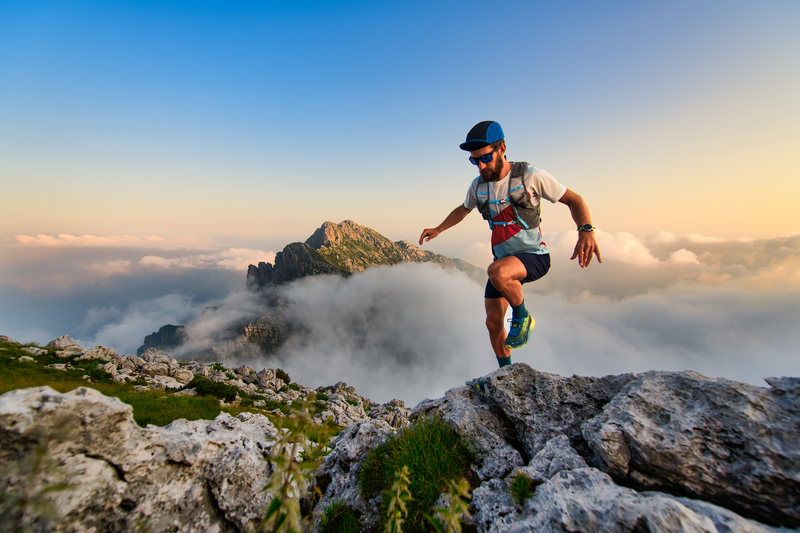
94% of researchers rate our articles as excellent or good
Learn more about the work of our research integrity team to safeguard the quality of each article we publish.
Find out more
OPINION article
Front. Photonics , 07 February 2025
Sec. Biophotonics
Volume 6 - 2025 | https://doi.org/10.3389/fphot.2025.1535133
Historically, dermatological diagnosis and treatment planning have relied on subjective observations and basic imaging techniques. While these approaches have yielded significant advancements, their limitations are increasingly evident in a world moving toward precision medicine (Seck et al., 2020). Two-dimensional imaging fails to capture the skin’s complex topography and depth, often leading to a mono-dimensional understanding of multifaceted conditions (Xu et al., 2019; Yew et al., 2014). This gap is now being addressed by the advent of 3D skin mapping technology. Using innovative imaging techniques, 3D skin mapping generates high-resolution, three-dimensional models of the skin, offering a detailed representation of surface and subsurface features (Gevaux et al., 2019). This technology facilitates a deeper understanding of individual skin characteristics, allowing for highly personalized treatment strategies. Beyond its transformative impact in medical dermatology, 3D mapping is also gaining prominence in aesthetic applications, where precision and patient satisfaction are equally critical.
At its core, 3D skin mapping utilizes advanced imaging technologies such as structured light scanning, photogrammetry and laser-assisted imaging (Quinzi et al., 2022). These methods create intricate, multi-layered representations of the skin, capturing parameters such as texture, elasticity, pigmentation and vascularity, while incorporating depth and spatial relationships into the analysis (Gevaux et al., 2019). Dynamic 3D mapping tools go further by visualizing changes in skin morphology over time, providing valuable insights into the progression of conditions and the efficacy of treatments (Voegeli et al., 2019; Shaiek et al., 2023). For example, in acne scars, these tools measure scar depth and volume to assess improvements following treatments like subcision or fractional laser therapy (Salameh et al., 2022). Psoriasis patients benefit from detailed monitoring of lesion thickness and affected area distribution, facilitating objective evaluations of therapeutic response. Similarly, in melasma, 3D mapping quantifies pigmentation density and irregularities, enabling clinicians to customize laser parameters for optimal results (Kallipolitis et al., 2025; Gaurav et al., 2024). By quantitatively measuring parameters such as wrinkle depth or scar volume, 3D mapping enables dermatologists to track patient progress with scientific accuracy, moving beyond subjective descriptions to data-driven outcomes. Beyond these conditions, hyperspectral imaging combined with AI algorithms, enhances early detection and characterization of skin cancers like melanoma and basal cell carcinoma. By utilizing these advanced capabilities, clinicians can deliver highly targeted and effective treatments tailored to individual patients’ needs.
Two-dimensional (2D) imaging has been a foundational tool in dermatology, offering valuable insights into surface-level features such as pigmentation, texture, and vascular abnormalities. While effective for basic assessments, 2D mapping tools are inherently limited by their inability to capture depth, volume, and the spatial relationships of skin structures. This limitation often results in incomplete analyses, particularly for conditions that require a detailed understanding of skin morphology, such as scars, wrinkles, or deep pigmentation disorders (Cerminara et al., 2023).
In contrast, 3D skin mapping provides a comprehensive representation of the skin by integrating depth and spatial data into multi-layered models. These detailed visualizations enable dermatologists to quantify parameters such as wrinkle depth, scar volume, and pigmentation density, offering a level of precision unattainable with 2D imaging. For instance, while a 2D photograph can document pigmentation patterns, a 3D scan can measure the thickness and distribution of the pigment across multiple layers, facilitating more targeted treatment planning (Kurzyk et al., 2024). Furthermore, 3D mapping tools allow for dynamic tracking of changes over time, making them invaluable for monitoring treatment efficacy or the progression of chronic skin conditions. The ability to visualize improvements in three dimensions enhances clinical accuracy and improves patient communication and satisfaction by providing tangible evidence of progress (Bannister et al., 2023).
