Explore article hub
- 1Faculty of Medicine, Université Libre de Bruxelles, Brussels, Belgium
- 2Clinical-Research Interface, Genomics England, London, United Kingdom
- 3Department of Medicine, University of Cambridge, Cambridge, United Kingdom
- 4National Reference Centre for Staphylococci and Enterococci, Division of Nosocomial Pathogens and Antibiotic Resistances, Department of Infectious Diseases, Robert Koch Institute (Wernigerode branch), Wernigerode, Germany
- 5Sydney Infectious Diseases Institute, University of Sydney, Sydney, NSW, Australia
- 6Centre for Infectious Diseases and Microbiology – Public Health, Institute of Clinical Pathology and Medical Research, New South Wales Health Pathology, Sydney, NSW, Australia
- 7Infectious Disease Preparedness, Statens Serum Institut, Copenhagen, Denmark
- 8Department of Microbiology, Faculty of Medicine, The Chinese University of Hong Kong, Prince of Wales Hospital, Hong Kong, Hong Kong SAR, China
- 9Shenzhen Research Institute, The Chinese University of Hong Kong, Shenzhen branch, Shenzhen, China
- 10European Society for Clinical Microbiology and Infectious Diseases Study Group on Public Health Microbiology (ESGPHM)
- 11European Society for Clinical Microbiology and Infectious Diseases Study Group on Epidemiological Markers (ESGEM)
Abstract
This article advocates for mobilizing pathogen genomic surveillance to contain and mitigate health threats from infectious diseases and antimicrobial resistance (AMR), building upon successes achieved by large-scale genome sequencing analysis of SARS-CoV-2 variants in guiding COVID-19 monitoring and public health responses and adopting a One Health approach. Capabilities of laboratory-based surveillance and epidemic alert systems should be enhanced by fostering (i) universal access to real-time whole genome sequence (WGS) data of pathogens to inform clinical practice, infection control, public health policies, and vaccine and antimicrobial drug research and development; (ii) integration of diagnostic microbiology data, data from testing asymptomatic individuals, pathogen sequence data, clinical data, and epidemiological data into surveillance programs; (iii) stronger cross-sectorial collaborations between healthcare, public health, animal health, and environmental surveillance and research using One Health approaches, toward understanding the ecology and transmission pathways of pathogens and AMR across ecosystems; (iv) international collaboration and interconnection of surveillance networks, harmonization of laboratory methods, and standardization of surveillance methods for global reporting, including on pathogen genomic variant or strain nomenclature; (v) responsible data sharing between surveillance networks, databases, and platforms according to FAIR (findability, accessibility, interoperability, and reusability) principles; and (vi) research on genomic surveillance system implementation and its cost-effectiveness for different pathogens and AMR threats across different settings. Regional and global One Health policies and governance initiatives should foster the concerted development and efficient utilization of pathogen genomic surveillance to protect the health of humans, animals, and the environment.
Key points
- Insights gained from the systemic use of whole genome sequencing (WGS) to monitor novel variants of SARS-CoV-2 during the COVID-19 pandemic have accelerated the wider integration of WGS for infectious disease and antimicrobial resistance (AMR) surveillance and a One Health approach to inform prevention and control strategies, including vaccination.
- Pandemic preparedness requires worldwide deployment of agile integrated genomic, clinical, and epidemiologic surveillance systems with seamless interoperability and global interconnectivity—allowing real-time monitoring of the emergence, spread, virulence, and evolution of pathogens, using a One Health perspective.
- One Health genomic surveillance requires wider cross-sectorial collaboration and data sharing in line with ethical regulations, between laboratories, health agencies, surveillance databases, and the broader scientific community—using FAIR principles regarding findability, accessibility, interoperability, and reusability.
- International harmonization of methods and nomenclature for genomic surveillance as well as support for timely data sharing are key steps to enable coordinated global responses to cross-border threats from infectious diseases and AMR.
- Continued investment to enhance laboratory capacity, provide access to validated genomic data analytical tools, train an expert workforce (including health professionals), and achieve a comprehensive digital health information infrastructure must address international resource inequalities to ensure universal access.
- Academic, public health, and non-governmental organizations should share best practices to advance the equitable deployment of pathogen genomics through collaborative initiatives at regional and global levels.
Introduction
The successes and challenges encountered by public health surveillance systems deployed during the COVID-19 pandemic offer lessons for surveillance of other infectious diseases and antimicrobial resistance (AMR). The pandemic emphasized the urgent need to modernize and strengthen health services and public health surveillance, and it demonstrated that epidemic response systems are a key aspect of health systems’ resilience (1, 2). This entails marshaling scientific innovation and technology in the fight not only against COVID-19 but also against evolving health threats from emerging pathogens and the rise in AMR. Recent global estimates have confirmed that AMR is a leading cause of death around the world, making it a global public health priority (3).
The widespread use of whole genome sequencing (WGS) to detect and characterize novel variants of SARS-CoV-2 has been instrumental in guiding the risk assessment and public health management of the COVID-19 pandemic at the local, national, and international levels (4). Further, it has allowed the detection and monitoring of potential immune-escape SARS-CoV-2 variants to guide the development and effective use of COVID-19 vaccines (5–8). As such, availability of WGS capacity for pathogen surveillance is integral to recommendations on future pandemic preparedness (2). Motivated by this progress, an acceleration of a decade-long paradigm shift from traditional sector-specific epidemiological surveillance based on case notification to real-time, genomic-enhanced surveillance systems that span across sectors for the prevention and control of infectious diseases is occurring (4, 9, 10). These new systems integrate the epidemiological context with pathogen sequence data in a One Health framework to provide more precise risk assessment. In turn, this informs the design and evaluation of adaptive responses as pathogens emerge, spread, and evolve in terms of their host range, pathogenicity, drug resistance, or capacity to escape vaccines (4, 11, 12). However, health inequalities and public health system vulnerabilities need to be addressed to ensure global access to WGS-based methods to enhance infectious disease surveillance and provide timely epidemic alerts and responses.
Building upon an appraisal of the role played by WGS in detecting novel SARS-CoV-2 variants and monitoring viral spread, this article advocates for extended use of genomic surveillance in the risk assessment and mitigation of health threats from other infectious diseases and AMR. To this end, we make recommendations on laboratory technology and practice changes, capacity building, cross-sectorial collaborations, public health system developments, and data-sharing governance, as well as research opportunities to strengthen local, national, and worldwide capabilities of integrated WGS-based surveillance of infectious threats to inform rapid epidemic response and curtail future pandemics (Figure 1).
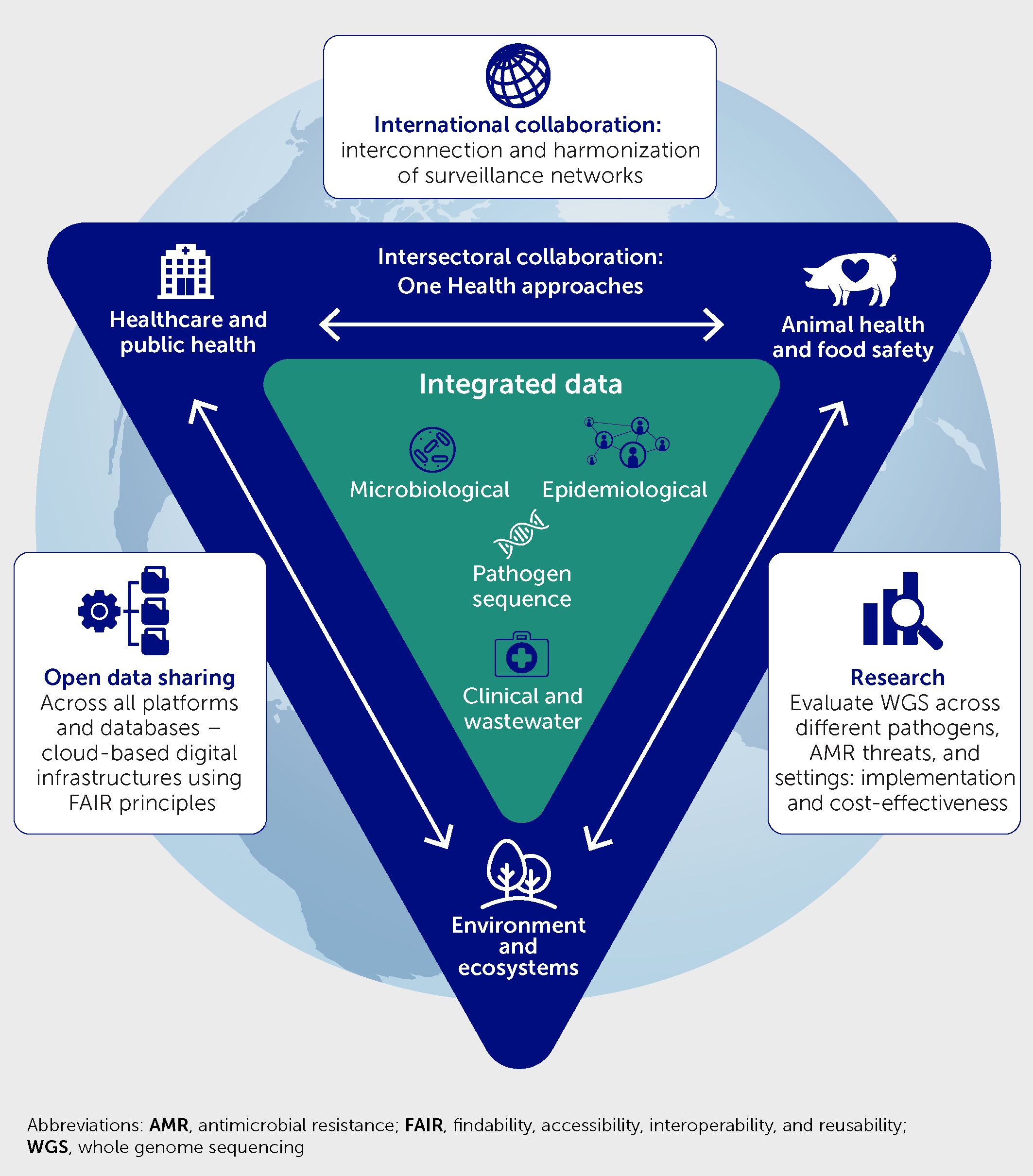
Figure 1 Framework of recommendations to enhance laboratory-based infection surveillance and epidemic alert systems via universal access to real-time whole genome sequencing analysis, with integration of these data with microbiology, clinical, environmental, and epidemiological data; strengthened cross-sectoral One Health collaborations; international collaboration to interconnect surveillance networks and harmonize methods; open data sharing; and research to evaluate the benefits.
Strengths and weaknesses of COVID-19 monitoring and responses
Several strengths and weaknesses have been observed globally when applying genomic surveillance to the monitoring of and response to the COVID-19 pandemic.
Strengths
New and emerging SARS-CoV-2 variants, particularly those with characteristics related to high transmissibility, illness severity, or immune response evasion, have repeatedly threatened the global pandemic response. Thus, the ability to rapidly detect, monitor, and understand the evolution of the virus using WGS has been crucial.
The ability to respond quickly has relied on collaboration between diverse academic and public health professionals to increase sequencing capacity, develop methodologies to further improve the quality and efficiency of the data generated, and perform analyses required for genomic surveillance. Figure 2 shows examples of consortia and networks that have undertaken this work.
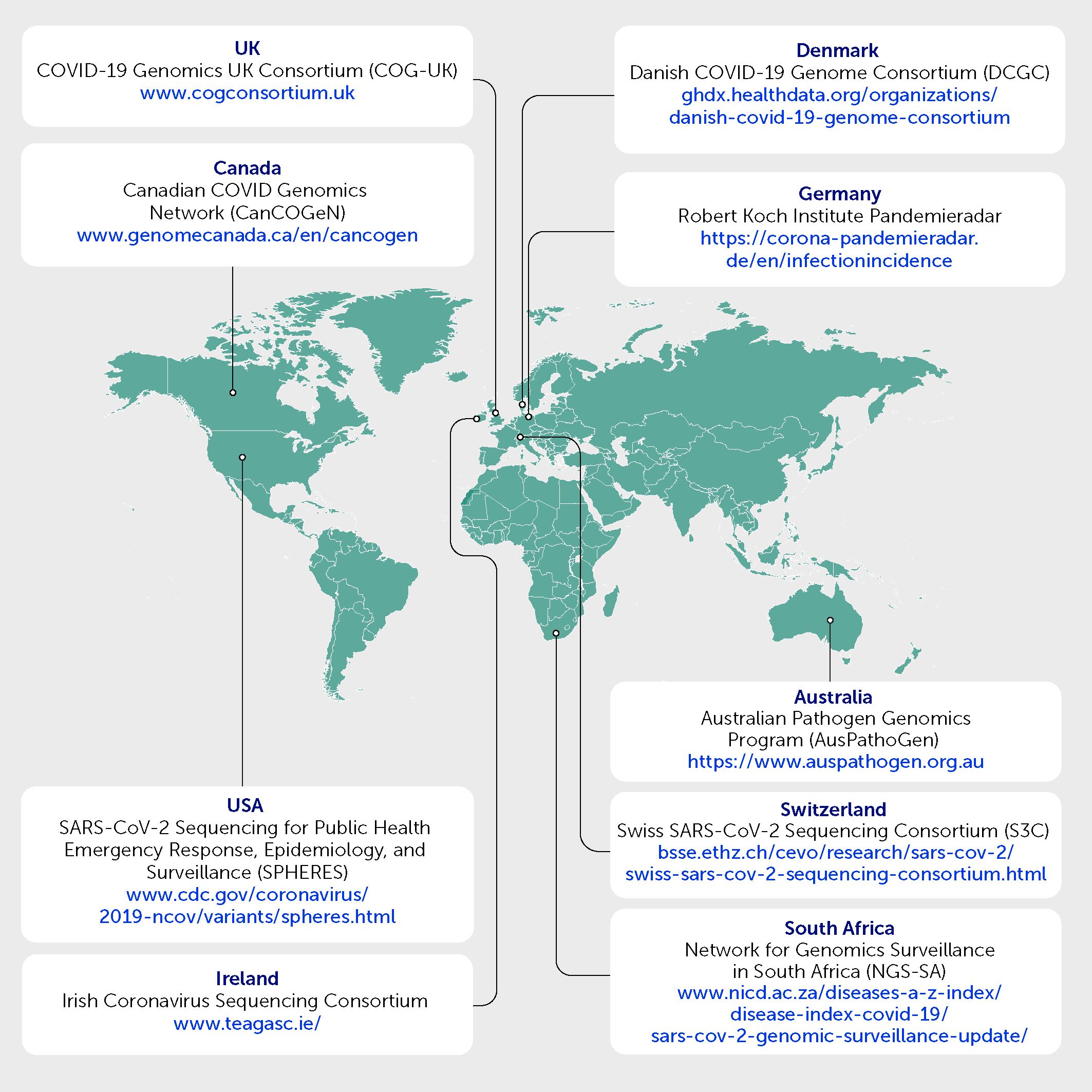
Figure 2 Examples of consortia and networks whereby academic and public health professionals have collaborated to undertake genomic surveillance for SARS-CoV-2.
The increased sharing of sequencing data and protocols (e.g., https://www.protocols.io) has created an ecosystem of critique and review that has led to continuous improvements to sequencing methods, analytical tools, knowledge sharing, and joint recognition on research publications worldwide. Genomic surveillance during the pandemic was readily achieved globally through the integration of SARS-CoV-2 sequence data into the Global Initiative on Sharing All Influenza Data (GISAID; https://gisaid.org/) database within the World Health Organization (WHO) Global Influenza Surveillance and Response System (GISRS). This initiative was initially established to help understand how influenza viruses evolve and spread during epidemics and pandemics (13). The platform promotes sharing of genetic sequences and clinical and epidemiological data regarding human influenza to link with geographical data associated with avian and animal viruses. GISAID involves public-private partnerships and academic institutions in many countries supporting data sharing and collaboration among researchers. A GISAID SARS-CoV-2 database was quickly implemented based on the influenza model; it was extremely useful, but criticisms were raised about governance and a lack of full public availability of data.
The sharing of SARS-CoV-2 sequence data via the GISAID database enabled countries around the world to understand which variants were emerging and circulating nationally and internationally. This allowed for early responses to mitigate the transmission of novel variants across borders, and for the amendment of testing and sequencing procedures to detect new variants. This was exemplified by the identification of the Alpha variant using WGS data generated by the COVID-19 Genomics UK (COG-UK) Consortium, which explained changes to the epidemiological characteristics and transmission dynamics of SARS-CoV-2, as well as the later identification and tracking of the Delta and Omicron variants of concern (VOCs) (14). Analysis of SARS-CoV-2 genomes and linkage to clinical data have informed medical improvements, including evaluations of vaccine efficacy against specific variants of SARS-CoV-2 and research on the susceptibility of viral variants to therapeutics against COVID-19 (8, 15–18).
Pre-existing sequencing infrastructure and expertise in pathogen genomics and bioinformatics within academic institutions and public health institutes were key success drivers for countries that rapidly introduced genomic surveillance of SARS-CoV-2. There was an urgent need to handle, store, and interpret large genomics datasets to enable genome-based decision-making processes. In the United Kingdom, the Cloud Institute for Microbial Bioinformatics was launched in 2014, with support from the Medical Research Council, and this pre-existing open, cloud-based infrastructure and expertise enabled COG-UK to rapidly share genome data and develop tools to interpret large datasets in a secure environment. In addition, the ARTIC network (https://artic.network/), built on previous experience in responding to other viral outbreaks, rapidly developed sequencing protocols and analytic tools for SARS-CoV-2 that were used worldwide. This highlights the importance of continued investments to support technical infrastructure, methodological development, training, and public health decision-making for genomic surveillance (19). Such investments reduce sequencing costs and turnaround times, provide better flexibility in sample types, and improve data quality, thereby increasing the affordability, scalability, and robustness of sequencing procedures (20, 21).
