Explore article hub
- 1Department of Microbiology and Immunology, University of British Columbia, Vancouver, BC, Canada
- 2Keenan Research Centre for Biomedical Science, Critical Care Medicine, St. Michael’s Hospital, University of Toronto, Toronto, ON, Canada
- 3Department of Molecular Biology and Biochemistry, Simon Fraser University, Burnaby, BC, Canada
Abstract
Sepsis is an abnormal, life-threatening response to infection that leads to (multi-)organ dysfunction and failure. It causes ~20% of deaths worldwide each year, and most deaths related to severe COVID-19 share various molecular features with sepsis. Current treatment approaches (antimicrobials and supportive care) do not address the complexity of sepsis or its mechanistic heterogeneity between and within patients over time. Systems immunology methods, including multiomics (notably RNA sequencing transcriptomics), machine learning, and network biology analysis, have the potential to transform the management paradigm toward precision approaches. Immune dysfunctions evident very early in sepsis drive the development of novel diagnostic gene expression signatures (e.g., cellular reprogramming) that could inform early therapy. Sepsis patients can now be categorized into “endotypes” based on unique immune dysfunction mechanisms corresponding to varying severity and mortality rates, raising the prospect of endotype-specific diagnostics and patient-specific immune-directed therapy. Longitudinal within-patient analyses can also reveal mechanisms (including epigenetics) that drive differential sepsis trajectories over time, enabling the prospect of disease stage-specific therapy during and after hospitalization, including for post-sepsis and long COVID syndromes. Achieving this transformation will require addressing barriers to systems immunology research, including its cost and resource-intensiveness, the relatively low volume of available data, and lack of suitable animal models; it will also require a change in the mindset of healthcare providers toward precision approaches. This should be prioritized in multistakeholder collaborations involving research communities, healthcare providers/systems, patients, and governments to reduce the current high disease burden from sepsis and to mitigate against future pandemics.
Key points
- Sepsis, a complex and heterogeneous immune dysregulation syndrome triggered by infection, is a major under-recognized global cause of mortality and of pandemic-related deaths.
- The multifaceted nature of sepsis requires systems immunology approaches such as comprehensive omics investigations and high-end bioinformatics analyses, including machine learning.
- Systems immunology is beginning to transform sepsis care toward precision medicine approaches, including diagnostics to enable early detection and immune-directed therapies tailored for specific sepsis subtypes (endotypes) and stages in disease trajectories over time.
- Advances in epigenetics could inform future therapies against long-term post-sepsis syndrome and long COVID.
- Research and development toward precision sepsis care should be at the heart of pandemic mitigation planning to provide a pathogen-agnostic means to reduce mortality.
Introduction: sepsis as a major medical emergency
Of all major causes of death and disability, arguably the least acknowledged is sepsis. Sepsis is formally defined as an abnormal, life-threatening response to infection that leads to (multi)organ dysfunction and failure (1). The global burden of sepsis is far higher than previously thought: an estimated 48.9 million cases caused 11 million deaths in 2017 alone (2), representing 19.7% of all deaths worldwide. Sepsis incidence and mortality rates show large interregional differences, with the greatest burden in lower-income areas (2). More recently, COVID-19 infected >690 million and killed 6.9–18 million people (as of October 2023) (3, 4) and, while caused by a novel virus (SARS-CoV-2), in its most severe form it has striking parallels to severe sepsis (5–7). Indeed, systems immunology studies have revealed various molecular features shared by patients with COVID-19 or non-COVID-19 severe sepsis; after a week in the intensive care unit (ICU), these groups are indistinguishable in terms of gene expression (8).
Critically, sepsis in its most severe forms (i.e., leading to organ failure) can be a major killer, with overall in-hospital case fatality rates averaging >22% (2). Septic shock, defined by hypotension and hyperlactatemia that require vasopressor use, has even higher in-hospital mortality rates of 30–50% (9). Even surviving a bout of sepsis does not guarantee future health: so-called “post-sepsis syndrome” (10)—and its counterpart, long COVID (11)—is associated with long-term major health problems, with up to 40% of survivors suffering physical, cognitive, and/or physiological effects, while >70% of patients who survived a first episode of sepsis were readmitted into acute care in the following year (10). Sepsis primarily affects already clinically vulnerable patients, including those of older age or who are immunosuppressed (e.g., due to AIDS, cancer, or immunosuppressant medications) (12). Patients aged over 65 years account for the majority of sepsis cases (13) and thus the incidence is expected to increase as populations age.
The acute and longer-term complications confer enormous direct healthcare costs and indirect socioeconomic costs that are potentially greater than those of coronary heart disease or stroke (14). The enormous contribution of sepsis is often under-recognized in reports of disease burden. For example, the World Health Organization (WHO) lists the major global causes of death as ischemic heart disease (16%), stroke (11%), and chronic obstructive pulmonary disease (COPD; 6%) (15, 16). Conversely, if one collects diseases into syndromes, cardiovascular diseases (~30%) and cancers (16.4%) are the major killers (17). Thus, despite having mortality and morbidity rates rivalling these major diseases, sepsis does not appear in the top 10 global causes of death. Why is this? First and foremost, sepsis is not usually a line item on any country’s death statistics, since it tends to be associated with diverse infections and often involves co-morbidities (17) (underlying ailments that predispose patients to a higher probability of severe sepsis, such as diabetes, hypertension, heart disease, malignancy, end-stage renal disease, and COPD). Thus, sepsis is usually buried beneath other statistics. A second reason is the massive heterogeneity of sepsis (18–20) in terms of its underlying causes, clinical presentation and symptomology, and rate of progression, as discussed further below. Third, sepsis is difficult to define, especially in the very early stages of a patient’s medical journey (21). Indeed, there have been three diverse international consensus statements on the characteristics of sepsis and these shifting sands have not helped to crystalize a workable definition of early sepsis (1, 20). This lack of a definition can not only result in a delay in therapy, which is associated with worse morbidity and mortality rates (22, 23), but also contributes to deemphasizing sepsis as the underlying cause of downstream issues. Moreover, significant barriers to understanding the larger scope/burden of sepsis remain, including coding of infection and organ dysfunction to ensure consistency, measurement of quality metrics, and benchmark strategies to evaluate outcomes.
Currently, sepsis management focuses on antimicrobials and supportive care, measures that fail to address the complex immune system dysfunctions that underlie sepsis and its heterogeneity between and within patients over time. This article describes how systems immunology methods are now starting to address these challenges—substantially elucidating the many mechanisms involved and transforming the current treatment paradigm toward a future of precision care.
Specifically, we explore how these techniques are elucidating.
(i). programming, i.e., common aspects of immune dysfunction evident very early in sepsis (at first clinical presentation) are driving the development of novel diagnostic signatures,
(ii). inter-patient heterogeneity, i.e., how the sepsis syndrome can be categorized into five distinct and diverse mechanistic groupings (endotypes) to allow novel, precision approaches to diagnosis and immune-directed therapy, and
(iii). within-patient staging, i.e., how a greater understanding of the mechanisms driving the progression of sepsis and the associated long-term disease trajectories should enable disease stage-specific therapies as well as treatments for post-sepsis complications, including long COVID.
Finally, we argue that collaborative multidisciplinary and multi-stakeholder research and action to transform the diagnosis and management of sepsis should be a priority to mitigate against future pandemics, as well as the present and rising burden from sepsis.
Immunity as a complex system of responses
Fundamentally, immunity enables an organism to resist a particular infectious disease or pathogen. It involves a complex and integrated system of interwoven responses guided by specific cells and proteins. These responses are both hard-wired into the organism and dynamic in the sense that they can be influenced by induction and repression of specific genes, proteins, chemicals, metabolites, and regulatory elements, and adjusted through adaptive mechanisms that include gene rearrangements and epigenetic modulation. Estimates of the numbers of individual proteins/genes involved in the immune response vary (24), but there are likely as many as 5,000 out of the ~11,000 expressed genes in humans, attesting to and reflecting the complexity of immunity. Systems biology tools such as NetworkAnalyst (25, 26) display how proteins interact in complex ways with many so-called “hub proteins” that in turn interact with tens to hundreds of other elements. Critically, the immune system overlaps extensively with other bodily systems such as the endocrine/hormonal, nervous, circulatory, renal, digestive, reproductive, muscular, and respiratory systems; thus, the immune system is integrated into the way the body functions as a whole. Although immunology is often viewed as a series of critical events involving specific elements, it is ultimately a system with components that cannot be considered in isolation (27), i.e., requiring a “systems biology” approach rather than a reductionist approach.
Generally, the immune system is considered to involve two arms: innate and adaptive immunity (28, 29). Innate immunity is considered the body’s first line of defense, but it comprises elements that function continuously during immune responses to foreign agents/pathogens. Adaptive (acquired) immunity involves antigen-specific responses. However, these distinctions are somewhat blurred, and overlaps exist between these systems. For example, innate immunity can involve some level of specificity (e.g., trained immunity), while the effectors of adaptive immunity are often major components involved in innate immunity. These overlaps highlight the importance of applying systems approaches to provide holistic insights.
Critically, sepsis is an immune dysfunction syndrome (30), so understanding sepsis will necessarily involve understanding why and how the immune system becomes dysfunctional. Like the immune system, sepsis has always been considered complex, perhaps underpinning the poor definition of this syndrome. Nevertheless, as described below, it is increasingly clear that the complexity of sepsis in terms of causes, symptoms, disease progression, and outcomes can be understood by using artificial intelligence (AI) strategies to identify mechanistic endotypes (disease subtypes with different pathophysiological mechanisms) (31, 32, 34, 35). These AI strategies are enabling new insights, novel diagnostics, and the prediction of new and personalized therapeutics tailored to a patient’s specific type of sepsis.
Using systems immunology to understand complex biology
Systems immunology is a branch of systems biology that involves the computational/mathematical analysis and modeling of immunology as a complex biological system (36). Many strategies can be used to maximize the collection of information on immune system components, allowing elucidation of how these parts interrelate and ultimately determine the events triggered when the immune system is disturbed. This is of fundamental importance for understanding a complex, heterogeneous syndrome involving immune dysfunction, such as sepsis. However, only recently have the ever-evolving methods of systems biology begun to be properly applied to deciphering sepsis.
Omics-based analysis in sepsis
Systems immunology analyzes genome-wide information by utilizing advanced computational and statistical analyses of omics data, including sophisticated AI and machine learning (ML) methods, network biology, biomarker discovery, and imputing function to create new hypotheses and ultimately learn more about complex systems such as sepsis (Figure 1). It requires aggregation of at least some (genome-wide) omics data, such as an understanding of genetic variation between subjects (i.e., mutations associated with function defined through genome-wide association studies; GWAS), altered transcriptome (mRNA) and microRNA expression (transcriptome and microRNAome), varied protein and/or lipid expression or modifications (proteome and lipidome), modulated metabolites (metabolome), and environmentally driven reversible changes to how the body reads its DNA sequences (epigenome).
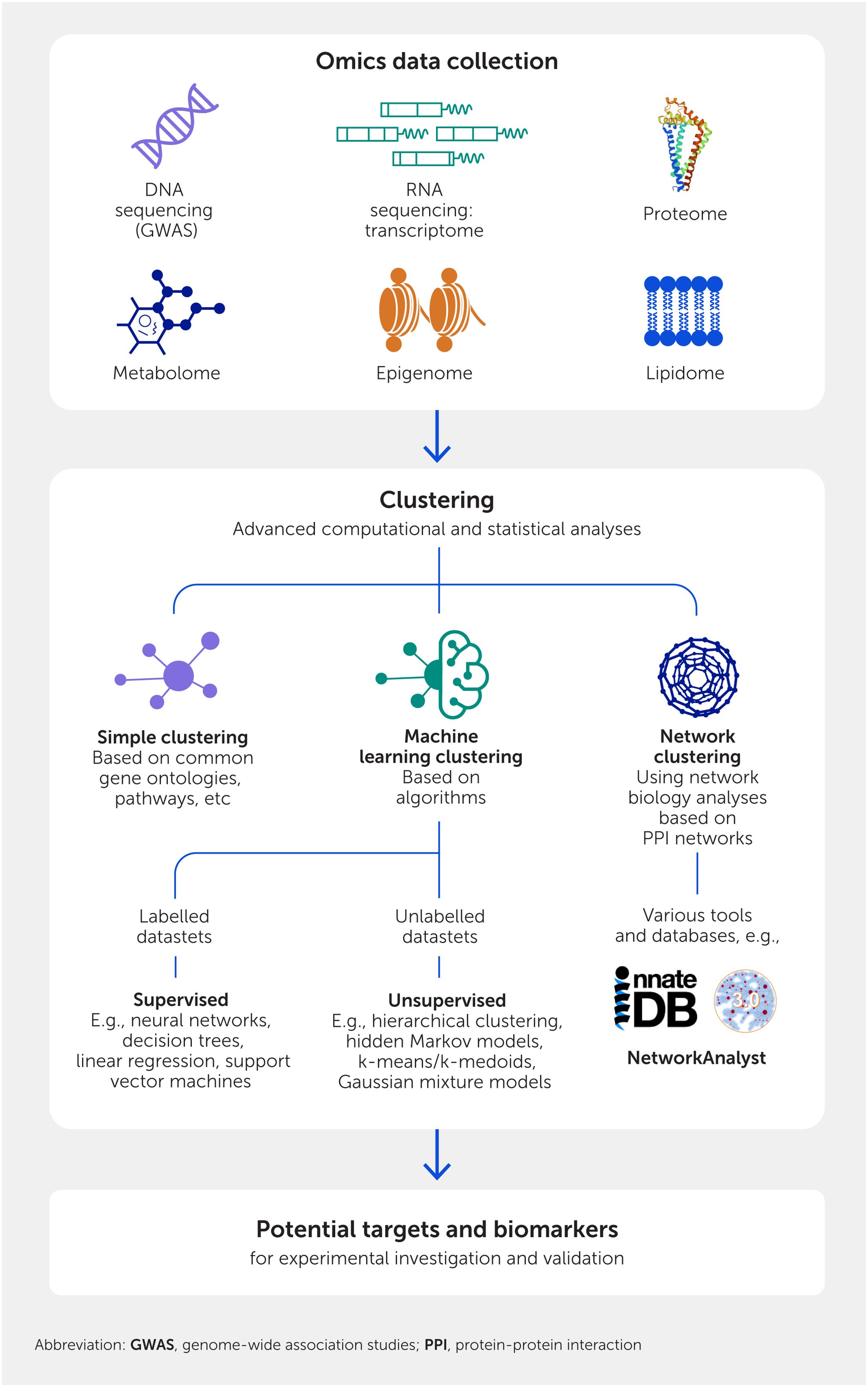
Figure 1. Methods of systems biology and immunology. The first layer involves high-throughput techniques that collect information in a genome-wide fashion. These data are then analyzed by various clustering methods that provide hypotheses for wet lab testing.
Each of these elements can be determined on a genomic scale, although the depth of accurate coverage varies. Generally, the transcriptome, acquired by high-throughput sequencing of complementary DNA obtained by reverse transcription of expressed messenger RNAs (a process termed RNA-Seq), provides the most comprehensive and accurate “real-time” data.
Clustering
The key to systems biology and immunology is to utilize these datasets to identify differences that occur according to a treatment or disease condition (e.g., differential gene expression). Collectively, these differences determine emergent properties (i.e., those not immediately obvious individually but revealed through the analysis of interacting components within a system) and inform novel biology related to a particular treatment or disease condition. Systems analysis moves beyond the traditional analysis of a few differentially abundant elements (e.g., known genes and their expressed products) to collate elements with common features—a process termed clustering. Thus, individual components have varying, but generally limited, importance to a system, but how they integrate and operate together is the critical, informative aspect for finding new biology.
