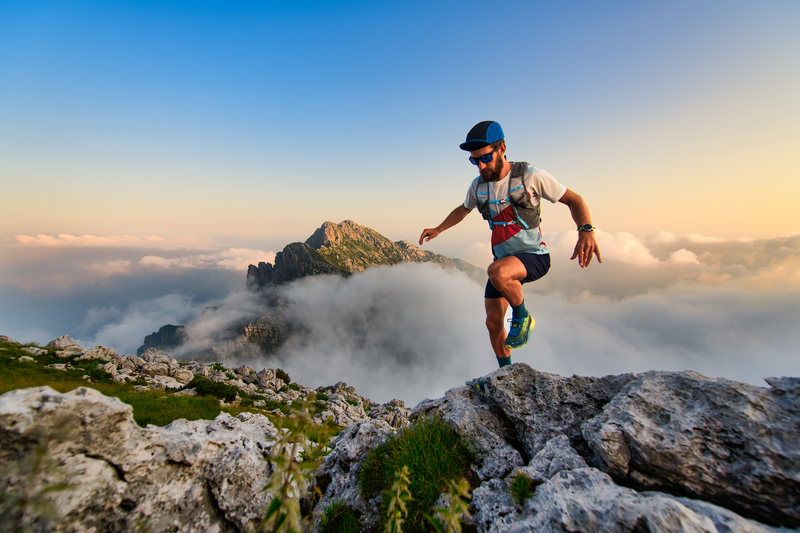
94% of researchers rate our articles as excellent or good
Learn more about the work of our research integrity team to safeguard the quality of each article we publish.
Find out more
ORIGINAL RESEARCH article
Front. Organ. Psychol. , 12 March 2025
Sec. Performance and Development
Volume 3 - 2025 | https://doi.org/10.3389/forgp.2025.1538438
This article is part of the Research Topic Affective and Behavioral Dynamics in Human-Technology Interactions of Industry 5.0 View all articles
The integration of AI technologies in aerospace manufacturing is significantly transforming critical operational processes, impacting decision-making, efficiency, and workflow optimization. Explainability in AI systems is essential to ensure these technologies are understandable, trustworthy, and effectively support end-users in complex environments. This study investigates the factors influencing the explainability of AI-based Decision Support Systems in aerospace manufacturing from the end-users' perspective. The study employed a Closed Card Sorting technique involving 15 professionals from a leading aerospace organization. Participants categorized 15 AI features into groups—enhances, is neutral to, and hinders explainability. Qualitative feedback was collected to understand participants' reasoning and preferences. The findings highlighted the importance of user support features in enhancing explainability, such as system feedback on user inputs and error messages with guidance. In contrast, technical jargon was consistently perceived as a hindrance. Transparency of algorithms emerged as the highest-priority feature, followed by clarity of interface design and decision rationale documentation. Qualitative insights emphasized the need for clear communication, intuitive interfaces, and features that reduce cognitive load. The study provides actionable insights for designing AI-based DSSs tailored to the needs of aerospace professionals. By prioritizing transparency, user support, and intuitive design, designers and developers can enhance system explainability and foster user trust. These findings support the human-centric development of AI technologies and lay the groundwork for future research exploring user-centered approaches in different high-stakes industrial contexts.
The transition from Industry 4.0 to Industry 5.0 marks a significant paradigm shift in industrial advancement (Mourtzis et al., 2022). This evolution moves the focus from the automation and digitization of the 4th industrial revolution to a new era prioritizing human-centric development in research and industrial practices (Ghobakhloo et al., 2023). While Industry 4.0 sets the stage by integrating cutting-edge technologies such as robotics and machine learning, Industry 5.0 focuses on human-centric development, emphasizing the importance of human-machine collaboration for more resilient and sustainable work environments (Möller et al., 2022).
The transition between Industry 4.0 and Industry 5.0 presents challenges, particularly in effectively integrating these two paradigms. Xu et al. (2021) highlight that while Industry 4.0 is mainly driven by technology, Industry 5.0 adopts a value-driven approach, focusing on reintroducing human decision-making into organizational processes. To address this challenge, Golovianko et al. (2023) propose a hybrid model that combines the technological efficiency of Industry 4.0 with the human-centered values of Industry 5.0. This model aims to harness the strengths of both paradigms to create more sustainable and resilient industrial systems. Leng et al. (2022) further stress that Industry 5.0 places human wellbeing at the heart of manufacturing systems, seeking social objectives beyond mere employment and economic growth. This human-centered focus aligns with the emphasis on sustainability and resilience, underscoring the need for future industrial systems to balance efficiency, social responsibility, and environmental sustainability.
Therefore, the three core pillars of Industry 5.0—human-centrism, sustainability, and resilience—aim to build industrial systems that are both adaptable and enduring (Alves et al., 2023). A human-centric approach focuses on enhancing human capabilities through AI and robotics, ensuring that these technologies complement rather than replace human roles and skills (Alves et al., 2023). This paradigm emphasizes the need for intuitive, transparent, and trustworthy technologies that align with human needs, reinforcing the growing importance of eXplainable AI (XAI) in ensuring that technological advancements remain accessible and aligned with these principles (Gordini et al., 2024). XAI systems are specifically designed to enhance transparency and interpretability, making their internal processes clear and understandable to users (Arrieta et al., 2020). Explainability is essential for helping end-users grasp the complex decision-making processes of AI algorithms, fostering trust and enabling more effective human-AI collaboration (Longo et al., 2024).
Recent research on XAI emphasizes the need to improve AI explainability (Saeed and Omlin, 2023). Taj and Zaman (2022) explore how XAI systems can facilitate the transition to Industry 5.0 by leveraging advanced technologies to enhance human-machine collaboration in smart industries. Rožanec et al. (2023) propose a human-centric AI architecture for Industry 5.0, where explainability is key to the effective deployment of AI systems in industrial settings. Additionally, studies by Chamola et al. (2023) and Cimino et al. (2023) highlight the importance of trustworthy and explainable AI in reducing bias and ensuring transparency, particularly in critical sectors. They underscore the need for transparent explanation models to build human trust in AI-based systems. Lastly, Minh et al. (2022) review the XAI methods, categorizing them into pre-modeling, interpretable, and post-modeling explainability, and discuss some challenges, such as balancing performance with explainability while addressing security and policy concerns.
Thus, explainability emerges as a crucial factor in the design of AI systems. This is especially relevant for Decision Support Systems (DSSs), defined as computer-based systems that assist in organizational decision-making (Srinivasan et al., 2024). According to Chander et al. (2022), DSSs can effectively support management, operations, and planning activities when integrated with AI. These systems help make decisions in dynamic and difficult-to-predict environments. They typically process large volumes of data, provide user-friendly interfaces, and use models to analyze information, make decisions, and solve problems (Srinivasan et al., 2024).
DSSs are widely used in sectors like aerospace manufacturing, helping workers handle complex tasks and make more informed, effective, and efficient decisions (Felsberger and Reiner, 2020). The aerospace sector can be considered critical due to its accent on safety, the complexity of its systems, and strict adherence to national and international regulations, all of which ensure the reliable and safe operation of aircraft in a field that significantly impacts global connectivity, defense, and economy (Zuluaga et al., 2024). In such environments, a transparent understanding of AI's decision-making is essential for fostering effective collaboration between humans and AI, benefiting overall operational efficiency.
Developing explainable AI-based DSSs involves understanding end-user requirements for what makes an AI system explainable to them. This step is essential for understanding their needs regarding comprehension of the AI's logic and functions. Adopting a user-centered approach ensures that AI tool development aligns with user experiences and expectations, increasing the chances of AI solutions being adopted for real-world problems and improving transparency and trustworthiness.
This article investigates end-user requirements for explainability in AI-based DSSs in aerospace manufacturing. The study involves sector workers classifying AI features influencing DSSs' explainability. The results reveal which features are perceived to improve, remain neutral, or hinder the clarity of AI logic explanations. This research is intended to propose a user-informed framework to guide AI system development.
Explainability in AI systems is closely tied to foundational principles of Human-Computer Interaction (HCI), which focuses on designing systems that align with users' cognitive processes, practical needs, and situational contexts (Kästner and Crook, 2024). HCI provides a conceptual framework for understanding how users engage with complex technologies, emphasizing that trust, usability, and clarity are fundamental to effective human-computer interactions. HCI principles foster clear and meaningful interactions, ensuring that systems perform their intended functions and enhance user confidence in decision-making processes (Lim et al., 2009).
