- 1Section of Structural and Synthetic Biology, Department of Infectious Disease, Imperial College London, London, United Kingdom
- 2London Biofoundry, Imperial College Translation and Innovation Hub, London, United Kingdom
- 3UK Dementia Research Institute Care Research and Technology Centre, Imperial College London, London, United Kingdom
A Viewpoint on the Frontiers in Science Lead Article
Real-time genomic surveillance for enhanced control of infectious diseases and antimicrobial resistance
Key points
- Pathogen surveillance forms only the first part of outbreak investigation and containment. Without suitable supporting measures, it cannot effectively prevent the evolution of outbreaks and epidemics into pandemics.
- There is limited political will to fund dedicated supporting infrastructure for pandemic preparedness, and therefore this infrastructure needs to form part of national research and development, biomanufacturing, and diagnostics healthcare ecosystems.
Introduction
The lead article by Struelens et al. (1) offers a comprehensive examination of the evolution of pathogen surveillance during the SARS-CoV-2 pandemic and maps out how further investment, the development of global standards, and a renewed focus on One Health policies and initiatives can play an important role in the monitoring and control of infectious diseases and antimicrobial resistance.
We view pathogen surveillance initiatives as the important first step of any public health intervention as the information garnered can be used to tailor subsequent containment strategies. However, any subsequent interventions rely heavily on supporting healthcare and biomanufacturing infrastructure to investigate the cause of syndromic clusters (such as acute flaccid paralysis, hemorrhagic fever, or pneumonia of unknown etiology) and develop diagnostic tests and countermeasures to contain them before they evolve into epidemics and pandemics.
In this viewpoint, we first discuss some of the limitations of surveillance strategies and then share how our experiences during the SARS-CoV-2 pandemic support our view that pandemic preparedness and healthcare system resilience will benefit substantially from the development of multiple local “biotech” ecosystems with both expertise and equipment that can be repurposed at a moment’s notice.
The limitations of pathogen surveillance
In late December 2019, a cluster of pneumonia cases of unknown etiology was reported in Wuhan, People’s Republic of China (2) and within 2 weeks the causal pathogen’s genomic sequence was made publicly available, enabling the rapid development of diagnostic assays (3). This case contrasts with that of the Ebola outbreak in 2013 in West Africa where poor diagnostic preparedness (4) led to a 3-month delay in identifying the outbreak, and, despite the low reproductive number (5), the delayed implementation of diagnostics infrastructure meant that the outbreak was only contained in 2016.
The rapid identification of the pathogen responsible for the COVID-19 pandemic represents close to the ideal case when it comes to hospital surveillance systems that link closely with public health authorities to investigate disease clusters. This was potentially because the virus first emerged in a country that scores highly on the Global Health Security Index, specifically for Laboratory Systems Strength and Quality (6), which clearly played a role in the rapid investigation of the outbreak. However, many of the places where emerging zoonotic diseases are likely to first present do not have resilient surveillance and diagnostics infrastructure (7).
Some efforts to strengthen surveillance infrastructure have focused on implementing sequencing capacity-building efforts in low- and middle-income countries (LMICs) (8) and exploring more cost-effective forms of surveillance such as wastewater testing. Wastewater surveillance, a promising modality in highly populated areas, still relies largely on wastewater treatment plants or formal sewerage systems. This is a significant limitation of this approach, as in many parts of the world there is little correlation between population density and the presence of wastewater treatment plants (Figure 1), making it of limited utility to replace current case notification-based surveillance. As a part of the Millenium Development Goals (MDGs), there are efforts to invest in WASH (access to sanitation, hygiene, and clean drinking water) initiatives, but many of these projects have decades-long time frames and have seen little progress in the last 24 years (Figure 1). Companies such as Ginkgo Bioworks are also focusing on building biosecurity “radar” systems by performing aircraft wastewater testing through collaborations with governments around the world. These efforts can serve as valuable proxies for traditional surveillance, providing insight into community transmission levels (12) in countries without adequate surveillance infrastructure. An early understanding of the epidemiology can then allow for interventions to be planned and implemented in both the source and destination countries. However, Ginkgo’s effort, focused on testing those traveling through air transit hubs, relies on the assumption that those traveling via air are broadly representative of the underlying population (and infectious disease risk) in a country. This is more likely to be an appropriate approximation for those in high-income countries (HICs) but is heavily biased toward certain socio-demographic groups that can afford air travel in LMICs.