As shown in the United Kingdom and Africa, distributed sequencing through a “hub and spoke” model enabled regional sequencing sites to ensure technical capabilities and expertise, and to work closely with local hospitals to rapidly sequence and analyze WGS data, while enabling public health agencies to focus on national public health decision-making (19, 22). The relationships fostered between healthcare and academic professionals demonstrate the value of closer intersectoral links and the alignment of clinical care with research and innovation.
Weaknesses
Public health services faced a complex and sometimes conflicting array of priorities during the COVID-19 pandemic, including the testing and sequencing of samples from populations in the community, in healthcare settings, and at borders. This resulted in governance and management challenges that hindered decision-making and the streamlining of processes.
Key vulnerabilities in genomic surveillance systems were recognized during the COVID-19 pandemic. Access to reagents and consumables necessary for sequencing was hindered by global shortages, limiting sequencing capacity and revealing a need to strengthen supply chain resilience akin to corresponding reflections regarding diagnostics and pharmaceuticals (23–25). Better communication and collaboration between laboratories, as well as a central and more sophisticated stock management system, could enable better resource management across laboratories (22, 26). The lack of integration of surveillance systems and healthcare systems at a national level, together with a lack of data sharing standards, hampered data sharing and the linkage of clinical and sequencing data in many countries at the beginning of the pandemic. Further advances in informatics and interoperability between databases are needed to link large genomic datasets with other research and clinical datasets to support clinical decision-making and multi-layered research investigations. Challenges were also encountered with sample collection, sample storage, and logistics. These included the identification of a suitable point of contact at each testing laboratory, data protection and confidentiality issues, ethical clearances to access and send samples with corresponding metadata, access to affordable transport that could provide dry ice when required, and point-to-point delivery of samples. COVID-19-related staff absences further stretched workforce capacity, leading to staff feeling overwhelmed by requests to transfer samples with corresponding clinical metadata to national surveillance systems (27), added on to routine clinical testing. Owing to the lack of guidance for reporting WGS results from academic institutions, reports were often not standardized and there was no clear process for validating them (21, 26).
The worldwide deployment of SARS-CoV-2 genomic surveillance capacities was geographically uneven and followed a variety of implementation strategies adapted to national health system organizations and resources. Available sequencing capacities could not readily meet the increasing demands owing to limitations in infrastructure and trained personnel. This proved particularly problematic in low-income settings, for example in many parts of Africa, where a lack of existing sequencing infrastructure and expertise in genome analyses hindered the management and initial response to COVID-19 (22). Global analysis of viral sequence data deposited in public repositories has allowed quantitative assessments of national SARS-CoV-2 genomic surveillance programs in terms of case coverage, timeliness of reporting, and completeness of data sharing, broken down by sampling strategies and sequencing technology (28–30). The analysis has shown that, during the first 2 years of the pandemic, the SARS-CoV-2 genomic surveillance landscape displayed marked heterogeneity of outputs by country and economic income level. While 78% of high-income countries (HICs) sequenced >0.5% of reported COVID-19 cases, only 42% of low- and middle-income countries (LMICs) achieved that coverage level (29). The mean sequencing coverage ranged by region, from 0.1% in the Eastern Mediterranean region to 3.4% in Europe (28). Mean turnaround reporting time was shorter in HICs (24 days) and in Europe (18 days) than in other income groups or regions (28). Coverage increased and turnaround times shortened gradually during the pandemic (29, 30).
Global SARS-CoV-2 data sharing was also very uneven geographically. Uploading of VOC sequences in the public domain was incomplete in many countries and metadata release into GISAID was inadequate, with 63% of global sequences missing patient demographic information and more than 95% missing clinical information (28).
In Africa during 2020, 72% of genome sequencing capacity was concentrated in four countries (31). The SARS-CoV-2 genomic surveillance capacity rapidly expanded after coordination and mobilization of resources from 55 countries, with logistical support by the Africa Centres for Disease Control and Prevention (CDC), the WHO, and public-private funding, as part of the African Pathogen Genomics Initiative (31–34). This was achieved through increased continental coordination, strengthening of infrastructure for sequencing and data sharing, and workforce training in genomics and bioinformatics. Turnaround times sufficient to inform public health decisions were related to proximal access to sequencing services (32). The African SARS-CoV-2 genomic surveillance collaboration delivered important early warnings by discovering several emerging VOCs in South Africa (32).
Common implementation enablers can be learned from these experiences. In high-, low-, and middle-income countries, mobilizing sequencing expertise and resources across sectors and jurisdictions, adopting representative and targeted national sampling strategies, investing in sequencing infrastructure, analytics, and expert workforce training, and providing public health surveillance centers access to data sharing and analysis platforms were key improvements that allowed the gradual implementation of effective national and regional SARS-CoV-2 genomic surveillance programs for early detection and tracking of new variants of interest (16, 19–21, 26, 32, 35–37).
WGS advances to inform surveillance, clinical care, and infection control
WGS was enthusiastically introduced into medical microbiology as the one-size-fits-all microbial typing technique. Providing the entire genetic information of a microorganism, it achieves the ultimate depth of discrimination to infer or disprove a potential relatedness between microbial pathogens. WGS-based strain typing has advantages in terms of standardization, scalability, reliability, speed, and accuracy and has largely replaced other phenotypic and genetic typing techniques (11, 38, 39). Advances such as multiplexing have reduced the costs of WGS, while novel kits and procedures have sped up sample preparation, sequencing, and post-sequencing analyses (40, 41). Thus, genome-based strain typing has proven suitable for timely outbreak detection of viral, bacterial, and eukaryotic pathogens in community and hospital settings (42–45). In addition, WGS data have improved pathogen surveillance by allowing clinical, epidemiological, and in-depth strain characteristics to be integrated—thereby fostering and streamlining infection prevention and control measures at the local, national, and even global levels (46–48).
Public health surveillance
Current WGS methods have revolutionized the design and efficiency of pathogen surveillance by accelerating the sequencing and analysis process and increasing sample throughput. Samples are no longer collected by clinical and epidemiological suspicion: rather, prospective isolate collections are massively genome-sequenced and subjected to automated and parallelized data analysis to investigate the phylogenetic relatedness of strains, generate transmission hypotheses, and identify potential sources. This has been successfully introduced for genome-based surveillance of endemic diseases and conditions, e.g., foodborne and sexually transmissible pathogens, Mycobacterium tuberculosis, and AMR. WGS is also a key tool for the investigation and public health management of emerging pathogens, as was illustrated during the recent mpox global epidemic (49). WGS methods first used a decade ago in a limited number of well-equipped public health laboratories in high-income countries are now being successfully deployed in most regions of the world (50–54). Genome-wide high-resolution pathogen typing and surveillance has been implemented in many national and international action plans and is supported by leading health organizations such as the WHO (55–57), the United States CDC (58), the European Centre for Disease Prevention and Control (ECDC) (10, 59), and the United Kingdom National Institute for Health and Care Research (60). This new strategic approach foresees an epidemiological confirmation of genome-based transmission hypotheses and transforms pathogen surveillance from monitoring disease incidence trends to delineating their transmission pathways at a highly scalable resolution level (9, 42, 43).
Monitoring pathogen evolution, virulence, and vaccine escape
Pathogen genomic surveys and surveillance programs have shown the value of genomic analyses to monitor pathogen diversity and evolution, track population distribution of lineages and phenotypic variants of public health importance, characterize changes in virulence factors, and predict antigen matches with current and candidate vaccines and neutralizing antibodies, both for viral and bacterial diseases. The integration between epidemiology and phylogenetic analysis into the evolutionary epidemiology approach, using phylogeographic and phylodynamic computational analyses, has been widely applied to decipher the evolution of genomic diversity and reconstruct routes and timing of transmission of major viral pathogens, including the influenza virus, hepatitis viruses, human immunodeficiency virus, Zika, Chikungunya (61), SARS-CoV-2 (62–66), and mpox viruses (49, 67). Genomic surveillance of the influenza virus has become an integral part of GISRS to inform the yearly vaccine design cycle, by continuous monitoring of the rate of viral evolution and identifying novel variants, for both influenza and SARS-CoV-2 viruses (56). Phylogenetic and phylodynamic studies of SARS-CoV-2 virus diversification, lineage transmissibility, and fitness can help design vaccines, monitor the cross-immunity of circulating lineages, and evaluate vaccine selective pressure on lineage-specific, immune escape mutations that may affect the efficacy of vaccines and therapeutic monoclonal antibodies (5, 7, 15, 18, 68). For example, the identification of mutations in the spike protein of SARS CoV-2 has informed our understanding of immunity in populations and the adaptation of diagnostic assays and vaccine production (69–72). Further integrating high-resolution pathogen phylogenomic data into mathematical disease transmission models offers potential for more precise forecasting and prediction of infectious disease epidemics and the effect of health interventions (73).
The era of vaccine development based on molecular and genome data was introduced almost 20 years ago under the term “reverse vaccinology” (74). The use of genome data again pushed this field forward (75), leading to innovations such as genome-guided RNA vaccines against cancers (76, 77). Prominent examples of gene-guided vaccine development already exist for bacterial pathogens. Genomic surveillance is increasingly informing both vaccine development and adaptive updates of antigen composition and vaccination schemes to improve and sustain prevention of bacterial diseases. WGS analysis has become of paramount importance in monitoring vaccine-preventable bacterial pathogen diversity and population dynamics, and in identifying virulence traits and determinants of immune escape, including antigen gene mutations, recombination, and deletion in major pathogens, such as Streptococcus pneumoniae (78), Neisseria meningitidis (79, 80), and Bordetella pertussis (81, 82).
Clinical care and infection control
By rapidly and inexpensively characterizing the entire genome of a pathogen, WGS data analyses provide attractive tools for comprehensive, fast, and reliable microbial diagnostics (46, 49). The genome-wide approach has allowed the simultaneous prediction of drug resistance to guide antimicrobial therapy and virulence assessment (e.g., the production of toxins) to support adjunctive patient treatment (83–85). Reasonable attempts have been undertaken to derive the huge armamentarium of possible resistance mutations and genes from genome data. Web-based tools and international databases that predict antimicrobial susceptibilities are continuously updated, assessed, and validated and have reached a level of accuracy comparable or even superior to phenotypic susceptibility testing (86–88). Even multifaceted, complex, and multifactorial resistance mechanisms can be predicted from microbial genome data as an input (89–91). High-performance computing using machine learning algorithms and artificial intelligence (AI) allows AMR to be derived from large genome datasets, even in the absence of knowledge on resistance mechanisms or reference templates (92–95).
WGS data can also guide the development of novel diagnostics. Analysis of the genomic information of an emerging pathogen (or a new variant thereof) in comparison with a large dataset of already available genome data allows the derivation of discriminatory and diagnostically relevant markers (11, 96, 97).
Classical diagnostic procedures, some also using WGS data, mainly started with the isolated and identified strain of a microorganism. However, WGS-based approaches do not necessarily require a pure culture of an isolate (Figure 3). Significant advancements have been made in the last 10 years to improve culture-independent diagnostics and strain typing. Finding pathogen-specific signatures in complex sample material paved the way for metagenomic diagnostics and strain typing to guide therapy and infection prevention and control measures in the veterinary, food safety, and public health sectors (39, 46, 62, 96, 98). Meanwhile, metagenomic diagnostics is becoming an established field in human medical and veterinary microbiology with an immense potential that is actively advanced by an engaged scientific community. Metagenomic analysis of human, animal, and environmental samples also offers high sensitivity and flexibility for detection and quantitation of known and novel pathogens for public health surveillance (99). Requirements for standardization (in sampling, data generation, and analyses), comparatively high analytical cost, and certain legal and regulatory barriers currently hinder a wider medical application of this approach (100, 101). However, these barriers are expected to be resolved in a stepwise manner in the coming years, building on current standardization steps (102).
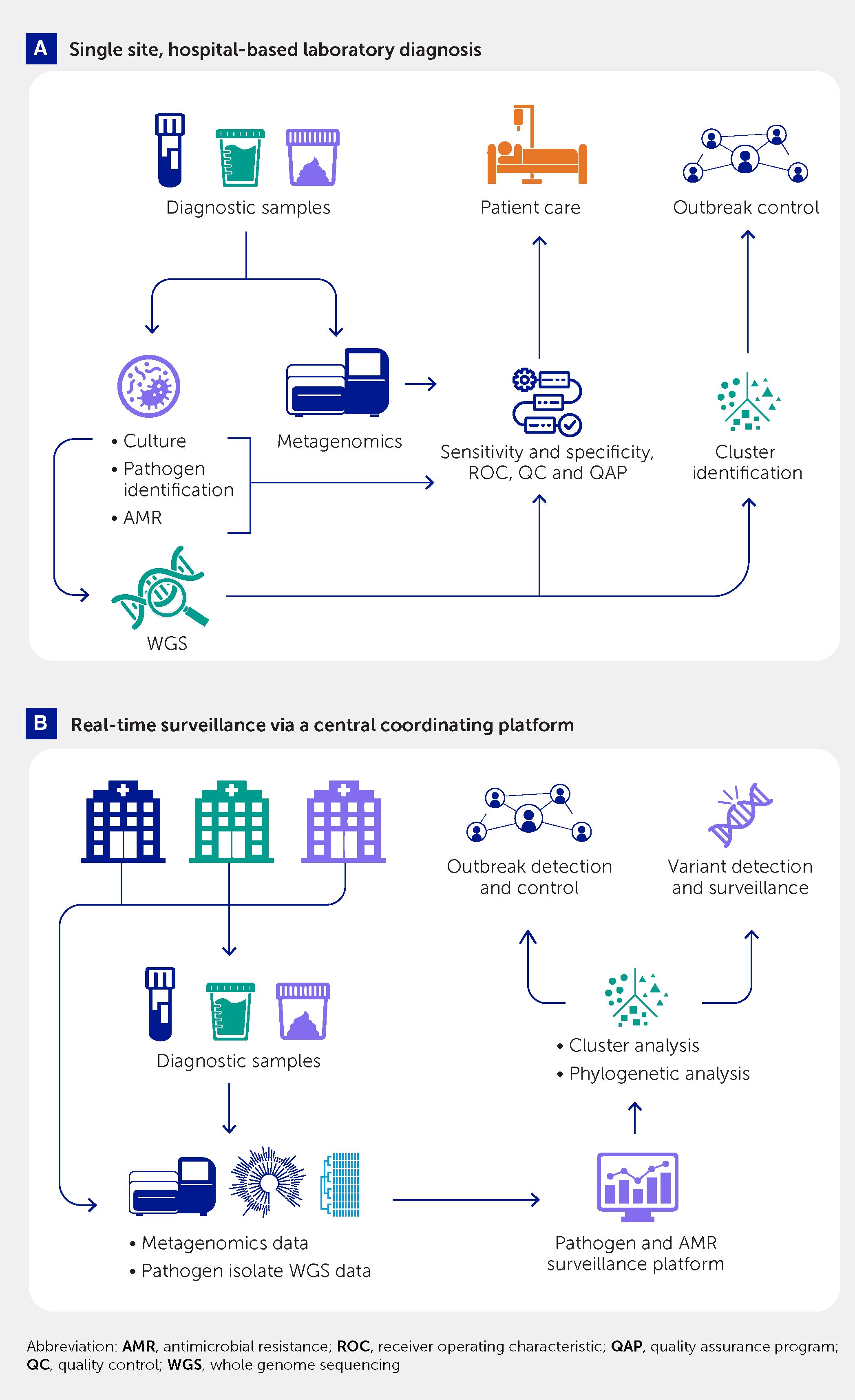
Figure 3 Use of culture-based whole genome sequencing and metagenomic pathogen detection and characterization for (A) single-center diagnostic support to patient care and hospital outbreak control and (B) a multicenter platform for real-time, public health–oriented genomic surveillance and outbreak control.
Next-generation and third-generation (long-read) sequencing
Technically, next-generation sequencing (NGS) describes a procedure involving the massive and parallel amplification and subsequent sequencing of DNA fragments of varying but comparably small lengths. This is accompanied by some limitations and drawbacks for downstream processing (103), for example, assembly breaks at repetitive elements and a missing differentiation between the chromosomal and plasmid contigs. Long-read sequencing (third-generation sequencing) circumvents these problems, but at the expense of higher quality input samples, higher costs, and higher computational demands, as well as potential for lower sequencing accuracy. Newer long-read sequencing technologies, such as those developed by Oxford Nanopore Technologies (ONT), have now reduced costs and improved accuracy, scalability, affordability, and flexibility (104). They are readily employable in low-resource settings (46). Until now, long-read sequencing projects have been mainly research-oriented but, with the possibilities of multiplexing and parallelization, the cost-per-sample can be drastically reduced and throughput greatly increased (105, 106). Combining the analysis of long- and short-read data in an aggregated form has been a useful approach for diagnostic and public health-oriented WGS applications, combining the advantages of both technologies for outbreak and phylogenetic analyses, resistance and virulence prediction, and genomic pathogen surveillance (107–109). Long-read sequencing using nanopore-based technologies with software optimization reaches high accuracy levels that may equal those of short-read sequencing data, removing the need for the latter for applications such as determination of bacterial drug susceptibility and delineation of transmission clusters in outbreak investigations (104). Recent technological advancements such as ultra-rapid genomic sequencing, single-cell sequencing, and sophisticated AI/machine learning algorithms for reference-free and stringent data analysis are propelling this field forward (110–114).
