Explore article hub
- 1Department of Surgery, University of Pittsburgh, Pittsburgh, PA, United States
- 2Department of Immunology, Center for Systems Immunology, University of Pittsburgh, Pittsburgh, PA, United States
- 3Department of Mathematical Sciences, Indiana University - Purdue University Indianapolis, Indianapolis, IN, United States
- 4Laboratory of Synthetic, Perceptive, Emotive and Cognitive Systems (SPECS), Donders Centre of Neuroscience, Donders Centre for Brain, Cognition and Behaviour, Faculty of Science and Engineering, Radboud University, Nijmegen, Netherlands
- 5True Health Initiative, The Health Sciences Academy, London, United Kingdom
- 6Tangelo - Intend, Inc., Birmingham, MI, United States
Abstract
As populations worldwide show increasing levels of stress, understanding emerging links among stress, inflammation, cognition, and behavior is vital to human and planetary health. We hypothesize that inflammation is a multiscale driver connecting stressors that affect individuals to large-scale societal dysfunction and, ultimately, to planetary-scale environmental impacts. We propose a “central inflammation map” hypothesis to explain how the brain regulates inflammation and how inflammation impairs cognition, emotion, and action. According to our hypothesis, these interdependent inflammatory and neural processes, and the inter-individual transmission of environmental, infectious, and behavioral stressors—amplified via high-throughput digital global communications—can culminate in a multiscale, runaway, feed-forward process that could detrimentally affect human decision-making and behavior at scale, ultimately impairing the ability to address these same stressors. This perspective could provide non-intuitive explanations for behaviors and relationships among cells, organisms, and communities of organisms, potentially including population-level responses to stressors as diverse as global climate change, conflicts, and the COVID-19 pandemic. To illustrate our hypothesis and elucidate its mechanistic underpinnings, we present a mathematical model applicable to the individual and societal levels to test the links among stress, inflammation, control, and healing, including the implications of transmission, intervention (e.g., via lifestyle modification or medication), and resilience. Future research is needed to validate the model’s assumptions and conclusions against empirical benchmarks and to expand the factors/variables employed. Our model illustrates the need for multilayered, multiscale stress mitigation interventions, including lifestyle measures, precision therapeutics, and human ecosystem design. Our analysis shows the need for a coordinated, interdisciplinary, international research effort to understand the multiscale nature of stress. Doing so would inform the creation of interventions that improve individuals’ lives; enhance communities’ resilience to stress; and mitigate the adverse effects of stress on the world.
Key points
- Understanding the links among stress, inflammation, mental state, and behavior is vitally important to human and planetary health.
- According to our hypothesis, inflammation acts as a multiscale driver connecting stressors that affect individuals to large-scale societal dysfunction and ultimately to planetary-scale impacts on the environment, which in turn drive inflammatory stress via a positive feedback loop.
- We propose a “central inflammation map” hypothesis to explain how the brain regulates inflammation and how inflammation impairs perception, emotion, cognition, consciousness, and behavior.
- The interdependent inflammatory and neural processes, and the inter-individual transmission of environmental and infectious stressors—amplified via high-throughput digital global communications—culminate in a multiscale, runaway, feed-forward process that could detrimentally affect human decision-making and behavior at scale, ultimately impairing our ability to address these same stressors at both the individual and population levels.
- We propose a mathematical model that can be used to elucidate and test the links between stress, inflammation, neural control/cognition, and healing, with resultant implications on stress transmission, possible intervention (e.g., via lifestyle modification or medication), and resilience.
- A coordinated, interdisciplinary, international research effort is needed to define interventions that would improve the lives of individuals and the resilience of communities to stress—involving multilayered, multiscale stress mitigation interventions covering lifestyle measures, precision therapeutics, and human ecosystem design.
Introduction: living in a state of inflammation
Inflammation is a biological process that has evolved to allow organisms to sense and respond to both beneficial and excessive stress caused by internal or external stimuli (1–5). It is a highly conserved process regulated by complex immunological, neural, and hormonal mechanisms (6). Perturbations in these mechanisms underlie a variety of acute and chronic inflammatory diseases (e.g., infectious diseases, critical illness, cardiovascular disease, cancer, and autoimmune diseases) that collectively are a major global health burden (7).
Today, most people are living in what is arguably a relatively peaceful era compared with much of the 20th century and before. Nevertheless, there is a sense that much is amiss at the individual, community, national, and global levels. Recent reports suggest that the global population is under more stress than ever (8–11). This process is worsening, given common exposure to concerns about climate change and planetary health (12), social upheaval, economic uncertainty, food insecurity, war, and—to many people—the impression of seemingly endless waves of disease affecting the planet (13–16). The ever-increasing delineation of stressors has led to the term “exposome,” used to define the sum of harmful environmental exposures (17). These issues are amplified by disparities and marked variations in the social determinants of health (18, 19). These cumulative stressors have been referred to as a “polycrisis”, with initial attempts to begin to define this term and its societal manifestations (20).
The sense of dread stemming from the “polycrisis” further escalates stress and its somatic expression. Exposure to cumulative stressors is not a new phenomenon, of course. What is unprecedented, however, is the rate and density of the transfer of stress within and between populations globally via the Internet. For example, the rise in adolescent depression (a co-morbidity of stress) correlates closely with the rise in social media use (21). While the impact of social media recommendation algorithms is complex, and social media use can stave off stress by allowing users to express their feelings, these algorithms can also trigger radicalization pathways driven by negative narratives, heighten a sense of danger, and thus increase stress (22). These pathways are shaped algorithmically and emerge because of the exploitation of the causal coupling of stressors, emotions, and choice behavior in the service of economics (23).
We suggest that this increased sense of constant and ubiquitous danger is itself perhaps as insidious a stressor as the events being communicated through social media, and further suggest a direct link of inflammation to this pervasive sense of stress. Many studies suggest that all stress, whether “real” or virtual, induces an inflammatory response, impacting organs, neural pathways, experience, and behavior throughout life (24, 25). The converse is also true: neural mechanisms that evolved to regulate inflammation (26–28) may become overwhelmed when stress is constant and omnipresent, thereby impairing cognition (29, 30) and augmenting the perception and experience of stress. Indeed, as the mind is built around self-constructed world models, constant exposure to stressors will bias these internal models that shape our perception, experience, and behavior (29). This raises the question of whether the notion of inflammation should be expanded beyond the biological substrate and symptom networks. Notably, the network approach to psychopathology posits that mental disorders can be conceptualized and studied as causal systems of mutually reinforcing symptoms (31).
Here, we present the hypothesis that inflammation acts as an embedded, multiscale driver connecting most or all stressors affecting individuals to large-scale societal dysfunction and, ultimately, to planetary-scale impacts on the environment, which in turn drive inflammatory stress via a positive feedback loop. Our framework is based on the intertwined nature of the inflammation of organ systems, neural processes, cognition, experience, behavior, and inter-individual stress transmission, and the application of the principles of inflammatory stress in cells and individuals to populations of individuals acting in concert. As the central nervous system (CNS) regulates inflammation, and inflammatory mediators affect neurotransmission, we hypothesize that pro-inflammatory stress impairs human mental processes at multiple levels. We argue that these interdependent inflammatory and neural processes, and the inter-individual transmission of environmental, microbial, and informational stressors—amplified via high-throughput digital global communications (i.e., the Internet and social media)—culminate in a multiscale, runaway, feed-forward process that could detrimentally affect human decision-making and behavior, ultimately impairing our ability to address these very same individual and global stressors. At scale, this could have far-reaching societal and environmental consequences, potentially contributing to the chaotic and counter-intuitive responses of large swathes of the global population to stressors as diverse as global climate change, conflict, and the COVID-19 pandemic. As noted above, stress-impaired judgment may propagate a self-fulfilling sense of pervasive danger, causing further stress and establishing a runaway positive feedback loop of stress through behavioral feedback (32). Inflammation is thereby recast as a multi-scale process linking molecular interactions to global societal and planetary outcomes.
After detailing this hypothesis, we test its qualitative implications using a mathematical model linking the relevant processes and use insights from this exercise to inform multi-scale, multi-layered mitigation strategies and areas for further interdisciplinary research.
Stress and inflammation: from cell to globe
A working definition of stress
“Stress” generally refers to stimuli that evoke a defensive response—encapsulated famously for humans as “fight or flight.” While fight or flight most vividly pertains to the actions of an autonomous, individual animal, we suggest that this concept may be adapted to the defensive actions of cells, cell communities, organs, organ systems, individuals, and communities of individuals. As such, we hypothesize that when stress is sustained beyond certain individual resilience parameters, one enters a regime of learned helplessness (33), which has been connected with societally adverse behaviors such as the adherence to conspiracy theories (34).
Generally, an organism that interprets stress as an “action” occurring against it reacts in a manner analogous to fight, flight, or surrender. Given a suitable provocation (i.e., stressor), cells and unicellular organisms may express a range of defensive biochemical and biological responses, including the synthesis of various molecular compounds, structural alterations (such as encystment), and, at the extreme, apoptosis or other modes of programmed cell death (35). Below, we present a working definition of inflammation and detail the interrelationships between inflammation and stress.
A working definition of inflammation
In multicellular organisms, stress is transmitted and amplified by inflammatory cells and their products. Indeed, given an appropriate and sufficient stress signal, most cells of the body will mount a reaction that qualifies as inflammatory (36–38). White blood cells, for instance, are deployed en masse within the body to counter an immunogenic provocation. Whereas the chemicals generated by unicellular organisms are directed almost exclusively “outward” (compounds that alter gene expression, i.e., epigenetic actions, being an exception), those produced by multicellular organisms may exert effects directed both outward and inward. Examples of inward effects include those mediated by compounds that bind to receptors within the same body to amplify, reduce, or otherwise alter the choreography of cells or the cascade of other chemical mediators and any chemotactic effects that alter the targeted actions of dedicated immune cells (2). In higher organisms, cells initiate alarm/danger signals—also known as damage-associated molecular pattern (DAMP) molecules—that activate tissue-resident inflammatory cells to secrete inflammatory chemokines and cytokines (4, 39), which in turn stimulate the production of oxygen and nitrogen radicals and other cell toxins (35). This cascade leads to the creation of more DAMPs by the inflammatory cells as well as damage to additional parenchymal, i.e., organ-specific, cells, driving a feed-forward loop of damage → inflammation → damage at the cell/microbiota, tissue, organ, and, ultimately, whole-organism level (2, 40) (Figure 1). External stressors can also trigger epigenetic effects, i.e., producing stable changes in the genome (41). For example, depression-related, stress-associated epigenetic changes have been reported in various genes, including those involved in glucocorticoid signaling, serotonergic signaling, and neurotrophins (30).
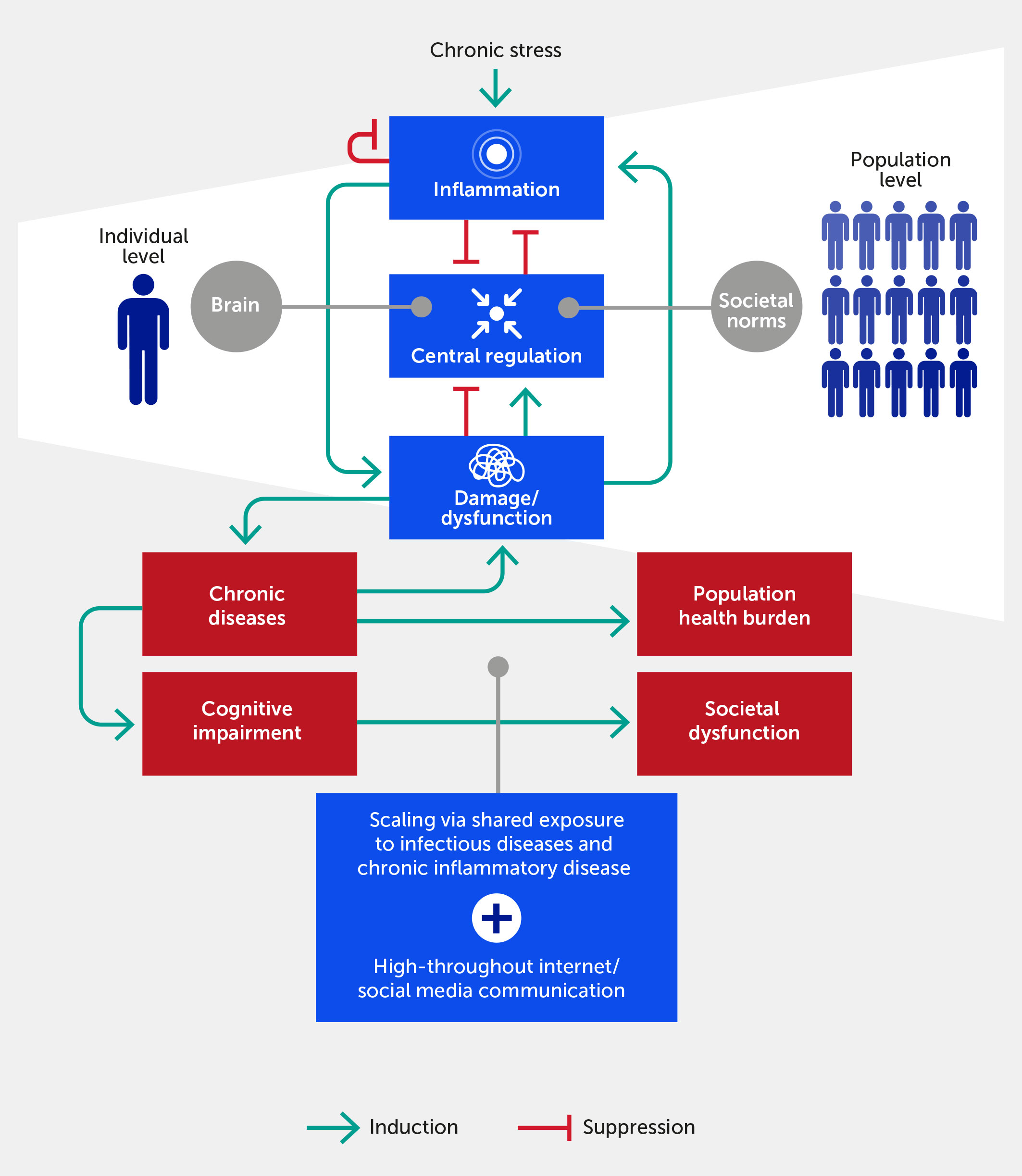
Figure 1 The inflammatory response in individuals feeds forward via its impact on physiologic damage/dysfunction. This damage impairs neurological and epigenetic function and the ability of the nervous system to regulate inflammation (i.e., distress), although we argue that within a certain range (i.e., eustress) stress can improve central control via enhanced resilience. Given ubiquitous exposure to infectious diseases, acute and chronic inflammatory disease factors, and the transmission of stress via the Internet/social media communication, this process scales to the population level. In this paradigm, chronic, stress-induced inflammation adversely impacts societal function if one views societal norms and processes as the analogue of biological allostatic/homeostatic mechanisms. We propose that the positive feedback loop between damage and inflammation can be generalized to the cognitive and population domains. Forward arrows indicate activation or promotion of a particular interaction, while blunted arrows indicate inhibition.
