et al., 2017).
Research traditions in agrarian development, including the Sustainable Livelihoods Approach and broader capabilities-based models, have enriched concepts of adaptation and adaptive capacity. In particular, a household's economic, physical, natural, social capitals (including informal and formal institutional access) have often been theorized as foundational indicators of livelihood planning and adaptation to social-ecological change (Chambers and Conway, 1992; Scoones, 1998; Adger and Kelly, 1999). More recently, a growing set of work has empirically shown that such capitals, or lack thereof, shape agricultural adaptive capacity, and adaptation across regions in the global South (Sugden et al., 2014; Wood et al., 2014; Perez et al., 2015; Kabir et al., 2017; Chen et al., 2018; Sujakhu et al., 2018). We use the term “capital” to employ Sen's (1981) concepts of “endowments” and “entitlements” as the set of resources that a person or household can acquire through their owned assets, relations, and market and non-market exchanges.
There are multiple pathways through which capital supports adaptation. Sen's (1981) original work on food insecurity highlighted that endowments and entitlement relations serve critical roles in market exchange and thus, food security. Others go beyond market exchange, emphasizing the roles of social and communal exchange in shaping entitlement structures (Leach et al., 1999). According to scholars like Ifejika Speranza et al. (2014), Patnaik et al. (2017), and Abid et al. (2017), knowledge sharing and learning, access to agricultural institutions, and endowments and entitlements to capital are critical factors that underpin livelihood adaptation and resilience. Bebbington (1999) argues household capitals provide meaning and drive “emancipatory action” by challenging the structures under which life and livelihood exist (Obrist et al., 2010). This collective set of works—much of it including theoretical and indicator-based frameworks—suggest household capital can serve diverse market, social exchange, knowledge sharing, value-based, and/or emancipatory roles in shaping livelihood adaptation processes.
This paper, analyzing a large set of data from over 1,000 farm households across Bihar and Haryana (India), sets out to empirically assess the extent to which “household capital” mediates the relationship between socio-economically differentiated households and their crop, livestock, and land management adaptations. In doing so, it contributes to three key research and policy gaps in the broader agricultural adaptation scholarship.
First, frameworks that theorize capital as a key process and mechanism underlying livelihood adaptation require validation using household-level data across diverse cultural and institutional contexts. Following Birkenholtz (2012), researchers often adopt “intensive”—qualitative village-scale studies on power, culture, and institutions that mediate adaptation in social-ecological systems (e.g., Cote and Nightingale, 2012)—or, “extensive” analyses where generic indicators, such as census data, are used to predict adaptability across large spatial scales. The use of empirical household-level data in different regional institutional contexts can provide policy-makers clarity over the roles and efficacy of capital in adaptation. Second, and importantly, much of the existing agricultural adaptation research explores either the extent to which certain household characteristics (e.g., education level, gender, household size) predict adaptation outcomes, or explores the extent to which household capitals (e.g., financial capital, infrastructure, access to weather information) enable adaptation (e.g., Deressa et al., 2010; Below et al., 2012; Wood et al., 2014; Kabir et al., 2017; Sujakhu et al., 2018). While such cases can provide evidence in support of capital, its key limitation is the inability to assess the extent to which household capital mediates the relationship between particular socio-economically differentiated households and their adaptation activities. Mediation analysis provides policy-makers with key information not only on the role of capital in mediating adaptability at the meso-scale, but also enables them to identify who is adopting agricultural adaptation and who is in need of stronger state and extension support—an important empirical gap for promoting evidence-based and equitable policy interventions (see Andersson and D'Souza, 2014; Totin et al., 2018). Finally, more empirical evidence is needed to better understand mechanisms for improving agricultural adaptability of marginalized social groups, particularly women (Davidson, 2016), given such groups are expected to experience climatic and broader social-ecological change impacts more intensely than other members in rural society (Vermeulen et al., 2012).
We begin this paper by describing our dataset and the analytical techniques used (sections Dataset and Study Site and Structural Equation Modeling: A Brief Overview). We then explain the value of structural equation modeling, given it has not been widely applied in the agricultural livelihood adaptation literature (but see Niles et al., 2013; Le Dang et al., 2014; Toma et al., 2018). In section Results, we present our results, with a specific focus on the extent to which capital mediates the relationship between household characteristics and crop, land, and farm (livestock) adaptation strategies in the IGP. We then discuss key findings from our results and provide recommendations for policy-makers focused on improving adaptation for marginalized groups (section Discussion: Key Findings for Policy-Makers). We conclude, learning from our nuanced set of results, providing avenues for future research in adaptation studies.
Dataset and Study Site
This paper uses the Climate Change, Agriculture, and Food Security (CCAFS) 2010–2011 household survey dataset collected by the CGIAR-led CCAFS research program in South Asia (India, Nepal, and Bangladesh) (CCAFS, 2015: http://doi.org/10.7910/DVN/IUJQZV). Standardized baseline instruments were used to collect information on household-level demographics, assets, livelihood information, experienced crises, current risk management strategies, and adaptive management practices from all CCAFS research sites (e.g., Bhatta et al., 2013; Singh, 2013). We focus our analysis within India. The data was collected by the CCAFS research team using a specified baseline sampling framework (per Kristjanson et al., 2011). Within Bihar and Haryana, selected “sites” were sampled. The specific criteria for site selection included but were not limited to considerations around agro-ecological production, socio-economic and demographic information, anticipated environmental change, and different land tenure arrangements (ibid, see p. 6). The sample population comes from eight sites in Bihar and one site in Haryana (Karnal District). Following the CCAFS sampling framework, a 100 km2 block or area for each site was developed. The CCAFSs research team then selected seven villages at random from that block (Kristjanson et al., 2011; Bhatta et al., 2013; Singh, 2013). Once the villages were selected, a comprehensive list of households was generated using voter lists and census data (ibid). From here, 20 households were randomly selected per village with the baseline instrument executed by trained enumerators in Hindi (ibid). The dataset we use contains records for 1,259 agricultural households across nine sub-regional sites across both states (Figure 1). For a more complete description of study sites and sampling methodology, please refer to baseline survey reports (Kristjanson et al., 2011; Bhatta et al., 2013; Singh, 2013).
Our central research objective involved providing recommendations that improve farm household adaptation in different demographic and social-ecological contexts. For that reason, we are not interested in undertaking a comparative state-wise analysis; instead, we direct interested readers to related research describing major differences between these sampled sites (e.g., Bhatta et al., 2013; Singh, 2013). That said, there are important differences between the districts. Karnal is a well-off district (1.5 million people, 417 inhabited villages) in north-eastern Haryana, with about 45% of “main workers” (>6 months of work per year) engaged in agricultural activities (Government of India, 2011a). Known as the “rice bowl” of Haryana, Karnal has been the highest-ranking district in the state on a per hectare yield (ibid, p. 17). Households sampled commonly own large livestock, and cultivate rice, wheat, vegetables, and engage in other related livelihood, likely including paper mills, sugar factories, agricultural implements (Government of India, 2011a; Singh, 2013). Vaishali, located in central Bihar, is one example of a much larger site (3.5 million people, 1,422 inhabited villages) and is heavily focused on rainfed agriculture, largely wheat, maize, and rice (Government of India, 2011b; Bhatta et al., 2013). There are differences in caste structure and distribution in our sample (i.e., Economically Backward Caste is specific to Bihar; General Caste concentration is higher in Haryana); land size (i.e., historical process in Haryana leading to larger land-sizes, Rathakrishnan and Kumar, 2014); agricultural crop production (i.e., Haryana mainly grows wheat and rice, with Bihar growing rice, wheat, and maize mix); and in hydro-climatic risks (i.e., vast majority in Bihar reported having experienced such risks). Table 1 compares demographic, social, and economic household-level information across both states. More, and clear from the Table 1, the recorded observations are unevenly distributed between Haryana (n = 140) and Bihar (n = 1,119).
Structural Equation Modeling: A Brief Overview
We developed a series of structural equation models (SEMs) using R-3.4.2 (Lavaan package) and Stata 13.1. The use of two statistical programs served as a means of quality assurance and control (we report statistics using R-3.4.2). SEMs are a powerful multivariate technique used to statistically assess complex system-wide relationships (Grace, 2006; Grace et al., 2010; Kenny, 2014). An important value-added contribution of the SEM approach is the ability to assess hypothesized relationships between variables, using observed and non-measured (“latent”) variables (ibid). We apply SEM using “mediated modeling” (Baron and Kenny, 1986; MacKinnon et al., 2007; Hayes, 2009; Zhao et al., 2010). Where conventional techniques use x and y variables, SEMs allow for the inclusion of a third component—a mediator. A mediator represents a well–theorized variable believed to affect the relationship between, in our case, a given household characteristic (x) and household adaptation behaviors (y). A hypothesized mediator can explain an observed effect either completely, or partially (Baron and Kenny, 1986). Following Baron and Kenny (1986), Zhao et al. (2010) outline “non-mediation” (a direct-only effect), “full mediation” (an indirect-only, without a “direct” effect), and “partial mediation” (a complementary, or competitive effect) as possible relationships (see Figure 2). These effects can be in the same or different directions. Iacobucci (2008) and Zhao et al. (2010, p. 198) suggest that full mediation remains the “gold standard” but is not commonly found in practice. The meaningful presence of a mediator provides an opportunity to better understand forces underlying and shaping observed adaptation patterns across differentiated strata of rural households, and thus, serves as a critical entry-point for targeted policy interventions.