Integrating genomic, clinical, and epidemiological data for enhanced surveillance
Sequencing data integration
The utility of pathogen sequencing data significantly increases when it is linked to clinical, phenotypic, or epidemiological data (i.e., “metadata”). Such data linkage between healthcare records in agencies and institutions can improve health outcomes and the effectiveness of public health surveillance—delivering clinical, public health, and economic benefits (115–118). For example, genomic data sharing has illuminated multi-jurisdictional outbreaks and cross-border spread of communicable diseases (11, 63, 119, 120).
Figure 4 outlines the integration of laboratory, clinical, and epidemiological metadata with pathogen sequencing data obtained from clinical samples using different sequencing approaches. The sequencing data include files generated by sequencing instruments (i.e., “raw” sequencing data stored as fastQ files) and inferred by bioinformatics pipelines (e.g., consensus genomes stored as fastA files, variant files, or network representation files). The genomic data are accompanied by laboratory findings obtained from other frontline diagnostic tests on the sample and stored in the laboratory information systems. For example, the genomic sequence of M. tuberculosis should be linked to results of associated phenotypic antimicrobial susceptibility testing (83). Other types of metadata include case demographics, clinical diagnoses with co-morbidities coded using international standards, past exposures to infected cases, treatment outcomes, and use of healthcare resources (e.g., hospital admissions and/or vaccinations). There is also a case for integrating disease occurrence metadata into relevant and validated phylogenetic models to improve epidemiological inferences from pathogen genomes (65). Occurrence metadata reflect background prevalence of the infection or disease and capture cases diagnosed by frontline diagnostic tests but without accompanying sequenced genomes and the time of their diagnosis.
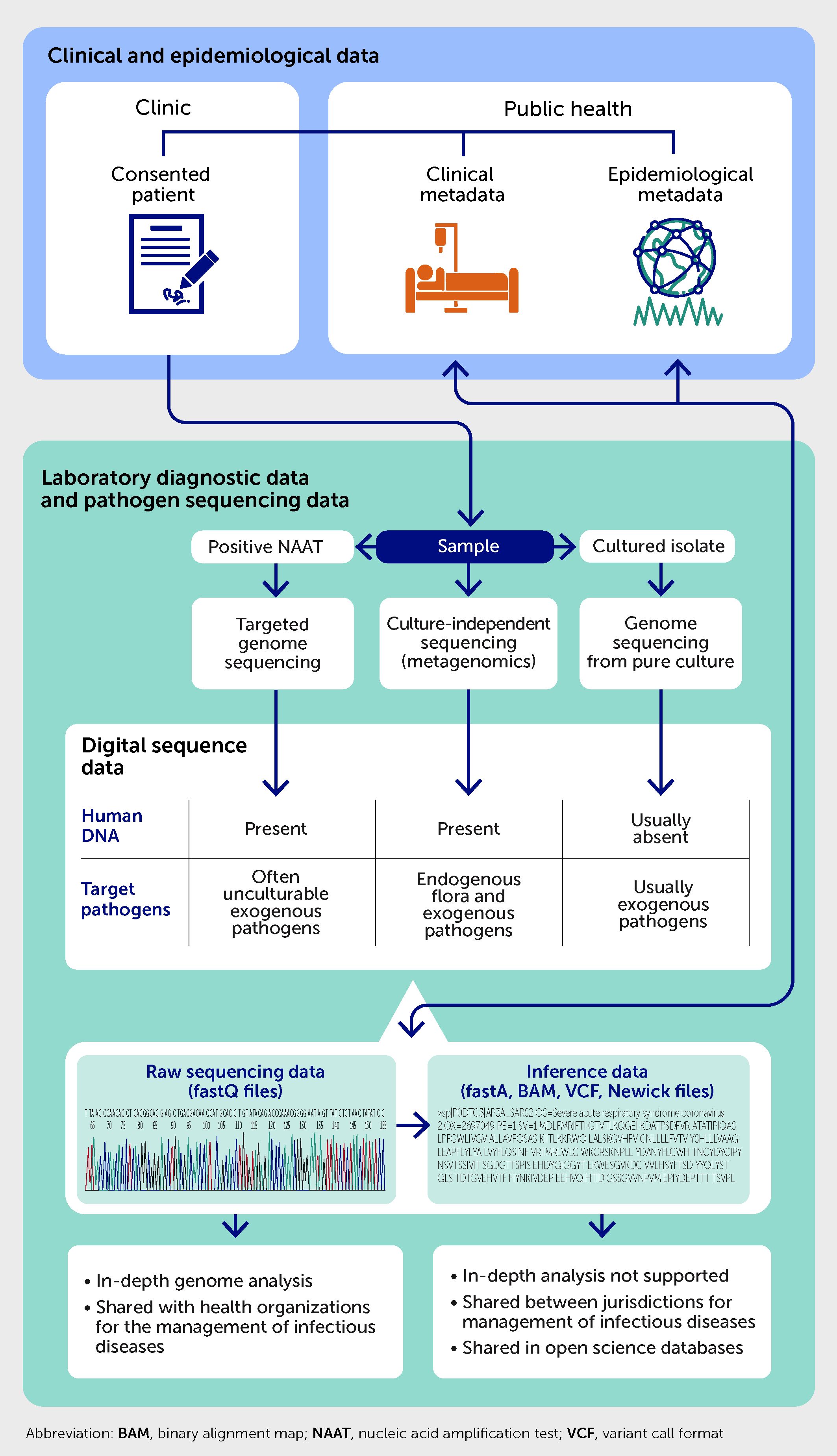
Figure 4 Integration of laboratory, clinical, and public health metadata with pathogen genome sequencing data.
The amount of microbial sequencing data generated in clinical, public health, and research laboratories has been rapidly expanding. Even before the COVID-19 pandemic, it was suggested that genomics would produce more data than social media, earth sciences, or astronomy (121). These projections have been fulfilled by the immense volumes gathered by the ongoing genomic surveillance of SARS-CoV-2 variants. Open databases such as GISAID now contain over 16 million SARS-CoV-2 genomes recovered from cases across all continents.
Data integration requires digital infrastructure for the collection, storage, and linkage of sequencing data and sample-associated metadata, as well as the sentinel and syndromic disease surveillance data produced across national and international networks (122, 123). As the data are produced by multiple pathology and clinical service providers, federated data storage solutions are usually employed and optimized for data heterogeneity and volume (e.g., genomic data warehouses). The portability of de-identified and often encrypted health data uploaded to the cloud can provide significant leverage to a growing number of data users interested in secondary analyses of the pathogen sequencing data for infectious disease management, prevention, and research. These analyses demand large-scale data aggregation and the use of third-party algorithms and tools designed for users with different backgrounds (39, 64).
Integration and responsible sharing of sequencing data should be governed by the findability, accessibility, interoperability, and reusability (FAIR) principles of Open Science. The core elements of such data sharing include transparency regarding the purpose, collection, use, and exchange of data; data quality, standardization, and security; and recognition and attribution, thereby fostering trust, integrity, and reciprocity. The sharing and mobilization of data empowers research and development, but genomic data generated and shared by data donors must also comply with relevant local policies and regulations.
Design of national genomic surveillance systems
The uptake and success of national genomic surveillance systems depends on the access to sequencing and data sharing across different sectors and jurisdictions. The main success factors are metadata and sample volume, density of sequencing, and timeliness of sequencing and data sharing.
The more contextual metadata are linked to genomic sequencing data, the greater the accuracy of a surveillance signal (124) and the potential value of such combined data for decision-making (125). The value of sequencing data is also directly related to the volume of shared data available for analysis. Larger sets of shared genome sequences are more representative of current disease activity and are more likely to allow the identification of clusters of infections with a common source. For example, for every 1000 genomes of foodborne bacteria (e.g., Listeria monocytogenes) added to a database, there is a reduction of approximately six cases of illness per pathogen per year (126). The utility of pathogen sequencing data is also a function of sequencing density (i.e., the proportion of cases of a disease from which genomes of relevant pathogens are sequenced and shared) and the timeliness of data sharing (Figure 5). The shorter the time between outbreak onset and the sharing of pathogen sequences associated with the outbreak, the higher the utility of these sequences for infection control and public health response. Density of sampling reflects the local or national ability to sequence target genomes in positive clinical samples from a representative proportion of diagnosed cases. The sequencing density varies for different diseases and can be limited by access to positive clinical samples and genome-sequencing technology.
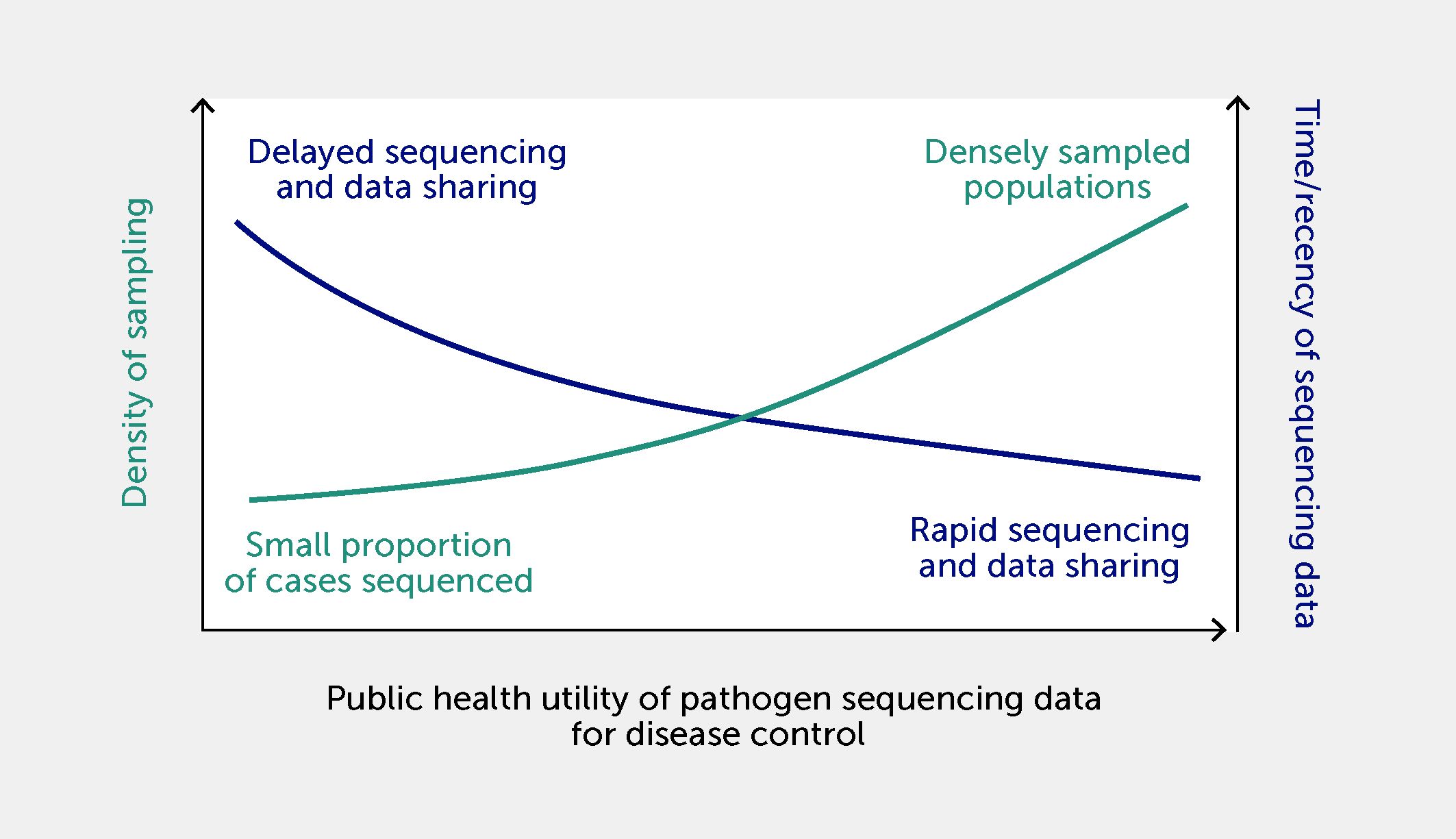
Figure 5 The relationship between density of disease sampling for sequencing, timeliness of sequencing data sharing, and utility of data for infection control and public health responses. Utility is increased by a shorter time between outbreak onset and the sharing of pathogen sequences associated with the outbreak, and by a high density of sampling (i.e., a higher proportion of sequencing of positive clinical samples from diagnosed cases within a population).
New applications continue to emerge to exploit the ultimate resolution offered by WGS, such as the detection of emerging pathogen variants or mixed infections (127). Nevertheless, the design of genomic surveillance systems should be guided by required sensitivity, specificity, and timeliness, as well as by national capacity in pathogen sequencing, to match local public health response capacity and to address specific operational public health objectives. The detection of clusters in an outbreak can be delayed if sampling density is limited and few cases are sequenced. The resolution of outbreak detection necessary to support public health responses can be estimated using both real and simulated genomic data. This approach informed the design of SARS-CoV-2 genomic surveillance sampling strategies in countries with adequate SARS-CoV-2 polymerase chain reaction (PCR) testing and sequencing capacity: simulations demonstrated that the impact of sequencing fewer cases depends on the size of the outbreaks, and on the genetic and spatiotemporal similarity of index cases of the outbreaks (128). Furthermore, these assessments can quantify the minimum number of sequenced cases required to detect and monitor new virus variants, while also considering representative sampling of cases and potential sampling biases. Insufficient timeliness of genome sequencing and reporting was found to be a frequent limiting factor for practical use of SARS-CoV-2 genomic data to inform transmission control interventions in acute healthcare settings (129).
The design of laboratory surveillance systems should balance national needs of data synthesis and response coordination with jurisdictional priorities for informing local public health responses and managing organizational data assets (57, 130). In this context, genomic data and metadata sharing often revolves around existing health data protection regulations. While some successful national systems are very centralized, others are based on decentralized primary bioinformatics analyses, open-source cloud-hosted shared pipelines, and restricted exchange of sequence and metadata between federal member states as well as across sectors—with shrinking data exchange volume from local to federal levels (131).
Benefits and challenges of pathogen sequencing data sharing
Benefits of genomic data sharing include more efficient public health surveillance (enabling better targeted and nuanced interventions or “precision public health”), the ability to examine local data in the global context in real time, and opportunities for research and development from data re-use (4, 12, 132, 133). The risks of data sharing are largely of concern to data donors and relate to patient privacy, confidentiality, and data protection and security. Although the risk of re-identification of patients from publicly shared health data is considered low, the risk assessment does not merely depend on the statistical probability of record attribution; it is also affected by the sensitivity of the data. For example, for COVID-19—a condition with a relatively small degree of potential invasion of privacy—the risk of re-identification of <10% has been considered acceptable. For high sensitivity data (e.g., associated with sexually transmitted infections) some guidelines recommend a threshold of 5% (134). Re-identification risks can also be reduced by the removal of human-like DNA sequences from the microbial sequence files before archiving them in any public database. Sequencing data donors have also been increasingly concerned about legal and ethical implications of incidental findings by data recipients, misuse of data, profiteering from re-use of data with limited attribution of intellectual property, and a lack of accountability among data recipients owing to distributed and unpredictable data re-use (135, 136).
National agencies are responsible for collating and mobilizing public health data to improve population health, as data hoarding and poor data stewardship can hinder genomic surveillance (137, 138). However, the collation and storage of extensive metadata for individual genomic results can be laborious and escalates the consequences of data security breaches. To minimize such risks and costs, only minimal sets of metadata are usually shared with microbial genomes, especially in publicly accessible databases. For example, for SARS-CoV-2 genomic surveillance, minimum contextual data could consist of the patient’s age (often displayed in 10-year intervals), gender, sample collection date, geographical region of collection, and the variant/lineage designation of the microorganism. Such sharing of microbial genomic data and metadata associated with diseases affecting humans and animals, and involving cases across different jurisdictions, requires trust and collaboration between all relevant sectors (i.e., human and animal health, food, and environment) and stakeholders (e.g., government, commercial, and not-for-profit entities) (139).
Consensus-based guidelines are needed to ensure responsible integration and mobilization of pathogen sequencing data, in accordance with ethical and legal requirements that guarantee equal and fair distribution of risks and benefits without an increase in global inequality. The anticipatory use of integrated data analysis for comprehensive disease surveillance and awareness will facilitate better informed and more efficient mitigation of the risk and impact of future pandemics.
Strengthening One Health genomic surveillance
One Health collaborations
“One Health” is an integrated, unifying approach that aims to balance and optimize the health of people, animals, and the environment (140). Both “One Health” and “Planetary Health” concepts have gained prominence as we acknowledge how people, animals, plants, and our environment are interconnected and interact, and how changes to ecosystems can create emerging threats with serious consequences for our health. The COVID-19 pandemic exemplified the importance of using a One Health approach to prevent, predict, detect, and respond to emerging global zoonotic health threats. SARS-CoV-2 is an example of a zoonotic pathogen (2) and human–animal and animal–human transmission events were detected during epidemic waves, e.g., in Denmark (141). Indeed, the majority of emerging pathogens are zoonotic and arise or originate from an animal source or reservoir. WGS has shown diagnostic value for early warning of potential cross-species spillover events of zoonotic pathogens, such as avian influenza (142, 143). A One Health approach is vital for ensuring infectious disease preparedness. To achieve this goal, developing and deploying “agnostic” or broad-range targeted metagenomic sequencing of samples from atypical diseases in humans and animals as well as from their environment has been advocated as the most promising One Health preparedness strategy for detecting the emergence of novel Disease X (99, 144).