The embedded, multiscale, feed-forward character of inflammation is necessary to respond rapidly to threat or injury, possibly across generations, but is also a key driver of pathology across all scales of organization (42–44). This is coupled with pre-existing causes of inflammation in the form of acute and chronic diseases (7). The enhanced state of alarm in our society means that a large proportion of the human population is potentially predisposed to stress-induced inflammation at any given moment. Importantly, stress may be either constructive (i.e., eustress) or destructive (i.e., distress), and inflammation may be either well-regulated and enhance function or dysregulated and promote dysfunction. This realization, in turn, offers an initial basis to prioritize and manage stress responses and to differentiate between essential, constructive inflammatory responses versus dysfunctional inflammation. The aforementioned “inward versus outward” paradigm can be elaborated further inward, given that individuals can be thought of as complex “communities of the self” (45), especially when considering the microbiome (46) in addition to our diverse cells and tissues. Similarly, given the newly gained access to information broadcasting through social networks, humans have strongly amplified their ability for outward action.
Multiscale interactions between stress and inflammation propagate within and across individuals
We consider biological agents as comprising embedded networks, from genes and neurons to thoughts and behaviors (47). Our cells are subject to nearly constant stress, and the imperative to maintain homeostasis is thought to have led to the evolution of multiple mechanisms for sensing, responding, and adapting to both exogenous (e.g., due to microbial infection, injury, or noxious stimuli) and endogenous (metabolic) stressors throughout our lifetime; indeed, the induction of stress response mechanisms is an important feature of aging (43, 48–50). Moreover, when stressed, our commensal microbiota can become virulent and promote further pro-inflammatory stress (51). Homeostasis itself is regulated through allostatic processes (52) that adapt homeostatic control loops to internal needs and external opportunities, finding organismic stability through continuous homeostatic change. However, these regulatory loops can go awry when perturbed, such as in settings of sepsis (51, 53) or trauma/hemorrhage (54).
We propose that these same principles of stress and inflammation pertain to communities, or populations, of individuals acting in concert (Figure 1). In support of this idea, the constellation of stressors, including climate change and environmental pollution, has been linked to a significant reduction in growth, survival, and microbiome diversity of plant communities (55). In the human context, a key example of a community-wide acute stress response is any version of civil strife or warfare, while a chronic one is a reduction of birth rates and life expectancy. As we extend this paradigm to the population level, we note that a stress or provocation (e.g., war or pandemic) incites a coordinated response among the component parts (e.g., civilians, politicians, individual soldiers, or healthcare workers) of a superorganism engaging with the same, small suite of response options (i.e., fight, flight, or surrender). At the biological level, individuals communicate stress to other individuals in a process called “stress contagion” (56, 57)—e.g., via pheromones (58)—and thereby likely exacerbate their inflammatory responses. We hypothesize that this is a further cause of community-wide, and possibly global, stress communication, in addition to shared exposure to infectious stressors and highly prevalent chronic inflammatory diseases (Figure 1). Indeed, the hypothesis that psychopathology can be understood in terms of symptom networks provides a direct causal link between biological substrates and pathways of stress and psychological, social, and cultural pathways (47). We advance that these pathways have become perturbed pathologically not only through the aggregate effects of the modern exposome (12, 59) but also through the potential amplification of such effects via high-throughput information channels in the Internet age (60, 61).
We hypothesize that dysregulated inflammation ensues when multiscale stress response mechanisms are no longer sufficient to mitigate stress, and when the combination of internal and external stress exceeds our individual or collective stress resilience (Figure 1). At baseline, the inflammatory state is constrained via multiple points of control (36) because the inflammatory response has evolved to ramp up vigorously through positive feedback to deal rapidly with relatively non-specific threats (2). In contrast, the resolution of inflammation occurs more slowly (62), suggesting that inflammatory responses are regulated by a race between the feed-forward processes that propagate self-sustaining inflammation versus inflammation resolution processes with fewer positive-feedback loops (40).
These multiscale induction and resolution feedback loops manifest within individuals both spatially and temporally (63). While inflammation is at some level inherently local (i.e., occurring in a tissue or organ), the inflammatory response can propagate across multiple tissues and manifest systemically (2, 42, 53, 54, 63), for example, in the pathology of critical illness (63–65) and other diseases. Notably, negative feedback on inflammation can manifest locally, systemically (if the inflammatory response spills over into the systemic circulation), and centrally (via neural regulatory mechanisms; see below) (6, 66, 67) (Figure 1). We propose that this type of propagation—with the aforementioned causes and consequences—also applies to populations of humans responding to stress signals from other individuals and their natural and human-made environment.
We suggest that the rapid ramp-up of inflammation is the Achilles’ heel of this generally beneficial process, i.e., that the flow of inflammatory stress from the individual to the population and back is at the root of global-scale stress and its associated pathologies, including cognitive and thus behavioral dysfunction (Figure 1). In complex biological systems, robustness and functional flexibility are realized through a paradoxical fragility in the so-called “constraints that deconstrain” (68, 69). In our paradigm, the tissue-embedded and inherent feed-forward nature of the inflammatory response—coupled with close interactions with the control systems of the CNS—creates both robustness and fragility. Robustness occurs via the multilayered, multiscale, dynamic, and reciprocal control mechanisms that link stress, inflammation, and neural control and behavioral function. Simultaneously, fragility occurs via dysregulated inflammation rippling rapidly throughout multiple tissues in a multiscale fashion, leading to dysfunction in multiple organs as well as dysregulated emotion, cognition, experience, and behavior—which can be transmitted between individuals.
The brain is a key substrate in this stress, inflammation, and dysfunction cascade. On one hand, the hypothalamic–pituitary–adrenal (HPA) and sympathetic–adrenal–medullary (SAM) axes link stress to brain function, including executive control (70) and the immune system (71). Indeed, there are indications of a direct link between mood disorders and elevated levels of inflammatory markers in the blood (72). In addition, the brain will drive the actions that act as stressors on the outside world, potentially setting in motion collective stress cascades in the population.
Our paradigm extends beyond a purely bottom-up perspective on stress and inflammation and includes a top-down component. For instance, alarm in the form of interpersonal threats—whether direct (73) or over long distances via digital media (22, 61, 74)—along with anxiety over the state of society, climate change, infectious disease, socioeconomic status, etc. (8, 13–15, 75), can be considered just as pro-inflammatory as explicitly biological stimuli such as severe infections or chronic illness. These interpersonal stresses propagate among individuals within and between populations, rippling inward and outward to impact global processes in multiple ways (Figure 1). As in biological settings of inflammation, the repeated re-initiation of relatively low-level inflammatory responses can lower the activation threshold for positive feedback in multiple individuals within a community or society. This phenomenon, called “priming” in the cellular/molecular context of inflammation, is part of a broader preconditioning phenomenon that also includes the negative feedback process of tolerance/desensitization. At the population level, we suggest that priming leads to a state in which stress can explode across a society, even in the (apparent) absence of a single, defined pro-inflammatory stimulus, due to a diminished resolution response. The parallel process of tolerance/desensitization to ever-increasing personal and societal harm is driven by excess negative feedback (76, 77). Both processes comprise the phenomenon of preconditioning, can be modeled mathematically (78, 79), and are a key aspect of the global inflammatory paradigm advanced here.
According to our paradigm, the current intensity of stress and its multiscale transmission might be expected to translate into a comparable concentration of societal disorder and violence amplifying stress and inflammation. That this apparently does not occur (80) suggests a commensurate amplification of “controller” functions in modern societies, from the authority of science and medicine and trust in our institutions, to multinational organizations such as the United Nations and the North Atlantic Treaty Organization (NATO), to the interdependence fostered by global commerce (81). Yet all these control functions are currently being questioned and have arguably degraded. The challenge now is how humans can best prepare themselves for the emerging negative scenario: a new adversarial era of instability due to a combination of global stress and ecological collapse.
The brain and inflammatory stress
The “central inflammation map” hypothesis
We propose that the nervous system and cognition in individuals and the forms of symbolic exchange it affords—and the conceptually parallel role that societal rules play in communities of individuals—link stress, inflammation, and individual and societal dysfunction. As noted above, higher organisms have evolved a multiscale negative feedback architecture to control inflammation, in part involving neural mechanisms. A key neural mechanism that regulates inflammation both locally and systemically involves the vagus nerve (26), with neural circuits acting to limit the degree to which inflammatory mediators are expressed following a pro-inflammatory stimulus (27, 28). Recent evidence suggests that the vagus nerve not only regulates the degree to which inflammation is induced, but also limits the spread of inflammation across tissues and organs (82). We extend this paradigm by hypothesizing that, under physiologic conditions, neural control of inflammation occurs in part via a type of “hardware abstraction layer,” a term we borrow from computer science and that refers to the logical division of code in which computer hardware is controlled through software (83). Another way to think about this is in terms of the “constraints that deconstrain”: by having evolved specific protocols and substrates (i.e., constraints), biological systems afford a broad set of a priori unknown adaptations (i.e., de-constrained) in functional expression (84, 85). We hypothesize that the neural-immunological architecture builds on central neural regulation, invoking and involving the same inflammatory cytokines that drive inflammation in peripheral tissues. By this we mean that the brain can express the same inflammatory mediators expressed in inflamed distal organs to build a whole-body map of inflammation; we hypothesize that this “central inflammation map” is a mechanism for central regulation of the inflammatory response. As an example, this hypothesis is supported by research in rodent models showing that intrabronchial administration of a bacterial-derived immunostimulant (Gram-negative endotoxin) in a manner that does not result in the systemic manifestation of inflammatory mediators results in both lung and brain expression of the key inflammatory mediator interleukin (IL)-1β (86). Conversely, injection of IL-1β into the nucleus tractus solitarius in the brainstem is sufficient to replicate the functional derangements in lung physiology induced by endotoxins (87) and the toxin bleomycin (88). Another example is the putative causal link among stress, inflammation, and hippocampus and medial prefrontal cortex dysfunction, which can be seen as the result of multiple neurotoxic processes (89). One of these processes is HPA axis dysfunction, caused by chronic stress and enhanced production of cell-mediated immune cytokines, leading to serotonin depletion, increased glutamate and oxidative stress, and reduced inhibitory control mediated by gamma-amino butyric acid (GABA), in turn resulting in cellular damage and volume reductions (90, 91).
Thus, our hypothesis integrates inflammation into how we sense and appraise reality and shape it through our actions, closing a continuous behavioral feedback loop between the individual and their physical and social world (29). Further, we suggest that this paradigm offers a mechanism whereby stress and chronic inflammation could result in dysregulated cognition and action, which unchecked could, in turn, drive psychological dysfunction at the individual level and, ultimately, drive societal dysfunction. Initial support for this hypothesis comes from the finding that individuals with neuroimmune disorders often experience “brain fog” (92) that can impair their decision-making (93). Additional support comes from the known associations of cognitive derangements with immune dysregulation (29, 30) and the direct relation between stress and memory (94)—which, however, seems robust against “everyday” stress (95).
A related point is that the cytokines expressed in the brain putatively communicate inflammation in distal organs and positively regulate their expression. Continuing with the example of IL-1β, this cytokine can induce further expression of itself (96). We can speculate that repeated bouts of inflammation, coupled with the constant activation of neural sensing and regulatory pathways in response to real or perceived stress, might underlie the reported role of IL-1β in the pathobiology of neurodegenerative conditions such as Alzheimer’s disease (97) and other aspects of cognition (29, 30), and also lead to increased sensitization to stress and inflammation pathways. Once these effects affect action control, they can spill out into the outside world.
Below, we discuss additional factors that impact stress, inflammation, and neural function, how they link individuals to their environment, and how these factors might scale from the individual to the population and back.
Sleep disturbance exacerbates inflammatory stress
Sleep disturbance is one key factor that contributes to the ongoing cycle of inflammation and potential degradation of well-being and cognition. At the individual level, sleep is affected adversely by inflammation, which in turn impairs cognitive function both in the short and long term (98). Restorative sleep is highly dependent upon hormonal cycles, known generally as circadian rhythms. From a global perspective, these cycles represent an alignment of biorhythms with planetary cycles of light and darkness (99); notably, circadian rhythms have been implicated in allostasis and resilience to stress (100). While the pineal hormone, melatonin, is most prominently associated with variations in alertness and somnolence (101), its effects extend to influences on other hormones, including cortisol, catecholamines, growth hormone, leptin, and ghrelin (102, 103). Chronic inflammation is both a cause and effect of hormonal perturbations, notably persistent elevations in cortisol and catecholamines. In turn, these disturbances can foster insulin insensitivity and elevate insulin levels, thereby further impairing endocrine homeostasis (104, 105). Importantly, the disruption of circadian rhythms in the context of the lifestyle noted above is associated with further adverse impacts on metabolism (106). Finally, recent studies also connect cognitive dysfunction induced by sleep deprivation to disturbances of the gut microbiome (107), supporting the aforementioned impact of cumulative stress on communities of organisms (55) through the adverse effects of sleep dysregulation on cognition and action.
Over time, the hormonal repercussions of inflammation tend to disrupt appetite signaling and favor overconsumption of food and the growth (in both size and number) of adipocytes (108). Fatty infiltration of other cells (notably hepatocytes) also occurs, resulting in metabolic dysfunction and further inflammation (109, 110). These pathways sabotage circadian rhythmicity, impairing sleep via a hormonal mechanism (111). Excessive weight gain and adiposity may also impair sleep via a mechanical pathway, since these are linked to laxity in the posterior palate, snoring, and sleep apnea (112). Cumulatively, these factors increase stress on the organism.
