- 1Department of Neurology and Stroke, Hertie Institute for Clinical Brain Research, University of Tübingen, Tübingen, Germany
- 2Institute of Medical Psychology and Behavioral Neurobiology, University of Tübingen, Tübingen, Germany
- 3Deutsches Resilienz Zentrum, University Medical Center Mainz, Mainz, Germany
For the last 30 years, non-invasive brain stimulation (NIBS) approaches, using transcranial magnetic stimulation (TMS) and transcranial direct or alternating current stimulation (TCS), have treated the brain as a black box, ignoring its internal state at the time of stimulation. While inter-individual variability is long known to undermine the replicability of NIBS effects (Figure 1A), intra-individual variability across and within sessions has only recently gained attention (Ziemann and Siebner, 2015). NIBS effects are state-dependent on a time scale of minutes to hours, depending on the immediate history of neural activity (Silvanto et al., 2008) and synaptic plasticity (Ziemann and Siebner, 2008; Karabanov et al., 2015). However, brain states also change on the time scale of seconds to milliseconds, as neurons are heavily influenced by the temporospatial dynamics of spontaneous network activity, governed by rhythmic fluctuations in neural excitability (Buzsáki and Draguhn, 2004; Schroeder and Lakatos, 2009) under the control of ascending neuromodulatory systems and thalamo- and cortico-cortical projections (Lee and Dan, 2012; Harris, 2013; Zagha and McCormick, 2014). Frequency, amplitude, and phase of neuronal oscillations constitute transient local, network, or even global brain states that not only determine the fate of incoming sensory stimuli (VanRullen and Koch, 2003; Sadaghiani et al., 2010), but also affect both the immediate (“online”) neuronal response to NIBS and the subsequent after-effects (“offline”) resulting from NIBS-induced synaptic changes. It has therefore been suggested to not only optimize NIBS protocols based on neuroimaging data to account for individual differences in functional neuroanatomy (Bergmann et al., 2016; Thut et al., 2017) but also to take the current oscillatory brain state into account (Bergmann et al., 2016; Karabanov et al., 2016; Zrenner et al., 2016). Technical advances allow to assess ongoing multi-channel EEG data in real-time (Bergmann et al., 2012b; Thies et al., 2018; Zrenner et al., 2018) and modify stimulation parameters on the fly (Habibollahi Saatlou et al., 2018) to apply brain state-dependent brain stimulation (BSDBS).
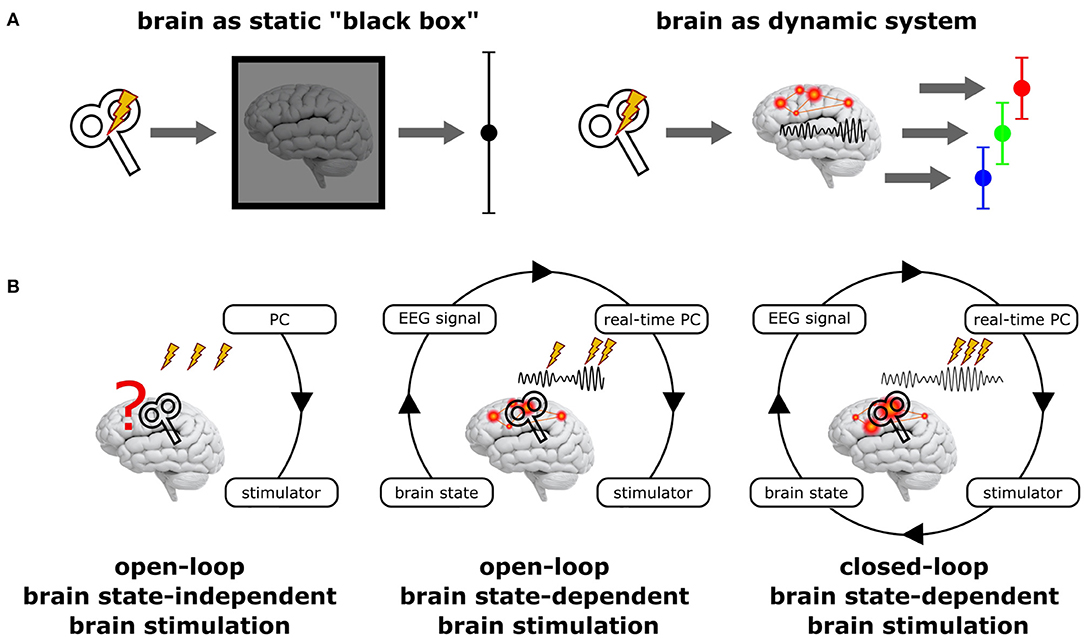
Figure 1. Principal scenarios of brain stimulation with respect to the current brain state. (A) Standard NIBS approaches treat the brain as a static “black box” (left), disregarding its variable internal state and may hence result in highly variable stimulation effects. In contrast, treating the brain as the dynamic system it actually is (right) may reveal very different (state-dependent) effects, but each of them being more homogenous. (B) Open-loop brain state-independent brain stimulation neglects the current brain state; no neuroimaging method and no real-time system is necessary to control the stimulation. Open-loop brain state-dependent brain stimulation (BSDBS) uses concurrent neuroimaging (e.g., EEG) and real-time signal analysis to monitor the current brain state and to adjust and trigger brain stimulation accordingly, however, without systematically changing the monitored target brain state (e.g., TMS triggered by the amplitude or phase of a certain EEG oscillation to assess state-specific corticospinal excitability but without considerable effect on the monitored oscillation). Closed-loop BSDBS additionally requires that the monitored brain state is actually changed by the stimulation, allowing to control the expression of a certain brain state.
