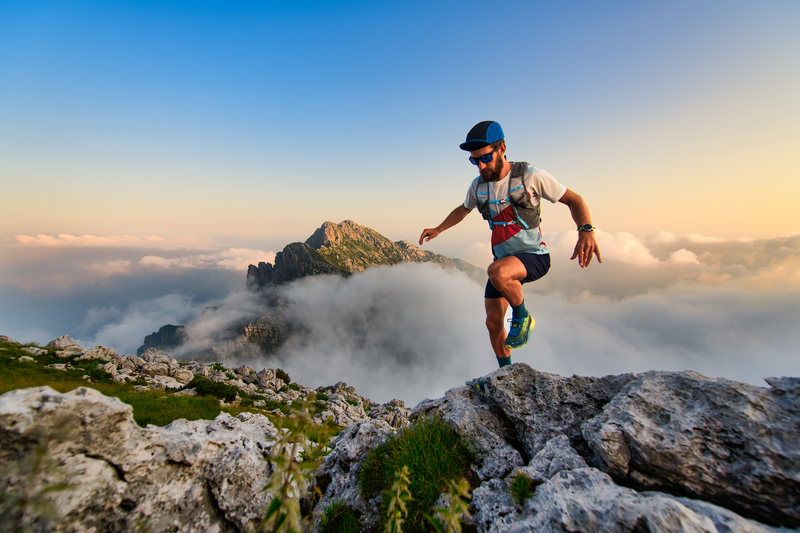
94% of researchers rate our articles as excellent or good
Learn more about the work of our research integrity team to safeguard the quality of each article we publish.
Find out more
ORIGINAL RESEARCH article
Front. Pharmacol.
Sec. Experimental Pharmacology and Drug Discovery
Volume 16 - 2025 | doi: 10.3389/fphar.2025.1565860
This article is part of the Research Topic Intelligent Computing for Integrating Multi-Omics Data in Disease Diagnosis and Drug Development View all articles
The final, formatted version of the article will be published soon.
You have multiple emails registered with Frontiers:
Please enter your email address:
If you already have an account, please login
You don't have a Frontiers account ? You can register here
Protein-protein interactions (PPIs) are critical for understanding the molecular mechanisms underlying various biological processes, particularly in microbes associated with cardiovascular disease. Traditional experimental methods for detecting PPIs are often time-consuming and costly, leading to an urgent need for reliable computational approaches. In this study, we present a novel model, the deep Denoising Autoencoder for Protein-Protein Interaction (DAEPPI), which leverages the denoising autoencoder and CatBoost algorithm to predict PPIs from the evolutionary information of protein sequences. Our extensive experiments demonstrate the effectiveness of the DAEPPI model, achieving average prediction accuracies of 97.85% and 98.49% on yeast and human datasets, respectively. Comparative analyses with existing effective methods further validate the robustness and reliability of our model in predicting PPIs. Additionally, we explore the application of DAEPPI in the context of cardiovascular disease, showcasing its potential to uncover significant interactions that could contribute to understanding disease mechanisms. Our findings indicate that DAEPPI is a powerful tool for advancing research in proteomics and could play a pivotal role in the identification of novel therapeutic targets in cardiovascular disease.
Keywords: protein-protein interactions, cardiovascular disease, Deep denoising autoencoder, CatBoost, Evolutionary information
Received: 23 Jan 2025; Accepted: 17 Feb 2025.
Copyright: © 2025 Li, Zhou, Luo, Tang, Li and He. This is an open-access article distributed under the terms of the Creative Commons Attribution License (CC BY). The use, distribution or reproduction in other forums is permitted, provided the original author(s) or licensor are credited and that the original publication in this journal is cited, in accordance with accepted academic practice. No use, distribution or reproduction is permitted which does not comply with these terms.
* Correspondence:
Yang Li, Hefei University of Technology, Hefei, China
Wenhua He, The Fourth Hospital of Changsha (Integrated Traditional Chinese and Western Medicine Hospital of Changsha, Changsha Hospital of Hunan Normal University), Changsha, China
Disclaimer: All claims expressed in this article are solely those of the authors and do not necessarily represent those of their affiliated organizations, or those of the publisher, the editors and the reviewers. Any product that may be evaluated in this article or claim that may be made by its manufacturer is not guaranteed or endorsed by the publisher.
Research integrity at Frontiers
Learn more about the work of our research integrity team to safeguard the quality of each article we publish.