The stressful effects of inflammation on sleep are reciprocal. Impaired sleep further disrupts circadian patterns, exacerbating hormonal disturbances. Increased chronic pain and psychological duress impair sleep and increase inflammatory responses, while sleep deprivation is associated with reductions in pain tolerance and psychological stress tolerance that potentiate the effects of both pain and stress. The result of these interactions is an adverse feedback loop, with inflammation disturbing sleep and sleep deprivation amplifying inflammation (104, 105, 113), setting in motion coupled multi-scale symptom pathways that amplify stress.
Resource competition drives inflammatory stress
Competition for survival essentials—water, food, shelter, and a mate—is a primordial and universal source of stress on individuals and populations. This can be further complemented with social survival essentials underlying flourishing such as bonding, affirmation, and recognition. The degree of stress varies with the intensity of such competition and the urgency of the unmet need. Competition for limited resources varies directly with population density, which translates into a fundamental trigger of stress-associated inflammation and impacts on cognition and behavior, as described above. Animal studies consistently demonstrate adverse effects of crowding on stress and inflammatory responses (114–116). Human studies of the impact of crowding, and related phenomena such as socioeconomically related violence (117–119) and work-related stress (120–122), suggest the same, though it is difficult to tease apart the impacts of chronic disease and other factors from that of work stress (123, 124). Both animal and human research have implicated various pathways, including disruptions of the HPA and microbiome (114, 125).
The potential for these environmental factors to trigger inflammatory responses within communities acquires greater importance in the context of population growth—the global human population has increased four-fold over the past century (126). As suggested earlier, the inflammatory responses of populations may be considered analogous to those of individuals: i.e., fight, flight, or surrender. In the case of survival essentials such as food and water, surrender is potentially suicidal. As global pressures on such resources increase, options for “flight” to safety become ever more limited. The inevitable result is more recourse to the “fight” response, whether between individuals within a population or between populations. The population density that intensifies competition and stress also serves to (a) deplete critical resources and engender shortages that aggravate the urgencies of competition and (b) situate competitors increasingly closer to one another in ever greater numbers. Importantly, contemporary competition-driven stress need not be caused by proximal stimuli alone: with the Internet and various forms of nearly instantaneous communication, the stress of competition for resources has become more intense and shifted to the social domain by the algorithmic exploitation of psychological needs. Competition is dissociated from spatial locations (i.e., it can now appear from any corner of the planet owing to globalization), compressed in time, and focused on new qualities, proxies, and currencies, such as social affirmation. This scaling and intensification of competition through digital means will, directly and indirectly, increase stress levels, further exacerbating inflammation. This is illustrated by the relationship between social media use and adolescent depression and suicide (21). Effectively, these digital means can now become a social and cultural substrate of inflammation, which continuously drives further stress.
We hypothesize that, in combination, these factors turn the human population into an incubator of stress and inflammation, accompanied by cognitive and behavioral dysfunction and, consequently, conflict. Conflict and competition further tax the stress tolerance of individuals, potentially making each less rational and more volatile. Disparities in general conditions—social and environmental—and in the social determinants of health amplify these stressors (18, 19) and further amplify conflict. Such volatility among individuals further inflames the body politic, triggering a self-perpetuating stress-enhancing positive feedback loop.
One final consideration is that the removal of functional opportunities to express native tendencies—for cells, individuals, or populations—might invite dysfunctional expressions of the same tendencies. For example, it has been argued that the eradication of various parasites from select populations has resulted in dramatic increases in autoimmune disease (127). Whether an analogous eradication of physical exertion from the modern experience of survival has contributed to hostile dysfunctions is a matter of conjecture. Cultural tensions related to modern policing methods do suggest that, as with cells, the cultivated actions of individuals and groups can be misdirected from function to dysfunction rather readily (128).
Modeling individual to global transmission of multiscale inflammatory stress
Model interactions
Our hypothesis is based on the intertwined nature of stress, inflammation, and neural dysfunction, and the propagation of these individual stressors both directly and indirectly within a population to ultimately impact societal function (Figure 1). As a first step, we have designed a high-level mathematical model that incorporates the following key variables: stress (S), inflammation (M), neural control (C), healing/restoration of function (H), and intervention (I). We note that, at present, the model is not calibrated to data and is simulated using arbitrary units (AU), given that massive, multiscale data acquisition and computational analysis across all these processes is needed to test these hypotheses and understand the complex dynamics underlying this stress-inflammation system. Figure 2 depicts the basic interactions among these variables, which are translated into the mathematical model (detailed in the Appendix). Notably, this model is relevant to two different interpretations of the interplay of stress and inflammation: an individual interpretation and a societal interpretation. Further, this framework can account for both eustress and distress.
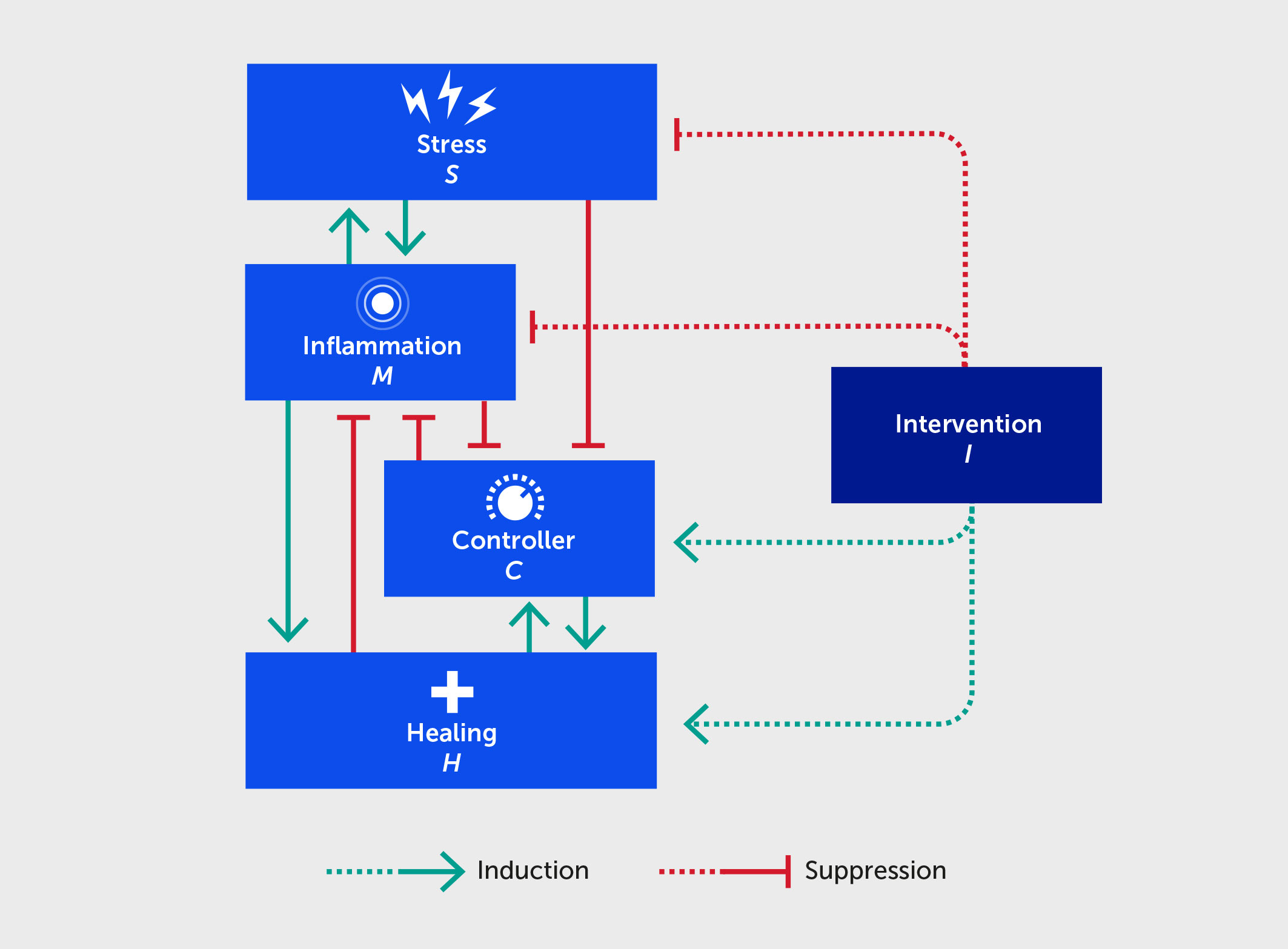
Figure 2 Schematic for a mathematical model describing the interactions between stress (S), inflammation (M), controller (C), healing (H), and an intervention (I) benefitting each of these (dashed lines). Forward green arrows indicate activation or promotion of a particular interaction, while blunted red arrows indicate inhibition.
The stress variable can comprise an unlimited number of internal and external stresses on the system. For example, internal stressors could include an unhealthy diet, physical inactivity (i.e., sedentary lifestyle), sleep deficiency, addictions, chronic illness, dysbiosis, and mental health conditions (e.g., depression, anxiety, and associated medications), or a lack of positive psychology/social connection (i.e., social isolation) (44). External stressors could include microbial infection, climate and natural environment factors, political upheaval, overcrowding, socioeconomic status, direct and digital social interactions, or other sources of digital stress (e.g., constant and easily accessible social media feeds and the news cycle). The model allows for any combination of such stress inputs and their weighting according to empirical data.
In the model, exposure to stressors triggers a varying degree of inflammatory response. At the individual level, an increase in the body’s inflammatory response causes more stress on the body, yielding a self-sustaining cycle of stress → inflammation → stress (i.e., leading to distress). Applying a societal interpretation, societal stressors trigger unrest that leads to a state of alarm and panic (i.e., “inflamed state” of a population) where societal rules are not obeyed. Further, we would argue that societal inflammation causes increased environmental stress because stressed, inflamed, and consequently cognitively impaired humans are more likely to make harmful decisions that contribute to environmental degradation, yielding a similar self-sustaining stress and inflammation cycle as generated on an individual level.
Since the CNS partially regulates the inflammatory response, the controller variable in the model is conceived as neural control at the individual level. At the societal level, the controller is a proxy of acquired societal norms and the organizations and other mechanisms that sustain these norms. The controller works to reduce and prevent an overwhelming inflammatory state abstracted at the whole-person level, just as acquired norms help reduce societal unrest (Figure 2). A highly inflamed state degrades the ability of the CNS to further regulate stress and inflammation, just as citizen unrest leads to actions that degrade adherence to societal norms, rules, and laws. Inflammation promotes healing in the model, since the primary purpose of inflammation is to heal the body (e.g., from infection) and to re-establish stability that is within allostatic bounds. The process of healing provides feedback to reduce inflammation. The healing variable in the model can be interpreted as a measure of deviation from homeostatic balance. On an individual level, the healing variable in the model is linked to the level of damage or DAMPs in the system; at a societal level, as stability reemerges, the healing variable could be linked to the relaxation or removal of laws and regulations put in place to address societal disorder.
Finally, the model can also account for the effects of outside interventions that inhibit stress and inflammation, promote healing, and restore control in a healthy and sustainable range. For example, these could include changes in diet, exercise, sleep, or medication at the individual level and societal trends, governmental subsidies, and rules at the societal level.
Model simulations: stress transmission, control, and intervention
When assessing the outcomes of all model simulations, comparing the steady state levels of stress, inflammation, controller, and healing levels with their respective baseline levels (dark blue) at time 0 will provide insight into whether stress added to the system causes detrimental outcomes (e.g., uncontrolled inflammation) in the system or if baseline is reestablished, regardless of all model variables being simulated in AUs. Once a dataset becomes available, inflammatory mediator levels and neurological function biomarkers could be used to calibrate the baseline initial conditions used in the system for a moderate level of input stress; those specific variables would be represented in appropriate units.
For this in silico exercise, the model is tested with six abstract stress factor inputs (which could correspond to any of the factors listed above) that are equally weighted concerning their impact on the overall system. Simulating the model shows how rising stress increases inflammation while impairing neural control of inflammation (Figure 3). When all stress input factors are set at their reference level (fi = 0.5), the system is considered to be in a baseline state with moderate stress and inflammation levels (see dark blue curves; units are arbitrary). Figure 3 simulates the upper and lower bounds of the six stress inputs: the red curves correspond to all high stress (all fi = 1) and the teal curves to low stress (all fi = 0). Here, the system is simulated in the absence of any intervention.
