Explore article hub
- 1Horticulture and Product Physiology, Wageningen University, Wageningen, Netherlands
- 2Horticultural Sciences, University of Florida, Gainesville, FL, United States
- 3Plant Sciences (IBG-2), Forschungszentrum Jülich GmbH, Jülich, Germany
- 4Department of Natural Sciences, Macquarie University, Sydney, NSW, Australia
- 5Department of Economic Analysis, University of the Basque Country (UPV/EHU), Bilbao, Spain
- 6National Key Laboratory of Efficient Plant Carbon Capturing, CAS Center for Excellence in Molecular Plant Sciences, Shanghai Institute of Plant Physiology and Ecology, Chinese Academy of Sciences, Shanghai, China
- 7Queensland Alliance for Agriculture and Food Innovations, The University of Queensland, St Lucia, QLD, Australia
- 8Institute of Biology II, Faculty of Biology, University of Freiburg, Freiburg, Germany
- 9Centre for Integrative Biological Signalling Studies (CIBSS), University of Freiburg, Freiburg, Germany
- 10Department of Plant Sciences, University of California, Davis, Davis, CA, United States
Abstract
Vertical farming is considered to be a key enabler for transforming agrifood systems, especially in or nearby urbanized areas. Vertical farming systems (VFS) are advanced indoor cropping systems that allow for highly intensified and standardized plant production. The close control of environmental parameters makes crop production stable and repeatable, ensuring year-round uniform product quality and quantity irrespective of location. However, due to continuous changes in plant physiology and development, as well as frequent changes in electricity prices, the optimum conditions for crop production and its associated costs can change within days or even minutes. This makes it beneficial to dynamically adjust setpoints for light (intensity, spectrum, pattern, and daylength), CO2, temperature, humidity, air flow, and water and nutrient availability. In this review, we highlight the beneficial effects that dynamic growth conditions can have on key plant processes, including improvements in photosynthetic gas exchange, transpiration, organ growth, development, light interception, flowering, and product quality. Our novel findings based on modeling and experimentation demonstrate that a dynamic daily light intensity pattern that responds to frequent changes in electricity prices can save costs without reducing biomass. Further, we argue that a smart, dynamic VFS climate management requires feedback mechanisms: several mobile and immobile sensors could work in combination to continuously monitor the crop, generating data that feeds into crop growth models, which, in turn, generate climate setpoints. In addition, we posit that breeding for the VFS environment is at a very early stage and highlight traits for breeding for this specialized environment. We envision a continuous feedback loop between dynamic crop management, crop monitoring, and trait selection for genotypes that are specialized for these conditions.
Key points
- Vertical farming systems (VFS) offer innovative ways to cultivate crops in controlled environments and may help address challenges in local food security and sustainability.
- VFS are often operated using constant environmental conditions, but diurnal and developmental changes in plant physiology, as well as diurnal changes in electricity prices, call for dynamic environmental control.
- VFS are sensitive to electricity prices, but electricity consumption can be adjusted to save costs through variable light intensity without compromising plant growth.
- Several end-of-production treatments for crops grown in VFS allow for optimizing product quality—including extended shelf life and increased concentrations of health-promoting metabolites—while avoiding growth penalty.
- The implementation of integrated plant monitoring and modeling in cultivation systems is crucial for achieving dynamic control of climate conditions.
- More focus should be given to breeding crops that are specific to controlled environments, as this will help address a bottleneck in a wider adoption of VFS.
Introduction
Vertical farming systems (VFS) are advanced indoor cropping systems that allow for intensified and standardized production of fresh and nutritious vegetables, herbs, microgreens, and fruits in close proximity to customers (1, 2). Crop production in VFS relies on controlled environments: light (intensity, spectrum, profile, and daylength), carbon dioxide (CO2) concentration, temperature, air humidity, air flow, plant density, and water and nutrient availability are tightly regulated. Crop yields can be strongly increased through this control; for example, in experiments and simulations, yields of wheat (Triticum aestivum) and rice (Oryza sativa) were found to be 10–60 times greater per unit production area compared to world average annual yields (3, 4). However, this increase in productivity requires much greater inputs than other cropping systems, especially in energy and electronics. This greater demand for inputs is amplified by the ability to stack layers of production area, which additionally multiplies VFS outputs per unit ground area (3). Given that food production must increase 25−70% by the year 2050 (5)—a problem exacerbated by global development of mega-cities, growing climate uncertainty, and geopolitical instability—VFS is seen to contribute to achieving local food security. In addition, higher quality food can be grown in VFS, potentially improving nutritional value and food safety and reducing yield losses (6–8). In a more populated world, production in VFS may be accomplished with less land, water, and nutrient inputs and will benefit from a continued increase in the share of renewable energy (9). However, realizing this vision is currently difficult due to the high investment, electricity, and operational costs of VFS, as well as a lack of knowledge of sets of environmental conditions that optimize crop production and product quality.
Maintaining a constant environment in VFS is often considered an advantage over other cropping systems as it ensures year-round, on-demand, and uniform product quality and quantity, regardless of location or season. Also, it removes uncertainty over “Genotype × Environment” interactions that can slow down crop selection in plant breeding. Environmental constancy means reproducibility, and—at least theoretically—total control over every aspect of plant production. Additionally, unlike in the open field, growing conditions can be researched experimentally, and breeding for generally applicable, reproducible growing conditions can take place in the same environment as production, speeding up implementation and replication of growing conditions. However, the genotype and the developmental stage strongly dictate VFS demands for electricity, CO2, water, and nutrients and can also change diurnally. Since electricity is traded in day-ahead markets, prices tend to be driven by demand, and the lack of large-scale storability increases volatility of electricity prices much more than those of other commodities. In many electricity markets, prices change within a quarter of an hour. Consequently, the dynamics in electricity prices as well as in plant physiology and development mean that the relevant timescales for VFS environmental changes are in the range of several minutes to every few days.
Implementing more dynamic environmental control could positively impact crop growth, crop yield, energy use efficiency, and cost-effectiveness of VFS. In this review, we provide examples that highlight how dynamic climate control strategies influence plant growth and development that could be used to transform VFS-wide energy use efficiency, energy costs, and product quality. We then outline crucial developments for improved monitoring of plant physiology and consider how the breeding of genotypes specifically fit for VFS may enable next-generation crop production in VFS.
Plant growth and physiology under a dynamic environment
Optimizing photosynthesis and growth through dynamic climate settings
Plants constantly exchange gases with their environment. They take up CO2 and emit O2 when illuminated (photosynthesis), a process which is reversed in darkness (mitochondrial respiration). Additionally, plants take up water and nutrients through their roots and lose water vapor through their leaves (transpiration), during both the day and night. Photosynthetic gas exchange is environment-driven as it depends on light intensity, light spectrum, CO2 concentration, air humidity, temperature, and air movement, among other factors. While growers manage to fine-tune environmental parameters to optimize plant growth, gas exchange can sometimes vary even under perfectly stable environmental conditions. Across a number of species, photosynthesis declined by 10−40% in single leaves and whole plants when monitored during the day under constant growth conditions (10–13). Under the same conditions, stomatal conductance (which affects transpiration and photosynthesis) was found to vary by 30-60% (10–13). Together, these changes in plant physiology under stable climate conditions result in strong diurnal changes in light and water use efficiencies. A strategy for these changes would be to apply a higher light intensity in the first half of the photoperiod, when photosynthesis shows a higher light use efficiency. However, experimental evidence that such a strategy would improve whole-plant light use efficiency throughout the production cycle is lacking.
Plant growth is strongly affected by the total amount of light received, a response that is visible across species and treatments (Figure 1). At the same time, timing and patterns of light intensity during the photoperiod seem to have limited effects, as shown in tomato (Solanum lycopersicum), lettuce (Lactuca sativa), and chrysanthemum (Chrysanthemum morifolium): plants had similar growth and time-integrated photosynthesis rates when illuminated constantly during the photoperiod and when illuminated under controlled fluctuations in light intensity (15–17), or even when partly illuminated during random moments in the night (night breaks) instead of only during the day (18). This flexibility in plant response to light intensity patterns provides opportunities to address the high electricity demands of VFS, where electricity use represents 20−40% of production costs (19, 20) and artificial lighting consumes 60−85% of electricity (19, 21–23). These high electricity demands make growers vulnerable to electricity price volatility. Fortunately, this volatility also presents opportunities to profit from periods of low electricity prices (24). Usually, the price of electricity is known one day in advance, changes frequently throughout the day, and tends to be lower during the night (Figure 2A). Wholesale electricity prices can sometimes be negative (Figure 2B) due to an overload of the electricity grid, and at these times growers can earn money by using electricity (26). As the share of wind- and solar-derived electricity increases, electricity prices will probably become more volatile, increasing the relevance of dynamic VFS electricity consumption.
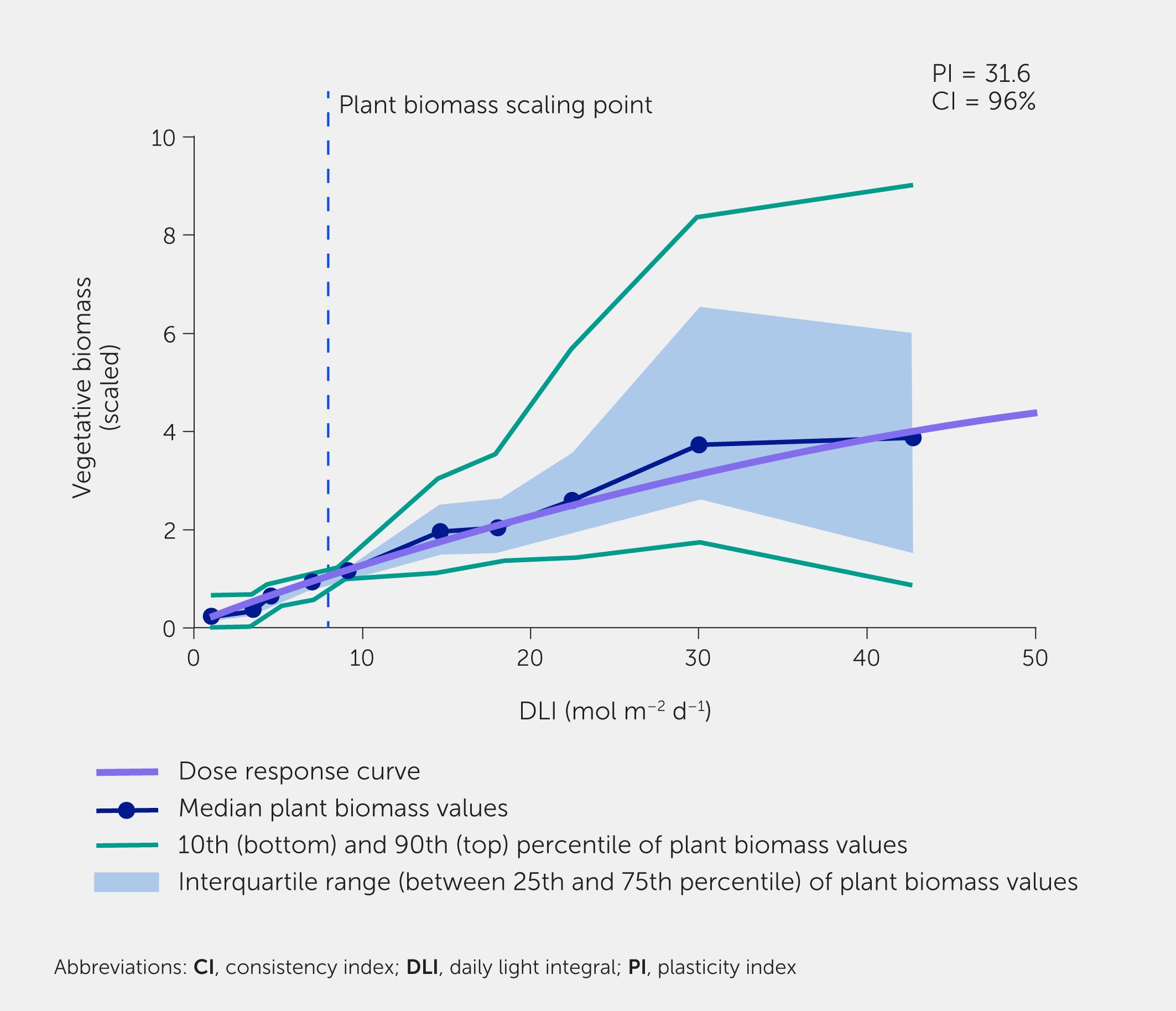
Figure 1. Plant biomass as a function of daily light integral (DLI), obtained using a meta-phenomics approach. Meta-analysis, based on 145 herbaceous species in 113 experiments, grown at various photosynthetic photon flux densities (PPFD). Each species in each experiment was grown at two or more light intensities while keeping other conditions similar. For each of these conditions, the DLI was calculated, which is the integral of PPFD over the photoperiod. Plant biomass per experiment and species was scaled to 8 mol m−2 d−1 (dashed blue line). Blue dots indicate median value for each decile of observations, grouped by their DLI. The purple line is the dose-response curve, fitted through all data. The blue-shaded area indicates the range between the 25th and 75th percentile of the scaled biomass value. The green lines indicate the 10th and 90th percentile. PI, Plasticity index, the ratio between the fitted scaled value at a DLI of 50 and 1 mol m−2 d−1; CI, Consistency index, percentage of species x experiment combinations where the biomass was higher for the treatment with the highest light intensity than for the treatment with the lowest light intensity. For more details on methodology, see (14).
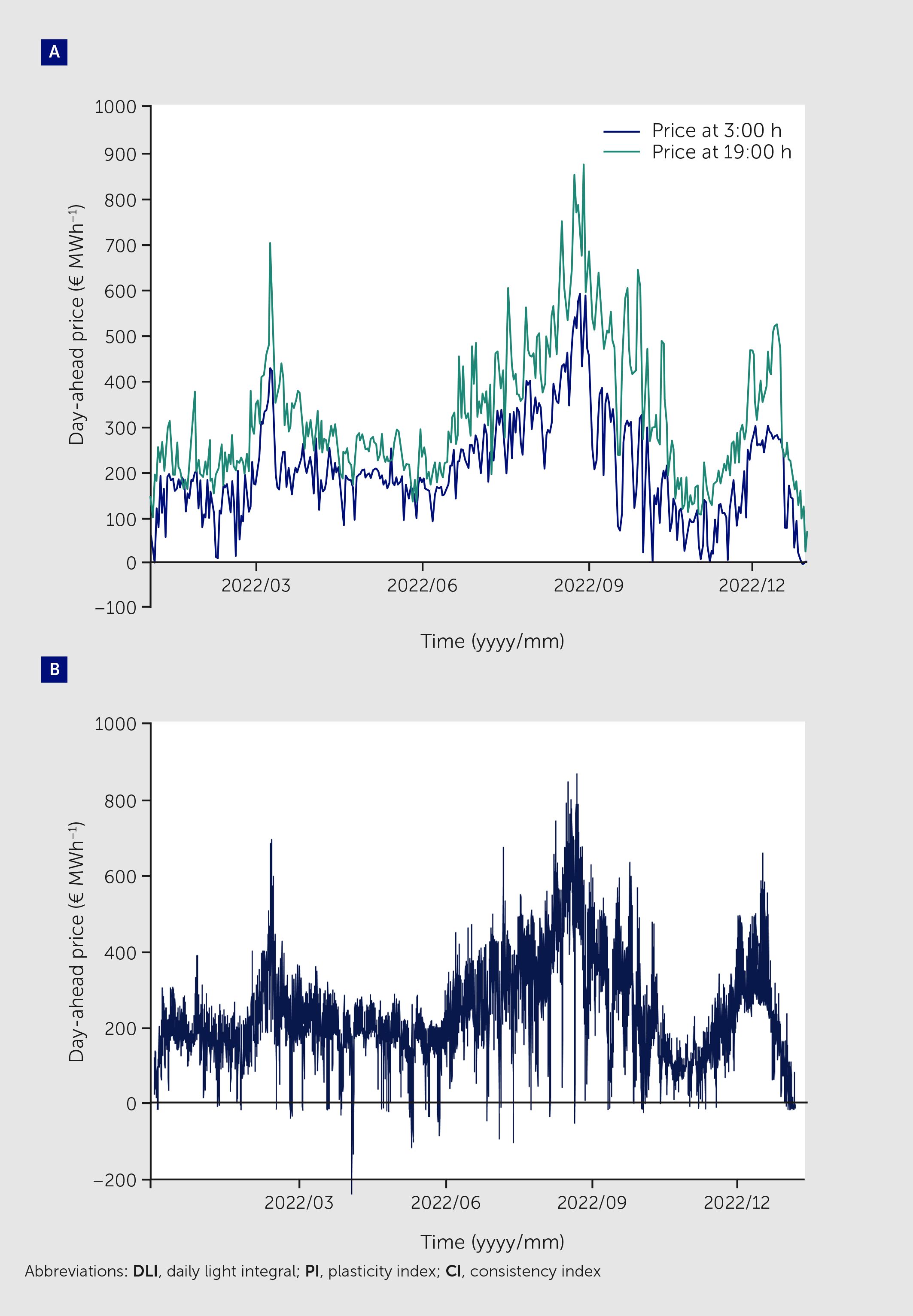
Figure 2. Day-ahead electricity prices in the Netherlands during 2022. (A) Daily prices at 3:00 and 19:00, to exemplify that electricity prices tend to be lower in the night (off-peak demand) than during the day (peak demand). (B) hourly prices, spanning from 871 € MWh−1 to –222.36 € MWh−1. Data were obtained from an online repository at ENTSO-E (25).