AMR is also an archetypical One Health issue by nature. A recent, mixed-method study estimated that 4.95 million (95% uncertainty interval 3.62–6.57) deaths were associated with bacterial AMR in 2019 globally, including 1.27 million (0.911–1.71) deaths attributable to bacterial AMR (3). Excessive use of antibiotics in healthcare, animal husbandry, and aquaculture all contribute to the increasing levels of AMR observed worldwide in many pathogens. Indeed, globally, two thirds of antibiotic usage is agricultural (i.e., for farm animals and crops) (145). Moreover, resistant organisms and genetic determinants of AMR spread across ecosystems—i.e., between animal and environmental reservoirs and human populations via various pathways (146). A One Health approach to AMR surveillance and control is therefore vital (Figure 6). It requires an improved understanding of these pathways and how they can be tackled. It is also crucial to understand how environmental pressure gives rise to new resistance mechanisms.
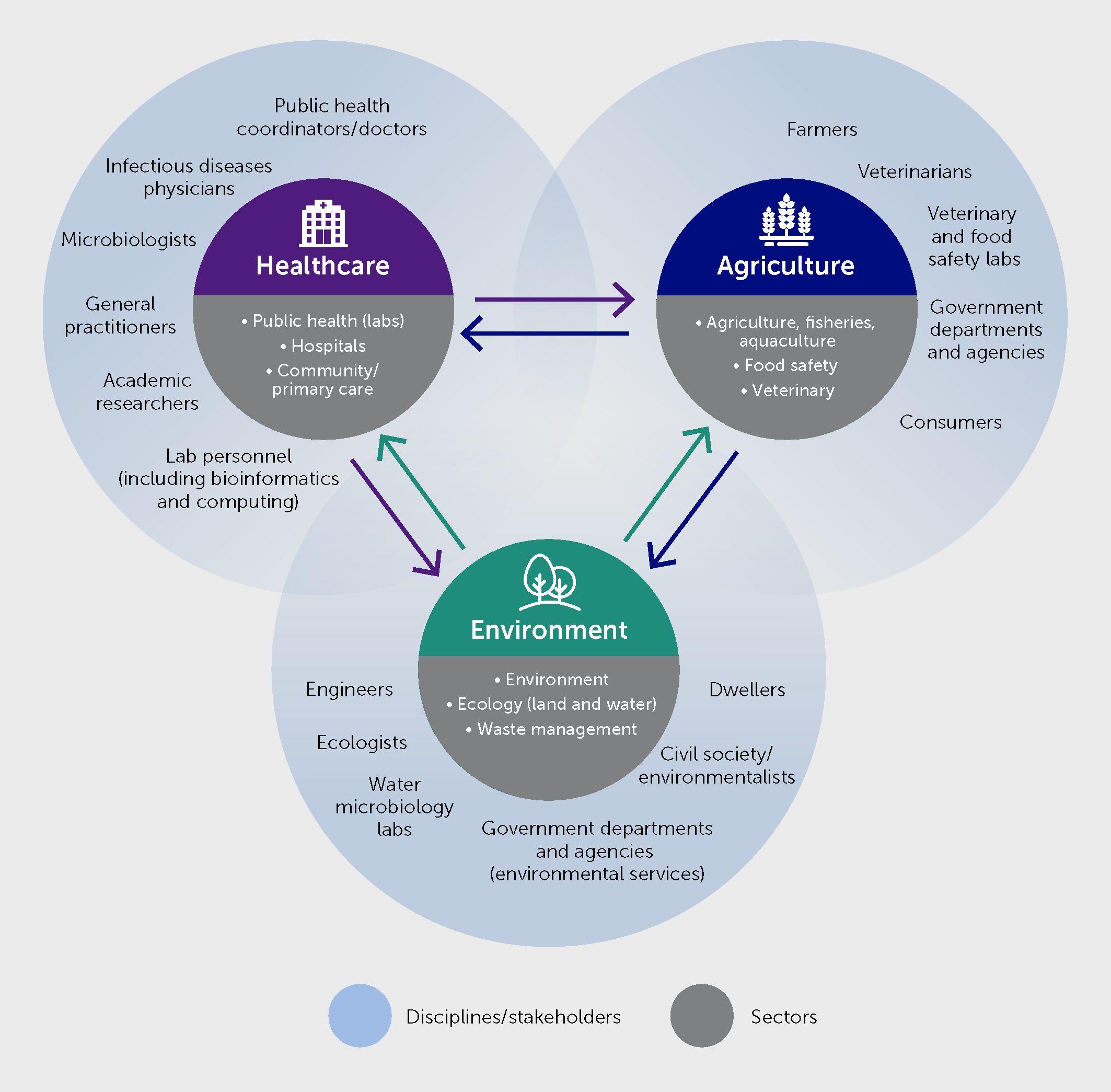
Figure 6 Multisectoral and multidisciplinary partners’ involvement for infectious diseases and antimicrobial resistance (AMR) surveillance and control under One Health.
The One Health approach mobilizes multiple sectors, disciplines, and communities at various levels of society to work together to understand and address the root causes of disease and generate long-term sustainable solutions. Conceptually, a global One Health collaboration has been formalized by WHO, the Food and Agriculture Organization of the United Nations (FAO), the United Nations Environment Programme (UNEP), and the World Organization for Animal Health (WOAH) (147). This “One Health Quadripartite” aims to support multisectoral approaches to reduce health threats at the human-animal-ecosystem interface, coordinate and engage partnerships, and facilitate interactions of the multiple objectives with a central goal. Moreover, the regional centers for disease control in many WHO regions have already adopted One Health programs for disease surveillance, e.g., the United States CDC (148), ECDC (149), and Africa CDC (150). In Europe, European Union (EU) agencies across relevant sectors have established a One Health cross-agency task force (151, 152). At the country level, strengthening the intersectoral collaboration and communication between different sectors and actors is also paramount. Countries are encouraged to work together on designing and improving their One Health approaches by sharing experiences and best practices.
Harmonizing methods and applications across sectors
Figure 6 illustrates how One Health genomic surveillance needs to span across sectors. Several separate surveillance schemes for human and animal pathogens are well established. In contrast, the sharing and usage of surveillance data across the sectors is less well explored, even though various models exist to harness high-throughput sequencing technology for integrated surveillance. FAIR principles can guide improvements in the sharing and usage of data across sectors.
Pathogens and AMR determinants can often be directly detected using the same molecular methods regardless of whether the sample is from a human, an animal, or the environment. This is particularly relevant to metagenomic pathogen surveillance (99, 153). This applies to methods used in bioinformatic pipelines and phylogenetic analyses. However, sample collection, preparation, and the interpretation of results may require specific approaches and knowledge depending on the surveillance objective. Harmonizing methods requires—and supports—cross-sectorial collaborations. Application of the same methods in laboratories across sectors and for different sample types, as well as the establishment of One Health laboratories, supports the adaptability of these systems to new pathogens and may provide opportunities for flexibly redirecting or scaling up capacities.
Harmonizing surveillance methods across sectors enables the generation of comparable and interoperable data from different sources. Nevertheless, sharing and using data for surveillance across sectors often requires an understanding of relevant intersectoral differences, for example regarding biological characteristics of hosts and pathogens, sampling strategies, and terminology (154).
In the United States, real-time genomic surveillance programs for foodborne pathogens have been developed in the last decade to support food safety and public health, including the GenomeTrakr program operated by the Food and Drug Administration (FDA) and the PulseNet program coordinated by the CDC (126, 155). Similar national programs for One Health real-time genomic surveillance of foodborne infections have also been successfully developed in other areas, including Europe and Australia (50, 119). These programs have demonstrated enhanced sensitivity over traditional surveillance for early outbreak detection and have proved effective, e.g., in preventing foodborne infections caused by Salmonella (119, 156), L. monocytogenes (157), and Shiga toxin-producing Escherichia coli (STEC) (158). They also showed cost-effectiveness when optimized WGS technology was used appropriately (159).
Wastewater surveillance
Wastewater surveillance is a timely example of an approach that can be a cost-effective, complementary component of integrated One Health surveillance of infectious diseases and AMR. Wastewater molecular analysis by PCR and metagenomic sequencing can provide a variant-specific, community-representative picture of human pathogen prevalence and early warning of local spread, as shown for SARS-CoV-2, influenza, mpox, and vaccine-derived polio viruses (153). Wastewater surveillance for mapping SARS-CoV-2 distribution and the magnitude of the infected population exemplifies how rapidly changing pathogens can be tracked across large populations (160). Wastewater-based surveillance has been shown to be useful at time points with less extensive testing, providing a quick overview of the geographical spread of, e.g., a new SARS-CoV-2 variant (8). After having been widely applied for the surveillance of SARS-CoV-2 in numerous countries, it is time to focus on harmonizing the approaches and widen the use of the approach to other pathogens, including emerging new pathogens and variants (153). In Europe, a large consortium (EU-WISH) with this aim was launched in 2023.
Wastewater surveillance has also been shown to be a promising tool for broad range monitoring of bacterial AMR. Using metagenomic analysis of AMR gene distribution in urban sewage around the world, Hendriksen et al. observed that local sanitation standards and healthcare conditions correlate with the abundance and type of AMR determinants (90). This mapping of local resistome profiles has expanded to over 100 countries (161). Such a metagenomic epidemiological approach measures the collective signature of microbial carriage across a community. However, many aspects are still unresolved. Current technology is not clear in terms of sensitivity, specificity, and plausibility. Since AMR pathogens may also propagate and live as saprophytic bacteria in the aquatic environment and sewage, wastewater surveillance of AMR fundamentally differs from that of viral pathogens. Therefore, there are still challenges in developing standardized and optimized methodologies for wastewater AMR metagenomic surveillance and in interpretation of data for conducting quantitative microbial risk assessment.
Genomic surveillance implementation: challenges and perspectives
How robust is the business case for transitioning to genomic surveillance for public health? Assessments of the health and economic impacts of WGS use for routine pathogen surveillance have focused on selected foodborne infections, healthcare-associated infections, multidrug-resistant bacteria, tuberculosis, influenza, and SARS-CoV-2 (47, 83, 84, 118, 126, 129, 143, 157, 159, 162–164). Systematic reviews indicate that these evaluation studies have predominantly addressed single pathogens, measured outbreak detection outputs, used heterogeneous observational study designs, were limited to high income countries, and largely ignored indirect impacts on healthcare systems and costs (159, 162, 164). While all studies supported the use of pathogen WGS for surveillance, the available evidence of its cost-effectiveness is still limited and of low strength and generalizability (162, 164). An economic and health impact modelling study for England and the United States suggests that implementing extensive WGS surveillance of priority healthcare-associated infection pathogens in acute care institutions would substantially reduce morbidity and mortality while achieving healthcare cost savings in both countries (163).
Comparisons of WGS approaches with other approaches and evaluation of One Health-ness of integrated methods can inform the selection of approaches best suited to different settings. These need to be harmonized, scalable, and robust; for some settings, genomic surveillance approaches may not yet meet these requirements. Genomic surveillance can provide helpful information to better design and assess public health policies, including for drug treatment and vaccination. In the context of infections that are increasingly difficult to treat, caused by multidrug-resistant pathogens, findings from genomic surveillance can help guide empirical antimicrobial treatment and evaluate its medium-term impact on resistance prevalence. For example, the genomic analysis of the Neisseria gonorrhoeae population structure over several years by the European Gonococcal Antimicrobial Surveillance Programme showed the decrease in prevalence of an epidemic lineage resistant to extended-spectrum cephalosporins after changing treatment recommendations for gonorrhea with cefixime to a combination of ceftriaxone and azithromycin (165). Likewise, genomic surveillance studies are increasingly used to monitor and map the prevalence of virulent bacterial lineages and vaccine escape variants to assess vaccine effectiveness and guide immunization policies against S. pneumoniae (78), N. meningitidis (80), and B. pertussis (82).
A structured evaluation framework has been proposed to measure the public health impact of genomic surveillance interventions, assess its local determinants, and guide stepwise implementation (166). Further real-world evaluation of One Health gains and cost-effectiveness of genomic surveillance programs are needed across a wider range of surveillance objectives, pathogens, health sectors, population settings, and income levels.
What is the current state of genomic surveillance implementation worldwide and what are the obstacles to overcome? One Health genomic surveillance is currently expanding globally in pathogen scope, surveillance objectives, policy use, and geographical coverage, albeit at different speeds between countries. Unfortunately, few global genomic surveillance programs allow for measuring uptake and performance over time by pathogen, objective, country, and laboratory. This gap is largely due to a lack of widely used public sequence data repositories outside SARS-CoV-2 and influenza GISAID databases.
Nevertheless, evidence from regional and national implementation surveys indicates that WGS is increasingly used in routine public health surveillance worldwide. In Europe, national and regional genomic surveillance is gradually becoming mainstream for monitoring pathogens that are prioritized through participative development of EU/EEA consensus strategies (10, 59). Early adoption started in 2014 in higher income countries, with strong collaboration between well-resourced genomic research communities and public health and food safety laboratories (43, 131, 156, 157), and this expanded further to many other countries within a few years (50). Despite the pressing focus on SARS-CoV-2, the number of countries routinely using WGS for national surveillance of other pathogens continued to increase between 2018 and 2021 in the EU/European Economic Area (EEA), as reported in the EULabCap monitoring system (167). In 2021, national or subnational reference laboratories from 25 EU/EEA countries (86%) used WGS for routine surveillance of at least one pathogen, including more than half (16 countries) using the technology for multidrug-resistant M. tuberculosis surveillance and around two thirds for L. monocytogenes and N. meningitidis surveillance (18 and 20 countries, respectively) (167).
Genomic surveillance for public health and food safety is also routine in North America. Since 2019, real-time genomic surveillance of foodborne pathogens such as Campylobacter, STEC, Salmonella, Vibrio, and Listeria has been implemented in all state and provincial public health laboratories within the PulseNet programs in the United States (https://www.cdc.gov/pulsenet/index.html) and Canada (https://www.canada.ca/en/public-health/programs/pulsenet-canada.html). These surveillance programs allow for high-sensitivity outbreak detection and One Health investigation of foodborne infections combined with the GenTrackr genomic monitoring system for source tracing in the United States food sector (126). Progress in this area has been slower in other regions of the world. Following the vision to extend genomic surveillance programs throughout the PulseNet International network (168), a survey of public health laboratories from 33 countries indicated that only 8% used WGS for routine surveillance of foodborne pathogens in 2020, ranging from none in Africa and the Middle East to less than 10% of laboratories in the Asia-Pacific, Latin American, and Caribbean regions (169). The main implementation barriers in these laboratories included insufficient funding and gaps in bioinformatic expertise and tools. Among priority needs for further onboarding of the technology was the provision of a software toolkit that is free, standardized, and validated for WGS data management, analysis, visualization, reporting, and sharing within a global database (169).
Regarding AMR genomic surveillance, more than half (16) of EU/EEA countries were already using WGS for routine characterization of carbapenemase-producing Enterobacterales at the national reference laboratory level in 2018 (170). As participants in European-wide networks, such as European Antimicrobial Resistance Genes Surveillance Network (EURGen-Net) and the Epidemic Intelligence Information System, these reference laboratories routinely share and analyze genomic and epidemiologic data via the ECDC EpiPulse platform (120, 167). Multicenter AMR genomic surveillance networks have been successfully established across regions, including in Australia (47), Colombia, India, Nigeria, and the Philippines (54, 171).
Building global governance and international integration of pathogen genomic surveillance
The COVID-19 pandemic underscored that infectious diseases are a global threat requiring a coordinated and global preparedness-and-response paradigm based on surveillance with equitable implementation, including in lower- and middle-income countries (1, 2, 172). In this section we provide a framework for the governance and integration of pathogen genomic surveillance into this paradigm (Figure 7).