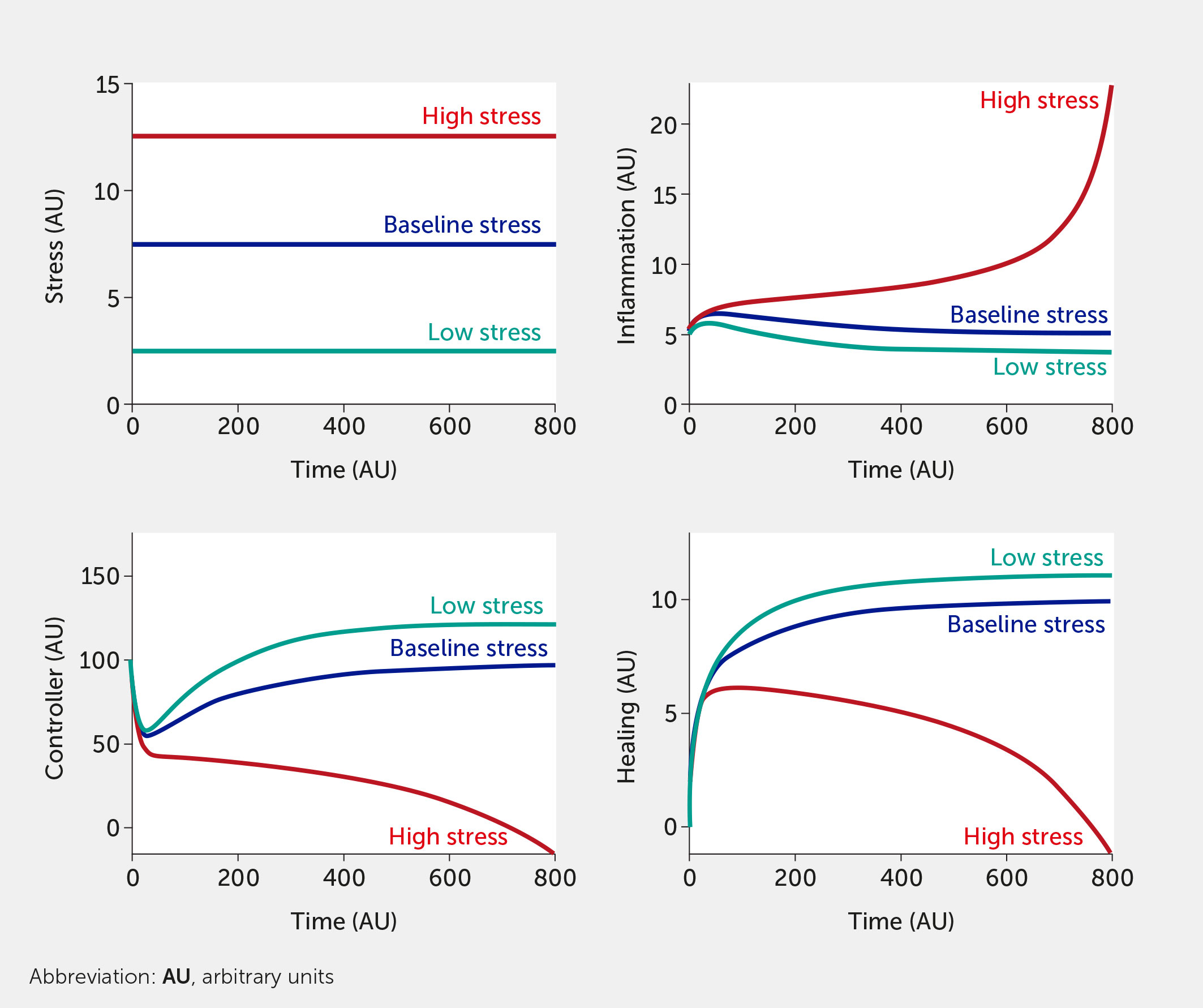
Figure 3 Model predictions for stress, inflammation, controller, and healing levels as a function of time given six equally weighted stress factor inputs of low (all fi = 0, teal), baseline (all fi = 0.5, dark blue), or high (all fi = 1, red) stress in the absence of intervention (i.e., I = 0 in all model equations).
Combinations of varying degrees of stress factors can also be simulated. For example, an input of f1 = 1, with all other factors f2 = f3 = f4 = f5 = f6 = 0.5, would show a substantial increase in stress and inflammation to the system (compared with baseline), illustrating how a single component of lifestyle stress could cause severe harm to the body. However, the model is currently predicated on the assumption that high stress in one aspect of a lifestyle or moderate stress in multiple areas will yield a similar inflammatory response. Such a model outcome could be used to show why diet and sleep habits that are perceived as relatively harmless could, in fact, be highly detrimental to an individual (or community) in a highly inflamed state, since these factors interact synergistically to induce high levels of stress.
Crucially, this modelling framework is scalable from the individual to the population. In Figure 4, the model is used to simulate the effect of stress transmitted by others. The vertical axis plots an individual’s stress level (here varied from 0–10); the horizontal axis shows an additional model input corresponding to the stress transmitted to an individual by others. Since this is a model stress input, it varies from 0 to 1: 0 corresponds to the absence of stress transmission (shown as the region to the left of the dotted lines) and 1 denotes the greatest level of stress that can be induced by a population. In Figure 4A, total stress in the system is indicated by the color map, where red indicates the highest stress levels. The color map in Figure 4B shows the level of controller function in the system; as expected, regions of high-stress lead to regions of controller dysfunction.
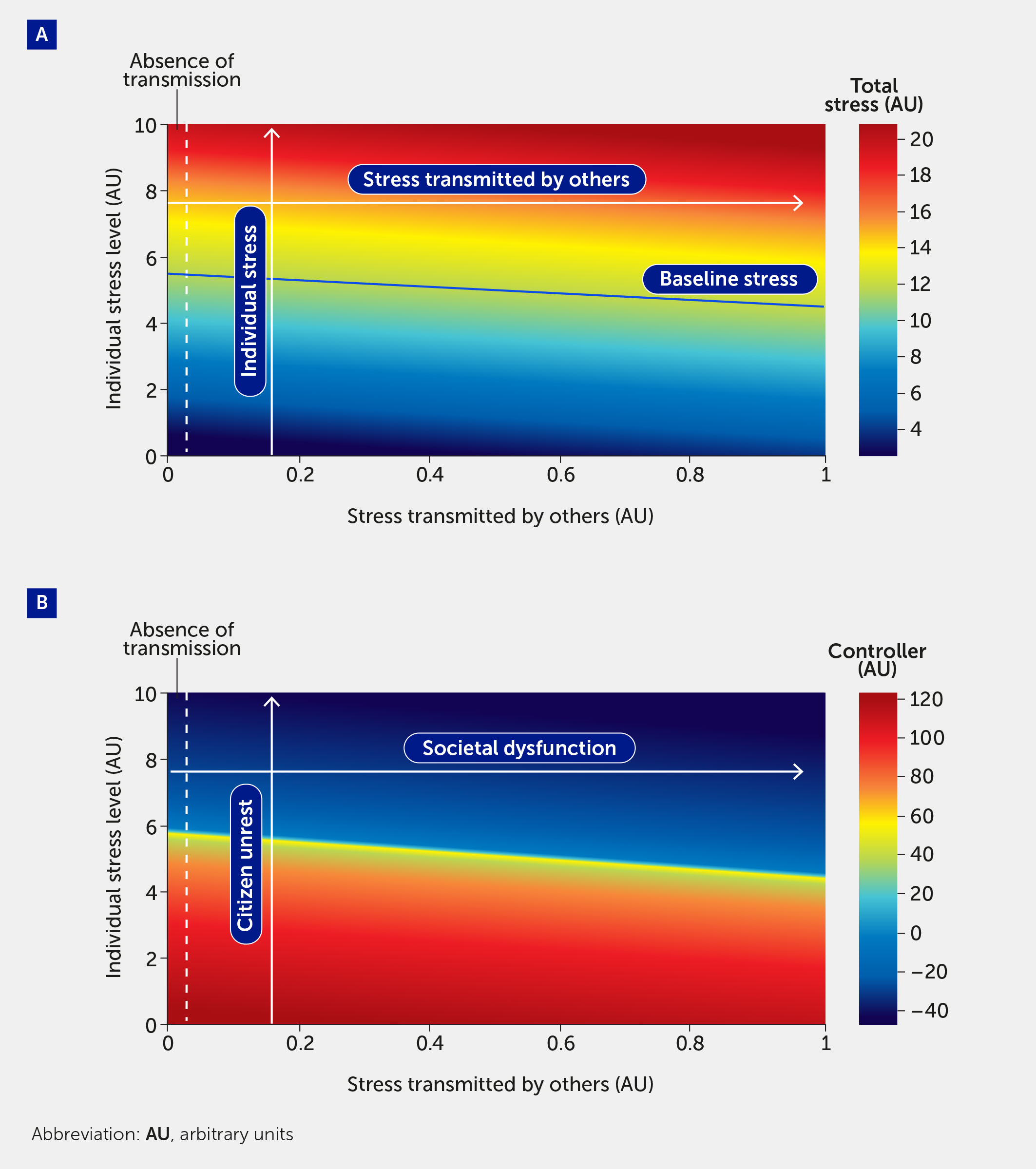
Figure 4 The impact of stress transmitted by others is quantified by total stress experienced by an individual (A) and controller function (B). As total stress (A) increases (red region), controller function (B) decreases (blue region). Regions to the left of the vertical dashed line indicate stress and controller levels felt by an individual in the absence of stress transmitted to that individual by others. A baseline level of stress (solid blue line), corresponding to all stress inputs fi = 0.5, is provided for comparison. The labels in panel B provide the societal interpretation of the impact of stress transmitted by others. Specifically, an increase in “individual stress level” corresponds to a higher level of citizen unrest, and a higher level of “stress transmitted by others” corresponds to increased societal dysfunction.
An emergent feature of this model is that the controller, which acts to stabilize the individual and population, can transition to a state in which it is not only incapable of dealing with stress, but where, in fact, it drives dysfunction. We interpret negative values for the controller function as indicating a scenario in which the controller is causing a deregulation of the system, which would be manifested in harmful decision-making. The boundary of the blue region in Figure 4B corresponds to the tipping point from reversible to irreversible societal dysfunction. From here, the model can be used to estimate the number of individuals necessary to cause a system to collapse.
The modeling framework can also assess the potential impact of interventions. Currently, the intervention (variable “I”) is modelled in a way that inhibits stress and inflammation and promotes the controller and healing function. This formalism was chosen to test the broad impact of interventions, but we envision modifying this so that a specific intervention only alters one function (e.g., only the controller versus only the inflammatory response). Two different individual-level interventions are introduced in Figure 5 and Figure 6. In Figure 5, a lifestyle intervention—e.g., exercising moderately/vigorously a few days per week (44, 129) or cognitive behavioral therapies (24, 44), known to reduce mortality in large epidemiological studies (130)—is included on the assumption that its efficacy is approximately 30%. Figure 6 simulates a medical drug intervention assumed to be 100% effective (a clear over-estimation used merely to test the model) with an onset of therapeutic benefit observed a short time after administration, which would be consistent with the time needed for an antidepressant drug to take full effect (131, 132); other relevant types of drugs (or devices) could also be modeled with differences in kinetics. In these simulations, the drug has a faster and greater effect than the single lifestyle intervention and shows a particularly greater benefit at high baseline stress levels. However, this gap is probably too narrow if one assumes much lower (and more realistic) drug efficacy.
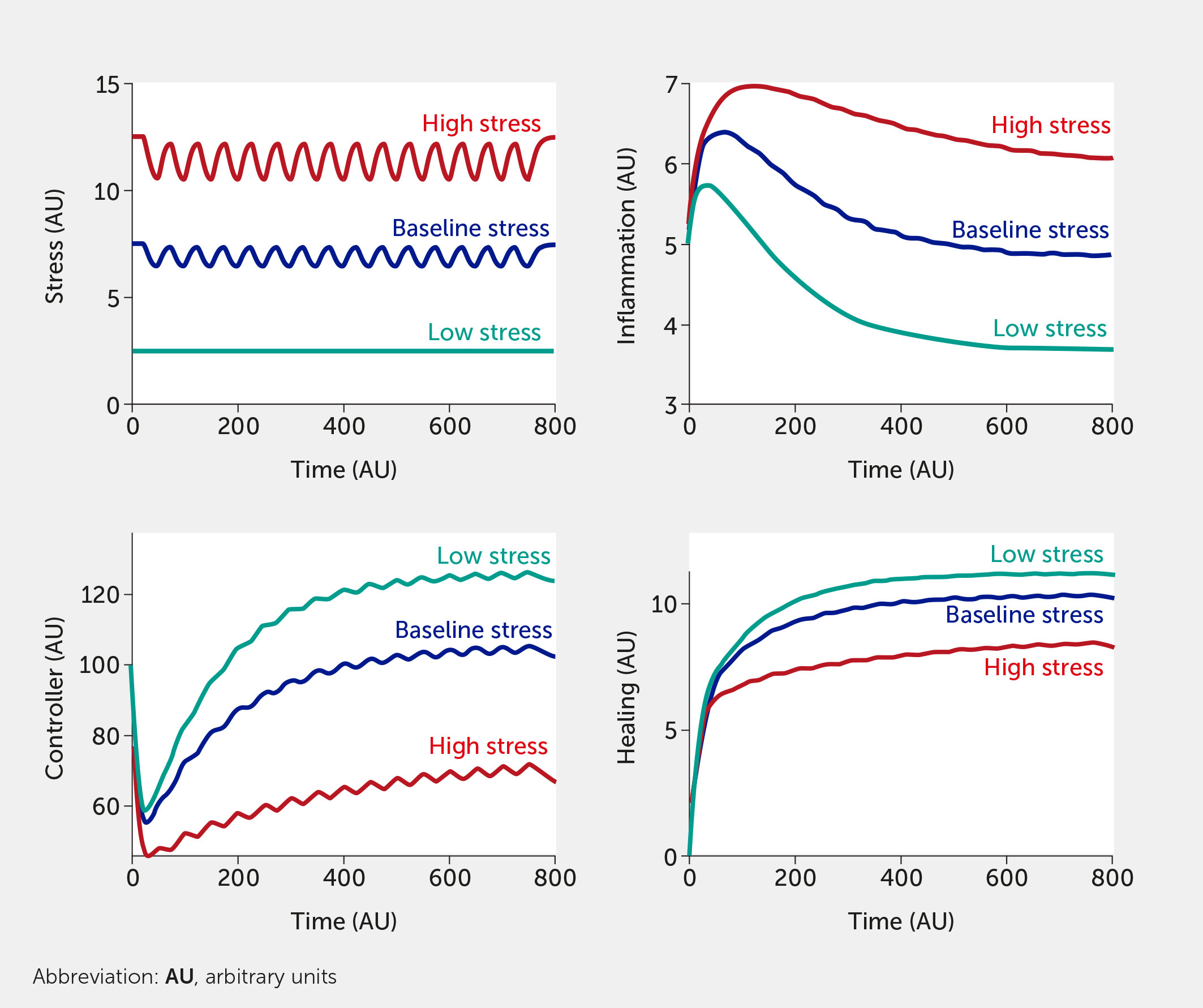
Figure 5 Model predictions for stress, inflammation, controller, and healing levels as a function of time given inputs of low (all fi = 0, teal), baseline (all fi = 0.5, dark blue), or high (all fi = 1, red) stress in the presence of a periodic intervention with 30% efficacy (e.g., exercise).