Open-Loop vs. Closed-Loop BSDBS
BSDBS is often equated with closed-loop stimulation, which is not justified in most cases (Figure 1B). A closed-loop circuit in the strict sense continuously monitors a specific parameter of a system (e.g., a certain state-space of the brain) and adjusts a control signal (e.g., brain stimulation) accordingly to achieve and maintain a desired set-point of the monitored parameter (e.g., a specific brain state), just like a thermostat measures room temperature and regulates hot water influx to a radiator in order to achieve and maintain a predefined room temperature. But if the control signal has no effect on the monitored parameter (e.g., if brain stimulation does not effectively alter the monitored brain state), the loop remains open, even though the stimulation was applied in a brain state-dependent fashion.
Applications for BSDBS
While BSDBS may have the potential to reduce the variability of NIBS effects (Figure 1A), it first and foremost provides a unique opportunity to study the neurophysiology and function of brain states, in particular neuronal oscillations. NIBS in general can be used online to quantify network properties (such as cortical excitability or connectivity), interfere with task-related neuronal activity (to impair behavioral performance), or modulate the level and timing of neuronal activity (e.g., to entrain neuronal oscillations and affect associated cognitive function); alternatively, NIBS can also be used offline to change synaptic efficacy, inducing LTP- and LTD-like changes in cortical excitability and connectivity (for a conceptual introduction to NIBS approaches see Bergmann et al., 2016). Importantly, all these strategies can be also employed in a brain state-dependent manner to study neuronal oscillations. Real-time EEG-triggered TMS has been used to quantify the excitability profile of specific oscillations: corticospinal excitability is larger during the peak (up-state) than the trough (down-state) of the sleep slow oscillation (<1 Hz) (Bergmann et al., 2012a), whereas it is increased during the trough compared to the peak of the 8–14 Hz sensorimotor mu-alpha rhythm (Zrenner et al., 2018) and is positively related to mu-alpha amplitude (Thies et al., 2018). TMS may also be used to interfere with information processing that is time-locked to specific oscillatory events or phases to probe their causal role for a cognitive function, such as memory reactivation during slow oscillation-spindle-ripple coupling (Staresina et al., 2015) or visual processing during alpha-gamma coupling (Jensen et al., 2014). Eventually, BSDBS may also be used to modulate, i.e., up- and down-regulate neuronal oscillations via the repeated time-locked stimulation of specific oscillatory phases, in analogy to the EEG-triggered auditory closed-loop modulation of sleep slow oscillations (Ngo et al., 2013). Recent TACS studies demonstrated feasibility of semi-closed-loop BSDBS (with brain state monitoring being interrupted during TACS application due to massive stimulation artifacts) for slow oscillations (Ketz et al., 2018) and spindles (Lustenberger et al., 2016), as well as tremor modulation (using peripheral accelerometry as a proxy for the neuronal tremor signal) (Brittain et al., 2013). But also offline BSDBS has been developed: Inspired by seminal work in rodents demonstrating that LTP- and LTD-like plasticity can be induced by bursts of electric stimulation timed to the peak or trough of the ongoing hippocampal theta oscillation (Huerta and Lisman, 1995, 1996), Zrenner et al. (2018) recently used EEG-triggered TMS bursts to induce phase-dependent plasticity in the human motor cortex with respect to the sensorimotor mu-alpha rhythm (Zrenner et al., 2018). Repeatedly targeting the more excitable oscillatory phase, one may tap into the same neural mechanism that underlies the proposed role of cross-frequency phase-amplitude coupling (PAC) in synaptic plasticity (Bergmann and Born, 2018).