The applications of 3D skin mapping span the spectrum of dermatological practice, encompassing both medical and cosmetic domains (Fu et al., 2021; Fu et al., 2018). In medical dermatology, it is proving invaluable for diagnosing and managing chronic skin conditions such as acne scars, psoriasis and melasma. By offering a comprehensive understanding of the distribution and depth of affected areas, 3D mapping enhances the precision of interventions. For example, in excisions and biopsies, 3D imaging provides high-resolution visualizations of skin lesions, allowing clinicians to accurately define lesion margins and assess their depth. This precision ensures biopsies target the most diagnostically relevant areas and excisions are performed with clear boundaries, reducing the risk of incomplete removal while optimizing cosmetic outcomes (Selkin et al., 2001; Guitera et al., 2012). 3D skin mapping also plays a critical role in dermatologic surgery, providing detailed pre-operative insights into the structure, elasticity, and vascularity of the surrounding tissue. These insights help surgeons tailor their approaches for procedures such as tumor removal or skin grafting, minimizing scarring and ensuring natural results, particularly in cosmetically sensitive areas like the face. Post-operatively, 3D imaging facilitates precise monitoring of healing, scar formation, and recurrence, enhancing patient care and satisfaction (Breuninger and Adam, 2014).
In aesthetic medicine, where precision and natural outcomes are crucial, 3D skin mapping has become a cornerstone for personalized treatment. It enables clinicians to analyze facial symmetry, contour, and volume loss, creating bespoke plans for dermal fillers, botulinum toxin, and other interventions (Seck et al., 2020). Another example is the detailed evaluation of wrinkle depth which can guide the selection of treatment modalities, such as fractional lasers, radiofrequency devices or dermal fillers, ensuring precise application tailored to the severity and location of the wrinkles. Furthermore, 3D imaging can identify subtle asymmetries and imperfections that may not be apparent to the naked eye, empowering clinicians to refine their techniques and deliver superior results. Another emerging application is in laser-based treatments, where the success of procedures often hinges on precise targeting. 3D skin mapping can optimize laser settings based on the unique attributes of the skin, reducing the risk of complications such as post inflammatory hyperpigmentation (PIH), especially in patients with darker skin tones. Moreover, the technology enhances post-treatment evaluations, enabling clinicians to assess outcomes objectively and adjust protocols for subsequent sessions. As technology evolves, integration with artificial intelligence (AI) is poised to revolutionize the field further. AI-powered 3D skin analysis can provide predictive insights, helping clinicians forecast treatment outcomes with greater accuracy and refine their strategies in real time.
Additionally, the development of portable and cost-effective 3D imaging systems promises to make these advancements accessible to a broader range of practitioners, democratizing precision medicine in aesthetics. Furthermore, 3D skin mapping has demonstrated remarkable utility in patient education (Frommherz et al., 2023). By providing a visual representation of the patient’s skin condition and the potential benefits of treatment, 3D skin mapping also fosters better understanding and alignment of expectations. That is to say, patients who see their skin in three dimensions often feel more engaged and reassured, leading to higher satisfaction and compliance with treatment plans.
Recent studies demonstrate the efficacy of these modalities in skin cancer diagnostics. Lin et al. assessed the Spectrum-Aided Vision Enhancer (SAVE) for detecting melanoma subtypes, achieving high sensitivity and specificity. This study focused on four melanoma types, acral lentiginous melanoma, melanoma in situ, nodular melanoma, and superficial spreading melanoma, using spectral information to enhance visualization and diagnostic accuracy. The researchers highlighted SAVE’s ability to detect early-stage melanomas that may otherwise evade traditional imaging techniques (Lin et al., 2024). Similarly, Huang et al. employed hyperspectral imaging combined with YOLOv5, a state-of-the-art machine learning algorithm, for automated skin cancer classification. This study demonstrated the robustness of combining imaging technology with AI by accurately categorizing various types of skin cancer. The integration of YOLOv5 enabled real-time image processing, offering clinicians a highly efficient diagnostic tool. The findings underscored the potential for hyperspectral imaging in routine clinical workflows, particularly for screening high-risk populations (Huang et al., 2023). The broader implications of these findings are reflected in reviews such as those by Lu, Parasca, and Dhawan et al., which highlighted the potential of hyperspectral imaging in automated tumor classification, demonstrating improved diagnostic accuracy in skin cancer detection (Lu and Fei, 2014; Parasca et al., 2024; Dhawan et al., 2009). These contributions collectively underscore the transformative potential of hyperspectral and multispectral imaging in clinical and research settings.
These modalities complement 3D mapping by offering additional layers of diagnostic precision, particularly in detecting subtle changes in pigmentation and vascularity. Their ability to analyze spectral signatures provides a more nuanced understanding of skin conditions, making them valuable tools for both research and clinical practice.
Several advanced 3D skin mapping devices are available on the market, offering cutting-edge imaging solutions for clinical and aesthetic dermatology. The VECTRA WB360 Imaging System by Canfield Scientific captures the entire skin surface in high resolution in a single shot, making it ideal for monitoring pigmented lesions and dispersed skin diseases (Marchetti et al., 2023). Similarly, the 3D Next MP Series provides total-body mapping, creating a 3D avatar for detecting skin cancer and other disorders, offering detailed insights within seconds (Cerminara et al., 2023). For facial analysis, the LifeViz® Mini by QuantifiCare is a portable and precise tool widely used in aesthetic practices, while the VISIA® Skin Analysis System excels in comprehensive skin assessments, capturing high-resolution images to analyze features such as texture, pigmentation, and vascularity (Goldie et al., 2021; Salti et al., 2023). These devices highlight the integration of advanced imaging technologies into dermatology, enhancing diagnostic accuracy and enabling highly personalized treatment plans significantly. Adding to these tools, Line-field Confocal Optical Coherence Tomography (LC-OCT) represents a significant advancement in non-invasive imaging technology. By combining optical coherence tomography and confocal microscopy, LC-OCT delivers quasi-isotropic spatial resolution at the cellular level, enabling detailed in-vivo visualization of the skin’s structure (Jdid et al., 2024). LC-OCT is particularly effective in diagnosing and monitoring conditions such as basal cell carcinoma, where its ability to differentiate malignant lesions from benign growths offers clinicians an unparalleled level of precision (Michielon et al., 2024). Moreover, LC-OCT has proven valuable in assessing inflammatory skin diseases, pigmentation disorders, and even treatment responses, expanding its utility further in both clinical and aesthetic dermatology. Together, these advanced imaging tools underline the transformative role of three-dimensional graphing of the skin in enhancing diagnostic accuracy, treatment planning, and overall patient outcomes.