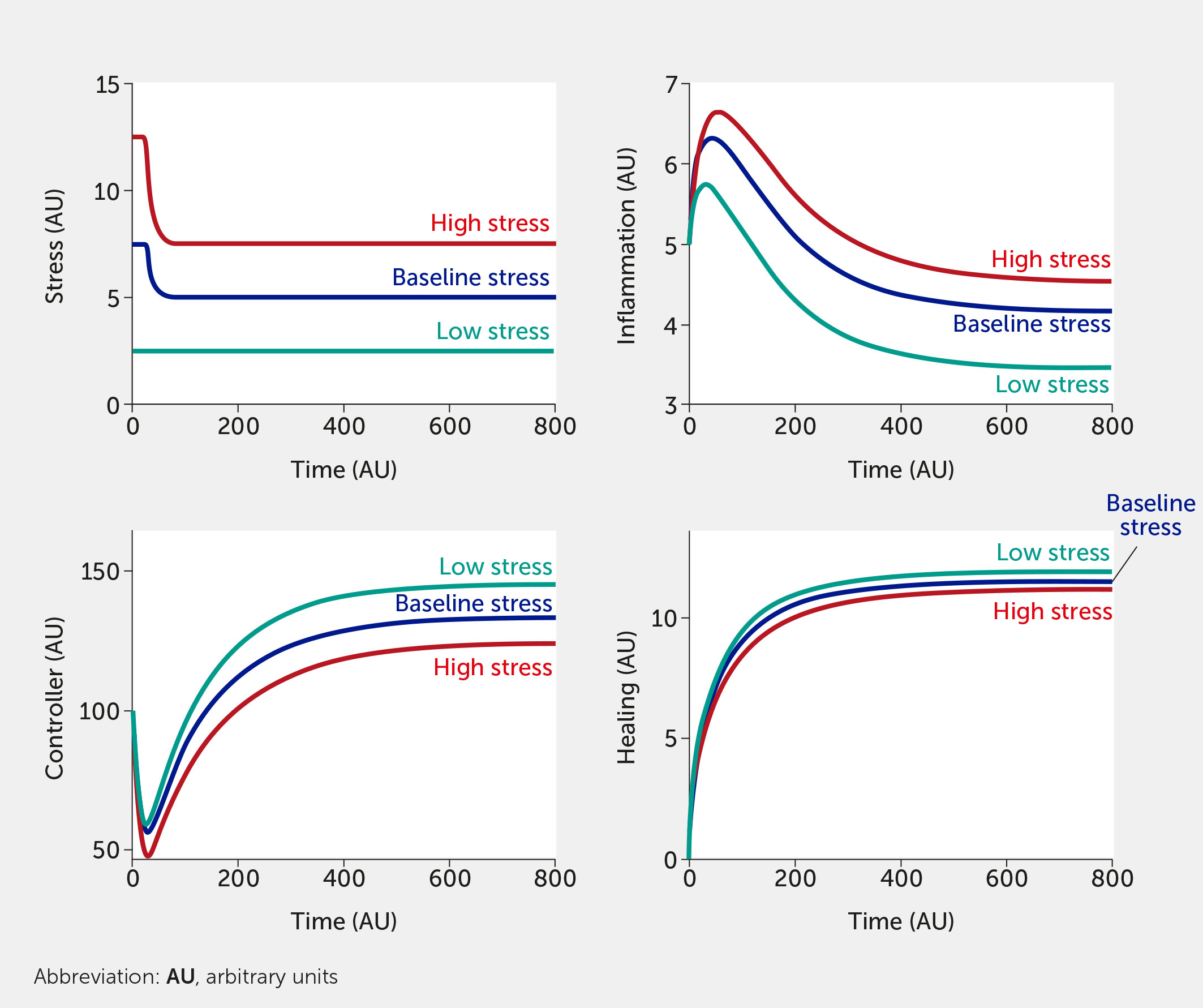
Figure 6 Model predictions for stress, inflammation, controller, and healing levels as a function of time given inputs of low (all fi = 0, teal), baseline (all fi = 0.5, dark blue), or high (all fi = 1, red) stress in the presence of a sustained intervention with 100% efficacy (e.g., medication) initiated at time = 25.
Inherent in our model is the concept of resilience. Figure 7 depicts the impact of increasing the resilience parameter, Scrit, slightly, in the absence of intervention. Model results are shown for the conditions of high stress, although the effect of increasing Scrit is evident at any level of stress. Increasing the resilience threshold from an arbitrary initial value of 6 to 7 reduced the system response from an extremely high level of inflammation to a much lower level (a little higher than baseline) while simultaneously increasing controller function and healing. This increased threshold corresponds to an individual or society that is sufficiently resilient to withstand a higher stress level.
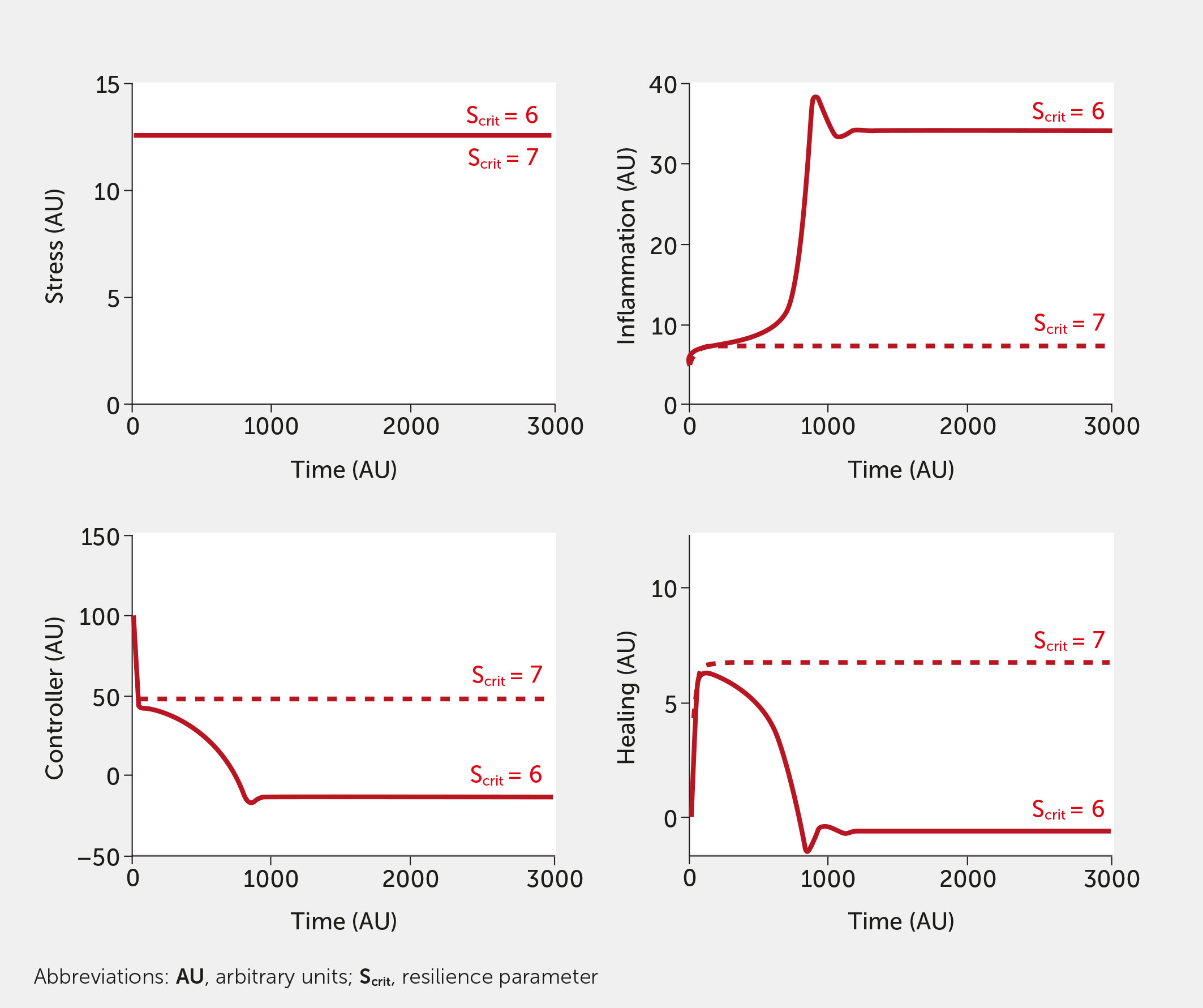
Figure 7 Impact of the resilience parameter (Scrit). Model predictions for stress, inflammation, controller, and healing levels as a function of time given inputs of high stress (all fi = 1, red) in the absence of intervention for a baseline (Scrit = 6, solid) and elevated (Scrit = 7, dashed) level of Scrit.
The model is intended to illustrate the complex relationship among various endogenous and exogenous factors involved in stress and inflammation and its propagation in individuals and collectives. It aims to show that using a modeling approach can assist us further in analyzing, monitoring, and predicting the impact of interventions on individuals and the population at large. Given the urgent need to better understand the multi-scale nature of stress and inflammation, because of its large-scale impact on society, we argue that such models must become part of the stress and inflammation control toolbox to inform rational collective decision-making.
Conclusion: a multilayered, multiscale approach to mitigating global inflammatory stress
We have argued for the global pathophysiologic and cognitive implications of pervasive, overwhelming stress while also suggesting how lower stress levels could improve resilience. Notably, the scientific community has begun to acknowledge the “polycrisis” nature of the intertwined stressors we have described here, but the proposed solutions center on traditional reductionist pharmacological interventions (133). In contrast, the model we propose, by its very nature, manifests and is regulated at multiple scales. Thus, the challenge lies in structuring—and customizing, for the individual and society—a commensurate set of multiscale interventions to mitigate the harmful effects of distress while acknowledging the value of eustress. To identify and validate such multiscale interventions, we seek to leverage the power of mathematical modelling: our example here—linking stress, inflammation, and cognition—may reveal potentially non-intuitive explanations for the behavior and relationships among cells, organisms, and superorganisms (i.e., communities of organisms), such as the interplay of multiple individual stressors that can lead to a virtually irrecoverable, pro-inflammatory, forward-feedback loop. We are aware that the field of mathematical modeling is mature and has evolved in many domains. Yet, so far, these multi-scale effects have been too complex to fully master and represent a challenge for the field. Clearly, much more work is needed to advance models of multiscale stress and inflammation, to validate them, and to obtain consensus on their underlying assumptions; to incorporate additional factors/variables that are currently only implicit—e.g., factors such as circadian rhythms, the multifaceted impacts of which have been modeled mathematically (134); to obtain data to verify and validate core qualitative assumptions of this model at both the individual and population levels; and to make the model more quantitative by obtaining prospective data in large-scale studies. Encouraging, early efforts in this regard have been published, wherein aggregated datasets have supported a picture of stress-related immune dysfunction in the context of socioeconomic disadvantage (135). Furthermore, other modeling formalisms (e.g., machine learning, once sufficient data are obtained) should be explored in tandem. This multi-pronged process would require a coordinated, interdisciplinary, international effort that engages key stakeholders, and we hope this article stimulates the broader research community to provide input in this regard.
The goal of this large-scale effort would be to define interventions that would improve the lives of individuals and the resilience of communities to stress. Key insights from the modeling of stress-induced inflammation and its impact on cognitive/executive function at the individual level—and the extrapolation of these insights to the society level—suggest the need for multiscale interventions to mitigate the detrimental effects of stress. This is an important observation because it implies that, to control stress and inflammation, unitary interventions that target a single level of this multiscale etiology will not suffice and may even aggravate the control problem. As an example, we can consider the seemingly paradoxical symptomatology of sepsis, which at first glance appears both unstructured and uncontrollable. Recent simulation studies (136–138) and synthetic biology-based in vivo studies (139) raise the possibility of rational, model-based control of this seemingly intractable syndrome. In this context, stress mitigation means attenuating positive feedback within and across layers. The initial mathematical model presented here can be used to describe stress conditions under which the controller will successfully re-establish homeostasis, compared with a collapse of the system under strikingly high levels of inflammation. Interventions can be evaluated to determine optimal dose timing and efficacy needed to restore inflammation to baseline levels and which pathways should be targeted to yield most improvement. We suggest that, at the individual level, anti-inflammatory drugs are not the answer, or at least not the whole answer, because they reduce inflammation indiscriminately and thus may mitigate the beneficial effects of eustress. Instead, we suggest (not surprisingly) that improving lifestyle (nutrition, sleep, exercise, etc.) would be of benefit (44), as of course would reducing the exposure to stress (140), which would correspond to a lowering of fi values in the model. There may also be a role for “nutraceutical” interventions such as probiotics to modulate the microbiome (141). Based on the correlative evidence cited above and our model simulations, we suggest that a key aspect of stress relief involves reducing the number, frequency, and duration of stressful interactions/events (e.g., reducing social media usage), and to create more space for the individual, both literally and figuratively. Modifications of the physical/built environment to provide more areas with a calm atmosphere are also likely to be of benefit (142). Our conceptual framework also raises the question of how to respond to digital stress and inflammation caused by the free market of “surveillance capitalism” (23). Here, one response could be to instill resilience through continuous education of citizens on the master narratives that anchor our societies and their norms and values (143).
Regarding therapeutics, we suggest the need to identify drugs that combat conditions such as depression via modulating the associated inflammatory responses (144) or drugs that antagonize pathways that sustain inflammation, e.g., drugs targeting type 17 immune responses (145, 146). Neuromodulation is another key area that should be explored, as this may improve controller function and reduce inflammation (147). Finally, as evidence suggests that pro-inflammatory stress might be imprinted epigenetically and potentially be heritable (148), the long-term, trans-generational impact of these mechanisms (and therapies) warrants further investigation.
Though halting and uneven from a global perspective, a paradigm shift is underway from reactive, reductive medicine to personalized, predictive, and proactive medicine based on extensive molecular profiling combined with bioinformatics and computational modeling (149, 150). This transition is especially important in the context of lifestyle medicine, where patients, physicians, and institutions could leverage emerging technologies to address chronic inflammation in a highly personalized fashion (44). An early and successful example of this transition is the application of individualized digital brain health approaches in stroke rehabilitation derived from advanced theoretical and modeling frameworks (151). As suggested previously (152) and demonstrated here, tools such as mechanistic mathematical modeling can be used to describe, reproduce, and predict complex interactions involving stress, resilience, inflammation, and lifestyle.