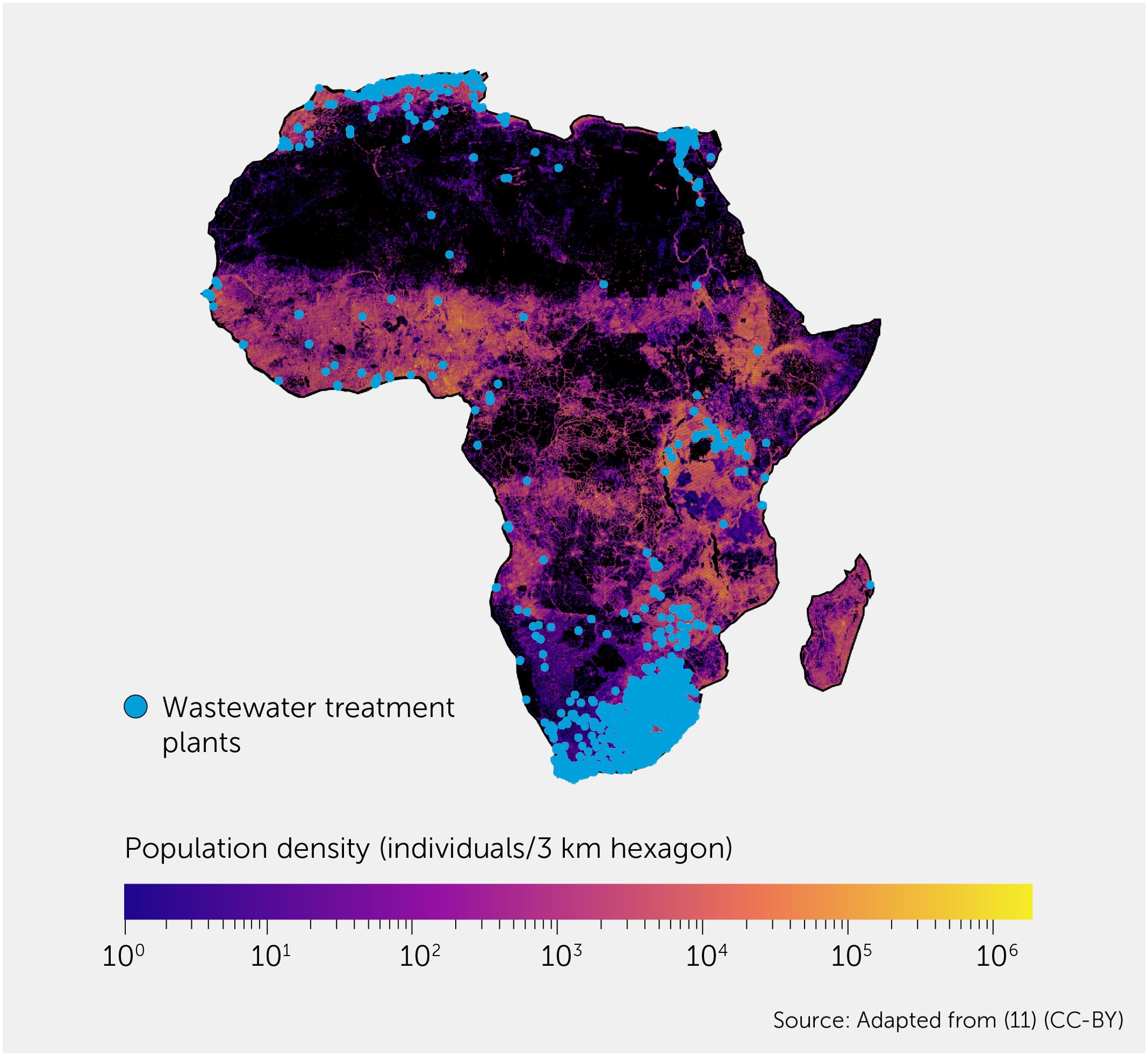
Figure 1. Distribution of wastewater treatment plants (WWTPs) on the background of population density in Africa. Population density is plotted using Kontur population data at 3km hexagon resolution (9). WWTP data are from the HydroWASTE v1 dataset (10). This diagram is adapted from (11).