There are three types of clustering (Figure 1). Simple clustering takes all elements analyzed and clusters them into groups with known common features, maximizing similarities such as hierarchical functional attributes (e.g., gene ontology terms; https://geneontology.org/), pathways they belong to (e.g., https://reactome.org/), or their common regulatory elements (e.g., TRANSFAC; http://gene-regulation.com/pub/databases.html). This can be very useful, especially when combined with the other methods outlined below. However, the redundancy of genes and their products, which can act in many different functions and pathways (particularly within the immune system), can limit the value of this approach and the insights delivered. New concepts have been largely based on the other two clustering approaches.
The second approach is ML (37, 38), a branch of AI. ML involves computational analysis of large volumes of data, enabling algorithms to learn and find patterns, classify data, and/or make predictions. The two most commonly used ML strategies in analyzing omics data are supervised and unsupervised reinforcement learning. Supervised ML involves feeding the computer with labelled datasets (e.g., with different properties such as sepsis versus healthy) to train algorithms to classify data and/or predict outcomes accurately; common methods are neural networks, decision trees, linear regression, and support vector machines. Unsupervised learning uses algorithms to analyze and cluster unlabeled datasets, enabling the discovery of hidden patterns or data groupings without predetermined labels, and subsequently determines if one of the groups is enriched for a particular attribute (e.g., which cluster has higher sepsis mortality rates). Common methods are hierarchical clustering, hidden Markov models (also useful for classification), k-means/k-medoids, and Gaussian mixture models. The fundamental idea is to use these powerful algorithms to classify and find patterns in data associated with a clinical or biological variable of interest, and thus generate new, non-obvious hypotheses for experimentation and validation.
A third widely used method is network biology analysis based on protein:protein interaction (PPI) networks (25). PPIs may be physical (e.g., proteins bind to one another), biochemical (e.g., consecutive enzymes in a pathway), or regulatory (e.g., a transcription factor regulating the expression of another protein). They are curated into large databases as part of the International Molecular Exchange (IMEx) Consortium, with the major immune database being InnateDB (39). PPIs are functional interactions, even in the absence of specific knowledge of a particular pathway involvement. Thus, drawing networks based on PPIs, which is performed using two major programs, NetworkAnalyst (25) (https://www.networkanalyst.ca/) and Cytoscape (40) (https://cytoscape.org/), can help to define how individual elements contribute to a system. This network biology perspective can be applied to individual omics datasets or even to multiple types of omics data to fully capture the interplay of elements within the system. The multiomics approach involves converting each omics data point to a corresponding gene/protein product: GWAS to their corresponding gene/protein, transcripts to expressed proteins, metabolites to the proteins involved in their production or degradation, and epigenetic markers to the adjacent genes they affect. For example, to understand the developmental trajectory of newborns, the transcriptome, proteome, and metabolome identified in multiple omics datasets were integrated, revealing a programmed pattern of changes that occur during the first week of life, demonstrating that different omics methods report on individual aspects of the same biological processes and describe the underlying molecular events/pathways (26). In addition to PPIs, one can also create metabolic, epigenetic, genetic interaction, gene/transcriptional/regulatory, and cell signaling networks, or networks that encompass any other set of properties that can be assessed on a genome-wide scale. These different types of omics studies have not yet been combined for sepsis, but this is now possible using PPI network approaches, as well as multivariate integrative feature selection ML methods such as DIABLO (Data Integration Analysis for Biomarker discovery using Latent cOmponents) (26). Integrating transcriptomics, genomics, epigenomics, proteomics, metabolomics, lipidomics, and/or microbiomics will almost certainly improve our understanding of sepsis and has the potential to provide new targets for intervention and new biomarkers to characterize the nature of a patient’s sepsis, which could tangibly improve patient care and health outcomes. We suggest that multiomics methods should be increasingly deployed in sepsis research to further characterize this complex disease since, in our experience, they strongly reinforce models derived from single omics data. Exploring the methodologies, algorithms, and frameworks employed to integrate and interpret such vast amounts of data will undoubtedly contribute to the overall understanding of how these recent innovations can be translated into tangible benefits for patient care and improved health outcomes.
Limitations
Recently, major attempts have been made to understand critical illness with deep heterogeneity such as sepsis and to derive treatments from a mechanistic perspective using systems immunology methods (41–43). These attempts are transforming our thinking from clinical symptom-based approaches to diagnostics and treatments based on underlying mechanisms and evidence. As we discuss below, these methods are starting to yield host-based molecular diagnostics that can assess the risk that a patient will progress to sepsis and (multi)-organ failure or further deteriorate, differentiate between bacterial and viral infections as the triggering agent, define groupings (endotypes) of sepsis patients with different underlying immune processes, and clarify post-sepsis syndrome. However, given the massive complexity of critical illness and sepsis, they provide guideposts rather than definitive approaches, and the current review aims to clarify the types of insights provided by systems immunology and the potential for transformation of therapeutic approaches.
It is worthwhile to describe the limitations of such approaches. First and foremost, as mentioned above, these methods do not deliver definitive mechanisms or “truths” but rather are powerful hypothesis-generating mechanisms that require experimental validation. This is especially relevant in the context of sepsis, since model systems (animals and organoids) for sepsis substantially lack precision and do not fully capture the mechanisms involved in human sepsis. Second, most omics data are based on bulk analyses (e.g., blood, tissue, or organ transcriptomes and proteomes) and can be influenced by cell type composition, although cellular deconvolution algorithms such as CIBERSORTx (44) can tease out the influence of cell type proportions on omics data. Although this can be overcome to some extent, such as by using single-cell RNA-Seq transcriptomics (45), such methods sacrifice resolution for specificity, delivering only a fraction of differentially expressed transcripts (especially those with high abundance and larger changes in expression), which skews the dataset and can limit the accuracy or ability to discern patterns. Third, there are several factors influencing sepsis presentation and severity, including the individual infectious agents, sex, age, endotypes, disease progression stage and trajectory, pre-existing medical conditions, and treatment effects, as well as many other currently unknown factors (18–20) (Figure 2). Another limitation lies in how such data will be translated into clinical gains. We have described diagnostic approaches that are indeed playing out in real time. However, these do not provide yes/no answers but rather must be couched in terms of probability. For example, an early sepsis diagnostic that can accurately identify >90% of patients who will go on to sepsis, in combination with conventional measurements, will provide valuable input to attending physicians to make decisions about that patient’s future clinical care.
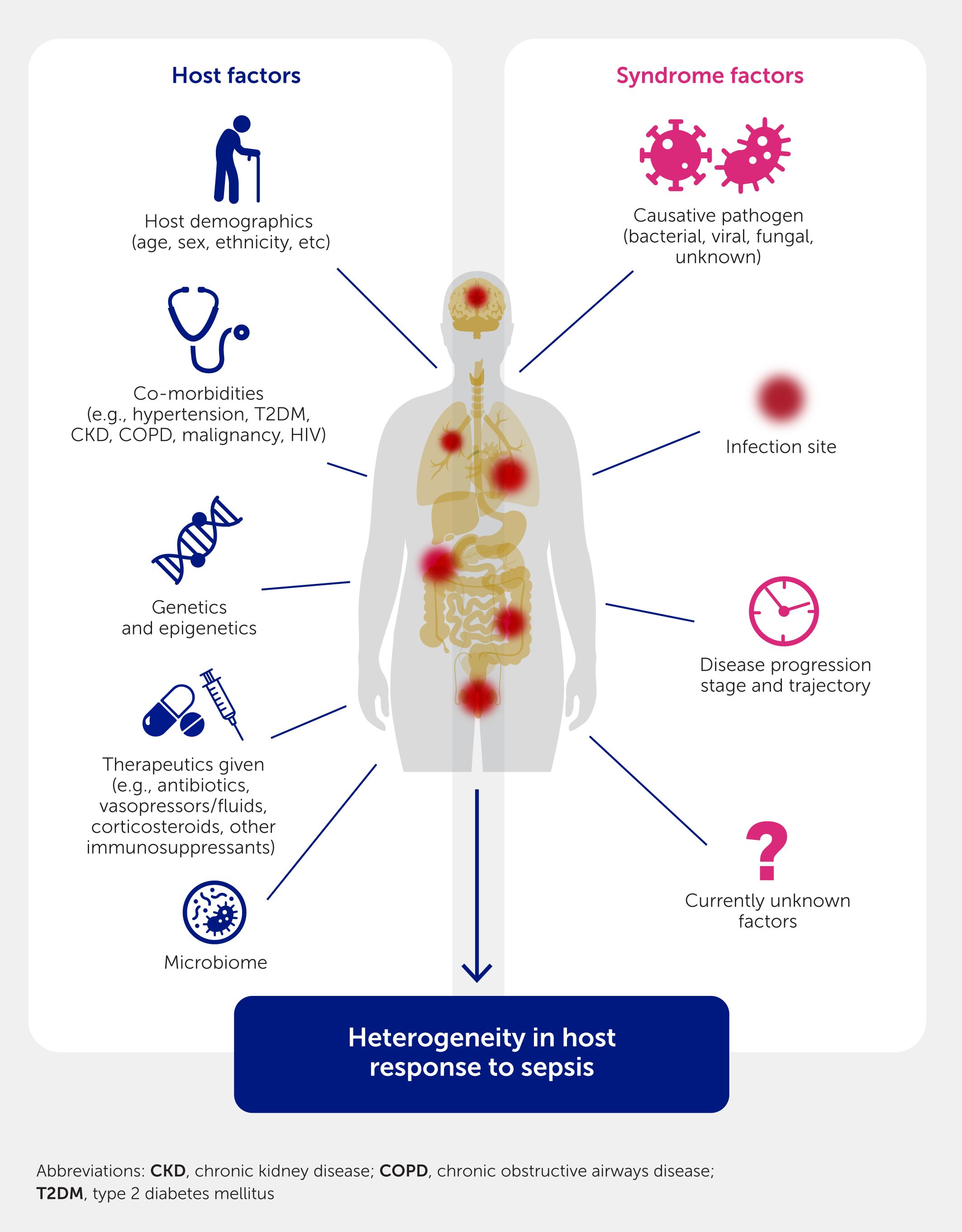
Figure 2. The host response in sepsis can be influenced by multiple factors, resulting in a highly heterogeneous disease.
All of these factors can drive the extreme heterogeneity now evident in sepsis. Fortunately, the perturbations that occur during sepsis are very substantial, creating signals that can be analyzed productively using computational approaches, as described below.
A key barrier to systems immunology research to date has been that it is expensive and resource-intensive. These approaches require highly trained, collaborative teams with a range of expertise. Consequently, the majority of studies have been limited to a few high-income countries, especially the United States, with relatively few in lower-income regions where the major burden of sepsis exists, and there have been no global research initiatives on sepsis, except for Sepsis-3 (1).
Another barrier is the limited volume of systems immunology data, resulting from small study sizes, especially for single-cell approaches. Systems approaches work better with large sample sizes for discovery and validation; otherwise, there are high risks of both type 1 and 2 errors, and results suffer from variations in the demographics and etiology of the sampled populations, clinical metadata collected, and analytical methodologies.
Finally, other research barriers include the paucity of informative diagnostics, a lack of legitimate animal models of sepsis for testing investigational drugs, and, crucially, a lack of endotype-specific models.
Using systems immunology to facilitate early sepsis diagnosis
The high mortality rate of sepsis can likely be attributed to two factors: difficult and often delayed diagnosis and the lack of sepsis-specific treatments. The difficulty of sepsis diagnosis, especially early in disease, can result in delayed treatment with antibiotics (or monoclonal antibodies and antivirals in the case of COVID-19). This delay has been documented to drastically increase mortality rates: for every hour that appropriate antibiotic therapy is delayed, the in-hospital mortality rate can increase very significantly (46), with numbers often cited of up to 7.6% in septic shock (22).
Challenges in sepsis diagnosis
The first symptoms of sepsis are non-specific (e.g., altered temperature, blood pressure, respiratory rate, and heart rate), making early diagnosis difficult (47). Based on the most recent Sepsis-3 criteria (1), sepsis is diagnosed if there is documented or suspected infection along with an indication of organ dysfunction, represented by an increase of two or more points in the Sequential Organ Failure Assessment (SOFA) score (1). The SOFA score assesses the organ function of six systems, namely the cardiovascular, respiratory, hepatic, renal, neurological, and coagulation systems, using appropriate clinical measures (48). Sepsis has been historically described as “severe sepsis” when such acute organ dysfunction occurs (49). Unfortunately, the surrogate early-SOFA score, termed qSOFA, is quite inaccurate in diagnosing progression to sepsis, with a sensitivity of 46% in a recent meta-analysis (50), meaning that over half of sepsis patients can be missed. For infection detection, the gold standard is bacterial identification in a blood or tissue culture (47). However, in a large meta-analysis, around half of sepsis cases were culture-negative (51), and even the suites of new rapid identification methods using mass spectrometry or multiplex polymerase chain reaction (PCR) assays (52) still fail to identify a causative pathogen in most cases. This could be because the progression of sepsis probably does not require the continued presence of the live pathogen that triggered the initial immune dysfunction, or it could reflect sepsis caused by non-culturable bacterial pathogens, fungi, or viruses—e.g., in the case of COVID-19 sepsis (4, 5). Moreover, even culture positivity may not necessarily be associated with “true” sepsis, reflecting our inability to distinguish colonization from infection, particularly in patients with significant comorbidities that affect the SOFA score (such as acute myocardial infarction) or non-sepsis physiological disturbances (such as asymptomatic bacteriuria).
Systems immunology-based diagnosis
To address the difficulty of pathogen detection and the imprecision of qSOFA, many have attempted to leverage changes in the host response as a proxy for infection, especially since this appears to be the definitive cause of the associated organ failure and mortality. A variety of blood biomarkers, mainly reflective of inflammation, have been developed to diagnose sepsis with varying accuracies. C-reactive protein and procalcitonin are considered to have the greatest potential, but others include interleukin (IL)-6, a soluble triggering receptor expressed on myeloid cells-1 (TREM-1), presepsin (soluble cleaved CD14), and calprotectin (53). Generally speaking, these tests do not work well in early sepsis and are not very specific or predictive (54).
Conversely, gene expression signatures are gaining popularity in sepsis diagnosis and prognosis, with a variety proposed based on whole-blood transcriptomics (55–61). These signatures are specific groups of genes, the expression of which is correlated with a specific clinical feature (in this case, sepsis). Using the systems immunology methods described above, signatures are often identified from an initial population of genes that are differentially expressed in sepsis compared with a control group (e.g., healthy patients) and can be further refined through ML/clustering methods to identify the top genes with discriminatory value (35). Table 1 describes a range of signatures and their performance using a common set of seven studies encompassing 1661 patients by comparing gene expression levels to two housekeeping genes (that do not change in sepsis); these signatures varied in their stated purpose, their accuracy/area under the curve (AUC), and the number of patients investigated. In many cases they were calculated from gene expression data obtained when patients already had sepsis. Nevertheless, the best tests are able to accurately classify 68–84% of patients while largely or completely failing to classify patients with different degrees of severity for cancer, cardiac disease, and inflammatory bowel disease.