A prime example of clinical utility of 3D skin mapping is wrinkle analysis and treatment optimization. In patients seeking anti-aging treatments, the technology provides precise measurements of wrinkle depth, length, and distribution across the face (Matias et al., 2015). For instance, a patient depicting pronounced nasolabial folds and periorbital fine lines may undergo a 3D scan to quantify these features. This data can guide the selection of energy-based device parameters, such as fractional laser or radiofrequency microneedling settings, ensuring that energy is concentrated on deeper wrinkles while avoiding unnecessary treatment of unaffected areas. Over successive sessions, the same technology allows clinicians to track reductions in wrinkle depth and improvements in skin texture, providing objective data to evaluate treatment efficacy. In the case of melasma, where pigmentation patterns are irregular and diffused, 3D skin mapping offers a detailed visualization of melanin distribution across the skin (Kurzyk et al., 2024; Ahmedt-Aristizabal et al., 2023; Ghose et al., 2023). This feature could enable clinicians to develop laser treatment protocols optimized for each patient’s pigmentation density and depth. For example, a dermatologist could use 3D imaging to adjust the parameters of a picosecond laser for a patient with melasma on their cheeks, ensuring safe and effective treatment with minimal risk of PIH. In aesthetic medicine, 3D skin mapping is revolutionizing procedures such as dermal filler treatments. Consider a patient seeking midface volume restoration. While traditional methods rely on manual palpation and subjective visual assessment, 3D imaging allows the clinician to visualize the loss of volume in the cheeks and nasolabial folds in detail. Using this data, the injector can plan precise filler placement to restore volume symmetrically (Musolff et al., 2024). Post-treatment scans provide immediate feedback, allowing the patient to see the subtle yet impactful changes achieved. Another compelling example is skin tightening procedures, such as those using ultrasound or radiofrequency devices, which could also benefit from 3D mapping. For instance, a patient undergoing treatment for lower face laxity might have their skin’s elasticity and collagen density measured pre-treatment. These baseline images guide the clinician in selecting appropriate treatment parameters. Follow-up scans, taken months later, objectively demonstrate improvements in skin tightness, helping to quantify treatment outcomes and reinforce patient satisfaction (Hua et al., 2018). In patients requiring correction from previous mal-conducted aesthetic procedures, 3D devices capacitate clinicians to spot clear-cut scope of the damage, thus exact solutions to these complications.
Along with this, LC-OCT has emerged as a promising non-invasive imaging modality, with studies demonstrating its efficacy in various dermatological and systemic applications (Musolff et al., 2024). Literature reports highlight its high sensitivity and specificity in the diagnosis of skin cancers, including melanoma and basal cell carcinoma through detailed visualization of the dermo-epidermal junction and cellular morphology (Latriglia et al., 2023; Perez-Anker et al., 2024). In Assi et al.'s studies, LC-OCT, combined with deep learning, enabled precise quantification of facial dermal aging in healthy Caucasian females (Assi et al., 2024; Ali et al., 2024). The imaging technique captured key markers of aging, offering objective and reproducible measurements that could enhance diagnostics and treatment monitoring. In inflammatory skin diseases, such as psoriasis and eczema, LC-OCT has been shown to accurately depict epidermal thickening, vascular changes, and inflammatory infiltrates, facilitating precise disease staging and treatment monitoring (Cappilli et al., 2024). Additionally, research has underscored its potential in detecting early microvascular changes associated with systemic conditions like lupus and diabetes (Ferreira et al., 2023). In aesthetic dermatology, LC-OCT has also been used to assess structural changes post-treatment, such as collagen remodeling and epidermal texture improvements following laser therapy or injectable procedures (Dryżałowska et al., 2024). By providing detailed, real-time insights into these changes, LC-OCT demonstrates its capacity to guide and refine treatment protocols. Therefore, LC-OCT is a cutting-edge imaging tool that enables highly personalized treatment strategies in aesthetic and dermatological care. By quantifying markers such as collagen density, elastin integrity, and dermal thickness, LC-OCT provides clinicians with precise, patient-specific insights into the aging process. These detailed assessments allow for tailored interventions that address the unique skin characteristics of each individual. For example, patients with thinning dermal layers or decreased skin density may be addressed with injectable biostimulators or dermal fillers, optimizing placement to achieve natural, balanced results. The ability to map these aging markers ensures treatments are neither overtreating nor underperforming.
These findings underscore the versatility of LC-OCT, offering a reliable, real-time, and non-invasive solution for diagnosis and treatment monitoring across a broad spectrum of clinical applications.
One of the most practical benefits of 3D skin mapping lies in its ability to engage patients and monitor their progress over time. By offering a detailed, three-dimensional visualization of their skin, patients gain a clearer understanding of their conditions and the potential benefits of proposed treatments. This transparency builds trust as areas of concern and projected outcomes are made tangible, fostering confidence in the treatment process. For example, a patient undergoing anti-aging treatments such as fractional lasers or chemical peels can see before-and-after images that objectively highlight changes in wrinkle depth, skin texture, and pigmentation. Another key advantage is that it is a non-invasive and painless method, ensuring patient comfort and making it ideal for sensitive skin conditions and regular follow-ups. This blend of precision, visual clarity, and comfort not only enhances satisfaction, but also empowers patients to actively engage in their care (Shaiek et al., 2023). For patients, 3D models offer an unparalleled educational tool, offering a deeper understanding of their treatment journey (Fuentes et al., 2020). A patient considering botulinum toxin for forehead lines may feel uncertain about the procedure. By showing them a 3D model of their current wrinkles and demonstrating how the treatment will affect the underlying musculature, clinicians can provide a clearer picture of expected results, addressing concerns and managing expectations (Fuentes et al., 2020). By integrating 3D skin mapping into their practice, clinicians create a more engaging and reassuring experience for patients. This process would empower patients to make informed decisions and actively participate in their care, ultimately driving higher satisfaction and compliance.