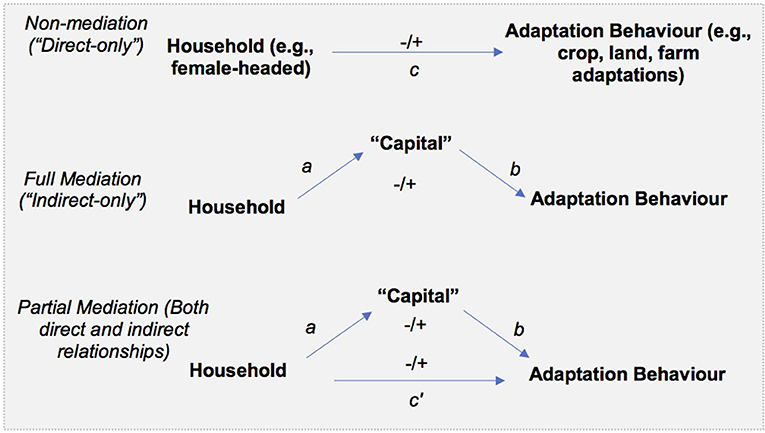
Figure 2. Diagram illustrating different mediation effects. Top: Non-mediation or the total effect (c). Middle: Full mediation (a × b); Bottom: Partial mediation where the indirect path (a × b), or the mediated effect explains only some of the relationship. Adapted from Zhao et al. (2010).
Explained below, we use three dependent variables: (i) crop management strategies; (ii) land management strategies; and (iii) farm or animal/fish management strategies in both Bihar and Haryana (i.e., six SEMs in total). Each model is comprised of three components: (i) a well-theorized set of social and economic household-level variables that have a theoretical association with adaptation and adaptability (our independent variables); (ii) an aggregated household-level capital variable (our mediator), hypothesized to mediate the effect between a household and its adaptation behavior; and (iii) the number of crop, livestock, and land adaptive behaviors engaged in by households (our dependent variables). We carefully specified each of the three components using literature reviews as well as well-accepted statistical measures, with the processes outlined below. We used the maximum likelihood robust (MLR) estimation in computing the effects of each SEM. The MLR computes robust (Huber-White) standard errors and a scaled test statistic equal to the Yuan-Bentler test statistic and can help protect against heteroscedasticity and non-normality (Lai, 2018).
Household-Level Social and Economic Variables (Independent Variables)
As signaled in our introduction, not all farm households are able to access agricultural adaptation solutions evenly and equitably. We adopt a theoretical framework where adaptation processes are differently shaped by social and economic systems that characterize household difference. This perspective leans on a rich tradition of social constructivism and coupled social-ecological models of vulnerability in agrarian systems (e.g., Watts and Bohle, 1993; Blaikie et al., 1994; Ribot, 1995). We selected a subset of independent variables within or based off of the CCAFS household survey, including gender, caste, land ownership, education, distance to urban roads, and family dependents—all of which have been theorized or evidenced in the global and Indian livelihoods adaptation literature to affect adaptation behavior (Table 2). To protect against multicollinearity in our models, we followed a stepwise model building procedure to narrow the list of independent household variables. In our stepwise approach we examined correlation matrices amongst included variables and SEM model fit statistics [e.g., Comparative Fit Index (CFI), Root Mean Square Error of Approximation (RMSEA), Standardized Root Mean Square Residual (SRMR) and Akaike Information Criterion (AIC)] to guide inclusion/exclusion decisions (Kenny, 2014). Households with identifiable social and economic characteristics serve as clear entry-points for policy-making intervention, if capital is shown to mediate their relationship with adaptation outcomes. Our models used 959 and 139 household observations for Bihar and Haryana, respectively. We excluded observations where records were incomplete or erroneous (e.g., incomplete or incorrect land units). In Haryana, we also excluded the sole “Economically Backwards Caste” observation (n = 1) to avoid inappropriate estimates. Table 2 summarizes the household variables and rationalizes their inclusion in our SEMs.
Household Capital (The Mediator)
In our introduction, we highlighted that household capital is often understood as an indicator of adaptability. To construct our capital variable, we used nearly 80 different household measurements in the CCAFS survey instrument, which represent human, natural, financial, physical, manufactured, and social capital (see Table 3). Supplementary Material #1 lists the used set of binary measurements of capital which were grouped as six indices, namely; (1) received weather information in the last year; (2) household production diversity; (3) household commercialization diversity; (4) household asset index; (5) household infrastructure index; and (6) household social capital index (Table 3). Table 3 describes these six data-driven indices and relates them to commonly referenced capital categories within the Sustainable Livelihoods Approach (SLA, e.g., Scoones, 1998). As shown in Supplementary Material #1, the number of underlying constructs used to measure each capital index varies from five (received weather) to 21 (household asset index).
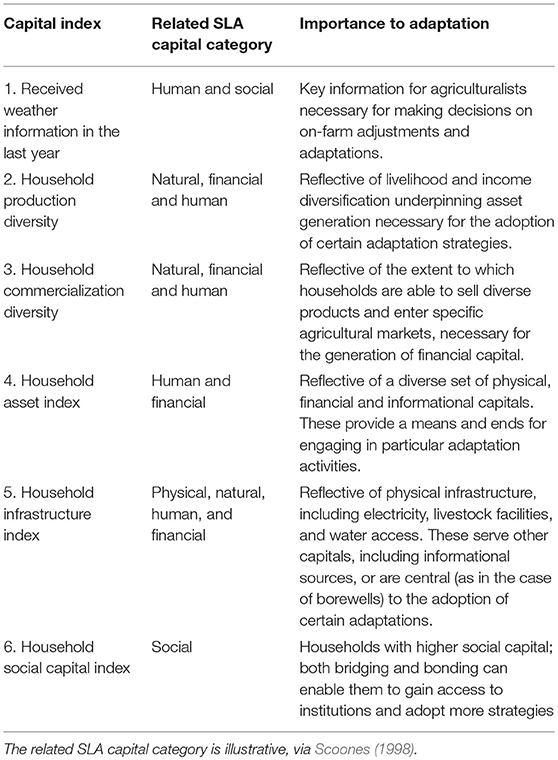
Table 3. Description of the six capital data-driven indices and evidence of their relationships or associations with adaptation.
We used a multi-step data reduction statistical process to go from nearly 80 inquired upon variables to one aggregated capital variable, largely to retain statistical power. First, we constructed standardized indices for each of the six different capitals-indices. Six index values were produced for each of the 1,259 households. Second, using these values, we ran correlation matrices and a Cronbach's Alpha test (n = 1,259, standardized α coefficient = 0.78) finding that these six capital indices had high internal reliability amongst themselves. Third, we took the Cronbach's Alpha value as justification for the inclusion of a Confirmatory Factor Analysis (CFA) within each SEM to construct a single latent capital variable, which is not measured but inferred as the underlying construct influencing each of the six measured or observed capitals indices. A higher relative latent capital score could indicate a sizeable concentrations of few capital categories, or fewer holdings across several categories. This latent variable constructed within each SEM serves as the single mediating variable and allows for the SEM to test the mediating role of capital between household characteristics and adaptive behaviors. To account for correlations between capital variables, we allowed the error terms of the measured capital indices to co-vary in the SEM.
The choice to aggregate the household capital indices emerged as a result of testing and comparing a number of different SEM model structures to obtain the best model fit and the most parsimonious model. We tested SEM structures with no household capital aggregation (i.e., six mediating variables), SEM structures with some aggregation (e.g., three latent variables), and the final aggregated model with all six household capitals composing one latent capital variable. The aggregated model was the optimal solution in terms of model fit statistics and parsimony. Furthermore, the combination of capitals indices into a latent, aggregated variable was in line with our theoretical understanding of capitals as interrelated and interlocking.