Recent advancements in HCI research have underscored the importance of tailoring AI explanations to users' unique needs. To do so, Margetis et al. (2021) stress the importance of adopting a human-centered design, proposing a framework for integrating the “human-in-the-loop” paradigm to ensure that AI systems are technically proficient and aligned with human needs and cognitive processes. This perspective integrates human input throughout the AI lifecycle to enhance system performance and user experience.
Chromik and Butz (2021) discuss the essential role of Explanation User Interfaces (EUIs) in XAI, emphasizing that their design should account for the social and iterative nature of explanations. They propose specific design principles to ensure that EUIs accommodate diverse user groups and real-world applications, fostering more effective human-AI interaction. In particular, AI explanations should be tailored to users' expertise and decision-making contexts, evolving dynamically through iterative feedback to remain relevant and comprehensible. In line with this, Liao and Varshney (2021) highlight the necessity of contextualizing AI explanations, ensuring that information is accessible, actionable, and directly relevant to users' tasks and goals. This requires consideration of user expertise, operational environments, and the cognitive demands of interpreting AI outputs, particularly in high-stakes domains where explainability significantly impacts safety and efficiency.
HCI methodologies, such as participatory design and usability evaluations, offer practical tools for developing user-centered systems that prioritize explainability. Participatory design involves actively engaging end-users in the design process, allowing developers to incorporate real-world user feedback into system functionalities and interfaces (Amershi et al., 2019). Usability evaluations, on the other hand, test systems in simulated or actual use cases to identify potential barriers to understanding and interaction (Chen et al., 2022). These methodologies are valuable for AI-based systems, where explainability often hinges on how well users interpret and act on system outputs.
Additionally, as Ehsan and Riedl (2020) discussed, reflective sociotechnical approaches emphasize integrating user values and social contexts into system design. Such approaches are relevant in high-stakes environments where transparency and acceptance are critical for fostering trust. For example, in industrial applications, AI systems that explain their decision-making processes in ways that resonate with users' roles and responsibilities are more likely to gain acceptance and be used effectively.
When grounded in HCI principles, explainable AI research can better address end-users' diverse and dynamic needs. Xu et al. (2023) note that this alignment ensures that AI systems are technically robust and user-centric, empowering users to make sense of complex outputs and confidently engage with the system. In other words, the alignment between HCI principles and explainable AI facilitates more effective and human-centered applications of AI, particularly in industrial and operational domains.
Current research on XAI attempts to identify the system features that affect end-users understanding and interpretation of AI outcomes. Recent studies show a strong interest in how AI's transparency and understandability features influence its explainability. Bhagya et al. (2023) underscore the importance of clearly presenting algorithms' logic and AI systems' decision-making processes, making even complex systems like deep neural networks more accessible to users. The focus on clarity is supported by the argument that the understanding and acceptance of AI systems are improved when they are transparent and interpretable (Casacuberta et al., 2022; Joyce et al., 2023). Additionally, real-time explanatory features can simplify models' complexity, enhancing the integration of advanced technology with user experience (Khakurel and Rawat, 2022; Sammani et al., 2023).
User support features also impact explainability. Arya et al. (2022) emphasize the importance of comprehensive tutorials explaining how systems work and detailed documentation in making AI systems more user-friendly. Additionally, practical user guidance, such as informative messages that detect potential errors or anomalies, can reduce cognitive burden and enhance user interaction with AI (Bauer et al., 2023; Bobek et al., 2024). Supporting this perspective, findings from Kim et al. (2023) underscore the role of consistent feedback from systems to users in bolstering human comprehension, while Werz et al. (2024) found that system error detection helps identify which aspects of AI decision-making require clearer explanations to users.
The design of system interfaces significantly impacts explainability. A study conducted by Encarnação et al. (2022) illustrates how AI visualization tools, such as interactive graphs, can simplify the interpretation of complex algorithms, aiding users in understanding the systems' outcomes. Mucha et al. (2021) also focus on the importance of detailed reporting on the systems' decisions in improving the efficacy of end-user interactions with these systems. Further emphasizing this point, Costa and Silva (2022) and Reddy (2024) highlight that intuitive layout and user-centric design principles in interface development make AI-based DSSs more comprehensible and accessible, fostering user engagement.
Finally, systems' communication and language features may influence the perception and understanding of AI outputs. Simplified language and knowledge-intensive processing probes enhance user comprehension of AI systems (Guersenzvaig and Casacuberta, 2023; Sheth et al., 2021). Jin et al. (2023) recommend customizing explainability approaches to match user understanding levels, employing clear and relatable language. Furthermore, Hohenstein et al. (2023) propose that AI employs language that resonates with social dynamics to cultivate and deepen human relationships.
These design features are critical for promoting the acceptance of AI systems, with trust being a central factor (Morandini et al., 2023). Choung et al. (2023) emphasize that trust in AI is crucial for users' intention to adopt AI technologies, highlighting the need for AI systems to be transparent, understandable, and thus reliable in their functionality. Similarly, Kelly et al. (2023) stress that perceived usefulness and trust are two key predictors of users' intention to adopt AI technologies, further underscoring the importance of these factors in AI acceptance. These insights emphasize the need to design AI-based DSSs that foster user trust and acceptance by encouraging engagement and ensuring effective HCI.
To conclude, understanding which system features affect end-users understanding and interpretation of AI outcomes form the foundation for designing AI-based Decision Support Systems that align with the high-stakes demands of aerospace manufacturing. By integrating transparency, user support, communication features and intuitive interfaces, these systems can address the unique challenges faced by professionals in this field.
The aerospace manufacturing industry, renowned for its high-stakes and complex operations, increasingly recognizes the necessity of AI-based DSSs. This sector is characterized by intricate design processes, the management of numerous components, adherence to stringent safety standards, and coordination of a global supply chain. In these processes, integrating AI-based DSSs may improve workforce performance (Morandini et al., 2024). By providing data-driven recommendations, AI systems facilitate accurate demand prediction and efficient inventory management, mitigating the financial implications of excess stock or shortages (Kumar, 2018).
Decision-making processes in aerospace manufacturing are particularly complex due to the high stakes in production and operations (Lin et al., 2018). Here, DSSs assist workers in navigating this environment by providing analytical insights. These systems can analyze vast data sets, revealing patterns and suggesting solutions, which is vital to this sector's high precision and accuracy (Felsberger and Reiner, 2020). Currently, DSSs are utilized for a range of functions. For instance, in planning and scheduling, tasks are used to ensure precise coordination of resource allocation to meet demanding timelines; in design, tasks are employed to predict performance and enhance material selection, while in the supply chain, forecast demands and manage inventories, ensuring the efficient flow of components (Felsberger and Reiner, 2020).
Integrating AI into these systems marks a significant evolution in their capabilities. AI-driven DSSs offer more efficient and accurate data processing, leading to nuanced recommendations that traditional systems might not discern. Advanced data analysis translates into more informed decisions and improves resource management, risk evaluation, and maintenance planning (Bousdekis et al., 2021). Integrating AI, DSSs evolve from tools that aggregate information to ones that actively contribute to strategic decision-making, thus elevating the efficiency and the scope of innovation within aerospace manufacturing.
In this context, understanding human requirements for applying AI-based DSSs can improve decision-making and optimize production processes. The effectiveness of interactions between professionals and these systems relies on the AI's explainability features. Therefore, investigating aerospace manufacturing professionals' perceptions can provide insights into how these features influence their practical application. Their usability could significantly improve if AI-based DSSs exhibit characteristics that make them more transparent and understandable to these specialized end-users.
This study utilized the Closed Card Sorting technique to explore the perceptions of aerospace manufacturing professionals regarding their explainability requirements in an AI-based DSS. Closed Card Sorting is a structured, user-centered research method effective for gaining insights into how individuals categorize specific information, revealing their thought processes and preferences (Kuniavsky, 2003). This technique was chosen for its ability to focus on participants' priorities and foster consensus while ensuring robust results with a sample of 15 participants (Lantz et al., 2019). Additionally, its adaptability to hybrid environments further supported diverse participant engagement, ensuring comprehensive insights. The method employed in this study is summarized in Figure 1, which provides a visual overview of the main research phases, including the approach definition, the development of materials, participant recruitment, data collection, and data analysis. Card Sorting methods are various and can be adapted according to the specific situation and requirements of the study. To define the procedure of the present work, we expressly referred to the work and recommendations of Conrad et al. (2019) and Conrad and Tucker (2019).
The items used in the Card Sorting exercise were selected following a structured process informed by academic literature and expert feedback. Researchers analyzed the relevant literature to identify categories of features influencing explainability. This analysis identified four key features most pertinent to the aerospace manufacturing context: (i) transparency and understandability, (ii) user support, (iii) interface design, and (iv) systems' communication and language. Within each category, items were chosen based on their relevance and applicability to AI-based DSSs, with a focus on features frequently emphasized in the literature as critical for enhancing explainability.
