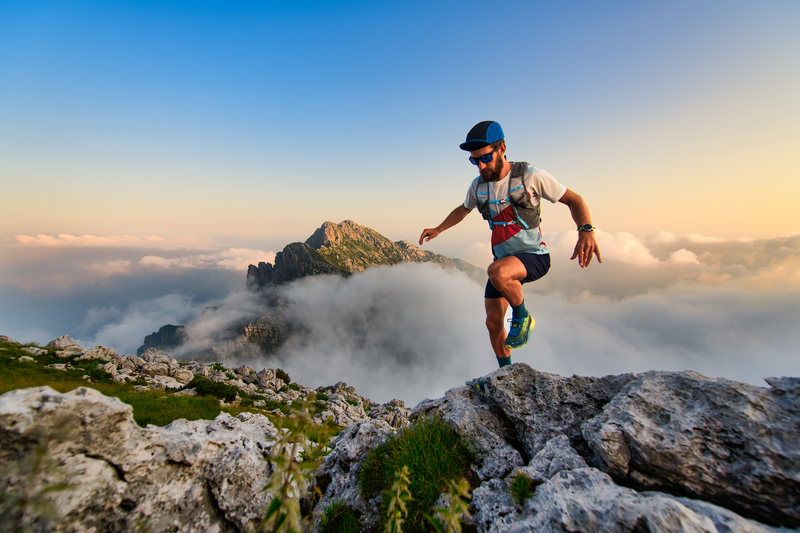
94% of researchers rate our articles as excellent or good
Learn more about the work of our research integrity team to safeguard the quality of each article we publish.
Find out more
ORIGINAL RESEARCH article
Front. Res. Metr. Anal. , 07 January 2025
Sec. Research Methods
Volume 9 - 2024 | https://doi.org/10.3389/frma.2024.1431298
This article is part of the Research Topic Network Analysis of Social Media Texts View all 6 articles
Big Data communication researchers have highlighted the need for qualitative analysis of online science conversations to better understand their meaning. However, a scholarly gap exists in exploring how qualitative methods can be applied to small data regarding micro-bloggers' communications about science articles. While social media attention assists with article dissemination, qualitative research into the associated microblogging practices remains limited. To address these gaps, this study explores how qualitative analysis can enhance science communication studies on microblogging articles. Calls for such qualitative approaches are supported by a practical example: an interdisciplinary team applied mixed methods to better understand the promotion of an unorthodox but popular science article on Twitter over a 2-year period. While Big Data studies typically identify patterns in microbloggers' activities from large data sets, this study demonstrates the value of integrating qualitative analysis to deepen understanding of these interactions. In this study, a small data set was analyzed using NVivo™ by a pragmatist and MAXQDA™ by a statistician. The pragmatist's multimodal content analysis found that health professionals shared links to the article, with its popularity tied to its role as a communication event within a longstanding debate in the health sciences. Dissident professionals used this article to support an emergent paradigm. The analysis also uncovered practices, such as language localization, where a title was translated from English to Spanish to reach broader audiences. A semantic network analysis confirmed that terms used by the article's tweeters strongly aligned with its content, and the discussion was notably pro-social. Meta-inferences were then drawn by integrating the findings from the two methods. These flagged the significance of contextualizing the sharing of a health science article in relation to tweeters' professional identities and their stances on health-related issues. In addition, meta-critiques highlighted challenges in preparing accurate tweet data and analyzing them using qualitative data analysis software. These findings highlight the valuable contributions that qualitative research can make to research involving microblogging data in science communication. Future research could critique this approach or further explore the microblogging of key articles within important scientific debates.
Since 1997, social media platforms have presented new opportunities for academic experts to engage in two-way communication with peers and other networked publics. The networked public sphere (Benkler, 2006) encompasses all spaces that enable the formation of online public discourse. These may be connected through users (in both content production and consumption), signals such as hyperlinks, or shared content. Scholars increasingly use popular digital platforms such as LinkedIn and Twitter (now called X)1 to promote their latest publications and build their reputations. This “pushed” dissemination of scholarship to the networked public differs from traditional academic dissemination. Conventional journal articles and conferences rely on readers pulling their information from a knowledge base that is typically restricted to users from subscribing to academic institutions.
This study examines the dynamics of sharing scientific articles on Twitter, highlighting its role as a platform for science communication and public engagement. This popular microblogging platform has between 550 and 360 million monthly active users worldwide. Micro-bloggers create short messages for real-time communication. This genre's immediate nature supports its users with staying up-to-date on current events. Scholars post on microblogs to communicate online events linking to their publications, encouraging networked discussions on state-of-the-art research.
The importance of such public engagement is recognized through the emergence of altmetrics. Its “alternative bibliometrics” complements traditional citation-based metrics by adding social media outreach to the quantitative analysis of scholarly output and publication (Priem et al., 2012). Altmetrics monitors the sharing of research publications on social media, reference managers, scholarly blogs, and mass media coverage (Moed, 2016). Within popular social network sites, traces of users' deliberations, conversations, and amplification of research articles are tracked. Altmetrics mirrors how network users' actions become a Big Data by-product. This involves the algorithmic processing of extensive digital footprints, or “data traces,” representing user activities on various platforms (Latzko-Toth et al., 2017). Altmetric reports provide insights into the online attention that scholarly documents receive (Fraumann, 2017) and the impact of policy research (Fang, 2021). Twitter metrics for research publication amplification are the most popular data sources that provide the basis for altmetrics (Haustein, 2019).
In science communication (SciComm), quantitative researchers have employed Big Data to investigate the sharing and amplification of science articles on microblogging networks. Related topics that such scholarship explores have included how Twitter users' activity around research publications can be characterized (Díaz-Faes et al., 2019), scientists' sharing of article links (Maleki, 2014), the relationship between Twitter altmetric results and citation results (Costas et al., 2015), the increasing value that academic journals see in promoting their articles on Twitter (Erskine and Hendricks, 2021), the quality of engagement from Twitter audiences with scientific studies (Didegah et al., 2018), and how articles are referenced in Twitter conversations, as part of broader argumentative patterns (Foderaro and Lorentzen, 2023).
SciComm researchers exploring microblogging conversations have called for the use of qualitative approaches that might add new insights regarding digital discourse related to science article shares (Foderaro and Lorentzen, 2023; Lorentzen et al., 2019; Nelhans and Lorentzen, 2016). Including qualitative research approaches inevitably entails a shift to small data projects since these do not use the proliferation of digital traces that Big Data ones do. This approach allows for detailed analysis using manual methods. Small data projects focus on data provided in tightly controlled ways using sampling techniques that limit their scope, temporality, and size (Kitchin and McArdle, 2016). An emergent approach, “small data,” combines basic quantitative metrics with a close reading of the selected microblogging data (Stephansen and Couldry, 2014).
Researchers have described how small data approaches can complement other qualitative methods, such as interviews (Latzko-Toth et al., 2017). However, for researchers focused solely on using small data to study microblogging, no specific rationale is available for qualitative research's potential contribution. This gap starkly contrasts with how qualitative methods for studying social media communication are well-established in their support for a fuller understanding of the context of media practices (Boyd and Crawford, 2012; Quan-Haase et al., 2015). Such methods support a fuller description of the social context of scientific research (Allen and Howell, 2020), plus how practices for a scientific article's sharing may be linked to agreement or dissent in broader science controversies (Venturini and Munk, 2021). The sharing of articles depends on the individual, making it worthwhile for researchers to situate how such practices relate to individuals' identity work. Health practitioners present themselves online by following the strategies of experts. These range from describing their credentials to active listening and making referrals (Rudolf von Rohr et al., 2019).
To address the missing rationale, this study sought to answer the question:
RQ. What role do qualitative methods play in researching Twitter data for a popular science article's sharing?
This question addresses a gap in the literature. Communication research into scientific Twitter often employs Big Data approaches, characterized by large sample sizes. Without a clear rationale for the value of small data approaches, the potential contribution of qualitative research to understanding microblogging communications risks being overlooked. As Borgman (2015) suggests, data can be understood as “big” or “little,” depending on how they are analyzed and utilized. Small approaches, when appropriately scaled to the phenomenon of interest, can still yield significant insights. This article provides a practical example, demonstrating distinctive insights derived from two qualitative lenses applied to the sharing of a science article on Twitter.
The Introduction grounds the salience of this study's research question by first tackling the emergence of new digital genres for science dissemination. Subsequently, the study addresses the importance and use of the “Scientific Twitter” genre. Finally, the introduction calls for qualitative analysis of microblogging data related to scientific conversations.