Adaptive Behaviors (Dependent Variables)
Crop, land-management, and livestock adaptations are key undertakings popular in the IGP region of India (Ojha et al., 2014; Khatri-Chhetri et al., 2016; Bhatta et al., 2017). In our sites, households adapt to a wide range of climatic and non-climatic stressors and opportunities. Supplementary Material #2 provides a list of reasons why farmers engage in land, crop, and livestock adaptations. In both Bihar and Haryana, common reasons for land and crop adaptations include better crop yields and prices, new market opportunities, less rainfall, lower groundwater tables, less productive land, and more expensive labor. Livestock adaptations are occurring largely to fetch households better prices across both states. In Haryana, more specifically, more cold spells and the risk of pest and diseases were cited by over 30% of households as being an important reason for adopting land/crop, and livestock adaptations, respectively. Farmers have differential capacity to engage in these strategies to redress harm or capitalize on opportunities resultant from hydro-climatic change. The CCAFS instrument surveyed over 50 different adaptation strategies nested under the aforementioned categories of crop, land, and farm adaptation behaviors. Land management behaviors include altered irrigation practices, water conservation strategies, planting times, integrated crop management, and intercropping. Crop adaptations include introducing higher yielding, flood, drought, and disease resistant variants. Farm (livestock) management strategies include fodder storage, new types of breeds or livestock, and farming of improved pastures. In line with Section Household Capital (The Mediator), we formulated three dependent variables (unstandardized count values) based on over 50 listings by using simple data aggregation techniques. Our decision to quantify adaptation behaviors into three aggregated count variables originated from our research objective to explore adaptation actions for broader agricultural categories (crop, land, livestock). By examining crop, land management, and livestock changes, our analysis is specific enough to target relevant policies that are in place but still allows us to derive meaning across heterogenous sites. Rather than making inferences over the importance of individually specific adaptation strategies within diverse regions, we use the overall quantity of adaptation categories as an index on which to examine adaptation. Each crop, land, and farm adaptation index represent the number of unique adaptation strategies a household has adopted. Supplementary Material File #3 lists all adaptation strategies by the crop, land, and farm groupings. Figure 3 provides a schema of our mediated models.
Results
This section synthesizes the detailed set of results for the six structural equation models across Bihar and Haryana for households' three types of adaptation behaviors: (i) land management, (ii) crop management, and (iii) farm (livestock) management adaptation strategies. We present the coefficient value and p-value in parentheses below with regards to the results in-text. The beta value can be interpreted as the average change in the dependent variable (i.e., number adaptation behaviors) for every one unit increase in the predictor variable. Table 4 shows a simplified chart highlighting instances where household capital fully mediates the relationships between a given household characteristic and their adaptive behaviors (see Supplementary Material #4 for the complete summarization).
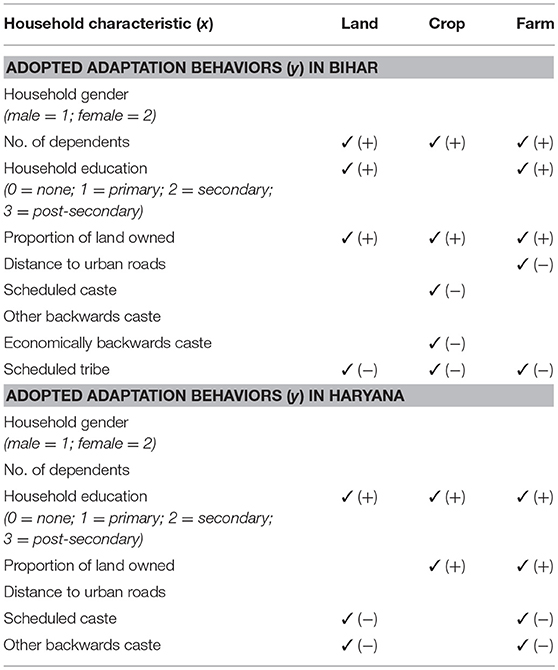
Table 4. Instances where household capital fully mediates (indirect-only) the relationships between a given household characteristic and their adaptation behavior (✓ = p < 0.05) in Bihar and Haryana (± indicates direction of the relationship).
Land Management Adaptations
The land adaptation model in Bihar has an acceptable goodness of fit score (Robust CFI: 0.846; Robust RMSEA: 0.088; SRMR: 0.056) while the Haryana model has an excellent fit (Robust CFI: 0.96; Robust RMSEA: 0.061; SRMR: 0.04; Table 5). Several similarities exist between both states. First, we find a significant positive relationship between household capital, and adopted land management adaptations in Bihar (β = 6.59, p ≤ 0.01) and Haryana (β = 8.92, p ≤ 0.01). Second, we observe a significant positive indirect-only relationship between household education level and the number of land adaptations adopted in Bihar (β = 0.49, p ≤ 0.01) and Haryana (β = 0.50, p ≤ 0.05). That is, household capital fully mediates the relationship between higher educated households and their higher adoption of land adaptation strategies.
We also find several differences between both states. A first difference involves the proportion of land a household owns. In Bihar, a significant positive indirect-only relationship exists between the number of adopted land adaptation strategies and the proportion of land owned (β = 0.37, p ≤ 0.01). Said differently, household capital fully mediates the relationship between households with a greater proportion of owned land and their higher uptake of land management activities. In Haryana, a significant negative direct relationship exists between the adoption of land adaptations and the proportion of land owned (β = −0.95, p ≤ 0.05). Here, household capital—although higher for those with a greater owned share of land—serves no statistical role in explaining land-based adaptations.
Second, in Bihar a significant positive indirect-only relationship exists between the number of household dependents and number of land adaptations (β = 0.09, p ≤ 0.01). In other words, households with more dependents adopt more land-based adaptations—an effect mediated by household capital. We do not observe this effect in Haryana. The third difference concerns households located farther from urban roads. A significant positive direct relationship exists between the distance to urban roads and the number of land-based adaptations in both Bihar (β = 2.90, p ≤ 0.01) and Haryana (β = 29.72, p ≤ 0.01). Interestingly, households that are farther from urban roads engage in significantly more land-based adaptations in each state. Conflictingly, a significant negative indirect relationship also exists in Bihar (β = −1.18, p ≤ 0.05) indicating “competitive mediation” (Zhao et al., 2010). Unpacking this, distance from an urban road has a significant negative effect on household capital (β = −0.18, p ≤ 0.01), yet household capital has a significant positive effect on land adaptation. While households farther from roads engage in more strategies (the direct effect), more road distance equates to less capital simultaneously available for adaptation. Hence, an omitted mechanism likely exists for explaining the positive effect in Bihar (Zhao et al., 2010).
Last, we focus on the complex relationships between caste and land management changes. The results are reported in relation to the General Caste level1. In Bihar, a significant negative indirect-only relationship exists between Scheduled Tribes (β = −0.63, p < 0.01) and the number of land adaptations—an effect fully mediated by household capital, which is on average lower for ST compared to upper castes. Although this effect was significant, we caution readers of the low sample size (n = 8) in the Bihar dataset (see section Limitations). We find significant positive direct (β = 1.24, p ≤ 0.01) and a significant negative indirect (β = −0.49, p ≤ 0.01) relationships between Economically Backwards Castes (EBC) and the number of land adaptations undertaken (“competitive mediation”). That is, EBC households adopt more land management strategies in comparison to General Caste households; however, this is likely explained by an omitted mediator given their lower capital scores detract from adaptation uptake. In Haryana, a significant negative indirect-only relationship exists between both Scheduled Castes (SC) (β = −3.15, p ≤ 0.01) and Other Backward Castes (OBC) (β = −2.06, p ≤ 0.01), and their land adaptations. Here, household capital—much lower for SC and OBC—mediates the relationship between these lower castes and their fewer uptake of land-based adaptation strategies.
Crop Management Adaptations
The crop adaptation models in Bihar (Robust CFI: 0.895; Robust RMSEA: 0.070; SRMR: 0.044) and Haryana have excellent goodness-of-fit scores (Robust CFI: 0.979; Robust RMSEA: 0.043; SRMR: 0.037). We find three similar relationships between both states. First, consistent with land-based adaptations (section Land Management Adaptations), there is a significant positive relationship between the household capital latent variable and adopted crop management adaptations in Bihar (β = 1.48, p ≤ 0.05) and Haryana (β = 3.61, p ≤ 0.01). Second, we find significant positive relationships between household education level and the number of adopted crop adaptations. Here, household capital partially mediates this relationship in Bihar (direct: β = 0.27, p ≤ 0.01; indirect: β = 0.08, p ≤ 0.05), and all of it in Haryana (β = 0.21, p ≤ 0.05). Third, the proportion of land owned has a significant positive indirect-only relationship with crop adaptations in Bihar (β = 0.06, p ≤ 0.05) and Haryana (β = 0.38, p ≤ 0.05). That is, household capital (increasing with the share land ownership) fully mediates this relationship.