To ensure these items' clarity and contextual fit, two rounds of discussions were conducted with two experts affiliated with the target aerospace organization. These experts, actively involved in research and development in AI, reviewed the proposed items and provided feedback. Through this iterative process, a final set of fifteen items was identified, comprising five for transparency and understandability features, four for user support features, three for the design of system interfaces, and three for systems' communication and language features. This preparation ensured the robustness and contextual relevance of the Card Sorting exercise.
Participants were guided through a structured Card Sorting task to evaluate their perceptions of AI-based DSS features. Before beginning the exercise, they were briefed on the procedure and the purpose of the study. Participants were informed that the task involved categorizing cards, each containing an item representing a feature of an AI-based DSS, into one of three predefined groups: enhances explainability, neutral to explainability, or hinders explainability. To ensure relevance to their professional expertise, participants evaluated features specifically designed for a DSS supporting planning and scheduling tasks and key functions that are common across all their roles. This approach allowed for a consistent and domain-relevant assessment, ensuring that participants' insights were grounded in real-world applications while maintaining comparability across different roles and organizational contexts.
They were tasked with answering the question, “Please indicate if the following AI system features enhance, are neutral to, or hinder its explainability.” It was emphasized that there were no right or wrong answers, with the goal being to capture their genuine opinions. The task was neutrally framed to ensure participants were not led toward specific categorizations.
The exercise was conducted using a digital interface that allowed participants to drag and drop the cards into the appropriate groups. During the process, participants were asked to explain and justify their reasoning for assigning each item to a specific group. These explanations were recorded, transcribed, and systematically analyzed to provide deeper insights into participants' thought processes. After completing the categorization, participants ranked the items within each group based on their perceived importance to explainability. Using the same drag-and-drop interface, they assigned rankings, where a value of one indicated the most important item within the group.
The Card Sorting sessions were conducted between October 2023 and January 2024. Seven sessions were carried out in person at the organization's sites in Hamburg (Germany). Four sessions were conducted in English, while three were conducted in German with the assistance of a native-speaking interpreter to accommodate participants' language preferences. The remaining eight sessions were conducted online with English-speaking participants only. This approach avoided the potential complexity and noise associated with using interpreters in online settings. With participants' consent, all sessions were audio-recorded for subsequent transcription and analysis.
Participants were reassured that the study was exploratory and aimed at improving user-centered AI design rather than evaluating their views on workplace practices. Open feedback was encouraged throughout the process to address potential AI adoption concerns and identify and mitigate potential biases.
The materials used in the study were developed to ensure a structured and ethical approach to data collection. Participants received an invitation document outlining the study's objectives, the significance of their involvement, and a detailed informed consent form. This form adhered to the General Data Protection Regulation (GDPR), outlining the nature of their participation, data handling procedures, and measures to protect confidentiality.
The Card Sorting exercise was conducted using Qualtrics software (Tharp and Landrum, 2017), which was selected for its user-friendly interface and ability to support both in-person and virtual environments. This flexibility ensured that participants could engage with the study seamlessly, regardless of the mode of participation. By replicating the Card Sorting process effectively across different settings, the software facilitated a consistent and reliable data collection experience for both participants and researchers.
The study involved 15 high-level experts from a leading organization in the aerospace manufacturing industry. Participants were selected based on their managerial roles in decision-making processes and their prospective use of AI-based DSSs. The group consisted of 11 males and four females (Mean age = 41.6, SD = 11.8), holding high-level positions across the organization's sites in France, Germany, Spain, and the UK. Participants had a minimum of 2 years of experience within the target organization and between 5 and 16 years of experience in the aircraft manufacturing sector.
To ensure participants could provide meaningful insights, eligibility criteria required them to be current employees involved in aircraft manufacturing, assembly, or logistics, with roles relevant to the tasks supported by DSS tools. Participants were also required to have at least 4 years of experience in these processes and to be potential end-users of new DSS implementations. This selection ensured they possessed the expertise to evaluate these systems' challenges and requirements associated with explainability.
The inclusion of professionals from diverse geographical locations and functional areas enabled the study to capture a comprehensive perspective on operational practices across different contexts. Participants' roles often involved critical decision-making, risk management, and operational optimization—key areas where DSS tools are expected to significantly impact (Felsberger and Reiner, 2020).
The measures used in this study were selected based on insights from academic literature, highlighting the significance of specific features related to transparency and understandability, user support, interface design, and communication features in influencing AI-based DSSs' explainability. The following items associated with these categories were chosen:
• Transparency of algorithms: evaluates the extent to which AI algorithms' inner workings and logic are made explicit and understandable to end-users (Ara, 2024; Srinivasan et al., 2024; Shakir et al., 2024).
• Decision rationale documentation: assesses the provision of comprehensive documentation explaining the rationale behind AI system decisions (Casacuberta et al., 2022; Joyce et al., 2023).
• Contextual information on data: examines the availability of contextual information regarding the data used by the AI system, aiding users in understanding the context in which decisions are made (Kozielski, 2023; Srinivasan et al., 2024).
• Real-time explanation of choices: focuses on the system's capability to provide real-time explanations for its decision-making, simplifying complex models for users (Ara, 2024; Sammani et al., 2023; Khakurel and Rawat, 2022).
• Consistency of decision outcomes: gauges the consistency of outcomes produced by the AI system, ensuring that users can rely on predictable and coherent results (MoDastoni, 2023; Srinivasan et al., 2024).
• User guide and documentation: assesses the presence of user guides and documentation that elucidate how the AI system functions (Arya et al., 2022; Bobek et al., 2024).
• Availability of tooltips or help sections: examines the accessibility of tooltips or help sections within the system interface, providing users with on-demand assistance (Arya et al., 2022; MoDastoni, 2023; Bobek et al., 2024).
• Error messages and guidance: evaluates the presence and effectiveness of error messages and guidance that assist users in rectifying issues or anomalies (Bauer et al., 2023; Werz et al., 2024).
• Availability of tutorial or training sessions: explores the availability of tutorial or training sessions aimed at enhancing users' understanding and interaction with the system (Arya et al., 2022; Gaur et al., 2020; Werz et al., 2024).
• Clarity of interface design: assesses the clarity and user-friendliness of the system's interface, focusing on intuitive design principles (Costa and Silva, 2022; Panigutti et al., 2023; Reddy, 2024).
• Visual aids, like charts or graphs: evaluate the inclusion of visual aids to support users in interpreting complex AI outcomes (Cabour et al., 2021; Encarnação et al., 2022; Gaur et al., 2020).
• Detailed reporting features: examines the presence of detailed reporting features that provide insights into the AI system's decision-making process (Cabour et al., 2021; Mucha et al., 2021).
• Use of technical jargon: considers technical language and jargon within the AI system's communication and assesses its impact on user comprehension (Guersenzvaig and Casacuberta, 2023; Valentino and Freitas, 2022).
• System feedback on user inputs: evaluates the system's ability to provide feedback on user inputs and actions, enhancing user engagement and understanding (Lai et al., 2023; Daly et al., 2021; Sheth et al., 2021).
• Users' testimonials or case studies: explores the inclusion of users' testimonials or case studies to illustrate the practical impact and benefits of the AI system, enhancing the perception and understanding of its outputs (Shin, 2021; Hohenstein et al., 2023).
Following the principles of the Card Sorting technique, both quantitative and qualitative data were collected, analyzed, and compared. The quantitative analysis involved using descriptive statistics to understand the data distribution. Additionally, the ranking scores assigned by participants to each AI feature that enhances explainability were analyzed to identify central tendencies and variabilities, providing insights into the perceived importance of these features in improving explainability.
The qualitative feedback provided by participants during the task was analyzed using thematic analysis, following the framework outlined by Braun and Clarke (2006). This approach was chosen for its flexibility in identifying recurring patterns in qualitative data while allowing for an in-depth interpretative analysis of participants' reasoning behind their categorization choices.
An inductive coding process was used, meaning that themes were not predefined but instead emerged from participants' responses. The analysis followed the six-phase approach of thematic analysis: (i) familiarization with the data; (ii) generation of initial codes; (iii) searching for themes; (iv) reviewing themes; (v) defining and naming themes; and (vi) producing the final report (Braun and Clarke, 2006).
Two researchers independently coded the transcribed feedback using open coding, where key concepts and meaningful phrases were identified and grouped into categories. Through an iterative comparison process, these categories were refined into higher-order themes, ensuring consistency in interpretation. Inter-coder reliability was achieved through discussion and consensus.