The emergence of Web 2.0 technologies enabled the networked public to exercise public voice, such as students who become online content creators (Brown et al., 2016). New communication formats (“TikToks,” retweets) serve a wide range of communicational purposes, from entertainment to deliberation on scholarship. Simultaneously, the emergence of new content genres promotes online communication, e.g., Reddit science discussion boards (Pflugfelder and Mahmou-Werndli, 2021). Science communication has witnessed the evolution of ScienceTok, post-peer-review, and Scientific Twitter—the focus of this study. Scientific tweeting is a new science communication genre (Weller et al., 2011), in which scientists microblog about the successes and failures in their fieldwork and ask for advice (Bonetta, 2009). Scholars microblog to share their findings, deliberate on science topics, and stay abreast of the literature. Researchers may microblog about careers, grants, science policy, and other issues. As such, this genre of science popularization is recognized as a new form of scientific output that shares characteristics with other forms of scientific discourse (Costas et al., 2015).
The scientific Twitter genre is non-trivial due to its popularity with researchers: Twitter has been one of the most popular digital platforms among researchers because it supports open engagement with science (Cormier and Cushman, 2021). Over 290,000 scholars on World of Science and Altmetric.com have Twitter accounts (Costas et al., 2020). Scientists who used it potentially comprised 1–5% of its 187 million user base in 2017 (Costas et al., 2017). Between August 2011 and October 2017, over 3.5 million unique Twitter profiles shared tweets that included scholarly output (Díaz-Faes et al., 2019).
Twitter's qualities as a digital platform that is open by default, cheap to access, quick to learn via “texting,” and easy to use contributed to it becoming ubiquitous (Murthy, 2018). Twitter has emerged as the microblog of choice for scientists to communicate with like-minded peers, members of the public, organizations, and the media (Collins et al., 2016). Scholars in academic institutions use Twitter to showcase their professional expertise (Vainio and Holmberg, 2017), connect with colleagues, and share peer-reviewed literature (Priem and Costello, 2010) and self-authored content. They tweet to educate others (Noakes, 2021), follow article discussions, add commentary (Van Noorden, 2014), participate in asynchronous journal clubs (Chary and Chai, 2020), and critique articles almost immediately after publication (Mandavilli, 2011; Yeo et al., 2017). Such communal feedback can complement traditional peer review methods, providing an additional layer of engagement (Sarkar et al., 2022). Scientists use Twitter to engage in discussions with science policymakers (Kapp et al., 2015) and to live-tweet during conferences (Kapp et al., 2015; Collins et al., 2016).
Scientific institutions use Twitter to promote events such as festivals (Su et al., 2017), while its features also facilitate multilingual and EFL (English as a Foreign Language) communication in virtual academic conferences (Márquez and Porras, 2020). Science organizations use hashtags and other affordances for their community-building practices (Su et al., 2017).
Twitter is a popular data source for health-related research, ranging from professional education in healthcare to big data and sentiment analysis (Yeung et al., 2021). As a communication tool, Twitter supports the exploration of the outcomes of divergent styles of science communication, such as the efficacy of humor in tweets (Yeo et al., 2020) and how types of humor are associated with retweets, likes, and comments (Su et al., 2022). Scientists joke about their work using the satirical hashtag #overlyhonestmethods (Simis-Wilkinson et al., 2018).
Limited research has focused exclusively on microblogging conversations in the sharing of science articles (Foderaro and Lorentzen, 2023). Few quantitative studies have addressed this (Nelhans and Lorentzen, 2016). Researchers have explored users whose science tweets link to articles (Maleki, 2014); patterns in Twitter conversations on health (Ola and Sedig, 2020); demonstrating a method for studying the use of scientific sources on Twitter (Foderaro and Lorentzen, 2022); and the argument stages in climate change threads (Foderaro and Lorentzen, 2023).
This study responds to calls for qualitative analysis of Twitter data in science-related conversations and arguments (Lorentzen et al., 2019; Nelhans and Lorentzen, 2016; Pearce et al., 2019). Quantitative researchers have shown that extensive conversations about research can be collected from Twitter. This scientific dialogue is suitable for various automated analysis methods. Since such analysis cannot say much about how Twitter users interact, a need to contextualize conversations via qualitative methods was identified:
Pearce et al. (2019) conducted a systematic and critical literature review focusing on discussions regarding climate change via social media. The review found a substantial bias toward Twitter studies. Gaps were identified regarding qualitative analyses, studies of visual communication, and alternative digital platforms to Twitter. In response to this call, Foderaro and Lorentzen (2023) investigated the practices of argumentation on Twitter by collecting conversational threads focused on climate change. They highlighted that little scholarly attention has been given to interactions in a conversational context. Their content analysis applied coding to tweets at different stages of argument and how linked and embedded sources were used.
Additionally, the plausibility and soundness of a message were coded, alongside the consistency and trustworthiness of linked sources, plus adequacy for the target audience. Foderaro and Lorentzen found that arguing parties were unable to convince each other even with reasonable arguments. This outcome was attributed to the absence of shared values or common premises among participants (p. 145). Consequently, it was more important for Twitter conversationalists to convince their audiences rather than change their opponents' minds.
Another example of research into Twitter conversation undertaken by Lorentzen et al. (2019) analyzed scientific study links. Information retrieval techniques compared segments of these conversations to locate differences and similarities between and within discussions. A qualitative analysis of tweet practices explored the use of unusual terms and categorized distinct conversational properties and academic-linking styles. The authors identified a need to identify controversial issues discussed on Twitter with more sophisticated machine-learning approaches or via qualitative methods. Lorentzen et al. recommended studying complete conversations to better explain how people interact on Twitter.
Qualitative research can address such communications' meanings and their relationship to micro-bloggers' identity work in presenting themselves in the way they do. The calls from SciComm scholars resonate with those from the field of digital discourse analysis. Its researchers are urged to move their field forward by studying the relational practices in social media, users' identity work, and sociability (Garcés-Conejos Blitvich and Bou-Franch, 2019).
The authors' literature review process focused on the role that qualitative methods might play in understanding microblogging practices, with the article being shared in the health sciences field.
Several studies address article sharing and amplification via Twitter. These drew on quantitative or mixed-methods methods to research how epidemiologists exploit the emerging genres of Twitter for public engagement (Tardy, 2023); Twitter (and Facebook and Instagram)'s use by eye-specialist journals, professional societies, and organizations (Cohen and Pershing, 2022); how surgeons use social media platforms (including Twitter) for research communication and impact (Grossman et al., 2021), likewise for biomedical scientists, and how their microblogging choices influence their followings (Sarkar et al., 2022).
Scholars have characterized the landscape of precision nutrition content on Twitter, with a specific focus on nutrigenetics and nutrigenomics (Batheja et al., 2023), and explored how sharing visual abstracts promotes wider suicide prevention research dissemination and altmetrics engagement (Hoffberg et al., 2020). Researchers have explored the anti-vaccination movement's referencing of vaccine-related research articles (Van Schalkwyk et al., 2020), plus changes in eight COVID-19 conspiracy theory discussions over time (Erokhin et al., 2022).
Regarding scholarship on the promotion of health science articles, scholars have described a year-long scientific Twitter campaign, #365Papers, that tweeted one peer-reviewed publication related to cancer and exercise/physical activity per day (Zadravec et al., 2021). Findings suggested this daily campaign stimulated peer and public engagement and dialogue around new scientific publications. Incorporating prominent research field figures into the campaign process assisted strongly with its outreach. A study into the short-term impact of a #TweetTheJournal social media promotion for select open-access psychological journals' articles found that the campaign resulted in a statistically significant, higher Altmetric attention score (Ye and Na, 2018). Researchers have compared the user engagement performance of articles that the Cell journal posted on Twitter and Facebook. An examination of 324 posts suggested that user engagement positively impacted article visits (Cui et al., 2023). Research into stroke-related journals' Twitter usage found that their more frequently tweeted articles tended to have higher citation rates (Sousa et al., 2022). An analysis of 110 articles from PeerJ found that while social media attention follows publication, it does not last long (Cui et al., 2023). This finding resonated with Zhang et al. (2023)'s articles representing recent scientific achievements, which may be tweeted for longer after catching society's attention.
While the social media sharing of health science articles can have a positive influence on their dissemination and citation rates, no research examples could be found that solely focused on specific articles' Twitter communication or proposed a rationale for the role of qualitative research with microblogging data.
Lacking an exemplar to follow, this study developed a qualitative-led approach to grow an understanding of Twitter communications around a scientific article. The six-phased research process comprised: (i) selecting an article to focus on from a long-running scientific debate; (ii) identifying what hyperlinks related to the article could be shared on Twitter; (iii) importing tweet shares into NVivo™ and benchmarking them vs. altmetrics, (iv) preparing a codebook and coding the data in NVivo™; (v) importing the conversations into MAXQDA™ for coding; and (vi) comparing a multimodal content- and semantic network analysis to discuss meta-inferences.
