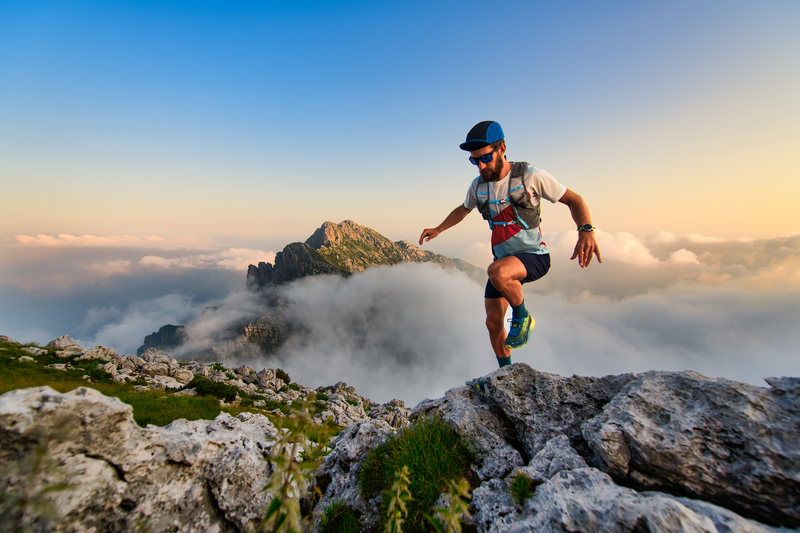
94% of researchers rate our articles as excellent or good
Learn more about the work of our research integrity team to safeguard the quality of each article we publish.
Find out more
ORIGINAL RESEARCH article
Front. Pharmacol.
Sec. Translational Pharmacology
Volume 16 - 2025 | doi: 10.3389/fphar.2025.1567544
This article is part of the Research Topic Emerging Horizons of Metformin: Exploring Recent Advances and Addressing Challenges in Research and Clinical Utilization View all 5 articles
The final, formatted version of the article will be published soon.
You have multiple emails registered with Frontiers:
Please enter your email address:
If you already have an account, please login
You don't have a Frontiers account ? You can register here
Background: Osteoarthritis (OA) and impaired glucose tolerance (IGT) frequently coexist, leading to compounded clinical and metabolic challenges. This study investigates the effects of metformin in improving both clinical outcomes (pain, stiffness, physical function) and metabolic parameters (inflammatory markers, lipid profile, BMI) in patients with knee OA and IGT.The study included 60 patients diagnosed with knee OA and IGT. Participants were divided into two groups: 26 patients received standard OA treatment without metformin (Without Metf), while 34 received metformin (500 mg twice daily) for three months, in addition to standard treatment (With Metf). Clinical assessments (WOMAC, Lequesne Algofunctional Index, KOOS, VAS) and metabolic markers (CRP, NLR, SOD, lipid profile, BMI) were measured before treatment, after one month, and after three months.The With Metf group showed significantly greater improvements in pain, stiffness, physical function, and quality of life compared to the Without Metf group. Metformin also led to significant reductions in inflammatory markers and improvements in lipid profiles and metabolic health indicators.The With Metf group demonstrated enhanced BMI, waist-to-hip ratio, and waist-to-height ratio.Furthermore, the need for increased NSAID doses was predicted by factors such as pain severity and inflammatory markers.Metformin effectively alleviates osteoarthritis symptoms and improves metabolic health in patients with both OA and IGT. Further research is needed to explore its long-term effects on joint health, inflammatory markers, and its potential role in OA management in patients without IGT.
Keywords: Osteoarthritis, Impaired glucose tolerance, Metformin, Inflammatory markers, disease-modifying therapy
Received: 27 Jan 2025; Accepted: 27 Feb 2025.
Copyright: © 2025 Halabitska, Petakh and Kamyshnyi. This is an open-access article distributed under the terms of the Creative Commons Attribution License (CC BY). The use, distribution or reproduction in other forums is permitted, provided the original author(s) or licensor are credited and that the original publication in this journal is cited, in accordance with accepted academic practice. No use, distribution or reproduction is permitted which does not comply with these terms.
* Correspondence:
Iryna Halabitska, Ternopil State Medical University, Ternopil, 46002, Ternopil Oblast, Ukraine
Disclaimer: All claims expressed in this article are solely those of the authors and do not necessarily represent those of their affiliated organizations, or those of the publisher, the editors and the reviewers. Any product that may be evaluated in this article or claim that may be made by its manufacturer is not guaranteed or endorsed by the publisher.
Research integrity at Frontiers
Learn more about the work of our research integrity team to safeguard the quality of each article we publish.