The identified themes aligned with the same four categories established in the quantitative analysis, reinforcing the key dimensions influencing explainability in AI-based DSSs. We integrated qualitative thematic insights with the quantitative ranking data to obtain an understanding of the features that participants considered most impactful in enhancing (or not enhancing) explainability in AI-based DSSs.
Table 1 presents the distribution of participant categorizations for each system feature into three distinct groups: Enhances explainability (Group 1), Neutral to explainability (Group 2), and Hinders explainability (Group 3), based on absolute values.
Table 1. Distribution of participants' categorization (in absolute values) of each system feature into the groups.
Notably, within Group 1, features like Availability of tooltips or help sections (12 participants), Consistency of decision outcomes (12 participants), Error messages and guidance (12 participants), and Clarity of interface design (11 participants) received the highest allocations, emphasizing their potential to enhance AI-based DSS explainability. Conversely, Group 3 highlighted the Use of technical jargon as a dominant hindrance, with 10 participants categorizing it as such. This result reflects a shared concern about the negative impact of overly technical language on clarity. Group 2 displayed more varied allocations across features, representing those perceived as having a neutral effect on explainability, such as Contextual information on data (5 participants) and Transparency of algorithms (8 participants).
Descriptive statistics and frequency analyses were conducted to quantify participants' average perceptions regarding how system features influence explainability within the three groups (see Figure 2). Group 1 displayed a substantial mean value of 9.00 (SD = 3.20) and a median of 10, indicating that these features significantly enhance the clarity of explanations. The range from 1 to 12 illustrates the varying degrees of enhancement across features within this group.
In contrast, Group 2 exhibited a moderate mean of 4.20 (SD = 1.93) and a median of 4, suggesting that these features are moderately neutral in their impact on explanation clarity, with perceptions being relatively consistent among participants. Group 3 showed a low mean value of 1.60 (SD = 2.23) and a median of 1, implying that these features are rarely categorized as hindering clarity. However, the wide range from 0 to 9 signifies significant variation in the perceived hindrance levels of individual features.
Additionally, frequency analysis revealed that Group 1 constituted the majority at 60% (135 counts), followed by Group 2 at 28.9% (65 counts), and Group 3 at 11.1% (25 counts).
Descriptive analyses were also run to understand how categories of features were perceived regarding enhancing, neutral or hindering explainability. User support features emerged as the category with the highest mean score (M = 11.25), signifying items within this category were overwhelmingly perceived as enhancing explainability. On the other hand, Systems' communication and language features had the lowest (M = 6.33), indicating that items pertaining to this category were perceived as hindering explainability. Meanwhile, Transparency and understandability features and the system interface design occupied the middle ground (M = 9.4 and 10.67, respectively), suggesting that features belonging to these categories were perceived as neutral regarding their impact on explainability.
Descriptive analyses illustrated the specific AI features participants believed were essential to enhance explainability. Figure 3 represents a boxplot showcasing rankings' central tendencies and variabilities (1 = higher importance) for each feature inserted in Group 1. The box plot shows the InterQuartile Range (IQR), median rankings, overall distribution, and outliers.
Among the most highly prioritized features, Transparency of algorithms received a median rank of 2, reflecting strong consensus (IQR 1–3), followed closely by Clarity of interface design with a median rank of 3, indicating its importance and similar agreement (IQR 1–3). System feedback on user inputs ranked slightly lower, with a median of 3.5 and a broader IQR of 1–4, indicating a more varied perception of its significance.
Mid-range features included Decision rationale documentation, User guide and documentation, and Error messages and guidance, all with median ranks of 6.5 and 7, respectively, with IQRs of 2, suggesting some variability in participants' responses. Error messages and guidance featured an outlier at rank 12, indicating a notably less favorable view from one participant.
Several features ranked in the middle range, including Availability of tooltips or help sections and Contextual information on data, with median ranks of 5.5 but with IQRs of 3 and 4, pointing to more substantial divergence in opinion. Visual aids and Real-time explanations of choices had median rankings of 5, with IQRs of 4.5, suggesting moderate variability in their perceived importance for enhancing explainability.
At the lower end of the rankings, Consistency of decision outcomes and Detailed reporting features were ranked at 5. The former had a wider IQR of 2–5 and an outlier at rank 10, indicating a notable departure from consensus. In contrast, Detailed reporting features had a higher dispersion (IQR 2–9) without outliers, showing more uniform participant expectations. The availability of tutorial or training sessions had a median rank of 7.5 and an IQR of 3, suggesting varying perceived importance levels. Users' testimonials or case studies ranked lowest, with a median of 8.5 (IQR 2.5), indicating that it was considered the least important feature on average. However, an outlier at rank 13 emphasized a divergent view. Finally, the Use of technical jargon was uniquely ranked by one participant (value = 4).
Qualitative feedback collected during the Card Sorting technique reveals in-depth perceptions of AI system features that influence explainability, accompanied by reasons behind their categorization. The participants' feedback from the audio recordings was transcribed using Notta Pro software (Notta.ai, n.d.) by one author. Subsequently, two authors independently analyzed the transcriptions, identifying key themes. They reached a consensus on the content, which was then reviewed and discussed with the other three authors. After a final discussion, all five authors agreed on the key insights.
Analysis of these responses revealed recurring themes, particularly the importance of transparency, trust, usability, and system communication. While many responses aligned with existing literature on the necessity of transparency for AI adoption and understanding, several new insights emerged. These included participants' expectations for real-time explanations and a preference for simplified communication. Furthermore, the feedback highlighted specific preferences regarding how transparency should be presented, especially in high-stakes environments such as aerospace manufacturing. While some themes confirmed extant literature, others—such as the demand for system-generated error messages and more explicit user feedback—offered new perspectives on how AI systems can better support end-users.
The feedback came from a diverse set of participants across various roles in the aerospace manufacturing sector. Although the sample size was limited, data saturation was achieved as no new significant themes emerged during the final stages of analysis. This suggests that the sample was sufficiently representative and could provide meaningful insights into the key features influencing explainability.
The following sections provide a more detailed breakdown of these results, specifically examining the qualitative feedback related to transparency, user support, system interfaces, and communication features.
The emphasis on transparency and understandability features was evident, with participants advocating for more transparent AI algorithms and decision-making processes. For instance, one participant noted, “I'd like a decision support system to provide information options through being transparent…providing clear explanations on why this decision is suggested.” Another participant emphasized the importance of clarity in the rationale behind decisions, stating, “When the system clearly explains the reasons for its decisions, it feels more like a reliable partner than just a tool.”
The role of transparency in enhancing explainability was further reinforced by participants who highlighted the significance of decision rationale documentation. One participant shared, “Transparency of algorithms enhances clarity of the decision or recommendation of the system. In this regard, documentation, for example, would be invaluable.” Similarly, another participant underscored the need for supporting materials, stating, “Having documentation on the decision is important as you need to know the limitations of the system, so you can understand how to use it properly.”
Differently, the opinions on contextual information on data varied: while one participant argued that “Contextual information on data might be overloading,” others considered it a priority, noting its potential to enhance clarity. As one participant explained, “Contextual information on data is a priority because I think it enhances clarity of explanation. You need to know the context to understand the decision fully.”
Participants also reflected on the importance of real-time explanations, emphasizing that while this feature can enhance transparency, its necessity depends on the context of the application. One explained, “Having real-time explanation of choices definitely helps enhance explainability, but I think if there's some delay—like a minute or so—I would not expect an instant explanation. So, yes, it is important, but other features related to transparency and understandability are more useful for me.” This perspective highlights that while immediacy in explanations is valuable, it may not always be a priority compared to other transparency-related features.
Feedback on user support features underscored the necessity of clear error messages and easily accessible help for enhanced explainability. A participant expressed, “I think it's helpful for me to have clear error messages and guidance,” reflecting the need for direct and actionable support. Another participant emphasized, “Error messages are crucial as they offer immediate guidance during tool use, eliminating the need to seek external testimonials or case studies. [This feature] precedes user testimonials in usefulness.” This highlights the value of immediate, system-generated feedback in reducing dependency on external resources.
Similarly, participants highlighted the importance of having tutorials and training sessions. One participant shared, “It's nice to have tutorial or training sessions available, even if, from my experience, it's rarely used. But I think that if it's good, it can be used more often.” Another added, “Having tutorials and training is essential due to organizational high turnover,” suggesting that structured training resources are necessary for maintaining operational efficiency. A third participant noted, “Training sessions are rated higher than the user guide and case studies for their direct impact on user comprehension,” emphasizing their practical utility in building familiarity with the system.