These renewed surveillance efforts are still in their infancy and are likely to yield interesting public health data that can guide vaccination strategies for known pathogens and provide more insight into disease dynamics. Our concern, however, is that many of these initiatives are better suited for implementation in HICs, and the sole reliance on surveillance systems could easily lead to outbreaks rapidly evolving into pandemics. This was clearly the case with SARS-CoV-2, where rapid identification of the underlying pathogen did not lead to rapid containment largely due to the lack of supporting diagnostics infrastructure to help isolate and contain the initial outbreak.
Rapidly developing clinical diagnostics in a pandemic
A key challenge during the early stages of the pandemic was access to the consumables used to perform diagnostic tests. Our dependence on just-in-time manufacturing (production directly coupled with demand and without stockpiling of additional materials) means that optimized production lines have restricted capacity to increase supply. With many robotic automation systems competing for the same plastic pipette tips and consumables, there was very limited scope to rapidly scale diagnostic efforts.
Diagnostic testing strategies differed between countries. The United Kingdom made an early decision to perform all testing centrally through Public Health England (now the United Kingdom Health Security Agency). This meant that in March 2020 the entire testing capacity of the United Kingdom was less than 2,000 samples per day (13), which contrasted with the approaches adopted by other European countries that have local biomanufacturing capabilities, such as Germany, where a distributed network of 300 laboratories could perform 160,000 tests per week by mid-March (14).
Based in the London Biofoundry and Imperial College London, some of our research focuses on the development of novel diagnostics using synthetic biology tools, and we leverage laboratory automation in our academic setting to rapidly develop and optimize new diagnostic assays. Our access to laboratory automation not typically used for diagnostics that could be rapidly repurposed would prove to be indispensable when developing diagnostics for clinical use, even though, before the end of January 2020, nothing that we had previously developed had been used in clinical decision making.
In 9 weeks, a small team of fewer than 10 people brought an idea to the diagnostic frontline (15), performing testing in United Kingdom Accreditation Service (UKAS) ISO 15189–accredited laboratories on patients and clinical staff. By the end of 2020, testing was being performed in two high-throughput laboratories, and the close working relationship between academics and clinical laboratory staff allowed for the prototyping of new approaches to increase testing capacity further (16). We offered more than 5% of the national Pillar 1 (patient and hospital staff diagnostic testing) capacity during the critical initial stages of the pandemic and, when our solution was scaled, we went on to provide Pillar 2 testing (population testing) as a part of the National Lighthouse Lab program, as well as contributing approximately 5% of the national capacity of Pillar 1 testing through our work together with North West London Pathology (Imperial College Healthcare NHS Trust). When the labs ceased operations in 2022, almost 2 million reverse transcriptase polymerase chain reaction (RT-PCR) tests had been carried out with our accredited workflows.
Looking back on the past 4 years, it is easy to appreciate that the position we found ourselves in could be considered unique: a melting pot of clinical, virological, and diagnostic development, and laboratory automation expertise and equipment ready to spring into action. However, we believe that national pandemic preparedness frameworks can build and leverage relationships like these to create a resilient ecosystem that can spring into action when the need arises.