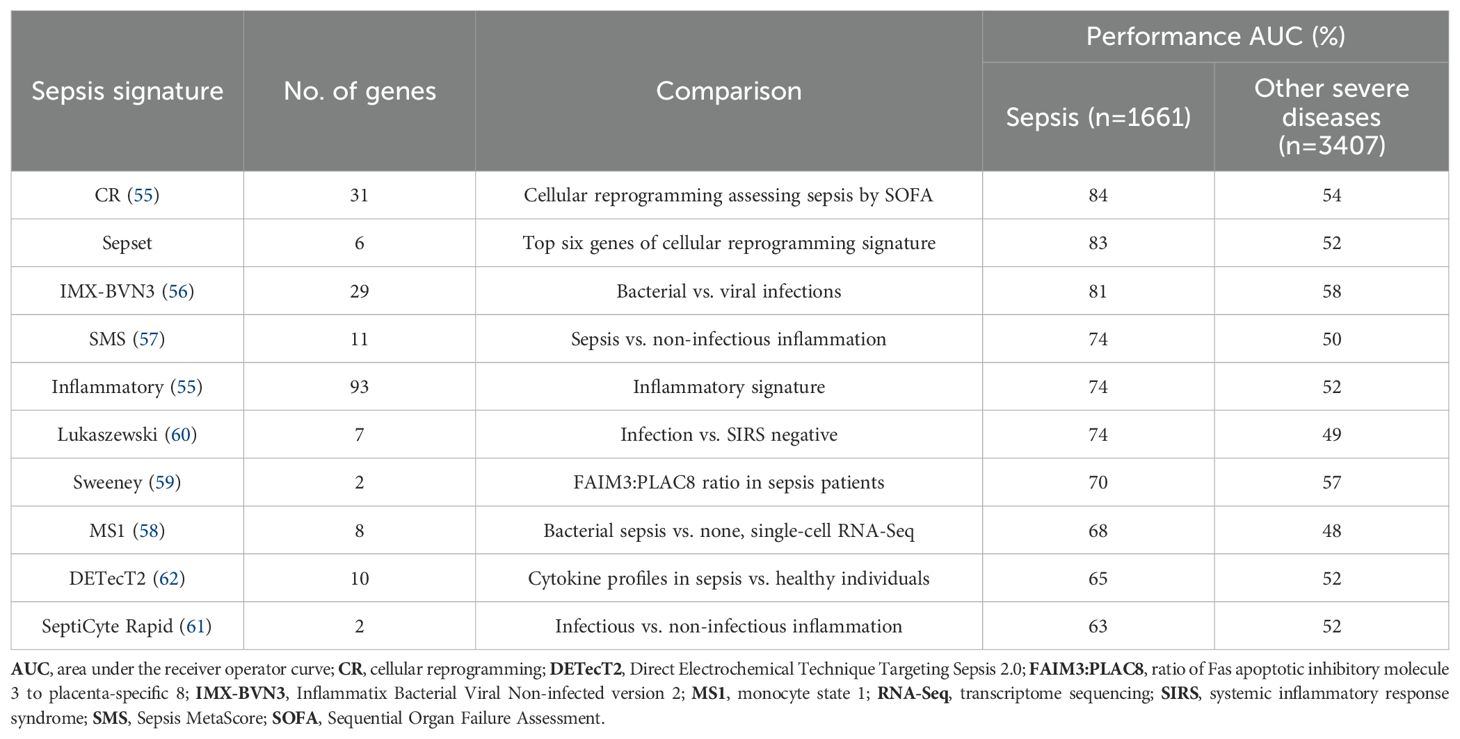
Table 1. Comparative performance of sepsis-related signatures. The “sepsis” column shows the predictive value of the signature (higher is better) while the “other severe diseases” column shows the specificity for sepsis (closer to 50% is better). Signatures were generally derived from machine learning approaches to obtain diagnostic signatures using the authors' own and/or public data (55–62). Seven published datasets of differentially expressed genes (32, 34, 63–67) from 1661 patients at various stages of hospital entry, from the emergency room to early intensive care unit, were analyzed for this review using known signatures of varying sizes (except for the inflammation comparison set, which was from a subset of human volunteers injected with bacterial endotoxin), using a common pair of housekeeping genes as a reference. As a control, the ability of the signature to predict severe versus mild disease states in a combined 3407 patients with severe inflammatory bowel disease (68), stage 3–4 cancer (https://www.cancer.gov/tcga), cardiogenic shock (GSE93101 dataset), and coronary artery disease (69) was tested. Area under the receiver operator curve (AUC) was assessed courtesy of Dr. Peter Zhang (University of British Columbia, Vancouver, BC, Canada), as a measure of accuracy; for example, when AUC = 0.83 (83%), a model based on the signature has an 83% chance of predicting a higher risk of sepsis for a random high-severity sample compared with a random low-severity sample. Note: these data are not a comment on the performance of any specific commercial test or signature since the methods for detection and standardization will vary from those used here to perform a comparative assessment.
Many signatures are based on ML algorithms and scored similarly to the “inflammatory” signature (60–74% AUC) derived from genes active in a non-specific inflammatory response. However, two signatures stand out: the cellular reprogramming (CR; or endotoxin tolerance) signature and its condensed version, SepsetER. The CR signature, generated through systems immunology methods, was developed based on a hypothesis regarding the nature of the early immune dysfunction in sepsis (55) and has been shown to predict progression to severe sepsis and organ failure with relative accuracy (35, 55). CR refers to the process by which innate immune cells such as monocytes or macrophages lose their ability to respond appropriately to pathogens after two or more challenges with bacterial lipopolysaccharide (LPS) or other agonists of Toll-like receptors (55, 70, 71) (Figure 3). CR starts as early as the first clinical presentation of sepsis, and CR monocytes are observed in sepsis (72) with impaired antigen presentation, dysregulated cytokine production (55, 70, 71), and the ability to suppress T-cell function (73). It was hypothesized that an initial pre-clinical infectious challenge represents an initial stimulation (74), and a later infection or the systemic spread of the initial infection acts to subsequently induce CR, allowing it to be detected early in sepsis (55) and thus making it an effective early signature. Considering the innate immune system’s role as the first layer of protection against pathogens and as the initiator of adaptive immune responses, it is not surprising that CR monocytes also affect T-cell functionality (73). In CR monocytes, production of cytokines such as transforming growth factor (TGF-β) and IL-10, decreased antigen presentation, and decreased expression of costimulatory molecules result in decreased T-cell proliferation and interferon (IFN)-γ production. Thus, in sepsis, CR may potentially bridge interactions between an overactive innate immune response and a suppressed adaptive response. Intriguingly, a recent single-cell transcriptome sequencing study to classify the effects of dexamethasone on patients revealed five out of six markers of the CR signature were upregulated in the monocytes of patients who died despite treatment, while at least three were relatively suppressed in patients who survived (75). This suggests that the CR signature might be useful to monitor therapeutic success.
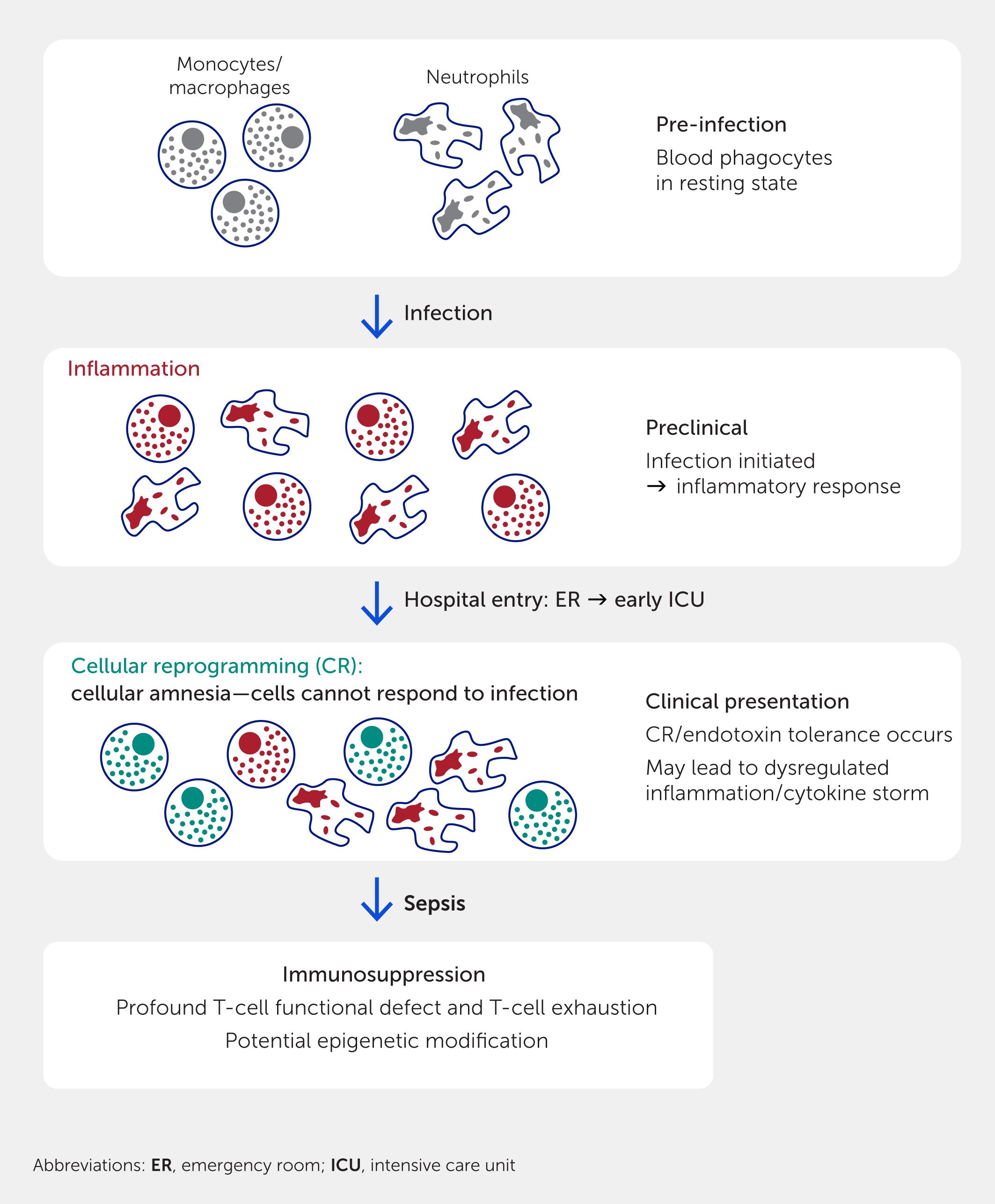
Figure 3. Genesis of cellular reprogramming and other immune suppression and their relationship to sepsis. Cellular reprogramming (also termed endotoxin tolerance) is initiated by two successive treatments with bacterial signature molecules such as lipopolysaccharide. The first treatment stimulates inflammation as an antimicrobial defense mechanism. The second (or perhaps prolonged treatment with the bacterial signature molecule) results in monocytes and macrophages being unable to respond to further bacterial stimuli, an amnesic state termed cellular reprogramming (monocytes and macrophages become a special class of M2 cells). Subsequently, T-cell deficiencies, including immunosuppression and T-cell exhaustion, occur.
Notably, studies with a large number of adults, some of whom went on to develop sepsis, have indicated that transcriptional changes might occur prior to symptom onset (60). This suggests it might be possible to predict the onset of sepsis very early in disease. We have made similar observations in neonates (76).
Implications for early intervention
Since sepsis is an immune dysfunction disease, the early use of immune-corrective therapies could perhaps boost the patient’s immunity and promote the efficacy of front-line treatments such as antibiotics. Although sepsis was originally considered to be a hyperinflammatory disease, more than 100 failed clinical trials using anti-inflammatory therapies (77) have led to a paradigm shift in understanding immune dysfunction in sepsis, with it now understood to involve immunosuppression. Proposed in 1996 by Dr. Roger Bone, this model represented a major breakthrough in our understanding that sepsis is not just inflammation (78) but instead involves what was termed a “compensatory anti-inflammatory response syndrome” (CARS; although the term “compensatory response” may not be correct per se but instead reflects a distinct phase of immune dysfunction). The important concept was that a later immunosuppressive phase dominates the immune response, predisposing patients to nosocomial secondary infections and eventual death. This was supported by findings of apoptosis of CD4+ and CD8+ T-cells, B-cells, and dendritic cells (79); expansion of immunosuppressive cells such as regulatory T-cells (79, 80) and myeloid-derived suppressor cells (81); impaired neutrophil function (82); and cellular reprogramming of antigen-presenting cells such as monocytes (55, 72). These general themes have been recently supported by systems immunology investigations (83). For example, single-cell studies demonstrated a unique monocyte phenotype in sepsis (58) and that patients with severe COVID-19 infections (i.e., viral sepsis) have a dysregulated myeloid compartment (84). Similarly, a recent single-cell transcriptome study (75) on the use of dexamethasone in more severely afflicted COVID-19 patients revealed a monocyte subset that was responsive to dexamethasone and provided a dexamethasone-responsive gene expression signature and a signature (possibly the CR signature, see above) for therapeutic success. Despite these themes, heterogeneity in sepsis is a major issue in this regard and it is noted in the dexamethasone study that up to 30% of patients treated with this immune-modulatory agent died in the RECOVERY trial (85).
Deriving systems-based endotypes: towards precision sepsis care
Previous clinical research has inferred that sepsis is too difficult to characterize comprehensively and mechanistically due to inter-individual variability in symptoms, infectious triggers, time course of disease, pre-existing medical issues/co-morbidities, and patient genetics, age, and demographics (17, 19). This variability is likely responsible for the diverse spectrum of disease manifestation, ranging from relatively mild, transient disease to severe symptoms requiring ventilation that result in organ dysfunction, septic shock, and death. Thus, attempts to identify a one-size-fits-all treatment (i.e., using antimicrobials and supportive care) have seemed doomed to fail, notwithstanding that the diagnostic signatures described in the previous section indicate that there are some common elements to most cases of severe sepsis.
Systems immunology approaches and unsupervised ML methods (e.g., clustering) now allow sepsis patients to be categorized (31, 32, 34, 35) into “endotypes” based on unique immune dysfunction mechanisms corresponding to varying severity and mortality rates (Figure 4). Defined as subtypes of a disease with different pathophysiological mechanisms, endotypes have been substantially described in asthma and other allergic diseases (83). Excitingly, they offer the potential to triage patients to enable different immune-supportive therapies.