The availability of user guides and documentation also emerged as a critical feature. A participant explained, “User guide and documentation are crucial for me. […] Even though we have competent planning teams, the learning curve is not as steep as I'd like due to the system's robustness.” Another echoed this sentiment, linking the importance of guides to accessibility: “[Regarding the] availability of tutorials, I think the same as user guide,” underscoring their complementary role in fostering system usability.
Participants appreciated interfaces that support the easy comprehension of AI decisions, confirming the role of the design of system interfaces in influencing explainability. One participant remarked, “Clarity of interface design and visual aids like charts or graphs can significantly reduce the cognitive load,” emphasizing the role of design in improving understandability. Another noted, “Visual aids [can enhance explainability], yes. But it depends on the system and user inputs; typically, the user can ask the system something.”
The importance of visual aids, such as charts or graphs, was consistently highlighted. One participant stated, “Visual aids are indispensable; we explain everything visually.” Another elaborated, “Graphs and charts can help in reducing the cognitive workload and effort. But if it's easy to use, it doesn't change anything about whether I understood why the decision was made. I think [explainability] doesn't depend on the interface.” This suggests that while visual aids are highly beneficial for reducing cognitive effort, their influence on understanding decision-making processes may vary.
Some participants viewed visual aids as more relevant to interface usability. For example, one shared, “Visual aids can be helpful for the reporting, and, of course, it helps to understand, but it's not necessary to have that.” These insights reflect a nuanced perspective, where visual tools are recognized as valuable for presenting information and reducing cognitive load but not universally essential for fostering explainability.
Systems' communication and language features were common concerns among the participants. “The use of technical jargon can reduce the clarity of explanations, especially for those unfamiliar with the technical issues,” a participant noted, advocating for more straightforward language. Another participant added, “It depends on the population. If you have a very well-skilled population, it does not matter, but it can hinder people who are not aware of technical jargon. Sometimes technical jargon is not commonly used across the whole company.” The potential for disengagement caused by excessive or overly complex information was also raised, with one participant explaining, “Too much information can overwhelm users, leading to disengagement.”
The value of system feedback on user inputs was consistently highlighted. A participant remarked, “System feedback on user inputs definitely enhances explainability. Because I'm interested in understanding if what I'm doing is right or sufficient step by step.” Another agreed, stating, “Yes, I would think that it helps,” reflecting the consensus that such feedback supports real-time decision-making and builds user confidence.
Opinions on users' testimonials or case studies were more nuanced. While some participants viewed them as less critical than error messages, which offer immediate guidance during tool use, others highlighted their role in fostering trust. One participant explained, “I think the system should always provide case studies. However, it doesn't necessarily assist with the explanation. Consistency is essential because if the system provides different information at different times, it doesn't make sense, and trust in the system is lost.” These insights underline the importance of clear, consistent communication in enhancing explainability and maintaining user trust.
This study revealed that aerospace professionals place significant importance on specific AI-based DSS features in enhancing explainability. Notably, most participants assigned features related to user support to the group of features that enhanced explainability. These findings are in line with a previous study by Ridley (2022) that highlighted that combining user support and interface design could improve user trust in AI systems. Trust, from a psychological lens, is a fundamental factor in human-automation interaction (Hoff and Bashir, 2015), and explainability serves as a mechanism to strengthen trust by reducing uncertainty and increasing perceived system reliability. Thus, the participants collective perception underscores these features' critical role in enhancing user understanding, as they directly address common user challenges and provide immediate assistance, making the AI systems' operations more intuitive and accessible.
Participants also emphasized the role of tutorials and training sessions, particularly in organizations with high turnover rates, highlighting how these resources facilitate operational efficiency. These findings echo Bhima et al. (2023), who underscore the importance of employee training programs in maximizing the adoption of AI systems and fostering organizational efficiency. Similarly, Soulami et al. (2024) emphasize that well-structured training initiatives are crucial for enhancing employee adaptability and wellbeing during AI integration, thereby supporting overall organizational performance.
Some specific features deviate from the general trend of their respective category. For example, system feedback on user inputs is identified as significantly enhancing explainability, highlighting the importance of including such features in AI systems despite the broader category of systems' communication and language features being perceived as a hindrance to explainability. From a psychological perspective, system feedback strengthens user trust and engagement by confirming that the AI is responsive to user actions (Madhavan and Wiegmann, 2007). The reciprocity of human-AI interaction emerges here as a critical factor in automation reliance, as users are more likely to develop a calibrated level of trust when they receive consistent, meaningful feedback on their inputs. Rather than being perceived as mere system outputs, interactive feedback mechanisms foster a more dynamic and transparent exchange between users and AI, reinforcing explainability and trust. This supports existing findings that emphasize the need for AI systems to communicate in a clear and tailored manner, ensuring that explanations remain accessible and user-friendly (Sheth et al., 2021).
Moreover, the categorization of the technical jargon by most participants as hindering explainability highlights the negative impact of overly technical language in the aerospace domain. While four participants placed it in the neutral group and one in the group that enhances explainability, the overall results reflect a shared concern that such language can create barriers to effective communication and understanding. This suggests that simplifying the language used in AI interfaces and documentation could significantly improve user understanding and confidence in AI systems (Pieters, 2011). Participants also expressed concerns about the overwhelming nature of excessive or overly detailed information, emphasizing the need for a balanced approach in system communication to prevent disengagement. Excessive detail can in fact lead to information overload (Eppler and Mengis, 2004), thereby increasing frustration and decreasing user engagement. A well-calibrated level of detail ensures that information remains accessible without overwhelming the user, fostering more effective human-AI interaction.
Features related to Transparency and understandability were generally perceived as enhancing explainability, which aligns with previous studies (Ehsan et al., 2021). However, results on the specific features of this category show that a significant portion of participants consider them very important, while other perceptions tend to be neutral. Despite this discrepancy, the need to clarify how AI systems reach decisions emerged as essential for increasing explainability and fostering trust in the systems, fostering HCI. Real-time explanations, though valued, were noted as less critical than other features, such as decision rationale documentation or contextual information, emphasizing the need for prioritization based on user-specific contexts and requirements.
The analysis of rankings highlights participants' priorities and needs for enhancing explainability. Here, Transparency of algorithms emerged as the highest priority, indicating a solid consensus on the need to make AI algorithms' inner workings explicit and understandable to users. This reinforces that understanding AI decision-making processes empowers users, increasing reliability and trust (Ezer et al., 2019).
Clarity of interface design ranked second in importance, reinforcing the idea that user-friendly interfaces are crucial for effective interaction with AI-based DSSs. This priority aligns intending to make AI accessible and usable for aerospace professionals, where intuitive design can reduce cognitive load and enhance overall user satisfaction (Panigutti et al., 2023; Ridley, 2022; Salimiparsa et al., 2021).
Decision rationale documentation followed closely, highlighting the value of providing comprehensive documentation to explain AI system decisions. This documentation serves as a bridge between technical processes and end-users understanding, catering to the diverse needs of aerospace professionals with varying technical expertise. In line with previous findings (Salimiparsa et al., 2021), participants further acknowledged the complementary role of visual aids, such as charts and graphs, in reducing cognitive load. However, their utility was viewed as context-dependent.
The feedback collected reflects the diversity of the participants' roles in aerospace manufacturing, with varying levels of experience and familiarity with AI tools influencing their responses. While the categorization of features was broadly applicable, the participants' experiences with tools like user guides, error messages, and training sessions could influence their assessment of these features' usefulness. This variance in experience highlights the importance of considering user background when interpreting feedback, as prior exposure to well-designed resources could create a more favorable perception compared to those who have encountered less effective support tools.
The findings of this study highlight opportunities for refining the development of explainable AI-based DSSs by focusing on user-centered principles. Practical implications extend to how these insights can inform actionable design and implementation strategies in high-stakes industries like aerospace manufacturing.
User support, transparency, interface design, and communication features represent interconnected dimensions influencing explainability. In line with previous studies (Ehsan et al., 2021; Panigutti et al., 2023; Ridley, 2022), our findings suggest that, when designing XAI DSSs, adopting a comprehensive approach that integrates these dimensions cohesively could enhance system usability and trust. For example, transparency features are not just isolated elements but can complement user support mechanisms by providing contextual clarity that reinforces error messages or guidance.
Interface design plays a key supporting role in bridging complexity and accessibility. The integration of visual aids, while not universally prioritized by participants, could be selectively employed to align with task-specific needs, as suggested in recent research (Nazemi et al., 2024). This highlights the importance of tailoring system interfaces to balance cognitive load while maintaining focus on decision-making processes, as discussed for DSS in other critical domains such as healthcare (Salimiparsa et al., 2021) or aviation (Mietkiewicz et al., 2024).