Challenges and Future Perspectives for BSDBS
So far BSDBS mainly relies on EEG due to its high temporal resolution, the ease of application and real-time data extraction, and its principal compatibility with NIBS. However, similar (MEG) or complementary (fMRI or fNIRS) neuroimaging techniques should be employed for BSDBS in the future, even though their combination with NIBS is more challenging (Bergmann et al., 2016). The accessibility of deep brain structures together with its excellent spatial resolution and whole brain coverage renders fMRI a highly promising tool to extract more complex brain states, e.g., using multi-voxel pattern classification, even though its low temporal resolution prevents a direct assessment of most neuronal oscillations. Regarding real-time signal analysis, the major challenge is to improve oscillatory brain-state extraction by developing better temporal and spatial filters, and more robust signal forecasting, which can be particularly demanding for oscillations with low signal-to-noise-ratio, non-sinusoidal waveforms, and high variability in amplitude and frequency over time. Also the spatial component of brain states and the adaptation of stimulation parameters should be considered. Automated robotic coil navigation (Harquel et al., 2017) or novel multi-channel coils (Koponen et al., 2018) principally allow to select stimulation sites in real-time, e.g., to follow traveling waves or to target different network nodes. Also NIBS intensity or frequency can be adapted online, e.g., to compensate spontaneous fluctuations in excitability or oscillatory frequency. Yet, the most important challenge will be to develop truly closed-loop BSDBS (Figure 1B) that allows to continuously monitor the brain signals of interest, while concurrently applying NIBS to achieve and maintain the desired brain state and to control perception or behavior. The successful real-time removal of TMS/TCS stimulation artifacts (Walter et al., 2012; Herring et al., 2015; Rogasch et al., 2017) and EEG correlates of multisensory co-stimulation during TMS (Herring et al., 2015; Conde et al., in press) and TCS (Schutter, 2016; Herring et al., 2018) is therefore a core developmental goal for the near future.
BSDBS is still in its very early stage, and many exciting applications yet remain to be uncovered. Importantly, to exploit the full potential of this novel technique, it needs to be applied in a hypothesis-driven manner, with a decent neurophysiological understanding of the target brain state, and carefully adapted to the research question at hand. It should not be considered as a new standard formula to improve any NIBS setup, but as an important step toward a higher degree of flexibility, specificity, and precision in NIBS.
Author Contributions
The author confirms being the sole contributor of this work and has approved it for publication.
Conflict of Interest Statement
The author declares that the research was conducted in the absence of any commercial or financial relationships that could be construed as a potential conflict of interest.
Acknowledgments
This publication was supported by the Deutsche Forschungsgemeinschaft (DFG, grant no. 362546008 to TB) and the Open Access Publishing Fund of the University of Tübingen.
References
Bergmann, T. O., and Born, J. (2018). Phase-amplitude coupling: a general mechanism for memory processing and synaptic plasticity? Neuron 97, 10–13. doi: 10.1016/j.neuron.2017.12.023
Bergmann, T. O., Karabanov, A., Hartwigsen, G., Thielscher, A., and Siebner, H. R. (2016). Combining non-invasive transcranial brain stimulation with neuroimaging and electrophysiology: current approaches and future perspectives. Neuroimage 140, 4–19. doi: 10.1016/j.neuroimage.2016.02.012
Bergmann, T. O., Mölle, M., Diedrichs, J., Born, J., and Siebner, H. R. (2012a). Sleep spindle-related reactivation of category-specific cortical regions after learning face-scene associations. Neuroimage 59, 2733–2742. doi: 10.1016/j.neuroimage.2011.10.036
Bergmann, T. O., Mölle, M., Schmidt, M. A., Lindner, C., Marshall, L., Born, J., et al. (2012b). EEG-guided transcranial magnetic stimulation reveals rapid shifts in motor cortical excitability during the human sleep slow oscillation. J. Neurosci. 32, 243–253. doi: 10.1523/JNEUROSCI.4792-11.2012
Brittain, J. S., Probert-Smith, P., Aziz, T. Z., and Brown, P. (2013). Tremor suppression by rhythmic transcranial current stimulation. Curr. Biol. 23, 436–440. doi: 10.1016/j.cub.2013.01.068
Buzsáki, G., and Draguhn, A. (2004). Neuronal oscillations in cortical networks. Science 304, 1926–1929. doi: 10.1126/science.1099745
Conde, V., Tomasevic, L., Akopian, I., Stanek, K., Saturnino, G.B., Thielscher, A., et al. (in press). The non-transcranial TMS-evoked potential is an inherent source of ambiguity in TMS-EEG studies. Neuroimage doi: 10.1016/j.neuroimage.2018.10.052.