To optimize costs under variable electricity prices, one can aim to maintain the same total carbon fixation per day despite varying light intensity. We attempted to model this by predicting the leaf photosynthesis rate under dynamically changing light intensity using leaf-level gas exchange models (27–29). An optimization algorithm (Supplementary Data Sheet 1) altered light intensity every hour over a 17 h photoperiod to minimize daily electricity costs while maintaining diurnal leaf carbon gain (Figure 3). An extra hour of lighting was added to a standard 16 h photoperiod (31) to give the algorithm more freedom to distribute light over the photoperiod, while keeping the same daily light integral (DLI) as the constant light treatment. Using this smart lighting strategy, electricity costs for lighting were reduced by 12%, while calculated daily carbon gain was maintained (Figure 3). However, diurnal variations in light intensity are known to induce physiological and morphological (acclimation) responses (12, 32) that are difficult to predict and hence were not accounted for in the optimization algorithm. Also, the effectiveness of such algorithms to save electricity costs in VFS remains to be tested, especially at the whole-canopy level.
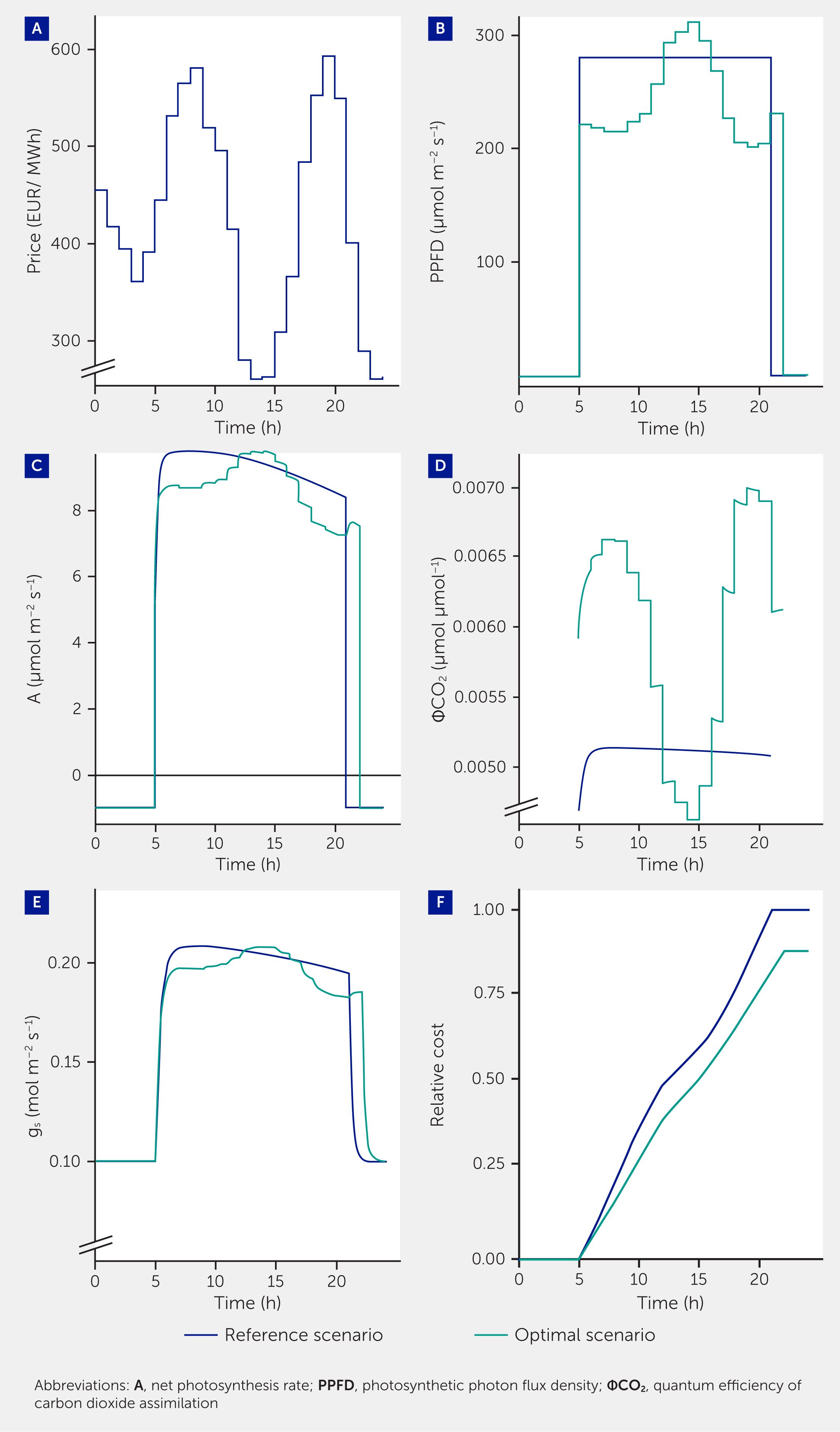
Figure 3. Distributing light intensity based on electricity cost can reduce costs in vertical farming systems (VFS). (A) Electricity price; (B) photosynthetic photon flux density (PPFD); (C) net photosynthesis rate (A); (D) quantum efficiency of CO2 assimilation (ΦCO2); (E) stomatal conductance (gs), and (F) relative cost of electricity over a 24h period. The blue line represents a reference scenario using constant light intensity during a 16 h photoperiod. The green line represents an optimal scenario, where the electricity cost over a 24h period was minimized (relative to the reference scenario) whilst maintaining the same daily net photosynthesis rate at the leaf level during a 17 h photoperiod. The dynamic leaf stomatal conductance and photosynthesis model used was derived from Lawson and Vialet-Chabrand (30).
Then, we aimed to determine how strongly the growth of “typical” VFS leafy herbs and vegetables—namely basil (Ocimum basilicum), pak choi (Brassica rapa subsp. chinensis), rucola (Diplotaxis tenuifolia), and spinach (Spinacia oleracea)—would be affected by hourly alterations in light intensity (Figure 4; for details on experimental procedures see Supplementary Data Sheet 2). We found that, compared to a constant light intensity, marketable fresh weight was unaffected in all genotypes (P>0.05 in all cases; Figures 4B, D, F, H) under either a regularly alternating high/low light intensity pattern (400/50 μmol m−2 s−1) or an irregularly changing light intensity pattern (range: 50−500 μmol m−2 s−1; Figure 4A); all three had the same DLI (12.96 mol m−2 d−1) and photoperiod (16 h). The irregular light intensity regime was designed to mimic changes in lamp output based on diurnally changing electricity prices (Figure 2). Further, we observed that, in pak choi (Figure 4E) and rucola (Figure 4G), specific leaf area was significantly increased under both dynamic light intensity patterns compared to constant light, suggesting that these genotypes formed thinner leaves—thus using leaf biomass more efficiently to capture light—when grown under dynamic light. Similar results were observed in tomato (16, 17) and in a meta-analysis across several other species (33); it may be that thinner leaves allow plants to use frequently changing light intensities more efficiently. Altogether, growth under a dynamic light intensity pattern such as is realistic in a VFS did not result in reduced growth, and some genotypes seemed to form thinner leaves when growing under dynamic light. However, it should be kept in mind that it is very likely that many combinations of light intensities (e.g., very large amplitudes and darkness) and frequencies (e.g., long exposure to very low light or darkness) exist that will substantially reduce growth, so caution should be exercised when applying a dynamic light intensity pattern.
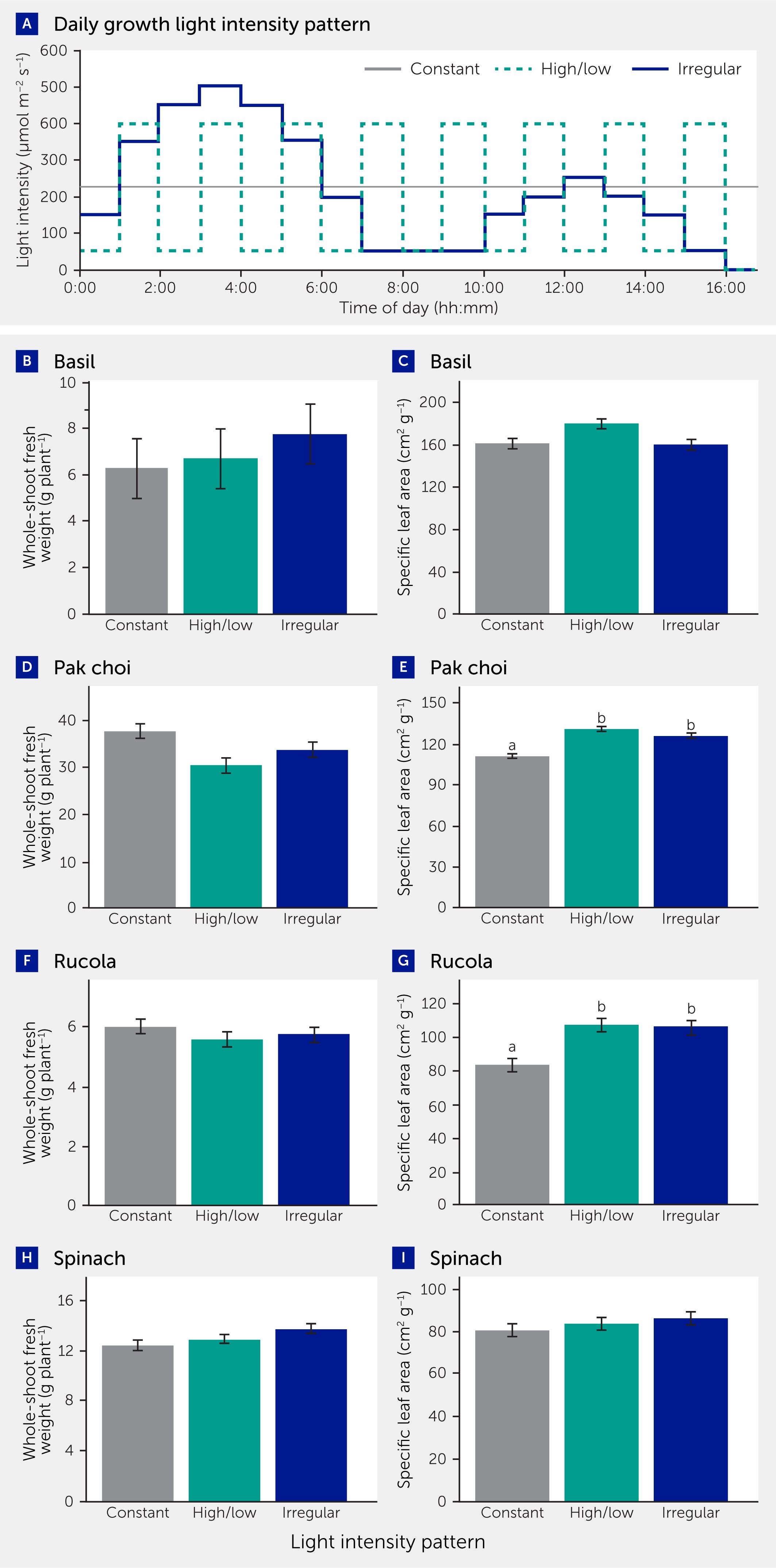
Figure 4. Biomass is not reduced under dynamic light patterns compared to constant light in four vertical farming systems crop species. (A) Treatment light intensity patterns. (B, D, F, H) Whole-shoot fresh weight in basil, pak choi, rucola, and spinach. (C, E, G, I) Specific leaf area, expressed in cm2 leaf area divided by g leaf dry weight. Plants were grown under the respective treatments for 30 (pak choi, rucola, spinach) and 40 days (basil). For more details on experimental procedures, see Supplementary Data Sheet 2. Bars in B-I depict average ± standard error of means, n=3, with 3−5 replicate plants per repetition. Different lowercase letters indicate significant (P<0.05) differences between treatments. Absence of letters indicates non-significant treatment effects.
Similar to lighting, air flow rates can be used to optimize leaf boundary conductance, which strongly affects stomatal responses to vapor pressure deficit, with greater responses under a higher air flow rate (34). Efficiently using frequent electricity price changes by adjusting light output (and potentially temperature, CO2 concentration, and air flow rates) therefore seems like a true opportunity for VFS, but this will require sophisticated plant monitoring and modeling approaches (see section on “Monitoring and prediction of plant physiology”).
In contrast to hourly changes in light intensity discussed above, the theory that high-frequency light intensity fluctuations (pulsed light whose intensity changes several times per second) may increase photosynthetic light use efficiency (compared to constant light of the same average intensity) has been debunked several times (35–37). However, pulsing specific wavelengths for several seconds at a time may have unique effects on plant morphology (see section on “Vegetative growth and development”).
In addition to light, plants also have a remarkable ability to acclimate to changes in temperature, enabling flexibility in VFS climate control [within up to ±6°C of average temperature (38, 39)] that may save energy (40). Fluctuations in temperature can lead to asynchrony between carbon supply (source) by photosynthesis and carbon demand (sink) by plant organs (41). Temporary storage and remobilization of carbohydrates allow plants to buffer these changes and act “as a battery” (39). A useful concept that integrates supply of and demand for carbohydrates is the photothermal ratio, which is the ratio between daily average light intensity and daily average temperature (42). Dynamic changes in temperature had minimal to no effects on biomass in tomato, probably due to dynamic carbohydrate storage (39). When production exceeds demand, such as when low temperature is combined with high light intensity (i.e., a high photothermal ratio), non-structural carbohydrates accumulate as starch and soluble sugars (43). During periods of low carbon supply, such as during the night or warm days with low light intensity (low photothermal ratio), remobilization of carbohydrates can support metabolism (44). This storage and re-mobilization occurs across multiple timescales: from one day, during which the photoperiod and circadian clock affect carbohydrate concentrations (45), to several days, when temperature and carbon status (source/sink balance) both determine carbohydrate remobilization (46). Together, DLI and temperature can be manipulated to use plants “as a battery”.
Vegetative growth and development
Plant growth in controlled environment agriculture is strongly driven by the amount of light intercepted by the plant, which in turn depends on projected leaf area. Therefore, optimizing vegetative growth in VFS can be achieved by an efficient and rapid establishment of leaf area, gradual increases in DLI over the growing period (47), dynamic plant spacing (48, 49), or a combination of these strategies. Especially when plants are young, rapid development and expansion of leaves are desirable to maximize light capture, photosynthesis, and growth, as light interception drives whole-canopy photosynthesis (50).
A common model suggests that organ expansion is largely controlled by the hydraulic status of the plant, where turgor pressure drives expansion and cell wall strength confines it (51, 52). Expansion may thus be maximized by dynamically manipulating the hydraulic status of the plant through environmental changes at either end of the rootzone-plant-atmosphere continuum. Conversely to this increase in expansion, a sudden increase in the electrical conductivity (EC) of the nutrient solution decreases its water potential, in turn decreasing water uptake (53), water potential in the elongation zone (54, 55), and leaf elongation rates (56). By contrast, in rose (Rosa hybrida), a sudden reduction in nutrient solution EC (1.0 to 0.1 dS m−1) resulted in a transient ~30% increase in stem elongation rate (57). Compared to a constant daily EC, a combination of low daytime and high nighttime EC in tomato increased fruit yield and plant biomass while reducing the incidence of blossom-end rot (58); see also section on “Product quality”). The opposite day/night combination caused negative effects (58), suggesting that high nighttime and low daytime EC can be beneficial for growth and yield. Altogether, a dynamic EC in the nutrient solution seems effective in modulating rates of organ expansion, but contradictory effects across studies and species suggest that this strategy requires further investigation.
According to the hydraulic model, elongation could also be regulated by changing the atmosphere around the plant: increasing air humidity (leading to reduced transpiration rate) has been shown to transiently increase elongation rate (59). Also, long-term exposure to high air humidity can increase disease pressure (60) and can cause stomata to be unresponsive to stimuli that usually induce closure, causing rapid wilting after harvest (61). Dynamically changing air humidity for several hours at a time may induce transient increases in leaf elongation rate without these negative side-effects, but whether this can improve yield over a growing cycle is unknown. Controlling stomatal conductance may be another method to modulate elongation: reducing blue light (B; 400−500 nm) intensity increases elongation rates—a response partially attributed to stomatal closure and less negative water potential in leaf expansion zones (62).