Clear and consistent communication features are critical when technical jargon hinders understanding. Streamlined, plain-language strategies that match users' expertise can enhance accessibility and foster confidence. Coupled with consistent and reliable system feedback, such an approach can strengthen trust, improve users' satisfaction, and encourage user engagement (Folorunsho et al., 2024).
Despite the significant contributions of this study, some limitations should be acknowledged. First, the generalizability of the findings is limited by the focus on aerospace manufacturing professionals. While the perspectives of these high-level experts are highly relevant to the industry, the results may not fully apply to other sectors or broader populations. Second, including German-speaking participants with interpretation support introduces the potential for subtle nuances or variations in understanding and interpretation, which could influence the data accuracy. Finally, the relatively small sample size of 15 participants, though sufficient for exploratory purposes, limits the generalizability of the findings and may not capture the full spectrum of perspectives within the aerospace manufacturing sector. Specifically, it may affect the interpretation of descriptive statistics and their broader applicability. Consequently, the quantitative results should be interpreted cautiously, as they primarily provide an overview of participants' categorizations rather than definitive statistical generalizations. Future studies should address these limitations by expanding the sample size, diversifying the participant pool, and minimizing potential language-related biases.
In the current industrial landscape, aerospace manufacturing is significantly shifting toward integrating AI technologies into its workflows. This transformation reflects the broader aspirations of Industry 5.0, which emphasizes advancing technological innovation while upholding human-centric values.
This study highlights the dimensions for improving the explainability of AI-based Decision Support Systems from the perspective of aerospace professionals. Transparency, clarity, and ease of use emerge as guiding principles for developing these systems, ensuring their alignment with the needs and expectations of end-users.
As the aerospace sector innovates, future research could focus on creating robust guidelines for developing human-centered AI-based DSS. These efforts can empower human abilities, enhance operational effectiveness, and support the safety and reliability critical to high-stakes industries such as aerospace manufacturing.
The raw data supporting the conclusions of this article will be made available by the authors, without undue reservation.
SM: Data curation, Formal analysis, Methodology, Software, Visualization, Writing – original draft, Writing – review & editing, Conceptualization, Investigation, Validation. FF: Conceptualization, Formal analysis, Funding acquisition, Methodology, Project administration, Resources, Software, Writing – original draft, Writing – review & editing, Supervision. MH: Conceptualization, Resources, Validation, Writing – original draft, Writing – review & editing. SQ-A: Resources, Validation, Writing – original draft, Writing – review & editing. LP: Conceptualization, Funding acquisition, Investigation, Project administration, Supervision, Writing – original draft, Writing – review & editing, Resources.
The author(s) declare that financial support was received for the research and/or publication of this article. This study was part of the TUPLES project-Trustworthy Planning and Scheduling with Learning and Explanations. The project has received funding from the European Commission’s Horizon Europe Research and Innovation program under the Grant Agreement No. 101070149.
The authors declare that the research was conducted in the absence of any commercial or financial relationships that could be construed as a potential conflict of interest.
The author(s) declare that Gen AI was used in the creation of this manuscript. Generative AI tools were utilized in specific, limited aspects of this manuscript's preparation. Specifically, we employed large language models to assist with initial manuscript formatting and basic language editing. However, all substantive content—including the research design, data collection, analysis, and interpretation of results—was conducted by Unibo and airbus team. The core work including card sorting study design, participant interactions, data analysis, and development of findings and conclusions, was performed by authors in Hamburg. This use of AI tools was limited to auxiliary support in manuscript preparation.
All claims expressed in this article are solely those of the authors and do not necessarily represent those of their affiliated organizations, or those of the publisher, the editors and the reviewers. Any product that may be evaluated in this article, or claim that may be made by its manufacturer, is not guaranteed or endorsed by the publisher.
Alves, J., Lima, T. M., and Gaspar, P. D. (2023). Is Industry 5.0 a human-centred approach? A systematic review. Processes 11:193. doi: 10.3390/pr11010193
Amershi, S., Weld, D., Vorvoreanu, M., Fourney, A., Nushi, B., Collisson, P., et al. (2019). “Guidelines for human-AI interaction,” in Proceedings of the 2019 CHI Conference on Human Factors in Computing Systems (New York, NY: ACM Press), pp. 1–13. doi: 10.1145/3290605.3300233
Ara, M. (2024). Enhancing AI Transparency for Human Understanding: A Comprehensive Review (Preprints.org). St. Louis, MO: Saint Louis University. doi: 10.20944/preprints202410.0262.v1
Arrieta, A. B., Díaz-Rodríguez, N., Del Ser, J., Bennetot, A., Tabik, S., Barbado, A., et al. (2020). Explainable Artificial Intelligence (XAI): Concepts, taxonomies, opportunities and challenges toward responsible AI. Inform. Fusion 58, 82–115. doi: 10.1016/j.inffus.2019.12.012
Arya, V., Bellamy, R. K., Chen, P. Y., Dhurandhar, A., Hind, M., Hoffman, S. C., et al. (2022). AI explainability 360: Impact and design. Proc. AAAI Conf. Artif. Intellig. 36, 12651–12657. doi: 10.1609/aaai.v36i11.21540
Bauer, K., von Zahn, M., and Hinz, O. (2023). Expl (AI) ned: The impact of explainable artificial intelligence on users' information processing. Inform. Syst. Res. 34, 1582–1602. doi: 10.1287/isre.2023.1199
Bhagya, J., Thomas, J., and Raj, E. D. (2023). “Exploring explainability and transparency in deep neural networks: a comparative approach,” in 2023 7th International Conference on Intelligent Computing and Control Systems (ICICCS) (Madurai: IEEE), 664–669.
Bhima, B., Zahra, A., and Nurtino, T. (2023). “Enhancing organizational efficiency through the integration of artificial intelligence in management information systems,” in APTISI Transactions on Management (ATM). Tangerang: Universitas Raharja.
Bobek, S., Korycińska, P., Krakowska, M., Mozolewski, M., Rak, D., Zych, M., et al. (2024). User-centric evaluation of explainability of AI with and for humans: a comprehensive empirical study. arXiv [preprint] arXiv:2410.15952. doi: 10.48550/arXiv.2410.15952
Bousdekis, A., Lepenioti, K., Apostolou, D., and Mentzas, G. (2021). A review of data-driven decision-making methods for industry 4.0 maintenance applications. Electronics 10:828. doi: 10.3390/electronics10070828
Braun, V., and Clarke, V. (2006). Using thematic analysis in psychology. Qual. Res. Psychol. 3, 77–101. doi: 10.1191/1478088706qp063oa
Cabour, G., Morales, A., Ledoux, É., and Bassetto, S. (2021). Towards an explanation space to align humans and explainable AI teamwork. arXiv [preprint] arXiv:2106.01503. doi: 10.48550/arXiv.2106.01503
Casacuberta, D., Guersenzvaig, A., and Moyano-Fernández, C. (2022). Justificatory explanations in machine learning: for increased transparency through documenting how key concepts drive and underpin design and engineering decisions. AI Soc. 2022, 1–15. doi: 10.1007/s00146-022-01389-z
Chamola, V., Hassija, V., Sulthana, A. R., Ghosh, D., Dhingra, D., and Sikdar, B. (2023). A review of trustworthy and explainable artificial intelligence (XAI). IEEE Access. 11, 78994–79015. doi: 10.1109/ACCESS.2023.3294569
Chander, B., Pal, S., De, D., and Buyya, R. (2022). “Artificial intelligence-based Internet of Things for Industry 5.0,” in Artificial Intelligence-based Internet of Things Systems (Cham: Springer), 3–45. doi: 10.1007/978-3-030-87059-1_1
Chen, V., Johnson, N., Topin, N., Plumb, G., and Talwalkar, A. (2022). Use-case-grounded simulations for explanation evaluation. Adv. Neural Inf. Process. Syst. 35, 1764–1775. doi: 10.48550/arXiv.2206.02256
Choung, H., David, P., and Ross, A. (2023). Trust in AI and its role in the acceptance of AI technologies. Int. J. Human–Comp. Intera. 39, 1727–1739. doi: 10.1080/10447318.2022.2050543
Chromik, M., and Butz, A. (2021). “Human-XAI interaction: a review and design principles for explanation user interfaces,” in Human-Computer Interaction–INTERACT 2021: 18th IFIP TC 13 International Conference (Bari: Springer International Publishing), 619–640.