A long-standing academic debate in the health sciences contrasts proponents of the 'cholesterol' model (CM) with those advocating an alternative insulin resistance (IR) paradigm. They argue for an IR paradigm of chronic ill health and low-carbohydrate, healthy fat (LCHF) lifestyles (Noakes et al., 2023b). South Africa has seen many communication episodes involving leading scholars in this debate (http://bit.ly/2OSMfUx). In November 2019, the researchers selected a popular article from among these episodes. Its small dataset of users and tweets seemed feasible for manual analysis via qualitative data analysis software (QDAS). This article (Webster et al., 2019) illuminated how 24 South Africans put their diabetes in remission by following an LCHF diet sustainably with minimal support from health professionals. In February 2024, Digital Science's Altmetric platform showed that this study had achieved an attention score of 93. This placed it among the top 5% of all research outputs Altmetric calculates (Altmetric, 2021). On X, the article had been featured in 158 posts from 137 users, with an upper bound of 632,585 followers.
An iterative process was followed to identify the publication links that might be shared (see Figure 1) via Twitter in relation to Webster et al. (2019). These were divided into shares from (i) academic publishers and (ii) other sources.
Figure 1. Diverse academic publications that might feature in a journal article science communication event.
Under (i), micro-bloggers shared a DOI link, three Dovepress URLs, and two PubMed URLs. Six URLs under (ii) included a critical letter, an author's reply, two blog posts, and Reddit forum discussions. Data extracts were run by Younglings Africa in April 2022 for (i) and (ii), which produced a spreadsheet for each hyperlink's shares. These started from the article's digital publication (5th of December 2019) up to March 2021. This data was extracted the following month.
Quantitative researchers have flagged pertinent concerns regarding flawed science communication on Twitter, plus related limitations in assuming that tweet counts used in Altmetrics denote valuable public outreach (Robinson-Garcia et al., 2017). This study followed their recommendations for removing mechanical (re)tweets while focusing on original tweets linked to science article shares. The analysts checked Twitter users' account activities, plus linked profiles, to ensure they were genuine, not Twitter bots. Automated “contributors” to Scientific Twitter are a real threat in skewing Altmetrics results (Arroyo-Machado et al., 2023).
For accurate and comprehensive tweet data, the research team relied on the extraction code that queried Twitter's API. It also provides data provision services (Bruns and Burgess, 2016). To double-check the accuracy of Webster et al.'s (2019) sharing, (i) extractions were benchmarked against the Altmetric results. This revealed that a few tweets had not been extracted. Webster et al. (2019)'s lead author had deactivated his Twitter account. Deletion of accounts and their tweets is the main reason for scientific publication mentions becoming unavailable, followed by the suspension or protection of tweeters' accounts (Fang et al., 2020). Another issue was that data extractions did not mention any URL in replies to retweets or longer versions of a URL being used. These “missed tweets” were manually captured in a dedicated spreadsheet. Following its import into NVivo™ for Mac, the file's tweets and user account information matched the early March Altmetrics report for Twitter shares (Digital Science, 2022).
Social semiotics is an approach to communication that strives to understand how people communicate by various means in specific social settings (Hodge and Kress, 1988). A social semiotic multimodal content analysis focuses on how people make signs in the context of interpersonal and institutional power relations to achieve specific aims. Modes of communication offer historically specific and socially and culturally shared options for communicating or semiotic resources. Researchers have analyzed how such resources are used in microblogging. Michele Zappavigna analyzed Twitter posts to explore ambient affiliation in expressions of self-deprecation, addiction, and frazzled parenting (Zappavigna, 2014). Her research demonstrated that identities can be thought of as bonds when approached in terms of the social relations they enact. Identities might be regarded as patterns of values when considered in terms of the meanings that they negotiate in discourse.
The semiotic resources that tweeters of Webster et al. (2019) used (such as @mentions, # hashtags, types of URL, and article screengrabs) were defined in the pragmatist's codebook. It was iteratively refined in response to interpretation needs and new literature. Coding the data revealed surprising interpretation challenges. The concept of “level of tweet reply” was added to easily locate a tweet's order in a thread. This ranged from first, as a “deliberation,” to being the “15th reply to a 14th tweet,” An example of the ongoing literature review informing needed coding changes concerned the schema from Nelhans' Twitter conversation patterns related to studies (Nelhans and Lorentzen, 2016, p. 28–9). Its outline was adopted to remedy missed codes and for labeling refinement.
Scholars should integrate different analytical methods for a more comprehensive understanding of Twitter discussions (Dai and Higgs, 2023). A statistician completed a semantic network analysis (SNA) to add to understandings derived from the pragmatists' work. This approach has been well-used for studying the discourse surrounding social movements and public health crises on microblogging platforms. SNA can offer novel perspectives regarding the structural relationships and meanings embedded within tweet content. SNA is a word analysis that explores the proximity of words in a text, whether in pairs or groups. In relation to Webster et al.'s (2019) shares, the SNA spotlighted the semantic connections between words, topics, and users in this data. This was intended to support insights into how the digital public disseminated, discussed, and perceived the article. Understanding the influential actors within this network and how they framed their messaging and interacted with others in discussing the prevalent themes.
There is a wide variety of SNA approaches, but for this study, the statistician's approach was process-driven in working with static Twitter data in MAXQDA™ for the first time. This involved becoming familiar with the many microblogging data fields in QDAS and how codes for content and user information could overlap. The pragmatist shared his codebook and spreadsheet extracts, which the statistician imported into both MAXQDA™ and ATLAS.ti™. For the first SNA step (S1), she created a visual word cloud view of the quantitative data. The next step (S2) explored the proximity of all codes, which proved sufficient for the analysis.
To prepare (S1) word cloud visualizations, the statistician initially searched for words to auto-code by doing a word frequency map in MAXQDA™. These results were checked against ATLAS.ti's, and they matched. All words that featured, whether from profiles or tweet content, were then sampled to produce a word cloud of the most popular terms. This defined prevalent topics within that article's sharing network. Subsequently, the statistician developed a (S2) proximity of codes map. She explored how closely the codes occurred to each other by coding co-occurrences and exploring their proximity visually through network maps. MAXQDA™ displayed the codes according to their “close instance,” how closely they feature together. Each SNA step involved an interplay between quantitative and qualitative approaches. While the steps primarily relied on quantitative analysis, interpreting the visual maps required qualitative insights. Overall, the process was qualitatively driven, with the analyst acting as a “research instrument” to identify the most salient codes.
The final phase involved a comparison of the multimodal content and SNA's results in a mixed-methods approach (Venkatesh et al., 2023). MAXQDA™ is a QDAS tool that supports a statistician's mixed methods with (live) Twitter data (Noakes et al., 2023a), while NVivo™ supports multimodal tweet analysis best. Both QDAS offer quantitative reporting options for either style of analysis. Though it focuses on the contributions of qualitative research, this study foregrounds its aspects from both lenses. This follows the recommendation of Schoonenboom and Johnson (2017) for a mixed-methods research approach to be qualitative dominant or qualitatively driven.
Genuine mixed-method projects conclude with a meta-inference that connects or integrates various claims (Schoonenboom, 2022). A meta-inference is an “overall conclusion, explanation, or understanding developed through an integration of the inferences obtained from the qualitative and quantitative strands of a mixed methods study” (Tashakkori and Teddlie, 2008, p. 102). A meta-inference and its internal structure are developed in successive steps of claim integration, whereby two or more simpler claims are integrated into one more complex claim (Schoonenboom, 2022).
In phase vi, the researchers sought to establish meta-inferences across the presented findings by following the ideal seven-step process. The first three involve defining separate inferences: (i) knowledge-based, (ii) experience-based, and (iii) data-driven, qualitative, and quantitative ones. The investigators then design (iv) inference association maps before (v) eliminating speculative inferences. (vi) Meta inferences are then generated and finalized, as the authors contrast claims to explore which were confirmatory, explanatory, or seemed to feature juxtapositions and contradictions. Step (vii) uses “working backward heuristics” as a meta-inference validation tool. Following the overall comprehensive process enables researchers to “apply analytical, reflexive, and visual tools concurrently to make explicit how meta inferences are linked to the knowledge base, the researchers” experiences, and the actual qualitative and quantitative data from the participants (Younas et al., 2023, p. 289).
In response to this study's research question, this section presents key findings from the pragmatist's and statistician's analyses:
The study incorporated a social semiotic multimodal content analysis. The analysis produced four claims for the article's sharing. It was (M1) predominately shared by IR/LCHF proponents. In contrast, (M2) critics replied to their tweets. The article's sharers followed a (M3) myriad of practices in sharing links, and their conversational threads were (M4) pro-social.