An unintended consequence of rapid leaf and canopy development can sometimes be the formation of low-quality leaves, which have a short shelf life after harvest or, in the case of tipburn, may not be marketable at all. The development of high-quality leaves, at least for leafy greens, is linked to slowly growing small cells, reduced cell wall extensibility, and fewer stomata (63). Modification of the lettuce cell wall, using transgenic lettuce with a reduction in the cell-wall-loosening enzyme xyloglucan endotransglucosylase/hydrolase (XTH), was associated with an extended shelf life (64). Also, while more expanded leaves may benefit light capture, excessively long stems may be undesirable in VFS due to lodging.
Light quality is a crucial parameter driving plant growth and physiological responses. Plants detect specific wavelengths via an elaborate set of photoreceptors: UV resistance locus 8 (UVR8), cryptochromes, phototropins, and phytochromes. Phytochromes primarily mediate plant responses to red (R) and far-red (FR; 700−800 nm) light and undergo reversible photoconversion between an inactive (Pr) and active (Pfr) state. The Pr state is primarily converted to Pfr by R, in turn limiting elongation growth. In contrast, Pfr is inactivated by FR, allowing elongation growth to occur. Elongation growth under low R:FR allows plants to outcompete neighboring vegetation for light when growing at high density (65). Plant responses to blue (B) light are predominately mediated by cryptochromes and phototropins (66, 67), and, to a lesser extent, phytochromes (68). In the shade, the fraction of B decreases (69, 70), and plants under such conditions display similar shade avoidance responses to those under low R:FR (71, 72). The combination of low R:FR with low B enhances petiole elongation more strongly than their combined action (73), highlighting the potential of applying these conditions in VFS to promote growth. Given that a low R:FR has been associated with accelerated flowering in some species (74–76), while low B has not (75, 77), it may be interesting to use a low R:FR ratio for expansion when flowering is desired and a low B fraction for expansion when flowering is unwanted.
Red LEDs have a higher efficacy than blue LEDs in µmol of photons produced per unit of electrical energy used (78). However, growing plants under monochromatic R results in excessive elongation (79) and decreased photosynthetic performance (80) due to a lack of activation of UV-A/blue-light-sensitive cryptochromes (80). Once cryptochrome photoreceptors are activated, deactivation in the absence of B can take several minutes (81), depending on temperature (82). Hence, it may be possible to maintain active cryptochrome photoreceptors when monochromatic R is interspersed with pulses of B, removing the red light syndrome while promoting plant growth at a reduced energy input compared to a constant application of B.
Applying short FR illumination periods at the end of the photoperiod can induce stem elongation and leaf expansion (83–85). Although effects tend to be weaker than applying FR for the entire photoperiod, the effectiveness per photon is higher at the end of the photoperiod (86). In lettuce, end-of-day (EOD) FR was more effective when applied alone rather than together with other wavelengths (85). The effectiveness of EOD FR may be because Pfr reverts slowly to Pr in darkness, and the slowly declining fraction of Pfr continues to suppress elongation in the dark until it has largely reverted to Pr. In contrast, an end-of-day FR treatment quickly and fully reverts Pfr to Pr, resulting in maximal elongation in the dark.
It may also be possible to apply fewer photons to achieve equivalent photomorphological effects using appropriately timed light pulses. In seedlings of turnip (B. rapa) and kale (B. napus), applying 5 s of FR (100 µmol m−2 s−1), followed by 5 s of darkness (repeatedly applied for 96 h) resulted in similar hypocotyl elongation as 5 s of FR and 10 s of darkness, indicating the same photomorphological effect while using 33% less light (87). In Arabidopsis thaliana, this effect was extended to an FR/dark ratio of 5/40 with no difference in hypocotyl length (87).
While the effect of FR on plant morphology through the shade avoidance syndrome might be desirable in VFS (i.e., initial elongation growth or early flowering), FR was shown to impair plant resistance toward pathogens (88–90). Understanding how plants detect and process light signals is key to designing dynamic light settings that promote growth while preserving disease resistance.
Apart from nutrient solution EC, humidity, and light spectrum, dynamic changes in temperature—especially the difference between day and night temperatures (DIF)—have been shown to be highly correlated with plant growth, with a positive DIF (higher day than night temperature) resulting in taller plants (91). Among many other species, striking responses were observed in Easter lily (Lilium longiflorum), where the same DIF resulted in similar heights at anthesis, even when average daily temperatures differed by up to 15°C (92). While DIF can be used to achieve a specific morphological response (taller plants with positive DIF and shorter plants with negative DIF), it could also be used to maintain uniformity across seasons. It is probably more cost-effective to maintain a lower average daily temperature in winter and a higher average daily temperature in summer. Also, as LED lamps emit heat as well as light, a positive DIF is probably more energetically favorable than a constant daily temperature. The average daily temperature influences plant height, with higher temperatures resulting in taller plants in some species (93), but, more importantly, higher average daily temperature increases rates of flower and leaf development (94, 95). To achieve a short phenotype in conditions where it may be too expensive to decrease the temperature for long periods of time, it may be beneficial to provide low temperatures for only ~2 h at a specific time of day (91, 95). This concept is called drop or dip, and while it is often reported to be most effective at the end of the night or the beginning of the photoperiod (91), results are inconsistent (96–98).
Stepwise increases in DLI [from 140 to 300 μmol m−2 s−1 photosynthetic photon flux density (PPFD)] every three days resulted in a 12% higher lettuce dry weight compared to a constant DLI when using equal total light sums during the crop cycle. This effect was attributed to the fact that plants could use high light intensity better at a high leaf area index (47). However, this study also revealed that, while the incident light use efficiency was highest for an increasing light intensity, intercepted light use efficiency was highest for a decreasing regime (47). Variable spacing may additionally maximize light use efficiency without inducing negative trade-offs that arise due to competition between plants (48, 49, 99, 100).
Given that the CO2 concentration for maximum plant growth is 1000-1200 ppm (101), which is substantially above the current atmospheric CO2 concentration [~420 ppm (102)], CO2 is typically enriched in VFS. In most cases, VFS rely on compressed gas for CO2 enrichment, although cooperation with neighboring CO2-producing industries is a possibility (103). Use of compressed gas should make it feasible and possibly more cost-effective to dynamically regulate CO2 concentration diurnally and throughout development, but this option is under-researched (103). What has been established is that nocturnal CO2 enrichment does not benefit growth, while CO2 enrichment during the photoperiod does (104). Also, fast changes in CO2 concentration (several changes per 10 min periods) resulted in greater biomass reductions compared to constant CO2 of the same average concentration (105, 106). However, whether slower changes in CO2 during the photoperiod or the crop cycle could benefit growth, cost savings, or product quality is unknown.
Flowering and fruiting
Leafy vegetables are the primary crops grown in VFS and are not grown to flower and fruit, as flowering decreases their value. However, the production of fruiting crops, edible flowers, and seeds (including speed breeding, a method to rapidly generate seeds for breeders) is now becoming increasingly relevant in VFS (107, 108). Hence flower induction, pollination, and fruit development must also be considered in the scope of dynamic VFS. For maximum yield in a fruiting crop, dynamic environmental control needs to strike a balance between vegetative growth on the one hand and optimum flowering and fruit development on the other.
Strawberries (Fragaria × ananassa) are of the perpetual or the seasonal flowering type, with perpetual types developing flowers and fruits under any daylength and seasonal flowering types developing flowers in long nights (short days). Young plants of either type are typically propagated via runners from mother plants and are initially grown under long-day conditions (typically a ≥16 h photoperiod). During subsequent development, interactions between daylength and temperature can influence vegetative growth and flower appearance. In seasonal flowering strawberries, maintaining long days or providing night-break lighting in short days can extend the vegetative period (109, 110), thereby ensuring sufficient vegetative growth to support fruit production. Under natural winter/spring daylengths in a greenhouse, night-break lighting with a high FR fraction stimulated leaf growth, boosting source strength for subsequent fruit growth (111).
Flowering and flower bud development of cannabis (Cannabis sativa), another short-day VFS crop, require photoperiods of <12−14 h (112), which limits its potential production rate due to a reduced DLI. Cannabis is typically grown under long-day conditions for several weeks before transitioning to short-day conditions that induce flowering. Chrysanthemum, another short-day plant, flowered when short days were extended to long days by pure B, as long as growth light contained no FR (113, 114), but whether this works in cannabis is unknown.
Following floral induction, dynamic environmental manipulation may improve pollination and fruit development. High air humidity can reduce fruit set in tomato and sweet pepper (Capsicum annuum), as it limits pollen release from the anthers (115, 116). On the other hand, high humidity promotes pollen adhesion to the stigmatic area and, thus, pollen germination (117). Because anthers generally open 2−4 h after sunrise, fruit set may be improved by first maintaining a low air humidity during the early morning and then increasing air humidity to promote pollen germination and fertilization during the day. Applying the opposite regime might prevent fruit set and could thus be used to reduce seasonal variations in fruit set.
High temperature increases the rate of bolting in lettuce (118), and FR accelerates flowering in several crops (119). However, high temperature also decreases fruit set (120). Therefore, it may be beneficial to initially apply high temperature to accelerate flowering and then to reduce it to ensure pollination and fruit set. In tomato, the heat-sensitive period for pollen production occurs during pollen mother cell meiosis, typically 1−2 weeks before flowering (121). In sweet pepper, the susceptible period for fruit abortion ranged from a few days before anthesis to two weeks after anthesis (122). During these sensitive periods, temperature must remain within a narrow range to safeguard yield (123).
During fruit growth, the addition of FR increases fruit sink strength in tomato, resulting in a larger fraction of dry mass partitioned to fruits and increased yield (124). Furthermore, FR applications during this phase increase foraging by bumblebees and, thus, pollination (125). However, these yield improvements from FR are achieved by reducing the partitioning of carbohydrates to the leaves, which may restrict source strength and plant growth. Also, in sweet pepper plants grown in a VFS-like environment, FR caused fruit abortion (126). FR can thus enhance fruit production, but its application should be approached cautiously due to its effects on the sink-source balance and its different effects in different crops.
Product quality
Quality is determined by a range of physiological and biochemical characteristics, including visual appearance, texture, taste, aroma, shelf life, nutritional compounds, and compounds attributed to physiological disorders. Often, environmental treatments aimed at improving quality tend to hinder yield: for example, in red lettuce, a large fraction of B enhanced anthocyanin concentrations and reduced fresh weight by 10−20% (127). As antioxidants, anthocyanins are often considered healthy (128), but they are also non-photosynthetic pigments that diminish the light available to photosynthesis, thereby potentially reducing growth. Such growth-quality trade-offs call for a dynamic strategy that first maximizes plant growth and development while optimizing product quality shortly before harvest through the use of “end-of-production” (EOP) treatments (129, 130). For instance, for leafy greens capable of anthocyanin production, reducing B initially and increasing its fraction toward the end of the production cycle may ensure sufficient yield and anthocyanin formation at harvest.
Several physiological disorders—tipburn in cabbage (B. oleracea) and lettuce leaves, calyx burn in strawberry fruit, blackheart in celery (Apium graveolens), and blossom-end rot in tomato and pepper fruits—are caused by a local deficiency of calcium (131, 132). These disorders are characterized as (typically dark) necrotic cells in developing organs. Calcium deficiencies result from inadequate uptake, transport, and/or local supply of calcium below concentrations required by a developing organ (133, 134) and typically occur when growth rates of that organ are high (135). Unlike other nutrients, calcium cannot be redistributed between organs through transport in the phloem, and its long-distance transport is restricted to the xylem. Low organ transpiration rate means low xylem flow and low calcium flux to the organ (131, 136). For example, tipburn typically occurs in the small immature leaves within a head of lettuce, which transpire less than mature leaves, due to the high humidity around young leaves. High rates of air flow close to young leaves can increase transpiration and reduce tipburn (137, 138), but high air flow can stunt growth (138, 139). Optimal timing of high air flow, to minimize tipburn without yield penalty, should be investigated. Another method to reduce tipburn is to concomitantly increase relative air humidity (> 95%) and decrease rootzone EC at night (140–142). Root pressure is caused by an accumulation of ions in the root xylem, inducing a more negative osmotic potential and high influx of water into the xylem, subsequently driving water upward (143). Moreover, calcium transport to the fruit and leaves are inversely related (133). To fulfill fruit calcium requirements, it is important to prevent high rates of whole-plant transpiration when fruits are developing. Leaf calcium-deficiency-related disorders tend to appear at specific moments in the production cycle, thus high temperature and high DLI could be provided initially for rapid growth and be decreased before symptoms begin to appear. It should also be noted that great genotypic variation exists, such as tipburn severity in lettuce (144, 145), so breeding is an additional instrument to minimize the symptoms caused by calcium-deficiency-related disorders.
The sensory and nutritional quality of plant products is greatly determined by the presence and concentrations of specific metabolites. Targeted short-term stresses can increase metabolite concentrations, since plants produce specialized metabolites to defend themselves against (a-)biotic stress. For example, high light intensity applied at EOP increased the concentrations of phenolic compounds and ascorbic acid in lettuce and basil (129, 130, 146). Contents of desired metabolites, such as cannabinoids in cannabis (147) or zeaxanthin, can be elevated by changing the light spectrum or by regular application of high light intensity flashes (148). Specific climate conditions can also be applied diurnally to maximize the synthesis of specialized compounds: for example, the composition of essential oils and other metabolites changed with the time of day in basil and thyme (Thymus vulgaris) (149–151). In addition to dynamic lighting strategies, controlled deficit irrigation increased concentrations of total soluble solids in tomato, enhancing flavor while marginally affecting yield (152).
In leafy vegetables that are grown under low light intensities, accumulation of nitrate can occur (153, 154), which is a human health risk (155). This problem may be tackled by applying light at EOP: an intensity of 35 μmol m−2 s−1 PPFD for one night was sufficient to reduce leaf nitrate concentrations in spinach by ~60% (156). Another possibility may be to reduce nitrate concentration in the nutrient solution shortly before harvest.
Shelf life is crucial for products derived from VFS, as it allows for retention of quality on products displayed in shops and kept in consumers’ homes. Shelf life is determined by physiological processes that ultimately lead to deterioration of quality features, including phytonutrient concentrations (157, 158). Compared to outdoor production, VFS probably reduce the number of microbes associated with spoilage on the surface of plant products, leading to reduced spoilage and potentially longer shelf life (159). Shelf life can be further improved by optimizing cultivation conditions, such that product quality takes longer to drop to unacceptable levels at the post-harvest stage. For example, increasing light intensity at EOP resulted in an extended shelf life of lettuce, probably due to higher carbohydrate and ascorbic acid contents (130): ascorbic acid diminishes the brown edges at the cut site of fresh-cut lettuce (130), while carbohydrates are substrates for respiration and can function as precursors for several antioxidants (160). Clarkson et al. (161) showed that harvesting at the end of the photoperiod, when concentrations of carbohydrates are maximal, can extend shelf life, a technique that can be easily applied in VFS. Generally, there is great potential for VFS to achieve desired product quality while maintaining high yields through dynamic growth conditions, but much remains to be understood to attain this goal.
Future developments
Monitoring and prediction of plant physiology
Because interactions between plants and their environments are highly complex, treating VFS as a dynamic system will work much more reliably if there is a deep understanding of how a given genotype responds to its environment, for example through assisted automated feedback. VFS offer unprecedented possibilities to monitor and manipulate the environment, but—like other growing systems (162)—they lack sensors that reliably and continuously monitor a representative fraction of the crop and provide actionable knowledge. Hence, while growers can monitor and change the environmental conditions continuously, they rely on their experience to judge the effects that those conditions have on their crop. Even so, the most experienced grower has a temporally and spatially limited overview of the crop: the grower can only see long-term changes (over several days), and local differences between plants may be difficult to discern. Furthermore, given that crop behavior can change diurnally [e.g., gas exchange (163) and leaf angle (164)] and throughout development (e.g., leaf morphology), climate variables need to be continuously adjusted to balance plant needs and VFS energy expenditures. Also, intelligently adjusting environmental conditions based on electricity prices (24) [see section on “Optimizing photosynthesis and growth through dynamic climate settings”] requires crop monitoring. Similarly, automated methods of early stress detection—and differentiation between stresses—are at an early stage of development. Scale of measurement and rate at which data can be converted into actionable knowledge are important considerations for the choice of sensors.
While many commercial systems provide insights into various aspects of plant physiology, these are typically too expensive to use on a large scale. Also, they often lack straightforward interpretations of plant environmental responses and hence are not often used for crop monitoring. The solution to crop monitoring will not come from a single sensor but rather a combination of sensors that inform on key physiological processes (and key environmental factors) that together provide a comprehensive picture and context of plant status. Given that there is a large suite of sensors that can provide signals on plant status, we constructed an overview of sensors that we deem suitable to provide actionable knowledge on relevant plant biological properties in a dynamic VFS context, and ranked them by throughput (Table 1). From this overview, several conclusions can be drawn: i) sensors use a wide variety of signals, including optical, audible, olfactory, and electrical signals; ii) while some sensors measure small areas and must be attached to single plants to function, others cover large areas and/or can be mobile, thereby greatly enhancing their throughput (number of plants measured per hour per sensor); and iii) sensors provide information across a large range of processes pertaining to plant growth (e.g., from photosynthetic electron transport rate to whole-plant growth), and these processes differ widely in how quickly they show a measurable change in the measured property or process (quantified as tΔ in Table 1).