Cimino, A., Elbasheer, M., Longo, F., Nicoletti, L., and Padovano, A. (2023). Empowering field operators in manufacturing: a prospective towards industry 5.0. Procedia Comput. Sci. 217, 1948–1953. doi: 10.1016/j.procs.2022.12.395
Conrad, L. Y., Demasson, A., Gorichanaz, T., and VanScoy, A. (2019). Exploring card sort methods: Interaction and implementation for research, education, and practice. Proc. Assoc. Inform. Sci. Technol. 56, 525–528. doi: 10.1002/pra2.81
Conrad, L. Y., and Tucker, V. M. (2019). Making it tangible: hybrid card sorting within qualitative interviews. J. Document. 75, 397–416. doi: 10.1108/JD-06-2018-0091
Costa, A., and Silva, F. (2022). “Interaction design for AI systems: an oriented state-of-the-art,” in 2022 International Congress on Human-Computer Interaction, Optimization and Robotic Applications (HORA) (Ankara: IEEE), 1–7.
Daly, E., Mattetti, M., Alkan, Ö., and Nair, R. (2021). User Driven Model Adjustment via Boolean Rule Explanations. arXiv. doi: 10.1609/aaai.v35i7.16737
Ehsan, U., Liao, Q. V., Muller, M., Riedl, M. O., and Weisz, J. D. (2021). “Expanding explainability: towards social transparency in AI systems,” in CHI Conference on Human Factors in Computing Systems (CHI '21), May 8–13, 2021, Yokohama, Japan (New York, NY: ACM Press), 1–19. doi: 10.1145/3411764.3445188
Ehsan, U., and Riedl, M. O. (2020). “Human-centered explainable AI: Towards a reflective sociotechnical approachm” in HCI International 2020 - Late Breaking Papers: Multimodality and Intelligence, eds. C. Stephanidis, M. Kurosu, H. Degen, and L. Reinerman-Jones (Cham: Springer International Publishing), 449–466. doi: 10.1007/978-3-030-60117-1_3
Encarnação, L. M., Kohlhammer, J., and Steed, C. A. (2022). Visualization for AI Explainability. IEEE Comput. Graph. Appl. 42, 9–10. doi: 10.1109/MCG.2022.3208786
Eppler, M. J., and Mengis, J. (2004). The concept of information overload: a review of literature from organization science, accounting, marketing, MIS, and related disciplines. Inform. Soc. 20, 325–344. doi: 10.1080/01972240490507974
Ezer, N., Bruni, S., Cai, Y., Hepenstal, S. J., Miller, C. A., and Schmorrow, D. D. (2019). “Trust engineering for human-AI teams,” in Proceedings of the Human Factors and Ergonomics Society Annual Meeting, Vol. 63 (Los Angeles, CA: SAGE Publications), 322–326.
Felsberger, A., and Reiner, G. (2020). Sustainable industry 4.0 in production and operations management: a systematic literature review. Sustainability 12:7982. doi: 10.3390/su12197982
Folorunsho, S., Adenekan, O., Ezeigweneme, C., Somadina, I., and Okeleke, P. (2024). Leveraging technical support experience to implement effective AI solutions and future service improvements. Int. J. Appl. Res. Soc. Sci. 6:8. doi: 10.51594/ijarss.v6i8.1425
Gaur, M., Desai, A., Faldu, K., and Sheth, A. (2020). Explainable AI Using Knowledge Graphs. San Diego, CA: ACM CoDS-COMAD Conference.
Ghobakhloo, M., Iranmanesh, M., Tseng, M. L., Grybauskas, A., Stefanini, A., and Amran, A. (2023). Behind the definition of Industry 5.0: a systematic review of technologies, principles, components, and values. J. Indust. Prod. Eng. 40, 432–447. doi: 10.1080/21681015.2023.2216701
Golovianko, M., Terziyan, V., Branytskyi, V., and Malyk, D. (2023). Industry 4.0 vs. industry 5.0: co-existence, transition, or a hybrid. Proced. Comp. Sci. 217, 102–113. doi: 10.1016/j.procs.2022.12.206
Gordini, A., Pozzi, M., Lombardi, M., Sergeys, T., Guns, T., De Cesarei, A., et al. (2024). “Trustworthiness of AI in planning and scheduling: the experience of the TUPLES project,” in Decision Science Alliance International Summer Conference (Cham: Springer Nature Switzerland), 328–342.
Guersenzvaig, A., and Casacuberta, D. (2023). Justificatory explanations: a step beyond explainability in machine learning. Eur. J. Public Health 33:873. doi: 10.1093/eurpub/ckad160.873
Hoff, K. A., and Bashir, M. (2015). Trust in automation: Integrating empirical evidence on factors that influence trust. Human Fact. 57, 407–434. doi: 10.1177/0018720814547570
Hohenstein, J., Kizilcec, R. F., DiFranzo, D., Aghajari, Z., Mieczkowski, H., Levy, K., et al. (2023). Artificial intelligence in communication impacts language and social relationships. Sci. Rep. 13:5487. doi: 10.1038/s41598-023-30938-9
Jin, W., Li, X., and Hamarneh, G. (2023). The XAI alignment problem: Rethinking how should we evaluate human-centred AI explainability techniques. arXiv [preprint] arXiv:2303.17707. doi: 10.48550/arXiv.2303.17707
Joyce, D. W., Kormilitzin, A., Smith, K. A., and Cipriani, A. (2023). Explainable artificial intelligence for mental health through transparency and interpretability for understandability. NPJ Digital Med. 6:6. doi: 10.1038/s41746-023-00751-9
Kästner, L., and Crook, B. (2024). Explaining AI through mechanistic interpretability. Eur. J. Philos. Sci. 14:52. doi: 10.1007/s13194-024-00614-4
Kelly, S., Kaye, S. A., and Oviedo-Trespalacios, O. (2023). What factors contribute to the acceptance of artificial intelligence? A systematic review. Telemat. Inform. 77:101925. doi: 10.1016/j.tele.2022.101925
Khakurel, U., and Rawat, D. B. (2022). “Evaluating explainable artificial intelligence (XAI): algorithmic explanations for transparency and trustworthiness of ML algorithms and AI systems,” in Artificial Intelligence and Machine Learning for Multi-Domain Operations Applications IV (Washington, DC: SPIE), 65–75.
Kim, S. S. Y., Watkins, E. A., Russakovsky, O., Fong, R., and Monroy-Hernández, A. (2023). “Help me help the AI”: understanding how explainability can support human-AI interaction. in Proceedings of the 2023 CHI Conference on Human Factors in Computing Systems (CHI '23), April 23–28, 2023, Hamburg, Germany (New York, NY: ACM Press), 1–17. doi: 10.1145/3544548.3581001
Kozielski, M. (2023). Contextual explanations for decision support in predictive maintenance. Applied Sci. 13:10068. doi: 10.3390/app131810068
Kumar, P. (2018). Development of Demand Forecast and Inventory Management Decision Support System Using AI Techniques (Doctoral dissertation). National Institute of Technology Karnataka, Surathkal.
Kuniavsky, M. (2003). Contextual Inquiry, Task Analysis, Card Sorting. Observing the User Experience. San Francisco, CA: Morgan Kaufmann, 148–177.