Centralized versus decentralized testing strategies
The population testing strategy eventually adopted in the United Kingdom was a centralized approach with several “Lighthouse Labs” and courier transport of testing kits to and from members of the public with symptoms. Equipment was privately purchased or requisitioned from universities and many academics volunteered their time. The centralized approach, although simpler in terms of supply chain management (which overcame many of the resourcing challenges faced by smaller diagnostic labs), had very long turnaround times. On average, results were provided 30 hours after samples were collected, not including the time it took for the testing kit to be shipped to homes (17).
Faster test turnarounds profoundly reduce onward transmission of SARS-CoV-2. In Austin, Texas, communication of results within 24 hours of sample collection reduced transmission by 68% (18). The testing program that we ran with our clinical laboratory partners had a target turnaround time of 15 hours, and our university testing program returned most results within 6 hours of sample submission, proving the value of distributed, rather than centralized, testing laboratories.
The rapid turnarounds in our university program were complemented by next-generation sequencing and an in-house S-gene target failure assay, which were used to rapidly detect new circulating variants and clusters of infection. This information was passed on to university management who were able to use the information to rapidly inform existing and new policy.
Building biotech ecosystems for pandemic preparedness
It is estimated that a US$124 billion investment is needed over the next 5 years for capacity building to ensure a global prevention, detection, and response system compliant with the International Health Regulations (19). However, it is unclear exactly where this funding could be sourced from. The Pandemic Fund, a new funding mechanism proposed to support some pandemic preparedness activities, would provide only a fraction of the required funding and has not been successful in raising the annual contributions it predicts are needed to achieve its goals. Of the annual US$10.5 billion it deems necessary (20), just US$1.6 billion has been contributed so far (21). There is little incentive for governments to fund initiatives that will not see any output during a typical election cycle. This leads us to believe that we have not learned any lessons from the COVID-19 pandemic, and there is destined to be a chronic underfunding of pandemic preparedness initiatives. But is there another way to build resilience or is the only solution to directly invest in pandemic preparedness infrastructure?
The global response to COVID-19 faltered in part because of the lack of local biomanufacturing capacity. Without forethought, we regularly optimize for price over resilience, which has both national and global health security implications. We believe that by driving investment and incubating collaboration in biotech ecosystems and making these public-private partnerships an integral part of health security we can change this dynamic, and that this approach would not be exclusive to HICs. This approach would directly benefit and grow the national bioeconomies of supporting countries while indirectly strengthening resilience to pandemic threats—creating excess capacity that could be mobilized as distributed diagnostic networks during disease outbreaks.
Our experience showed what is possible, even without the financial and staffing resources of other academic initiatives in the United Kingdom (22). All the initiatives in the United Kingdom were ad-hoc and took several months to scale but at the same time laid the foundation for a rapid implementation of similar approaches in the future. Most of the uncoordinated diagnostic testing initiatives in the United Kingdom involved the close collaboration of academics and diagnostic laboratory staff, but there is no reason why small and large biotech companies cannot form part of these ecosystems of specialist expertise and equipment. Now that a foundation for these collaborations has been built and protocols and software have been developed, it is time to define the role that these organizations can play in future outbreaks.
Acknowledgments
We thank the United Kingdom (UK) Dementia Research Institute for rapidly funding the initial workflow development and for continuing support via the UK Dementia Research Institute Care Research and Technology Centre based at Imperial College London and the University of Surrey, United Kingdom. We would like to thank the original team in the London Biofoundry who helped to deliver the initial workflow, bringing the concept from bench to diagnostic laboratory so quickly (Michael Crone, Miles Priestman, Marta Ciechonska, and Marko Storch). We would like to thank Kirsten Jensen and Andrew Griffiths for logistics and project management support. We would like to thank the team at North West London Pathology (Paul Randell, Panagiotis Pantelidis, Arthi Anand, and Cindy Madona) who helped us to validate and scale our solution for clinical testing. We would like to thank Graham Taylor, Aileen Rowan, Anjna Badhan, and Lesley Rawlinson for their help in delivering Imperial College Student and Staff testing, variant testing, and next-generation sequencing, and the Imperial College Lighthouse Laboratory. We would like to thank all the staff who worked in the Imperial College Lighthouse Laboratory—without you this work would not have had the same impact.