The future of 3D skin mapping lies in its continued evolution and integration with complementary technologies. Advances in AI are set to enhance the capabilities of 3D imaging by automating analysis and identifying patterns that may elude human observation (Haykal, 2024a). For instance, AI algorithms could predict the likelihood of treatment success based on the unique attributes of the patient’s skin, paving the way for reliably predictive dermatology. This is a transformative development, as it moves dermatology from a reactive approach to a proactive one, allowing clinicians to select the most effective treatments tailored to individual needs (Haykal, 2024b). By integrating real-time data from imaging, AI can forecast outcomes such as wrinkle reduction, scar improvement, or pigmentation correction with remarkable accuracy, minimizing trial-and-error in treatment plans (Grech et al., 2024). AI’s impact is driven by advanced techniques like deep learning and machine learning models. Deep learning algorithms, especially convolutional neural networks (CNNs), excel at recognizing complex dermatological patterns in imaging data, such as early signs of melanoma or subtle pigmentation changes (Singh et al., 2024). Machine learning models analyze multi-dimensional patient data including age, skin type, and lesion characteristics to predict treatment responses and optimize protocols. Generative adversarial networks (GANs) simulate realistic “before-and-after” outcomes, improving patient communication and expectation management (Chan et al., 2020). These AI techniques not only refine diagnostic precision, but also enable dynamic adaptation of treatments based on real-time imaging and patient feedback. This synergy between AI and 3D imaging enables highly personalized treatment plans, more accurate monitoring of progress, and optimized protocols, ensuring superior and efficient patient care (Li et al., 2022).
Additionally, the development of portable, cost-effective devices is another promising avenue. As hardware becomes more compact and affordable, the barriers to entry will diminish, democratizing access to this technology (Balado et al., 2025). Such advancements could bring 3D skin mapping into the realm of primary care or remote dermatology, where its potential for improving diagnostic accuracy and patient outcomes is immense (Luo et al., 2023). Integration with wearable devices and other biometric tools could also expand the scope of 3D skin mapping (Haykal, 2024c). By combining imaging data with real-time metrics such as hydration levels, UV exposure, and temperature fluctuations, through which clinicians could gain a more holistic understanding of skin health. This multidimensional approach would enable the creation of highly personalized skincare regimens that address not only the current state of the skin, but also its dynamic responses to environmental and lifestyle factors.
While currently hypothetical, integrating 3D skin mapping with diagnostic tools like spectral imaging or biomarkers has the potential to 1 day support the early detection of systemic conditions with skin manifestations. For example, combining lesion imaging with systemic biomarkers might aid in monitoring diseases like lupus or diabetes. Although such applications are not yet realized, they underscore the potential of 3D skin mapping to advance personalized and multidisciplinary healthcare (Giavina-Bianchi and Ko, 2024).
Several 3D skin mapping devices have received regulatory approvals, such as FDA clearance in the United States and CE marking in Europe, demonstrating their safety and efficacy for clinical use. For instance, the VECTRA WB360 Imaging System by Canfield Scientific is FDA-cleared for clinical applications, including monitoring pigmented lesions and early detection of skin cancer (VECTRA® WB360s, 2024). Similarly, the VISIA Skin Analysis System, which is both FDA-approved and CE-marked, is widely used in aesthetic practices for comprehensive facial assessments, analyzing features like wrinkles, pigmentation, and vascularity (Canfieldsci, 2024). The LC-OCT device is CE-marked in Europe and has been validated in clinical studies for non-invasive imaging of basal cell carcinoma and other dermatological conditions (Musolff et al., 2024). These regulatory certifications highlight the reliability and clinical relevance of 3D skin mapping technologies, enabling their adoption in both medical and aesthetic dermatology.
Despite its transformative potential, the integration of 3D skin mapping into routine dermatological practice faces several obstacles. One of the primary challenges is the high cost of technology. Advanced imaging systems, such as the VECTRA WB360, can cost upwards of €70,000, with additional expenses for maintenance, software updates, and staff training (Singh et al., 2024). These costs are often prohibitive for smaller clinics or practitioners in resource-limited settings. Clinical trials also encounter financial barriers, as they require multiple devices for different sites, skilled operators, and secure storage for the large datasets generated. Such expenses strain research budgets, particularly in smaller-scale studies, limiting the broader validation of 3D skin mapping in diverse populations (Ahmed et al., 2023). Another significant barrier is the lack of standardization in imaging protocols and data interpretation (Kukk et al., 2024). Different devices rely on varying algorithms and methodologies, leading to inconsistencies in results across practices and multi-centered studies. This variability undermines the reliability of 3D skin mapping as a universal tool and complicates collaborative decision-making. Additionally, data management poses logistical challenges. The detailed models generated by these systems produce large datasets that require secure storage and efficient retrieval systems. In an era where data privacy is a growing concern, ensuring the protection of patient information is paramount (Paul et al., 2023). For example, while maintaining records of a patient’s skin morphology enables clinicians to assess condition progression or treatment efficacy, robust data storage solutions are essential. Moreover, limited awareness among practitioners often leads to underutilization, while insufficient clinical validation for certain applications reduces confidence in its broader use. Integration into existing workflows can be challenging due to the time required for imaging, analysis, and staff training (Mennella et al., 2024).