Habibollahi Saatlou, F., Rogasch, N. C., McNair, N. A., Biabani, M., Pillen, S. D., Marshall, T. R., et al. (2018). MAGIC: an open-source MATLAB toolbox for external control of transcranial magnetic stimulation devices. Brain Stimul. 11, 1189–1191. doi: 10.1016/j.brs.2018.05.015
Harquel, S., Diard, J., Raffin, E., Passera, B., Dall'Igna, G., Marendaz, C., et al. (2017). Automatized set-up procedure for transcranial magnetic stimulation protocols. Neuroimage 153, 307–318. doi: 10.1016/j.neuroimage.2017.04.001
Harris, K. D. (2013). Top-down control of cortical state. Neuron 79, 408–410. doi: 10.1016/j.neuron.2013.07.034
Herring, J. D., Esterer, S., Marshall, T. R., Jensen, O., and Bergmann, T. O. (2018). Low-frequency alternating current stimulation rhythmically suppresses gamma-band oscillations and impairs perceptual performance. Neuroimage 184, 440–449. doi: 10.1016/j.neuroimage.2018.09.047
Herring, J. D., Thut, G., Jensen, O., and Bergmann, T. O. (2015). Attention modulates TMS-locked alpha oscillations in the visual cortex. J. Neurosci. 35, 14435–14447. doi: 10.1523/JNEUROSCI.1833-15.2015
Huerta, P. T., and Lisman, J. E. (1995). Bidirectional synaptic plasticity induced by a single burst during cholinergic theta oscillation in CA1 in vitro. Neuron 15, 1053–1063. doi: 10.1016/0896-6273(95)90094-2
Huerta, P. T., and Lisman, J. E. (1996). Low-frequency stimulation at the troughs of theta-oscillation induces long-term depression of previously potentiated CA1 synapses. J. Neurophysiol. 75, 877–884. doi: 10.1152/jn.1996.75.2.877
Jensen, O., Gips, B., Bergmann, T. O., and Bonnefond, M. (2014). Temporal coding organized by coupled alpha and gamma oscillations prioritize visual processing. Trends Neurosci. 37, 357–369. doi: 10.1016/j.tins.2014.04.001
Karabanov, A., Thielscher, A., and Siebner, H. R. (2016). Transcranial brain stimulation: closing the loop between brain and stimulation. Curr. Opin. Neurol. 29, 397–404. doi: 10.1097/WCO.0000000000000342
Karabanov, A., Ziemann, U., Hamada, M., George, M. S., Quartarone, A., Classen, J., et al. (2015). Consensus paper: probing homeostatic plasticity of human cortex with non-invasive transcranial brain stimulation. Brain Stimul. 8, 442–454. doi: 10.1016/j.brs.2015.01.404
Ketz, N., Jones, A. P., Bryant, N. B., Clark, V. P., and Pilly, P. K. (2018). Closed-loop slow-wave tACS improves sleep-dependent long-term memory generalization by modulating endogenous oscillations. J. Neurosci. 38, 7314–7326. doi: 10.1523/JNEUROSCI.0273-18.2018
Koponen, L. M., Nieminen, J. O., and Ilmoniemi, R. J. (2018). Multi-locus transcranial magnetic stimulation-theory and implementation. Brain Stimul. 11, 849–855. doi: 10.1016/j.brs.2018.03.014
Lee, S. H., and Dan, Y. (2012). Neuromodulation of brain States. Neuron 76, 209–222. doi: 10.1016/j.neuron.2012.09.012
Lustenberger, C., Boyle, M. R., Alagapan, S., Mellin, J. M., Vaughn, B. V., and Fröhlich, F. (2016). Feedback-controlled transcranial alternating current stimulation reveals a functional role of sleep spindles in motor memory consolidation. Curr. Biol. 26, 2127–2136. doi: 10.1016/j.cub.2016.06.044
Ngo, H. V., Martinetz, T., Born, J., and Mölle, M. (2013). Auditory closed-loop stimulation of the sleep slow oscillation enhances memory. Neuron 78, 545–553. doi: 10.1016/j.neuron.2013.03.006
Rogasch, N. C., Sullivan, C., Thomson, R. H., Rose, N. S., Bailey, N. W., Fitzgerald, P. B., et al. (2017). Analysing concurrent transcranial magnetic stimulation and electroencephalographic data: a review and introduction to the open-source TESA software. Neuroimage 147, 934–951. doi: 10.1016/j.neuroimage.2016.10.031