Most of the participants who shared and discussed the science article were health professionals (see Table 1). They typically present their occupations and related expertise online to establish credibility and trust (Sillence, 2010). The high number of profiles mentioning their “occupation” and “expertise” agreed with Vainio and Holmberg's (2017) finding that tweeters who share popularly-tweeted scientific articles tend to describe themselves with these two categories.
Online commentators' profiles reflect the effect (Barnes, 2018), and many of the article's sharers' profiles took a stance that endorsed low-carbohydrate diets. Specific expressions for LCHF support ranged from lengthy self-descriptions (e.g., “40 years old but fitter and stronger than when I was 30 thanks to Keto, then carnivore for the last 3 years”) to the simple (“Unashamedly carnivore”) or via hashtags that ranged from the broad #LCHF movement to the specific #ketones. Four authors indicated that they had written pro-IR publications (ranging from capsules on various health topics in French at Dogmez-vous to blogposts for DietDoctor.com and two books—“Quick Keto” and “The Banting 7-Day Meal Plans”). Promotion of the LCHF lifestyle (or “diet”) denoted support for the IR paradigm, as its proponents believe that “(LCHF) food is medicine.” Just as “Medical” and “Diet follower or nutritional advice” role descriptions could denote IR support, so did “sports-related” ones. Mentioning belonging to a fitness chain for extreme conditioning program training could denote support for LCHF if that chain endorses LCHF as foundational to wellbeing.
It was unsurprising that publications for the IR paradigm were almost exclusively shared by users expressing support for the LCHF lifestyle (or “diet”). This matches Vainio and Holmbergs' finding that scientific articles are tweeted to promote ideological views, especially when an article represents a topic that divides general opinion (Vainio and Holmberg, 2017). As cultural sociology explains, when individuals classify objects, they simultaneously classify themselves (Bourdieu, 1986). LCHF proponents who shared the Webster et al. (2019) article earlier connoted being up-to-date with current IR research developments. Micro-bloggers' academic and scientific role mentions did not address the IR, or cholesterol, paradigm. Rather, “scholarly roles” focused on degrees and work achievements, while “science roles” foregrounded scientific goals, philosophies, and caveats on the Twitter content (“Spreading scientific information, not medical advice”).
The authors and the funding organization behind their manuscript first shared links to Webster et al. (2019). Their deliberations soon attracted like-minded health professionals who supported IR interventions. In contrast, no deliberations from critics were found in the original article shares. The choice to tweet a science article's link is tied to online identity work since micro-bloggers follow narrativization processes in making aspects of themselves and their interests visible (Dayter, 2016; Sadler, 2021). This occurs in Twitter profiles, original posts, and in what tweeters reshare and like. Such construction of digital identity is not a static process but an ongoing and reflexive construction of selfhood (Cover, 2014). Promoting pro-IR articles via posts is congruent with LCHF proponents' ongoing digital identity work. By contrast, sharing evidence for an unconventional LCHF approach would question an orthodox health scientist's identity.
Twitter users drew on a surprisingly wide variety of communication practices while sharing the research article's link. Table 2 shows their codes: Examples of academic shares included one as part of the reading list for a lecture and being linked from a conference presentation. Two participants in the article's study mentioned contributing their data. A few doctors shared the article for affirming their treatment protocols, as did nutritionists and dietitians. The article was linked to #WORLDdiabetes Day as part of an LCHF lifestyle promotion. The article's key point(s) were translated by Spanish and Portuguese health micro-influencers for their Twitter followers.
A microblogging conversation begins with an original post and may branch into various threads through replies. These replies can lead to consensual, discursive, or confrontational exchanges (Barnes, 2018). Although the majority of Twitter discussions around Webster et al. (2019) did not generate replies, those that did were constructive and focused on the article's content. Conversations involved knowledgeable contributors, whether IR/LCHF proponents or their critics. Contributing to their genuine identities, such experts carried the formal norms for civil communication into the scientific Twitter genre. The consensual threads included a request for further information, an agreement marking a thread's conclusion, and praise for the article. The discursive threads featured a query on why Professor Noakes's response letter did not include references and when a rebuttal to his response might be expected from the original letter's writer. While there were relatively few confrontational threads, critics did emphasize the article's methodological limitations. A tweet claim that diabetes might be “reversed” was corrected with the observation that it is rather put in “remission.”
The statistician developed six main claims from her SNA. The first (S1) confirmed that Twitter posts were strongly tied to Webster et al.'s (2019) focus on diet and diabetes. The second (S2) revealed academic links were shared repeatedly by prominent IR advocates. Finally, the (S3) most repeated shares came from England and South Africa. The fourth (S4) collaborated on the findings of the multimodal content analysis regarding who was involved and the content they shared. The fifth claim (S5) stemmed from a critique of the automated quantitative methods in QDAS inaccurately reporting results for “sentiment.” In response, qualitative-led studies could help accurately identify sentiment and users' stances. Since the researchers' close views of “clean” data flagged a few other concerns, finding (S6) concerned how qualitative approaches could add valuable meta-criticisms of Twitter data.
A proximity map (Figure 2) shows the most popular words in yellow for Webster et al.'s (2019) article. The most prevalent topics mentioned in tweets are shown in blue, users in red, and their countries in purple. The left-hand side of the proximity map suggests a heavy overlap between Webster et al.'s frequently used terms and the tweet content that users created in sharing and discussing that article. In contrast, the right-hand side features many of the article's terms that were not included in tweets.
Webster et al. (2019)'s most frequent sharers are shown in Figure 3. (i) Academic links are shown in blue, and (ii) the others in red. Few accounts shared the link more than once or chose to share links from academic publishers and other sources. Amongst the repeat sharers, prominent IR advocacy organizations (such as @LowCarbCanberra) and individuals (like @JeffreyGerberMD) largely posted academic publication links. This reflects the credibility of these sources within the academic use of Twitter for scientific communication.
The inaccurate results for the two outliers are an issue with automated link share reporting. They did not “reshare” the article four and three times, as shown. Instead of representing individual shares, these were erroneously counted multiple times from a seven-tweet thread. An informal link's one-time share became recounted as “seven.”
Figure 4 shows that the most influential tweeters and shares of Webster et al. (2019) emanated from England and South Africa. Additionally, users in Australia, Brazil, Chile, Canada, India, New Zealand, Poland, Scotland, Singapore, Spain, and the United States of America shared the article.
A relational analysis illustrated the proximity and interconnections between codes.
The analyst produced an intersection, co-occurrence, and proximity map. The proximity maps were the most useful in showing the proximity of codes for tweets across all the spreadsheets. In contrast, the intersection and co-occurrence maps were limited to showing URL-sharing data within individual spreadsheets. Figure 5 shows user profiles in red, tweet content in blue, and locations in purple. A (#) accompanies each legend, denoting its specific code number.
Overall, the semantic proximity map collaborated with the findings of the multimodal content analysis regarding who was involved and what they communicated:
Regarding the content discussed, the content nodes on the right for “diabetes” and “diet” were strongly linked to many others. The terms “weight” and “medical” were tied to the research article's content, as were those that stretch across the bottom, such as “individual,” “self-selected,” and “high fat.” In the map's top left were terms tied to the upsides described in Webster et al.'s (2019) LCHF case studies, such as “improve,” “blood,” and “sugar.”
Figure 5 shows the article's fourth author, Professor Tim Noakes, the most mentioned sharer. His status as an international sport science expert and use of provocative posts linking IR to other scientific controversies help explain his centrality to Twitter engagements. This is similar to how entrepreneurs' provocative tweets on new ventures help drive interaction with their content (Seigner et al., 2023).
The statistician initially used Atlas.ti and MAXQDA's automated tools for sentiment analysis. In checking both results, she found many instances where sentiment was incorrectly described as negative where it should have been positive. The following tweet, originally in Portuguese: “Research by Dr. Tim Noakes achieved total or partial REMISSION (!!) of Type 2 Diabetes in 24 subjects who followed the Ketogenic/Low Carb Diet for 6–35 months, no medication required! Incredible news” was coded as slightly negative, though the content is positive. This suggested a methodological limitation of quantitative approaches, such as QDAS, in accurately analyzing static Twitter data.
The SNA uncovered concerns with incorrect automated counts for link shares (in S2) and false results for sentiment analysis (S5). This indicates that microblogging statistics may oversimplify complex categories, leading to inaccurate comparisons. Quantitative simplifications may fail to capture the nuanced complexities of qualitative data. In response, the sixth finding was that a close reading of Twitter data presents a distinct opportunity for meta-critique. Qualitative research can support critiques of microblogging data sources. The results are derived from the automated analyses of data whose categorization is inept.