Moreover, the deployment of AI and machine learning models in dermatology raises critical ethical considerations. Patient privacy and data security are paramount, especially given the sensitivity of dermatological imaging data. Compliance with regulations like the General Data Protection Regulation (GDPR) is essential to ensure ethical data handling (Carmi et al., 2023). Transparency in AI decision-making processes is another critical aspect. Patients and clinicians should be informed about how algorithms generate recommendations to foster trust and accountability. Furthermore, the underrepresentation of diverse skin tones and conditions in training datasets poses a risk of exacerbating healthcare disparities, highlighting the need for inclusive data collection to create equitable and effective models. Informed consent for data use and the education of patients and clinicians about AI’s capabilities and limitations are critical to prevent misconceptions and over-reliance. Ensuring diverse representation in training datasets is vital to create models that are effective across varied skin types and conditions (Daneshjou and Kittler, 2024). Ethical frameworks must prioritize fairness, inclusivity, and the mitigation of biases to achieve truly equitable care.
3D skin mapping is more than just an imaging tool; it signifies a transformative shift toward precision and personalization in dermatology. By offering detailed, objective insights into the skin’s structure and condition, this technology enables clinicians to assess individual variations in skin health, from pigmentation and texture to vascular structures and volume loss. These insights empower practitioners to deliver targeted, customized treatments that address the unique concerns and aesthetic goals of each patient, enhancing both clinical outcomes and patient satisfaction. Another potential asset of adopting 3D imaging in dermatology is its possibility to integrate with AI, therefore laying firm grounds for clinicians and patients’ education. However, challenges remain, particularly in areas such as the high cost of attaining and maintaining 3D imaging devices, the need for specialized training, and the lack of standardized protocols for data storage and interpretation. Additionally, future research must prioritize reducing costs, standardizing imaging protocols, and validating the technology across diverse populations. Expanding integration with AI, conducting longitudinal studies on chronic skin conditions, and improving workflow optimization are also critical steps toward seamless clinical adoption. Overcoming these barriers requires collaborative efforts among researchers, clinicians, and manufacturers to deem the technology more accessible and user-friendly. Despite these challenges, the potential benefits outweigh the obstacles by far, as 3D skin mapping stands poised to redefine diagnostics, treatment planning, and follow-up care.
DH: Conceptualization, Data curation, Formal Analysis, Funding acquisition, Investigation, Methodology, Project administration, Resources, Software, Supervision, Validation, Visualization, Writing–original draft, Writing–review and editing.
The author(s) declare that no financial support was received for the research, authorship, and/or publication of this article.
The author declares that the research was conducted in the absence of any commercial or financial relationships that could be construed as a potential conflict of interest.
The author(s) declare that no Generative AI was used in the creation of this manuscript.
All claims expressed in this article are solely those of the authors and do not necessarily represent those of their affiliated organizations, or those of the publisher, the editors and the reviewers. Any product that may be evaluated in this article, or claim that may be made by its manufacturer, is not guaranteed or endorsed by the publisher.
Ahmed, M. I., Spooner, B., Isherwood, J., Lane, M., Orrock, E., and Dennison, A. (2023). A systematic review of the barriers to the implementation of artificial intelligence in healthcare. Cureus 15 (10), e46454. doi:10.7759/cureus.46454
Ahmedt-Aristizabal, D., Nguyen, C., Tychsen-Smith, L., Stacey, A., Li, S., Pathikulangara, J., et al. (2023). Monitoring of pigmented skin lesions using 3D whole body imaging. Comput. Methods Programs Biomed. 232, 107451. doi:10.1016/j.cmpb.2023.107451
Ali, A., Colombe, L., Mélanie, P., Agnes, P., Meryem, N., Samuel, R., et al. (2024). Comparison of facial skin ageing in healthy Asian and Caucasian females quantified by in vivo line-field confocal optical coherence tomography 3D imaging. Skin. Res. Technol. Off. J. Int. Soc. Bioeng. Skin. ISBS Int. Soc. Digit. Imaging Skin. ISDIS Int. Soc. Skin. Imaging ISSI 30 (9), e13643. doi:10.1111/srt.13643
Assi, A., Fischman, S., Lopez, C., Pedrazzani, M., Grignon, G., Missodey, R., et al. (2024). Evaluating facial dermis aging in healthy Caucasian females with LC-OCT and deep learning. Sci. Rep. 14 (1), 24113. doi:10.1038/s41598-024-74370-z
Balado, J., Garozzo, R., Winiwarter, L., and Tilon, S. (2025). A systematic literature review of low-cost 3D mapping solutions. Inf. Fusion 114, 102656. doi:10.1016/j.inffus.2024.102656
Bannister, J. J., Wilms, M., Aponte, J. D., Katz, D. C., Klein, O. D., Bernier, F. P., et al. (2023). Comparing 2D and 3D representations for face-based genetic syndrome diagnosis. Eur. J. Hum. Genet. 31 (9), 1010–1016. doi:10.1038/s41431-023-01308-w
Breuninger, H., and Adam, P. (2014). 3D Histology Eval. Dermatologic Surg., 133. doi:10.1007/978-1-4471-4438-0
Canfieldsci (2024). VISIA skin analysis | Canfield scientific. Available at: https://www.canfieldsci.com/imaging-systems/visia-complexion-analysis/(Accessed November 18, 2024).