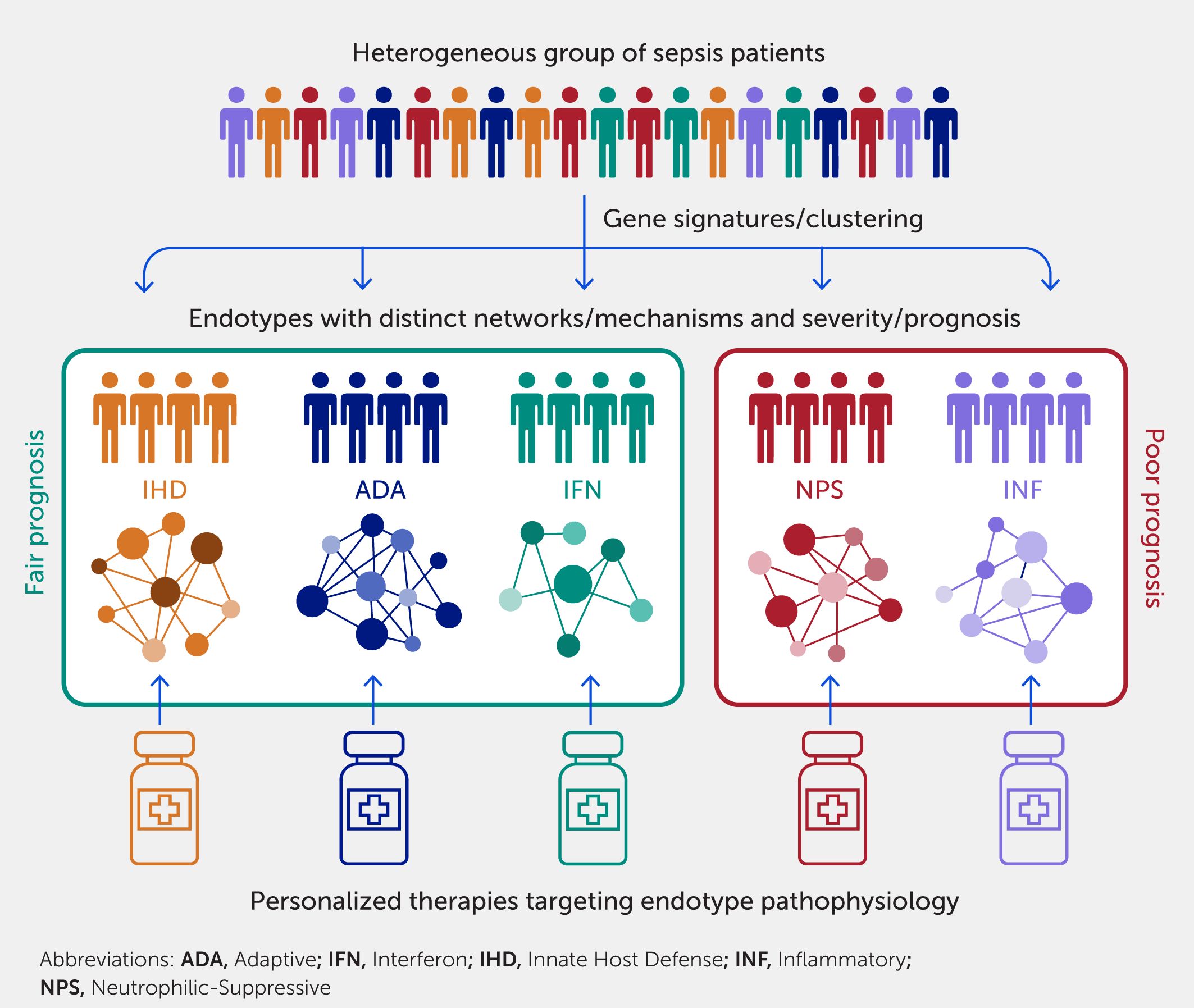
Figure 4. Endotypes uncover novel mechanisms and have clinical implications on prognosis and treatment of sepsis. Endotypes, which are subtypes of a disease with distinct mechanisms, can be identified using systems immunology approaches such as clustering. Gene signatures can then be derived for classification of patients into endotypes, which can aid prognostication and triaging. In (35), five endotypes were discovered and named after their predominant mechanisms: Neutrophilic-Suppressive (NPS) and Inflammatory (INF) had patients with poor prognosis; Interferon (IFN), Adaptive (ADA), and Innate Host Defense (IHD) had fair prognosis. As these endotypes also have distinct mechanisms, targeted therapies can be developed to provide personalized treatments rather than a “one-size-fits-all” approach, which has not been historically successful in treating heterogeneous diseases such as sepsis.
Using ML methods, patients can be divided into endotypes based on their common differential expression of an exclusive set of genes not found in any other patient endotype. A variety of sepsis endotypes have been proposed to stratify patients at ICU admission, including sepsis response signatures (SRS) 1/2 (32), Molecular Diagnosis and Risk Stratification of Sepsis (MARS) 1–4 (33, 34), and inflammopathic/adaptive/coagulopathic (86). Recently, using RNA-Seq transcriptomic data, five endotypes were identified in patients in the emergency department, well before any formal diagnosis (35), indicating that they are actionable and could be used to guide therapy. Each was distinguished by ~200 differentially expressed genes unique to the particular endotypes. The two most severe endotypes, termed Neutrophilic-Suppressive (NPS) and Inflammatory (INF), are almost diametrically opposed in terms of their mechanistic basis, the first reflecting immune suppression and the second hyperinflammatory immune stimulation. The remaining three were less severe endotypes termed Interferon (IFN), Adaptive (ADA), and Innate Host Defense (IHD) (35). Generally, these endotypes were also identified in early ICU patients, patients with severe COVID-19 disease, and large sepsis datasets from other studies (35, 63). The extent to which these endotypes are stable throughout the course of sepsis has not been studied. However, a recent study has linked neutrophils to immune suppression and disease (87), consistent with the observation that the NPS endotype has very high mortality.
The specific gene sets identified in other studies (32, 34, 35, 86) as discriminating between sets of endotypes vary, likely reflecting (in part) the patient inclusion criteria and methods used. However, these different endotype classification systems share trends, in that endotypes that capture processes related to higher levels of inflammation and/or lower adaptive immune function are associated with worse prognoses.
Embracing the concept of sepsis endotypes will warrant a rethinking of patient triage and treatment by the healthcare community; to facilitate this mindset change, researchers are now developing endotype-specific diagnostics. Regarding treatment, a computational approach (Figure 5) was recently used to predict drugs that are effective for subsets of endotype-specific differentially expressed genes (63). This comprises mapping these genes to PPI networks, identifying the hubs (genes with the largest numbers of interconnections in the network and thus considered to have maximal involvement in the biological processes described by the network), and then identifying existing approved (potentially repurposed) drugs that target these genes (see below). Another method is to use the Drug Signatures Database (DSigDB) (88), a repository of medications approved by the United States Food and Drugs Administration (FDA) and their experimentally validated interactions (e.g., antagonism) with genes/proteins. This can be used to assess the enrichment of particular drug signatures among endotype-specific differentially expressed genes. These methods enabled identification, for example, of dexamethasone as a potential drug for treating the NPS endotype (63). Indeed, dexamethasone has shown efficacy in assisting some patients with early severe sepsis (85, 89) and yet is somewhat counterintuitive, especially since it is considered anti-inflammatory and many other anti-inflammatory regimens have failed in sepsis (77). This provides an excellent example of how omics might realign patients with their appropriate therapy. Intriguingly, recent studies indicated that, in COVID-19 sepsis, dexamethasone triggers a defined subset of monocytes and reverses aberrant monocyte regulation (75); taken together with the observation that dexamethasone only works in a subset of patients (85), this might indicate that this monocyte subset is favored in the NPS endotype.
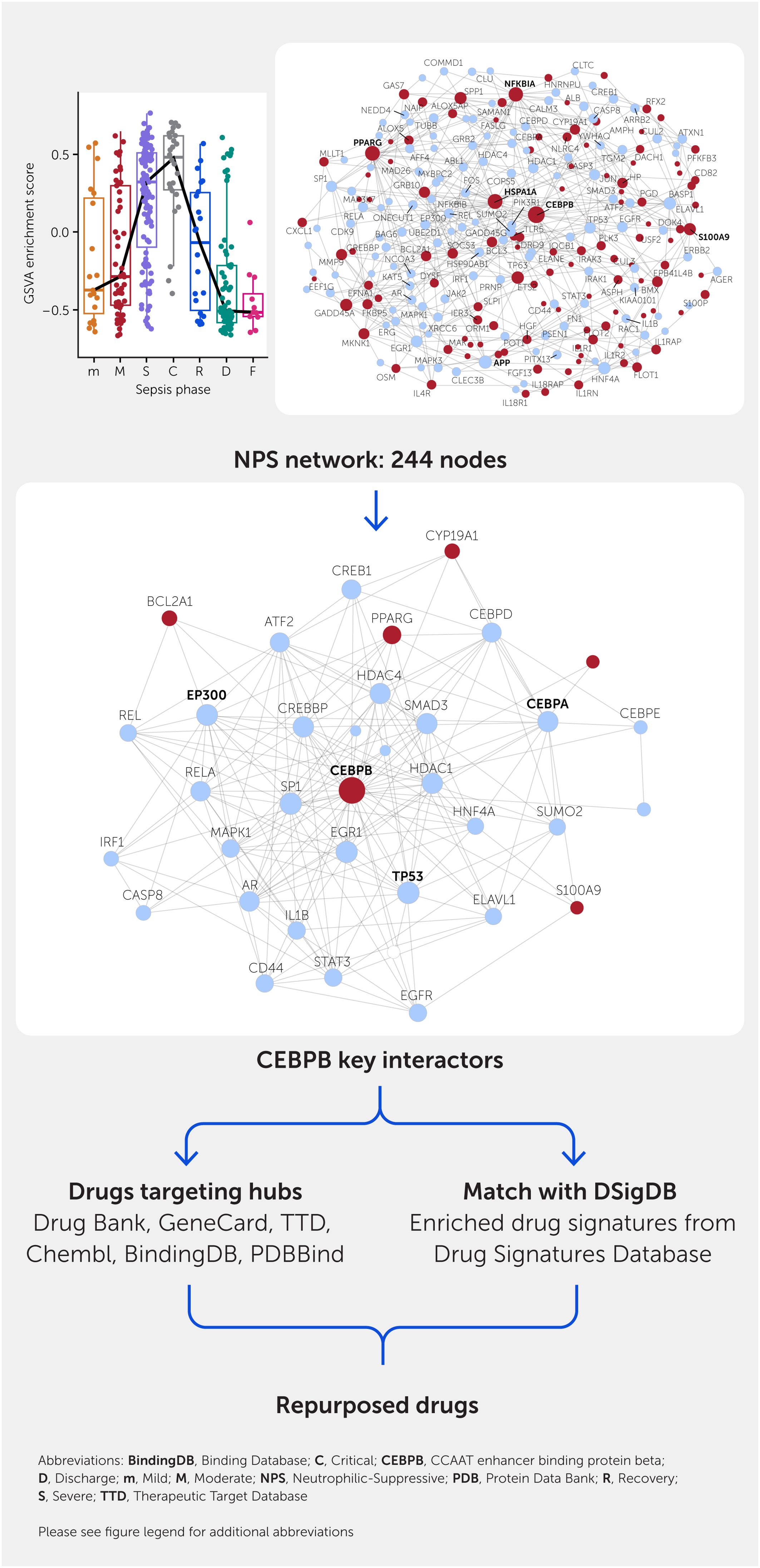
Figure 5. Repurposed drug predictions from transcriptomic data. This is illustrated for the set of genes uniquely expressed in patients of the NPS endotype but, as shown on the upper part, this endotype is especially relevant to patients in the Critical phase of sepsis [phases are abbreviated as Mild (m), Moderate (M), Severe (S), Critical (C), Recovery (R), and Discharge (D)]. A minimally connected protein—protein interaction network (top right) is drawn from the differentially expressed (red nodes) genes including close interactors that are not themselves differentially expressed (blue nodes). To obtain candidate targets, highly connected hubs (larger nodes with connections to the maximum number of other nodes) are deleted within NetworkAnalyst, and nodes that become less connected are considered to be the most important for the interaction network for the particular hub (shown for CEBPB top right). Known drugs (candidates for repurposing) targeting the hubs are extracted from a variety of candidate databases. Conversely, DSigDB comprising enriched drug—gene signatures are extracted from the Drug Signatures Database and examined for enrichment in the gene expression protein—protein interaction network. Any candidate drugs obtained will be known to be safe for human use but will need to be specifically tested in the context of sepsis to justify use in sepsis patients. The general effectiveness of this method is revealed by the fact that it predicts drugs with known efficacy in at least a subset of sepsis patients and/or in animal models. Abbreviations: ABL1, ABL proto-oncogene 1, non-receptor tyrosine kinase; AFF4, ALF transcription elongation factor 4; AGER, advanced glycosylation end-product specific receptor; AKR1B1, aldo-keto reductase family 1 member B; ALB, albumin; ALOX5, arachidonate 5-lipoxygenase; ALOX5AP, arachidonate 5-lipoxygenase activating protein; ALPK3, alpha kinase 3; AMPH, amphiphysin; APP, amyloid beta precursor protein; AR, androgen receptor; AREG, amphiregulin; ARRB2, arrestin beta 2; ASPH, aspartate beta-hydroxylase; ASZ1, ankyrin repeat, SAM and basic leucine zipper domain-containing 1; ATF2, activating transcription factor 2; ATXN1, ataxin 1; BAG6, BAG cochaperone 6; BASP1, brain-abundant membrane-attached signal protein 1; BCL2A1, BCL2-related protein A1; BCL3, BCL3 transcription coactivator; BMX, BMX non-receptor tyrosine kinase; CALM3, calmodulin 3; CASP3, caspase 3; CASP8, caspase 8; CCM2, CCM2 scaffold protein; CD44, CD44 molecule (IN blood group); CD82, CD82 molecule; CDK9, cyclin dependent kinase 9; CEBPA, CCAAT enhancer binding protein alpha; CEBPB, CCAAT enhancer binding protein beta; CEBPD, CCAAT enhancer binding protein delta; CEBPE, CCAAT enhancer binding protein epsilon; CLEC3B, C-type lectin domain family 3 member B; CLTC, clathrin heavy chain; CLU, clusterin; COMMD1, copper metabolism domain-containing 1; COPS5, COP9 signalosome subunit 5; CREB1, cAMP-responsive element-binding protein 1; CREBBP, CREB-binding protein; CUL2, cullin 2; CUL3, cullin 3; CXCL10, C-X-C motif chemokine ligand 10; CXXC1, CXXC finger protein 1; CYP19A1, cytochrome P450 family 19 subfamily A member 1; DACH1, dachshund family transcription factor 1; DLC1, DLC1 Rho GTPase-activating protein; DOK4, docking protein 4; DYSF, dysferlin; EEF1G, eukaryotic translation elongation factor 1 gamma; EFNA1, ephrin A1; EGFR, epidermal growth factor receptor; EGR1, early growth response 1; ELANE, elastase, neutrophil expressed; ELAVL1, ELAV-like RNA binding protein 1; EP300, E1A-binding protein p300; EPB41L4B, erythrocyte membrane protein band 4.1-like 4B; ERBB2, erb-b2 receptor tyrosine kinase 2; ERG, ETS transcription factor ERG; ETS2, ETS proto-oncogene 2, transcription factor; FASLG, Fas ligand; FBF1, Fas binding factor 1; FDXR, ferredoxin reductase; FGF13, fibroblast growth factor 13; FKBP5, FKBP prolyl isomerase 5; FLOT1, flotillin 1; FLOT2, flotillin 2; FN1, fibronectin 1; FOS, Fos proto-oncogene, AP-1 transcription factor subunit; GADD45A, growth arrest and DNA damage-inducible alpha; GADD45G, growth arrest and DNA damage-inducible gamma; GDNF, glial cell-derived neurotrophic factor; GRB10, growth factor receptor-bound protein 10; GRB2, growth factor receptor-bound protein 2; GSVA, gene set variation analysis; HDAC1, histone deacetylase 1; HDAC4, histone deacetylase 4; HGF, hepatocyte growth factor; HNF4A, hepatocyte nuclear factor 4 alpha; HNRNPU, heterogeneous nuclear ribonucleoprotein U; HP, haptoglobin; HSP90AB1, heat shock protein 90 alpha family class B member 1; HSPA1A, heat shock protein family A (Hsp70) member 1A; IER3, immediate early response 3; IL18R1, interleukin 18 receptor 1; IL18RAP, interleukin 18 receptor accessory protein; IL1B, interleukin 1 beta; IL1R1, interleukin 1 receptor type 1; IL1R2, interleukin 1 receptor type 2; IL1RAP, interleukin 1 receptor accessory protein; IL1RN, interleukin 1 receptor antagonist; IL4R, interleukin 4 receptor; IL6, interleukin 6; IQCB1, IQ motif-containing B1; IRAK1, interleukin 1 receptor-associated kinase 1; IRAK3, interleukin 1 receptor-associated kinase 3; IRF1, interferon regulatory factor 1; JAK2, Janus kinase 2; JUN, Jun proto-oncogene, AP-1 transcription factor subunit; KAT5, lysine acetyltransferase 5; MAK, male germ cell-associated kinase; MAP3K7, mitogen-activated protein kinase kinase kinase 7; MAPK1, mitogen-activated protein kinase 1; MAPK3, mitogen-activated protein kinase 3; MED26, mediator complex subunit 26; MKNK1, MAPK interacting serine/threonine kinase 1; MLLT1, MLLT1 super elongation complex subunit; MMP9, matrix metallopeptidase 9; MYBPC2, myosin-binding protein C2; NAIP, NLR family apoptosis inhibitory protein; NCOA3, nuclear receptor coactivator 3; NEDD4, NEDD4 E3 ubiquitin protein ligase; NFKBIA, NFKB inhibitor alpha; NFKBIB, NFKB inhibitor beta; NLRC4, NLR family CARD domain-containing 4; NPS, Neutrophilic-Suppressive; ONECUT1, one cut homeobox 1; ORM1, orosomucoid 1; OSM, oncostatin M; PCLAF, PCNA clamp-associated factor; PFKFB3, 6-phosphofructo-2-kinase/fructose-2,6-biphosphatase 3; PGD, phosphogluconate dehydrogenase; PIK3R1, phosphoinositide-3-kinase regulatory subunit 1; PITX3, paired-like homeodomain 3; PLK3, polo-like kinase 3; POT1, protection of telomeres 1; PPARG, peroxisome proliferator activated receptor gamma; PRNP, prion protein (Kanno blood group); PSEN1, presenilin 1; RAC1, Rac family small GTPase 1; REL, REL proto-oncogene, NF-kB subunit; RELA, RELA proto-oncogene, NF-kB subunit; RFX2, regulatory factor X2; RNASE1, ribonuclease A family member 1, pancreatic; S100A9, S100 calcium-binding protein A9; S100P, S100 calcium-binding protein P; SAMSN1, SAM domain, SH3 domain and nuclear localization signals 1; SLPI, secretory leukocyte peptidase inhibitor; SMAD3, SMAD family member 3; SOCS3, suppressor of cytokine signaling 3; SOS1, SOS Ras/Rac guanine nucleotide exchange factor 1; SP1, Sp1 transcription factor; SPP1, secreted phosphoprotein 1; STAT3, signal transducer and activator of transcription 3; SUMO2, small ubiquitin-like modifier 2; TDRD9, tudor domain-containing 9; TGM2, transglutaminase 2; TLR5, toll-like receptor 5; TP53, tumor protein p53; TP63, tumor protein p63; TUBB, tubulin beta class I; TUBB2A, tubulin beta 2A class IIa; UBE2D1, ubiquitin-conjugating enzyme E2 D1; USF2, upstream transcription factor 2, c-fos interacting; XRCC6, X-ray repair cross complementing 6; YWHAQ, tyrosine 3-monooxygenase/tryptophan 5-monooxygenase activation protein theta.