Statements
Author contributions
PF: Conceptualization, Supervision, Writing – original draft, Writing – review & editing. MC: Conceptualization, Data curation, Investigation, Visualization, Writing – original draft, Writing – review & editing.
Funding
The author(s) declare financial support was received for the research, authorship, and/or publication of this article. PF receives funding from UK Dementia Research Institute (UKDRI) Care Research and Technology Centre funded by the Medical Research Council (MRC), Alzheimer’s Research UK, Alzheimer’s Society (grant number: UKDRI-7002). The funder was not involved in the study design, collection, analysis, interpretation of data, the writing of this article or the decision to submit it for publication.
Conflict of interest
The authors declare that the research was conducted in the absence of financial relationships that could be construed as a potential conflict of interest.
Publisher’s note
All claims expressed in this article are solely those of the authors and do not necessarily represent those of their affiliated organizations, or those of the publisher, the editors and the reviewers. Any product that may be evaluated in this article, or claim that may be made by its manufacturer, is not guaranteed or endorsed by the publisher.
References
1. Struelens MJ, Ludden C, Werner G, Sintchenko V, Jokelainen P, Ip M. Real-time genomic surveillance for enhanced control of infectious diseases and antimicrobial resistance. Front Sci (2024) 2:1298248. doi: 10.3389/fsci.2024.1298248
2. World Health Organization. Listings of WHO’s response to COVID-19 (2020). Available at: https://www.who.int/news/item/29-06-2020-covidtimeline
3. Lu X, Wang L, Sakthivel SK, Whitaker B, Murray J, Kamili S, et al. US CDC Real-time reverse transcription PCR panel for detection of severe acute respiratory syndrome coronavirus 2. Emerg Infect Dis (2020) 26(8):1654–65. doi: 10.3201/eid2608.201246
4. Perkins MD, Dye C, Balasegaram M, Bréchot C, Mombouli JV, Røttingen JA, et al. Diagnostic preparedness for infectious disease outbreaks. Lancet (2017) 390(10108):2211–14. doi: 10.1016/s0140-6736(17)31224-2
5. Chowell G, Nishiura H. Transmission dynamics and control of Ebola virus disease (EVD): a review. BMC Med (2014) 12:196. doi: 10.1186/s12916-014-0196-0
6. Bell JA, Nuzzo JB. Global Health Security Index: advancing collective action and accountability amid global crisis, 2021 (2021). Available at: https://ghsindex.org/wp-content/uploads/2021/12/2021_GHSindexFullReport_Final.pdf.
7. Allen T, Murray KA, Zambrana-Torrelio C, Morse SS, Rondinini C, Di Marco M, et al. Global hotspots and correlates of emerging zoonotic diseases. Nat Commun (2017) 8(1):1124. doi: 10.1038/s41467-017-00923-8
8. Kanteh A, Manneh J, Sanyang B, Kujabi MA, Jallow HS, Dabiri Damilare K, et al. Simple and structured model to build sequencing capacity in west Africa. Lancet Glob Health (2022) 10(9):e1240–1. doi: 10.1016/s2214-109x(22)00319-9
9. Kontur. Kontur Population: global population density for 3km H3 hexagons (2023). Available at: https://data.humdata.org/dataset/kontur-population-dataset-3km.