This work may also serve to drive more theoretical or philosophical discussions. At the risk of anthropomorphism, it may be argued that this paradigm can be extended further outward to a global level, wherein the collective actions of humans are the stress in this “Anthropocene era”, and the planet displays an inflammatory response in the form of global warming and associated climate change (153). Whether this is a manifestation of dysfunctional inflammation or effective self-defense remains to be determined and might be a matter of perspective, as the interests of planetary life in general and those of our species potentially diverge. However, our model proposes that the human population and its artifacts can also be seen as a substrate of inflammation and stress, potentially threatening stable co-existence with the planet at large. Clearly, this is a very speculative, philosophically oriented hypothesis. Taken together, we hope that the paradigm and model presented here drive further dialogue, discussion, and research into the potential mechanisms that link stress, inflammation, and cognition from the personal to the global level.
Supplementary material
The Supplementary Material for this article can be found online at: https://www.frontiersin.org/articles/10.3389/fsci.2023.1239462/full#supplementary-material
Statements
Author contributions
YV: Writing – original draft, Writing – review & editing, Conceptualization, Investigation, Methodology, Project administration, Supervision, Visualization. JA: Writing – original draft, Writing – review & editing, Conceptualization, Formal Analysis, Funding acquisition, Investigation, Methodology, Resources, Software, Visualization. PFMJV: Writing – original draft, Writing – review & editing, Conceptualization, Investigation. DLK: Writing – original draft, Writing – review & editing, Conceptualization, Investigation.
All of the authors contributed equally.
Data availability statement
The original contributions presented in the study are included in the article/supplementary material. Further inquiries can be directed to the corresponding author.
Funding
YV was supported by the following grants/contracts: U.S. Defense Advanced Research Projects Agency D20AC00002; U.S. Department of Defense W81XWH-18-2-0051, W81 XWH-15-1-0336, and W81XWH-15-PRORP-OCRCA; and NIH U01EB021960-01A1, RO1-GM107231, R01CA214865, UO1-DK072146, and P50-GM-53789. JA gratefully acknowledges NSF DMS-1654019, NSF DMS-1852146, and NIH R01EY030851. PFMJV is supported by the European Commission through AISN (HE, 101057655), EBRAINS-HEALTH (HE, 101058516), PHRASE (EIC, 101058240), NEST (AAL-2020-7-227-CP).
Conflict of interest
YV is a cofounder of, and stakeholder in, Immunetrics, Inc. and a consultant to Anuna AI. PFMJV is the founder of, and stakeholder in, Eodyne Systems s.l. and Sapiens5 Holding BV. DLK was employed by Tangelo - Intend, Inc. Neither these companies nor the funders mentioned above were involved in the study design, data collection, analysis, interpretation of data, the writing of this article, or the decision to submit it for publication. The companies mentioned above also did not provide funding for the study.
The remaining author declares that the research was conducted in the absence of any commercial or financial relationships that could be construed as a potential conflict of interest.
The authors YV and PV declared that they are editorial board members of Frontiers, at the time of submission. This had no impact on the peer review process and the final decision.
Publisher’s note
All claims expressed in this article are solely those of the authors and do not necessarily represent those of their affiliated organizations, or those of the publisher, the editors and the reviewers. Any product that may be evaluated in this article, or claim that may be made by its manufacturer, is not guaranteed or endorsed by the publisher.
References
1. Coussens LM, Werb Z. Inflammation and cancer. Nature (2002) 420(6917):860–7. doi: 10.1038/nature01322
2. Medzhitov R. Origin and physiological roles of inflammation. Nature (2008) 454(7203):428–35. doi: 10.1038/nature07201
3. Moseley P. Stress proteins and the immune response. Immunopharmacology (2000) 48(3):299–302. doi: 10.1016/s0162-3109(00)00227-7
4. Gallucci S, Matzinger P. Danger signals: SOS to the immune system. Curr Opin Immunol (2001) 13(1):114–9. doi: 10.1016/s0952-7915(00)00191-6
5. Nathan C, Ding A. Nonresolving inflammation. Cell (2010) 140(6):871–82. doi: 10.1016/j.cell.2010.02.029
6. Dick TE, Molkov Y, Nieman G, Hsieh Y, Jacono FJ, Doyle J, et al. Linking inflammation and cardiorespiratory variability in sepsis via computational modeling. Front Physiol (2012) 3:222. doi: 10.3389/fphys.2012.00222
7. GBD 2019 Diseases and Injuries Collaborators. Global burden of 369 diseases and injuries in 204 countries and territories, 1990–2019: a systematic analysis for the Global Burden of Disease Study 2019. Lancet (2020) 396(10258):1204–22. doi: 10.1016/S0140-6736(20)30925-9
8. American Psychological Association. Stress in America 2022: Concerned for the future, beset by inflation. Washington, DC: American Psychological Association (2022). Available at: https://www.apa.org/news/press/releases/stress/2022/concerned-future-inflation.
10. Daniali H, Martinussen M, Flaten MA. A global meta-analysis of depression, anxiety, and stress before and during COVID-19. Health Psychol (2023) 42(2):124–38. doi: 10.1037/hea0001259
11. Nochaiwong S, Ruengorn C, Thavorn K, Hutton B, Awiphan R, Phosuya C, et al. Global prevalence of mental health issues among the general population during the coronavirus disease-2019 pandemic: a systematic review and meta-analysis. Sci Rep (2021) 11(1):10173. doi: 10.1038/s41598-021-89700-8
12. Logan AC, Prescott SL, Haahtela T, Katz DL. The importance of the exposome and allostatic load in the planetary health paradigm. J Physiol Anthropol (2018) 37(1):15. doi: 10.1186/s40101-018-0176-8
13. Levy BS, Patz JA. Climate change, human rights, and social justice. Ann Glob Health (2015) 81(3):310–22. doi: 10.1016/j.aogh.2015.08.008
14. Koenen KC, Ratanatharathorn A, Ng L, McLaughlin KA, Bromet EJ, Stein DJ, et al. Posttraumatic stress disorder in the World Mental Health Surveys. Psychol Med (2017) 47(13):2260–74. doi: 10.1017/S0033291717000708
15. Hossain MM, Tasnim S, Sultana A, Faizah F, Mazumder H, Zou L, et al. Epidemiology of mental health problems in COVID-19: a review. F1000Res (2020) 9:636. doi: 10.12688/f1000research.24457.1
16. Santomauro DF, Mantilla Herrera AM, Shadid J, Zheng P, Ashbaugh C, Pigott DM, et al. Global prevalence and burden of depressive and anxiety disorders in 204 countries and territories in 2020 due to the COVID-19 pandemic. Lancet (2021) 398(10312):1700–12. doi: 10.1016/S0140-6736(21)02143-7
17. Vineis P, Robinson O, Chadeau-Hyam M, Dehghan A, Mudway I, Dagnino S. What is new in the exposome? Environ Int (2020) 143:105887. doi: 10.1016/j.envint.2020.105887
18. Evans RG, Barer ML, Marmor TR. Why Are Some People Healthy and Others Not?: the determinants of health of populations. Ebook ed. New York: De Gruyter (2021).
19. Castagné R, Delpierre C, Kelly-Irving M, Campanella G, Guida F, Krogh V, et al. A life course approach to explore the biological embedding of socioeconomic position and social mobility through circulating inflammatory markers. Sci Rep (2016) 6(1):25170. doi: 10.1038/srep25170
20. Hoyer D, Bennett JS, Reddish J, Holder S, Howard R, Benam M, et al. Navigating polycrisis: long-run socio-cultural factors shape response to changing climate. Philos Trans R Soc Lond B Biol Sci (2023) 378(1889):20220402. doi: 10.1098/rstb.2022.0402
21. Twenge JM. Why increases in adolescent depression may be linked to the technological environment. Curr Opin Psychol (2020) 32:89–94. doi: 10.1016/j.copsyc.2019.06.036
22. Wolfers LN, Utz S. Social media use, stress, and coping. Curr Opin Psychol (2022) 45:101305. doi: 10.1016/j.copsyc.2022.101305
23. Zuboff S. The age of surveillance capitalism: the fight for a human future at the new frontier of power. London: Profile books (2018).
24. Straub RH, Cutolo M. Psychoneuroimmunology-developments in stress research. Wien Med Wochenschr (2018) 168(3–4):76–84. doi: 10.1007/s10354-017-0574-2
25. Chiang JJ, Lam PH, Chen E, Miller GE. Psychological stress during childhood and adolescence and its association with inflammation across the lifespan: a critical review and meta-analysis. Psychol Bull (2022) 148(1–2):27–66. doi: 10.1037/bul0000351
27. Zanos TP, Silverman HA, Levy T, Tsaava T, Battinelli E, Lorraine PW, et al. Identification of cytokine-specific sensory neural signals by decoding murine vagus nerve activity. Proc Natl Acad Sci USA (2018) 115(21):E4843–52. doi: 10.1073/pnas.1719083115
28. Kressel AM, Tsaava T, Levine YA, Chang EH, Addorisio ME, Chang Q, et al. Identification of a brainstem locus that inhibits tumor necrosis factor. Proc Natl Acad Sci USA (2020) 117(47):29803–10. doi: 10.1073/pnas.2008213117
29. Bilbo SD, Schwarz JM. Early-life programming of later-life brain and behavior: a critical role for the immune system. Front Behav Neurosci (2009) 3:14.2009. doi: 10.3389/neuro.08.014.2009
30. Patterson SL. Immune dysregulation and cognitive vulnerability in the aging brain: interactions of microglia, IL-1β, BDNF and synaptic plasticity. Neuropharmacology (2015) 96(A):11–8. doi: 10.1016/j.neuropharm.2014.12.020
31. Borsboom D, Cramer AO. Network analysis: an integrative approach to the structure of psychopathology. Annu Rev Clin Psychol (2013) 9:91–121. doi: 10.1146/annurev-clinpsy-050212–185608
32. Verschure PFMJ, Voegtlin T, Douglas RJ. Environmentally mediated synergy between perception and behaviour in mobile robots. Nature (2003) 425(6958):620–4. doi: 10.1038/nature02024
33. Seligman ME. Learned helplessness. Annu Rev Med (1972) 23:407–12. doi: 10.1146/annuRevme.23.020172.002203
34. Farhart C, Miller J, Saunders K. Conspiracy stress or relief? Learned helplessness and conspiratorial thinking. In: Barker DC, Suhay E, editors. The Politics of Truth in Polarized America. Oxford: Oxford University Press (2021) 223–56. doi: 10.1093/oso/9780197578384.003.0011
35. Zhou DR, Eid R, Boucher E, Miller KA, Mandato CA, Greenwood MT. Stress is an agonist for the induction of programmed cell death: a review. Biochim Biophys Acta Mol Cell Res (2019) 1866(4):699–712. doi: 10.1016/j.bbamcr.2018.12.001
36. Nathan C. Points of control in inflammation. Nature (2002) 420(6917):846–52. doi: 10.1038/nature01320
37. Nathan C, Sporn M. Cytokines in context. J Cell Biol (1991) 113(5):981. doi: 10.1083/jcb.113.5.981
38. Medzhitov R, Janeway C Jr. Innate immunity. N Engl J Med (2000) 343(5):338–44. doi: 10.1056/NEJM200008033430506
39. Andersson U, Tracey KJ. HMGB1 is a therapeutic target for sterile inflammation and infection. Annu Rev Immunol. (2011) 29:139–62. doi: 10.1146/annurev-immunol-030409-101323
40. Vodovotz Y, Csete M, Bartels J, Chang S, An G. Translational systems biology of inflammation. PloS Comput Biol (2008) 4(4):e1000014. doi: 10.1371/journal.pcbi.1000014
41. Park C, Rosenblat JD, Brietzke E, Pan Z, Lee Y, Cao B, et al. Stress, epigenetics and depression: a systematic review. Neurosci Biobehav Rev (2019) 102:139–52. doi: 10.1016/j.neubioRev2019.04.010
42. Kotas ME, Medzhitov R. Homeostasis, inflammation, and disease susceptibility. Cell (2015) 160(5):816–27. doi: 10.1016/j.cell.2015.02.010
43. Furman D, Campisi J, Verdin E, Carrera-Bastos P, Targ S, Franceschi C, et al. Chronic inflammation in the etiology of disease across the life span. Nat Med (2019) 25(12):1822–32. doi: 10.1038/s41591-019-0675-0
44. Vodovotz Y, Barnard N, Hu FB, Jakicic J, Lianov L, Loveland D, et al. Prioritized research for the prevention, treatment, and reversal of chronic disease: recommendations from the Lifestyle Medicine Research Summit. Front Med (Lausanne) (2020) 7:585744(959). doi: 10.3389/fmed.2020.585744
46. Reynoso-García J, Miranda-Santiago AE, Meléndez-Vázquez NM, Acosta-Pagán K, Sánchez-Rosado M, Díaz-Rivera J, et al. A complete guide to human microbiomes: body niches, transmission, development, dysbiosis, and restoration. Front Syst Biol (2022) 2:951403. doi: 10.3389/fsysb.2022.951403
47. Wiers RW, Verschure P. Curing the broken brain model of addiction: neurorehabilitation from a systems perspective. Addict Behav (2021) 112:106602. doi: 10.1016/j.addbeh.2020.106602
48. Rea IM, Gibson DS, McGilligan V, McNerlan SE, Alexander HD, Ross OA. Age and age-related diseases: role of inflammation triggers and cytokines. Front Immunol (2018) 9:586. doi: 10.3389/fimmu.2018.00586
49. Franceschi C, Garagnani P, Parini P, Giuliani C, Santoro A. Inflammaging: a new immune-metabolic viewpoint for age-related diseases. Nat Rev Endocrinol (2018) 14(10):576–90. doi: 10.1038/s41574-018-0059-4
50. Haigis MC, Yankner BA. The aging stress response. Mol Cell (2010) 40(2):333–44. doi: 10.1016/j.molcel.2010.10.002
51. Alverdy J, Stern E. Effect of immunonutrition on virulence strategies in bacteria. Nutrition (1998) 14(7–8):580–4. doi: 10.1016/s0899-9007(98)00008-2
52. Schulkin J, Sterling P. Allostasis: a brain-centered, predictive mode of physiological regulation. Trends Neurosci (2019) 42(10):740–52. doi: 10.1016/j.tins.2019.07.010
53. Zamora R, Korff S, Mi Q, Barclay D, Yin J, Schimunek L, et al. A computational analysis of dynamic, multi-organ inflammatory crosstalk induced by endotoxin in mice. PloS Comput Biol (2018) 6:e100658. doi: 10.1371/journal.pcbi.1006582
54. Shah AM, Zamora R, Korff S, Barclay D, Yin J, El-Dehaibi F, et al. Inferring tissue-specific, TLR4-dependent type 17 immune interactions in experimental trauma/hemorrhagic shock and resuscitation using computational modeling. Front Immunol (2022) 13:908618. doi: 10.3389/fimmu.2022.908618
55. Zandalinas SI, Fritschi FB, Mittler R. Global warming, climate change, and environmental pollution: recipe for a multifactorial stress combination disaster. Trends Plant Sci (2021) 26(6):588–99. doi: 10.1016/j.tplants.2021.02.011
56. Bolger N, DeLongis A, Kessler RC, Wethington E. The contagion of stress across multiple roles. JMF (1989) 51(1):175–83. doi: 10.2307/352378
57. Liu CH, Doan SN. Psychosocial stress contagion in children and families during the COVID-19 pandemic. Clin Pediatr (Phila) (2020) 59(9–10):853–5. doi: 10.1177/0009922820927044
58. Calvi E, Quassolo U, Massaia M, Scandurra A, D’Aniello B, D’Amelio P. The scent of emotions: A systematic review of human intra- and interspecific chemical communication of emotions. Brain Behav (2020) 10(5):e01585. doi: 10.1002/brb3.1585
59. Vermeulen R, Schymanski EL, Barabási AL, Miller GW. The exposome and health: where chemistry meets biology. Science (2020) 367(6476):392–6. doi: 10.1126/science.aay3164
60. Afifi TD, Zamanzadeh N, Harrison K, Acevedo Callejas M. WIRED: the impact of media and technology use on stress (cortisol) and inflammation (interleukin IL-6) in fast paced families. Comput Hum Behav (2018) 81:265–73. doi: 10.1016/j.chb.2017.12.010
61. Lee DS, Way BM. Social media use and systemic inflammation: the moderating role of self-esteem. Brain Behav Immun Health (2021) 16:100300. doi: 10.1016/j.bbih.2021.100300
62. Willoughby DA, Moore AR, Colville-Nash PR, Gilroy D. Resolution of inflammation. Int J Immunopharmacol (2000) 22(12):1131–5. doi: 10.1016/s0192-0561(00)00064-3
63. Shah AM, Zamora R, Vodovotz Y. Interleukin-17 as a spatiotemporal bridge from acute to chronic inflammation: novel insights from computational modeling. Wires Mech Dis (2023) 15(3):e1599. doi: 10.1002/wsbm.1599
64. Cox CE. Persistent systemic inflammation in chronic critical illness. Respir Care (2012) 57(6):859–64; discussion 864. doi: 10.4187/respcare.01719
65. Gentile LF, Cuenca AG, Efron PA, Ang D, Bihorac A, McKinley BA, et al. Persistent inflammation and immunosuppression: a common syndrome and new horizon for surgical intensive care. J Trauma Acute Care Surg (2012) 72(6):1491–501. doi: 10.1097/TA.0b013e318256e000
66. An G, Nieman G, Vodovotz Y. Computational and systems biology in trauma and sepsis: current state and future perspectives. Int J Burns Trauma (2012) 2(1):1–10.