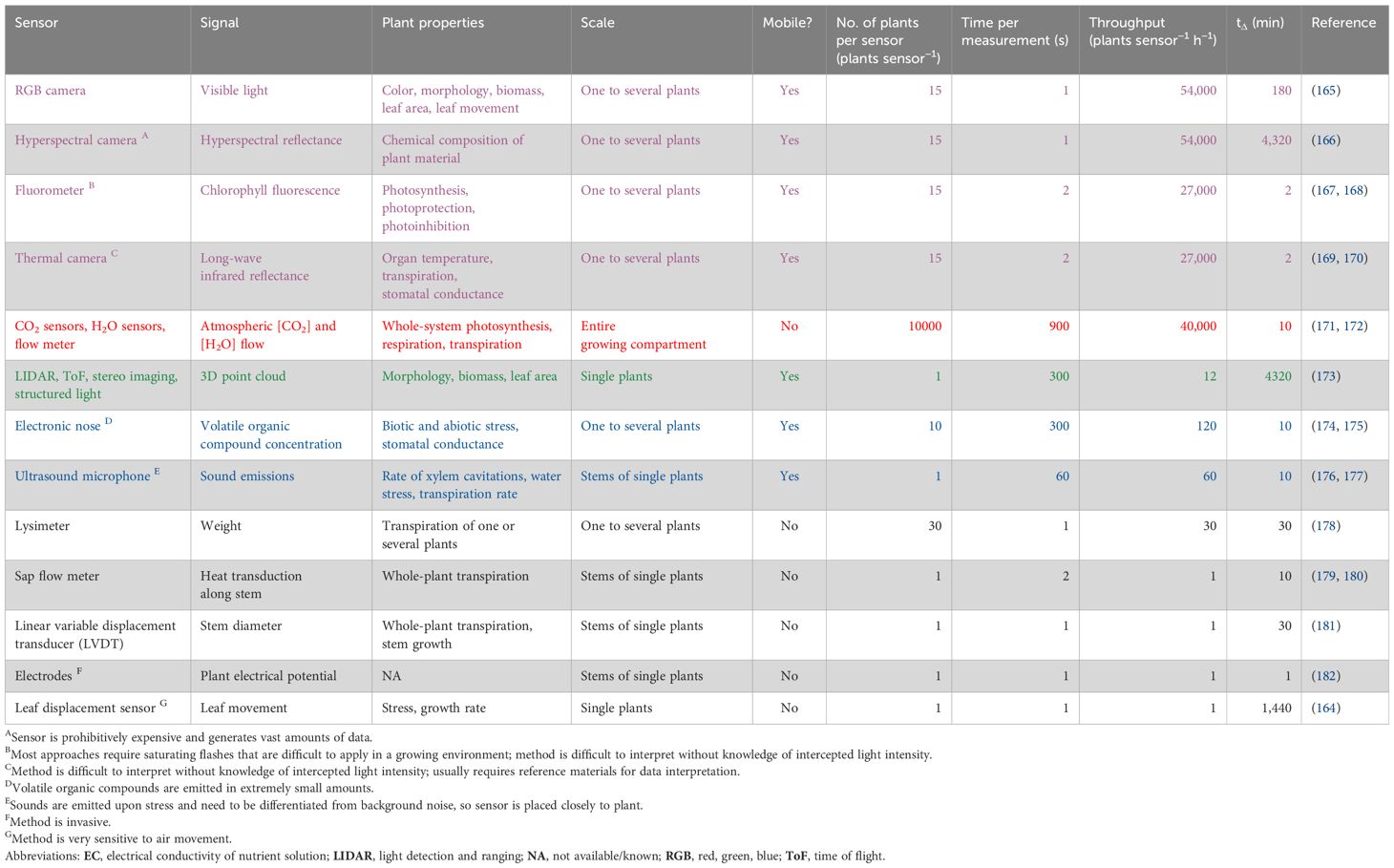
Table 1. Sensors for crop monitoring in vertical farming systems (VFS), ranked by throughput. Important considerations for use of a given sensor include the spatial scale of measurement, sensor mobility, number of plants that can be measured per hour (throughput), plant properties or processes that the sensor provides information on, and the time that it takes to detect a change in a measured property or process (tΔ). For mobile sensors, throughput was calculated as the number of plants that can be captured per measurement (plants sensor−1), multiplied by the duration of measurement (required time per measurement); for immobile sensors that are attached to one plant, throughput was set to 1 plant sensor−1 h−1. Values for throughput and tΔ presented in this table are based on referenced literature as well as own user experience and should be interpreted as rough estimates only. Sensors are color-coded according to how they are grouped in the text.
The largest group of sensors in Table 1 uses optical signals in the short- and longwave range (RGB, hyperspectral, chlorophyll fluorescence, and thermal imaging); therefore, they can be used as cameras, allowing for mobile, quick, and remote data acquisition. These sensors allow for a high degree of automation and are already in use in high-throughput plant phenotyping platforms (183). Another sensor approach (CO2 sensors, H2O sensors, and flow meters) assumes that a VFS can be used as a large cuvette, which exchanges CO2 and water vapor with its environment. Assuming that the crop is enclosed in a box, all inputs and outputs to the system (CO2 dosing, fertigation, and removal of water vapor from the air) are potentially quantifiable through the sensors that monitor aerial CO2 concentration and humidity, as well as whole-system irrigation input. This may enable the monitoring of long-term trends in whole-system photosynthesis and transpiration (184). While this approach has been shown to work in research greenhouses (171, 172), it might be challenging to use in crop production due to lack of proper air mixing (leading to inaccurate sampling), leaks, and standardization when plants are added or removed. To solve the last problem and update knowledge on biomass and leaf area, light detection and ranging (LIDAR), time-of-flight (ToF), stereo imaging, and structured light can be used to acquire 3D point clouds. If time series of various physiological and architectural parameters can be monitored, patterns of plant growth and development can, in principle, be derived. Such data sets, together with key environmental data, enable the development of highly mechanistic models of plant growth, which can be used to develop digital twins and decision support tools for VFS. Such combined datasets could be used to feed artificial intelligence models to enable control of microenvironments inside VFS.
Electronic noses and ultrasound microphones can sample part of the system, as they can measure signals that are emitted when plants are stressed. Electronic noses measure volatile organic compounds, and ultrasound microphones detect sounds emitted due to xylem embolisms that occur under drought stress (176, 177). The remaining five sensors in Table 1 (leaf displacement sensor, linear variable displacement transducer, lysimeter, sap flow meter, and electrodes) are all immobile, which strongly limits their throughput but does enable long-term monitoring on individual plants. Also, it should be noted that several researchers have recently reported on “plant wearable sensors”, which show some promise in plant monitoring (185). However, these are not included here, since they are often cumbersome to attach to plants (i.e., expensive to operate) and generate data that are often difficult to interpret.
The rate at which actionable knowledge can be generated will depend on both sensor throughput and time it takes to detect a change in a measured property or process (tΔ). Plotting throughput vs. tΔ for all sensor types (Figure 5) revealed that sensors disperse widely along both axes, suggesting that all combinations exist: low-throughput sensors that measure slow changes in plant status (e.g., leaf displacement sensors), high-throughput sensors that measure rapid changes (e.g., thermal cameras and fluorometers), and several others in between. Depending on the application, combining fast, mobile sensors with slow, immobile sensors seems a promising strategy for achieving well-informed and automated decision-making inside dynamically regulated VFS. However, to provide actionable information, data generated by sensors need to be curated, analyzed, and interpreted correctly, which remains a bottleneck, as does the lack of affordable sensors.
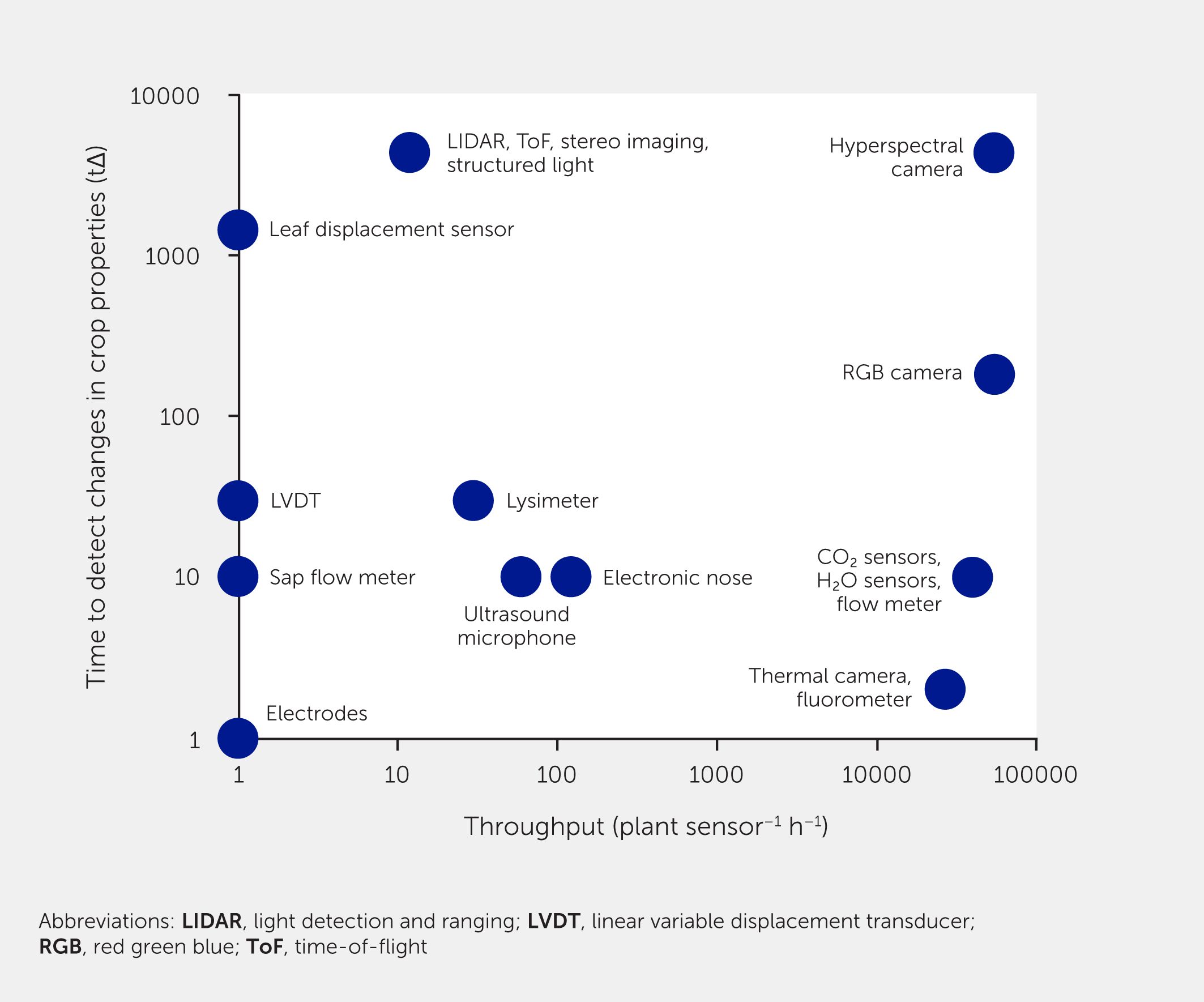
Figure 5. Sensors for crop monitoring in vertical farming systems. Sensors are plotted by throughput vs. time required to detect a change in crop property that a given sensor measures (tΔ; log-log plot). Numbers presented in this figure are best estimates, based on referenced literature (Table 1) as well as user experience, and should be interpreted as rough estimates only.
Mathematical models, including physiological and machine learning-based models, will play a crucial role in the integration of information from different sensors. Models can guide decision-making to optimize on-the-fly growth environment optimization. Among different models developed at various spatial and temporal scales, dynamic systems models of canopy photosynthesis can integrate data of canopy architecture and physiological parameters from different layers of a canopy (186). Predicted canopy-wide photosynthesis can be used to determine light intensity setpoints for maximal productivity or photosynthetic light use efficiency, for example in the context of changing electricity prices (Figures 2, 3). Such sensor-model systems can also help avoid extreme setpoints, such that when the model suggests setpoints under extremely low or high electricity prices, it does so within boundaries that are defined by prior knowledge and sensor data. Furthermore, with the development of models simulating the dynamic acclimation of the photosynthetic apparatus to light intensity and quality (187), light intensity can be optimized to maximize whole-canopy photosynthesis for several days, with a full consideration of canopy formation and, hence, light interception history of different leaves.
Breeding for the VFS environment
Controlled environments are often used to develop new germplasm—but what about breeding for production in VFS (188)? A breeder’s goal is to coalesce a set of desirable traits that express appropriately in a specific environment, and a crop bred for the field may not grow at its maximum genetic potential in an artificial environment. Plant genetic improvement for the field must consider an ever-changing environment, replete with biotic and abiotic stresses and potentially wild extremes. Breeders of open field crops thus need to develop genotypes that produce marketable products regardless of environmental fluctuations. A VFS is a far departure from sun, soil, and outdoor stresses, even with controlled changes in the VFS environment, as discussed in this review. The lack of variety designed for highly controlled environments can therefore be considered a bottleneck. Also, over the last century, plant genetic improvement has focused on production traits: improvements in size, disease resistance, yield, and post-harvest storage life have sacrificed quality traits like flavor, texture, color, and aroma (189). In VFS, on the other hand, due to favorable environmental conditions and fewer disease threats, breeders can select for performance under a discrete set of conditions that focus on consumer-centered qualities and high growth rates under a relatively constant climate with comparably low light intensity, elevated [CO2], and otherwise benign conditions (188).
Apart from the development of a highly compact, early-yielding tomato variety with high harvest index (190), few published examples of plants bred specifically for VFS exist. The paucity may be because proprietary lines comprise the best examples, and information about those is not disclosed. Some unpublished examples do exist and, as always, necessity was the mother of invention: cannabis growers were the pioneers in this space, as controlled environment agriculture offered discreet, secret production. The use of indoor locations led cannabis growers to desire compact plants. Cannabis sativa grows tall compared to C. indica, which produces compact plants with short internodes. Other favorable traits include flowering on long days and an evenly spread leaf area to optimize light interception. Breeders have selected for flower buds to be produced close to a compact stem or buds to be produced in clusters on different branches. The downside of compact, dense plants is the incidence of fungal diseases. Cannabis breeders also have selected for cannabinoids, as well as for traits that facilitate growth in controlled environments: heat tolerance, disease resistance, and resistance to insects and arachnids.
Inside VFS, there is great potential to breed for improved photosynthetic properties. First, light intensity is generally lower than in the field, which makes it possible to breed for crops with higher photosynthetic rate under low light, a feature that has so far received little attention. In rice, photosynthesis under low light intensity differed ~2.8× between cultivars (191). It is expected that this trait can be used as a breeding target in any species. Furthermore, although VFS can supply a stable light environment, light intensity inside a leaf is highly spatially and temporally heterogeneous. Due to optical scattering and focusing effects inside the leaf, light intensity hitting a particular chloroplast may be up to 15 times higher than the incident light on the leaf (192). Due to tiny changes in leaf position caused by high air flow or intrinsically triggered leaf movement, strong changes in incident light intensity can occur (192), as can lamp output that responds to changes in electricity prices (see section on “Photosynthesis and transpiration”). Breeding for VFS crops with an enhanced capacity to respond to fluctuating light intensity thus seems useful, and the potential for improving these properties has been demonstrated in the lab and field (193–196).
An attractive breeding priority might be cultivars that possess great environmental plasticity and thus produce different traits in response to varying environmental conditions (197). This contrasts with what is expected of field plants, where phenotypic consistency is desired in a changing environment. Classical breeding techniques for field crops emphasized production traits such as disease resistance, shipping quality, and shelf life. This led to the selection of mass-produced fruits and vegetables that were lacking in sensory quality (189). Selection for controlled environment agriculture allows a shift to improved flavors and aromas, due to the short distance between VFS and end consumers. For instance, FR increases the aroma, perception of sweetness, and improved texture of tomatoes more than R or B against a background of solar light (198, 199). Enhancement of FR sensing circuits, perhaps by breeding genotypes that are hypersensitive to the activation of phytochromes, may result in better tasting fruits. Also, depending on whether flowering is desired or unwanted, varieties that feature an insensitivity or hypersensitivity of floral initiation to specific wavebands may be bred.
An important prerequisite for agricultural production is the ease of harvest (200). Controlled environments are much more amenable to robotic harvest than the field because of their standardized conditions. Robots can work in conditions that are perilous for humans, such as on tall buildings or under high-intensity UV light (201). In some crops, the efficiency and feasibility of automated harvest is strongly affected by plant architecture, as this is guided by machine vision to detect ripe fruits (202). Breeding for uniformity in fruit size, ripening, color, and distribution may thus aid in automatic harvest. For example, the JOINTLESS gene in tomatoes controls the detachment force (203); selective breeding to alter fruit abscission may benefit robotic harvest, as whole trusses of ripe fruit could be harvested together. Similarly, peppers that produce widely spaced fruits rather than fruit clusters are more amenable to robotic harvest (204). Control of peduncle growth is critical to mechanical harvesting in cucumber (Cucumis sativus) (205). In these cases, the genetics that underlie these traits are at least partially understood.