10. Macedo HE, Lehner B, Nicell J, Grill G, Li J, Limtong A, et al. Distribution and characteristics of wastewater treatment plants within the global river network. Earth Syst Sci Data (2022) 14:559–77. doi: 10.5194/essd-14-559-2022
11. Crone MA. Developing standards and workfows for high-throughput and surveillance diagnostics applications [Thesis]. London: Imperial College London (2023). doi: 10.25560/107682
12. Smith TC, Bart SM, Loh SM, Rothman J, Grubaugh ND, Gardner L, et al. SARS-CoV-2 Sample Positivity in Travellers Can Predict Community Prevalence Rates: Data from the Traveller-Based Genomic Surveillance Programme. SSRN (2024). [Preprint]. doi: 10.2139/ssrn.4720735
13. Rough E. Coronavirus: testing for Covid-19 (2020). Available at: https://commonslibrary.parliament.uk/research-briefings/cbp-8897/.
14. Eckner C. How Germany has managed to perform so many Covid-19 tests. The Spectator (2020). Available at: https://www.spectator.co.uk/article/how-Germany-has-managed-to-perform-so-many-covid-19-tests/.
15. Crone MA, Priestman M, Ciechonska M, Jensen K, Sharp DJ, Anand A, et al. A role for biofoundries in rapid development and validation of automated SARS-CoV-2 clinical diagnostics. Nat Commun (2020) 11(1):4464 [Correction published: Nat Commun (2020) 11(1):4793]. doi: 10.1038/s41467-020-18130-3
16. Crone M, Randell P, Herm Z, Anand A, Missaghian-Cully S, Perelman L, et al. Rapid design and implementation of an adaptive pooling workflow for SARS-CoV-2 testing in an NHS diagnostic laboratory: a proof-of-concept study. Wellcome Open Res (2021) 6:268. doi: 10.12688/wellcomeopenres.17226.1
17. United Kingdom Health Security Agency. NHS Test and Trace statistics (England): methodology (2022). Available at: https://www.gov.uk/government/publications/nhs-test-and-trace-statistics-england-methodology/nhs-test-and-trace-statistics-england-methodology.
18. Wang X, Du Z, James E, Fox SJ, Lachmann M, Meyers LA, et al. The effectiveness of COVID-19 testing and contact tracing in a US city. Proc Natl Acad Sci USA (2022) 119:e2200652119. doi: 10.1073/pnas.2200652119
19. Eaneff S, Graeden E, McClelland A, Katz R. Investing in global health security: estimating cost requirements for country-level capacity building. PloS Glob Public Health (2022) 2(12):e0000880. doi: 10.1371/journal.pgph.0000880
20. World Health Organization, World Bank. Analysis of Pandemic Preparedness and Response (PPR) architecture, financing needs, gaps and mechanisms (2022). Available at: https://thedocs.worldbank.org/en/doc/5760109c4db174ff90a8dfa7d025644a-0290032022/original/G20-Gaps-in-PPR-Financing-Mechanisms-WHO-and-WB-pdf.pdf.
21. World Bank. Financial Intermediary Funds (FIFs): The Pandemic Fund (2024). Available at: https://fiftrustee.worldbank.org/en/about/unit/dfi/fiftrustee/fund-detail/pppr.
Keywords: diagnostics, healthcare resilience, bioeconomy, containment, pandemic, surveillance
Citation: Crone MA and Freemont PS. Thinking beyond pathogen surveillance: building resilient biotech ecosystems to combat the next pandemic. Front Sci (2024) 2:1412291. doi: 10.3389/fsci.2024.1412291
Received: 04 April 2024; Accepted: 05 August 2024;
Published: 10 September 2024.
Edited and Reviewed by:
Richard V. Goering, Creighton University, United StatesCopyright © 2024 Crone and Freemont. This is an open-access article distributed under the terms of the Creative Commons Attribution License (CC BY). The use, distribution or reproduction in other forums is permitted, provided the original author(s) and the copyright owner(s) are credited and that the original publication in this journal is cited, in accordance with accepted academic practice. No use, distribution or reproduction is permitted which does not comply with these terms.
*Correspondence: Michael A. Crone, Y3JvbmUubWljaGFlbEBnbWFpbC5jb20=; Paul S. Freemont, cC5mcmVlbW9udEBpbXBlcmlhbC5hYy51aw==