Why sepsis patients separate into different endotypes is not well understood. Potential causes could be individual factors such as host genetics, microbiome, age, co-morbidities, infecting pathogen, and treatments received (17, 19). It could also reflect the disease stage, since not all patients present to hospital at the same stage (19) (as explored below). Regardless, the presence of endotypes highlights the potential for and importance of personalized treatments for sepsis and is one of the areas of highest potential for future sepsis studies. We see a future where personalized treatments will replace the current inflexible “one-size-fits-all” approach of sepsis bundles and years of failed clinical trials (77).
Deciphering sepsis progression using systems immunology
The European Group on Immunology of Sepsis has identified “the dynamics of sepsis-associated immunological alterations” (90) as one of the major gaps in sepsis research. Indeed, time after illness onset is important in interpreting the underlying genomic response (91). Sepsis immune dysfunction is highly complex and varies substantially over time (8, 91, 92), which can further contribute to already-existing heterogeneity. Since patients arrive at hospital at varying stages in their disease progression, analyzing a single timepoint (e.g., at hospital or ICU admission) is valuable for determining predictive biomarkers/diagnostics and endotypes for potential early immune-modulatory drug treatments but cannot address this heterogeneity over time. Instead, analyzing trends is likely to be more informative (8, 64, 93). Sampling at multiple timepoints during and after hospitalization is necessary to elucidate disease trajectories, i.e., analyzing how gene expression changes within individual patients due to disease progression enables a mechanistic understanding of why some die while others do not. Analysis of within-patient temporal trends also helps to overcome the obstacle of inter-individual heterogeneity, since patients act as their own baseline and comparator at different timepoints, thereby accounting for inherent genetic, infectious trigger, microbiome, co-morbidity, and demographic differences. In addition, understanding the dynamics of the immune response in sepsis, which likely evolves over time, can also inform future personalized treatment with different medications required for targeting different time-dependent responses associated with progression, lethality, and post-sepsis complications (64).
Eight early systems-level transcriptomic (microarray) studies were summarized previously (91). Identified trends during sepsis progression included increased suppression of adaptive immune genes, disrupted cell cycle control, increased neutrophil-specific proteases, and increases in selected inflammatory cytokines. An examination of the findings from endotype studies (31, 32, 34, 35) have implicated particular endotypes as having more dominant individual characteristics than others. More detailed transcriptomic studies have examined the progression of COVID-19-induced sepsis but generally have had small sample sizes (<15 patients) and variable classification of disease phases (94–96). These showed consistent elevations of inflammatory mediators, dysregulated coagulation, and decreases in adaptive immunity during the most severe stages of COVID-19, followed by reversal of these pathological processes during recovery stages.
A recent longitudinal investigation (8) of 42 ICU patients with sepsis (20 with and 22 without COVID-19) characterized blood transcriptomes by RNA-Seq at ICU admission and approximately 1 week later. All patients demonstrated common underlying immune dysfunction, with upregulation of immune processes such as IL-1 signaling and interleukin-6/Janus kinase (JAK)/signal transducer and activator of transcription-3 (STAT3) signaling throughout the disease course. Interestingly, at ICU admission, COVID-19 patients had 1,215 differentially expressed genes compared with non-COVID-19 sepsis patients, primarily supportive of a robust antiviral response (8). However, after a week in the ICU, the number of differentially expressed genes dropped to just nine, even after correcting for cellular proportions, making these two disease groups transcriptionally almost indistinguishable. Only through such a longitudinal systems immunology approach could this convergence be observed, supporting the idea that severe COVID-19 is a form of sepsis and that immune dysregulation, and not the pathogen, is the main issue (Figure 6A).
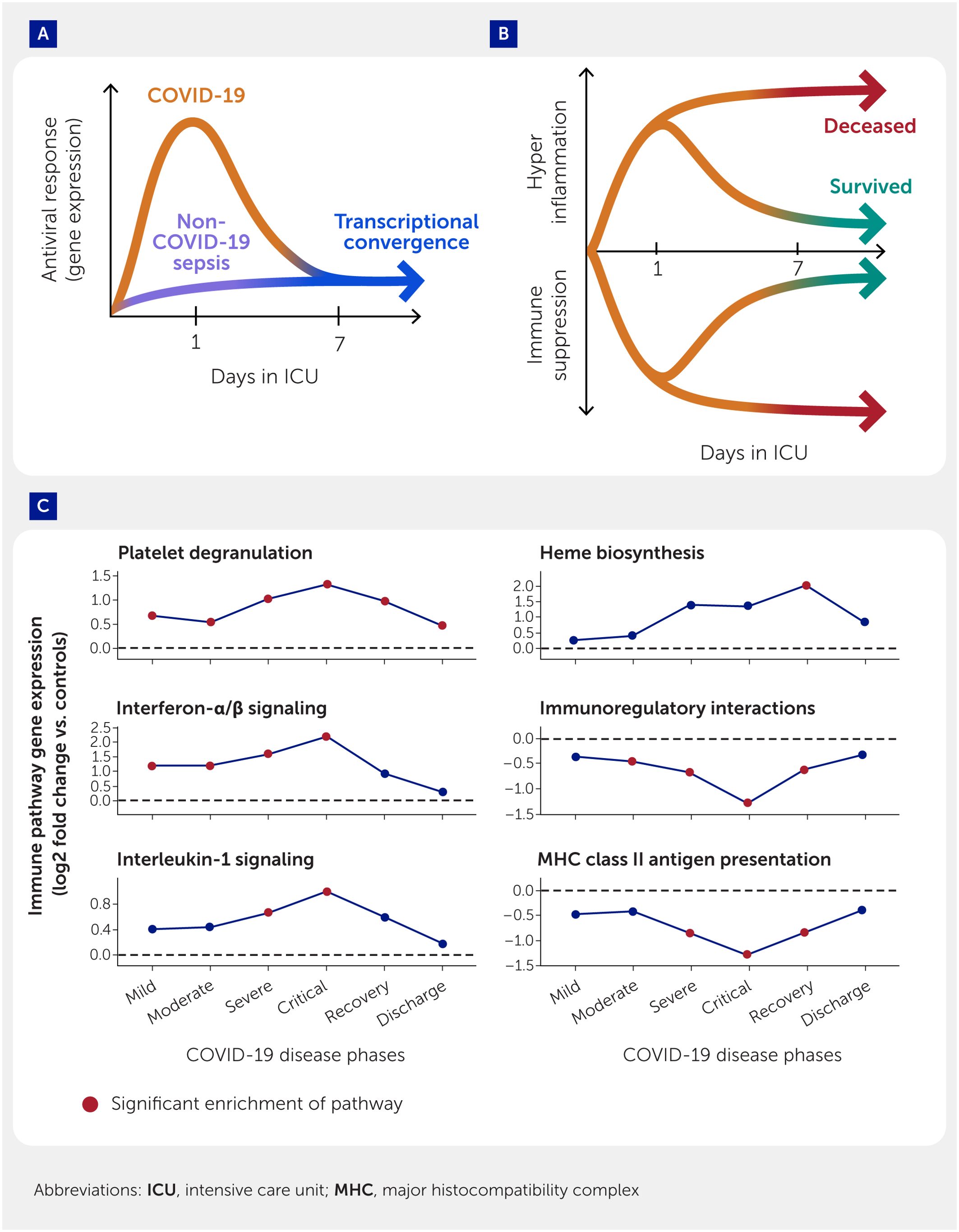
Figure 6. Longitudinal systems immunology analyses highlight mechanistic temporal changes in sepsis and COVID-19. (A) Schematic representing findings uncovered by systems immunology approaches that COVID-19 patients initially had a strong antiviral response at Day 1 of intensive care unit (ICU) admission but became transcriptionally similar to non-COVID-19 sepsis patients at Day 7 in the ICU. (B) Eventual mortality of sepsis patients in the ICU was associated with persistent immune dysfunction in terms of both uncontrolled inflammation and adaptive immunity suppression (orange line). (C) Distinct pathways are activated at six different disease phases of COVID-19. Line graphs representing trends of pathway enrichment with Mild, Moderate, Severe, Critical, Recovery, and Discharge phases. Points are the median log2 fold change of the set of differentially expressed genes of each pathway. Red points denote significant enrichment of pathway at that phase.
A subsequent analysis of these patients observed major differences in temporal patterns of gene expression in patients who died as opposed to those who survived (64). Non-survivors had many more “persistently altered” genes (i.e., 4,289 genes that remained up- or downregulated at both timepoints) when compared with survivors (1,186 genes); this was also observed using two publicly available datasets of COVID-19 and sepsis patients. Persistently downregulated genes included those involved in T-cell signaling, whereas persistent upregulation occurred in selected innate immune and metabolic pathways. This strongly suggests that immune dysfunction was resolved in survivors but not in patients who died (Figure 6B), i.e., persistent immune dysfunction predicts mortality. This was generally consistent with the concept of a 10-gene differential expression signature that was correlated with eventual mortality (AUC 75% in all-cause sepsis and 86% in COVID-19 sepsis) (35, 63). Using systems biology methods, a broad variety of immunomodulatory drug candidates, e.g., corticosteroids, were predicted that could potentially target this persistent immune dysfunction (64).
Disease progression was also investigated in a larger cohort of 128 hospitalized COVID-19 patients (300 blood samples subjected to RNA-Seq analysis) (97). Assessing severity according to the SOFA score, ICU admission, and general trajectories of severity (e.g., downward trend in SOFA score), six disease phases were proposed for COVID-19 patients. Each phase was distinct in both enriched differentially expressed pathways and a variety of clinical metadata, suggesting that the phases capture distinct stages of COVID-19 illness. Overall, many genes were differentially expressed between the successive Mild, Moderate, Severe, Critical, Recovery, and Discharge phases, with each phase characterized by particular pathways (Figure 6C).
Substantial further research is necessary to elucidate the complexities of sepsis progression (especially all-cause sepsis). However, the exceptional remodeling of immune functions over time was very prominent in the aforementioned study. Notably, a variety of immune pathways were highly dysregulated, including adaptive immune pathways being highly enriched in downregulated genes from the Moderate to Recovery phases. Other adaptive immune pathways were also downregulated, starting at the Critical phase. Traditionally, innate immune pathways, including IL-1 signaling and IL-4/13 signaling, were often enriched only in the most severe disease phases. Conversely, genes associated with neutrophil degranulation were significantly upregulated at all phases, suggesting underlying processes that were activated throughout the entire disease timeline as well as discharge.
These observed gene expression differences allowed the identification of disease stage-specific repurposed drugs (following the method illustrated in Figure 5 for the NPS endotype, which is prominent in the Critical phase of sepsis), which could differentially target particular disease stages, potentially facilitating time-dependent sepsis treatment (97). In the initial stages of sepsis, the highest hit was the antihistamine cyproheptadine, which is currently being investigated in COVID-19 (clinicaltrials.gov NCT04820751) due to its function as an anti-serotonergic, with the possibility of improving organ dysfunction. At the Critical stage of sepsis, the top hit was metformin, which influences energy metabolism; observational studies have linked metformin use to decreased COVID-19 severity (98).
Understanding post-sepsis syndrome and long COVID
In addition to its high case-fatality rate, sepsis often has major downstream consequences for its survivors (10). In the post-discharge period, sepsis patients are frequently readmitted to hospital for other recurrent infections, such as pneumonia or urinary tract infections (99), consistent with an impaired immune system that predisposes survivors to infections. Post-sepsis immune dysfunction is likely a continuation or sequelae of the dysregulated immune response during the septic event (30). Indeed, at least half of all survivors have long-term consequences, and one-third die within 1 year after discharge (100). These dire prognoses are a result of “post-sepsis syndrome”, which has not been adequately researched (10, 100) as most studies and clinical trials focus on acute sepsis outcomes such as organ failure and in-hospital mortality. However, these long-term consequences gained renewed interest in the COVID-19 pandemic due to the emergence of the highly related syndrome “long COVID”, which affects up to 50–70% of COVID-19 patients (101).
Post-sepsis syndrome and long COVID
Post-sepsis syndrome is characterized by frequent hospital readmissions and increased mortality due to persistent immune dysfunction, cardiovascular disease, functional disability, psychiatric changes, and cognitive impairment, causing poor quality of life and increasing the burden on healthcare systems (10, 99, 100). Prior sepsis is the most common reason for 30-day unplanned rehospitalization, with staggering healthcare costs (>US$10,000 per patient) exceeding diseases such as acute myocardial infarction, chronic obstructive pulmonary disease, heart failure, and pneumonia; the indirect costs are estimated at billions of dollars annually (14, 102). Sepsis survivors have higher mortality rates than non-septic critical illness survivors (10). Furthermore, long-term sequelae cannot be attributed solely to poor health status prior to sepsis in >20% of sepsis survivors (99, 100). Post-sepsis issues are poorly understood and need to be investigated (30); encouragingly, the upsurge of long COVID has increased research dramatically. Intriguingly, latent class analysis identified clinical subphenotypes of sepsis at ICU discharge with distinct clinical biomarker profiles, suggesting persistent dysfunction of specific biological pathways (103).