67. An G, Nieman G, Vodovotz Y. Toward computational identification of multiscale tipping points in multiple organ failure. Ann BioMed Eng (2012) 40:2412–24. doi: 10.1007/s10439-012-0565-9
68. Kitano H. Systems biology: a brief overview. Science (2002) 295(5560):1662–4. doi: 10.1126/science.1069492
69. Csete ME, Doyle JC. Reverse engineering of biological complexity. Science (2002) 295(5560):1664–9. doi: 10.1126/science.1069981
70. Shansky RM, Lipps J. Stress-induced cognitive dysfunction: hormone-neurotransmitter interactions in the prefrontal cortex. Front Hum Neurosci (2013) 7:123. doi: 10.3389/fnhum.2013.00123
71. Glaser R, Kiecolt-Glaser JK. Stress-induced immune dysfunction: implications for health. Nat Rev Immunol (2005) 5(3):243–51. doi: 10.1038/nri1571
72. Mechawar N, Savitz J. Neuropathology of mood disorders: do we see the stigmata of inflammation? Transl Psychiatry (2016) 6(11):e946. doi: 10.1038/tp.2016.212
73. Bush NR, Aschbacher K. Immune biomarkers of early-life adversity and exposure to stress and violence-Searching outside the streetlight. JAMA Pediatr (2020) 174(1):17–9. doi: 10.1001/jamapediatrics.2019.3882
74. Lee DS, Jiang T, Crocker J, Way BM. Social media use and its link to physical health indicators. Cyberpsychol Behav Soc Netw (2022) 25(2):87–93. doi: 10.1089/cyber.2021.0188
75. Kraft P, Kraft B. Explaining socioeconomic disparities in health behaviours: a review of biopsychological pathways involving stress and inflammation. Neurosci Biobehav Rev (2021) 127:689–708. doi: 10.1016/j.neubioRev2021.05.019
76. Day J, Rubin J, Vodovotz Y, Chow CC, Reynolds A, Clermont G. A reduced mathematical model of the acute inflammatory response II. Capturing scenarios of repeated endotoxin administration. J Theor Biol (2006) 242(1):237–56. doi: 10.1016/j.jtbi.2006.02.015
77. Rivière B, Epshteyn Y, Swigon D, Vodovotz Y. A simple mathematical model of signaling resulting from the binding of lipopolysaccharide with toll-like receptor 4 demonstrates inherent preconditioning behavior. Math Biosci (2009) 217(1):19–26. doi: 10.1016/j.mbs.2008.10.002
78. Kumar R, Clermont G, Vodovotz Y, Chow CC. The dynamics of acute inflammation. J Theor Biol (2004) 230(2):145–55. doi: 10.1016/j.jtbi.2004.04.044
79. Day JD, Park S, Ranard BL, Singh H, Chow CC, Vodovotz Y. Divergent COVID-19 disease trajectories predicted by a DAMP-centered immune network model. Front Immunol (2021) 12:754127. doi: 10.3389/fimmu.2021.754127
80. Pinker S. The better angels of our nature: why violence has declined. New York: Penguin Books (2012).
81. Friedman TL. The world is flat 3.0. A brief history of the twenty-first century (further updated and expanded). New York: Farrar, Straus and Giroux (2007).
82. Shah AM, Ruben Z, Barclay D, Yin J, El-Dehaibi F, Addorisio M, et al. Computational inference of chemokine-mediated roles for the vagus nerve in modulating intra- and inter-tissue inflammation. Front Syst Biol (2024) 4:1266279. doi: 10.3389/fsysb.2024.1266279
83. Popovici K, Jerraya A. Hardware abstraction layer. In: Ecker W, Müller W, Dömer R, editors. Hardware-dependent Software. Dordrecht: Springer Netherlands (2009). p. 67–94. doi: 10.1007/978-1-4020-9436-1_4
84. Kirschner MW, Gerhart JC, Norton J. The plausibility of life resolving Darwin’s Dilemma. New Haven, CT: Yale University Press (2005).
85. Doyle JC, Csete M. Architecture, constraints, and behavior. Proc Natl Acad Sci USA (2011) 108(Suppl 3):15624–30. doi: 10.1073/pnas.1103557108
86. Jacono FJ, Mayer CA, Hsieh YH, Wilson CG, Dick TE. Lung and brainstem cytokine levels are associated with breathing pattern changes in a rodent model of acute lung injury. Respir Physiol NeuroBiol (2011) 178(3):429–38. doi: 10.1016/j.resp.2011.04.022
87. Hsieh YH, Litvin DG, Zaylor AR, Nethery DE, Dick TE, Jacono FJ. Brainstem inflammation modulates the ventilatory pattern and its variability after acute lung injury in rodents. J Physiol (2020) 598(13):2791–811. doi: 10.1113/JP279177
88. Litvin DG, Denstaedt SJ, Borkowski LF, Nichols NL, Dick TE, Smith CB, et al. Peripheral-to-central immune communication at the area postrema glial-barrier following bleomycin-induced sterile lung injury in adult rats. Brain Behav Immun (2020) 87:610–33. doi: 10.1016/j.bbi.2020.02.006
89. Belleau EL, Treadway MT, Pizzagalli DA. The impact of stress and major depressive disorder on hippocampal and medial prefrontal cortex morphology. Biol Psychiatry (2019) 85(6):443–53. doi: 10.1016/j.biopsych.2018.09.031
90. Bandelow B, Baldwin D, Abelli M, Bolea-Alamanac B, Bourin M, Chamberlain SR, et al. Biological markers for anxiety disorders, OCD and PTSD: a consensus statement. Part II: neurochemistry, neurophysiology and neurocognition. World J Biol Psychiatry (2017) 18(3):162–214. doi: 10.1080/15622975.2016.1190867
91. Faye C, McGowan JC, Denny CA, David DJ. Neurobiological mechanisms of stress resilience and implications for the aged population. Curr Neuropharmacol (2018) 16(3):234–70. doi: 10.2174/1570159X15666170818095105
92. Theoharides TC, Stewart JM, Hatziagelaki E, Kolaitis G. Brain “fog,” inflammation and obesity: key aspects of neuropsychiatric disorders improved by luteolin. Front Neurosci (2015) 9:225. doi: 10.3389/fnins.2015.00225
93. Yelland GW. Gluten-induced cognitive impairment (“brain fog”) in coeliac disease. J Gastroenterol Hepatol (2017) 32(Suppl 1):90–3. doi: 10.1111/jgh.13706
94. Luethi M, Meier B, Sandi C. Stress effects on working memory, explicit memory, and implicit memory for neutral and emotional stimuli in healthy men. Front Behav Neurosci (2008) 2:5.2008. doi: 10.3389/neuro.08.005.2008
95. Lukasik KM, Waris O, Soveri A, Lehtonen M, Laine M. The relationship of anxiety and stress with working memory performance in a large non-depressed sample. Front Psychol (2019) 10:4. doi: 10.3389/fpsyg.2019.00004
96. Yazdi AS, Ghoreschi K. The interleukin-1 family. Adv Exp Med Biol (2016) 941:21–9. doi: 10.1007/978–94–024–0921–5_2
97. Heneka MT, Kummer MP, Stutz A, Delekate A, Schwartz S, Vieira-Saecker A, et al. NLRP3 is activated in Alzheimer’s disease and contributes to pathology in APP/PS1 mice. Nature (2013) 493(7434):674–8. doi: 10.1038/nature11729
98. Atrooz F, Salim S. Sleep deprivation, oxidative stress and inflammation. Adv Protein Chem Struct Biol (2020) 119:309–36. doi: 10.1016/bs.apcsb.2019.03.001
99. Rosato E, Kyriacou CP. Origins of circadian rhythmicity. J Biol Rhythms (2002) 17(6):506–11. doi: 10.1177/0748730402238232
100. Rao R, Androulakis IP. The physiological significance of the circadian dynamics of the HPA axis: interplay between circadian rhythms, allostasis and stress resilience. Horm Behav (2019) 110:77–89. doi: 10.1016/j.yhbeh.2019.02.018
101. Pevet P, Challet E, Felder-Schmittbuhl MP. Melatonin and the circadian system: keys for health with a focus on sleep. Handb Clin Neurol (2021) 179:331–43. doi: 10.1016/B978-0-12-819975-6.00021-2
102. Maitra S, Bhattacharya D, Das S, Bhattacharya S. Melatonin and its anti-glioma functions: a comprehensive review. Rev Neurosci (2019) 30(5):527–41. doi: 10.1515/revneuro-2018–0041
103. Tordjman S, Chokron S, Delorme R, Charrier A, Bellissant E, Jaafari N, et al. Melatonin: pharmacology, functions and therapeutic benefits. Curr Neuropharmacol (2017) 15(3):434–43. doi: 10.2174/1570159X14666161228122115
104. Kim TW, Jeong JH, Hong SC. The impact of sleep and circadian disturbance on hormones and metabolism. Int J Endocrinol (2015) 2015:591729. doi: 10.1155/2015/591729
105. Ditmer M, Gabryelska A, Turkiewicz S, Białasiewicz P, Małecka-Wojciesko E, Sochal M. Sleep problems in chronic inflammatory diseases: prevalence, treatment, and new perspectives: a narrative review. J Clin Med (2021) 11(1). doi: 10.3390/jcm11010067
106. Bae SA, Fang MZ, Rustgi V, Zarbl H, Androulakis IP. At the interface of lifestyle, behavior, and circadian rhythms: metabolic implications. Front Nutr (2019) 6:132. doi: 10.3389/fnut.2019.00132
107. Wang Z, Chen WH, Li SX, He ZM, Zhu WL, Ji YB, et al. Gut microbiota modulates the inflammatory response and cognitive impairment induced by sleep deprivation. Mol Psychiatry (2021) 26(11):6277–92. doi: 10.1038/s41380-021-01113-1
108. Gregor MF, Hotamisligil GS. Inflammatory mechanisms in obesity. Annu Rev Immunol (2011) 29:415–45. doi: 10.1146/annurev-immunol-031210-101322
109. Luci C, Bourinet M, Leclère PS, Anty R, Gual P. Chronic inflammation in non-alcoholic steatohepatitis: molecular mechanisms and therapeutic strategies. Front Endocrinol (Lausanne) (2020) 11:597648. doi: 10.3389/fendo.2020.597648
110. Lakhani HV, Sharma D, Dodrill MW, Nawab A, Sharma N, Cottrill CL, et al. Phenotypic alteration of hepatocytes in non-alcoholic fatty liver disease. Int J Med Sci (2018) 15(14):1591–9. doi: 10.7150/ijms.27953
111. Wang XL, Li L. Circadian clock regulates inflammation and the development of neurodegeneration. Front Cell Infect MicroBiol (2021) 11:696554. doi: 10.3389/fcimb.2021.696554
112. Dempsey JA, Veasey SC, Morgan BJ, O’Donnell CP. Pathophysiology of sleep apnea. Physiol. Rev (2010) 90(1):47–112. doi: 10.1152/physRev00043.2008
113. Garbarino S, Lanteri P, Bragazzi NL, Magnavita N, Scoditti E. Role of sleep deprivation in immune-related disease risk and outcomes. Commun Biol (2021) 4(1):1304. doi: 10.1038/s42003-021-02825-4
114. Delaroque C, Chervy M, Gewirtz AT, Chassaing B. Social overcrowding impacts gut microbiota, promoting stress, inflammation, and dysglycemia. Gut Microbes (2021) 13(1):2000275. doi: 10.1080/19490976.2021.2000275
115. Yin Z, Lam TJ, Sin YM. The effects of crowding stress on the non-specific immuneresponse in fancy carp (Cyprinus carpio L.). Fish Shellfish Immunol (1995) 5(7):519–29. doi: 10.1016/S1050-4648(95)80052-2
116. Lin W, Li L, Chen J, Li D, Hou J, Guo H, et al. Long-term crowding stress causes compromised nonspecific immunity and increases apoptosis of spleen in grass carp (Ctenopharyngodon idella). Fish Shellfish Immunol (2018) 80:540–5. doi: 10.1016/j.fsi.2018.06.050
117. Finegood ED, Chen E, Kish J, Vause K, Leigh AKK, Hoffer L, et al. Community violence and cellular and cytokine indicators of inflammation in adolescents. Psychoneuroendocrinology (2020) 115:104628. doi: 10.1016/j.psyneuen.2020.104628
118. Lee MJ, Rittschof CC, Greenlee AJ, Turi KN, Rodriguez-Zas SL, Robinson GE, et al. Transcriptomic analyses of black women in neighborhoods with high levels of violence. Psychoneuroendocrinology (2021) 127:105174. doi: 10.1016/j.psyneuen.2021.105174
119. Miller GE, Chen E, Finegood E, Shimbo D, Cole SW. Prospective associations between neighborhood violence and monocyte pro-inflammatory transcriptional activity in children. Brain Behav Immun (2022) 100:1–7. doi: 10.1016/j.bbi.2021.11.003
120. Booth A, Cowell J. Crowding and health. J Health Soc Behav (1976) 17(3):204–20. doi: 10.2307/2136543
121. Bellingrath S, Weigl T, Kudielka BM. Chronic work stress and exhaustion is associated with higher allostastic load in female school teachers. Stress (2009) 12(1):37–48. doi: 10.1080/10253890802042041
122. Lee W, Kang SK, Choi WJ. Effect of long work hours and shift work on high-sensitivity C-reactive protein levels among Korean workers. Scand J Work Environ Health (2021) 47(3):200–7. doi: 10.5271/sjweh.3933
123. Emeny RT, Zierer A, Lacruz ME, Baumert J, Herder C, Gornitzka G, et al. Job strain-associated inflammatory burden and long-term risk of coronary events: findings from the Monica/KORA Augsburg case-cohort study. Psychosom Med (2013) 75(3):317–25. doi: 10.1097/PSY.0b013e3182860d63
124. Kaltenegger HC, Becker L, Rohleder N, Nowak D, Weigl M. Associations of working conditions and chronic low-grade inflammation among employees: a systematic review and meta-analysis. Scand J Work Environ Health (2021) 47(8):565–81. doi: 10.5271/sjweh.3982
125. Liu J, Huang S, Zhang X, Li G, Batsuren E, Lu W, et al. Gut microbiota reflect the crowding stress of space shortage, physical and non-physical contact in Brandt’s voles (Lasiopodomys brandtii). MicroBiol Res (2021) 255:126928. doi: 10.1016/j.micres.2021.126928
126. Roser M, Ritchie H, Ortiz-Ospina E, Rodés-Guirao L. World population growth. Our World in Data. (2013). Accessible at: https://ourworldindata.org/.