Cappilli, S., Paradisi, A., Di Stefani, A., Palmisano, G., Pellegrino, L., D’Onghia, M., et al. (2024). Line-field confocal optical coherence tomography: a new skin imaging technique reproducing a “virtual biopsy” with evolving clinical applications in dermatology. Diagnostics 14 (16), 1821. doi:10.3390/diagnostics14161821
Carmi, L., Zohar, M., and Riva, G. M. (2023). The European General Data Protection Regulation (GDPR) in mHealth: theoretical and practical aspects for practitioners’ use. Med. Sci. Law 63 (1), 61–68. doi:10.1177/00258024221118411
Cerminara, S. E., Cheng, P., Kostner, L., Huber, S., Kunz, M., Maul, J. T., et al. (2023). Diagnostic performance of augmented intelligence with 2D and 3D total body photography and convolutional neural networks in a high-risk population for melanoma under real-world conditions: a new era of skin cancer screening? Eur. J. Cancer 190, 112954. doi:10.1016/j.ejca.2023.112954
Chan, S., Reddy, V., Myers, B., Thibodeaux, Q., Brownstone, N., and Liao, W. (2020). Machine learning in dermatology: current applications, opportunities, and limitations. Dermatol Ther. 10 (3), 365–386. doi:10.1007/s13555-020-00372-0
Daneshjou, R., and Kittler, H. (2024). Empowering the Next generation of artificial intelligence in dermatology: the datasets and benchmarks track of the journal of investigative dermatology. J. Invest. Dermatol 144 (3), 437–438. doi:10.1016/j.jid.2023.11.011
Dhawan, A. P., D’Alessandro, B., Patwardhan, S., and Mullani, N. (2009). Multispectral optical imaging of skin-lesions for detection of malignant melanomas. Annu. Int. Conf. IEEE Eng. Med. Biol. Soc. IEEE Eng. Med. Biol. Soc. Annu. Int. Conf. 2009, 5352–5355. doi:10.1109/IEMBS.2009.5334045
Dryżałowska, Z., Blicharz, L., Michalczyk, A., Koscian, J., Maj, M., Czuwara, J., et al. (2024). The usefulness of line-field confocal optical coherence tomography in monitoring epidermal changes in atopic dermatitis in response to treatment: a pilot study. Diagn Basel Switz. 14 (16), 1724. doi:10.3390/diagnostics14161724
Ferreira, A., Vieira, R. J., Furtado, M. J., Lume, M., Andrade, J. P., and Menéres, P. (2023). Detection of subclinical microvascular changes in systemic lupus erythematous using optical coherence tomography angiography: a systematic review and meta-analysis. Surv. Ophthalmol. 68 (6), 1115–1128. doi:10.1016/j.survophthal.2023.07.008
Frommherz, L., Hering, P., Stadler, P. C., Clanner-Engelshofen, B. M., and Reinholz, M. (2023). Silicone models for dermatological education: assessment of a new teaching tool by dermatologists. Dermatol Pract. Concept 13 (1), e2023049. doi:10.5826/dpc.1301a49
Fu, X., Cheong, Y. H., Ahamed, A., Zhou, C., Robert, C., Krikstolaityte, V., et al. (2021). Diagnostics of skin features through 3D skin mapping based on electro-controlled deposition of conducting polymers onto metal-sebum modified surfaces and their possible applications in skin treatment. Anal. Chim. Acta 1142, 84–98. doi:10.1016/j.aca.2020.10.056
Fu, X., Zeng, W., Ramírez-Pérez, A. C., and Lisak, G. (2018). 3-D and electrically conducting functional skin mapping for biomedical applications. Chem. Commun. Camb Engl. 54 (8), 980–983. doi:10.1039/c7cc09052h
Fuentes, S., Berlioz, M., Damián, F., Pradillos, J. M., Lorenzo, T., and Ardela, E. (2020). Patient and healthcare professional satisfaction with 3D imaging new technologies for medical purposes. Radiologia 62 (1), 46–50. doi:10.1016/j.rx.2019.06.001
Gaurav, V., Agrawal, S., Najeeb, A., Ahuja, R., Saurabh, S., and Gupta, S. (2024). Advancements in dermatological imaging modalities. Indian Dermatol Online J. 15 (2), 278–292. doi:10.4103/idoj.idoj_852_23
Gevaux, L., Adnet, C., and Seroul, P. (2019). Three-dimensional maps of human skin properties on full face with shadows using 3D-hyperspectral imaging. J. Biomed. Opt. 24, 1. doi:10.1117/1.JBO.24.6.066002
Ghose, S., Ju, Y., McDonough, E., Ho, J., Karunamurthy, A., Chadwick, C., et al. (2023). 3D reconstruction of skin and spatial mapping of immune cell density, vascular distance and effects of sun exposure and aging. Commun. Biol. 6 (1), 718–814. doi:10.1038/s42003-023-04991-z
Giavina-Bianchi, M., and Ko, J. (2024). Artificial intelligence in cutaneous lesions: where do we stand and what is Next? Front. Media SA. doi:10.3389/978-2-8325-4911-7
Goldie, K., Kerscher, M., Fabi, S. G., Hirano, C., Landau, M., Lim, T. S., et al. (2021). Skin quality – a holistic 360° view: consensus results. Clin. Cosmet. Investig. Dermatol 14, 643–654. doi:10.2147/CCID.S309374
Grech, V. S., Kefala, V., and Rallis, E. (2024). Cosmetology in the era of artificial intelligence. Cosmetics 11, 135. doi:10.3390/cosmetics11040135
Guitera, P., Menzies, S. W., Longo, C., Cesinaro, A. M., Scolyer, R. A., and Pellacani, G. (2012). In vivo confocal microscopy for diagnosis of melanoma and basal cell carcinoma using a two-step method: analysis of 710 consecutive clinically equivocal cases. J. Invest. Dermatol. 132 (10), 2386–2394. doi:10.1038/jid.2012.172