Finally, while many examples in this section emphasize the opportunities that lie in breeding for a constant environment in VFS, the next step can be imagined easily: breeding for a dynamic yet well-controlled environment with clearly defined boundaries per environmental variable. Such an environment will still be far removed from nature (due to the general constancy, repeatability, and controllability in VFS) and therefore should in theory be much easier to breed for than the field. Marker-assisted breeding may be used to speed up the development of lines for VFS, and gene editing may help instill traits across species.
Conclusions and outlook
In this review, we present many examples that show the benefits of managing the growth environment in VFS in a dynamic manner (Figure 6A). We also explain how—and how quickly—changes in the environment affect various processes in plants. Changes in VFS environments are broadly motivated by two factors, namely the physiology and development of the crop on the one hand (changing the “demand function” for specific inputs within the system) and changes in electricity prices on the other (changing the “supply function” of a major input to the system), which can be used to change the cost-effectiveness of VFS. A large suite of processes in the plant is affected by the environment, from short-term changes (e.g., photosynthesis rate) to longer-term processes that drive development (e.g., flower initiation; Figure 6A); therefore, it is not surprising that variations in the environment will affect these processes as well. Plants integrate and respond to all environmental stimuli at once, which can make it difficult to predict how plants will respond to any single stimulus (206). Therefore, we consider it crucial to implement dynamic climate control in VFS together with sensors that monitor relevant plant processes, at a high enough spatial and temporal resolution to make informed decisions, and models that use the information provided by the sensors to provide setpoints (Figure 6B). Given rapid developments in nanoscale sensor technology (207), as well as data integration and modeling approaches [including machine learning (208)], we foresee massive improvements in how dynamic climate setpoint optimization and plant monitoring could work together in future growing systems (162).
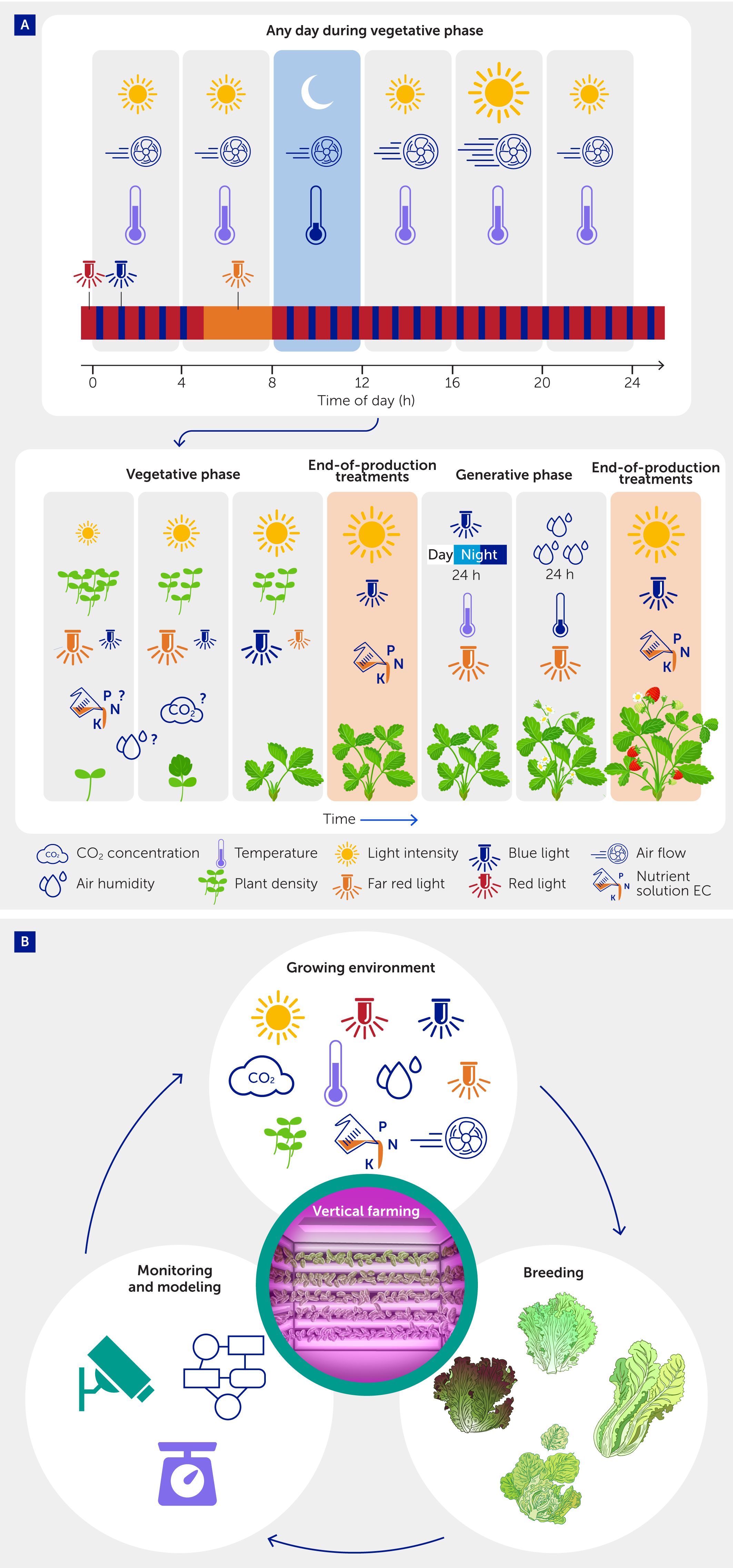
Figure 6. Future vision of a dynamic vertical farming system (VFS). (A) Examples of dynamic changes in the growth environment during different phases of the production cycle, as well as during any day during the vegetative phase. Depending on the product, the production cycle may end with the vegetative phase [when an end-of-production treatment (EOP) can be applied] or with seed/fruit formation after a transition to the vegetative phase (EOP may be applied to ripening fruits). Symbol size depicts relative intensity or concentration. Question marks signify unknown or contradictory effects for dynamic changes of given factors. All changes are depicted as examples only and are neither exact nor exhaustive; for details, see text (sections on “Photosynthesis and transpiration”, “Flowering and fruiting”, and “Product quality”). (B) An intelligent dynamic VFS should be integrated with crop monitoring and modeling, as well as with breeding that is specialized for this environment, in a continuous feedback loop between these domains that aims to optimize resource use efficiency, product quality, and energy costs.
It should be stressed that vertical farming, and the research thereof, is a relatively recent foray for the agricultural industry. Furthermore, much of VFS research to date has focused on cultivation in constant climate conditions. With the ever-increasing capacity to fine-tune and apply dynamic conditions in VFS, this review highlights some insights and approaches that we believe contribute to the advent of a truly dynamic VFS industry. Additionally, specific processes highlighted in this review have been tested at low spatial and temporal levels: these are often results of lab experiments tested on specific organs over short periods of time, not at canopy-scale or over longer time spans that include different phases of growth and development. Such experimentation should take place before implementing any proposed solution in VFS, as responses at a lower integration level often do not scale up to higher integration levels as expected (209). Several potential applications of dynamic VFS thus await experimental verification at the relevant spatial and temporal scales.
In the long term, fine-tuning of dynamic environmental conditions, monitoring, and breeding for growth under these conditions should go hand in hand (Figure 6B), in a continuous feedback loop between crop management, crop monitoring, and trait selection, to optimize cost-effectiveness, resource use efficiency, or product quality. Boundaries to dynamic environmental conditions will be defined clearly and will be based on knowledge of the plant’s biology, a constant stream of monitoring data and its interpretation using process-based and machine learning-based models, prices of inputs, and market demands. Finally, the current energy crisis, as well as questions about the environmental sustainability of VFS compared to other growing systems (22, 210, 211), may force us to rethink cultivation approaches and the proposed future role of VFS in agriculture. Making environmental conditions in VFS more dynamic is an important step toward making crop cultivation in VFS more sustainable and cost-effective.
Supplementary material
The Supplementary Material for this article can be found online at: https://www.frontiersin.org/articles/10.3389/fsci.2024.1411259/full#supplementary-material
Acknowledgments
The authors thank Wilma Slegers for drawing the conceptual figure and Arnold Moene, Peter Steeneken, Wim van Ieperen, and Ep Heuvelink for fruitful discussions on technical aspects of the paper.
Statements
Author contributions
EK: Conceptualization, Data curation, Formal Analysis, Investigation, Project administration, Visualization, Writing – original draft, Writing – review & editing. PK: Visualization, Writing – original draft, Writing – review & editing. SV-C: Data curation, Formal Analysis, Investigation, Writing – original draft, Writing – review & editing. KF: Writing – original draft. YL: Writing – original draft, Writing – review & editing. HP: Data curation, Formal Analysis, Investigation, Writing – original draft, Writing – review & editing. NW: Data curation, Formal Analysis, Investigation, Writing – original draft, Writing – review & editing. SS: Data curation, Formal Analysis, Investigation, Writing – original draft, Writing – review & editing. AC: Data curation, Formal Analysis, Investigation, Writing – original draft, Writing – review & editing. JB: Writing – original draft, Writing – review & editing. MK: Writing – original draft, Writing – review & editing. YJ: Writing – original draft, Writing – review & editing. SD: Writing – original draft, Writing – review & editing. CZ: Writing – original draft, Writing – review & editing. XZ: Writing – original draft, Writing – review & editing. KH: Writing – original draft, Writing – review & editing. JV: Writing – original draft, Writing – review & editing. EW: Writing – original draft, Writing – review & editing. PG: Writing – original draft, Writing – review & editing. SC: Writing – original draft, Writing – review & editing. GT: Writing – original draft, Writing – review & editing. LM: Conceptualization, Project administration, Visualization, Writing – original draft, Writing – review & editing.
Data availability statement
The raw data supporting the conclusions of this article will be made available by the authors, without undue reservation.
Funding
The author(s) declare financial support was received for the research, authorship, and/or publication of this article.
The authors PK, YL, JB, YJ, SD, KH, JV, EW, and LM received support from the TTW Perspectief programme “Sky High”, which is funded by the Dutch Research Council (NWO), AMS Institute, Bayer, Bosman van Zaal, Certhon, Fresh Forward, Grodan, Growy, Own Greens/Vitroplus, Priva, Signify, Solynta, Unilever, and Van Bergen Kolpa Architects, as well as the Foundation for Food and Agriculture (FFAR).
The authors SV-C, XZ, and MK received funding from the Merian Fund for the NWO-CAS projects “Twinergy” and “Greenfarm”.
The author SS received support from the “Synergia” program, which is funded by NWO (NWO Crossover grant 17626).
The author AC received funding from the research grant IT1461-22 provided by Basque Country (Spain).
The author SC received funding from the Deutsche Forschungsgemeinschaft (DFG, German Research Foundation) under Germany's Excellence Strategy (CIBSS – EXC-2189 – Project ID 390939984).
The funders were not involved in the study design, collection, analysis, interpretation of data, the writing of this article, or the decision to submit it for publication.
Conflict of interest
The authors declare that the research was conducted in the absence of any commercial or financial relationships that could be construed as a potential conflict of interest.
The authors EK, PK, YL, YJ, XX, KH, JV, EW, PG, SC and LM declared that they were an editorial board member of Frontiers, at the time of submission. This had no impact on the peer review process and the final decision.
Publisher’s note
All claims expressed in this article are solely those of the authors and do not necessarily represent those of their affiliated organizations, or those of the publisher, the editors and the reviewers. Any product that may be evaluated in this article, or claim that may be made by its manufacturer, is not guaranteed or endorsed by the publisher.
Abbreviations
B, blue light (400–500 nm); DIF, difference between day and night temperature (°C); DLI, daily light integral (mol photons m−2 d−1); EC, electrical conductivity (dS m−1); EOP, end of production; FR, far-red light (700–800 nm); G, green light (500–600 nm); LED, light-emitting diode; LIDAR, light detection and ranging; Pfr, far-red-absorbing (active) form of phytochrome; PPFD, photosynthetic photon flux density (μmol photons m−2 s−1; 400–700 nm); Pr, red-absorbing (inactive) form of phytochrome; R, red light (600–700 nm); ToF, time of flight; tΔ, time required to detect a change in plant process (min); VFS, vertical farming system.