While MAXQDA™ works very well with a dictionary for auto-coding, it did not work well with static Twitter data in spreadsheets. Although the dataset was relatively small, the software frequently crashed and operated sluggishly. In contrast, there was no automatic coding of words option in Atlas.ti. It does not support the required dictionary. Consequently, the statistician found that (lack of) QDAS support for static Twitter data analysis could be a concern.
The development of meta-inference is the final step in a mixed-methods research project (Schoonenboom, 2022). Following Younas et al. (2023), a seven-step process (i–vii) was followed for developing meta-inferences from findings. The work on the first stages (steps I, II, III, and V) is shown in Table 3 and (step vi) in Table 4. The researchers skipped step iv because comparing the various types of qualitative and quantitative inferences was irrelevant to the main research question's qualitative emphasis.
Table 4. Joint display of inferences and meta-inferences for analyses of a science article's sharing on Twitter.
In the initial stages of this article's fieldwork, the study adopted a multimodal content analysis strategy, followed by a semantic network one. The claims from these mono-methods are presented in Table 3's rows (iii) and (iv). The analysts then elaborated on the meta-inferences from the small data project step-by-step before eliminating the repetitive speculative inferences of row (v). Subsequently, a meta-inference confirmation determines the alignment between different sets of data in a research study to establish if they confirm or potentially expand each other.
Table 4 shows the points of explanation, confirmation, and contradiction that were established by comparing the analysts. The meta-inferences are listed in [brackets]. These were derived from the comparison and largely explained or confirmed the mono-methods' findings.
Two important contradictions emerged in the research process itself. First, there was mis-categorization by quantitative tools in their automated sentiment analysis and inaccurate article share tallying from threads. The researchers flagged that such inaccurate results can be spotted through meta-level critiques. This flags the need for an accurate description of categories based on rigorous qualitative research for apt categorization around meaning(s). Second, while QDAS tools are marketed as making Twitter analysis efficient, the statistician faced challenges using small static spreadsheets or being unable to auto-code depending on the QDAS she worked with.
The raw data supporting the conclusions of this article will be made available by the authors without undue reservation.
The studies involving humans were approved by the Cape Peninsula University Faculty of Health and Wellness Sciences Research Ethics Committee. The studies were conducted in accordance with the local legislation and institutional requirements. Written informed consent for participation was not required from the participants or the participants' legal guardians/next of kin in accordance with the national legislation and institutional requirements.
Written informed consent was not obtained from the individual(s) to publish any potentially identifiable images or data in this article because this information is in the public domain and is not controversial. Sharing pseudonymized tweets in an article does not place their authors in a negative light, so it holds no risk to authors' reputations.
Our research follows the “Ethical decision-making and Internet research (version 2.0) recommendations of the American Association of Internet Researchers” (AAoIR) Ethics Working Committee (Markham et al., 2012) and AAoIR's initial guidance (Ess and Jones, 2004). The ethics committee at the Cape Peninsula University of Technology's (CPUT) Faculty of Health and Wellness Sciences reviewed and approved our project's process and this output (2024URI_NEG_002). Our project complies with Twitter's criteria for data use.
Micro-bloggers are unlikely to expect a review of their tweets for research purposes. Most users expect to give their consent before their tweets are researched (Fiesler and Proferes, 2018). This concern must be weighed against the considerable effort required to provide full anonymity. The authors decided against full anonymization since it would reduce the richness of this study's illustrative examples. At the same time, while there appear to be no reputational risks in sharing tweeters' examples, this study does not show who authored particular tweets.
The findings from each mono-method and their combined meta-inferences suggest the potential contributions that qualitative research can make to studies that focus on small data from microblogging communications.
Qualitative research methods can uncover meanings from inside a communication phenomenon. These methods support explorations of who chooses to tweet scientific content, a detailed description of their practices, and how these may link back to individuals' identity work. A novel finding spotlighted the wide variety of users' sharing practices. Almost 20 different types were uncovered.
Qualitative research can provide a rich contextual framing for how micro-practices relate to important social dynamics. A sociological framing situates Webster et al.'s (2019) publication as a communication event within a long-running debate in the health sciences. Since their article motivated the emergent IR paradigm, its sharing presented opportunities for health professionals to do supportive identity work. As the LCHF interventions they prescribe draw on the IR paradigm, sharing its positive research developments would assist their professional credibility while growing their visibility to sympathetic networks and potential customers. In contrast, the article's critics did not promote its links, choosing to engage in pro-social threads with IR/LCHF proponents.
A qualitative process supported the identification of a wide variety of pro-social practices amongst Twitter sharers by Webster et al.'s (2019) study. This contrasts with quantitative scholarship reporting on the negative practices in Twitter shares for popular dentistry articles. Monomania underpinned their high tweet counts, with most tweeting being mechanical, seemingly devoid of human thought (Robinson-Garcia et al., 2017). Many duplicate tweets originated from centralized management accounts or bots. Few tweets represented genuine engagement with the shared articles, and very few were part of conversations. The contrast with the pro-social Webster et al. (2019) suggest the need to contextualize micro-level communications within higher-level social strata, such as the Global Health Science field and its key debates.
Qualitative research focusing on an in-depth exploration of small data can contribute to the research process by supporting meta-level critiques of missing data, (mis-) categorizations, and flawed automated (and manual) results.
This study contributes a rationale for the contribution of qualitative methods in researching with Twitter and other microblogging data. The study compared two qualitatively led analyses regarding the unusual topic of tweets that shared links to a science article in support of the emergent IR paradigm. A multimodal content analysis supported the rich-and-thick description of users' Twitter practices, showing that they have a much greater variety than previously described in the SciComm literature. This analysis revealed that many of these practices were related to users' professional contexts and digital identity work. Many health professionals' promotion of LCHF lifestyles seemed favored by the article's findings on IR. A semantic network analysis confirmed that communications related to the article, with proponents and critics having very different responses. While prominent proponents shared links repeatedly, critics never did, choosing to reply. The SNA flagged issues with the automated identification of sentiment, suggesting that qualitative research can contribute to accurately categorizing communicators' stances. Meta-inferences from both analyses suggested the important role of qualitative methods for supporting critiques of Twitter data, automated QDAS functions, and verifying the definition of categories that underpin automated results.
This research exhibits the eight “big tent” strengths expected for high-quality qualitative research (Tracy, 2019): It makes a (1) timely and interesting contribution to a neglected research subject by proposing a rationale for qualitative approaches to microblogging research with small data. The two research lenses of multimodal content and SNA were (2) rigorously applied; the meta-inference comparison supports the credibility of the research findings through coherence and expansion. Simultaneously, an in-depth review of the data on which the analysis was based helped ensure that key practices by micro-bloggers did not disappear due to Twitter data export and QDAS import cleaning practices. A conventional approach might only focus on English tweets. This would miss the valuable role of science promoters making translations for their non-English-speaking followers.
Furthermore, adding memos for translated tweets proved useful for incorporating a wider range of contributors. A similar issue emerged with sentiment analysis, where the researcher's knowledge of the text's content differed from MAXQDA's automated findings. Given that the positive, neutral, and negative results seemed inaccurate, they were excluded.
This study's manuscript evolved over a 4-year period. The authors are (3) sincere in sharing the challenges they experienced during the project. They are transparent in the phases and steps taken to prepare their analyses. The (4) credibility of the research is supported by both thick descriptions and the crystallization resulting from meta-inference development. An element of multivocality drew on researchers' perspectives with differing professional backgrounds: a statistician, a media studies pragmatist, an information technology qualitative analyst, and a transdisciplinary research expert. These researchers differed in their opinions on the value of academic Twitter but agreed that this study's findings would (5) resonate with other scholars. This study's findings are transferable to microblogging scholars who could benefit from learning about the roles of qualitative research in analyzing small data. This manuscript's organization and the aesthetic merit in its well-designed figures and tables should support resonance with readers. While the results of the Twitter analysis are not generalizable, a similar methodology could yield valuable insights into the sharing of other popular articles.
The study's topic has methodological (6) significance in assisting its readers in understanding how to analyze a science article's shares on a microblogging platform. The study has conceptual significance in building theory by presenting a fresh rationale. Suggestions for future research in the next section may contribute to heuristic significance by encouraging scholars to address the gaps it spotlights. As described under (7) Ethics, this project was covered by an institutional review. This study is (8) meaningfully coherent in following a clear line of inquiry. Its research question links the literature reviewed to the article's foci, methods, and findings.
Regarding weaknesses, the research team could only focus on learnings produced from two qualitatively led methods. Many other feasible approaches to researching small data, such as critical discourse analysis or grounded theory, could be explored.
There are many opportunities to build on this study's contributions. Researchers could explore and refine the role of qualitative methods in analyzing microblogging communications for science articles. Qualitative research that adds data outside small data microblogging extracts could strengthen the study's rationale.