Certain analyses of gene expression have been performed to compare patients with or without persistent post-COVID symptoms. A profiled cohort of 69 patients demonstrated evidence of persistent transcriptomic dysregulation up to 24 weeks post discharge in patients with persistent symptoms but unfortunately did not profile patients while hospitalized (104). A larger cohort of 165 patients was used to assess differences in samples collected in-hospital between patients who did or did not acquire long COVID symptoms at a median of 1 year after discharge (105). They observed a relationship between specific symptoms, immunoglobulin-related genes, and plasma cells in hospital but did not investigate gene expression at follow-up for comparison. The most comprehensive analysis to date has been a single-cell RNA-Seq cohort profiling peripheral blood mononuclear cells of 209 patients at clinical diagnosis, acute disease, and 2–3 months post disease onset (45). This study found four endotypes related to T-cell and monocyte gene expression patterns, highlighting the impact of heterogeneity in long COVID. Pathway analysis of the four patient endotypes revealed coordinated expression patterns across innate and adaptive immune cell types, with polarization reminiscent of the canonical type 1 and type 2 immune responses. Thus, the type 1 group was enriched in Th1-like signatures in CD4+ T-cells, M1-like pro-inflammatory signatures in monocytes, cytotoxic effector signatures in CD8+ T-cells and natural killer (NK) cells, and memory signatures in B cells. In contrast, the type 2 group was enriched in Th2-like CD4+ T-cell signatures, M2-like (anti-inflammatory) monocyte signatures, and a plasma B cell signature. The “intermediate” group exhibited a transitional immune status between types 1 and 2. The “naïve” group exhibited naive-like T and B cell signatures and resting NK cell signatures. Thus, major differences in single-cell immune responses that were associated with divergent acute severity and post-acute sequelae of COVID-19 (long COVID) were evident (45).
A bulk RNA-Seq whole-blood study (106) compared 10 patients with long COVID to 14 asymptomatic patients, examining both hospital and follow-up samples by RNA-Seq, to determine underlying differences between patients who developed long COVID and those without persistent post-COVID symptoms. Trajectory analysis (from hospital to follow up) was performed to account for individual factors, including genetics, demographics, diet, microbiome, and age. This analysis showed that massive temporal gene expression changes occurred in patients without post-COVID symptoms that did not occur in those who had symptoms. Specifically, these temporally differentially expressed genes (from hospital to follow up) reflected a decrease over time in clotting pathways and inflammation and an increase over time in activity of adaptive immunity pathways. Patients were separated into three endotypes with different mechanisms. The “Resolved” endotype (lowest rate of post-COVID symptoms) had strong inflammatory and hemostatic responses in hospital that resolved after discharge. Conversely, in the “Suppressive” and “Unresolved” endotypes, which had higher rates of patients with post-COVID symptoms, the inflammatory/hemostatic responses were persistently dampened and activated, respectively, reflecting deficient modulation of these perturbations. Endotypes could be accurately defined by specific gene expression signatures (involving six to seven genes) in the blood.
Epigenetic mechanisms in post-sepsis syndrome and long COVID
These observed gene expressions captured by transcriptomic studies after sepsis may be a consequence of long-term changes induced by epigenetics. Epigenetic modifications are often persistent regulatory mechanisms that control gene expression but are not related to changes in DNA sequence and include processes such as DNA methylation and histone modifications. Epigenetic modifications are not genetically determined per se but are thought to be environmentally induced in response to nutrients, pollution, toxicants, disease progression, aging, and inflammation. The long duration of sepsis in its first manifestation in many patients, as well as the longer-term persistence of its consequences, have led researchers to hypothesize that sepsis patients undergo epigenetic processes that influence post-sepsis events. Binnie et al. (107) recently reviewed many studies confirming this and revealing that epigenetic mechanisms are probably central to the pathogenesis of sepsis, influencing the early inflammatory response, sepsis-associated immune suppression, and lung injury. Epigenetic changes often influence transcription of the genes with which they are associated, enabling the possibility of mapping such changes on to protein interaction networks. Using this approach (64), 34 genes were recently shown to overlap between persistent genes in non-survivors, differentially methylated genes of sepsis patients identified in a previous study (108), and those of COVID-19 patients (109). These overlapping genes were mostly immune-related genes, including CD177, CD3D, and S100P. This finding suggests that epigenetic switches, known to be involved in sepsis (110), might be responsible for persistent dysregulation of genes. In other recent studies, epigenetic alterations in TMPRSS2, interferon-related genes, FURIN, and ADAM17 genes were important determinants of COVID-19 severity (111).
Such studies should be extended to post-sepsis/long COVID syndromes to identify potential epigenetic modification regulating the enduring immune dysfunction that appears to accompany these syndromes. Critically, a deeper understanding of epigenetic regulation has the potential to generate biomarkers for the aforementioned stages of sepsis and new or repurposed therapeutic options. Intriguing mouse studies have demonstrated that the DNA methyltransferase inhibitors azacitidine (112) and decitabine (112, 113), as well as the histone deacetylase inhibitors trichostatin A and sodium butyrate (114), can reverse some of the genotypic and phenotypic features of sepsis, indicating that epigenetic therapies may have potential in the treatment of sepsis, its associated organ failures, and possibly its downstream sequelae.
Sepsis caused most pandemic deaths and requires continued research
Early in the COVID-19 pandemic, it was recognized that most patients hospitalized for severe COVID-19 disease, and especially those who required respiratory support or died, had viral sepsis. This has been supported by studies of patients’ clinical records and systems immunology showing converging immune mechanisms over time between COVID-19 sepsis and non-COVID-19 (e.g., bacterial) sepsis (8). Consistent with this, both sepsis types are associated with signatures of cellular reprogramming, organ dysfunction, mortality, and endotypes (63). Thus, it could be argued that although efforts to identify the SARS-CoV-2 virus were critical to developing prophylactic measures (e.g., vaccines), the most severe manifestations of this disease were due to sepsis. Indeed, while the SARS-CoV-2 virus caused nearly 700 million infections, it was the 6.9 million deaths, primarily due to sepsis, that drove the strong public health responses, including isolation measures, and the consequent reduction in commerce.
Early suggestions that patients were acquiring secondary bacterial infections that were driving sepsis did not hold up to scrutiny (115). Rather, the virus itself seems to drive sepsis in a subset of individuals. We do not know the mechanisms behind virally driven sepsis. However, since conventional culture methods only identify bacteria as the cause of ~50% of sepsis cases at most (51), viral sepsis could be much more prevalent than previously supposed, as implied by metagenomic sequencing (116). Notably, the IFN endotype of sepsis was more strongly associated with COVID-19 sepsis than all-cause sepsis (63).
It is worth asking, then, how common sepsis is as a driver in pandemics generally? Influenza A virus caused three pandemics in the 20th century due to the Spanish flu (H1N1; 1918–20), Asian flu (H2N2; 1957–9), and Hong Kong flu (H3N2; 1968–9), killing many millions of people. It has been suggested that severe sepsis occurred in the more critically ill patients and was caused either directly by the influenza viruses or indirectly by secondary bacterial infections induced by weakened defenses due to influenza (117, 118). Indeed, influenza, an endemic disease that is a substantial cause of mortality, is associated with acute respiratory distress syndrome (ARDS), a manifestation that often accompanies sepsis (119), while post-acute complications of influenza resemble post-sepsis syndrome and long COVID (120). Further back in time, the bubonic plague killed 12–15 million people from 1855 to 1960 and even more during the Middle Ages. The septicemic form of this disease (typified by systemic infection caused by bacteremia) as well as its pneumonic form have a case-fatality ratio of 30–100% if left untreated. Thus, there is a strong likelihood that bubonic plague mortality is again due to sepsis.
Overall, we conclude that, regardless of the causative agent, sepsis has been a major factor in past and recent pandemics and will likely be a strong factor in the most severe forms of disease in future pandemics. Thus, novel diagnostic tools and drugs are urgently needed, not only to reduce the current, enormous global burden of sepsis, but also to avoid the medical calamity seen in the COVID-19 pandemic.
Such “pathogen-agnostic” measures could efficiently treat severe disease outcomes even before a pandemic pathogen has been identified and pathogen-specific diagnostics and therapies have been developed. This transformational concept should be at the heart of plans to mitigate against future pandemics; it should be a priority for the research community, healthcare systems, and ultimately governments when providing funding support. In addition to these insights, collaborations between scientists, physicians, and other healthcare providers, and most importantly patients and their families, should be initiated in order to deliver stakeholder-focused solutions. Pandemic preparedness is at the heart of many broad plans, including from the European Union (121), World Bank (122), and WHO (123), but concerningly none of these mention sepsis. Given the current limitations involved in studying sepsis (heterogeneity and the consequent requirement for precision approaches, lack of suitable animal models, and modest investment), it is specifically recommended that relevant stakeholders should come together (possibly under the banner of organizations such as the Surviving Sepsis campaign or the Global Sepsis Alliance) to establish an aggressive and fundable program to address these deficiencies. Recently, the 2030 Global Agenda for Sepsis (124) was launched as a multistakeholder strategic vision aimed at alleviating the significant human, societal, healthcare, and economic burden of sepsis. This multi-year strategic vision aims to reduce the global incidence of sepsis by at least 25%, improve survival rates by over 20%, and reduce the median costs per sepsis patient by 20% from 2017–2020 baselines. Its five strategic pillars include research and innovation specifically mentioning precision medicine to address heterogeneity, together with political leadership and multilateral cooperation, health system readiness, a “whole-of-society response”, and sepsis in pandemics and other emergencies.
Given the unique and data-driven insights offered by systems immunology, the approaches described here should form the foundation for research and innovation.
Conclusion
Sepsis is defined as a dysregulated, life-threatening response to infection that leads to (multi)organ dysfunction and failure. It has been poorly understood but causes 19.7% of all deaths and, more recently, caused almost all deaths during the COVID-19 pandemic. Systems immunology studies involving bioinformatic analysis (AI, network biology, clustering, etc.) of gene expression and other omics datasets derived from patients’ cells are starting to deliver new early diagnostics, clarify this complex disease as a series of mechanistically distinct endotypes, and provide a more profound understanding of the dynamics of sepsis both during and after hospitalization (i.e., post-sepsis syndrome and long COVID). New biomarkers and targeted treatments for sepsis are not only vital to reduce the current global toll of sepsis but also to better protect our populations against future pandemics.
A recent evaluation of an ML model of treatment outcomes in another complex disorder, schizophrenia, concluded that clinical prediction models in this setting are highly context-dependent and may have limited generalizability beyond the datasets on which they are based (125). This is likely also true for sepsis, and we propose here that these issues can be overcome in sepsis research by (i) capturing important heterogeneity through phenotyping, (ii) identifying trial/study-level characteristics that relate to patient outcomes (addressable using ML or Bayesian approaches), and (iii) utilizing longitudinal validation methods that reduce challenges related to heterogeneity and context while identifying temporal trends. Importantly, the aforementioned schizophrenia study (125) was limited in that it did not consider multiomics approaches. We submit that evidence of the robust performance of sepsis models developed using multiple omics and systems immunology methods strongly supports their generalizability and potential to drive substantial and urgent improvements in the care of this globally concerning condition.
Acknowledgments
The authors thank Dr. Peter Zhang (University of British Columbia, Vancouver, BC, Canada) for providing assistance in the analysis presented in Table 1.
Statements
Author contributions
RH: Writing – review & editing, Writing – original draft, Visualization, Validation, Supervision, Methodology, Funding acquisition, Conceptualization. AA: Visualization, Validation, Methodology, Formal Analysis, Funding acquisition, Data curation, Conceptualization, Writing – review & editing, Writing – original draft, Bioinformatic data analysis. CS: Writing – review & editing, Writing – original draft, Funding acquisition, Providing clinical perspective, Conceptualization. AL: Writing – review & editing, Writing – original draft, Methodology, Funding acquisition, Formal data analysis, Data curation, Conceptualization.
Data availability statement
The original contributions presented in the study are included in the article/supplementary material. Further inquiries can be directed to the corresponding author.
Ethics statement
The previous studies involving humans cited within this manuscript were approved by local ethics committees as described in the cited references. For the authors’ own cited research, the Clinical Research Ethics Board of the University of British Columbia provided ethics approval for all sequencing and bioinformatics studies, carried out in a manner blinded to patient identity (approval number H17-01208). These previous studies were conducted in accordance with the local legislation and institutional requirements. Written informed consent for participation in these previous studies was required from the participants or the participants’ legal guardians/next of kin in accordance with the national legislation and institutional requirements.
Funding
The author(s) declare that financial support was received for the research, authorship, and/or publication of this article. REWH, AHYL and CS wish to acknowledge separate funding from the Canadian Institutes for Health Research for their own sepsis and Systems Immunology research. REWH currently holds a University of British Columbia Killam Professorship. AA is funded by a Canada Graduate Scholarships Doctoral (CGS-D) program. The funders were not involved in the study design, collection, analysis, interpretation of data, the writing of this article or the decision to submit it for publication.
Conflict of interest
REWH is the inventor on patent filings covering sepsis endotypes (WO2022246553A1) and early sepsis diagnostics (e.g., EP3117030A4), corresponding to the peer-reviewed research findings published in references (35, 55). These technologies have been licensed to Sepset Biosciences Inc., which is partly owned by Asep Medical Inc., and REWH is a shareholder and CEO of Asep.
The remaining authors declare that the research was conducted in the absence of any commercial or financial relationships that could be construed as a potential conflict of interest.
CS declared that they were an editorial board member of Frontiers, at the time of submission. This had no impact on the peer review process and the final decision.
Publisher’s note
All claims expressed in this article are solely those of the authors and do not necessarily represent those of their affiliated organizations, or those of the publisher, the editors and the reviewers. Any product that may be evaluated in this article, or claim that may be made by its manufacturer, is not guaranteed or endorsed by the publisher.