Sadaghiani, S., Hesselmann, G., Friston, K. J., and Kleinschmidt, A. (2010). The relation of ongoing brain activity, evoked neural responses, and cognition. Front. Syst. Neurosci. 4:20. doi: 10.3389/fnsys.2010.00020
Schroeder, C. E., and Lakatos, P. (2009). Low-frequency neuronal oscillations as instruments of sensory selection. Trends Neurosci. 32, 9–18. doi: 10.1016/j.tins.2008.09.012
Schutter, D. J. (2016). Cutaneous retinal activation and neural entrainment in transcranial alternating current stimulation: a systematic review. Neuroimage 140, 83–88. doi: 10.1016/j.neuroimage.2015.09.067
Silvanto, J., Muggleton, N., and Walsh, V. (2008). State-dependency in brain stimulation studies of perception and cognition. Trends Cogn. Sci. 12, 447–454. doi: 10.1016/j.tics.2008.09.004
Staresina, B. P., Bergmann, T. O., Bonnefond, M., van der Meij, R., Jensen, O., Deuker, L., et al. (2015). Hierarchical nesting of slow oscillations, spindles and ripples in the human hippocampus during sleep. Nat. Neurosci. 18, 1679–1686. doi: 10.1038/nn.4119
Thies, M., Zrenner, C., Ziemann, U., and Bergmann, T. O. (2018). Sensorimotor mu-alpha power is positively related to corticospinal excitability. Brain Stimul. 11, 1119–1122. doi: 10.1016/j.brs.2018.06.006
Thut, G., Bergmann, T. O., Fröhlich, F., Soekadar, S. R., Brittain, J. S., Valero-Cabré, A., et al. (2017). Guiding transcranial brain stimulation by EEG/MEG to interact with ongoing brain activity and associated functions: a position paper. Clin. Neurophysiol. 128, 843–857. doi: 10.1016/j.clinph.2017.01.003
VanRullen, R., and Koch, C. (2003). Is perception discrete or continuous? Trends Cogn. Sci. 7, 207–213. doi: 10.1016/S1364-6613(03)00095-0
Walter, A., Ramos Murguialday, A., Rosenstiel, W., Birbaumer, N., and Bogdan, M. (2012). Coupling BCI and cortical stimulation for brain-state-dependent stimulation: methods for spectral estimation in the presence of stimulation after-effects. Front. Neural Circuits 6:87. doi: 10.3389/fncir.2012.00087
Zagha, E., and McCormick, D. A. (2014). Neural control of brain state. Curr. Opin. Neurobiol. 29, 178–186. doi: 10.1016/j.conb.2014.09.010
Ziemann, U., and Siebner, H. R. (2008). Modifying motor learning through gating and homeostatic metaplasticity. Brain Stimul. 1, 60–66. doi: 10.1016/j.brs.2007.08.003
Ziemann, U., and Siebner, H. R. (2015). Inter-subject and inter-session variability of plasticity induction by non-invasive brain stimulation: boon or bane? Brain Stimul. 8, 662–663. doi: 10.1016/j.brs.2015.01.409
Zrenner, C., Belardinelli, P., Müller-Dahlhaus, F., and Ziemann, U. (2016). Closed-loop neuroscience and non-invasive brain stimulation: a tale of two loops. Front. Cell. Neurosci. 10:92. doi: 10.3389/fncel.2016.00092
Keywords: transcrancial magnetic stimulation (TMS), transcranial alternating current stimulation (tACS), transcranial direct current stimulation (tDCS), real-time, EEG
Citation: Bergmann TO (2018) Brain State-Dependent Brain Stimulation. Front. Psychol. 9:2108. doi: 10.3389/fpsyg.2018.02108
Received: 10 September 2018; Accepted: 12 October 2018;
Published: 01 November 2018.
Edited by:
Juha Silvanto, University of Westminster, United KingdomReviewed by:
Domenica Veniero, University of Glasgow, United KingdomCopyright © 2018 Bergmann. This is an open-access article distributed under the terms of the Creative Commons Attribution License (CC BY). The use, distribution or reproduction in other forums is permitted, provided the original author(s) and the copyright owner(s) are credited and that the original publication in this journal is cited, in accordance with accepted academic practice. No use, distribution or reproduction is permitted which does not comply with these terms.
*Correspondence: Til O. Bergmann, dGlsLmJlcmdtYW5uQHVuaS10dWViaW5nZW4uZGU=