We also find several notable differences. In Bihar, a significant positive indirect-only relationship exists between the number of household dependents and the number of crop adaptations made (β = 0.02, p ≤ 0.05). In other words, household capital fully mediates the relationship between a higher number of dependents and higher adoption of crop adaptations. This relationship was not statistically observed in Haryana. Second, in Bihar, we find a significant positive direct effect between distance to urban roads, and the number of crop adaptations (β = 3.09, p ≤ 0.01); but, at the same time a significant negative indirect relationship also exists where household capital acts as a mediating factor which detracts from crop adaptations (β = −0.22, p ≤ 0.05). Distance from an urban road has a significant negative effect on household capital, which affects adaptation uptake. Thus, an omitted mechanism likely explains this positive relationship. In Haryana, a significant positive direct relationship exists between distance to urban road and the number of crop strategies adopted (β = 28.96, p ≤ 0.01).
Last, caste continues to demonstrate diverse relationships. In Bihar, a significant negative indirect-only relationship exists between Scheduled Tribes (β = −0.11, p ≤ 0.05), Scheduled Castes (β = −0.13, p < 0.05) and EBC (β = −0.102, p < 0.05), and crop-based adaptations—an effect mediated by household capital. In contrast, a significant positive direct effect (β = 0.62, p < 0.01) and a significant negative indirect effect (β = −0.08, p < 0.05) exist between OBC and the number of crop management changes (“competitive mediation”). In Haryana, significant negative direct and indirect relationships exist between SC (direct: β = −3.15, p ≤ 0.01; indirect: β = −1.14, p ≤ 0.01) and OBC (direct: β = −1.09 p ≤ 0.01; indirect: β = −0.64, p ≤ 0.05), and the number of crop adjustments made. This effect is partially mediated by their household capital—which is lower than General Castes. The full results for the crop model in Bihar and Haryana are presented in Table 6.
Farm (Animal/Fish) Adaptation Strategies
The farm adaptation models have acceptable goodness-of-fit scores in Bihar (Robust CFI: 0.837; Robust RMSEA: 0.090; SRMR: 0.052) and excellent fit in Haryana (Robust CFI: 0.955; Robust RMSEA: 0.060; SRMR: 0.04). In regard to farm (livestock) adaptations, we observe three important similarities between both states. First, and similar to the land and crop models, we find a significant positive relationship between the household capital latent variable and adopted farm management adaptations in Bihar (β = 4.15, p ≤ 0.01) and Haryana (β = 4.95, p ≤ 0.01). Second, a significant positive indirect-only relationship exists between household education level and the number of farm adaptations adopted in each state (Bihar: β = 0.27, p ≤ 0.01; Haryana: β = 0.30, p ≤ 0.05). That is, household capital—which, once again is on average higher for more educated households—fully mediates these relationships. Third, this same indirect relationship holds in each state for households with a greater proportion of land owned (Bihar: β = 0.21, p ≤ 0.01; Haryana: β = 0.50, p ≤ 0.05).
Like the above adaptations, differences exist. First, in Bihar, we find a significant positive indirect-only relationship between households with more dependents and the number of farm management adaptations (β = 0.06, p ≤ 0.01), but not in Haryana. Second, in Bihar, we see a significant negative indirect-only relationship between distance to urban roads and the number of farm adaptations adopted (β = −0.62, p ≤ 0.01). This effect was not found in Haryana. Last, as with land and crop adaptations, we observe a significant negative indirect-only effect between Scheduled Tribes (β = −0.30, p < 0.01) and the number of farm adaptations in Bihar. Competitive mediation exists for SC, EBC, and OBC households and their relationship with farm adaptation strategies wherein a significant positive direct effect co-exists with significant negative indirect effects. While SC, EBC, and OBC households adopt more farm management strategies in relation to their General Caste counterparts, household capital is not driving this relationship. Rather, it detracts from it, given these households have lower capital scores for adaptation. In contrast, in Haryana, we observe a significant negative indirect-only effect between SC (β = −1.67, p < 0.01) and OBC (β = −1.05, p < 0.01), and adopted farm adaptation activities—an effect fully mediated by household capital. We present clarified highlights of these trends in section Discussion: Key Findings for Policy-Makers.
Discussion: Key Findings for Policy-Makers
We found that household capital is a significant and positive predictor of the number of land, crop, and farm (livestock) strategies adopted by households across Haryana and Bihar (Tables 5–7). That is, a higher composition of household capital is related to households engaging in more adaptation behaviors, even under multiple research sites with differing demographic, agricultural, economic, and institutional contexts. More importantly, we found that household capital fully mediates adaptation behavior for a sub-set of the sampled population. This was for around 48% of the relationships between a given household characteristic and their adaptation behaviors. We highlight key findings below with the intent of showcasing policy recommendations (Table 8) that are aligned with supporting and enhancing adaptation for certain marginalized sub-groups within our sample.
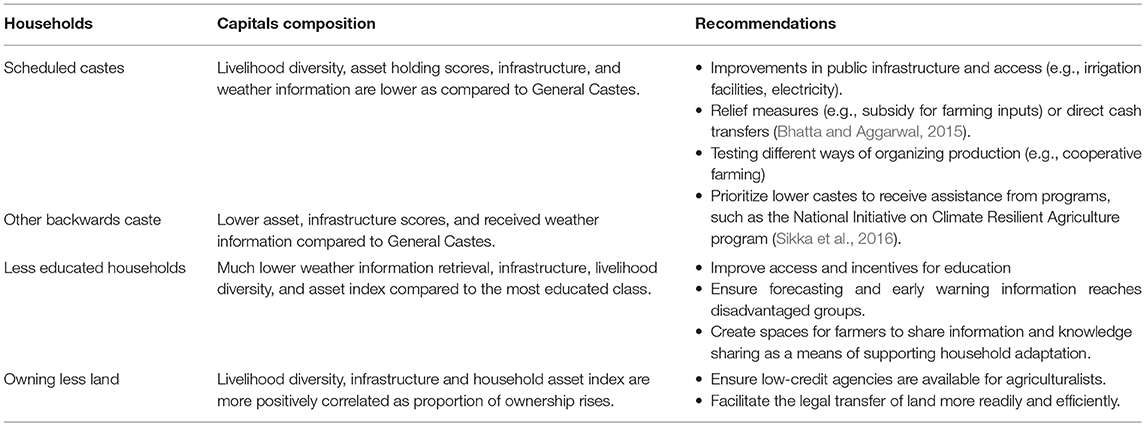
Table 8. Short-term policy recommendations across Bihar and Haryana for disadvantaged groups where household capital was observed to mediate adaptation.
Education and Land Ownership
First, more educated households engage in more adaptation strategies in both geographical contexts—an outcome fully mediated by their higher capital scores. As education increases, so do mean scores for each of the six capital variables, with noticeable improvements in owned assets and infrastructure, production diversity, and received weather and climate information. One possible, and well-theorized, explanation for this observed mediated pattern is that higher levels of education—linked with more assets (e.g., radios, television, internet, mobile phones) and exposure to climate information—could influence farmers' perception of climate risks and facilitate livelihood adaptations (see Deressa et al., 2009; Lutz et al., 2014; Kibue et al., 2016; Tripathi and Mishra, 2017). One key recommendation includes stronger diffusion of weather forecasting and early warning systems to facilitate adaptation, particularly for less-educated households (Aggarwal, 2008; Lobo et al., 2017; Table 8).
Second, households with a higher proportion of owned land adopt more adaptation strategies across both states—an outcome fully mediated by their higher composition of capital. Research shows that as the ownership of land2 increases, so can a household's capacity to invest in sustainable crop, farm and land based strategies (Deininger, 2003). One possible reason this pattern emerges is because such households are better positioned to “mobilize,” or gain access to more capital, either via institutional opportunities that exist with larger land ownership, or through incentives for land investments (e.g., ibid).
Caste and Community
Highlighted above, caste exhibits differentiated effects on agricultural adaptation. In certain cases, and particularly in Haryana, SC, and OBC's lower capital score fully mediates and explains lower adaptation action, relative to General Castes. The same can be said consistently for Scheduled Tribes in Bihar, and with EBC, SC, and OBC when it comes to crop adaptations. Thus, in a number of cases, capital fully mediates the lower adoption of agricultural management adaptations for lower castes in different institutional and geographic contexts. In more limited cases, notably land and farm adaptations in Bihar, we observe negative indirect effects as well as simultaneous positive direct and total effects of SC, EBC, and OBC households. This indicates higher adaptation uptake relative to General Castes. However, household capital is not driving this effect. While another mechanisms or mediator exists in these cases, we maintain that improving capital scores can in fact increase adaptation, even in the presence of other unaccounted mediators.