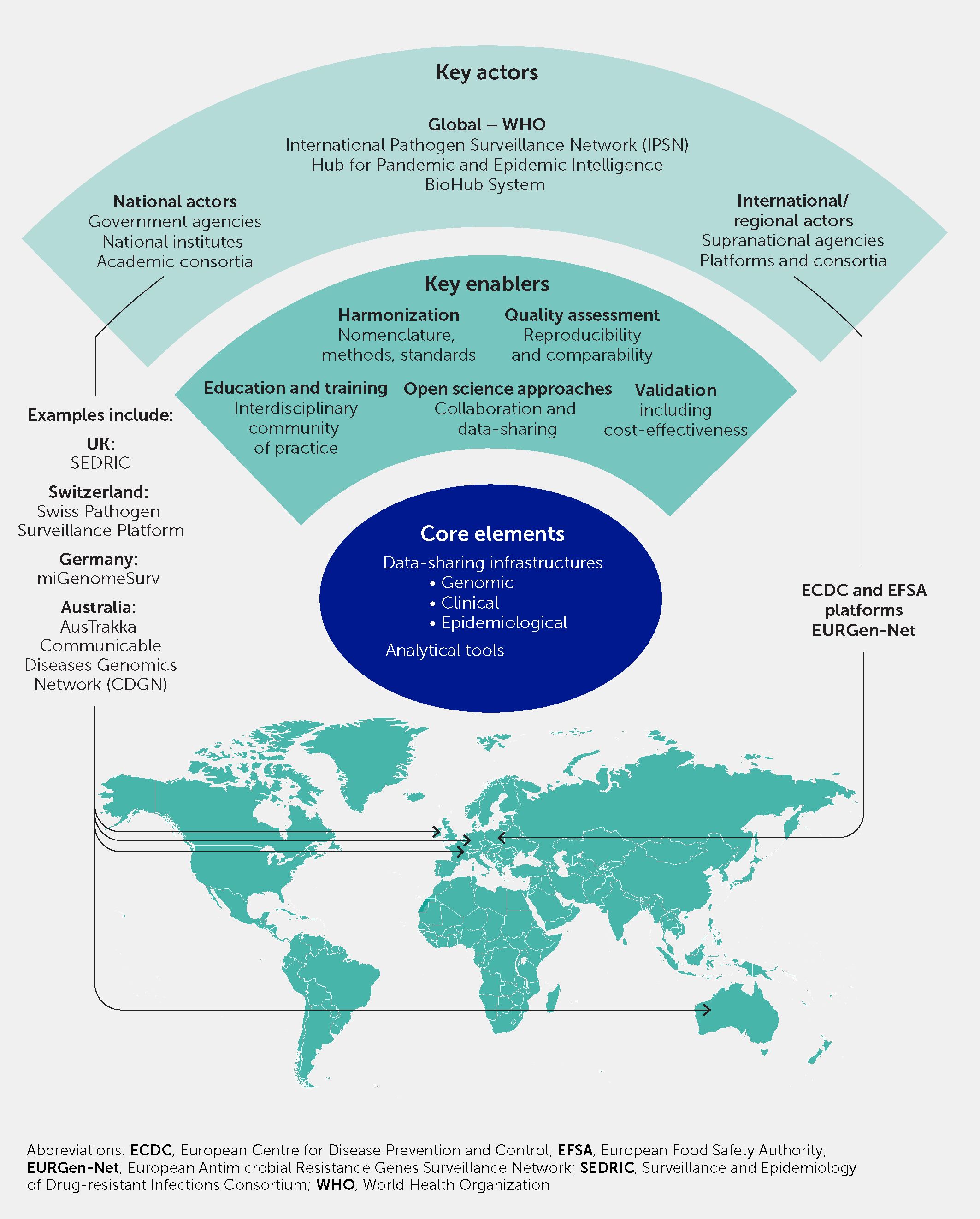
Figure 7 Global governance and international collaboration and harmonization is necessary to embed pathogen genomic surveillance into scalable responses to infectious diseases and antimicrobial resistance. This schematic illustrates the key actors, enabling factors, and core elements, with examples of existing initiatives and platforms that offer a basis on which to build.
Strengthening global governance and resources for pathogen genomic surveillance
As discussed above, international genomic surveillance during the COVID-19 pandemic was rapidly achieved through the release of SARS-CoV-2 data into the GISAID database. Additional tools enabling real-time genomic surveillance of COVID-19 at a global level include the public availability of sequence-based consensus nomenclature, such as Pango Lineages databases (https://cov-lineages.org) (68) for labeling and open access analysis, and visualization tools (e.g., www.nextstrain.org) for tracking viral phylogenetic lineages and variants. Consensus nomenclature was established by WHO with a multidisciplinary panel of experts to define and label SARS-CoV-2 genomic variants of interest and variants of concern, based on increased virulence, transmissibility, or immune escape (https://www.who.int/en/activities/tracking-SARS-CoV-2-variants/). Similar sets of publicly available databases and scientific resources are available for global genomic surveillance of other pathogens, including microbial genome databases such as PubMLST, which provides a gene-by-gene analysis-based strain nomenclature (173). Interactive online platforms and bioinformatics toolboxes such as Pathogenwatch (https://pathogen.watch/) and the Center for Genomic Epidemiology (https://www.genomicepidemiology.org) have been developed for integration and visualization of bacterial genomic and epidemiological data, and for the identification of high-risk epidemic clones and lineages (174).
We propose that the worldwide development of routine genomic surveillance for SARS-CoV-2 needs to be expanded to other epidemic-prone pathogens, for the tracking of AMR mechanisms, and for future pandemic preparedness. Clearly, this effort would require wider global public health and One Health commitment and agreement on technical standards for the responsible, voluntary, and secure sharing of coherent genome and health data beyond SARS-CoV-2 (175). Demonstrating the positive health impact and cost-effectiveness of local, national, and international genomic surveillance for different pathogens and AMR threats will help guide what data need to be collected and shared in a timely manner.
Recently, WHO, in line with the World Health Assembly Resolution 74.7, enacted governance principles for international genomic surveillance data sharing as part of a 10-year strategy for global pathogen genomic surveillance for epidemic preparedness (130). Novel resource centers have been established recently to support implementation processes, e.g., the WHO Hub for Pandemic and Epidemic Intelligence and the WHO BioHub System (https://pandemichub.who.int/pandemic-hub) for international sharing of biological samples (https://www.who.int/initiatives/who-biohub). Academic, public health, and non-governmental organizations should work in sharing best practices and technical solutions toward supporting the equitable deployment of pathogen genomics capabilities through collaborative initiatives at the regional and global levels, including well-established regional public health surveillance networks (167, 176) and the recently established International Pathogen Surveillance Network (IPSN) (177).
WHO has long-established flexible AMR phenotypic data reporting tools (e.g., WHONET 2022) for the Global Antimicrobial Resistance and Use Surveillance System (GLASS). They published an expert opinion on potential approaches to further integrate reporting of WGS data on AMR determinants into GLASS (55). A number of WHO Collaborating Centres for Antimicrobial Resistance Epidemiology and Surveillance (https://www.who.int/about/collaboration/collaborating-centres) support the technical implementation of the WHO Global strategic action plan on AMR and GLASS national programs.
Key efforts to build new public health infrastructures and processes for using actionable outputs from genomic surveillance should focus on enhancing the understanding of clinical and public health practitioners and health officials across sectors on how to interpret genomic epidemiology findings and translate them into action. First, this should include the development of novel training curricula and programs for surveillance data end-user health professionals as well as advanced meta-disciplinary specialty training for the new generation of genomic surveillance experts, such as genomic epidemiologists and public health bioinformaticians. Second, efforts should promote further collaboration and dialogue among virologists, microbiologists, evolutionary biologists, environmental scientists, epidemiologists, and public health and animal health practitioners into a transdisciplinary One Health community of practice. Third, connections should be strengthened between surveillance networks and open data research platforms hosting curated databases of genomic sequence data and interactive bioinformatic nomenclature assignment resources for specific viral, bacterial, fungal, and parasitic pathogens of public health importance. Fourth, external quality assessment and interlaboratory ring tests should be implemented to ensure reproducibility and comparability of derived consensus sequence data and assembly pipelines. It is key that these multilateral and multidisciplinary collaboration efforts build on the existing disease-specific research and surveillance networks, and that they benefit from close interaction with the cognate scientific as well as professional healthcare community (10, 57, 59).
Strengthening international partnerships and method harmonization
Network of networks
Several new international networks have been established to strengthen genomic pathogen surveillance. For example, several One Health initiatives have been established in Europe that closely collaborate with the Health Emergency Preparedness and Response Authority (HERA). Building links between such initiatives (e.g., DURABLE, EU-HIP, and EU-WISH) will support synergies and avoid duplication of efforts. Moreover, specific EU-funding has been allocated to strengthen national One Health integrated surveillance capacities (e.g., UNITED4Surveillance and OH4Surveillance).
Foodborne pathogen genomic surveillance.
Progress toward a global vision of international genomic data sharing for rapid, coordinated public health responses (168) has so far been achieved at the regional level, in particular for foodborne pathogens. In the EU, platforms for the sharing and analysis of standardized WGS data from national monitoring of foodborne pathogens by reference laboratories have been developed by the European Food Safety Authority (EFSA) for food isolates and the ECDC for human isolates. The ECDC supports Epidemic Intelligence Information System (EPIS) collaborations for foodborne pathogens and diseases, through which epidemiologists can securely share case data, and national reference laboratories can share and analyze genomic data via the EpiPulse platform (https://www.ecdc.europa.eu/en/publications-data/epipulse-european-surveillance-portal-infectious-diseases). The EU agencies collaborate with member state authorities to conduct genomics-informed, joint investigations of cross-border foodborne outbreaks and the identification of environmental reservoirs, food vehicles of infection, and potential contamination sources by combining surveillance data from different health sectors (59, 178, 179). In this way, they contribute in near real-time to international outbreak alerts and cross-sectoral rapid risk assessment to inform coordinated risk mitigation options. Developing further governance agreements and surveillance method harmonization will allow broadening these surveillance and alert systems into intersectoral global networks. New opportunities for One Health integration between human and animal health surveillance are opening, for example with the launch of the Animal Health Information System (WAHIS) by the WOAH (https://www.woah.org/en/oie-wahis-a-new-era-for-animal-health-data/).
AMR genomic surveillance
Even though AMR containment frameworks and action plans are in place in many countries, international collaborations are essential to address the cross-border spread of epidemic multidrug-resistant pathogens. Additionally, many low- and middle-income countries lack the necessary laboratory infrastructure and require support to achieve the goals stipulated in WHO strategies. Regional efforts to facilitate international genomic surveillance of epidemic AMR threats have been initiated. Pan-European genomic surveys of multidrug-resistant pathogens such as carbapenem-resistant Enterobacterales have provided baseline maps of their epidemic clonal structure and resistance plasmid spread in healthcare settings (107). Currently, the ECDC coordinates the EURGen-Net, which brings together reference laboratories from 37 European countries. The network has established centralized surveillance protocols and uses a joint platform for analyzing national genomic surveillance data on high-risk multidrug-resistant bacteria, such as carbapenemase-producing Klebsiella pneumoniae, for cross-border tracking of emerging epidemic clones (49, 52, 59, 120, 180).
Additional support, including from charitable foundations, is needed to enhance and harmonize training and data collection methods for real-time surveillance and timely provision of feedback on AMR threats. In the United Kindgom, the Surveillance and Epidemiology of Drug-resistant Infections Consortium (SEDRIC, www.sedric.org.uk) brings together experts to provide technical analysis, advice, and AMR advocacy. The Consortium aims to use these experts’ research outcomes to identify knowledge gaps and solutions that will influence changes in policy, practice, and funding in surveillance and epidemiology of drug-resistant infections. The SEDRIC Genomic surveillance Working Group focuses on data standards and harmonization for AMR surveillance. A laboratory information management system currently being piloted provides specimen management, bench workflows, result reporting, and automated and manual analysis of AMR data, together with an interface enabling local hospital systems to report to GLASS through National Focal Points via WHONet software.
Vaccine-preventable disease surveillance
As discussed above, global data standardization and sharing of One Health genomic surveillance data is well advanced for influenza and SARS-CoV-2, following the extensive use of the GISAID database and public sequence repositories together with lineage nomenclature open resource tools. Rapid progress has also been made toward standardized global genomic surveillance for tracking the population dynamics of antigenic variants of bacterial pathogens causing vaccine-preventable diseases, such as meningococcal disease, pertussis, and diphtheria. This is supported by scientific efforts to build publicly accessible, phylogenetically robust, global genomic strain and antigen allelic profile nomenclature databases. These surveillance genomic typing frameworks are based on the delineation of bacterial core genomes and design of open resource typing schemes of scalable resolution using selected gene subsets, following the multilocus sequence base typing (MLST) and multilevel genome typing (MGT) approaches (79–82, 181, 182). These genus- or species-specific genomic typing nomenclatures are very flexible in resolution and phenotype prediction, making them suitable for use in a range of public health applications, from global surveillance and prediction of vaccine effectiveness to outbreak investigation (79–82, 182). Future governance agreements based on expert consensus would benefit from using scientifically validated pathogen nomenclature as reporting standards for global surveillance.
Surveillance harmonization and synergies with open science repositories and analysis platforms
Further international collaboration is needed on developing common methods and nomenclature for genomic surveillance of epidemic-prone infectious diseases and multidrug-resistant organisms of public health concern. These efforts encompass the harmonization of epidemiological and environmental sampling schemes for surveillance programs, as well as transparency of bioinformatic analysis pipelines and agreement on microbiological, clinical, and epidemiological data semantics.
Gaps in genomic data management, sharing, and analytic capabilities are common barriers to wider implementation of real-time pathogen genomic surveillance of SARS-CoV-2 and other pathogens, as noted above. These gaps underline the added value of further developing unified and freely accessible bio-informatics ecosystems for public health agencies, first available at national and regional levels, that provide open-source integrated data processing, data sharing, genomic analytics, and reporting tools, including standardized metadata and automation support by application programming interfaces (APIs) (10, 183).
To enhance interpretation of pathogen and AMR genomic surveillance data and their translation into public health risk assessment and mitigation, it is also desirable to facilitate rapid data deposition in public sequence archives, such as the United States National Center for Biotechnology Information (https://www.ncbi.nlm.nih.gov/) and the European Nucleotide Archive (https://www.ebi.ac.uk/). These repositories, in turn, enable data use for research with scientifically curated genomic data platforms and genetic nomenclature resources. This allows inferences to be drawn on pathogen evolution, functional prediction of AMR, virulence, and antigen phenotype and the tracking of lineages causing disease across countries and settings. Currently, genomic sequence data can be directly deposited there using private subsets functionalities. Examples of open science resources include projects such as the K. pneumoniae Global Genomic Surveillance Platform (https://klebnet.org/) and scientific discussion forums, such as the Virological forum (https://virological.org/about), which allow for open analysis and interpretation of virus molecular evolution and epidemiology.
Conclusion
Optimizing WGS technology for genomic analysis of microbial pathogens with epidemic potential has demonstrated its ability to contribute to infectious disease discovery, early outbreak detection, and containment of epidemics caused by different pathogens and AMR. As a community, we must continue to address challenges such as those faced during the COVID-19 pandemic and further invest in the infrastructure required for global integrated One Health genomic surveillance to enable a timely, unified approach to future pandemic and public health threats from infectious diseases and AMR. Infectious disease preparedness covers many aspects where genomic approaches can make a major difference, from detection of outbreaks and the emergence and spread of new variants to informing decisions on interventions, including vaccines and antimicrobials.
As national, regional, and global institutions are building stronger pandemic preparedness systems following the COVID-19 pandemic, these efforts will greatly benefit from expanding pathogen sequencing capacity and the expertise of laboratories in low-, middle-, and high-income countries, together with modernizing health information systems. Progress is needed with pathogen genomic nomenclature standardization, surveillance, and interoperability of healthcare databases. A focus on seamless integration of genomic, microbiological, clinical, and epidemiological data from across sectors is also crucial. International agreements and cross-sectorial collaborations should foster consensus-based, national legislation- and FAIR-compliant, and responsible governance of data sharing within and between surveillance networks and health authorities. Translational research should identify implementation barriers and enablers in diverse settings. Assessment of cost effectiveness of genomic surveillance in reducing disease burden will help support data collection and sharing requirements to demonstrate the overall health and economic benefits. Implementing the collaborative and capacity-building steps outlined above equitably across the world, ushering in full-scale genomic surveillance of major human and zoonotic pathogens, will enhance our global epidemic intelligence and help curtail future pandemics.
Acknowledgments
The authors thank the following members of the European Society for Clinical Microbiology and Infectious Diseases Study Group on Public Health Microbiology (ESGPHM) and Study Group on Epidemiological Markers (ESGEM) for their critical review of the manuscript: ESGEM: Sylvain Brisse (France), Natacha Couto (UK), and ESGPHM: Surabhi Taori (UK).
Statements
Author contributions
MS: Conceptualization, Investigation, Methodology, Project administration, Visualization, Writing – original draft, Writing – review & editing. CL: Investigation, Visualization, Writing – original draft, Writing – review & editing. GW: Investigation, Visualization, Writing – original draft, Writing – review & editing. VS: Investigation, Visualization, Writing – original draft, Writing – review & editing. PJ: Investigation, Visualization, Writing – original draft, Writing – review & editing. MI: Investigation, Visualization, Writing – original draft, Writing – review & editing.
Data availability statement
The original contributions presented in the study are included in the article/supplementary material. Further inquiries can be directed to the corresponding author.
Funding
The author(s) declare financial support was received for the research, authorship, and/or publication of this article.
MJS was employed as Chief Microbiologist at ECDC, 2010–2020.
CL was employed as Director of Operations for COG-UK, 2020–2022. She is Head of Clinical Research Interface at Genomics England.
GW receives a grant from the Federal Ministry of Health to run the National Reference Centre for Staphylococci and Enterococci (current period: 2023–2025).
VS’s contribution was in part supported by the New South Wales Health Prevention Research Support Program.
PJ’s contribution was supported by the EU Horizon 2020 Research and Innovation Programme under grant agreement 773830 – One Health European Joint Programme, and the EU4Health programme under grant agreements 101140460 – EU-WISH, 101102774 – EU-HIP, and 101102733 – DURABLE.
MI is a Clinical Microbiologist at the Department of Microbiology, Faculty of Medicine, Chinese University of Hong Kong, and the Prince of Wales Hospital, Shatin, Hong Kong. She acknowledges the grant support on “One Health approach to Track and Target Antimicrobial Resistance (2020–2024) (CID_CUHK_C)” from the Health and Medical Research Fund, Food and Health Bureau of the Hong Kong Special Administrative Region.
Conflict of interest
The handling editor EF declared a shared research group ESGEM with the authors at the time of review.
The authors declare that the research was conducted in the absence of financial relationships that could be construed as a potential conflict of interest. As a retired Emeritus Professor, MJS is no longer gainfully employed. Co-authors CL, GW, VS, PJ, MI declare that their employer and funding sources were not involved in the conceptualization, the collection and synthesis of evidence, the writing of this article, or the decision to submit it for publication. Views and opinions expressed do not necessarily reflect those of the funders listed.
The authors MJS, GW, VS, MI declared that they were an editorial board member of Frontiers, at the time of submission. This had no impact on the peer review process and the final decision.