References
1. Ji Y, Kusuma P, Marcelis LFM. Vertical farming. Curr Biol (2023) 33(11):R471–3. doi: 10.1098/rspb.2016.2569
2. van Delden SH, SharathKumar M, Butturini M, Graamans LJA, Heuvelink E, Kacira M, et al. Current status and future challenges in implementing and upscaling vertical farming systems. Nat Food (2021) 2(12):944–56. doi: 10.1038/s43016-021-00402-w
3. Asseng S, Guarin JR, Raman M, Monje O, Kiss G, Despommier DD, et al. Wheat yield potential in controlled-environment vertical farms. Proc Natl Acad Sci USA (2020) 117(32):19131–5. doi: 10.1073/pnas.2002655117
4. Germer J, Sauerborn J, Asch F, de Boer J, Schreiber J, Weber G, et al. Skyfarming an ecological innovation to enhance global food security. J Verbr Lebensmittelsicherh (2011) 6(2):237–51. doi: 10.1007/s00003-011-0691-6
5. Hunter MC, Smith RG, Schipanski ME, Atwood LW, Mortensen DA. Agriculture in 2050: recalibrating targets for sustainable intensification. BioScience (2017) 67(4):386–91. doi: 10.1093/biosci/bix010
6. Avgoustaki DD, Xydis G. How energy innovation in indoor vertical farming can improve food security, sustainability, and food safety? Adv Food Secur Sustain (2020) 5:1–51. doi: 10.1016/bs.af2s.2020.08.002
7. Food and Agriculture Organization of the United Nations, International Fund for Agricultural Development, United Nations Children’s Fund, World Food Programme, World Health Organization. The state of food security and nutrition in the world 2023. Rome: FAO (2023). doi: 10.4060/cc3017en
8. Oh S, Lu C. Vertical farming - smart urban agriculture for enhancing resilience and sustainability in food security. J Hortic Sci Biotechnol (2023) 98(2):133–40. doi: 10.1080/14620316.2022.2141666
9. Ritchie H, Rosado P, Roser M. Share of electricity production by source, World [online] (2024). Available at: https://ourworldindata.org/grapher/share-elec-by-source
10. Lanoue J, Leonardos ED, Ma X, Grodzinski B. The effect of spectral quality on daily patterns of gas exchange, biomass gain, and water-use-efficiency in tomatoes and Lisianthus: an assessment of whole plant measurements. Front Plant Sci (2017) 8:1076. doi: 10.3389/fpls.2017.01076
11. Lanoue J, Leonardos ED, Grodzinski B. Effects of light quality and intensity on diurnal patterns and rates of photo-assimilate translocation and transpiration in tomato leaves. Front Plant Sci (2018) 9:756. doi: 10.3389/fpls.2018.00756
12. Matthews JSA, Vialet-Chabrand S, Lawson T. Acclimation to fluctuating light impacts the rapidity of response and diurnal rhythm of stomatal conductance. Plant Physiol (2018) 176(3):1939–51. doi: 10.1104/pp.17.01809
13. Mencuccini M, Mambelli S, Comstock J. Stomatal responsiveness to leaf water status in common bean (Phaseolus vulgaris L.) is a function of time of day. Plant Cell Environ (2000) 23(10):1109–18. doi: 10.1046/j.1365-3040.2000.00617.x
14. Poorter H, Niinemets Ü, Ntagkas N, Siebenkäs A, Mäenpää M, Matsubara S, et al. A meta-analysis of plant responses to light intensity for 70 traits ranging from molecules to whole plant performance. New Phytol (2019) 223(3):1073–105. doi: 10.1111/nph.15754
15. Bhuiyan R, van Iersel MW. Only extreme fluctuations in light levels reduce lettuce growth under sole source lighting. Front Plant Sci (2021) 12:619973. doi: 10.3389/fpls.2021.619973
16. Kaiser E, Matsubara S, Harbinson J, Heuvelink E, Marcelis LFM. Acclimation of photosynthesis to lightflecks in tomato leaves: interaction with progressive shading in a growing canopy. Physiol Plant (2018) 162(4):506–17. doi: 10.1111/ppl.12668
17. Zhang Y, Kaiser E, Marcelis LFM, Yang Q, Li T. Salt stress and fluctuating light have separate effects on photosynthetic acclimation, but interactively affect biomass. Plant Cell Environ (2020) 43(9):2192–206. doi: 10.1111/pce.13810
18. Kjaer KH, Ottosen C-O. Growth of chrysanthemum in response to supplemental light provided by irregular light breaks during the night. J Amer Soc Hort Sci (2011) 136(1):3–9. doi: 10.21273/JASHS.136.1.3
19. Avgoustaki DD, Xydis G. Energy cost reduction by shifting electricity demand in indoor vertical farms with artificial lighting. Biosyst Eng (2021) 211:219–29. doi: 10.1016/j.biosystemseng.2021.09.006
20. Kozai T. Contribution of PFALs to the sustainable development goals and beyond. In: Kozai T, Niu G, Masabni JG, editors. Plant factory basics, applications and advances. London: Academic Press (2022). 57–79. doi: 10.1016/B978-0-323-85152-7.00016-1
21. Ahamed MS, Sultan M, Monfet D, Rahman MS, Zhang Y, Zahid A, et al. A critical review on efficient thermal environment controls in indoor vertical farming. J Cleaner Prod (2023) 425:138923. doi: 10.1016/j.jclepro.2023.138923
22. Graamans L, Baeza E, van den Dobbelsteen A, Tsafaras I, Stanghellini C. Plant factories versus greenhouses: comparison of resource use efficiency. Agric Syst (2018) 160:31–43. doi: 10.1016/j.agsy.2017.11.003
23. Song R, Liu D, Pan Y, Cheng Y, Meng C. Container farms: energy modeling considering crop growth and energy-saving potential in different climates. J Cleaner Prod (2023) 420:138353. doi: 10.1016/j.jclepro.2023.138353
24. Mitchell C, Stutte GW. Sole-source lighting for controlled-environment agriculture. In: Lopez R, Runkle ES, editors. Light Management in Controlled Environments. Willoughby: Meister Media Worldwide (2015). 119–34. Available at: https://ntrs.nasa.gov/api/citations/20150009399/downloads/20150009399.pdf
25. European Network of Transmission System Operators for Electricity. Day-ahead electricity prices for the Netherlands. ENTSO-E Transparency Platform. Available at: https://transparency.entsoe.eu/transmission-domain/r2/dayAheadPrices/show?name=&defaultValue=false&viewType=GRAPH&areaType=BZN&atch=false&dateTime.dateTime=20.10.2023+00:00|CET|DAY&biddingZone.values=CTY|10YNL———L!BZN|10YNL———L&resolution.values=PT15M&resolution.values=PT30M&resolution.values=PT60M&dateTime.timezone=CET_CEST&dateTime.timezone_input=CET+(UTC+1)+/+CEST+(UTC+2)
26. Biber A, Felder M, Wieland C, Spliethoff H. Negative price spiral caused by renewables? Electricity price prediction on the German market for 2030. Electr J (2022) 35(8):107188. doi: 10.1016/j.tej.2022.107188
27. Vialet-Chabrand S, Dreyer E, Brendel O. Performance of a new dynamic model for predicting diurnal time courses of stomatal conductance at the leaf level. Plant Cell Environ (2013) 36(8):1529–46. doi: 10.1111/pce.12086
28. Vialet-Chabrand S, Matthews JSA, Brendel O, Blatt MR, Wang Y, Hills A, et al. Modelling water use efficiency in a dynamic environment: an example using Arabidopsis thaliana. Plant Sci (2016) 251:65–74. doi: 10.1016/j.plantsci.2016.06.016
29. Vialet-Chabrand S, Matthews JSA, Lawson T. Light, power, action! Interaction of respiratory energy- and blue light-induced stomatal movements. New Phytol (2021) 231(6):2231–46. doi: 10.1111/nph.17538
30. Lawson T, Vialet-Chabrand S. Speedy stomata, photosynthesis and plant water use efficiency. New Phytol (2019) 221(1):93–8. doi: 10.1111/nph.15330
31. Zelkind M, Livingston T, Verlage V. Indoor production of tomatoes. In: Kozai T, Niu G, Masabni JG, editors. Plant factory basics, applications and advances. London: Academic Press (2022). 295–305. doi: 10.1016/B978-0-323-85152-7.00008-2
32. Vialet-Chabrand S, Matthews JSA, Simkin AJ, Raines CA, Lawson T. Importance of fluctuations in light on plant photosynthetic acclimation. Plant Physiol (2017) 173(4):2163–79. doi: 10.1104/pp.16.01767
33. Morales A, Kaiser E. Photosynthetic acclimation to fluctuating irradiance in plants. Front Plant Sci (2020) 11:268. doi: 10.3389/fpls.2020.00268
34. Bunce JA. Effect of boundary layer conductance on the response of stomata to humidity. Plant Cell Environ (1985) 8(1):55–7. doi: 10.1111/j.1365-3040.1985.tb01209.x
35. Jishi T, Matsuda R, Fujiwara K. Effects of photosynthetic photon flux density, frequency, duty ratio, and their interactions on net photosynthetic rate of cos lettuce leaves under pulsed light: explanation based on photosynthetic-intermediate pool dynamics. Photosynth Res (2018) 136(3):371–8. doi: 10.1007/s11120-017-0470-z
36. Sager JC, Giger W. Re-evaluation of published data on the relative photosynthetic efficiency of intermittent and continuous light. Agric Meteorol (1980) 22(3–4):289–302. doi: 10.1016/0002-1571(80)90008-4
37. Tennessen DJ, Bula RJ, Sharkey TD. Efficiency of photosynthesis in continuous and pulsed light emitting diode irradiation. Photosynth Res (1995) 44(3):261–9. doi: 10.1007/BF00048599
38. de Koning A. Development and dry matter distribution in glasshouse tomato: a quantitative approach [thesis]. Wageningen: Wageningen University (1994). Available at: https://edepot.wur.nl/205947
39. Zepeda AC, Heuvelink E, Marcelis LFM. Non-structural carbohydrate dynamics and growth in tomato plants grown at fluctuating light and temperature. Front Plant Sci (2022) 13:968881. doi: 10.3389/fpls.2022.968881
40. van Beveren PJM, Bontsema J, van Straten G, van Henten EJ. Optimal control of greenhouse climate using minimal energy and grower defined bounds. Appl Energy (2015) 159:509–19. doi: 10.1016/j.apenergy.2015.09.012
41. Gibon Y, Bläsing OE, Palacios-Rojas N, Pankovic D, Hendriks JHM, Fisahn J, et al. Adjustment of diurnal starch turnover to short days: depletion of sugar during the night leads to a temporary inhibition of carbohydrate utilization, accumulation of sugars and post-translational activation of ADP-glucose pyrophosphorylase in the following light period. Plant J (2004) 39(6):847–62. doi: 10.1111/j.1365-313X.2004.02173.x
42. Poorter H, Fiorani F, Pieruschka R, Wojciechowski T, van der Putten WH, Kleyer M, et al. Pampered inside, pestered outside? Differences and similarities between plants growing in controlled conditions and in the field. New Phytol (2016) 212(4):838–55. doi: 10.1111/nph.14243
43. Palacio S, Hoch G, Sala A, Körner C, Millard P. Does carbon storage limit tree growth? New Phytol (2014) 201(4):1096–100. doi: 10.1111/nph.12602
44. Dietze MC, Sala A, Carbone MS, Czimczik CI, Mantooth JA, Richardson AD, et al. Nonstructural carbon in woody plants. Annu Rev Plant Biol (2014) 65(1):667–87. doi: 10.1146/annurev-arplant-050213-040054
45. Graf A, Schlereth A, Stitt M, Smith AM. Circadian control of carbohydrate availability for growth in Arabidopsis plants at night. Proc Natl Acad Sci USA (2010) 107(20):9458–63. doi: 10.1073/pnas.0914299107
46. Pilkington SM, Encke B, Krohn N, Höhne M, Stitt M, PYL ET. Relationship between starch degradation and carbon demand for maintenance and growth in Arabidopsis thaliana in different irradiance and temperature regimes. Plant Cell Environ (2015) 38(1):157–71. doi: 10.1111/pce.12381
47. Jin W, Ji Y, Larsen DH, Huang Y, Heuvelink E, Marcelis LFM. Gradually increasing light intensity during the growth period increases dry weight production compared to constant or gradually decreasing light intensity in lettuce. Sci Hortic (2023) 311. doi: 10.1016/j.scienta.2022.111807
48. Karpe M, Marcelis LFM, Heuvelink E. Dynamic plant spacing in tomato results in high yields while mitigating the reduction in fruit quality associated with high planting densities. Front Plant Sci (2024) 15:1386950. doi: 10.3389/fpls.2024.1386950
49. Prince RP, Bartok JW Jr. Plant spacing for controlled environment plant growth. Trans ASAE (1978) 21(2):332–6. doi: 10.13031/2013.35299
50. Beadle CL, Long SP. Photosynthesis - is it limiting to biomass production? Biomass (1985) 8(2):119–68. doi: 10.1016/0144-4565(85)90022-8
51. Boyer JS, Silk WK. Hydraulics of plant growth. Funct Plant Biol (2004) 31(8):761–73. doi: 10.1071/FP04062
52. Hilty J, Muller B, Pantin F, Leuzinger S. Plant growth: the what, the how, and the why. New Phytol (2021) 232(1):25–41. doi: 10.1111/nph.17610
53. Carvajal M, Martínez V, Alcaraz CF. Physiological function of water channels as affected by salinity in roots of paprika pepper. Physiol Plant (1999) 105(1):95–101. doi: 10.1034/j.1399-3054.1999.105115.x
54. Cramer GR, Bowman DC. Kinetics of maize leaf elongation: I. Increased yield threshold limits short-term, steady-state elongation rates after exposure to salinity. J Exp Bot (1991) 42(11):1417–26. doi: 10.1093/jxb/42.11.1417
55. Thiel G, Lynch J, Läuchli A. Short-term effects of salinity stress on the turgor and elongation of growing barley leaves. J Plant Physiol (1988) 132(1):38–44. doi: 10.1016/S0176-1617(88)80180-9
56. Munns R, Passioura JB, Guo J, Chazen O, Cramer GR. Water relations and leaf expansion: importance of time scale. J Exp Bot (2000) 51(350):1495–504. doi: 10.1093/jexbot/51.350.1495
57. Oki LR, Lieth JH. Effect of changes in substrate salinity on the elongation of Rosa hybrida L. “Kardinal” stems. Sci Hortic (2004) 101(1–2):103–19. doi: 10.1016/j.scienta.2003.09.013
58. Ieperen van W. Effects of different day and night salinity levels on vegetative growth, yield and quality of tomato. J Hortic Sci (1996) 71(1):99–111. doi: 10.1080/14620316.1996.11515386
59. Passioura JB, Munns R. Rapid environmental changes that affect leaf water status induce transient surges or pauses in leaf expansion rate. Funct Plant Biol (2000) 27(10):941–8. doi: 10.1071/PP99207
60. Roberts JM, Bruce TJA, Monaghan JM, Pope TW, Leather SR, Beacham AM. Vertical farming systems bring new considerations for pest and disease management. Ann Appl Biol (2020) 176(3):226–32. doi: 10.1111/aab.12587
61. Fanourakis D, Bouranis D, Giday H, Carvalho DRA, Rezaei Nejad A, Ottosen CO. Improving stomatal functioning at elevated growth air humidity: a review. J Plant Physiol (2016) 207:51–60. doi: 10.1016/j.jplph.2016.10.003
62. Barillot R, De Swaef T, Combes D, Durand JL, Escobar-Gutiérrez AJ, Martre P, et al. Leaf elongation response to blue light is mediated by stomatal-induced variations in transpiration in Festuca arundinacea. J Exp Bot (2021) 72(7):2642–56. doi: 10.1093/jxb/eraa585
63. Clarkson GJJ, O’Byrne EE, Rothwell SD, Taylor G. Identifying traits to improve postharvest processability in baby leaf salad. Postharvest Biol Technol (2003) 30(3):287–98. doi: 10.1016/S0925-5214(03)00110-8
64. Wagstaff C, Clarkson GJJ, Zhang F, Rothwell SD, Fry SC, Taylor G, et al. Modification of cell wall properties in lettuce improves shelf life. J Exp Bot (2010) 61(4):1239–48. doi: 10.1093/jxb/erq038
65. Smith H. Phytochromes and light signal perception by plants - an emerging synthesis. Nature (2000) 407(6804):585–91. doi: 10.1038/35036500
66. Hirose F, Inagaki N, Hanada A, Yamaguchi S, Kamiya Y, Miyao A, et al. Cryptochrome and phytochrome cooperatively but independently reduce active gibberellin content in rice seedlings under light irradiation. Plant Cell Physiol (2012) 53(9):1570–82. doi: 10.1093/pcp/pcs097
67. Inoue SI, Kinoshita T, Matsumoto M, Nakayama KI, Doi M, Shimazaki KI. Blue light-induced autophosphorylation of phototropin is a primary step for signaling. Proc Natl Acad Sci USA (2008) 105(14):5626–31. doi: 10.1073/pnas.0709189105
68. Shinomura T, Nagatani A, Hanzawa H, Kubota M, Watanabe M, Furuya M. Action spectra for phytochrome A- and B-specific photoinduction of seed germination in Arabidopsis thaliana. Proc Natl Acad Sci USA (1996) 93(15):8129–33. doi: 10.1073/pnas.93.15.8129
69. Franklin KA. Photomorphogenesis: plants feel blue in the shade. Curr Biol (2016) 26(24):R1275–6. doi: 10.1016/j.cub.2016.10.039
70. Gautier H, Varlet-Grancher C. Regulation of leaf growth of grass by blue light. Physiol Plant (2008) 98(2):424–30. doi: 10.1034/j.1399-3054.1996.980227.x
71. Ballaré CL. Keeping up with the neighbours: phytochrome sensing and other signalling mechanisms. Trends Plant Sci (1999) 4(3):97–102. doi: 10.1016/S1360-1385(99)01383-7
72. Keuskamp DH, Sasidharan R, Vos I, Peeters AJM, Voesenek LACJ, Pierik R. Blue-light-mediated shade avoidance requires combined auxin and brassinosteroid action in Arabidopsis seedlings. Plant J (2011) 67(2):208–17. doi: 10.1111/j.1365-313X.2011.04597.x