As an aggregated sample, General Castes have a higher mean score in each of the six major capitals, as compared to both SCs and OBCs. However, prominent differences exist with respect to certain capitals. Received weather information, infrastructure, and asset holdings are much lower for OBCs as compared to General Castes. The same holds true for SCs; however, their production diversity is substantially lower than General Castes. Based on our data analysis, SC and OBC households could more effectively adopt adaptation strategies if the household capitals mentioned above were strengthened. Further, a broader set of non-climate strategies such as effective soil, water, and nutrient management could be essential for enabling households to engage in climate change adaptation, including livelihood diversity (Chaudhari and Mishra, 2015).
The Climate Smart Village3 program adopted by the government of India in 151 vulnerable sites in India aims to mobilize and empower communities through decentralized decision making on coping and adapting while also stabilizing and improving agricultural production (Rao et al., 2016; Aggarwal et al., 2018). Aggarwal et al. (2018) demonstrate important gains in rice-wheat yields, income, water conservation, and emissions reduction with altered tillage practices, residue incorporation, and land-leveling in multiple sites in northern India. However, it remains unclear to what extent social and economic difference play in shaping adoption rates within villages. Improving the capital holdings of SC and OBC households could enable them to partake in these initiatives as well. In this line of reasoning, lower castes could be prioritized to receive assistance from ongoing programs, such as the National Initiative on Climate Resilient Agriculture Program, which facilitates access to agricultural machinery in support of climate resilient cultivation practices (Sikka et al., 2016).
Our results suggest household capital mediates the observed effects between adaptation, and certain factions of our sample that have lower education, less owned land, and belong to lower caste communities. These are broader, noteworthy results for policy approaches focused on just, and equitable adaptation and are not universal across all adaptation behaviors in both states. Nevertheless, where we do find evidence that “disadvantaged” groups adopt fewer strategies, policy interventions that raise household-level capital scores can be an immediate and effective means of increasing adaptation. Table 8 reflects illustrative examples where possible improvements in capital could be made for specific groups. While improvements in any capital category will, in theory, improve adaptation adoption, these recommendations reflect areas where mean scores of certain capitals are lower for certain disadvantaged members of socio-economic groups. As such, they reflect relevant areas for intervention.
Limitations
There are some important limitations of our approach we wish to address. We are using the number of adaptation strategies engaged in as an indicator of flexibility, choice, and response diversity (Bhatta et al., 2017). We assume more adaptations or innovations is a positive characteristic, even though we understand that some individual interventions or particular combinations thereof may eventually not result in climate-smart strategies in certain contexts. We recognize that this is an imperfect proxy for “vulnerability” (a relational concept we engage with in limited fashion in this paper) given households who adopted fewer strategies may have found success in these and opted not to pursue other strategies.
Second, the nature of our analysis does not allow us to infer whether specific household capitals, or varying combinations of capital, have a stronger effect on adoption rates. We cannot identify mediating relationships between independent and dependent variables at a level of specificity greater than the aggregated capital mediator (i.e., mediation by specific capital indices). However, we are able to examine relative capital indices for certain groups, which allow us to identify promising areas for increased efforts to enhance adaptation and adaptive capacity.
Third, while the CCAFS implements a random sampling design, more data from female-headed households is required in order to better assess differential adaptive capacities in the IGP. The sample size of female-headed households in both Haryana (n = 4) and Bihar (n = 28) was small and likely unsuitable for inferential testing. As a result, we caution against the interpretation that no observed adaptation differences in female and male-headed households exist. While we cannot provide a robust results, our descriptive statistical findings are aligned with scholarship recommending women's access to finance, social networks, knowledge, and their participation in formal agricultural institutions should be improved (e.g., Sugden et al., 2014; Chaudhari and Mishra, 2015). For example, in Bihar female-headed households (n = 28) had lower livelihood, commercial diversity, and asset holding scores compared to male-headed households, echoing findings that female ownership and access to assets, networks, and exclusion from formal agricultural institutions may be important factors shaping adaptation behavior (Ahmed and Fajber, 2009; Sugden et al., 2014; Perez et al., 2015; Mehar et al., 2016; Niles and Brown, 2017). We call for more research, particularly given the context where the scale and rate of male out-migration (especially in Bihar) is restructuring conventional gender roles in agriculture (Datta and Mishra, 2012; Aryal et al., 2014). The same disclosures apply equally to the effect of Scheduled Tribes on agricultural adaptations, particularly in Bihar where the sample size was small (n = 8).
Fourth, income is likely to be correlated with other household capital variables. We were unable to control for this variable, as it was unmeasured and unquantified in the household survey. Fifth, we acknowledge that adaptation decisions involve complex socio-cultural and human decision-making factors (e.g., Adger et al., 2012) in addition to household capital. That said, we hypothesize—using insights from the agrarian livelihoods, development, and environmental change literatures—capital remains but one critical entry point for improving adaptations, innovations, and experimentations. Last, we recognize there could be other estimations of the structural equation models used to better handle count data.
Future Research
The use of mediation allows us to evaluate the contribution household capital makes in affecting the relationships between socially-economically differentiated households and their adaptation behavior. In numerous cases, we find evidence of no mediation or partial mediation. The implications of both non-mediation and partial mediation are that our theorization and construction of the mediator variable are incomplete and room exists for exploring underlying drivers of adaptation adoption (Zhao et al., 2010). But in the case of non-mediation, we caution against a reading that capital itself is unimportant. Rather, this should be interpreted as capital—constructed here—requires expansion or refinement, or the underlying metrics through which capital is surveyed does not mediate adaptive behavior patterns. In addition to metric development, we speculate that there could be other, non-material forms of capital that are mediating the relationship between a given sub-set of households and their adaptive decisions. In cases of partial mediation, household capital mediates observed behavior, but cannot account for it in full. The above recommendations around metric re-examination and other, non-surveyed factors are future areas of research, particularly for “complementary” effects (same signs). In fewer cases, we see negative “competitive” relationships (opposite signs) between household capital and adaptation behavior (Zhao et al., 2010). This means that household capital mediates, all factors equal, relationships where a sub-set of households adopt less of a given strategy, such as crop variants; however, there is an additional factor at play that is allowing the uptake of said strategies. The identification of other factors—including those that extend beyond an individual household and branch into social-ecological systems properties supportive of adaptation—could help explain adaptation outcomes.
Conclusions
This paper developed six robust SEMs to explore the relationships between household capital and diverse agricultural adaptation strategies for over 1,000 randomly selected farm households in Bihar and Haryana. The models sought to identify the extent to which capital mediates the relationship between household social and economic difference, and adaptation strategies in a heterogeneous population with different geographical and institutional contexts. To accomplish this, we statistically constructed a single capital variable by aggregating nearly 80 different household measurements, which were reflective of households' financial, physical, human, natural, and social capital. We found household capital-holdings significantly predict the number of adaptation strategies households adopted. More specifically, we find in certain cases that households with a higher education level and a greater proportion of owned land adopt more adaptation strategies—an outcome fully mediated by their (higher) household capital scores. Further, the (lower) household capital scores often mediate the relationships between lower castes and their engagement with fewer agricultural adaptation strategies in key cases.
Our robust and carefully specified SEMs suggest that increased adoption of adaptation strategies can occur by raising the overall household capital score, especially for disadvantaged groups. In the short-term, we recommend programs that improve (i) access to public and private agricultural infrastructure for lower castes; (ii) education and shared knowledge spaces for less-educated households to increase adaptation; and (iii) the availability of low-interest loans and the more efficient legal transfer of land for agriculturalists owning a smaller proportion of their utilized land. While we recognize more adaptation does not overlap neatly with complex conceptions of vulnerability, we maintain that the ability to engage in more strategies is critical given the uncertainty posed by active processes of hydro-climatic change and approaching environmental limits (Zacharias et al., 2014). In sum, our results suggest that improvements in household capital can improve adaptability in the immediate term for households in the IGP.
Author Contributions
CHW organized the database. CHW and SS performed the statistical analysis. SS wrote the first draft of the manuscript. All authors contributed to the conception and design of the study, contributed to manuscript revision, read and approved the submitted version. MTN provided advice and oversight of the statistical approach.
Funding
Funding This work was supported by the National Socio-Environmental Synthesis Center (SESYNC) under funding received from the National Science Foundation DBI-1052875.
Conflict of Interest Statement
The authors declare that the research was conducted in the absence of any commercial or financial relationships that could be construed as a potential conflict of interest.
Acknowledgments
We extend our thanks to Drs. Ian Carroll, Kelly Hondula, and Philippe Marchand for their statistical support. We are grateful to Drs. Margaret Palmer, Jonathan Kramer, and Nicole Motzer for their support in hosting the SESYNC Proposal Workshop and in their continued guidance in support of this funded Graduate Student Pursuit Project. We thank the EDGES Research Collaborative for helpful feedback. We are grateful to the CGIAR Research Program on Climate Change, Agriculture and Food Security (CCAFS) for collecting the household data, and to the respondents who participated in the baseline survey. Finally, we thank the Editor and the two reviewers for their thoughtful and incisive comments on our submission. An earlier version of this paper was presented at the American Association of Geographers in Boston.