Publisher’s note
All claims expressed in this article are solely those of the authors and do not necessarily represent those of their affiliated organizations, or those of the publisher, the editors and the reviewers. Any product that may be evaluated in this article, or claim that may be made by its manufacturer, is not guaranteed or endorsed by the publisher.
Author disclaimer
Views and opinions expressed are those of the author(s) only and do not necessarily reflect those of the EU or the European Health and Digital Executive Agency (HaDEA). Neither the EU nor the granting authority can be held responsible for them.
References
1. Sirleaf EJ, Clark H. Report of the independent panel for pandemic preparedness and response: making COVID-19 the last pandemic. Lancet (2021) 398(10295):101–3. doi: 10.1016/S0140-6736(21)01095-3
2. Sachs JD, Karim SSA, Aknin L, Allen J, Brosbøl K, Colombo F, et al. The Lancet Commission on lessons for the future from the COVID-19 pandemic. Lancet (2022) 400(10359):1224–80. doi: 10.1016/S0140-6736(22)01585-9
3. Antimicrobial resistance collaborators. Global burden of bacterial antimicrobial resistance in 2019: a systematic analysis. Lancet (2022) 399(10325):629–55. doi: 10.1016/S0140-6736(21)02724-0
4. Oude Munnink BBO, Worp N, Nieuwenhuijse DF, Sikkema RS, Haagmans B, Fouchier RAM, et al. The next phase of SARS-CoV-2 surveillance: real-time molecular epidemiology. Nat Med (2021) 27(9):1518–24. doi: 10.1038/s41591-021-01472-w
5. Cardona-Ospina JA, Rojas-Gallardo DM, Garzón-Castaño SC, Jiménez-Posada EV, Rodríguez-Morales AJ. Phylodynamic analysis in the understanding of the current COVID-19 pandemic and its utility in vaccine and antiviral design and assessment. Hum Vaccin Immunother (2021) 17(8):2437–44. doi: 10.1080/21645515.2021.1880254
6. Spänig S, Eick L, Nuy JK, Beisser D, Ip M, Heider D, et al. A multi-omics study on quantifying antimicrobial resistance in European freshwater lakes. Environ Int (2021) 157:106821. doi: 10.1016/j.envint.2021.106821
7. Lassaunière R, Polacek C, Utko M, Sørensen KM, Baig S, Ellegaard K, et al. Virus isolation and neutralisation of SARS-CoV-2 variants BA.2.86 and EG.5.1. Lancet Infect Dis (2023) 23(12):e509–10. doi: 10.1016/S1473-3099(23)00682-5
8. Rasmussen M, Møller FT, Gunalan V, Baig S, Bennedbæk M, Christiansen LE, et al. First cases of SARS-coV-2 BA.2.86 in Denmark, 2023. Euro Surveill (2023) 28(36):2300460. doi: 10.2807/1560-7917.ES.2023.28.36.2300460
9. Struelens MJ, Brisse S. From molecular to genomic epidemiology: transforming surveillance and control of infectious diseases. Euro Surveill (2013) 18(4):20386. doi: 10.2807/ese.18.04.20386-en
10. European Centre for Disease Prevention and Control (ECDC). Expert Opinion on whole genome sequencing for public health surveillance. Stockholm: ECDC (2016).
11. Gardy JL, Loman NJ. Towards a genomics-informed, real-time, global pathogen surveillance system. Nat Rev Genet (2018) 19(1):9–20. doi: 10.1038/nrg.2017.88
12. Khoury MJ, Armstrong GL, Bunnell RE, Cyril J, Iademarco MF. The intersection of genomics and big data with public health: opportunities for precision public health. PloS Med (2020) 17(10):e1003373. doi: 10.1371/journal.pmed.1003373
13. Shu Y, McCauley J. GISAID: global initiative on sharing all influenza data - from vision to reality. Euro Surveill (2017) 22(13):30494. doi: 10.2807/1560-7917.ES.2017.22.13.30494
14. Nicholls SM, Poplawski R, Bull MJ, Underwood A, Chapman M, Abu-Dahab K, et al. The COVID-19 Genomics UK (COG-UK) consortium. CLIMB-COVID: continuous integration supporting decentralized sequencing for SARS-CoV-2 genomic surveillance. Genome Biol (2021) 22(1):196. doi: 10.1186/s13059-021-02395-y
15. Kemp SA, Collier DA, Datir RP, Ferreira IATM, Gayed S, Jahun A, et al. SARS-CoV-2 evolution during treatment of chronic infection. Nature (2021) 592(7853):277–82. doi: 10.1038/s41586-021-03291-y
16. Wright DW, Harvey WT, Hughes J, Cox M, Peacock TP, Colquhoun R, et al. Tracking SARS-CoV-2 mutations and variants through the COG-UK-Mutation Explorer. Virus Evol (2022) 8(1):veac023. doi: 10.1093/ve/veac023
17. Boshier FAT, Pang J, Penner J, Parker M, Alders N, Bamford A, et al. Evolution of viral variants in remdesivir-treated and untreated SARS-CoV-2-infected pediatrics patients. J Med Virol (2022) 94(1):161–72. doi: 10.1002/jmv.27285
18. Cox M, Peacock TP, Harvey WT, Hughes J, Wright DW, COVID-19 Genomics UK (COG-UK) Consortium, et al. SARS-CoV-2 variant evasion of monoclonal antibodies based on in itro studies. Nat Rev Microbiol (2023) 21(2):112–24. doi: 10.1038/s41579-022-00809-7
19. Crook D, Fingerhut H. Global governance of genomic pathogen surveillance. Opportunities and challenges. London: Tony Blair Institute for Global Change, Lawrence J. Eillison Institute for Transformative Medicine of USC, University of Oxford (2022). Available at: https://assets.ctfassets.net/75ila1cntaeh/6SQyBJPNAKwQGpCoXS34qz/8c734f522305de9eac0e687c8be1f9d3/Global_Health_Security_Consortium__Global_Governance_of_Genomic_Pathogen_Surveillance__June_2022.pdf.
20. Baker DJ, Aydin A, Le-Viet T, Kay GL, Rudder S, de Oliveira Martins L, et al. CoronaHiT: high-throughput sequencing of SARS-CoV-2 genomes. Genome Med (2021) 13(1):21. doi: 10.1186/s13073-021-00839-5
21. Marjanovic S, Romanelli RJ, Ali G-C, Leach B, Bonsu M, Rodriguez-Rincon D, et al. COVID-19 Genomics UK (COG-UK) consortium [final report]. Santa Monica, CA: RAND Corporation (2022). Available at: https://www.rand.org/pubs/research_reports/RRA1277-1.html.
22. Kanteh A, Manneh J, Sanyang B, Kujabi MA, Jallow HS, Damilare DK, et al. Simple and structured model to build sequencing capacity in west Africa. Lancet Glob Health (2022) 10(9):e1240–1-e1241. doi: 10.1016/S2214-109X(22)00319-9
23. American Society of Microbiology. Supply shortages impacting COVID-19 and non-COVID testing (2021). Available at: https://asm.org/Articles/2020/September/Clinical-Microbiology-Supply-Shortage-Collecti-1.
24. Hannay E, Fernández-Suárez M, Duneton P. COVID-19 diagnostics: preserving manufacturing capacity for future pandemics. BMJ Glob Health (2022) 7(2):e007494. doi: 10.1136/bmjgh-2021-007494
25. Fischer S, Knoll V, Alleweldt F, Vogler S. Potential measures to facilitate the productions of active pharmaceutical ingredients (APIs). Luxembourg: Policy Department for Economic, Scientific and Quality of Life Policies, European Parliament (2023). Available at: https://www.europarl.europa.eu/RegData/etudes/STUD/2023/740070/IPOL_STU(2023)740070_EN.pdf.
26. Wang J, Hawken SE, Jones CD, Hagan RS, Bushman F, Everett J, et al. Collaboration between clinical and academic laboratories for sequencing SARS-CoV-2 genomes. J Clin Microbiol (2022) 60(3):e0128821. doi: 10.1128/JCM.01288-21
27. Iacobucci G. Covid-19: staff absences are continuing to stretch NHS hospitals, say leaders. BMJ (2022) 376:o350. doi: 10.1136/bmj.o350
28. Chen Z, Azman AS, Chen X, Zou J, Tian Y, Sun R, et al. Global landscape of SARS-CoV-2 genomic surveillance and data sharing. Nat Genet (2022) 54(4):499–507. doi: 10.1038/s41588-022-01033-y
29. Brito AF, Semenova E, Dudas G, Hassler GW, Kalinich CC, Kraemer MUG, et al. Global disparities in SARS-CoV-2 genomic surveillance. Nat Commun (2022) 13(1):7003. doi: 10.1038/s41467-022-33713-y
30. Ohlsen EC, Hawksworth AW, Wong K, Guagliardo SAJ, Fuller JA, Sloan ML, et al. Determining gaps in publicly shared SARS-CoV-2 genomic surveillance data by analysis of global submissions. Emerg Infect Dis (2022) 28(13):S85–92. doi: 10.3201/eid2813.220780
31. Makoni M. Africa’s $100-million pathogen genomics initiative. Lancet Microbe (2020) 1(8):e318. doi: 10.1016/S2666-5247(20)30206-8
32. Tegally H, San JE, Cotten M, Moir M, Tegomoh B, Mboowa G, et al. The evolving SARS-CoV-2 epidemic in Africa: insights from rapidly expanding genomic surveillance. Science (2022) 378(6615):eabq5358. doi: 10.1126/science.abq5358
33. Christoffels A, Mboowa G, van Heusden P, Makhubela S, Githinji G, Mwangi S, et al. A Pan-African pathogen genomics data sharing platform to support disease outbreaks. Nat Med (2023) 29(5):1052–5. doi: 10.1038/s41591-023-02266-y
34. Onywera H, Ondoa P, Nfii F, Ogwell A, Kebede Y, Christoffels A, et al. Boosting pathogen genomics and bioinformatics workforce in Africa. Lancet Infect Dis (2024) 24(2):e106–12. doi: 10.1016/S1473-3099(23)00394-8
35. O’Toole Á, Hill V, Pybus OG, Watts A, Bogoch II, Khan K, et al. Tracking the international spread of SARS-CoV-2 lineages B.1.1.7 and B.1.351/501Y-V2 with grinch. Wellcome Open Res (2021) 6:121. doi: 10.12688/wellcomeopenres.16661.2
36. Hoang T, da Silva AG, Jennison AV, Williamson DA, Howden BP, Seemann T. AusTrakka: fast-tracking nationalized genomics surveillance in response to the COVID-19 pandemic. Nat Commun (2022) 13(1):865. doi: 10.1038/s41467-022-28529-9
37. Nadon C, Croxen M, Knox N, Tanner J, Zetner A, Yoshida C, et al. Public health genomics capacity assessment: readiness for large-scale pathogen genomic surveillance in Canada’s public health laboratories. BMC Public Health (2022) 22(1):1817. doi: 10.1186/s12889-022-14210-9
38. Gorrie CL, Da Silva AG, Ingle DJ, Higgs C, Seemann T, Stinear TP, et al. Key parameters for genomics-based real-time detection and tracking of multidrug-resistant bacteria: a systematic analysis. Lancet Microbe (2021) 2(11):e575–83. doi: 10.1016/S2666-5247(21)00149-X
39. Gangiredla J, Rand H, Benisatto D, Payne J, Strittmatter C, Sanders J, et al. GalaxyTrakr: a distributed analysis tool for public health whole genome sequence data accessible to non-bioinformaticians. BMC Genomics (2021) 22(1):114. doi: 10.1186/s12864-021-07405-8
40. Huptas C, Scherer S, Wenning M. Optimized Illumina PCR-free library preparation for bacterial whole genome sequencing and analysis of factors influencing de novo assembly. BMC Res Notes (2016) 9:269. doi: 10.1186/s13104-016-2072-9
41. Gaio D, Anantanawat K, To J, Liu M, Monahan L, Darling AE. Hackflex: low-cost, high-throughput, Illumina Nextera Flex library construction. Microb Genom (2022) 8(1):744. doi: 10.1099/mgen.0.000744
42. Peacock SJ, Parkhill J, Brown NM. Changing the paradigm for hospital outbreak detection by leading with genomic surveillance of nosocomial pathogens. Microbiol (Reading) (2018) 164(10):1213–9. doi: 10.1099/mic.0.000700
43. Chattaway MA, Dallman TJ, Larkin L, Nair S, McCormick J, Mikhail A, et al. The Transformation of reference microbiology methods and surveillance for Salmonella with the use of Whole Genome Sequencing in England and Wales. Front Public Health (2019) 7:317. doi: 10.3389/fpubh.2019.00317
44. Konings F, Perkins MD, Kuhn JH, Pallen MJ, Alm EJ, Archer BN, et al. SARS-CoV-2 Variants of Interest and Concern naming scheme conducive for global discourse. Nat Microbiol (2021) 6(7):821–3. doi: 10.1038/s41564-021-00932-w
45. Simpson VJ, Thriemer K, Tobon-Castano A, Trimarsanto H, Urbano Ferreira M, Vélez ID, et al. An open dataset of Plasmodium vivax genome variation in 1,895 worldwide samples. Wellcome Open Res (2022) 7:136. doi: 10.12688/wellcomeopenres.17795.1
46. Quick J, Loman NJ, Duraffour S, Simpson JT, Severi E, Cowley L, et al. Real-time, portable genome sequencing for Ebola surveillance. Nature (2016) 530(7589):228–32. doi: 10.1038/nature16996
47. Sherry NL, Gorrie CL, Kwong JC, Higgs C, Stuart RL, Marshall C, et al. Multi-site implementation of whole genome sequencing for hospital infection control: a prospective genomic epidemiological analysis. Lancet Reg Health West Pac (2022) 23:100446. doi: 10.1016/j.lanwpc.2022.100446
48. Rockett RJ, Arnott A, Lam C, Sadsad R, Timms V, Gray KA, et al. Revealing COVID-19 transmission in Australia by SARS-CoV-2 genome sequencing and agent-based modeling. Nat Med (2020) 26(9):1398–404. doi: 10.1038/s41591-020-1000-7
49. Rao AK, Schulte J, Chen TH, Hughes CM, Davidson W, Neff JM, et al. Monkeypox in a traveler returning from Nigeria – Dallas, Texas, July 2021. MMWR Morb Mortal Wkly Rep (2022) 71(14):509–16. doi: 10.15585/mmwr.mm7114a1
50. Revez J, Espinosa L, Albiger B, Leitmeyer KC, Struelens MJ, ECDC National Microbiology Focal Points and Experts Group. Survey on the use of whole-genome sequencing for infectious diseases surveillance: rapid expansion of European national capacities, 2015-2016. Front Public Health (2017) 5:347(5). doi: 10.3389/fpubh.2017.00347
51. Chewapreecha C, Holden MT, Vehkala M, Välimäki N, Yang Z, Harris SR, et al. Global and regional dissemination and evolution of Burkholderia pseudomallei. Nat Microbiol (2017) 2:16263. doi: 10.1038/nmicrobiol.2016.263
52. Aanensen DM, Carlos CC, Donado-Godoy P, Okeke IN, Ravikumar KL, NIHR Global Health Research Unit on Genomic Surveillance of Antimicrobial Resistance. Implementing whole-genome sequencing for ongoing surveillance of antimicrobial resistance: exemplifying insights into Klebsiella pneumoniae. Clin Infect Dis (2021) 73(Suppl_4):S255–7. doi: 10.1093/cid/ciab795
53. Lagrada ML, Argimón S, Borlasa JB, Abad JP, Gayeta JM, Masim ML, et al. Genomic surveillance of Salmonella spp. in the Philippines during 2013-2014. Trans R Soc Trop Med Hyg (2022) 116(12):1202–13. doi: 10.1093/trstmh/trac080
54. Okeke IN, Aboderin AO, Egwuenu A, Underwood A, Afolayan AO, Kekre M, et al. Establishing a national reference laboratory for antimicrobial resistance using a whole-genome sequencing framework: Nigeria’s experience. Microbiol (Reading) (2022) 168(8):001208. doi: 10.1099/mic.0.001208
55. World Health Organization. GLASS whole-genome sequencing for surveillance of antimicrobial resistance. Geneva: World Health Organization (2020). Available at: www.who.int/publications/i/item/9789240011007.
56. World Health Organization. Global genomic surveillance strategy for pathogens with pandemic and epidemic potential 2022–2032. Geneva: World Health Organization (2022). Available at: www.who.int/publications/i/item/9789240046979.
57. World Health Organization. Considerations for developing a national genomic surveillance strategy or action plan for pathogens with pandemic and epidemic potential. Geneva: World Health Organization (2023). Available at: www.who.int/publications/i/item/9789240076563.
58. Gwinn M, MacCannell D, Armstrong GL. Next-generation sequencing of infectious pathogens. JAMA (2019) 321(9):893–4. doi: 10.1001/jama.2018.21669
59. European Centre for Disease Prevention and Control. ECDC strategic framework for the integration of molecular and genomic typing into European surveillance and multi-country outbreak investigations 2019–2021 [ECDC technical report]. Stockholm (2019). Available at https://www.ecdc.europa.eu/en/publications-data/ecdc-strategic-framework-integration-molecular-and-genomic-typing-european.