While this study focused on a relatively small communication event, many examples of meaningful exchanges materialized, indicating the importance of studying events within long-running scientific debates. This resonates with Díaz-Faes et al.'s (2019) suggestion for the scholarship that explores Twitter shares by specific scientific communities. By focusing on tweet shares, our research contributes to the great imbalance in Twitter's use as a social media platform, which is over-researched (Tufekci, 2014). To combat this, scholars can explore science sharing via microblogging platforms outside the Anglosphere. Non-platform-specific creative situational approaches are needed since these provide a much-needed understanding of wider platform dynamics (Özkula et al., 2022).
The raw data supporting the conclusions of this article will be made available by the authors, without undue reservation.
The studies involving humans were approved by Cape Peninsula University Faculty of Health and Wellness Sciences Research Ethics Committee. The studies were conducted in accordance with the local legislation and institutional requirements. Written informed consent for participation was not required from the participants or the participants' legal guardians/next of kin in accordance with the national legislation and institutional requirements. Written informed consent was not obtained from the individual(s) for the publication of any potentially identifiable images or data included in this article because this information is in the public domain and is not controversial. Sharing pseudonymized tweets in an article does not place their authors in a negative light, so holds no risk to authors' reputations.
TN: Conceptualization, Data curation, Formal analysis, Funding acquisition, Investigation, Methodology, Project administration, Resources, Visualization, Writing – original draft, Writing – review & editing, Validation. CU: Formal analysis, Investigation, Methodology, Validation, Writing – original draft, Writing – review & editing, Data curation. PH: Formal analysis, Investigation, Writing – original draft, Writing – review & editing. IZ: Methodology, Resources, Supervision, Writing – original draft, Writing – review & editing.
The author(s) declare financial support was received for the research, authorship, and/or publication of this article. No funding was received for the research and authorship of this article. These were done at each author's own cost. The Noakes Foundation contributed to covering the costs of; the Twitter data extractions and their cleaning for analysis in QDAS, plus related software. The Faculty of Health and Wellness Sciences (FHWS) at the Cape Peninsula University of Technology will pay the overall Academic Publication Cost (APC). Each author will reimburse the FHWS a quarter of this APC charge from their research cost centers. The Noakes Foundation will cover the quarter for any author who cannot access their research cost center.
The authors would like to thank Professor Susanna Priest for her in-depth feedback on the original manuscript, which improved this study's focus. We thank Alwyn van Wyk at Younglings Africa and its software interns for extracting this study's Twitter data. Namely, Shane Abrahams, Tia Demas, Scott Dennis, Ruan Erasmus, Paul Geddes, Sonwabile Langa, Joshua Schell, and Zander Swanepoel. In addition, we are grateful to the senior software data analysts Darryl Chetty and Cheryl Mitchell for guiding these extractions and QDAS import preparations.
The authors declare that the research was conducted in the absence of any commercial or financial relationships that could be construed as a potential conflict of interest.
All claims expressed in this article are solely those of the authors and do not necessarily represent those of their affiliated organizations, or those of the publisher, the editors and the reviewers. Any product that may be evaluated in this article, or claim that may be made by its manufacturer, is not guaranteed or endorsed by the publisher.
1. ^Twitter was purchased by Elon Musk in October 2022. As part of his plans for expanding this digital platform to an “everything app,” Twitter was rebranded to “X” in late July 2023. Our research article refers to Twitter throughout to reflect that we report on “tweets” between late 2019 and the end of March 2021 from the “old” platform. Technically, these data extracts were not from an “X platform.” Referring to “Twitter” enables the authors to accurately speak to pre-August 2023 “Twitter research” in science communication. Attempting to term such scholarship as “X research” may well confuse readers by potentially conflating contemporary research into the X platform with scholarship done on Twitter that stretches back until 2007.
Allen, D., and Howell, J. (2020). Groupthink in Science Greed, Pathological Altruism, Ideology, Competition, and Culture, 1st Edn. Cham: Springer. doi: 10.1007/978-3-030-36822-7
Altmetric (2021). How Is the Altmetric Attention Score calculated? London: Digital Science. Available at: https://help.altmetric.com/support/solutions/articles/6000233311-how-is-the-altmetric-attention-score-calculated-
Arroyo-Machado, W., Herrera-Viedma, E., and Torres-Salinas, D. (2023). The Botization of Science? large-scale study of the presence and impact of Twitter bots in science dissemination. arXiv preprint arXiv:2310.12741. doi: 10.48550/arXiv.2310.12741
Barnes, R. (2018). Uncovering Online Commenting Culture: Trolls, Fanboys and Lurkers, 1 Edn. Springer. Available at: https://www.palgrave.com/gp/book/9783319702346 (accessed February 29, 2024).
Batheja, S., Schopp, E. M., Pappas, S., Ravuri, S., and Persky, S. (2023). Characterizing precision nutrition discourse on Twitter: quantitative content analysis. J. Med. Internet Res. 25, e43701–e43701. doi: 10.2196/43701
Benkler, Y. (2006). The Wealth of Networks: How Social Production Transforms Markets and Freedom. Yale University Press. Available at: http://www.jstor.org/stable/j.ctt1njknw (accessed February 2, 2024).
Borgman, C. L. (2015). Big Data, Little Data, No Data: Scholarship in the Networked World. Cambridge: The MIT Press.
Bourdieu, P. (1986). Distinction: a Social Critique of the Judgement of Taste/Translated By Richard Nice, New Edn. London: Routledge.
Boyd, D., and Crawford, K. (2012). Critical questions for big data. Inform. Commun. Soc. 15, 662–679. doi: 10.1080/1369118X.2012.678878
Brown, C., Czerniewicz, L., and Noakes, T. (2016). Online content creation: looking at students? social media practices through a connected learning lens. Learn. Media Technol. 41, 140–159. doi: 10.1080/17439884.2015.1107097
Bruns, A., and Burgess, J. (2016). “Methodological innovation in precarious spaces: the case of Twitter,” in Digital Methods for Social Science: An Interdisciplinary Guide to Research Innovation, eds. H. Snee, C. Hine, Y. Morey, S. Roberts, and H. Watson (London: Palgrave Macmillan UK), 17–33.
Chary, M. A., and Chai, P. R. (2020). Tweetchats, disseminating information, and sparking further scientific discussion with social media. J. Med. Toxicol. 16, 109–111. doi: 10.1007/s13181-020-00760-0
Cohen, S. A., and Pershing, S. (2022). #Ophthalmology: social media utilization and impact in ophthalmology journals, professional societies, and eye health organizations. Clin. Ophthalmol. 16, 2989–3001. doi: 10.2147/OPTH.S378795
Collins, K., Shiffman, D., and Rock, J. (2016). How are scientists using social media in the workplace? PLoS ONE 11:e0162680. doi: 10.1371/journal.pone.0162680
Cormier, M., and Cushman, M. (2021). Innovation via social media-the importance of Twitter to science. Res. Pract. Thromb. Haemost. 5:373. doi: 10.1002/rth2.12493
Costas, R., Mongeon, P., Ferreira, M. R., van Honk, J., and Franssen, T. (2020). Large-scale identification and characterization of scholars on Twitter. Quant. Sci. Stud. 1, 771–791. doi: 10.1162/qss_a_00047
Costas, R., van Honk, J., and Franssen, T. (2017). Scholars on Twitter: who and how many are they? arXiv preprint arXiv:1712.05667. doi: 10.48550/arXiv.1712.05667
Costas, R., Zahedi, Z., and Wouters, P. (2015). Do “altmetrics” correlate with citations? extensive comparison of altmetric indicators with citations from a multidisciplinary perspective. J. Assoc. Inform. Sci. Technol. 66, 2003–2019. doi: 10.1002/asi.23309
Cover, R. (2014). “Becoming and belonging: performativity, subjectivity and the cultural purposes of social networking,” in Identity Technologies: Constructing the Self Online, eds. A. Poletti and J. Rak (Madison, WI: University of Wisconsin Press), 55–69.
Cui, Y., Fang, Z., and Wang, X. (2023). Article promotion on Twitter and Facebook: a case study of cell journal. J. Inform. Sci. 49, 1218–1228. doi: 10.1177/01655515211059772
Dai, Z., and Higgs, C. (2023). Social network and semantic analysis of Roe v. Wade's Reversal on Twitter. Soc. Sci. Comput. Rev. 42:8944393231178602. doi: 10.1177/08944393231178602
Dayter, D. (2016). Discursive Self in Microblogging: Speech Acts, Stories and Self-Praise. John Benjamins Publishing Company. Available at: http://digital.casalini.it/9789027267528 (accessed February 12, 2024).
