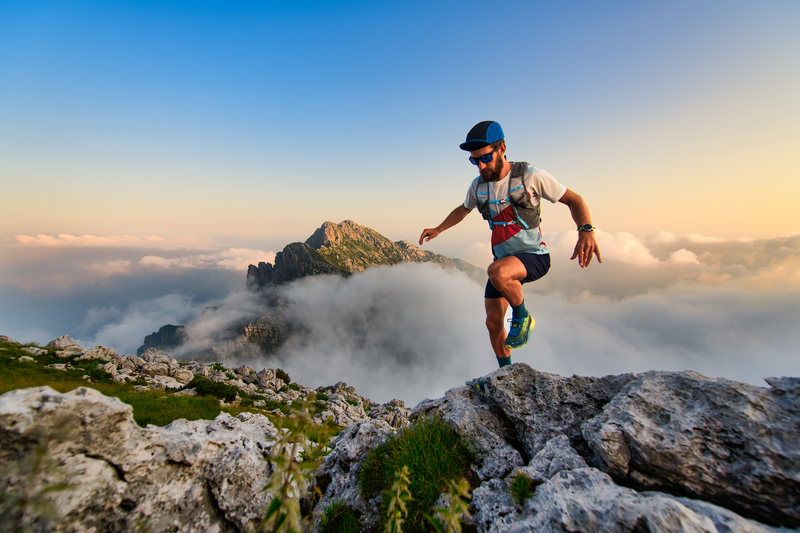
94% of researchers rate our articles as excellent or good
Learn more about the work of our research integrity team to safeguard the quality of each article we publish.
Find out more
REVIEW article
Front. Psychol.
Sec. Psychopathology
Volume 16 - 2025 | doi: 10.3389/fpsyg.2025.1492471
This article is part of the Research Topic Translational research advancements utilizing the Iowa Gambling Task in preclinical and clinical studies: 30 years of the IGT View all 6 articles
The final, formatted version of the article will be published soon.
You have multiple emails registered with Frontiers:
Please enter your email address:
If you already have an account, please login
You don't have a Frontiers account ? You can register here
The Iowa Gambling Task (IGT) is a widely used tool for assessing decision-making in clinical populations. In each trial of the task, participants freely select from different playing card decks that vary in the magnitude and frequency of rewards and punishments. Good decks offer relatively smaller rewards on each trial yielding greater overall winnings while bad decks result in a net loss over time as high penalties negate any rewards earned. Comparing participants' rate of selecting good to bad decks can provide insight into learning in uncertain conditions across time. However, inconsistent patterns of deficits and learning within clinical and control populations are often observed in the task (eg., in depression). Thus, a clearer mechanistic understanding of the IGT is needed to fully understand the decision-making process. The goal of the current review is to synthesize the predominant empirical and theoretical literature of the IGT using event-related potentials (ERPs) derived from electroencephalogram (EEG). The review then explores how modifications of the IGT allow for event-related potentials to be captured at each stage of decision-making. Lastly, the review discusses how the modified version with ERPs can be further applied to directly assess the impact of emotion processing on decision-making, using findings from depression research as an example.
Keywords: IGT, EEG, ERP, Iowa Gamble Task, clinical, Iowa Gambling Task (IGT)
Received: 07 Sep 2024; Accepted: 26 Feb 2025.
Copyright: © 2025 Latibeaudiere, Butler and Owens. This is an open-access article distributed under the terms of the Creative Commons Attribution License (CC BY). The use, distribution or reproduction in other forums is permitted, provided the original author(s) or licensor are credited and that the original publication in this journal is cited, in accordance with accepted academic practice. No use, distribution or reproduction is permitted which does not comply with these terms.
* Correspondence:
Max Jacob Owens, University of South Florida St. Petersburg, St. Petersburg, United States
Disclaimer: All claims expressed in this article are solely those of the authors and do not necessarily represent those of their affiliated organizations, or those of the publisher, the editors and the reviewers. Any product that may be evaluated in this article or claim that may be made by its manufacturer is not guaranteed or endorsed by the publisher.
Research integrity at Frontiers
Learn more about the work of our research integrity team to safeguard the quality of each article we publish.