References
1. Singer M, Deutschman CS, Seymour CW, Shankar-Hari M, Annane D, Bauer M, et al. The third international consensus definitions for sepsis and septic shock (Sepsis-3). JAMA (2016) 315(8):801–10. doi: 10.1001/jama.2016.0287
2. Rudd KE, Johnson SC, Agesa KM, Shackelford KA, Tsoi D, Kievlan DR, et al. Global, regional, and national sepsis incidence and mortality, 1990–2017: analysis for the global burden of disease study. Lancet (2020) 395(10219):200–11. doi: 10.1016/S0140-6736(19)32989-7
3. Worldometer. COVID-19 Coronavirus Pandemic [online] (2024). Available at: https://www.worldometers.info/coronavirus/
4. Wang H, Paulson KR, Pease SA, Watson S, Comfort H, Zheng P, et al. Estimating excess mortality due to the COVID-19 pandemic: a systematic analysis of COVID-19-related mortality, 2020–21. Lancet (2022) 399(10334):1513–36. doi: 10.1016/S0140-6736(21)02796-3
5. Vincent JL. COVID-19: it’s all about sepsis. Future Microbiol (2021) 16(3):131–3. doi: 10.2217/fmb-2020-0312
6. Gu X, Zhou F, Wang Y, Fan G, Cao B. Respiratory viral sepsis: epidemiology, pathophysiology, diagnosis and treatment. Eur Respir Rev (2020) 29(157):200038. doi: 10.1183/16000617.0038-2020
7. Olwal CO, Nganyewo NN, Tapela K, Djomkam Zune AL, Owoicho O, Bediako Y, et al. Parallels in sepsis and COVID-19 conditions: implications for managing severe COVID-19. Front Immunol (2021) 12:602848. doi: 10.3389/fimmu.2021.602848
8. An AY, Baghela A, Zhang P, Falsafi R, Lee AH, Trahtemberg U, et al. Severe COVID-19 and non-COVID-19 severe sepsis converge transcriptionally after a week in the intensive care unit, indicating common disease mechanisms. Front Immunol (2023) 14:1167917. doi: 10.3389/fimmu.2023.1167917
9. Hotchkiss RS, Moldawer LL, Opal SM, Reinhart K, Turnbull IR, Vincent JL. Sepsis and septic shock. Nat Rev Dis Primers (2016) 2(1):16045. doi: 10.1038/nrdp.2016.45
10. van der Slikke EC, An AY, Hancock REW, Bouma HR. Exploring the pathophysiology of post-sepsis syndrome to identify therapeutic opportunities. eBioMedicine (2020) 61:103044. doi: 10.1016/j.ebiom.2020.103044
11. Herminghaus A, Osuchowski MF. How sepsis parallels and differs from COVID-19. eBioMedicine (2022) 86:104355. doi: 10.1016/j.ebiom.2022.104355
12. Kalil AC, Opal SM. Sepsis in the severely immunocompromised patient. Curr Infect Dis Rep (2015) 17(6):487. doi: 10.1007/s11908-015-0487-4
13. Martin GS, Mannino DM, Moss M. The effect of age on the development and outcome of adult sepsis. Crit Care Med (2006) 34(1):15–21. doi: 10.1097/01.ccm.0000194535.82812.ba
14. Luijks ECN, Slikke EC, van Zanten ARH, Ter Maaten JC, Postma MJ, Hilderink HBM, et al. Societal costs of sepsis in the Netherlands. Crit Care (2024) 28(1):29. doi: 10.1186/s13054-024-04816-3
15. World Health Organization. The top 10 causes of death [online] (2024). Available at: https://www.who.int/news-room/fact-sheets/detail/the-top-10-causes-of-death
16. United States Centers for Disease Control and Prevention National Center for Health Statistics. Leading causes of death [online] (2024). Available at: https://www.cdc.gov/nchs/fastats/leading-causes-of-death.htm
17. Esper AM, Moss M, Lewis CA, Nisbet R, Mannino DM, Martin GS. The role of infection and comorbidity: factors that influence disparities in sepsis. Crit Care Med (2006) 34(10):2576–82. doi: 10.1097/01.CCM.0000239114.50519.0E
18. Scicluna BP, Klein Klouwenberg PMC, van Vught LA, Wiewel MA, Ong DSY, Zwinderman AH, et al. A molecular biomarker to diagnose community-acquired pneumonia on intensive care unit admission. Am J Respir Crit Care Med (2015) 192(7):826–35. doi: 10.1164/rccm.201502-0355OC
19. Leligdowicz A, Matthay MA. Heterogeneity in sepsis: new biological evidence with clinical applications. Crit Care (2019) 23(1):80. doi: 10.1186/s13054-019-2372-2
20. Hotchkiss RS, Monneret G, Payen D. Immunosuppression in sepsis: a novel understanding of the disorder and a new therapeutic approach. Lancet Infect Dis (2013) 13(3):260–8. doi: 10.1016/S1473-3099(13)70001-X
21. Sartelli M, Kluger Y, Ansaloni L, Hardcastle TC, Rello J, Watkins RR, et al. Raising concerns about the Sepsis-3 definitions. World J Emerg Surg (2018) 13:6. doi: 10.1186/s13017-018-0165-6
22. Kumar A, Roberts D, Wood KE, Light B, Parrillo JE, Sharma S, et al. Duration of hypotension before initiation of effective antimicrobial therapy is the critical determinant of survival in human septic shock. Crit Care Med (2006) 34(6):1589–96. doi: 10.1097/01.CCM.0000217961.75225.E9
23. Siewers K, Abdullah SMOB, Sørensen RH, Nielsen FE. Time to administration of antibiotics and mortality in sepsis. J Am Coll Emerg Physicians Open (2021) 2(3):e12435. doi: 10.1002/emp2.12435
24. InnateDB. Gene Lists (2014). Available at: https://www.innatedb.com/redirect.do?go=resourcesGeneLists
25. Zhou G, Soufan O, Ewald J, Hancock REW, Basu N, Xia J. NetworkAnalyst 3.0: a visual analytics platform for comprehensive gene expression profiling and meta-analysis. Nucleic Acids Res (2019) 47(W1):W234–41. doi: 10.1093/nar/gkz240
26. Lee AH, Shannon CP, Amenyogbe N, Bennike TB, Diray-Arce J, Idoko OT, et al. Dynamic molecular changes during the first week of human life follow a robust developmental trajectory. Nat Commun (2019) 10(1):1092. doi: 10.1038/s41467-019-08794-x
27. Subramanian N, Torabi-Parizi P, Gottschalk RA, Germain RN, Dutta B. Network representations of immune system complexity. Wiley Interdiscip Rev Syst Biol Med (2015) 7(1):13–38. doi: 10.1002/wsbm.1288
29. Iwasaki A, Medzhitov R. Control of adaptive immunity by the innate immune system. Nat Immunol (2015) 16(4):343–53. doi: 10.1038/ni.3123
30. van der Poll T, Shankar-Hari M, Wiersinga WJ. The immunology of sepsis. Immunity (2021) 54(11):2450–64. doi: 10.1016/j.immuni.2021.10.012
31. Maslove DM, Tang BM, McLean AS. Identification of sepsis subtypes in critically ill adults using gene expression profiling. Crit Care (2012) 16(5):R183. doi: 10.1186/cc11667
32. Davenport EE, Burnham KL, Radhakrishnan J, Humburg P, Hutton P, Mills TC, et al. Genomic landscape of the individual host response and outcomes in sepsis: a prospective cohort study. Lancet Respir Med (2016) 4(4):259–71. doi: 10.1016/S2213-2600(16)00046-1
33. Scicluna BP, van Vught LA, Zwinderman AH, Wiewel MA, Davenport EE, Burnham KL, et al. Classification of patients with sepsis according to blood genomic endotype: a prospective cohort study. Lancet Respir Med (2017) 5(10):816–26. doi: 10.1016/S2213-2600(17)30294-1
34. Burnham KL, Davenport EE, Radhakrishnan J, Humburg P, Gordon AC, Hutton P, et al. Shared and distinct aspects of the sepsis transcriptomic response to fecal peritonitis and pneumonia. Am J Respir Crit Care Med (2017) 196(3):328–39. doi: 10.1164/rccm.201608-1685OC
35. Baghela A, Pena OM, Lee AH, Baquir B, Falsafi R, An A, et al. Predicting sepsis severity at first clinical presentation: the role of endotypes and mechanistic signatures. eBioMedicine (2022) 75:103776. doi: 10.1016/j.ebiom.2021.103776
36. Forlin R, James A, Brodin P. Making human immune systems more interpretable through systems immunology. Trends Immunol (2023) 44(8):577–84. doi: 10.1016/j.it.2023.06.005
37. Auslander N, Gussow AB, Koonin EV. Incorporating machine learning into established bioinformatics frameworks. Int J Mol Sci (2021) 22(6):2903. doi: 10.3390/ijms22062903
38. Lee BD, Gitter A, Greene CS, Raschka S, Maguire F, Titus AJ, et al. Ten quick tips for deep learning in biology. PloS Comput Biol (2022) 18(3):e1009803. doi: 10.1371/journal.pcbi.1009803
39. Breuer K, Foroushani AK, Laird MR, Chen C, Sribnaia A, Lo R, et al. InnateDB: systems biology of innate immunity and beyond - recent updates and continuing curation. Nucleic Acids Res (2013) 41(D1):D1228–33. Available at: www.innateDB.ca
40. Shannon P, Markiel A, Ozier O, Baliga NS, Wang JT, Ramage D, et al. Cytoscape: a software environment for integrated models of biomolecular interaction networks. Genome Res (2003) 13(11):2498–504. doi: 10.1101/gr.1239303
41. Vodovotz Y. Towards systems immunology of critical illness at scale: from single cell ‘omics to digital twins. Trends Immunol (2023) 44(5):345–55. doi: 10.1016/j.it.2023.03.004
42. Seymour CW, Kennedy JN, Wang S, Chang CH, Elliott CF, Xu Z, et al. Derivation, validation, and potential treatment implications of novel clinical phenotypes for sepsis. JAMA (2019) 321(20):2003–17. doi: 10.1001/jama.2019.5791
43. Maslove DM, Tang B, Shankar-Hari M, Lawler PR, Angus DC, Baillie JK, et al. Redefining critical illness. Nat Med (2022) 28(6):1141–8. doi: 10.1038/s41591-022-01843-x
44. Chen B, Khodadoust MS, Liu CL, Newman AM, Alizadeh AA. Profiling tumor infiltrating immune cells with CIBERSORT. In: von Stechow L, editor. Cancer systems biology. New York: Humana Press (2018). 243–59. doi: 10.1007/978-1-4939-7493-1_12
45. Su Y, Yuan D, Chen DG, Ng RH, Wang K, Choi J, et al. Multiple early factors anticipate post-acute COVID-19 sequelae. Cell (2022) 185(5):881–895.e20. doi: 10.1016/j.cell.2022.01.014
46. Tang F, Yuan H, Li X, Qiao L. Effect of delayed antibiotic use on mortality outcomes in patients with sepsis or septic shock: a systematic review and meta-analysis. Int Immunopharmacol (2024) 129:111616. doi: 10.1016/j.intimp.2024.111616
47. Vincent J-L. The clinical challenge of sepsis identification and monitoring. PloS Med (2016) 13(5):e1002022. doi: 10.1371/journal.pmed.1002022
48. Lambden S, Laterre PF, Levy MM, Francois B. The SOFA score-development, utility and challenges of accurate assessment in clinical trials. Crit Care (2019) 23(1):374. doi: 10.1186/s13054-019-2663-7
49. Martin GS. Sepsis, severe sepsis and septic shock: changes in incidence, pathogens and outcomes. Expert Rev Anti Infect Ther (2012) 10(6):701–6. doi: 10.1586/eri.12.50
50. Wang C, Xu R, Zeng Y, Zhao Y, Hu X. A comparison of qSOFA, SIRS and NEWS in predicting the accuracy of mortality in patients with suspected sepsis: a meta-analysis. PloS One (2022) 17(4):e0266755. doi: 10.1371/journal.pone.0266755
51. Gupta S, Sakhuja A, Kumar G, McGrath E, Nanchal RS, Kashani KB. Culture-negative severe sepsis: nationwide trends and outcomes. Chest (2016) 150(6):1251–9. doi: 10.1016/j.chest.2016.08.1460
52. Tsalik EL, Jones D, Nicholson B, Waring L, Liesenfeld O, Park LP, et al. Multiplex PCR to diagnose bloodstream infections in patients admitted from the emergency department with sepsis. J Clin Microbiol (2010) 48(1):26–33. doi: 10.1128/JCM.01447-09
53. Kim MH, Choi JH. An update on sepsis biomarkers. Infect Chemother (2020) 52(1):1–18. doi: 10.3947/ic.2020.52.1.1
54. van Oers JAH, de Jong E, Kemperman H, Girbes ARJ, de Lange DW. Diagnostic accuracy of procalcitonin and C-reactive protein is insufficient to predict proven infection: a retrospective cohort study in critically ill patients fulfilling the Sepsis-3 criteria. J Appl Lab Med (2020) 5(1):62–72. doi: 10.1373/jalm.2019.029777
55. Pena OM, Hancock DG, Lyle NH, Linder A, Russell JA, Xia J, et al. An endotoxin tolerance signature predicts sepsis and organ dysfunction at initial clinical presentation. EBiomedicine (2014) 1(1):64–71. doi: 10.1016/j.ebiom.2014.10.003
56. Ram-Mohan N, Rogers AJ, Blish CA, Nadeau KC, Zudock EJ, Kim D, et al. Using a 29-mRNA host response classifier to detect bacterial coinfections and predict outcomes in COVID-19 patients presenting to the emergency department. Microbiol Spectr (2022) 10(6):e0230522. doi: 10.1128/spectrum.02305-22
57. Sweeney TE, Shidham A, Wong HR, Khatri P. A comprehensive time-course-based multicohort analysis of sepsis and sterile inflammation reveals a robust diagnostic gene set. Sci Transl Med (2015) 7(287):287ra71. doi: 10.1126/scitranslmed.aaa5993
58. Reyes M, Filbin MR, Bhattacharyya RP, Billman K, Eisenhaure T, Hung DT, et al. An immune-cell signature of bacterial sepsis. Nat Med (2020) 26(3):333–40. doi: 10.1038/s41591-020-0752-4
59. Sweeney TE, Khatri P. Comprehensive validation of the FAIM3:PLAC8 ratio in time-matched public gene expression data. Am J Respir Crit Care Med (2015) 192(10):1260–1. doi: 10.1164/rccm.201507-1321LE
60. Lukaszewski RA, Jones HE, Gersuk VH, Russell P, Simpson A, Brealey D, et al. Presymptomatic diagnosis of postoperative infection and sepsis using gene expression signatures. Intensive Care Med (2022) 48(9):1133–43. doi: 10.1007/s00134-022-06769-z
61. Balk R, Esper AM, Martin GS, Miller RR 3rd, Lopansri BK, Burke JP, et al. Validation of SeptiCyte RAPID to discriminate sepsis from non-infectious systemic inflammation. J Clin Med (2024) 13(5):1194. doi: 10.3390/jcm13051194
62. Tanak AS, Sardesai A, Muthukumar S, Prasad S. Simultaneous detection of sepsis host response biomarkers in whole blood using electrochemical biosensor. Bioeng Transl Med (2022) 7(3):e10310. doi: 10.1002/btm2.10310
63. Baghela A, An A, Zhang P, Acton E, Gauthier J, Brunet-Ratnasingham E, et al. Predicting severity in COVID-19 disease using sepsis blood gene expression signatures. Sci Rep (2023) 13(1):1247. doi: 10.1038/s41598-023-28259-y
64. An AY, Baghela A, Zhang P, Falsafi R, Lee AH, Trahtemberg U, et al. Persistence is key: unresolved immune dysfunction is lethal in both COVID-19 and non-COVID-19 sepsis. Front Immunol (2023) 14:1254873. doi: 10.3389/fimmu.2023.1254873
65. Kalantar KL, Neyton L, Abdelghany M, Mick E, Jauregui A, Caldera S, et al. Integrated host-microbe plasma metagenomics for sepsis diagnosis in a prospective cohort of critically ill adults. Nat Microbiol (2022) 7(11):1805–16. doi: 10.1038/s41564-022-01237-2
66. McClain MT, Constantine FJ, Henao R, Liu Y, Tsalik EL, Burke TW, et al. Dysregulated transcriptional responses to SARS-CoV-2 in the periphery. Nat Commun (2021) 12(1):1079. doi: 10.1038/s41467-021-21289-y