Haykal, D. (2024a). Emerging and pioneering AI technologies in aesthetic dermatology: sketching a path toward personalized, predictive, and proactive care. Cosmetics 11 (6), 206. doi:10.3390/cosmetics11060206
Haykal, D. (2024b). The suitability of AI in dermatology for enhanced skin care. J. Aesthetic Nurs. 13 (5), 240–251. doi:10.12968/joan.2024.0006
Haykal, D. (2024c). Unleashing the power of biosensors and artificial intelligence in dermatology. Aesthetic Surg. J. Open Forum 6, ojae030. doi:10.1093/asjof/ojae030
Hua, Q., Sun, J., Liu, H., Bao, R., Yu, R., Zhai, J., et al. (2018). Skin-inspired highly stretchable and conformable matrix networks for multifunctional sensing. Nat. Commun. 9 (1), 244. doi:10.1038/s41467-017-02685-9
Huang, H. Y., Hsiao, Y. P., Mukundan, A., Tsao, Y. M., Chang, W. Y., and Wang, H. C. (2023). Classification of skin cancer using novel hyperspectral imaging engineering via YOLOv5. J. Clin. Med. 12 (3), 1134. doi:10.3390/jcm12031134
Jdid, R., Pedrazzani, M., Lejeune, F., Fischman, S., Cazorla, G., Forestier, S., et al. (2024). Skin dark spot mapping and evaluation of brightening product efficacy using Line-field Confocal Optical Coherence Tomography (LC-OCT). Skin. Res. Technol. Off. J. Int. Soc. Bioeng. Skin. ISBS Int. Soc. Digit. Imaging Skin. ISDIS Int. Soc. Skin. Imaging ISSI 30 (2), e13623. doi:10.1111/srt.13623
Kallipolitis, A., Moutselos, K., Zafeiriou, A., Andreadis, S., Matonaki, A., Stavropoulos, T. G., et al. (2025). Skin image analysis for detection and quantitative assessment of dermatitis, vitiligo and alopecia areata lesions: a systematic literature review. BMC Med. Inf. Decis. Mak. 25 (1), 10. doi:10.1186/s12911-024-02843-2
Kukk, A. F., Scheling, F., Panzer, R., Emmert, S., and Roth, B. (2024). Non-invasive 3D imaging of human melanocytic lesions by combined ultrasound and photoacoustic tomography: a pilot study. Sci. Rep. 14 (1), 2768. doi:10.1038/s41598-024-53220-y
Kurzyk, A., Szumera-Ciećkiewicz, A., Miłoszewska, J., and Chechlińska, M. (2024). 3D modeling of normal skin and cutaneous squamous cell carcinoma. A comparative study in 2D cultures, spheroids, and 3D bioprinted systems. Biofabrication 16 (2), 025021. doi:10.1088/1758-5090/ad2b06
Latriglia, F., Ogien, J., Tavernier, C., Fischman, S., Suppa, M., Perrot, J. L., et al. (2023). Line-field confocal optical coherence tomography (LC-OCT) for skin imaging in dermatology. Life Basel Switz. 13 (12), 2268. doi:10.3390/life13122268
Li, Z., Koban, K. C., Schenck, T. L., Giunta, R. E., Li, Q., and Sun, Y. (2022). Artificial intelligence in dermatology image analysis: current developments and future trends. J. Clin. Med. 11 (22), 6826. doi:10.3390/jcm11226826
Lin, T. L., Lu, C. T., Karmakar, R., Nampalley, K., Mukundan, A., Hsiao, Y. P., et al. (2024). Assessing the efficacy of the spectrum-aided vision enhancer (SAVE) to detect acral lentiginous melanoma, melanoma in situ, nodular melanoma, and superficial spreading melanoma. Diagn Basel Switz. 14 (15), 1672. doi:10.3390/diagnostics14151672
Lu, G., and Fei, B. (2014). Medical hyperspectral imaging: a review. J. Biomed. Opt. 19 (1), 010901. doi:10.1117/1.JBO.19.1.010901
Luo, N., Zhong, X., Su, L., Cheng, Z., Ma, W., and Hao, P. (2023). Artificial intelligence-assisted dermatology diagnosis: from unimodal to multimodal. Comput. Biol. Med. 165, 107413. doi:10.1016/j.compbiomed.2023.107413
Marchetti, M. A., Nazir, Z. H., Nanda, J. K., Dusza, S. W., D'Alessandro, B. M., DeFazio, J., et al. (2023). 3D Whole-body skin imaging for automated melanoma detection. J. Eur. Acad. Dermatol Venereol. JEADV 37 (5), 945–950. doi:10.1111/jdv.18924
Matias, A. R., Ferreira, M., Costa, P., and Neto, P. (2015). Skin colour, skin redness and melanin biometric measurements: comparison study between Antera® 3D, Mexameter® and Colorimeter®. Skin. Res. Technol. Off. J. Int. Soc. Bioeng. Skin. ISBS Int. Soc. Digit. Imaging Skin. ISDIS Int. Soc. Skin. Imaging ISSI 21 (3), 346–362. doi:10.1111/srt.12199
Mennella, C., Maniscalco, U., De Pietro, G., and Esposito, M. (2024). Ethical and regulatory challenges of AI technologies in healthcare: a narrative review. Heliyon 10 (4), e26297. doi:10.1016/j.heliyon.2024.e26297
Michielon, E., Motta, A. C., Ogien, J., Oranje, P., Waaijman, T., Thakoersing, V., et al. (2024). Integration of line-field confocal optical coherence tomography and in situ microenvironmental mapping to investigate the living microenvironment of reconstructed human skin and melanoma models. J. Dermatol Sci. 115 (2), 85–93. doi:10.1016/j.jdermsci.2024.07.001
Musolff, N., Sanabria, B., Caetano, V., Pulgarin, L., Chaudhry, Z. S., and Rao, B. (2024). LC-OCT as a tool to visualize in vivo location of dermal fillers. Skin. Res. Technol. Off. J. Int. Soc. Bioeng. Skin. ISBS Int. Soc. Digit. Imaging Skin. ISDIS Int. Soc. Skin. Imaging ISSI 30 (8), e70014. doi:10.1111/srt.70014