73. de Wit M, Keuskamp DH, Bongers FJ, Hornitschek P, Gommers CMM, Reinen E, et al. Integration of phytochrome and cryptochrome signals determines plant growth during competition for light. Curr Biol (2016) 26(24):3320–6. doi: 10.1016/j.cub.2016.10.031
74. Devlin PF, Patel SR, Whitelam GC. Phytochrome E influences internode elongation and flowering time in Arabidopsis. Plant Cell (1998) 10(9):1479–87. doi: 10.1105/tpc.10.9.1479
75. Park Y, Runkle ES. Far-red radiation and photosynthetic photon flux density independently regulate seedling growth but interactively regulate flowering. Environ Exp Bot (2018) 155:206–16. doi: 10.1016/j.envexpbot.2018.06.033
76. Smith H, Whitelam GC. The shade avoidance syndrome: multiple responses mediated by multiple phytochromes. Plant Cell Environ (1997) 20(6):840–4. doi: 10.1046/j.1365-3040.1997.d01-104.x
77. Meng Q, Runkle ES. Regulation of flowering by green light depends on its photon flux density and involves cryptochromes. Physiol Plant (2019) 166(3):762–71. doi: 10.1111/ppl.12832
78. Kusuma P, Pattison PM, Bugbee B. From physics to fixtures to food: current and potential LED efficacy. Hortic Res (2020) 7(1):56. doi: 10.1038/s41438-020-0283-7
79. Kochetova GV, Avercheva OV, Bassarskaya EM, Kushunina MA, Zhigalova TV. Effects of red and blue LED light on the growth and photosynthesis of barley (Hordeum vulgare L.) seedlings. J Plant Growth Regul (2023) 42(3):1804–20. doi: 10.1007/s00344-022-10661-x
80. Hogewoning SW, Trouwborst G, Maljaars H, Poorter H, van Ieperen W, Harbinson J. Blue light dose-responses of leaf photosynthesis, morphology, and chemical composition of Cucumis sativus grown under different combinations of red and blue light. J Exp Bot (2010) 61(11):3107–17. doi: 10.1093/jxb/erq132
81. Herbel V, Orth C, Wenzel R, Ahmad M, Bittl R, Batschauer A. Lifetimes of Arabidopsis cryptochrome signaling states in vivo. Plant J (2013) 74(4):583–92. doi: 10.1111/tpj.12144
82. Pooam M, Dixon N, Hilvert M, Misko P, Waters K, Jourdan N, et al. Effect of temperature on the Arabidopsis cryptochrome photocycle. Physiol Plant (2021) 172(3):1653–61. doi: 10.1111/ppl.13365
83. Boccalandro HE, Rugnone ML, Moreno JE, Ploschuk EL, Serna L, Yanovsky MJ, et al. Phytochrome B enhances photosynthesis at the expense of water-use efficiency in Arabidopsis. Plant Physiol (2009) 150(2):1083–92. doi: 10.1104/pp.109.135509
84. López-Juez E, Buurmeijer WF, Heeringa GH, Kendrick RE, Wesselius JC. Response of light-grown wild-type and long hypocotyl mutant cucumber plants to end-of-day far-red light. Photochem Photobiol (1990) 52(1):143–9. doi: 10.1111/j.1751-1097.1990.tb01767.x
85. Zou J, Fanourakis D, Tsaniklidis G, Cheng R, Yang Q, Li T. Lettuce growth, morphology and critical leaf trait responses to far-red light during cultivation are low fluence and obey the reciprocity law. Sci Hortic (2021) 289. doi: 10.1016/j.scienta.2021.110455
86. Kalaitzoglou P, van Ieperen W, Harbinson J, van der Meer M, Martinakos S, Weerheim K, et al. Effects of continuous or end-of-day far-red light on tomato plant growth, morphology, light absorption, and fruit production. Front Plant Sci (2019) 10:322. doi: 10.3389/fpls.2019.00322
87. Song S, Kusuma P, Carvalho SD, Li Y, Folta KM. Manipulation of seedling traits with pulsed light in closed controlled environments. Environ Exp Bot (2019) 166. doi: 10.1016/j.envexpbot.2019.103803
88. Courbier S, Grevink S, Sluijs E, Bonhomme PO, Kajala K, Van Wees SCM, et al. Far-red light promotes Botrytis cinerea disease development in tomato leaves via jasmonate-dependent modulation of soluble sugars. Plant Cell Environ (2020) 43(11):2769–81. doi: 10.1111/pce.13870
89. Courbier S, Snoek BL, Kajala K, Li L, van Wees SCM, Pierik R. Mechanisms of far-red light-mediated dampening of defense against Botrytis cinerea in tomato leaves. Plant Physiol (2021) 187(3):1250–66. doi: 10.1093/plphys/kiab354
90. Ji Y, Ouzounis T, Courbier S, Kaiser E, Nguyen PT, Schouten HJ, et al. Far-red radiation increases dry mass partitioning to fruits but reduces Botrytis cinerea resistance in tomato. Environ Exp Bot (2019) 168:103889. doi: 10.1016/j.envexpbot.2019.103889
91. Myster J, Moe R. Effect of diurnal temperature alternations on plant morphology in some greenhouse crops—a mini review. Sci Hortic (1995) 62(4):205–15. doi: 10.1016/0304-4238(95)00783-P
92. Erwin JE, Heins RD, Karlsson MG. Thermomorphogenesis in lilium longiflorum. Am J Bot (1989) 76(1):47–52. doi: 10.1002/j.1537-2197.1989.tb11283.x
93. Grimstad SO, Frimanslund E. Effect of different day and night temperature regimes on greenhouse cucumber young plant production, flower bud formation and early yield. Sci Hortic (1993) 53(3):191–204. doi: 10.1016/0304-4238(93)90067-Z
94. Karlsson MG, Heins RD, Erwin JE. Quantifying temperature-controlled leaf unfolding rates in “Nellie White” Easter Lily. J Amer Soc Hort Sci (1988) 113(1):70–4. doi: 10.21273/JASHS.113.1.70
95. Moe R, Heins RD. Thermo- and photomorphogenesis in plants. Adv Floriculture Res Rep (2000) 6:52–64
96. Berghage R. Controlling height with temperature. HortTechnology (1998) 8(4):535–9. doi: 10.21273/HORTTECH.8.4.535
97. Moe R, Mortensen LM, Grimstad SO. Control of plant morphogenesis without growth retardants. In: Hayashi M, Kano A, Goto E, editors. International Symposium on Transplant Production Systems. Yokohama: Acta Horticulturae (1992). 319:323–8. doi: 10.17660/ActaHortic.1992.319.50
98. Titov AF, Shibaeva TG, Ikkonen EN, Sherudilo EG. Plant responses to a daily short-term temperature drop: phenomenology and mechanisms. Russ J Plant Physiol (2020) 67(6):1003–17. doi: 10.1134/S1021443720060187
99. Carotti L, Pistillo A, Zauli I, Meneghello D, Martin M, Pennisi G, et al. Improving water use efficiency in vertical farming: effects of growing systems, far-red radiation and planting density on lettuce cultivation. Agric Water Manag (2023) 285:108365. doi: 10.1016/j.agwat.2023.108365
100. Jin W, Urbina JL, Heuvelink E, Marcelis LFM. Adding far-red to red-blue light-emitting diode light promotes yield of lettuce at different planting densities. Front Plant Sci (2020) 11:609977. doi: 10.3389/fpls.2020.609977
101. Poorter H, Knopf O, Wright IJ, Temme AA, Hogewoning SW, Graf A, et al. A meta-analysis of responses of C3 plants to atmospheric CO2: dose–response curves for 85 traits ranging from the molecular to the whole-plant level. New Phytol (2022) 233(4):1560–96. doi: 10.1111/nph.17802
102. National Aeronautics and Space Administration. Carbon dioxide [online] (2024). Available at: https://climate.nasa.gov/vital-signs/carbon-dioxide/
103. Wang A, Lv J, Wang J, Shi K. CO2 enrichment in greenhouse production: towards a sustainable approach. Front Plant Sci (2022) 13:1029901. doi: 10.3389/fpls.2022.1029901
104. Baker JT, Lascano RJ, Yates C, III DCG. Nighttime CO2 enrichment did not increase leaf area or shoot biomass in cotton seedlings. Agric For Meteorol (2022) 320:108931. doi: 10.1016/j.agrformet.2022.108931
105. Allen LH, Kimball BA, Bunce JA, Yoshimoto M, Harazono Y, Baker JT, et al. Fluctuations of CO2 in Free-Air CO2 Enrichment (FACE) depress plant photosynthesis, growth, and yield. Agric Forest Meteorol (2020) 284:107899. doi: 10.1016/j.agrformet.2020.107899
106. Bunce JA. Responses of cotton and wheat photosynthesis and growth to cyclic variation in carbon dioxide concentration. Photosynthetica (2012) 50(3):395–400. doi: 10.1007/s11099-012-0041-7
107. Park Y, Gómez C, Runkle ES. Indoor production of ornamental seedlings, vegetable transplants, and microgreens. In: Kozai T, Niu G, Masabni JG, editors. Plant factory basics, applications and advances. London: Academic Press (2022). 351–75. doi: 10.1016/B978-0-323-85152-7.00020-3
108. SharathKumar M, Heuvelink E, Marcelis LFM. Vertical Farming: moving from genetic to environmental modification. Trends Plant Sci (2020) 25(8):724–7. doi: 10.1016/j.tplants.2020.05.012
109. Vince-Prue D, Guttridge CG, Buck MW. Photocontrol of petiole elongation in light-grown strawberry plants. Planta (1976) 131(2):109–14. doi: 10.1007/BF00389978
110. Vince-Prue D, Guttridge CG. Floral initiation in strawberry: spectral evidence for the regulation of flowering by long-day inhibition. Planta (1973) 110(2):165–72. doi: 10.1007/BF00384839
111. Lieten F. Effects of chilling and night-break treatment on greenhouse production of “Elsanta”. In: van der Scheer HATH, Lieten F, Dijkstra J, editors. III International Strawberry Symposium. Veldhoven: Acta Horticulturae (1997). 439(2):633–40. doi: 10.17660/ActaHortic.1997.439.105
112. Moher M, Jones M, Zheng Y. Photoperiodic response of in vitro Cannabis sativa plants. Hortscience (2021) 56(1):108–13. doi: 10.21273/HORTSCI15452-20
113. Kohler AE, Birtell EM, Runkle ES, Meng Q. Day-extension blue light inhibits flowering of Chrysanthemum when the short main photoperiod includes far-red light. J Am Soc Hortic Sci (2023) 148(2):89–98. doi: 10.21273/JASHS05294-23
114. SharathKumar M, Heuvelink E, Marcelis LFM, van Ieperen W. Floral induction in the short-day plant Chrysanthemum under blue and red extended long-days. Front Plant Sci (2020) 11:610041. doi: 10.3389/fpls.2020.610041
115. Bakker JC. The effects of air humidity on flowering, fruit set, seed set and fruit growth of glasshouse sweet pepper (Capsicum annuum L.). Sci Hortic (1989) 40(1):1–8. doi: 10.1016/0304-4238(89)90002-2
116. van Koot IJ, van Ravestijn W. The germination of tomato pollen on the stigma In: International Horticultural Congress. Proceedings of the 16th International Horticultural Congress. Gembloux: Editions J. Duculot (1962). 2:452–61
117. Picken AJF. A review of pollination and fruit set in the tomato (Lycopersicon esculentum Mill.). J Hortic Sci (1984) 59(1):1–13. doi: 10.1080/00221589.1984.11515163
118. Ito H, Kato T, Konno Y. Factors associated with the flower induction in lettuce. Tohoku J Agric Res (1963) 2:51–65. Available at: https://tohoku.repo.nii.ac.jp/record/101506/files/KJ00000714076.pdf
119. Demotes-Mainard S, Péron T, Corot A, Bertheloot J, Le Gourrierec J, Pelleschi-Travier S, et al. Plant responses to red and far-red lights, applications in horticulture. Environ Exp Bot (2016) 121:4–21. doi: 10.1016/j.envexpbot.2015.05.010
120. Abdul-Baki AA, Stommel JR. Pollen viability and fruit set of tomato genotypes under optimum-and high-temperature regimes. Hortscience (1995) 30(1):115–7. doi: 10.21273/HORTSCI.30.1.115
121. Karapanos IC, Shreef M, Thanopoulos C. Fruit set in solanaceous vegetable crops as affected by floral and environmental factors. Eur J Plant Sci Biotechnol (2008) 2(1):88–105. Available at http://www.globalsciencebooks.info/Online/GSBOnline/images/0812/EJPSB_2(SI1)/EJPSB_2(SI1)88-105o.pdf
122. Marcelis LF, Heuvelink E, Hofman-Eijer LRB, Bakker JD, Xue LB. Flower and fruit abortion in sweet pepper in relation to source and sink strength. J Exp Bot (2004) 55(406):2261–8. doi: 10.1093/jxb/erh245
123. Peet MM, Sato S, Gardner RG. Comparing heat stress effects on male-fertile and male-sterile tomatoes. Plant Cell Environ (1998) 21(2):225–31. doi: 10.1046/j.1365-3040.1998.00281.x
124. Ji Y, Nuñez Ocaña D, Choe D, Larsen DH, Marcelis LFM, Heuvelink E. Far-red radiation stimulates dry mass partitioning to fruits by increasing fruit sink strength in tomato. New Phytol (2020) 228(6):1914–25. doi: 10.1111/nph.16805
125. Meijer D, van Doesburg F, Jungerling L, Weldegergis BT, Kappers IF, Van Oystaeyen AV, et al. Supplemental far-red light influences flowering traits and interactions with a pollinator in tomato crops. Environ Exp Bot (2023) 213:105438. doi: 10.1016/j.envexpbot.2023.105438
126. Chen S, Marcelis LFM, Heuvelink E. Far-red radiation increases flower and fruit abortion in sweet pepper (Capsicum annuum L.). Sci Hortic (2022) 305. doi: 10.1016/j.scienta.2022.111386
127. Nicole CCS, Krijn MPCM, van Slooten U. Postharvest quality of leafy greens growing in a plant factory. In: Anpo M, Fukuda H, Wada T, editors. Plant factory using artificial light. Amsterdam: Elsevier (2019). 33–43. doi: 10.1016/B978-0-12-813973-8.00005-1
128. Liu Y, Tikunov Y, Schouten RE, Marcelis LFM, Visser RGF, Bovy A. Anthocyanin biosynthesis and degradation mechanisms in solanaceous vegetables: a review. Front Chem (2018) 6:52. doi: 10.3389/fchem.2018.00052
129. Larsen DH, Li H, van de Peppel AC, Nicole CCS, Marcelis LFM, Woltering EJ. High light intensity at end-of-production improves the nutritional value of basil but does not affect postharvest chilling tolerance. Food Chem (2022) 369:130913. doi: 10.1016/j.foodchem.2021.130913
130. Min Q, Marcelis LFM, Nicole CCS, Woltering EJ. High light intensity applied shortly before harvest improves lettuce nutritional quality and extends the shelf life. Front Plant Sci (2021) 12:615355. doi: 10.3389/fpls.2021.615355
131. Maruo T, Johkan M. Tipburn. In: Kozai T, Niu G, Takagaki M, editors. Plant factory. London: Academic Press (2020). 231–4. doi: 10.1016/B978-0-12-816691-8.00015-7
132. Saure MC. Causes of the tipburn disorder in leaves of vegetables. Sci Hortic (1998) 76(3–4):131–47. doi: 10.1016/S0304-4238(98)00153-8
133. Adams P, Ho LC. Effects of environment on the uptake and distribution of calcium in tomato and on the incidence of blossom-end rot. Plant Soil (1993) 154(1):127–32. doi: 10.1007/BF00011081
134. Thibodeau PO, Minotti PL. The influence of calcium on the development of lettuce tipburn. J Am Soc Hortic Sci (1969) 94(4):372–6. doi: 10.21273/JASHS.94.4.372
135. Marcelis LFM, Ho LC. Blossom-end rot in relation to growth rate and calcium content in fruits of sweet pepper (Capsicum annuum L.). J Exp Bot (1999) 50(332):357–63. doi: 10.1093/jxb/50.332.357
136. Frantz JM, Ritchie G, Cometti NN, Robinson J, Bugbee B. Exploring the limits of crop productivity: beyond the limits of tipburn in lettuce. J Am Soc Hortic Sci (2004) 129(3):331–8. doi: 10.21273/JASHS.129.3.0331
137. Haibo Y, Lei Z, Haiye Y, Yucheng L, Chunhui L, Yuanyuan S. Sustainable development optimization of a plant factory for reducing tip burn disease. Sustainability (2023) 15(6):5607. doi: 10.3390/su15065607
138. Lee JG, Choi CS, Jang YA, Jang SW, Lee SG, Um YC. Effects of air temperature and air flow rate control on the tipburn occurrence of leaf lettuce in a closed-type plant factory system. Hortic Environ Biotechnol (2013) 54(4):303–10. doi: 10.1007/s13580-013-0031-0
139. Ghosh R, Barbacci A, Leblanc-Fournier N. Mechanostimulation: a promising alternative for sustainable agriculture practices. J Exp Bot (2021) 72(8):2877–88. doi: 10.1093/jxb/erab036
140. Collier GF, Tibbitts TW. Effects of relative humidity and root temperature on calcium concentration and tipburn development in lettuce. J Am Soc Hortic Sci (1984) 109(2):128–31. doi: 10.21273/JASHS.109.2.128
141. Cresswell GC. Effect of lowering nutrient solution concentration at night on leaf calcium levels and the incidence of tipburn in lettuce (var. gloria). J Plant Nutr (1991) 14(9):913–24. doi: 10.1080/01904169109364252
142. Vanhassel P, Bleyaert P, Van Lommel J, Vandevelde I, Crappé S, Van Hese N, et al. Rise of nightly air humidity as a measure for tipburn prevention in hydroponic cultivation of butterhead lettuce. In: De Pascale S, Jiang WJ, Connellan G, editors. XXIX International Horticultural Congress on Horticulture: Sustaining Lives, Livelihoods and Landscapes (IHC2014): International Symposium on Innovation and New Technologies in Protected Cropping. Brisbane: Acta Horticulturae (2015). 1107:195–202. doi: 10.17660/ActaHortic.2015.1107.26
143. Singh S. Root pressure: getting to the root of pressure. In: Lüttge U, Cánovas F, Matyssek R, editors. Progress in Botany 77. Cham: Springer (2016). 105–50. doi: 10.1007/978-3-319-25688-7_3
144. Beacham AM, Hand P, Teakle GR, Barker GC, Pink DAC, Monaghan JM. Tipburn resilience in lettuce (Lactuca spp.) – the importance of germplasm resources and production system-specific assays. J Sci Food Agric (2023) 103(9):4481–8. doi: 10.1002/jsfa.12523