Díaz-Faes, A. A., Bowman, T. D., and Costas, R. (2019). Towards a second generation of 'social media metrics': characterizing Twitter communities of attention around science. PLoS ONE 14:e0216408. doi: 10.1371/journal.pone.0216408
Didegah, F., Mejlgaard, N., and Sørensen, M. P. (2018). Investigating the quality of interactions and public engagement around scientific papers on Twitter. J. Informetr. 12, 960–971. doi: 10.1016/j.joi.2018.08.002
Digital Science (2022). Overview of Twitter Attention for Article Published in Diabetes, Metabolic Syndrome and Obesity: Targets and Therapy, December 2019. Dove Medical Press. Available at: https://dovemedicalpress.altmetric.com/details/72148983/twitter
Erokhin, D., Yosipof, A., and Komendantova, N. (2022). COVID-19 conspiracy theories discussion on Twitter. Soc. Media Soc. 8:20563051221126051. doi: 10.1177/20563051221126051
Erskine, N., and Hendricks, S. (2021). The use of Twitter by medical journals: systematic review of the literature. J. Med. Internet Res. 23:e26378. doi: 10.2196/26378
Ess, C., and Jones, S. (2004). “Ethical decision-making and Internet research: recommendations from the aoir ethics working committee,” in Readings in Virtual Research Ethics: Issues and Controversies, 1 Edn, eds. C. Ess and S. Jones (Hershey, PA: IGI Global), 27–44.
Fang, Z. (2021). Towards Advanced Social Media Metrics: Understanding the Diversity and Characteristics of Twitter Interactions Around Science. Leiden: Leiden University.
Fang, Z., Dudek, J., and Costas, R. (2020). The stability of Twitter metrics: a study on unavailable Twitter mentions of scientific publications. J. Assoc. Inform. Sci. Technol. 71, 1455–1469. doi: 10.1002/asi.24344
Fiesler, C., and Proferes, N. (2018). “Participant” perceptions of Twitter research ethics. Soc. Media Soc. 4:2056305118763366. doi: 10.1177/2056305118763366
Foderaro, A., and Lorentzen, D. (2022). Facts and arguments checking: investigating the occurrence of scientific argument on Twitter. SSRN Electr. J. 2022:4141496. doi: 10.2139/ssrn.4141496
Foderaro, A., and Lorentzen, D. G. (2023). Argumentative practices and patterns in debating climate change on Twitter. Aslib J. Inform. Manag. 6, 131–148. doi: 10.1108/AJIM-06-2021-0164
Fraumann, G. (2017). Valuation of Altmetrics in Research Funding (M. Sc. Admin). University of Tampere, Finland. Available at: https://nbn-resolving.org/urn:nbn:de:0168-ssoar-58249-9
Garcés-Conejos Blitvich, P., and Bou-Franch, P. (2019). Introduction to analyzing digital discourse: new insights and future directions. Anal. Digit. Discour. 6, 3–22. doi: 10.1007/978-3-319-92663-6_1
Grossman, R., Sgarbura, O., Hallet, J., and Søreide, K. (2021). Social media in surgery: evolving role in research communication and beyond. Langenbeck's Archiv. Surg. 406, 505–520. doi: 10.1007/s00423-021-02135-7
Haustein, S. (2019). “Scholarly Twitter metrics,” in Springer Handbook of Science and Technology Indicators, eds. W. Glänzel, H. F. Moed, U. Schmoch, and M. Thelwall (Cham: Springer International Publishing), 729–760.
Hoffberg, A. S., Huggins, J., Cobb, A., Forster, J. E., and Bahraini, N. (2020). Beyond journals-visual abstracts promote wider suicide prevention research dissemination and engagement: a randomized crossover trial. Front. Res. Metr. Analyt. 5:564193. doi: 10.3389/frma.2020.564193
Kapp, J. M., Hensel, B. K., and Schnoring, K. T. (2015). Is Twitter a forum for disseminating research to health policy makers? Ann. Epidemiol. 25, 883–887. doi: 10.1016/j.annepidem.2015.09.002
Kitchin, R., and McArdle, G. (2016). What makes big data, big data? exploring the ontological characteristics of 26 datasets. Big Data Soc. 3:2053951716631130. doi: 10.1177/2053951716631130
Latzko-Toth, G., Bonneau, C., and Millette, M. (2017). Small data, thick data: thickening strategies for trace-based social media research. SAGE Handb. Soc. Media Res. Methods 13. 199–214. doi: 10.4135/9781473983847.n13
Lorentzen, D., Nelhans, G., Eklund, J., and Ekström, B. (2019). “On the potential for detecting scientific issues and controversies on Twitter: a method for investigation conversations mentioning research,” in 17th International Conference on Scientometrics & Informetrics, ISSI2019, 2–5 September, 2019, Rome.
Maleki, A. (2014). “Twitter users in science tweets linking to articles: the case of web of science articles with Iranian authors,” in SIGMET Workshop METRICS (Seattle, WA). Available at: https://www.researchgate.net/publication/337567330_Twitter_Users_in_Science_Tweets_Linking_to_Articles_The_Case_of_Web_of_Science_Articles_with_Iranian_Authors
Markham, A., Buchanan, E., and Committee, A. E. W. (2012). Ethical Decision-Making and Internet Research (Version 2.0) Recommendations from the AAoIR Ethics Working Committee. Chicago, IL: Association of Internet Researchers. Available at: https://aoir.org/reports/ethics2.pdf
Márquez, M. C., and Porras, A. M. (2020). Science communication in multiple languages is critical to its effectiveness. Front. Commun. 5:31. doi: 10.3389/fcomm.2020.00031
Moed, H. F. (2016). Altmetrics as traces of the computerization of the research process. Theor. Informetr. Schol. Commun. 21, 360–371. doi: 10.1515/9783110308464-021
Nelhans, G., and Lorentzen, D. (2016). Twitter conversation patterns related to research papers. Inform. Res. 21:n2. Available at: https://informationr.net/ir/21-2/SM2.html
Noakes, T. (2021). The value (or otherwise) of social media to the medical professional: some personal reflections. Curr. Allergy Clin. Immunol. 34, 3–9. doi: 10.10520/ejc-caci-v34-n1-a5
Noakes, T., Harpur, P., and Uys, C. (2023a). Noteworthy disparities with four CAQDAS tools: explorations in organising live Twitter data. Soc. Sci. Comput. Rev. 2023:e08944393231204163. doi: 10.1177/08944393231204163
Noakes, T., Murphy, T., Wellington, N., Kajee, H., Bullen, J., Rice, S., et al. (2023b). Ketogenic: the Science of Therapeutic Carbohydrate Restriction in Human Health. Elsevier Science. Available at: https://books.google.co.za/books?id=I1FVzwEACAAJ (accessed April 10, 2024).
Ola, O., and Sedig, K. (2020). Understanding discussions of health issues on Twitter: a visual analytic study. Onl. J. Publ. Health Inform. 12:e2. doi: 10.5210/ojphi.v12i1.10321
Özkula, S. M., Lompe, M., Vespa, M., Sørensen, E., and Zhao, T. (2022). When URLs on social networks become invisible: bias and social media logics in a cross-platform hyperlink study. First Monday 27:12568. doi: 10.5210/fm.v27i6.12568
Pearce, W., Niederer, S., Özkula, S. M., and Sánchez Querubín, N. (2019). The social media life of climate change: platforms, publics, and future imaginaries. WIREs Clim. Change 10:e569. doi: 10.1002/wcc.569
Pflugfelder, E. H., and Mahmou-Werndli, A. (2021). Impacts of genre and access on science discussions: “the New Reddit Journal of Science”. J. Sci. Commun. 20:A04. doi: 10.22323/2.20050204
Priem, J., and Costello, K. L. (2010). How and why scholars cite on Twitter. Proc. Am. Soc. Inform. Sci. Technol. 47, 1–4. doi: 10.1002/meet.14504701201
Priem, J., Piwowar, H. A., and Hemminger, B. M. (2012). Altmetrics in the wild: using social media to explore scholarly impact. arXiv preprint arXiv:1203.4745. doi: 10.48550/arXiv.1203.4745
Quan-Haase, A., Martin, K., and McCay-Peet, L. (2015). Networks of digital humanities scholars: the informational and social uses and gratifications of Twitter. Big Data Soc. 2:2053951715589417. doi: 10.1177/2053951715589417
Robinson-Garcia, N., Costas, R., Isett, K., Melkers, J., and Hicks, D. (2017). The unbearable emptiness of tweeting-about journal articles. PLoS ONE 12:e0183551. doi: 10.1371/journal.pone.0183551
Rudolf von Rohr, M.-T., Thurnherr, F., and Locher, M. A. (2019). Linguistic expert creation in online health practices. Anal. Digit. Discour. 8, 219–250. doi: 10.1007/978-3-319-92663-6_8
Sadler, N. (2021). Fragmented Narrative: Telling and Interpreting Stories in the Twitter Age. London: Routledge.