Parasca, S. V., Calin, M. A., Manea, D., and Radvan, R. (2024). Hyperspectral imaging with machine learning for in vivo skin carcinoma margin assessment: a preliminary study. Phys. Eng. Sci. Med. 47 (3), 1141–1152. doi:10.1007/s13246-024-01435-8
Paul, M., Maglaras, L., Ferrag, M. A., and Almomani, I. (2023). Digitization of healthcare sector: a study on privacy and security concerns. ICT Express 9 (4), 571–588. doi:10.1016/j.icte.2023.02.007
Perez-Anker, J., Soglia, S., Lenoir, C., Albero, R., Alos, L., García, A., et al. (2024). Criteria for melanocytic lesions in LC-OCT. J. Eur. Acad. Dermatol Venereol. JEADV 38 (10), 2005–2016. doi:10.1111/jdv.20079
Quinzi, V., Polizzi, A., Ronsivalle, V., Santonocito, S., Conforte, C., Manenti, R. J., et al. (2022). Facial scanning accuracy with Stereophotogrammetry and Smartphone technology in children: a systematic review. Children 9 (9), 1390. doi:10.3390/children9091390
Salameh, F., Shehadeh, W., Sprecher, E., and Artzi, O. (2022). A stereoscopic optical system for objective quantification of the change in cumulative acne scar depth following various treatment interventions. J. Cosmet. Dermatol 21 (5), 2099–2105. doi:10.1111/jocd.14334
Salti, G., Siquier-Dameto, G., Rharbaoui, S., Hernandez Malgapo, D. M., Innocenti, S., and Manni, M. (2023). An interim 6-month analysis of the dermatologic effects and midface volume correction with XTRCL filler in a prospective, single-center study. Dermatol Surg. 49 (10), 943–948. doi:10.1097/DSS.0000000000003878
Seck, A., Dee, H., Smith, W., and Tiddeman, B. (2020). 3D surface texture analysis of high-resolution normal fields for facial skin condition assessment. Skin. Res. Technol. Off. J. Int. Soc. Bioeng. Skin. ISBS Int. Soc. Digit. Imaging Skin. ISDIS Int. Soc. Skin. Imaging ISSI 26 (2), 169–186. doi:10.1111/srt.12793
Selkin, B., Rajadhyaksha, M., Gonzalez, S., and Langley, R. G. (2001). In vivo CONFOCAL MICROSCOPY IN DERMATOLOGY. Dermatol Clin. 19 (2), 369–377. doi:10.1016/S0733-8635(05)70274-6
Shaiek, A., Monot, M., Rubert, V., Cornillon, C., Vicic, M., Flament, F., et al. (2023). In vitro and in vivo validation of a new three-dimensional fringe projection-based device (AEVA-HE) dedicated to skin surface mapping. Skin. Res. Technol. Off. J. Int. Soc. Bioeng. Skin. ISBS Int. Soc. Digit. Imaging Skin. ISDIS Int. Soc. Skin. Imaging ISSI 29 (2), e13209. doi:10.1111/srt.13209
Singh, P., Bornstein, M. M., Hsung, R. T. C., Ajmera, D. H., Leung, Y. Y., and Gu, M. (2024). Frontiers in three-dimensional surface imaging systems for 3D face acquisition in craniofacial research and practice: an updated literature review. Diagnostics 14 (4), 423. doi:10.3390/diagnostics14040423
VECTRA® WB360s (2024). Google my maps. Available at: https://www.google.com/maps/d/viewer?mid=1i4U0HOpPsKkd6mo5FYoN7NegIg4XqQtb (Accessed November 24, 2024).
Voegeli, R., Gierschendorf, J., Summers, B., and Rawlings, A. V. (2019). Facial skin mapping: from single point bio-instrumental evaluation to continuous visualization of skin hydration, barrier function, skin surface pH, and sebum in different ethnic skin types. Int. J. Cosmet. Sci. 41 (5), 411–424. doi:10.1111/ics.12562
Xu, W., Paidi, S. K., Qin, Z., Huang, Q., Yu, C. H., Pagaduan, J. V., et al. (2019). Self-folding hybrid graphene skin for 3D biosensing. Nano Lett. 19 (3), 1409–1417. doi:10.1021/acs.nanolett.8b03461
Keywords: 3D skin mapping, personalized medicine, advanced imaging, dermatological diagnosis, aesthetic medicine
Citation: Haykal D (2025) 3D skin mapping for personalized dermatological treatment. Front. Photonics 6:1535133. doi: 10.3389/fphot.2025.1535133
Received: 26 November 2024; Accepted: 21 January 2025;
Published: 07 February 2025.
Edited by:
Shuo Chen, Northeastern University, ChinaReviewed by:
Kamran Avanaki, University of Illinois Chicago, United StatesCopyright © 2025 Haykal. This is an open-access article distributed under the terms of the Creative Commons Attribution License (CC BY). The use, distribution or reproduction in other forums is permitted, provided the original author(s) and the copyright owner(s) are credited and that the original publication in this journal is cited, in accordance with accepted academic practice. No use, distribution or reproduction is permitted which does not comply with these terms.
*Correspondence: Diala Haykal, ZG9jdGV1ci5oYXlrYWxAZ21haWwuY29t
†ORCID: Diala Haykal, orcid.org/0000-0001-7528-5088
Disclaimer: All claims expressed in this article are solely those of the authors and do not necessarily represent those of their affiliated organizations, or those of the publisher, the editors and the reviewers. Any product that may be evaluated in this article or claim that may be made by its manufacturer is not guaranteed or endorsed by the publisher.
Research integrity at Frontiers
Learn more about the work of our research integrity team to safeguard the quality of each article we publish.