Supplementary Material
The Supplementary Material for this article can be found online at: https://www.frontiersin.org/articles/10.3389/fsufs.2019.00001/full#supplementary-material
Footnotes
1. ^Often taken in many contexts as the highest-ranking caste.
2. ^A wider scholarship examines how land shortage, landholding size, and/or tenure can affect adaptation practices (e.g., Deressa et al., 2009; Shah, 2015; Singh et al., 2016).
3. ^Climate Smart Village program initiated by the CGIAR promotes practices such as agricultural diversification, conservation practices, tolerant variants, insurance-based programs and agro-advisory extension services (see Aggarwal et al., 2018).
References
Abid, M., Ngaruiya, G., Scheffran, J., and Zulfiqar, F. (2017). The role of social networks in agricultural adaptation to climate change: implications for sustainable agriculture in Pakistan. Climate 5:85. doi: 10.3390/cli5040085
Adger, W. N., Barnett, J., Brown, K., Marshall, N., and O'Brien, K. (2012). Cultural dimensions of climate change impacts and adaptation. Nat. Clim. Chang. 3, 112–117. doi: 10.1038/nclimate1666
Adger, W. N., and Kelly, P. M. (1999). Social vulnerability to climate change and the architecture of entitlements. Mitigat. Adapt. Strat. Global Change 4, 253–266. doi: 10.1023/A:1009601904210
Agard, J., Schipper, E. L. F., Birkmann, J., Campos, M., Dubeux, C., Nojiri, Y., et al. (2014). “Glossary,” in Climate Change 2014: Impacts, Adaptation, and Vulnerability. Part A: Global and Sectoral Aspects. Contribution of Working Group II to the Fifth Assessment Report of the Intergovernmental Panel on Climate Change, eds C. B. Field, V. R. Barros, D. J. Dokken, K. J. Mach, M. M. D, T. E. Bilir, et al. (Cambridge; New York, NY: Cambridge University Press), 1757–1776.
Aggarwal, P. K. (2008). Global climate change and Indian agriculture: Impacts, adaptation and mitigation. Ind. J. Agric. Sci. 78, 911–919.
Aggarwal, P. K., Jarvis, A., Campbell, B. M., Zougmoré, R. B., Khatri-Chhetri, A., Vermeulen, S. J., et al. (2018). The climate-smart village approach: framework of an integrative strategy for scaling up adaptation options in agriculture. Ecol. Soc. 23:14. doi: 10.5751/ES-09844-230114
Aggarwal, P. K., Joshi, P. K., Ingram, J. S. I., and Gupta, R. K. (2004). Adapting food systems of the Indo-Gangetic plains to global environmental change: key information needs to improve policy formulation. Environ. Sci. Policy 7, 487–498. doi: 10.1016/j.envsci.2004.07.006
Ahmed, S., and Fajber, E. (2009). Engendering adaptation to climate variability in Gujarat, India. Gender Dev. 17, 33–50. doi: 10.1080/13552070802696896
Andersson, J. A., and D'Souza, S. (2014). From adoption claims to understanding farmers and contexts: a literature review of Conservation Agriculture (CA) adoption among smallholder farmers in southern Africa. Agric. Ecosyst. Environ. 187, 116–132. doi: 10.1016/j.agee.2013.08.008
Apata, T. G., Samuel, K. D., and Adeola, A. O. (2009). “Analysis of climate change perception and adaptation among arable food crop farmers in South Western Nigeria,”in Presented at The International Association of Agricultural Economists, Beijing, China. 1–15.
Aryal, J., Farnworth, C. R., Khurana, R., Ray, S., and Sapkota, T. B. (2014). “Gender dimensions of climate change adaptation through climate smart agricultural practices in India,” in Innovation in Indian Agriculture: Ways Forward. New Delhi and Washington D.C.: Institute of Economic Growth (IEG) and International Food Policy Research Institute (IFPRI). 1–32.
Baron, R. M., and Kenny, D. A. (1986). The moderator-mediator variable distinction in social psychological research: conceptual, strategic, and statistical considerations. J. Pers. Soc. Psychol. 51, 1173–1182. doi: 10.1037/0022-3514.51.6.1173
Bastakoti, R. C., Bharati, L., Bhattarai, U., and Wahid, S. M. (2017). Agriculture under changing climate conditions and adaptation options in the Koshi Basin. Climate Dev. 9, 634–648. doi: 10.1080/17565529.2016.1223594
Bebbington, A. (1999). Capitals and capabilities: a framework for analyzing peasant viability, rural livelihoods and poverty. World Dev. 27, 2021–2044. doi: 10.1016/S0305-750X(99)00104-7
Below, T. B., Mutabazi, K. D., Kirschke, D., Franke, C., Sieber, S., Siebert, R., et al. (2012). Can farmers' adaptation to climate change be explained by socio-economic household-level variables? Global Environ. Change 22, 223–235. doi: 10.1016/j.gloenvcha.2011.11.012
Bhatta, G. D., and Aggarwal, P. K. (2015). Coping with weather adversity and adaptation to climatic variability: a cross-country study of smallholder farmers in South Asia. Climate Dev. 8, 145–157. doi: 10.1080/17565529.2015.1016883
Bhatta, G. D., Ojha, H. R., Aggarwal, P. K., Sulaiman, R. V, Sultana, P., Thapa, D., et al. (2017). Agricultural innovation and adaptation to climate change: empirical evidence from diverse agro-ecologies in South Asia. Environ. Dev. Sustain. 19, 497–525. doi: 10.1007/s10668-015-9743-x
Bhatta, G. D., Singh, R. K. P., and Kristjanson, P. (2013). Summary of Baseline Household Survey Results: Vaishali Site, Bihar State (Northeast India). Copenhagen, Denmark: CGIAR Research Program on Climate Change, Agriculture and Food Security (CCAFS), 1–34.
Birkenholtz, T. (2012). Network political ecology: method and theory in climate change vulnerability and adaptation research. Prog. Hum. Geogr. 36, 295–315. doi: 10.1177/0309132511421532
Blaikie, P., Cannon, T., Davis, I., and Wisner, B. (Eds.). (1994). At Risk: Natural Hazards, People's Vulnerability and Disasters. London: Routledge.
Bosher, L., Penning-Rowsell, E., and Tapsell, S. (2007). Resource accessibility and vulnerability in Andhra Pradesh: caste and non-caste influences. Dev. Change 38, 615–640. doi: 10.1111/j.1467-7660.2007.00426.x
CCAFS (2015). Climate Change and Food Security (CCAFS) – Baseline household level questionnaire. CGIAR Research Program on Climate Change, Agriculture and Food Security (Harvard Dataverse).
Chambers, R., and Conway, G. R. (1992). Sustainable rural livelihoods: practical concepts for the 21st century. Institute Dev. Stud. 1992, 1–29.
Chand, R., and Haque, T. (1998). Rice-wheat crop system in Indo-Gangetic region. Econom. Political Weekly 33, A108–A112.
Chaudhari, V. R., and Mishra, A. (2015). Multilevel policy responses to mainstream climate adaptation through watershed development in rainfed farming systems of India. Climate Dev. 8, 324–335. doi: 10.1080/17565529.2015.1064808
Chen, M., Wichmann, B., Luckert, M., Winowiecki, L., Förch, W., and Läderach, P. (2018). Diversification and intensification of agricultural adaptation from global to local scales. PLoS ONE 13, 1–27. doi: 10.1371/journal.pone.0196392
Cote, M., and Nightingale, A. J. (2012). Resilience thinking meets social theory: situating social change in socio-ecological systems (SES) research. Prog. Hum. Geogr. 36, 475–489. doi: 10.1177/0309132511425708
Cramer, L., Förch, W., Mutie, I., and Thornton, P. K. (2016). Connecting women, connecting men: how communities and organizations interact to strengthen adaptive capacity and food security in the face of climate change. Gend. Technol. Dev. 20, 169–199. doi: 10.1177/0971852416639771
Dasgupta, P., Morton, J. F., Dodman, D., Karapinar, B., Meza, F., Rivera-Ferre, M. G., et al. (2014). Rural Areas. In B. C. Field, V. R. Barros, D. J. Dokken, K. J. Mach, M. D. Mastrandrea, T. E. Bilir, et al. (Eds.), In: Climate Change 2014: Impacts, Adaptation, and Vulnerability. Part A: Global and Sectoral Aspects. Contribution of Working Group II to the Fifth Assessment Report of the Intergovernmental Panel on Climate Change. (pp. 613–657). Cambridge, U.K. and New York, NY, USA: Cambridge University Press.