60. NIHR Global Health Research Unit on Genomic Surveillance of AMR. Whole-genome sequencing as part of national and international surveillance programmes for antimicrobial resistance: a roadmap. BMJ Glob Health (2020) 5(11):e002244. doi: 10.1136/bmjgh-2019-002244
61. Ciccozzi M, Lai A, Zehender G, Borsetti A, Cella E, Ciotti M, et al. The phylogenetic approach for viral infectious disease evolution and epidemiology: an updating review. J Med Virol (2019) 91(10):1707–24. doi: 10.1002/jmv.25526
62. Wu A, Peng Y, Huang B, Ding X, Wang X, Niu P, et al. Genome composition and divergence of the novel coronavirus (2019-nCoV) originating in China. Cell Host Microbe (2020) 27(3):325–8. doi: 10.1016/j.chom.2020.02.001
63. Lemey P, Ruktanonchai N, Hong SL, Colizza V, Poletto C, Van den Broeck F, et al. Untangling introductions and persistence in COVID-19 resurgence in Europe. Nature (2021) 595(7869):713–7. doi: 10.1038/s41586-021-03754-2
64. Lane CR, Sherry NL, Porter AF, Duchene S, Horan K, Andersson P, et al. Genomics-informed responses in the elimination of COVID-19 in Victoria, Australia: an observational, genomic epidemiological study. Lancet Public Health (2021) 6(8):e547–56. doi: 10.1016/S2468-2667(21)00133-X
65. Featherstone LA, Zhang JM, Vaughan TG, Duchene S. Epidemiological inference from pathogen genomes: a review of phylodynamic models and applications. Virus Evol (2022) 8(1):veac045. doi: 10.1093/ve/veac045
66. Attwood SW, Hill SC, Aanensen DM, Connor TR, Pybus OG. Phylogenetic and phylodynamic approaches to understanding and combating the early SARS-CoV-2 pandemic. Nat Rev Genet (2022) 23(9):547–62. doi: 10.1038/s41576-022-00483-8
67. Ferrareze PAG, Pereira E, Costa RA, Thompson CE. Genomic characterization and molecular evolution of human monkeypox viruses. Arch Virol (2023) 168(11):278. doi: 10.1007/s00705-023-05904-5
68. Rambaut A, Holmes EC, O’Toole Á, Hill V, McCrone JT, Ruis C, et al. A dynamic nomenclature proposal for SARS-CoV-2 lineages to assist genomic epidemiology. Nat Microbiol (2020) 5(11):1403–7. doi: 10.1038/s41564-020-0770-5
69. Tao K, Tzou PL, Nouhin J, Gupta RK, de Oliveira T, Kosakovsky Pond SL, et al. The biological and clinical significance of emerging SARS-CoV-2 variants. Nat Rev Genet (2021) 22(12):757–73. doi: 10.1038/s41576-021-00408-x
70. Sonnleitner ST, Prelog M, Sonnleitner S, Hinterbichler E, Halbfurter H, Kopecky DBC, et al. Cumulative SARS-CoV-2 mutations and corresponding changes in immunity in an immunocompromised patient indicate viral evolution within the host. Nat Commun (2022) 13(1):2560. doi: 10.1038/s41467-022-30163-4
71. McLean G, Kamil J, Lee B, Moore P, Schulz TF, Muik A, et al. The impact of evolving SARS-CoV-2 mutations and variants on COVID-19 vaccines. mBio (2022) 13(2):e0297921. doi: 10.1128/mbio.02979-21
72. Planas D, Saunders N, Maes P, Guivel-Benhassine F, Planchais C, Buchrieser J, et al. Considerable escape of SARS-CoV-2 Omicron to antibody neutralization. Nature (2022) 602(7898):671–5. doi: 10.1038/s41586-021-04389-z
73. Stockdale JE, Liu P, Colijn C. The potential of genomics for infectious disease forecasting. Nat Microbiol (2022) 7(11):1736–43. doi: 10.1038/s41564-022-01233-6
74. Rappuoli R, Covacci A. Reverse vaccinology and genomics. Science (2003) 302(5645):602. doi: 10.1126/science.1092329
75. Donati C, Rappuoli R. Reverse vaccinology in the 21st century: improvements over the original design. Ann N Y Acad Sci (2013) 1285:115–32. doi: 10.1111/nyas.12046
76. Vormehr M, Schrörs B, Boegel S, Löwer M, Türeci Ö, Sahin U. Mutanome engineered RNA immunotherapy: towards patient-centered tumor vaccination. J Immunol Res (2015) 2015:595363. doi: 10.1155/2015/595363
77. Beck JD, Reidenbach D, Salomon N, Sahin U, Türeci Ö, Vormehr M, et al. mRNA therapeutics in cancer immunotherapy. Mol Cancer (2021) 20(1):69. doi: 10.1186/s12943-021-01348-0
78. Colijn C, Corander J, Croucher NJ. Designing ecologically optimized pneumococcal vaccines using population genomics. Nat Microbiol (2020) 5(3):473–85. doi: 10.1038/s41564-019-0651-y
79. Retchless AC, Fox LM, Maiden MCJ, Smith V, Harrison LH, Glennie L, et al. Toward a global genomic epidemiology of meningococcal disease. J Infect Dis (2019) 220(Suppl 4):S266–73. doi: 10.1093/infdis/jiz279
80. Rodrigues CMC, MacDonald L, Ure R, Smith A, Cameron JC, Maiden MCJ. Exploiting real-time genomic surveillance data to assess 4CMenB meningococcal vaccine performance in Scotland, 2015 to 2022. mBio (2023) 14(2):e0049923. doi: 10.1128/mbio.00499-23
81. Bridel S, Bouchez V, Brancotte B, Hauck S, Armatys N, Landier A, et al. A comprehensive resource for Bordetella genomic epidemiology and biodiversity studies. Nat Commun (2022) 13(1):3807. doi: 10.1038/s41467-022-31517-8
82. Payne M, Xu Z, Hu D, Kaur S, Octavia S, Sintchenko V, et al. Genomic epidemiology and multilevel genome typing of Bordetella pertussis. Emerg Microbes Infect (2023) 12(2):2239945. doi: 10.1080/22221751.2023.2239945
83. Lam C, Martinez E, Crighton T, Furlong C, Donnan E, Marais BJ, et al. Value of routine whole genome sequencing for Mycobacterium tuberculosis drug resistance detection. Int J Infect Dis (2021) 113(Suppl 1):S48–54. doi: 10.1016/j.ijid.2021.03.033
84. Lam MMC, Wick RR, Watts SC, Cerdeira LT, Wyres KL, Holt KE. A genomic surveillance framework and genotyping tool for Klebsiella pneumoniae and its related species complex. Nat Commun (2021) 12(1):4188. doi: 10.1038/s41467-021-24448-3
85. Hatcher J, Godambe S, Lyall H, Tyszczuk L, Stubbs F, Cummings N, et al. Healthcare-worker-associated outbreak of Panton-Valentine-leukocidin-producing meticillin-sensitive Staphylococcus aureus in a large neonatal unit in London: successful targeted suppression therapy following failure of mass suppression therapy. J Hosp Infect (2022) 122:148–56. doi: 10.1016/j.jhin.2021.12.023
86. Ellington MJ, Ekelund O, Aarestrup FM, Canton R, Doumith M, Giske C, et al. The role of whole genome sequencing in antimicrobial susceptibility testing of bacteria: report from the EUCAST Subcommittee. Clin Microbiol Infect (2017) 23(1):2–22. doi: 10.1016/j.cmi.2016.11.012
87. Bortolaia V, Kaas RS, Ruppe E, Roberts MC, Schwarz S, Cattoir V, et al. ResFinder 4.0 for predictions of phenotypes from genotypes. J Antimicrob Chemother (2020) 75(12):3491–500. doi: 10.1093/jac/dkaa345
88. Rebelo AR, Bortolaia V, Leekitcharoenphon P, Hansen DS, Nielsen HL, Ellermann-Eriksen S, et al. One day in Denmark: comparison of phenotypic and genotypic antimicrobial susceptibility testing in bacterial isolates from clinical settings. Front Microbiol (2022) 13:804627. doi: 10.3389/fmicb.2022.804627
89. Hendriksen RS, Bortolaia V, Tate H, Tyson GH, Aarestrup FM, McDermott PF. Using genomics to track global antimicrobial resistance. Front Public Health (2019) 7:242(7). doi: 10.3389/fpubh.2019.00242
90. Hendriksen RS, Munk P, Njage P, van Bunnik B, McNally L, Lukjancenko O, et al. Global monitoring of antimicrobial resistance based on metagenomics analyses of urban sewage. Nat Commun (2019) 10(1):1124. doi: 10.1038/s41467-019-08853-3
91. Feldgarden M, Brover V, Fedorov B, Haft DH, Prasad AB, Klimke W. Curation of the AMRFinderPlus databases: applications, functionality and impact. Microb Genom (2022) 8(6):mgen000832. doi: 10.1099/mgen.0.000832
92. Peiffer-Smadja N, Dellière S, Rodriguez C, Birgand G, Lescure FX, Fourati S, et al. Machine learning in the clinical microbiology laboratory: has the time come for routine practice? Clin Microbiol Infect (2020) 26(10):1300–9. doi: 10.1016/j.cmi.2020.02.006
93. Catalán P, Wood E, Blair JMA, Gudelj I, Iredell JR, Beardmore RE. Seeking patterns of antibiotic resistance in ATLAS, an open, raw MIC database with patient metadata. Nat Commun (2022) 13(1):2917. doi: 10.1038/s41467-022-30635-7
94. Kim JI, Maguire F, Tsang KK, Gouliouris T, Peacock SJ, McAllister TA, et al. Machine learning for antimicrobial resistance prediction: current practice, limitations, and clinical perspective. Clin Microbiol Rev (2022) 35(3):e0017921. doi: 10.1128/cmr.00179-21
95. Ruppé E, d’Humières C, Armand-Lefèvre L. Inferring antibiotic susceptibility from metagenomic data: dream or reality? Clin Microbiol Infect (2022) 28(9):1225–9. doi: 10.1016/j.cmi.2022.04.017
96. Hoffmann B, Scheuch M, Höper D, Jungblut R, Holsteg M, Schirrmeier H, et al. Novel orthobunyavirus in cattle, europe, 2011. Emerg Infect Dis (2012) 18(3):469–72. doi: 10.3201/eid1803.111905
97. Stanley S, Liu Q, Fortune SM. Mycobacterium tuberculosis functional genetic diversity, altered drug sensitivity, and precision medicine. Front Cell Infect Microbiol (2022) 12:1007958(12). doi: 10.3389/fcimb.2022.1007958
98. Wang H, Li X, Li T, Zhang S, Wang L, Wu X, et al. The genetic sequence, origin, and diagnosis of SARS-CoV-2. Eur J Clin Microbiol Infect Dis (2020) 39(9):1629–35. doi: 10.1007/s10096-020-03899-4
99. Ko KKK, Chng KR, Nagarajan N. Metagenomics-enabled microbial surveillance. Nat Microbiol (2022) 7(4):486–96. doi: 10.1038/s41564-022-01089-w
100. d’Humières C, Salmona M, Dellière S, Leo S, Rodriguez C, Angebault C, et al. The potential role of clinical metagenomics in infectious diseases: therapeutic perspectives. Drugs (2021) 81(13):1453–66. doi: 10.1007/s40265-021-01572-4
101. Purushothaman S, Meola M, Egli A. Combination of whole genome sequencing and metagenomics for microbiological diagnostics. Int J Mol Sci (2022) 23(17):9834. doi: 10.3390/ijms23179834
102. Sherry NL, Horan KA, Ballard SA, Gonçalves da Silva A, Gorrie CL, Schultz MB, et al. An ISO-certified genomics workflow for identification and surveillance of antimicrobial resistance. Nat Commun (2023) 14(1):60. doi: 10.1038/s41467-022-35713-4
103. Hilt EE, Ferrieri P. Next generation and other sequencing technologies in diagnostic microbiology and infectious diseases. Genes (Basel) (2022) 13(9):1566. doi: 10.3390/genes13091566
104. Hall MB, Rabodoarivelo MS, Koch A, Dippenaar A, George S, Grobbelaar M, et al. Evaluation of nanopore sequencing for Mycobacterium tuberculosis drug susceptibility testing and outbreak investigation: a genomic analysis. Lancet Microbe (2023) 4(2):e84–92. doi: 10.1016/S2666-5247(22)00381-0
105. Schmidt J, Blessing F, Fimpler L, Wenzel F. Nanopore sequencing in a clinical routine laboratory: challenges and opportunities. Clin Lab (2020) 66(6):10.7754/Clin.Lab.2019.191114. doi: 10.7754/Clin.Lab.2019.191114
106. Ben Khedher M, Ghedira K, Rolain JM, Ruimy R, Croce O. Application and challenge of 3rd generation sequencing for clinical bacterial studies. Int J Mol Sci (2022) 23(3):1395. doi: 10.3390/ijms23031395
107. David S, Cohen V, Reuter S, Sheppard AE, Giani T, Parkhill J, et al. Integrated chromosomal and plasmid sequence analyses reveal diverse modes of carbapenemase gene spread among Klebsiella pneumoniae. Proc Natl Acad Sci USA (2020) 117(40):25043–54. doi: 10.1073/pnas.2003407117
108. McHugh MP, Parcell BJ, Pettigrew KA, Toner G, Khatamzas E, El Sakka N, et al. Presence of optrA-mediated linezolid resistance in multiple lineages and plasmids of Enterococcus faecalis revealed by long read sequencing. Microbiol (Reading) (2022) 168(2):1137. doi: 10.1099/mic.0.001137
109. Egan SA, Kavanagh NL, Shore AC, Mollerup S, Samaniego Castruita JA, O’Connell B, et al. Genomic analysis of 600 vancomycin-resistant Enterococcus faecium reveals a high prevalence of ST80 and spread of similar vanA regions via IS1216E and plasmid transfer in diverse genetic lineages in Ireland. J Antimicrob Chemother (2022) 77(2):320–30. doi: 10.1093/jac/dkab393
110. Ameur A, Kloosterman WP, Hestand MS. Single-molecule sequencing: towards clinical applications. Trends Biotechnol (2019) 37(1):72–85. doi: 10.1016/j.tibtech.2018.07.013
111. Ying YL, Hu ZL, Zhang S, Qing Y, Fragasso A, Maglia G, et al. Nanopore-based technologies beyond DNA sequencing. Nat Nanotechnol (2022) 17(11):1136–46. doi: 10.1038/s41565-022-01193-2
112. Goenka SD, Gorzynski JE, Shafin K, Fisk DG, Pesout T, Jensen TD, et al. Accelerated identification of disease-causing variants with ultra-rapid nanopore genome sequencing. Nat Biotechnol (2022) 40(7):1035–41. doi: 10.1038/s41587-022-01221-5
113. Gorzynski JE, Goenka SD, Shafin K, Jensen TD, Fisk DG, Grove ME, et al. Ultrarapid nanopore genome sequencing in a critical care setting. N Engl J Med (2022) 386(7):700–2. doi: 10.1056/NEJMc2112090
114. Sundermann AJ, Chen J, Miller JK, Martin EM, Snyder GM, Van Tyne D, et al. Whole-genome sequencing surveillance and machine learning for healthcare outbreak detection and investigation: a systematic review and summary. Antimicrob Steward Healthc Epidemiol (2022) 2(1):e91. doi: 10.1017/ash.2021.241
115. Outhred AC, Jelfs P, Suliman B, Hill-Cawthorne GA, Crawford ABH, Marais BJ, et al. Added value of whole-genome sequencing for management of highly drug-resistant TB. J Antimicrob Chemother (2015) 70(4):1198–202. doi: 10.1093/jac/dku508
116. Allix-Beguec C, Arandjelovic I, Bi L, Beckert P, Bonnet M, Bradley P, et al. Prediction of susceptibility to first-line tuberculosis drugs by DNA sequencing. N Engl J Med (2018) 379(15):1403–415. doi: 10.1056/NEJMoa1800474
117. Mugwagwa T, Abubakar I, White PJ. Using molecular testing and whole-genome sequencing for tuberculosis diagnosis in a low-burden setting: a cost-effectiveness analysis using transmission-dynamic modelling. Thorax (2021) 76(3):281–91. doi: 10.1136/thoraxjnl-2019-214004
118. Ford L, Glass K, Williamson DA, Sintchenko V, Robson JMB, Lancsar E, et al. Cost of whole genome sequencing for non-typhoidal Salmonella enterica. PloS One (2021) 16(3):e0248561. doi: 10.1371/journal.pone.0248561
119. Ford L, Carter GP, Wang Q, Seemann T, Sintchenko V, Glass K, et al. Incorporating whole-genome sequencing into public health surveillance: lessons from prospective sequencing of Salmonella Typhimurium in Australia. Foodborne Pathog Dis (2018) 15(3):161–7. doi: 10.1089/fpd.2017.2352
120. Ludden C, Lötsch F, Alm E, Kumar N, Johansson K, Albiger B, et al. Cross-border spread of blaNDM-1- and blaOXA-48-positive Klebsiella pneumoniae: a European collaborative analysis of whole genome sequencing and epidemiological data, 2014 to 2019. Euro Surveill (2020) 25(20):pii=2000627. doi: 10.2807/1560-7917.ES.2020.25.20.2000627
121. Stephens ZD, Lee SY, Faghri F, Campbell RH, Zhai C, Efron MJ, et al. Big Data: astronomical or genomical? PloS Biol (2015) 13(7):e1002195. doi: 10.1371/journal.pbio.1002195
122. Meehan CJ, Goig GA, Kohl TA, Verboven L, Dippenaar A, Ezewudo M, et al. Whole genome sequencing of Mycobacterium tuberculosis: current standards and open issues. Nat Rev Microbiol (2019) 17(9):533–45. doi: 10.1038/s41579-019-0214-5
123. Griffiths EJ, Timme RE, Mendes CI, Page AJ, Alikhan NF, Fornika D, et al. Future-proofing and maximizing the utility of metadata: the PHA4GE SARS-CoV-2 contextual data specification package. GigaScience (2022) 11:1–3. doi: 10.1093/gigascience/giac003
124. Cori A, Nouvellet P, Garske T, Bourhy H, Nakouné E, Jombart T. A graph-based evidence synthesis approach to detecting outbreak clusters: an application to dog rabies. PloS Comput Biol (2018) 14(12):e1006554. doi: 10.1371/journal.pcbi.1006554
125. Grad YH, Lipsitch M. Epidemiologic data and pathogen genome sequences: a powerful synergy for public health. Genome Biol (2014) 15(11):538. doi: 10.1186/s13059-014-0538-4
126. Brown B, Allard M, Bazaco MC, Blankenship J, Minor T. An economic evaluation of the Whole Genome Sequencing source tracking program in the U.S. PloS One (2021) 16(10):e0258262. doi: 10.1371/journal.pone.0258262
127. Rockett RJ, Draper J, Gall M, Sim EM, Arnott A, Agius JE, et al. Co-infection with SARS-CoV-2 Omicron and Delta variants revealed by genomic surveillance. Nat Commun (2022) 13(1):2745. doi: 10.1038/s41467-022-30518-x
128. Suster CJE, Arnott A, Blackwell G, Gall M, Draper J, Martinez E, et al. Guiding the design of SARS-Cov-2 genomic surveillance by estimating the resolution of outbreak detection. Front Public Health (2022) 10:1004201. doi: 10.3389/fpubh.2022.1004201
129. Hare D, Dembicka KM, Brennan C, Campbell C, Sutton-Fitzpatrick U, Stapleton PJ, et al. Whole-genome sequencing to investigate transmission of SARS-CoV-2 in the acute healthcare setting: a systematic review. J Hosp Infect (2023) 140:139–55. doi: 10.1016/j.jhin.2023.08.002
130. World Health Organization. WHO Guiding principles for pathogen genome data sharing. Geneva: World Health Organization (2022). Available at: www.who.int/publications/i/item/9789240061743.