Sarkar, A., Giros, A., Mockly, L., Moore, J., Moore, A., Nagareddy, A., et al. (2022). Unlocking the microblogging potential for science and medicine. bioRxiv 2022.2004.2022.488804. doi: 10.1101/2022.04.22.488804
Schoonenboom, J. (2022). “Developing the meta-inference in mixed methods research through successive integration of claims,” in Routledge Handbook for Advancing Integration in Mixed Methods Research, 1 edn, eds. J. H. Hitchcock and A. J. Onwuegbuzie (London: Taylor & Francis), 55–70. Available at: https://library.oapen.org/handle/20.500.12657/53964 (accessed April 7, 2024).
Schoonenboom, J., and Johnson, R. B. (2017). How to construct a mixed methods research design. KZfSS Kölner Zeitschrift für Soziologie und Sozialpsychologie 69, 107–131. doi: 10.1007/s11577-017-0454-1
Seigner, B. D. C., Milanov, H., Lundmark, E., and Shepherd, D. A. (2023). Tweeting like Elon? provocative language, new-venture status, and audience engagement on social media. J. Bus. Ventur. 38:106282. doi: 10.1016/j.jbusvent.2022.106282
Sillence, E. (2010). Seeking out very like-minded others: exploring trust and advice issues in an online health support group. Int. J. Web Based Commun. 6, 376–394. doi: 10.1504/IJWBC.2010.035840
Simis-Wilkinson, M., Madden, H., Lassen, D., Su, L. Y.-F., Brossard, D., Scheufele, D. A., et al. (2018). Scientists joking on social media: an empirical analysis of #overlyhonestmethods. Sci. Commun. 40, 314–339. doi: 10.1177/1075547018766557
Sousa, J., Alves, I. N., Sargento-Freitas, J., and Donato, H. (2022). The Twitter factor: how does twitter impact #stroke journals and citation rates? Int. J. Stroke 18, 586–589. doi: 10.1177/17474930221136704
Stephansen, H. C., and Couldry, N. (2014). Understanding micro-processes of community building and mutual learning on Twitter: a 'small data' approach. Inform. Commun. Soc. 17, 1212–1227. doi: 10.1080/1369118X.2014.902984
Su, L. Y.-F., McKasy, M., Cacciatore, M. A., Yeo, S. K., DeGrauw, A. R., and Zhang, J. S. (2022). Generating science buzz: an examination of multidimensional engagement with humorous scientific messages on Twitter and Instagram. Sci. Commun. 44, 30–59. doi: 10.1177/10755470211063902
Su, L. Y.-F., Scheufele, D. A., Bell, L., Brossard, D., and Xenos, M. A. (2017). Information-sharing and community-building: exploring the use of Twitter in science public relations. Sci. Commun. 39, 569–597. doi: 10.1177/1075547017734226
Tardy, C. M. (2023). How epidemiologists exploit the emerging genres of twitter for public engagement. Engl. Spec. Purpos. 70, 4–16. doi: 10.1016/j.esp.2022.10.005
Tashakkori, A., and Teddlie, C. (2008). “7 quality of inferences in mixed methods research: calling for an integrative framework,” in Advances in Mixed Methods Research, ed. M. M. Bergman (New York, NY: SAGE Publications Ltd), 101–119.
Tracy, S. J. (2019). Qualitative Research Methods: Collecting Evidence, Crafting Analysis, Communicating Impact. Hoboken, NJ: John Wiley & Sons.
Tufekci, Z. (2014). “Big questions for social media big data: representativeness, validity and other methodological pitfalls,” in Proceedings of the International AAAI Conference on Web and Social Media. Oxford.
Vainio, J., and Holmberg, K. (2017). Highly tweeted science articles: who tweets them? an analysis of Twitter user profile descriptions. Scientometrics 112, 345–366. doi: 10.1007/s11192-017-2368-0
Van Noorden, R. (2014). Online collaboration: scientists and the social network. Nat. News 512:126. doi: 10.1038/512126a
Van Schalkwyk, F., Dudek, J., and Costas, R. (2020). Communities of shared interests and cognitive bridges: the case of the anti-vaccination movement on Twitter. Scientometrics 125:3551. doi: 10.1007/s11192-020-03551-0
Venkatesh, V., Brown, S., and Sullivan, Y. (2023). Conducting Mixed-Methods Research: From Classical Social Sciences to the Age of Big Data and Analytics. Blacksburg, VA: Virginia Tech Publishing.
Venturini, T., and Munk, A. K. (2021). Controversy Mapping: A Field Guide, 1 Edn. Cambridge: Polity Press.
Webster, C. C., Murphy, T. E., Larmuth, K. M., Noakes, T. D., and Smith, J. A. (2019). Diet, diabetes status, and personal experiences of individuals with type 2 diabetes who self-selected and followed a low carbohydrate high fat diet. Diabet. Metabol. Syndr. Obes. 12:2567. doi: 10.2147/DMSO.S227090
Weller, K., Dröge, E., and Puschmann, C. (2011). “Citation analysis in Twitter: approaches for defining and measuring information flows within tweets during scientific conferences,” in 8th Extended Semantic Web Conference (ESWC 2011) #MSM2011 (Heraklion). Available at: https://ceur-ws.org/Vol-718/msm2011_proceedings.pdf
Ye, Y. E., and Na, J.-C. (2018). To get cited or get tweeted: a study of psychological academic articles. Onl. Inform. Rev. 42, 1065–1081. doi: 10.1108/OIR-08-2017-0235
Yeo, S. K., Liang, X., Brossard, D., Rose, K. M., Korzekwa, K., Scheufele, D. A., et al. (2017). The case of #arseniclife: blogs and Twitter in informal peer review. Publ. Underst. Sci. 26, 937–952. doi: 10.1177/0963662516649806
Yeo, S. K., Su, L. Y.-F., Cacciatore, M. A., McKasy, M., and Qian, S. (2020). Predicting intentions to engage with scientific messages on Twitter: the roles of mirth and need for humor. Sci. Commun. 42, 481–507. doi: 10.1177/1075547020942512
Yeung, A. W. K., Kletecka-Pulker, M., Eibensteiner, F., Plunger, P., Völkl-Kernstock, S., Willschke, H., et al. (2021). Implications of Twitter in health-related research: a landscape analysis of the scientific literature. Front. Publ. Health 907:654481. doi: 10.3389/fpubh.2021.654481
Younas, A., Fàbregues, S., and Creswell, J. W. (2023). Generating metainferences in mixed methods research: a worked example in convergent mixed methods designs. Methodol. Innov. 16, 276–291. doi: 10.1177/20597991231188121
Zadravec, K., Weller, S., Meyers, L., Lane, K., Kong, J., and Campbell, K. (2021). The impact of #365Papers: a daily scientific Twitter campaign to disseminate exercise oncology literature. Internet J. Allied Health Sci. Pract. 19:2059. doi: 10.46743/1540-580X/2021.2059
Zappavigna, M. (2014). Enacting identity in microblogging through ambient affiliation. Discour. Commun. 8, 209–228. doi: 10.1177/1750481313510816
Keywords: content analysis, debate about health science, microblogging data, multimodal content analysis, research method, semantic network analysis, science communication, small data analysis
Citation: Noakes T, Uys CS, Harpur PA and van Zyl I (2025) A role for qualitative methods in researching Twitter data on a popular science article's communication. Front. Res. Metr. Anal. 9:1431298. doi: 10.3389/frma.2024.1431298
Received: 11 May 2024; Accepted: 09 December 2024;
Published: 07 January 2025.
Edited by:
Patrick Ngulube, University of South Africa, South AfricaReviewed by:
James Danowski, University of Illinois Chicago, United StatesCopyright © 2025 Noakes, Uys, Harpur and van Zyl. This is an open-access article distributed under the terms of the Creative Commons Attribution License (CC BY). The use, distribution or reproduction in other forums is permitted, provided the original author(s) and the copyright owner(s) are credited and that the original publication in this journal is cited, in accordance with accepted academic practice. No use, distribution or reproduction is permitted which does not comply with these terms.
*Correspondence: Travis Noakes, bm9ha2VzdEBjcHV0LmFjLnph
Disclaimer: All claims expressed in this article are solely those of the authors and do not necessarily represent those of their affiliated organizations, or those of the publisher, the editors and the reviewers. Any product that may be evaluated in this article or claim that may be made by its manufacturer is not guaranteed or endorsed by the publisher.
Research integrity at Frontiers
Learn more about the work of our research integrity team to safeguard the quality of each article we publish.