127. Velasquez-Manoff M. An epidemic of absence: a new way of understanding allergies and autoimmune diseases. New York: Scribner (2013).
128. DeVylder J, Fedina L, Link B. Impact of police violence on mental health: a theoretical framework. Am J Public Health (2020) 110(11):1704–10. doi: 10.2105/AJPH.2020.305874
129. Metsios GS, Moe RH, Kitas GD. Exercise and inflammation. Best Pract. Res. Clin Rheumatol (2020) 34(2):101504. doi: 10.1016/j.berh.2020.101504
130. Gebel K, Ding D, Chey T, Stamatakis E, Brown WJ, Bauman AE. Effect of moderate to vigorous physical activity on all-cause mortality in middle-aged and older Australians. JAMA Intern Med (2015) 175(6):970–7. doi: 10.1001/jamainternmed.2015.0541
131. Bighelli I, Castellazzi M, Cipriani A, Girlanda F, Guaiana G, Koesters M, et al. Antidepressants versus placebo for panic disorder in adults. Cochrane Database Syst Rev (2018) 4(4):CD010676. doi: 10.1002/14651858.CD010676.pub2
132. Taylor MJ, Freemantle N, Geddes JR, Bhagwagar Z. Early onset of selective serotonin reuptake inhibitor antidepressant action: systematic review and meta-analysis. Arch Gen Psychiatry (2006) 63(11):1217–23. doi: 10.1001/archpsyc.63.11.1217
133. Gomollón-Bel F, García-Martínez J. Chemical solutions to the current polycrisis. Angew Chem Int Ed Engl (2023) 62(25):e202218975. doi: 10.1002/anie.202218975
134. Rao R, Androulakis IP. Allostatic adaptation and personalized physiological trade-offs in the circadian regulation of the HPA axis: a mathematical modeling approach. Sci Rep (2019) 9(1):11212. doi: 10.1038/s41598-019-47605-7
135. Lam PH, Chen E, Chiang JJ, Miller GE. Socioeconomic disadvantage, chronic stress, and proinflammatory phenotype: an integrative data analysis across the lifecourse. PNAS Nexus (2022) 1(4):pgac219. doi: 10.1093/pnasnexus/pgac219
136. Petersen BK, Yang J, Grathwohl WS, Cockrell C, Santiago C, An G, et al. Deep reinforcement learning and simulation as a path toward precision medicine. J Comput Biol (2019) 26:597–604. doi: 10.1089/cmb.2018.0168
137. Cockrell RC, An G. Examining the controllability of sepsis using genetic algorithms on an agent-based model of systemic inflammation. PloS Comput Biol (2018) 14(2):e1005876. doi: 10.1371/journal.pcbi.1005876
138. Day JD, Cockrell C, Namas R, Zamora R, An G, Vodovotz Y. Inflammation and disease: modelling and modulation of the inflammatory response to alleviate critical illness. Curr Opin Syst Biol (2018) 12:22–9. doi: 10.1016/j.coisb.2018.08.008
139. Namas RA, Mikheev M, Yin J, Barclay D, Jefferson B, Mi Q, et al. An adaptive, negative feedback circuit in a biohybrid device reprograms dynamic networks of systemic inflammation in vivo. Front Syst Biol (2023) 2:926618. doi: 10.3389/fsysb.2022.926618
140. Fairbank EJ, McGrath JJ, Henderson M, O’Loughlin J, Paradis G. Social support and C-reactive protein in a Québec population cohort of children and adolescents. PloS One (2022) 17(6):e0268210. doi: 10.1371/journal.pone.0268210
141. Den H, Dong X, Chen M, Zou Z. Efficacy of probiotics on cognition, and biomarkers of inflammation and oxidative stress in adults with Alzheimer’s disease or mild cognitive impairment - a meta-analysis of randomized controlled trials. Aging (Albany NY) (2020) 12(4):4010–39. doi: 10.18632/aging.102810
143. Verschure PFMJ, Wierenga S. Future memory: a digital humanities approach for the preservation and presentation of the history of the Holocaust and Nazi crimes. Holocaust Stud (2022) 28(3):331–57. doi: 10.1080/17504902.2021.1979178
144. Kiecolt-Glaser JK, Derry HM, Fagundes CP. Inflammation: depression fans the flames and feasts on the heat. Am J Psychiatry (2015) 172(11):1075–91. doi: 10.1176/appi.ajp.2015.15020152
145. Miossec P, Kolls JK. Targeting IL-17 and TH17 cells in chronic inflammation. Nat Rev Drug Discovery (2012) 11(10):763–76. doi: 10.1038/nrd3794
146. Ghoreschi K, Balato A, Enerbäck C, Sabat R. Therapeutics targeting the IL-23 and IL-17 pathway in psoriasis. Lancet (2021) 397(10275):754–66. doi: 10.1016/S0140-6736(21)00184-7
147. Pavlov VA, Chavan SS, Tracey KJ. Molecular and functional neuroscience in immunity. Annu Rev Immunol (2018) 36:783–812. doi: 10.1146/annurev-immunol-042617-053158
148. Yehuda R, Lehrner A. Intergenerational transmission of trauma effects: putative role of epigenetic mechanisms. World Psychiatry (2018) 17(3):243–57. doi: 10.1002/wps.20568
149. Abul-Husn NS, Kenny EE. Personalized medicine and the power of electronic health records. Cell (2019) 177(1):58–69. doi: 10.1016/j.cell.2019.02.039
150. Ho D, Quake SR, McCabe ERB, Chng WJ, Chow EK, Ding X, et al. Enabling technologies for personalized and precision medicine. Trends Biotechnol (2020) 38(5):497–518. doi: 10.1016/j.tibtech.2019.12.021
151. Verschure PF, Pascoa F, Sharma V. Redefining stroke rehabilitation: Mobilizingthe embodied goal-oriented brain. Curr Opin Neurol (2023) 83:102807. doi: 10.1016/j.conb.2023.102807
152. Vodovotz Y. Computational modelling of the inflammatory response in trauma, sepsis and wound healing: implications for modelling resilience. Interface Focus (2014) 4(5):20140004. doi: 10.1098/rsfs.2014.0004
153. Tong S, Bambrick H, Beggs PJ, Chen L, Hu Y, Ma W, et al. Current and future threats to human health in the Anthropocene. Environ Int (2022) 158:106892. doi: 10.1016/j.envint.2021.106892
154. Galland L. Diet and inflammation. Nutr Clin Pract (2010) 25(6):634–40. doi: 10.1177/0884533610385703
Appendix
The mathematical model used to simulate the impact of different stress conditions on the inflammatory response and cognition comprises five ordinary differential equations that track changes in stress (S), inflammation (M), controller (C), healing (H), and intervention (I). The description of the model equations is written from the individual perspective but could be translated to a societal interpretation, as suggested above. All model variables and parameters are given in arbitrary units owing to a lack of data for calibration.
In Equation 1, the rate of change of stress is modelled to track stress levels in an individual. The first term represents how stress can deviate from a reference or baseline level of stress (Sref) assumed to be present in any individual. Six example factors (fi, where i = 1, 2, …, 6) that generate stress are included in the model. As described in the text, these could be interpreted as internal factors (e.g., diet, physical inactivity, sleep deficiency) or external factors (infection, climate or environment factors, digital stress)—any additional factors could be easily (and eventually) quantified. These stress factors are assumed to vary between 0 ≤ fi ≤ 1, where fi = 1 indicates the highest level of stress that can be invoked by that factor, e.g., the most pro-inflammatory diet (154), and fi = 0 indicates a reduction in the level of stress that could be caused by that factor, e.g., choosing a vegan diet that is minimally pro-inflammatory. A value fi = 0.5 indicates a baseline contribution that would lead to homeostasis based on that factor. The product of Sref and a linear function of these six factors (fi) gives the extent to which stress is increased or decreased from Sref based on the combination of factors for any given individual. These stress inputs are inhibited by outside intervention (I), given by the presence of (1 + I) in the denominator. The second term in Equation 1 represents the increase in stress due to the inflammatory response (a3 term), which is inhibited by healing (H/H∞ term).
In Equation 2, the pro-inflammatory response is triggered in the presence of stress (v1S) but is inhibited by neural control (kcC), healing (kHH), and outside intervention (kII). A natural decay is also assumed in the second term.
Equation 3 shows the rate of change in the control variable. The first term includes the concept of resilience within an individual. This model was designed to include the possibility that exposure to stress does not lead solely to distress; stress can be helpful (i.e., eustress) to a biological system (either individual or population). In the model, if stress remains below a threshold level (Scrit), then stress helps to improve the controller (which is how we define eustress functionally). However, stress increasing above Scrit acts detrimentally on the controller (the functional definition of distress). This parameter, Scrit, is individual-specific and thus can be varied to account for different levels of resilience in each individual. In the second term, healing (η1H) and intervention γ3I) help to promote the system’s neural control abilities, while inflammation decreases it (c2 + kMM). A natural decay term (μCC) is also included that serves to regulate controller function.
As shown in Equation 4, healing is assumed to increase with inflammation (a1M), neural control (a2C), and outside intervention (γ4I), with an assumed saturating effect and decrease with natural decay μH.
Finally, an external intervention is tracked by the model and is assumed to be initially zero. The model includes various time-dependent functions for intervention that are used to simulate a single, long-term intervention (e.g., treatment with medication, Eq. 5a) or multiple, short-term interventions (e.g., exercising a few times per week, Eq. 5b), where ti indicates the time at which treatment is administered. Future iterations of the model could allow intervention to depend on stress, since it could be assumed that a system undergoing higher stress will be treated with higher levels of intervention. All model variables and parameters are expressed in arbitrary units, and the description and values of the parameters are given in Table 1. Files with the open codes of the model are available in the Supplementary Data Sheet.
Keywords: stress, society, climate change, social media, inflammation, mathematical model
Citation: Vodovotz Y, Arciero J, Verschure PFMJ and Katz DL. A multiscale inflammatory map: linking individual stress to societal dysfunction. Front Sci (2024) 1:1239462. doi: 10.3389/fsci.2023.1239462
Received: 13 June 2023; Accepted: 17 November 2023;
Published: 12 March 2024.
Edited by:
Denise Kirschner, University of Michigan, United StatesReviewed by:
Pietro Ghezzi, University of Urbino Carlo Bo, ItalyIoannis P. Androulakis, Rutgers, The State University of New Jersey, United States
Copyright © 2024 Vodovotz, Arciero, Verschure and Katz. This is an open-access article distributed under the terms of the Creative Commons Attribution License (CC BY). The use, distribution or reproduction in other forums is permitted, provided the original author(s) and the copyright owner(s) are credited and that the original publication in this journal is cited, in accordance with accepted academic practice. No use, distribution or reproduction is permitted which does not comply with these terms.
*Correspondence: Yoram Vodovotz, dm9kb3ZvdHp5QHVwbWMuZWR1
†All authors have contributed equally to this work