131. Uelze L, Becker N, Borowiak M, Busch U, Dangel A, Deneke C, et al. Toward an integrated genome-based surveillance of Salmonella enterica in Germany. Front Microbiol (2021) 12:626941. doi: 10.3389/fmicb.2021.626941
132. Arnott A, Draper J, Rockett RJ, Lam C, Sadsad R, Gall M, et al. Documenting elimination of co-circulating COVID-19 clusters using genomics in New South Wales, Australia. BMC Res Notes (2021) 14(1):415. doi: 10.1186/s13104-021-05827-x
133. Robishaw JD, Alter SM, Solano JJ, Shih RD, DeMets DL, Maki DG, et al. Genomic surveillance to combat COVID-19: challenges and opportunities. Lancet Microbe (2021) 2(9):e481–4. doi: 10.1016/S2666-5247(21)00121-X
134. Song L, Liu H, Brinkman FSL, Gill E, Griffiths EJ, Hsiao WWL, et al. Addressing privacy concerns in sharing viral sequences and minimum contextual data in a public repository during the COVID-19 pandemic. Front Genet (2021) 12:716541. doi: 10.3389/fgene.2021.716541
135. Halabi S. Viral sovereignty, intellectual property, and the changing global system for sharing pathogens for infectious disease research. Ann Health Law (2019) 28:101. Available at: https://lawecommons.luc.edu/cgi/viewcontent.cgi?article=1468&context=annals.
136. Afolayan AO, Bernal JF, Gayeta JM, Masim ML, Shamanna V, Abrudan M, et al. Overcoming data bottlenecks in genomic pathogen surveillance. Clin Infect Dis (2021) 73(Suppl 4):S267–74. doi: 10.1093/cid/ciab785
137. Marshall E. Ethics in science: Is data-hoarding slowing the assault on pathogens? Science (1997) 275(5301):777–80. doi: 10.1126/science.275.5301.777
138. Toczydlowski RH, Liggins L, Gaither MR, Anderson TJ, Barton RL, Berg JT, et al. Poor data stewardship will hinder global genetic diversity surveillance. Proc Natl Acad Sci U.S.A. (2021) 118(34):e2107934118. doi: 10.1073/pnas.2107934118
139. Sintchenko V, Holmes EC. The role of pathogen genomics in assessing disease transmission. Brit Med J (2015) 350:h1314. doi: 10.1136/bmj.h1314
140. One Health High-Level Expert Panel (OHHLEP), Adisasmito WB, Almuhairi S, Behravesh CB, Bilivogui P, Bukachi SA, et al. One Health: A new definition for a sustainable and healthy future. PloS Pathog (2022) 18(6):e1010537. doi: 10.1371/journal.ppat.1010537
141. Hammer AS, Quaade ML, Rasmussen TB, Fonager J, Rasmussen M, Mundbjerg K, et al. SARS-CoV-2 transmission between mink (Neovison vison) and humans, Denmark. Emerg Infect Dis (2021) 27(2):547–51. doi: 10.3201/eid2702.203794
142. Briand FX, Schmitz A, Ogor K, Le Prioux A, Guillou-Cloarec C, Guillemoto C, et al. Emerging highly pathogenic H5 avian influenza viruses in France during winter 2015/16: phylogenetic analyses and markers for zoonotic potential. Euro Surveill (2017) 22(9):30473. doi: 10.2807/1560-7917.ES.2017.22.9.30473
143. Williams TGS, Snell LB, Alder C, Charalampous T, Alcolea-Medina A, Sehmi JK, et al. Feasibility and clinical utility of local rapid nanopore influenza A virus whole genome sequencing for integrated outbreak management, genotypic resistance detection and timely surveillance. Microb Genom (2023) 9(8):mgen001083. doi: 10.1099/mgen.0.001083
144. Aarestrup FM, Bonten M, Koopmans M. Pandemics- One Health preparedness for the next. Lancet Reg Health Eur (2021) 9:100210. doi: 10.1016/j.lanepe.2021.100210
145. Van Boeckel TP, Glennon EE, Chen D, Gilbert M, Robinson TP, Grenfell BT, et al. Reducing antimicrobial use in food animals. Consider user fees and regulatory caps on veterinary use. Science (2017) 357(6358):1350–2. doi: 10.1126/science.aao1495
146. Berendonk TU, Manaia CM, Merlin C, Fatta-Kassinos D, Cytryn E, Walsh F, et al. Tackling antibiotic resistance: the environmental framework. Nat Rev Microbiol (2015) 13(5):310–7. doi: 10.1038/nrmicro3439
147. World Health Organization. One Health initiative website. Available at: https://www.who.int/teams/one-health-initiative.
148. Centers for Disease Control and Prevention. One health. Available at: www.cdc.gov/onehealth/index.html.
149. European Centre for Disease Prevention and Control. Antimicrobial Resistance in the EU/EEA - A One Health response. ECDC (2022). Available at: https://www.ecdc.europa.eu/en/publications-data/antimicrobial-resistance-eueea-one-health-response.
150. Africa Centres for Disease Control and Prevention. One Health programme. Available at: https://africacdc.org/programme/surveillance-disease-intelligence/one-health/.
151. Bronzwaer S, Catchpole M, de Coen W, Dingwall Z, Fabbri K, Foltz C, et al. One Health collaboration with and among EU Agencies - Bridging research and policy. One Health (2022) 15:100464. doi: 10.1016/j.onehlt.2022.100464
152. European Food Safety Authority. One Health cross-Agency Task Force (2023). Available at: https://www.efsa.europa.eu/sites/default/files/documents/news/one-health-cross-agency-task-force.pdf.
153. Levy JI, Andersen KG, Knight R, Karthikeyan S. Wastewater surveillance for public health. Science (2023) 379(6627):26–7. doi: 10.1126/science.ade2503
154. Buschhardt T, Günther T, Skjerdal T, Torpdahl M, Gethmann J, Filippitzi ME, et al. A one health glossary to support communication and information exchange between the human health, animal health and food safety sectors. One Health (2021) 13:100263. doi: 10.1016/j.onehlt.2021.100263
155. Armstrong GL, MacCannell DR, Taylor J, Carleton HA, Neuhaus EB, Bradbury RS, et al. Pathogen genomics in public health. N Engl J Med (2019) 381(26):2569–80. doi: 10.1056/NEJMsr1813907
156. Waldram A, Dolan G, Ashton PM, Jenkins C, Dallman TJ. Epidemiological analysis of Salmonella clusters identified by whole genome sequencing, England and Wales 2014. Food Microbiol (2018) 71:39–45. doi: 10.1016/j.fm.2017.02.012
157. Moura A, Tourdjman M, Leclercq A, Hamelin E, Laurent E, Fredriksen N, et al. Real-time whole-genome sequencing for surveillance of Listeria monocytogenes, France. Emerg Infect Dis (2017) 23(9):1462–70. doi: 10.3201/eid2309.170336
158. Joensen KG, Scheutz F, Lund O, Hasman H, Kaas RS, Nielsen EM, et al. Real-time whole-genome sequencing for routine typing, surveillance, and outbreak detection of verotoxigenic Escherichia coli. J Clin Microbiol (2014) 52(5):1501–10. doi: 10.1128/JCM.03617-13
159. Alleweldt F, Kara Ş, Best K, Aarestrup FM, Beer M, Bestebroer TM, et al. Economic evaluation of whole genome sequencing for pathogen identification and surveillance – results of case studies in Europe and the Americas 2016 to 2019. Euro Surveill (2021) 26(9):1900606. doi: 10.2807/1560-7917.ES.2021.26.9.1900606
160. Kitajima M, Ahmed W, Bibby K, Carducci A, Gerba CP, Hamilton KA, et al. SARS-CoV-2 in wastewater: state of the knowledge and research needs. Sci Total Environ (2020) 739:139076. doi: 10.1016/j.scitotenv.2020.139076
161. Munk P, Brinch C, Møller FD, Petersen TN, Hendriksen RS, Seyfarth AM, et al. Genomic analysis of sewage from 101 countries reveals global landscape of antimicrobial resistance. Nat Commun (2022) 13(1):7251. doi: 10.1038/s41467-022-34312-7
162. Tran M, Smurthwaite KS, Nghiem S, Cribb DM, Zahedi A, Ferdinand AD, et al. Economic evaluations of whole-genome sequencing for pathogen identification in public health surveillance and health-care-associated infections: a systematic review. Lancet Microbe (2023) 4(11):e953–62. doi: 10.1016/S2666-5247(23)00180-5
163. Fox JM, Saunders NJ, Jerwood SH. Economic and health impact modelling of a whole genome sequencing-led intervention strategy for bacterial healthcare-associated infections for England and for the USA. Microb Genom (2023) 9(8):mgen001087. doi: 10.1099/mgen.0.001087
164. Price V, Ngwira LG, Lewis JM, Baker KS, Peacock SJ, Jauneikaite E, et al. A systematic review of economic evaluations of whole-genome sequencing for the surveillance of bacterial pathogens. Microb Genom (2023) 9(2):mgen000947. doi: 10.1099/mgen.0.000947
165. Sánchez-Busó L, Cole MJ, Spiteri G, Day M, Jacobsson S, Golparian D, et al. Europe-wide expansion and eradication of multidrug-resistant Neisseria gonorrhoeae lineages: a genomic surveillance study. Lancet Microbe (2022) 3(6):e452–63. doi: 10.1016/S2666-5247(22)00044-1
166. Ferdinand AS, Kelaher M, Lane CR, da Silva AG, Sherry NL, Ballard SA, et al. An implementation science approach to evaluating pathogen whole genome sequencing in public health. Genome Med (2021) 13(1):121. doi: 10.1186/s13073-021-00934-7
167. European Centre for Disease Prevention and Control. EU Laboratory Capability Monitoring System (EULabCap): report on 2021 survey of EU/EEA country capabilities and capacities. Stockholm: ECDC (2023). Available at: https://www.ecdc.europa.eu/en/publications-data/eu-laboratory-capability-monitoring-system-eulabcap-2021.
168. Nadon C, Van Walle I, Gerner-Smidt P, Campos J, Chinen I, Concepcion-Acevedo J, et al. PulseNet International: vision for the implementation of whole genome sequencing (WGS) for global food-borne disease surveillance. Euro Surveill (2017) 22(23):30544. doi: 10.2807/1560-7917.ES.2017.22.23.30544
169. Davedow T, Carleton H, Kubota K, Palm D, Schroeder M, Gerner-Smidt P, et al. PulseNet international survey on the implementation of whole genome sequencing in low and middle-income countries for foodborne disease surveillance. Foodborne Pathog Dis (2022) 19(5):332–40. doi: 10.1089/fpd.2021.0110
170. Brolund A, Lagerqvist N, Byfors S, Struelens MJ, Monnet DL, Albiger B, et al. Worsening epidemiological situation of carbapenemase-producing Enterobacteriaceae in Europe, assessment by national experts from 37 countries, July 2018. Euro Surveill (2019) 24(9):pii=1900123. doi: 10.2807/1560-7917.ES.2019.24.9.1900123
171. Kekre M, Arevalo SA, Valencia MF, Lagrada ML, Macaranas PKV, Nagaraj G, et al. Integrating scalable genome sequencing into microbiology laboratories for routine antimicrobial resistance surveillance. Clin Infect Dis (2021) 73(Suppl_4):S258–S266:S258-66. doi: 10.1093/cid/ciab796
172. Nachega JB, Nsanzimana S, Rawat A, Wilson LA, Rosenthal PJ, Siedner MJ, et al. Advancing detection and response capacities for emerging and re-emerging pathogens in Africa. Lancet Infect Dis (2023) 23(5):e185–9. doi: 10.1016/S1473-3099(22)00723-X
173. Jolley KA, Bray JE, Maiden MCJ. Open-access bacterial population genomics: BIGSdb software, the PubMLST.org website and their applications. Wellcome Open Res (2018) 3:124. doi: 10.12688/wellcomeopenres.14826.1
174. Argimón S, David S, Underwood A, Abrudan M, Wheeler NE, Kekre M, et al. Rapid genomic characterization and global surveillance of Klebsiella using Pathogenwatch. Clin Infect Dis (2021) 73(Suppl_4):S325–S335:S325-35. doi: 10.1093/cid/ciab784
175. Global Health Security Consortium. Global governance of genomic pathogen surveillance- opportunities and challenges (2022). Available at: https://institute.global/sites/default/files/2022-06/Global%20Health%20Security%20Consortium%2C%20Global%20Governance%20of%20Genomic%20Pathogen%20Surveillance%2C%20June%202022.pdf.
176. Albiger B, Revez J, Leitmeyer KC, Struelens MJ. Networking of public health microbiology laboratories bolsters Europe’s defenses against infectious diseases. Front Public Health (2018) 6:46. doi: 10.3389/fpubh.2018.00046
177. World Health Organization. International Pathogen Surveillance Network (IPSN) website. Available at: www.who.int/initiatives/international-pathogen-surveillance-network.
178. Painset A, Björkman JT, Kiil K, Guillier L, Mariet JF, Félix B, et al. LiSEQ – whole-genome sequencing of a cross-sectional survey of Listeria monocytogenes in ready-to-eat foods and human clinical cases in Europe. Microb Genom (2019) 5(2):e000257. doi: 10.1099/mgen.0.000257
179. Pijnacker R, Dallman TJ, Tijsma ASL, Hawkins G, Larkin L, Kotila SM, et al. An international outbreak of Salmonella enterica serotype Enteritidis linked to eggs from Poland: a microbiological and epidemiological study. Lancet Infect Dis (2019) 19(7):778–86. doi: 10.1016/S1473-3099(19)30047-7
180. European Centre for Disease Prevention and Control. Emergence of hypervirulent Klebsiella pneumoniae ST23 carrying carbapenemase genes in EU/EEA countries. In: Rapid Risk Assessment Report. ECDC, Stockholm (2021).
181. Maiden MC, Jansen van Rensburg MJ, Bray JE, Earle SG, Ford SA, Jolley KA, et al. MLST revisited: the gene-by-gene approach to bacterial genomics. Nat Rev Microbiol (2013) 11(10):728–36. doi: 10.1038/nrmicro3093
182. Guglielmini J, Hennart M, Badell E, Toubiana J, Criscuolo A, Brisse S. Genomic epidemiology and strain taxonomy of Corynebacterium diphtheriae. J Clin Microbiol (2021) 59(12):e0158121. doi: 10.1128/JCM.01581-21
Keywords: infectious diseases, antimicrobial resistance, public health, One Health, whole genome sequencing, pathogen genomics, genomic surveillance, pandemic
Citation: Struelens MJ, Ludden C, Werner G, Sintchenko V, Jokelainen P and Ip M. Real-time genomic surveillance for enhanced control of infectious diseases and antimicrobial resistance. Front Sci (2024) 2:1298248. doi: 10.3389/fsci.2024.1298248
Received: 21 September 2023; Accepted: 02 April 2024;
Published: 25 April 2024.
Edited by:
Edward Feil, University of Bath, United KingdomReviewed by:
David M. Engelthaler, Translational Genomics Research Institute (TGen), United StatesPaul Simon Freemont, Imperial College London, United Kingdom
Copyright © 2024 Struelens, Ludden, Werner, Sintchenko, Jokelainen and Ip. This is an open-access article distributed under the terms of the Creative Commons Attribution License (CC BY). The use, distribution or reproduction in other forums is permitted, provided the original author(s) and the copyright owner(s) are credited and that the original publication in this journal is cited, in accordance with accepted academic practice. No use, distribution or reproduction is permitted which does not comply with these terms.
*Correspondence: Marc J. Struelens, bWouc3RydWVsZW5zQGdtYWlsLmNvbQ==
†Present address: Catherine Ludden, Research and Innovation Office, St. James's Hospital, Dublin, Ireland