Datta, A., and Mishra, S. K. (2012). Glimpses of women's lives in rural Bihar: Impact of male migration. Indian J. Labour Econom. 54, 457–477. Available online at: http://hdl.handle.net/1765/34865
Davidson, D. (2016). Gaps in agricultural climate adaptation research. Nat. Clim. Chang. 6, 433–435. doi: 10.1038/nclimate3007
Deininger, K. W. (2003). Land Policies for Growth and Poverty Reduction. Washington, DC: The World Bank and Oxford University Press.
Deressa, T. T., Hassan, R. M., and Ringler, C. (2010). Perception of and adaptation to climate change by farmers in the Nile basin of Ethiopia. J. Agric. Sci. 149, 23–31. doi: 10.1017/S0021859610000687
Deressa, T. T., Hassan, R. M., Ringler, C., Alemu, T., and Yesuf, M. (2009). Determinants of farmers' choice of adaptation methods to climate change in the Nile Basin of Ethiopia. Global Environ. Change 19, 248–255. doi: 10.1016/j.gloenvcha.2009.01.002
Doss, C. R. (2001). Designing agricultural technology for African women farmers: lessons from 25 years of experience. World Dev. 29, 2075–2092. doi: 10.1016/S0305-750X(01)00088-2
Duncan, J. M., Tompkins, E. L., Dash, J., and Tripathy, B. (2017). Resilience to hazards: rice farmers in the Mahanadi Delta, India. Ecol. Soc. 22:3. doi: 10.5751/ES-09559-220403
Engle, N. L. (2011). Adaptive capacity and its assessment. Global Environ. Change 21, 647–656. doi: 10.1016/j.gloenvcha.2011.01.019
Government of Haryana (2011). Haryana State Action Plan on Climate Change. Government of Haryana. Available online at: http://www.moef.nic.in/sites/default/files/sapcc/Haryana.pdf
Government of India (2011a). District Census Handbook: Karnal (Haryana) (No. Series 07, Part XII-A). Haryana: Census of India (Directorate of Census Operations, Haryana), 1–338.
Government of India (2011b). District Census Handbook: Vaishali (Bihar). (No. Series-11 Part XII-A). Bihar: Census of India (Directorate of Census Operations, Bihar), 1–1142.
Grace, J. B. (2006). Structural Equation Modeling and Natural Systems. New York, NY: Cambridge University Press.
Grace, J. B., Anderson, T. M., Olff, H., and Scheiner, S. M. (2010). On the specification of structural equation models for ecological systems. Ecol. Monogr. 80, 67–87. doi: 10.1890/09-0464.1
Hayes, A. F. (2009). Beyond Baron and Kenny: statistical mediation analysis in the new millennium. Commun. Monogr. 76, 408–420. doi: 10.1080/03637750903310360
Iacobucci, D. (2008). Mediation Analysis. Series: Quantitative Applications in the Social Sciences. Thousand Oaks, CA: SAGE Publications Inc.
Ifejika Speranza, C., Wiesmann, U., and Rist, S. (2014). An indicator framework for assessing livelihood resilience in the context of social–ecological dynamics. Global Environ. Change 28, 109–119. doi: 10.1016/j.gloenvcha.2014.06.005
Immerzeel, W. W., van Beek, L. P. H., and Bierkens, M. F. P. (2010). Climate change will affect the Asian water towers. Science 328, 1382–1385. doi: 10.1126/science.1183188
Jones, L., and Boyd, E. (2011). Exploring social barriers to adaptation: insights from Western Nepal. Global Environ. Change 21, 1262–1274. doi: 10.1016/j.gloenvcha.2011.06.002
Jost, C., Kyazze, F., Naab, J., Neelormi, S., Kinyangi, J., Zougmore, R., et al. (2015). Understanding gender dimensions of agriculture and climate change in smallholder farming communities. Climate Dev. 8, 133–144. doi: 10.1080/17565529.2015.1050978
Kabir, M. J., Alauddin, M., and Crimp, S. (2017). Farm-level adaptation to climate change in Western Bangladesh: an analysis of adaptation dynamics, profitability and risks. Land Use Policy 64, 212–224. doi: 10.1016/j.landusepol.2017.02.026
Kelkar, U., Narula, K. K., Sharma, V. P., and Chandna, U. (2008). Vulnerability and adaptation to climate variability and water stress in Uttarakhand State, India. Global Environ. Change 18, 564–574. doi: 10.1016/j.gloenvcha.2008.09.003
Kenny, D. (2014). Structural Equation Modeling. Available online at: http://davidakenny.net/cm/causalm.htm. (Accessed December 3, 2018).
Khatri-Chhetri, A., Aryal, J., Sapkota, T. B., and Khurana, R. (2016). Economic benefits of climate-smart agricultural practices to smallholder farmers in the Indo-Gangetic Plains of India. Curr. Sci. 110, 1251–1256. doi: 10.18520/cs/v110/i7/1251-1256
Kibue, G. W., Liu, X., Zheng, J., Zhang, X., Pan, G., Li, L., et al. (2016). Farmers' perceptions of climate variability and factors influencing adaptation: evidence from Anhui and Jiangsu, China. Environ. Manage. 57, 976–986. doi: 10.1007/s00267-016-0661-y
Kristjanson, P., Bryan, E., Bernier, Q., Twyman, J., Meinzen-Dick, R., Kieran, C., et al. (2017). Addressing gender in agricultural research for development in the face of a changing climate: where are we and where should we be going? Int. J. Agric. Sustain. 15, 482–500. doi: 10.1080/14735903.2017.1336411
Kristjanson, P. M., Förch, W., Barahona, C., Garlick, C., and Thornton, P. K. (2011). CCAFS Baseline Household Level Survey: Manual for Survey Sites, 1–35. Available online at: https://cgspace.cgiar.org/handle/10568/41976
Lai, K. (2018). Estimating standardized SEM parameters given nonnormal data and incorrect model: methods and comparison. Struct. Equat. Model. 25, 600–620. doi: 10.1080/10705511.2017.1392248
Le Dang, H., Li, E., Nuberg, I., and Bruwer, J. (2014). Understanding farmers' adaptation intention to climate change: a structural equation modelling study in the Mekong Delta, Vietnam. Environ. Sci. Policy 41, 11–22. doi: 10.1016/j.envsci.2014.04.002
Leach, M., Mearns, R., and Scoones, I. (1999). Environmental entitlements: dynamics and institutions in community-based natural resource management. World Dev. 27, 225–247. doi: 10.1016/S0305-750X(98)00141-7
Lipper, L., Thornton, P., Campbell, B. M., Baedeker, T., Braimoh, A., Bwalya, M., et al. (2014). Climate-smart agriculture for food security. Nat. Clim. Chang. 4, 1068–1072. doi: 10.1038/nclimate2437
Lobo, C., Chattopadhyay, N., and Rao, K. V. (2017). Making smallholder farming climate-smart: integrated agrometeorlogical services. Econom. Political Weekly LLI 52, 53–58.
Lutz, W., Muttarak, R., and Striessnig, E. (2014). Universal education is key to enhanced climate adaptation. Science 346, 1061–1062. doi: 10.1126/science.1257975
MacKinnon, D. P., Fairchild, A. J., and Fritz, M. S. (2007). Mediation analysis. Annu. Rev. Psychol. 58, 593–614. doi: 10.1146/annurev.psych.58.110405.085542
Maddison, D. (2007). The Perception of and Adaptation to Climate Change in Africa (No. Policy Research Working Paper #4308). The World Bank (Development Research Group, Sustainable Rural and Urban Development Team), 1–51.