67. Arunachalam PS, Wimmers F, Mok CKP, Perera RAPM, Scott M, Hagan T, et al. Systems biological assessment of immunity to mild versus severe COVID-19 infection in humans. Science (2020) 369(6508):1210–20. doi: 10.1126/science.abc6261
68. Argmann C, Hou R, Ungaro RC, Irizar H, Al-Taie Z, Huang R, et al. Biopsy and blood-based molecular biomarker of inflammation in IBD. Gut (2023) 72(7):1271–87. doi: 10.1136/gutjnl-2021-326451
69. McCaffrey TA, Toma I, Yang Z, Katz R, Reiner J, Mazhari R, et al. RNA sequencing of blood in coronary artery disease: involvement of regulatory T cell imbalance. BMC Med Genomics (2021) 14(1):216. doi: 10.1186/s12920-021-01062-2
70. Cavaillon J-M, Adib-Conquy M. Bench-to-bedside review: endotoxin tolerance as a model of leukocyte reprogramming in sepsis. Crit Care (2006) 10(5):233. doi: 10.1186/cc5055
71. Pena OM, Pistolic J, Raj D, Fjell CD, Hancock REW. Endotoxin tolerance represents a distinctive state of alternative polarization (M2) in human mononuclear cells. J Immunol (2011) 186(12):7243–54. doi: 10.4049/jimmunol.1001952
72. Shalova IN, Lim JY, Chittezhath M, Zinkernagel AS, Beasley F, Hernández-Jiménez E, et al. Human monocytes undergo functional re-programming during sepsis mediated by hypoxia-inducible factor-1α. Immunity (2015) 42(3):484–98. doi: 10.1016/j.immuni.2015.02.001
73. Poujol F, Monneret G, Pachot A, Textoris J, Venet F. Altered T lymphocyte proliferation upon lipopolysaccharide challenge ex vivo. PloS One (2015) 10(12):e0144375. doi: 10.1371/journal.pone.0144375
74. Liu VX, Escobar GJ, Chaudhary R, Prescott HC. Healthcare utilization and infection in the week prior to sepsis hospitalization. Crit Care Med (2018) 46(4):513–6. doi: 10.1097/CCM.0000000000002960
75. Knoll R, Helbig ET, Dahm K, Bolaji O, Hamm F, Dietrich O, et al. The life-saving benefit of dexamethasone in severe COVID-19 is linked to a reversal of monocyte dysregulation. Cell (2024) 187(16):4318–4335.e20. doi: 10.1016/j.cell.2024.06.014
76. An AY, Acton E, Idoko OT, Shannon CP, Blimkie TM, Falsafi R, et al. Predictive gene expression signature diagnoses neonatal sepsis before clinical presentation. EBiomedicine (2024). 105411. doi: 10.1016/j.ebiom.2024.105411
77. Marshall JC. Why have clinical trials in sepsis failed? Trends Mol Med (2014) 20(4):195–203. doi: 10.1016/j.molmed.2014.01.007
78. Bone RC. Sir isaac newton, sepsis, SIRS, and CARS. Crit Care Med (1996) 24(7):1125–8. doi: 10.1097/00003246-199607000-00010
79. Bryn T, Yaqub S, Mahic M, Henjum K, Aandahl EM, Taskén K. LPS-activated monocytes suppress T-cell immune responses and induce FOXP3+ T cells through a COX-2–PGE2-dependent mechanism. Int Immunol (2008) 20(2):235–45. doi: 10.1093/intimm/dxm134
80. Jensen IJ, Sjaastad FV, Griffith TS, Badovinac VP. Sepsis-induced T cell immunoparalysis: the ins and outs of impaired T cell immunity. J Immunol (2018) 200(5):1543–53. doi: 10.4049/jimmunol.1701618
81. Mathias B, Delmas AL, Ozrazgat-Baslanti T, Vanzant EL, Szpila BE, Mohr AM, et al. Human myeloid-derived suppressor cells are associated with chronic immune suppression after severe sepsis/septic shock. Ann Surg (2017) 265(4):827–34. doi: 10.1097/SLA.0000000000001783
82. Shen X-F, Cao K, Jiang J-P, Guan W-X, Du J-F. Neutrophil dysregulation during sepsis: an overview and update. J Cell Mol Med (2017) 21(9):1687–97. doi: 10.1111/jcmm.13112
83. Agache I, Akdis CA. Precision medicine and phenotypes, endotypes, genotypes, regiotypes, and theratypes of allergic diseases. J Clin Invest (2019) 129(4):1493–503. doi: 10.1172/JCI124611
84. Schulte-Schrepping J, Reusch N, Paclik D, Baßler K, Schlickeiser S, Zhang B, et al. Severe COVID-19 is marked by a dysregulated myeloid cell compartment. Cell (2020) 182(6):1419–1440.e23. doi: 10.1016/j.cell.2020.08.001
85. RECOVERY Collaborative Group, Horby P, Lim WS, Emberson JR, Mafham M, Bell JL, et al. Dexamethasone in hospitalized patients with Covid-19. N Engl J Med (2021) 384(8):693–704. doi: 10.1056/NEJMoa2021436
86. Sweeney TE, Azad TD, Donato M, Haynes WA, Perumal TM, Henao R, et al. Unsupervised analysis of transcriptomics in bacterial sepsis across multiple datasets reveals three robust clusters. Crit Care Med (2018) 46(6):915–25. doi: 10.1097/CCM.0000000000003084
87. Kwok AJ, Allcock A, Ferreira RC, Cano-Gamez E, Smee M, Burnham KL, et al. Neutrophils and emergency granulopoiesis drive immune suppression and an extreme response endotype during sepsis. Nat Immunol (2023) 24(5):767–79. doi: 10.1038/s41590-023-01490-5
88. Yoo M, Shin J, Kim J, Ryall KA, Lee K, Lee S, et al. DSigDB: drug signatures database for gene set analysis. Bioinformatics (2015) 31(18):3069–71. doi: 10.1093/bioinformatics/btv313
89. Son JY, Shin S, Choi YJ. New evidence of potential benefits of dexamethasone and added on therapy of fludrocortisone on clinical outcomes of corticosteroid in sepsis patients: a systematic review and meta-analysis. J Pers Med (2021) 11(6):544. doi: 10.3390/jpm11060544
90. Rubio I, Osuchowski MF, Shankar-Hari M, Skirecki T, Winkler MS, Lachmann G, et al. Current gaps in sepsis immunology: new opportunities for translational research. Lancet Infect Dis (2019) 19(12):e422–36. doi: 10.1016/S1473-3099(19)30567-5
91. Sweeney TE, Wong HR. Risk stratification and prognosis in sepsis: what have we learned from microarrays? Clin Chest Med (2016) 37(2):209–18. doi: 10.1016/j.ccm.2016.01.003
92. Delano MJ, Ward PA. The immune system’s role in sepsis progression, resolution, and long-term outcome. Immunol Rev (2016) 274(1):330–53. doi: 10.1111/imr.12499
93. Maslove DM, Wong HR. Gene expression profiling in sepsis: timing, tissue, and translational considerations. Trends Mol Med (2014) 20(4):204–13. doi: 10.1016/j.molmed.2014.01.006
94. Yan Q, Li P, Ye X, Huang X, Feng B, Ji T, et al. Longitudinal peripheral blood transcriptional analysis reveals molecular signatures of disease progression in COVID-19 patients. J Immunol (2021) 206(9):2146–59. doi: 10.4049/jimmunol.2001325
95. Zheng H-Y, Xu M, Yang C-X, Tian R-R, Zhang M, Li J-J, et al. Longitudinal transcriptome analyses show robust T cell immunity during recovery from COVID-19. Signal Transduct Target Ther (2020) 5(1):294. doi: 10.1038/s41392-020-00457-4
96. Bernardes JP, Mishra N, Tran F, Bahmer T, Best L, Blase JI, et al. Longitudinal multi-omics analyses identify responses of megakaryocytes, erythroid cells, and plasmablasts as hallmarks of severe COVID-19. Immunity (2020) 53(6):1296–1314.e9. doi: 10.1016/j.immuni.2020.11.017
97. An AY, Baghela A, Zhang P, Blimkie T, Gauthier J, Kaufmann DE, et al. Dynamic gene expression analysis reveals distinct severity phases of immune dysregulation in COVID-19. BioRxIV [preprint] (2023). doi: 10.1101/2023.11.04.565404
98. Bailey CJ, Gwilt M. Diabetes, metformin and the clinical course of COVID-19: outcomes, mechanisms and suggestions on the therapeutic use of metformin. Front Pharmacol (2022) 13:784459. doi: 10.3389/fphar.2022.784459
99. Prescott HC, Langa KM, Iwashyna TJ. Readmission diagnoses after hospitalization for severe sepsis and other acute medical conditions. JAMA (2015) 313(10):1055–7. doi: 10.1001/jama.2015.1410
100. Prescott HC, Angus DC. Enhancing recovery from sepsis: a review. JAMA (2018) 319(1):62–75. doi: 10.1001/jama.2017.17687
101. Davis HE, McCorkell L, Vogel JM, Topol EJ. Long COVID: major findings, mechanisms and recommendations. Nat Rev Microbiol (2023) 21(3):133–46. doi: 10.1038/s41579-022-00846-2
102. Mayr FB, Talisa VB, Balakumar V, Chang CH, Fine M, Yende S. Proportion and cost of unplanned 30-day readmissions after sepsis compared with other medical conditions. JAMA (2017) 317(5):530–1. doi: 10.1001/jama.2016.20468
103. Soussi S, Sharma D, Jüni P, Lebovic G, Brochard L, Marshall JC, et al. Identifying clinical subtypes in sepsis-survivors with different one-year outcomes: a secondary latent class analysis of the FROG-ICU cohort. Crit Care (2022) 26(1):114. doi: 10.1186/s13054-022-03972-8
104. Ryan FJ, Hope CM, Masavuli MG, Lynn MA, Mekonnen ZA, Yeow AEL, et al. Long-term perturbation of the peripheral immune system months after SARS-CoV-2 infection. BMC Med (2022) 20(1):26. doi: 10.1186/s12916-021-02228-6
105. Thompson RC, Simons NW, Wilkins L, Cheng E, Del Valle DM, Hoffman GE, et al. Molecular states during acute COVID-19 reveal distinct etiologies of long-term sequelae. Nat Med (2022) 29(1):236–46. doi: 10.1038/s41591-022-02107-4
106. An AY, Baghela A, Zhang PGY, Blimkie TM, Gauthier J, Kaufmann DE, et al. Post-COVID symptoms are associated with endotypes reflecting poor inflammatory and hemostatic modulation. Front Immunol (2023) 14:1243689. doi: 10.3389/fimmu.2023.1243689
107. Binnie A, Tsang JLY, Hu P, Carrasqueiro G, Castelo-Branco P, Dos Santos CC. Epigenetics of sepsis. Crit Care Med (2020) 48(5):745–56. doi: 10.1097/CCM.0000000000004247
108. Binnie A, Walsh CJ, Hu P, Dwivedi DJ, Fox-Robichaud A, Liaw PC, et al. Epigenetic profiling in severe sepsis: a pilot study of DNA methylation profiles in critical illness. Crit Care Med (2020) 48(2):142–50. doi: 10.1097/CCM.0000000000004097
109. Castro de Moura M, Davalos V, Planas-Serra L, Alvarez-Errico D, Arribas C, Ruiz M, et al. Epigenome-wide association study of COVID-19 severity with respiratory failure. EBiomedicine (2021) 66:103339. doi: 10.1016/j.ebiom.2021.103339
110. Wu D, Shi Y, Zhang H, Miao C. Epigenetic mechanisms of immune remodeling in sepsis: targeting histone modification. Cell Death Dis (2023) 14(2):112. doi: 10.1038/s41419-023-05656-9
111. Ozturkler Z, Kalkan R. A new perspective of COVID-19 infection: an epigenetics point of view. Glob Med Genet (2022) 9(1):4–6. doi: 10.1055/s-0041-1736565
112. Huang X, Kong G, Li Y, Zhu W, Xu H, Zhang X, et al. Decitabine and 5-azacitidine both alleviate LPS induced ARDS through anti-inflammatory/antioxidant activity and protection of glycocalyx and inhibition of MAPK pathways in mice. BioMed Pharmacother (2016) 84:447–53. doi: 10.1016/j.biopha.2016.09.072
113. Singer BD, Mock JR, Aggarwal NR, Garibaldi BT, Sidhaye VK, Florez MA, et al. Regulatory T cell DNA methyltransferase inhibition accelerates resolution of lung inflammation. Am J Respir Cell Mol Biol (2015) 52(5):641–52. doi: 10.1165/rcmb.2014-0327OC
114. Zhang L, Jin S, Wang C, Jiang R, Wan J. Histone deacetylase inhibitors attenuate acute lung injury during cecal ligation and puncture-induced polymicrobial sepsis. World J Surg (2010) 34(7):1676–83. doi: 10.1007/s00268-010-0493-5
115. Feldman C, Anderson R. The role of co-infections and secondary infections in patients with COVID-19. Pneumonia (Nathan) (2021) 13(1):5. doi: 10.1186/s41479-021-00083-w
116. Zhou Y, Shi W, Wen Y, Mao E, Ni T. Comparison of pathogen detection consistency between metagenomic next-generation sequencing and blood culture in patients with suspected bloodstream infection. Sci Rep (2023) 13(1):9460. doi: 10.1038/s41598-023-36681-5
117. Florescu DF, Kalil AC. The complex link between influenza and severe sepsis. Virulence (2014) 5(1):137–42. doi: 10.4161/viru.27103
118. Cavaillon JM, Osuchowski MF. COVID-19 and earlier pandemics, sepsis, and vaccines: a historical perspective. J Intensive Med (2021) 1(1):4–13. doi: 10.1016/j.jointm.2021.04.003
119. Kalil AC, Thomas PG. Influenza virus-related critical illness: pathophysiology and epidemiology. Crit Care (2019) 23(1):258. doi: 10.1186/s13054-019-2539-x
120. Quinn KL, Stukel TA, Huang A, Abdel-Qadir H, Altaf A, Bell CM, et al. Comparison of medical and mental health sequelae following hospitalization for COVID-19, Influenza, and Sepsis. JAMA Intern Med (2023) 183(8):806–17. doi: 10.1001/jamainternmed.2023.2228
121. Wouters OJ, Forman R, Anderson M, Mossialos E, McKee M. The launch of the EU Health Emergency Preparedness and Response Authority (HERA): improving global pandemic preparedness? Health Policy (2023) 133:104844. doi: 10.1016/j.healthpol.2023.104844
122. Boyce MR, Sorrell EM, Standley CJ. An early analysis of the World Bank’s Pandemic Fund: a new fund for pandemic prevention, preparedness and response. BMJ Glob Health (2023) 8(1):e011172. doi: 10.1136/bmjgh-2022-011172
123. Gostin LO, Halabi SF, Klock KA. An international agreement on pandemic prevention and preparedness. JAMA (2021) 326(13):1257–8. doi: 10.1001/jama.2021.16104
124. Global Sepsis Alliance. 2030 global agenda for sepsis. GSA (2024). Available at: https://static1.squarespace.com/static/58a7025b8419c215b30b2df3/t/66eb2bf6ea445e649f46f905/1726688249207/2030_Global_Agenda_Sep18.pdf
Keywords: sepsis, systems immunology, diagnostics, endotypes, RNA-Seq, multiomics, pandemics
Citation: Hancock REW, An A, dos Santos CC and Lee AHY. Deciphering sepsis: transforming diagnosis and treatment through systems immunology. Front Sci (2025) 2:1469417. doi: 10.3389/fsci.2024.1469417
Received: 23 July 2024; Accepted: 11 November 2024;
Published: 30 January 2025.
Edited by:
Yoram Vodovotz, University of Pittsburgh, United StatesReviewed by:
Antonio Artigas, Parc Taulí Foundation, SpainJonathan Cohen, Brighton and Sussex Medical School, United Kingdom
Copyright © 2025 Hancock, An, dos Santos and Lee. This is an open-access article distributed under the terms of the Creative Commons Attribution License (CC BY). The use, distribution or reproduction in other forums is permitted, provided the original author(s) and the copyright owner(s) are credited and that the original publication in this journal is cited, in accordance with accepted academic practice. No use, distribution or reproduction is permitted which does not comply with these terms.
*Correspondence: Robert E. W. Hancock, Ym9iQGhhbmNvY2tsYWIuY29t