145. Macias-González M, Truco MJ, Bertier LD, Jenni S, Simko I, Hayes RJ, et al. Genetic architecture of tipburn resistance in lettuce. Theor Appl Genet (2019) 132(8):2209–22. doi: 10.1007/s00122-019-03349-6
146. Gómez C, Jiménez J. Effect of End-Of-Production high-energy radiation on nutritional quality of indoor-grown red-leaf lettuce. Hortscience (2020) 55(7):1055–60. doi: 10.21273/HORTSCI15030-20
147. Arora AS, Yun CM. Dynamic spectrum lighting impact on plant morphology and cannabinoid profile of medical and recreational cannabis – a novel leapfrog strategy towards shaping the future of horticulture lighting. Ind Crops Prod (2023) 199. doi: 10.1016/j.indcrop.2023.116799
148. Cohu CM, Lombardi E, Adams WW, Demmig-Adams B. Increased nutritional quality of plants for long-duration spaceflight missions through choice of plant variety and manipulation of growth conditions. Acta Astronaut (2014) 94(2):799–806. doi: 10.1016/j.actaastro.2013.10.009
149. Kaya DA, Arslan M, Rusu L-C. Effects of harvesting hour on essential oil content and composition of Thymus vulgaris. Farmacia (2013) 61(6):1194–203. Available at: https://farmaciajournal.com/arhiva/201306/art-16-kaya%201194-1203.pdf
150. Mith H, Yayi-Ladékan E, Sika Kpoviessi SD, Yaou Bokossa I, Moudachirou M, Daube G, et al. Chemical composition and antimicrobial activity of essential oils of Ocimum basilicum, Ocimum canum and Ocimum gratissimum in function of harvesting time. J Essent Oil Bear Plants (2016) 19(6):1413–25. doi: 10.1080/0972060X.2014.890076
151. Rusanov K, Kovacheva N, Rusanova M, Atanassov I. Traditional Rosa damascena flower harvesting practices evaluated through GC/MS metabolite profiling of flower volatiles. Food Chem (2011) 129(4):1851–9. doi: 10.1016/j.foodchem.2011.05.132
152. Khapte PS, Kumar P, Burman U, Kumar P. Deficit irrigation in tomato: agronomical and physio-biochemical implications. Sci Hortic (2019) 248:256–64. doi: 10.1016/j.scienta.2019.01.006
153. Gaudreau L, Charbonneau J, Vezina LP, Gosselin A. Effects of photoperiod and photosynthet1c photon flux on nitrate content and nitrate reductase activity in greenhouse-grown lettuce. J Plant Nutr (1995) 18(3):437–53. doi: 10.1080/01904169509364914
154. Proietti S, Moscatello S, Colla G, Battistelli Y. The effect of growing spinach (Spinacia oleracea L.) at two light intensities on the amounts of oxalate, ascorbate and nitrate in their leaves. J Hortic Sci Biotechnol (2004) 79(4):606–9. doi: 10.1080/14620316.2004.11511814
155. Ward MH, Jones RR, Brender JD, de Kok TM, Weyer PJ, Nolan BT, et al. Drinking water nitrate and human health: an updated review. Int J Environ Res Public Health (2018) 15(7):1557. doi: 10.3390/ijerph15071557
156. Steingröver E, Siesling J, Ratering P. Effect of one night with “low light” on uptake, reduction and storage of nitrate in spinach. Physiol Plant (1986) 66(3):557–62. doi: 10.1111/j.1399-3054.1986.tb05966.x
157. Piergiovanni L, Limbo S. Food shelf-life models. In: Accorsi R, Manzini R, editors. Sustainable food supply chains. London: Academic Press (2019). 49–60. doi: 10.1016/B978-0-12-813411-5.00004-1
158. Tanner D. Impacts of storage on food quality. In: Smithers GW, editor. Reference module in food science. Amsterdam: Elsevier (2016). doi: 10.1016/B978-0-08-100596-5.03479-X
159. Barth M, Hankinson TR, Zhuang H, Breidt F. Microbiological spoilage of fruits and vegetables. In: Sperber W, Doyle M, editors. Compendium of the microbiological spoilage of foods and beverages. New York: Springer (2009). 135–83. doi: 10.1007/978-1-4419-0826-1_6
160. Fernandes PAR, Coimbra MA. The antioxidant activity of polysaccharides: a structure-function relationship overview. Carbohydr Polym (2023) 314:120965. doi: 10.1016/j.carbpol.2023.120965
161. Clarkson GJJ, Rothwell SD, Taylor G. End of day harvest extends shelf life. HortSci (2005) 40(5):1431–5. doi: 10.21273/HORTSCI.40.5.1431
162. Steeneken PG, Kaiser E, Verbiest GJ, ten Veldhuis MC. Sensors in agriculture: towards an internet of plants. Nat Rev Methods Primers (2023) 3(1):60. doi: 10.1038/s43586-023-00250-x
163. Resco de Dios V. Circadian regulation and diurnal variation in gas exchange. Plant Physiol (2017) 175(1):3–4. doi: 10.1104/pp.17.00984
164. Geldhof B, Pattyn J, Eyland D, Carpentier S, Van de Poel B. A digital sensor to measure real-time leaf movements and detect abiotic stress in plants. Plant Physiol (2021) 187(3):1131–48. doi: 10.1093/plphys/kiab407
165. Hartmann A, Czauderna T, Hoffmann R, Stein N, Schreiber F. HTPheno: an image analysis pipeline for high-throughput plant phenotyping. BMC Bioinformatics (2011) 12:148. doi: 10.1186/1471-2105-12-148
166. Sarić R, Nguyen VD, Burge T, Berkowitz O, Trtílek M, Whelan J, et al. Applications of hyperspectral imaging in plant phenotyping. Trends Plant Sci (2022) 27(3):301–15. doi: 10.1016/j.tplants.2021.12.003
167. Baker NR. Chlorophyll fluorescence: a probe of photosynthesis in vivo. Annu Rev Plant Biol (2008) 59:89–113. doi: 10.1146/annurev.arplant.59.032607.092759
168. Murchie EH, Lawson T. Chlorophyll fluorescence analysis: a guide to good practice and understanding some new applications. J Exp Bot (2013) 64(13):3983–98. doi: 10.1093/jxb/ert208
169. Vialet-Chabrand S, Lawson T. Dynamic leaf energy balance: deriving stomatal conductance from thermal imaging in a dynamic environment. J Exp Bot (2019) 70(10):2839–55. doi: 10.1093/jxb/erz068
170. Vialet-Chabrand S, Lawson T. Thermography methods to assess stomatal behaviour in a dynamic environment. J Exp Bot (2020) 71(7):2329–38. doi: 10.1093/jxb/erz573
171. Kläring HP, Körner O. Design of a real-time gas-exchange measurement system for crop stands in environmental scenarios. Agronomy (2020) 10(5):1–22. doi: 10.3390/agronomy10050737
172. Wheeler RM, Corey KA, Sager JC, Knott WM. Gas exchange characteristics of wheat stands grown in a closed, controlled environment. Crop Sci (1993) 33(1):161–8. doi: 10.2135/cropsci1993.0011183X003300010029x
173. Li L, Zhang Q, Huang D. A review of imaging techniques for plant phenotyping. Sensors (Basel) (2014) 14(11):20078–111. doi: 10.3390/s141120078
174. Epping R, Koch M. On-site detection of volatile organic compounds (VOCs). Molecules (2023) 28(4):1598. doi: 10.3390/molecules28041598
175. Wilson AD. Diverse applications of electronic-nose technologies in agriculture and forestry. Sensors (Basel) (2013) 13(2):2295–348. doi: 10.3390/s130202295
176. Dutta S, Chen Z, Kaiser E, Matamoros PM, Steeneken PG, Verbiest GJ. Ultrasound pulse emission spectroscopy method to characterize xylem conduits in plant stems. Research (Wash DC) (2022) 2022:9790438. doi: 10.34133/2022/9790438
177. Khait I, Lewin-Epstein O, Sharon R, Saban K, Goldstein R, Anikster Y, et al. Sounds emitted by plants under stress are airborne and informative. Cell (2023) 186(7):1328–1336.e10. doi: 10.1016/j.cell.2023.03.009
178. Vadez V, Kholová J, Hummel G, Zhokhavets U, Gupta SK, Hash CT. LeasyScan: a novel concept combining 3D imaging and lysimetry for high-throughput phenotyping of traits controlling plant water budget. J Exp Bot (2015) 66(18):5581–93. doi: 10.1093/jxb/erv251
179. Clearwater MJ, Luo Z, Mazzeo M, Dichio B. An external heat pulse method for measurement of sap flow through fruit pedicels, leaf petioles and other small-diameter stems. Plant Cell Environ (2009) 32(12):1652–63. doi: 10.1111/j.1365-3040.2009.02026.x
180. Helfter C, Shephard JD, Martinez-Vilalta J, Mencuccini M, Hand DP. A noninvasive optical system for the measurement of xylem and phloem sap flow in woody plants of small stem size. Tree Physiol (2007) 27(2):169–79. doi: 10.1093/treephys/27.2.169
181. De Swaef T, Hanssens J, Cornelis A, Steppe K. Non-destructive estimation of root pressure using sap flow, stem diameter measurements and mechanistic modelling. Ann Bot (2013) 111(2):271–82. doi: 10.1093/aob/mcs249
182. Tran D, Dutoit F, Najdenovska E, Wallbridge N, Plummer C, Mazza M, et al. Electrophysiological assessment of plant status outside a Faraday cage using supervised machine learning. Sci Rep (2019) 9(1):17073. doi: 10.1038/s41598-019-53675-4
183. Fahlgren N, Gehan MA, Baxter I. Lights, camera, action: high-throughput plant phenotyping is ready for a close-up. Curr Opin Plant Biol (2015) 24:93–9. doi: 10.1016/j.pbi.2015.02.006
184. Bugbee B, Monje O. The limits of crop productivity: validating theoretical estimates and determining the factors that limit crop yields in optimal environments. Bioscience (1992) 42(7):494–502. doi: 10.2307/1311879
185. Lee G, Wei Q, Zhu Y. Emerging wearable sensors for plant health monitoring. Adv Funct Materials (2021) 31(52):2106475. doi: 10.1002/adfm.202106475
186. Zhu XG, Song Q, Ort DR. Elements of a dynamic systems model of canopy photosynthesis. Curr Opin Plant Biol (2012) 15(3):237–44. doi: 10.1016/j.pbi.2012.01.010
187. Graefe J, Yu W, Körner O. A photosynthetic light acclimation model accounting for the effects of leaf age, chlorophyll content, and intra-leaf radiation transfer. Front Plant Sci (2022) 13:889709. doi: 10.3389/fpls.2022.889709
188. Folta KM. Breeding new varieties for controlled environments. Plant Biol (Stuttg) (2019) 21(Suppl 1):6–12. doi: 10.1111/plb.12914
189. Folta KM, Klee HJ. Sensory sacrifices when we mass-produce mass produce. Hortic Res (2016) 3:16032. doi: 10.1038/hortres.2016.32
190. Kwon CT, Heo J, Lemmon ZH, Capua Y, Hutton SF, Van Eck J, et al. Rapid customization of Solanaceae fruit crops for urban agriculture. Nat Biotechnol (2020) 38(2):182–8. doi: 10.1038/s41587-019-0361-2
191. Qu M, Zheng G, Hamdani S, Essemine J, Song Q, Wang H, et al. Leaf photosynthetic parameters related to biomass accumulation in a global rice diversity survey. Plant Physiol (2017) 175(1):248–58. doi: 10.1104/pp.17.00332
192. Xiao Y, Tholen D, Zhu XG. The influence of leaf anatomy on the internal light environment and photosynthetic electron transport rate: exploration with a new leaf ray tracing model. J Exp Bot (2016) 67(21):6021–35. doi: 10.1093/jxb/erw359
193. Kromdijk J, Głowacka K, Leonelli L, Gabilly ST, Iwai M, Niyogi KK, et al. Improving photosynthesis and crop productivity by accelerating recovery from photoprotection. Science (2016) 354(6314):857–61. doi: 10.1126/science.aai8878
194. Papanatsiou M, Petersen J, Henderson L, Wang Y, Christie JM, Blatt MR. Optogenetic manipulation of stomatal kinetics improves carbon assimilation, water use, and growth. Science (2019) 363(6434):1456–9. doi: 10.1126/science.aaw0046
195. Qu M, Hamdani S, Li W, Wang S, Tang J, Chen Z, et al. Rapid stomatal response to fluctuating light: an under-explored mechanism to improve drought tolerance in rice. Funct Plant Biol (2016) 43(8):727–38. doi: 10.1071/FP15348
196. Qu M, Essemine J, Xu J, Ablat G, Perveen S, Wang H, et al. Alterations in stomatal response to fluctuating light increase biomass and yield of rice under drought conditions. Plant J (2020) 104(5):1334–47. doi: 10.1111/tpj.15004
197. Carvalho SD, Folta KM. Environmentally modified organisms – expanding genetic potential with light. Crit Rev Plant Sci (2014) 33(6):486–508. doi: 10.1080/07352689.2014.929929
198. Fanwoua J, Vercambre G, Buck-Sorlin G, Dieleman JA, de Visser P, Génard M. Supplemental LED lighting affects the dynamics of tomato fruit growth and composition. Sci Hortic (2019) 256. doi: 10.1016/j.scienta.2019.108571
199. Kim HJ, Yang T, Choi S, Wang YJ, Lin MY, Liceaga AM. Supplemental intracanopy far-red radiation to red LED light improves fruit quality attributes of greenhouse tomatoes. Sci Hortic (2020) 261:108985. doi: 10.1016/j.scienta.2019.108985
200. Kozai T, Niu G. Role of the plant factory with artificial lighting (PFAL) in urban areas. In: Kozai T, Niu G, Takagaki M, editors. Plant factory: an indoor vertical farming system for efficient quality food production (2nd edition). London: Academic Press (2019). 7–34. doi: 10.1016/B978-0-12-816691-8.00002-9
201. Jacobson TA, Kler JS, Hernke MT, Braun RK, Meyer KC, Funk WE. Direct human health risks of increased atmospheric carbon dioxide. Nat Sustain (2019) 2(8):691–701. doi: 10.1038/s41893-019-0323-1
202. Benavides M, Cantón-Garbín M, Sánchez-Molina JA, Rodríguez F. Automatic tomato and peduncle location system based on computer vision for use in robotized harvesting. Appl Sci (2020) 10(17):5887. doi: 10.3390/app10175887
203. Mao L, Begum D, Chuang HW, Budiman MA, Szymkowiak EJ, Irish EE, et al. JOINTLESS is a MADS-box gene controlling tomato flower abscission zone development. Nature (2000) 406(6798):910–3. doi: 10.1038/35022611
204. Bac CW, Van Henten EJ, Hemming J, Edan Y. Harvesting robots for high-value crops: state-of-the-art review and challenges ahead. J Field Robot (2014) 31(6):888–911. doi: 10.1002/rob.21525
205. Song ZC, Miao H, Zhang S, Wang Y, Zhang SP, Gu XF. Genetic analysis and QTL mapping of fruit peduncle length in cucumber (Cucumis sativus L.). PloS One (2016) 11(12):e0167845. doi: 10.1371/journal.pone.0167845
206. Merilo E, Jõesaar I, Brosché M, Kollist H. To open or to close: species-specific stomatal responses to simultaneously applied opposing environmental factors. New Phytol (2014) 202(2):499–508. doi: 10.1111/nph.12667
207. Zhang Q, Ying Y, Ping J. Recent advances in plant nanoscience. Adv Sci (Weinh) (2022) 9(2):e2103414. doi: 10.1002/advs.202103414
208. Zhang N, Zhou X, Kang M, Hu BG, Heuvelink E, Marcelis LFM. Machine learning versus crop growth models: an ally, not a rival. AoB Plants (2023) 15(2):plac061. doi: 10.1093/aobpla/plac061
209. Jarvis PG. Scaling processes and problems. Plant Cell Environ (1995) 18(10):1079–89. doi: 10.1111/j.1365-3040.1995.tb00620.x
210. Blom T, Jenkins A, Pulselli RM, van den Dobbelsteen AAJF. The embodied carbon emissions of lettuce production in vertical farming, greenhouse horticulture, and open-field farming in the Netherlands. J Cleaner Prod (2022) 377:134443. doi: 10.1016/j.jclepro.2022.134443
211. Nicholson CF, Harbick K, Gómez MI, Mattson NS. An economic and environmental comparison of conventional and controlled environment agriculture (CEA) supply chains for leaf lettuce to US cities. In: Aktas E, Bourlakis M, editors. Food supply chains in cities: modern tools for circularity and sustainability. Cham: Palmgrave Macmillan (2020). 33–68. doi: 10.1007/978-3-030-34065-0_2
Keywords: controlled environment agriculture, electricity costs, flowering, urban farming, photosynthesis, plant breeding, product quality, sensors
Citation: Kaiser E, Kusuma P, Vialet-Chabrand S, Folta K, Liu Y, Poorter H, Woning N, Shrestha S, Ciarreta A, van Brenk J, Karpe M, Ji Y, David S, Zepeda C, Zhu X-G, Huntenburg K, Verdonk JC, Woltering E, Gauthier PPG, Courbier S, Taylor G and Marcelis LFM. Vertical farming goes dynamic: optimizing resource use efficiency, product quality, and energy costs. Front Sci (2024) 2:1411259. doi: 10.3389/fsci.2024.1411259
Received: 02 April 2024; Accepted: 03 July 2024;
Published: 24 September 2024.
Edited by:
Genhua Niu, Texas A&M University, United StatesReviewed by:
Youssef Rouphael, University of Naples Federico II, ItalyRaymond M. Wheeler, National Aeronautics and Space Administration (NASA), United States
Copyright © 2024 Kaiser, Kusuma, Vialet-Chabrand, Folta, Liu, Poorter, Woning, Shrestha, Ciarreta, van Brenk, Karpe, Ji, David, Zepeda, Zhu, Huntenburg, Verdonk, Woltering, Gauthier, Courbier, Taylor and Marcelis. This is an open-access article distributed under the terms of the Creative Commons Attribution License (CC BY). The use, distribution or reproduction in other forums is permitted, provided the original author(s) and the copyright owner(s) are credited and that the original publication in this journal is cited, in accordance with accepted academic practice. No use, distribution or reproduction is permitted which does not comply with these terms.
*Correspondence: Leo F. M. Marcelis, bGVvLm1hcmNlbGlzQHd1ci5ubA==