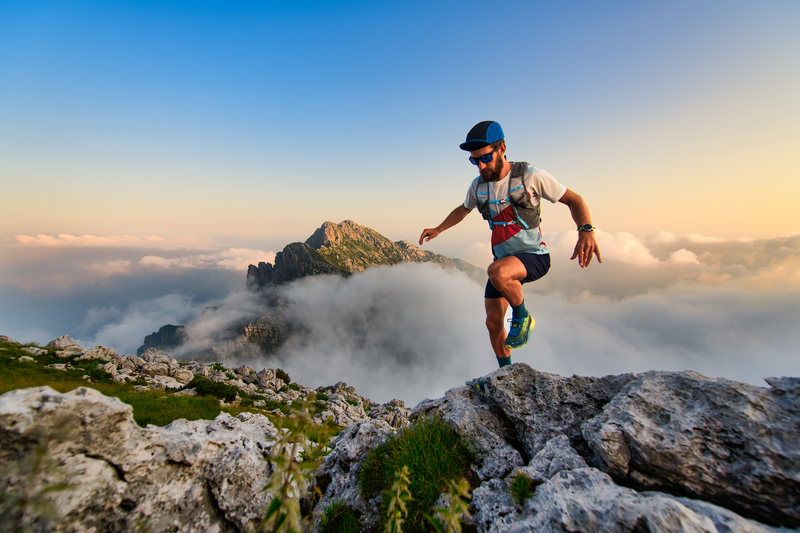
94% of researchers rate our articles as excellent or good
Learn more about the work of our research integrity team to safeguard the quality of each article we publish.
Find out more
ORIGINAL RESEARCH article
Front. Robot. AI
Sec. Robot Learning and Evolution
Volume 12 - 2025 | doi: 10.3389/frobt.2025.1492526
This article is part of the Research Topic Advancements in Neural Learning Control for Enhanced Multi-Robot Coordination View all 3 articles
The final, formatted version of the article will be published soon.
You have multiple emails registered with Frontiers:
Please enter your email address:
If you already have an account, please login
You don't have a Frontiers account ? You can register here
In the realm of real-time environmental monitoring and hazard detection, multi-robot systems present a promising solution for mapping and exploring dynamic fields, particularly where human intervention poses safety risks. This research introduces a strategy for path planning and control of a group of mobile sensing robots to efficiently explore and reconstruct a dynamic field consisting of multiple non-overlapping diffusion sources. Our approach integrates a reinforcement learningbased path planning algorithm to guide the multi-robot formation in identifying diffusion sources, with a clustering-based method for destination selection for the multi-robot formation once a new source is detected, to enhance coverage and accelerate exploration in unknown environments.Simulation results and real-world laboratory tests demonstrate the effectiveness of our approach in mapping and reconstructing the dynamic field. This study advances the field of multi-robot systems in environmental monitoring and has practical implications for rescue missions and field explorations.
Keywords: Multi-Robot Systems, source seeking, dynamic field reconstruction, reinforcement learning, mobile sensor networks
Received: 07 Sep 2024; Accepted: 17 Feb 2025.
Copyright: © 2025 Lu, Sobti, Talwar and Wu. This is an open-access article distributed under the terms of the Creative Commons Attribution License (CC BY). The use, distribution or reproduction in other forums is permitted, provided the original author(s) or licensor are credited and that the original publication in this journal is cited, in accordance with accepted academic practice. No use, distribution or reproduction is permitted which does not comply with these terms.
* Correspondence:
Wencen Wu, San Jose State University, San Jose, United States
Disclaimer: All claims expressed in this article are solely those of the authors and do not necessarily represent those of their affiliated organizations, or those of the publisher, the editors and the reviewers. Any product that may be evaluated in this article or claim that may be made by its manufacturer is not guaranteed or endorsed by the publisher.
Research integrity at Frontiers
Learn more about the work of our research integrity team to safeguard the quality of each article we publish.