Mehar, M., Mittal, S., and Prasad, N. (2016). Farmers coping strategies for climate shock: is it differentiated by gender? J. Rural Stud. 44, 123–131. doi: 10.1016/j.jrurstud.2016.01.001
Meshram, S. G., Singh, V. P., and Meshram, C. (2016). Long-term trend and variability of precipitation in Chhattisgarh State, India. Theor. Appl. Climatol. 129, 729–744. doi: 10.1007/s00704-016-1804-z
Niles, M. T., and Brown, M. E. (2017). A multi-country assessment of factors related to smallholder food security in varying rainfall conditions. Nat. Sci. Rep. 7, 1–11. doi: 10.1038/s41598-017-16282-9
Niles, M. T., Lubell, M., Van, R., and Haden (2013). Perceptions and responses to climate policy risks among California farmers. Global Environ. Change 23, 1752–1760. doi: 10.1016/j.gloenvcha.2013.08.005
Obrist, B., Pfeiffer, C., and Henley, R. (2010). Multi-layered social resilience: a new approach in mitigation research. Progr. Dev. Stud. 10, 283–293. doi: 10.1177/146499340901000402
Ojha, H. R., Sulaiman, R. V., Sultana, P., Dahal, K., Thapa, D., Mittal, N., et al. (2014). Is South Asian agriculture adapting to climate change? Evidence from the Indo-Gangetic Plains. Agroecol. Sustain. Food Syst. 38, 505–531. doi: 10.1080/21683565.2013.841607
Patnaik, U., Das, P. K., and Bahinipati, C. S. (2017). Development interventions, adaptation decisions and farmers' well-being: evidence from drought- prone households in rural India. Climate Dev. 2017, 1–17. doi: 10.1080/17565529.2017.1410084
Pearse, R. (2017). Gender and climate change. Wiley Interdiscip. Rev. Climate Change 8, e451–e416. doi: 10.1002/wcc.451
Perez, C., Jones, E. M., Kristjanson, P., Cramer, L., Thornton, P. K., Förch, W., et al. (2015). How resilient are farming households and communities to a changing climate in Africa? A gender-based perspective. Global Environ. Change 34, 95–107. doi: 10.1016/j.gloenvcha.2015.06.003
Rao, C. S., Gopinath, K. A., Prasad, J. V., Prasannakumar, N. S., and Singh, A. K. (2016). Climate resilient villages for sustainable food security in tropical India: concept, process, technologies, institutions, and impacts. Adv. Agronomy 140, 101–214. doi: 10.1016/bs.agron.2016.06.003
Rathakrishnan, L., and Kumar, K. R. (2014). Land institutions divided India economically: unbundling land institutions. J. Land Rural Stud. 2, 113–125. doi: 10.1177/2321024913515100
Reddy, D. N., and Mishra, S. (eds.). (2009). Agrarian Crisis in India. New Delhi: Oxford University Press.
Ribot, J. C. (1995). The causal structure of vulnerability: its application to climate impact analysis. GeoJournal 35, 119–122. doi: 10.1007/BF00814058
Robert, M. (2016). Modeling Adaptive Decision-Making of Farmer: An Integrated Economic and Management Model, With an Application to Smallholders in India. Ph.D. Dissertation. Université Paul Sabatier - Toulouse III.
Rodell, M., Velicogna, I., and Famiglietti, J. S. (2009). Satellite-based estimates of groundwater depletion in India. Nature 460, 999–1002. doi: 10.1038/nature08238
Sapkota, T. B., Jat, M. L., Aryal, J. P., Jat, R. K., and Khatri-Chhetri, A. (2015). Climate change adaptation, greenhouse gas mitigation and economic profitability of conservation agriculture: some examples from cereal systems of Indo-Gangetic Plains. J. Integr. Agric. 14, 1524–1533. doi: 10.1016/S2095-3119(15)61093-0
Scoones, I. (1998). Sustainable Rural Livelihoods: A Framework for Analysis (No. IDS Working Paper No. 72). Brighton: Institute of Development Studies. 1–22.
Sekar, I., and Pal, S. (2012). Rice and wheat crop productivity in the Indo-Gangetic Plains of India: changing pattern of growth and future strategies. Ind. J. Agric. Econom. 67, 238–252.
Sen, A. (1981). Poverty and Famines: An Essay on Entitlement and Deprivation. Oxford: Oxford University Press.
Shah, S. H. (2015). Water Variability, Livelihoods, and Adaptation: A Case Study From the Angat River Basin (Philippines). The University of British Columbia (Master's Thesis).
Shukla, R., Chakraborty, A., and Joshi, P. K. (2017). Vulnerability of agro-ecological zones in India under the earth system climate model scenarios. Mitigat. Adapt. Strategies Global Change 22, 399–425. doi: 10.1007/s11027-015-9677-5
Sikka, A. K., Rao, B. B., and Rao, V. U. M. (2016). Agricultural disaster management and contingency planning to meet the challenges of extreme weather events. Mausam 67, 155–168.
Singh, C., Dorward, P., and Osbahr, H. (2016). Developing a holistic approach to the analysis of farmer decision-making: implications for adaptation policy and practice in developing countries. Land Use Policy 59, 329–343. doi: 10.1016/j.landusepol.2016.06.041
Singh, R. K. P. (2013). Summary of Baseline Household Survey Results: Karnal, Haryana State, India. Copenhagen, Denmark: CGIAR Research Program on Climate Change, Agriculture and Food Security (CCAFS). 1–35.
Smit, B., and Wandel, J. (2006). Adaptation, adaptive capacity and vulnerability. Global Environ. Change 16, 282–292. doi: 10.1016/j.gloenvcha.2006.03.008
Sugden, F., Maskey, N., Clement, F., Ramesh, V., Philip, A., and Rai, A. (2014). Agrarian stress and climate change in the Eastern Gangetic Plains: Gendered vulnerability in a stratified social formation. Global Environ. Change 29, 258–269. doi: 10.1016/j.gloenvcha.2014.10.008
Sujakhu, N. M., Ranjitkar, S., Niraula, R. R., Salim, M. A., Nizami, A., Schmidt-Vogt, D., et al. (2018). Determinants of livelihood vulnerability in farming communities in two sites in the Asian Highlands. Water Int. 44, 1–18. doi: 10.1080/02508060.2017.1416445
Tesfaye, K., Aggarwal, P., Mequanint, F., Shirsath, P., Stirling, C., Khatri-Chhetri, A., et al. (2017). Climate variability and change in Bihar, India: challenges and opportunities for sustainable crop production. Sustainability 9:1998. doi: 10.3390/su9111998
Tian, Q., Jiang, L., Lemos, M., and Qi, S. (2018). Interactions of social, natural, and technological subsystems and synergy between development and adaptation to floods around Poyang Lake. Ann. GIS 24, 209–220. doi: 10.1080/19475683.2018.1471520
Toma, L., Barnes, A. P., Sutherland, L.-A., Thomson, S., Burnett, F., and Mathews, K. (2018). Impact of information transfer on farmers' uptake of innovative crop technologies: a structural equation model applied to survey data. J. Technol. Transf. 43, 864–881. doi: 10.1007/s10961-016-9520-5
Totin, E., Segnon, A., Schut, M., Affognon, H., Zougmore, R., Rosenstock, T., et al. (2018). Institutional perspectives of climate-smart agriculture: a systematic literature review. Sustainability 10, 1990–1920. doi: 10.3390/su10061990
Tripathi, A., and Mishra, A. K. (2017). Knowledge and passive adaptation to climate change: an example from Indian farmers. Climate Risk Manag. 16, 195–207. doi: 10.1016/j.crm.2016.11.002
Vermeulen, S. J., Campbell, B. M., and Ingram, J. S. I. (2012). Climate change and food systems. Annu. Rev. Environ. Resour. 37, 195–222. doi: 10.1146/annurev-environ-020411-130608
Watts, M. J., and Bohle, H. G. (1993). The space of vulnerability: the causal structure of hunger and famine. Prog. Hum. Geogr. 17, 43–67. doi: 10.1177/030913259301700103
Wood, S. A., Jina, A. S., Jain, M., Kristjanson, P., and DeFries, R. S. (2014). Smallholder farmer cropping decisions related to climate variability across multiple regions. Global Environ. Change 25, 163–172. doi: 10.1016/j.gloenvcha.2013.12.011
Zacharias, M., Kumar, S. N., Singh, S. D., Rani, D. N. S., and Aggarwal, P. K. (2014). Assessment of impacts of climate change on rice and wheat in the Indo-Gangetic plains. J. Agrometeorol. 16, 9–17.
Keywords: adaptation, agriculture, household capital, climate change, socio-environmental change, structural equation modeling, India
Citation: Shah SH, Wagner CH, Sanga U, Park H, Demange LHML, Gueiros C and Niles MT (2019) Does Household Capital Mediate the Uptake of Agricultural Land, Crop, and Livestock Adaptations? Evidence From the Indo-Gangetic Plains (India). Front. Sustain. Food Syst. 3:1. doi: 10.3389/fsufs.2019.00001
Received: 29 August 2018; Accepted: 03 January 2019;
Published: 30 January 2019.
Edited by:
Ademola Braimoh, World Bank, United StatesReviewed by:
Nicholas R. Magliocca, University of Alabama, United StatesIdowu O. Oladele, Sasakawa Africa Fund for Extension Education, South Africa
Copyright © 2019 Shah, Wagner, Sanga, Park, Demange, Gueiros and Niles. This is an open-access article distributed under the terms of the Creative Commons Attribution License (CC BY). The use, distribution or reproduction in other forums is permitted, provided the original author(s) and the copyright owner(s) are credited and that the original publication in this journal is cited, in accordance with accepted academic practice. No use, distribution or reproduction is permitted which does not comply with these terms.
*Correspondence: Sameer H. Shah, c2FtZWVyLnNoYWhAYWx1bW5pLnViYy5jYQ==