Lai, V., Zhang, Y., Chen, C., Liao, Q., and Tan, C. (2023). Selective explanations: leveraging human input to align explainable AI. Proc. ACM on Human-Comp. Interact. 7, 1–35. doi: 10.1145/3610206
Lantz, E., Keeley, J., Roberts, M., Medina-Mora, M., Sharan, P., and Reed, G. (2019). Card sorting data collection methodology: how many participants is most efficient? J. Classif. 8, 1–10. doi: 10.1007/s00357-018-9292-8
Leng, J., Sha, W., Wang, B., Zheng, P., Zhuang, C., Liu, Q., et al. (2022). Industry 5.0: Prospect and retrospect. J. Manuf. Syst. 65, 279–295. doi: 10.1016/j.jmsy.2022.09.017
Liao, Q. V., and Varshney, K. R. (2021). Human-centered explainable AI (XAI): from algorithms to user experiences. arXiv [preprint] arXiv:2110.10790. doi: 10.48550/arXiv.2110.10790
Lim, B. Y., Dey, A. K., and Avrahami, D. (2009). Why and why not explanations improve the intelligibility of context-aware intelligen systems. In Proceedings of the SIGCHI Conference on Human Factors in Computing Systems (CHI 2009), April 4–9, 2009, Boston, Massachusetts, USA (New York, NY: ACM Press), 2119–2128. doi: 10.1145/1518701.1519023
Lin, C. T., Hung, K. P., and Hu, S. H. (2018). A decision-making model for evaluating and selecting suppliers for the sustainable operation and development of enterprises in the aerospace industry. Sustainability 10:735. doi: 10.3390/su10030735
Longo, L., Brcic, M., Cabitza, F., Choi, J., Confalonieri, R., Del Ser, J., et al. (2024). Explainable Artificial Intelligence (XAI) 2.0: a manifesto of open challenges and interdisciplinary research directions. Inform. Fusion 106:102301. doi: 10.1016/j.inffus.2024.102301
Madhavan, P., and Wiegmann, D. A. (2007). Similarities and differences between human–human and human–automation trust: an integrative review. Theoret. Issues Ergon. Sci. 8, 277–301. doi: 10.1080/14639220500337708
Margetis, G., Ntoa, S., Antona, M., and Stephanidis, C. (2021). “Human-centered design of artificial intelligence,” in Handbook of Human Factors and Ergonomics (5th ed.), eds. G. Salvendy, and W. Karwowski (Hoboken, NJ: John Wiley & Sons), 1085–1106. doi: 10.1002/9781119636113.ch42
Mietkiewicz, J., Abbas, A., Amazu, C., Baldissone, G., Madsen, A., Demichela, M., et al. (2024). Enhancing control room operator decision making. Processes 12:328. doi: 10.3390/pr12020328
Minh, D., Wang, H. X., Li, Y. F., and Nguyen, T. N. (2022). Explainable artificial intelligence: a comprehensive review. Artif. Intellig. Rev. 2022, 1–66. doi: 10.1007/s10462-021-10088-y
MoDastoni, D. A. (2023). Exploring methods to make AI decisions more transparent and understandable for humans. Adv. Eng. Innovat. 3, 32–36. doi: 10.54254/2977-3903/3/2023037
Möller, D. P., Vakilzadian, H., and Haas, R. E. (2022). “From industry 4.0 towards industry 5.0.,” in 2022 IEEE International Conference on Electro Information Technology (EIT) (Mankato, MN: IEEE), 61–68.
Morandini, S., Fraboni, F., Hall, M., Quintana-Amate, S., and Pietrantoni, L. (2024). Human factors and emerging needs in aerospace manufacturing planning and scheduling. Cognit. Technol. Work. doi: 10.1007/s10111-024-00785-3
Morandini, S., Fraboni, F., Puzzo, G., Giusino, D., Volpi, L., Brendel, H., et al. (2023). Examining the Nexus Between Explainability of AI Systems and User's Trust: A Preliminary Scoping Review. Available online at: http://ceur-ws.org (accessed January 12, 2025).
Mourtzis, D., Angelopoulos, J., and Panopoulos, N. (2022). A literature review of the challenges and opportunities of the transition from industry 4.0 to society 5.0. Energies 15:6276. doi: 10.3390/en15176276
Mucha, H., Robert, S., Breitschwerdt, R., and Fellmann, M. (2021). “Interfaces for explanations in human-AI interaction: Proposing a design evaluation approach,” in CHI Conference on Human Factors in Computing Systems Extended Abstracts (CHI '21 Extended Abstracts), May 8–13, 2021, Yokohama, Japan (New York, NY: ACM Press), 1–6. doi: 10.1145/3411763.3451759
Nazemi, K., Secco, C., Sina, L., Eliseeva, U., Correll, E., and Blazevic, M. (2024). “Visual analytics for decision-making,” in Proceedings of the 28th International Conference on Information Visualisation (IV 2024), July 15–18, 2024, Paris, France (Washington, DC: IEEE Computer Society), 150–159. doi: 10.1109/IV.2024.00024
Notta.ai. (n.d.). AI-Powered Transcription and Note-Taking. Available online at: https://www.notta.ai/it (accessed January 12, 2025).
Panigutti, C., Beretta, A., Fadda, D., Giannotti, F., Pedreschi, D., Perotti, A., et al. (2023). Co-design of human-centered, explainable AI for clinical decision support. ACM Trans. Interact. Intellig. Syst. 13, 1–35. doi: 10.1145/3587271
Pieters, W. (2011). Explanation and trust: what to tell the user in security and AI? Ethics Inform. Technol. 13, 53–64. doi: 10.1007/s10676-010-9253-3
Reddy, S. (2024). Human-computer interaction techniques for explainable artificial intelligence systems. Res. Rev. 3, 1–7 doi: 10.46610/RTAIA.2024.v03i01.001
Ridley, M. (2022). Explainable artificial intelligence (XAI): adoption and advocacy. Inform. Technol. Librar. 41:2. doi: 10.6017/ital.v41i2.14683
Rožanec, J. M., Novalija, I., Zajec, P., Kenda, K., Tavakoli Ghinani, H., Suh, S., et al. (2023). Human-centric artificial intelligence architecture for industry 5.0 applications. Int. J. Prod. Res. 61, 6847–6872. doi: 10.1080/00207543.2022.2138611
Saeed, W., and Omlin, C. (2023). Explainable AI (XAI): A systematic meta-survey of current challenges and future opportunities. Knowl.-Based Syst. 263:110273. doi: 10.1016/j.knosys.2023.110273
Salimiparsa, M., Lizotte, D., and Sedig, K. (2021). “A user-centered design of explainable AI for clinical decision support,” in Proceedings of the 34th Canadian Conference on Artificial Intelligence (Canadian AI 2021), May 25–28, 2021, Online Conference (London, ON: University of Western Ontario).
Sammani, F., Joukovsky, B., and Deligiannis, N. (2023). “Visualizing invariant features in vision models,” in 2023 24th International Conference on Digital Signal Processing (DSP) (Rhodes: IEEE), 1–5.
Shakir, M. A., Abass, H. K., Jelwy, O. F., Al-Bayati, H. N. A., Salman, S. M., Mikhav, V., et al. (2024). “Developing interpretable models for complex decision-making,” in 2024 36th Conference of Open Innovations Association (FRUCT) (Lappeenranta: IEEE), 66–75.
Sheth, A., Gaur, M., Roy, K., and Faldu, K. (2021). Knowledge-intensive language understanding for explainable AI. IEEE Intern. Comp. 25, 19–24. doi: 10.1109/MIC.2021.3101919
Shin, D. (2021). The effects of explainability and causability on perception, trust, and acceptance: implications for explainable AI. Int. J. Hum. Comput. Stud. 146:102551. doi: 10.1016/j.ijhcs.2020.102551
Soulami, M., Benchekroun, S., and Galiulina, A. (2024). Exploring how AI adoption in the workplace affects employees: a bibliometric and systematic review. Front. Artif. Intell. 7:1473872. doi: 10.3389/frai.2024.1473872
Srinivasan, S., Hema, D. D., Singaram, B., Praveena, D., Mohan, K. B. K., and Preetha, M. (2024). Decision support system based on industry 5.0 in artificial intelligence. Int. J. Intellig. Syst. Appl. Eng. 12, 172–178.
Taj, I., and Zaman, N. (2022). Towards industrial revolution 5.0 and explainable artificial intelligence: challenges and opportunities. Int. J. Comp. Digit. Syst. 12, 295–320. doi: 10.12785/ijcds/120128
Tharp, K., and Landrum, J. (2017). “Qualtrics advanced survey software tools,” in Indiana University Workshop in Methods. Availabe online at: https://hdl.handle.net/2022/21933
Valentino, M., and Freitas, A. (2022). Scientific explanation and natural language: a unified epistemological-linguistic perspective for explainable AI. arXiv [preprint] arXiv:2205.01809. doi: 10.48550/arXiv.2205.01809
Werz, J. M., Borowski, E., and Isenhardt, I. (2024). Explainability as a means for transparency? Lay users' requirements towards transparent AI. Cognit. Comp. Intern. Things 124:124. doi: 10.54941/ahfe1004712
Xu, W., Dainoff, M. J., Ge, L., and Gao, Z. (2023). Transitioning to human interaction with AI systems: New challenges and opportunities for HCI professionals to enable human-centered AI. Int. J. Human–Comp. Interact. 39, 494–518. doi: 10.1080/10447318.2022.2041900
Xu, X., Lu, Y., Vogel-Heuser, B., and Wang, L. (2021). Industry 4.0 and Industry 5.0—Inception, conception and perception. J. Manufact. Syst. 61, 530–535. doi: 10.1016/j.jmsy.2021.10.006
Keywords: aerospace manufacturing, artificial intelligence, Decision Support Systems, explainability, XAI, Card Sorting, user-centered approach
Citation: Morandini S, Fraboni F, Hall M, Quintana-Amate S and Pietrantoni L (2025) User perspectives on AI explainability in aerospace manufacturing: a Card-Sorting study. Front. Organ. Psychol. 3:1538438. doi: 10.3389/forgp.2025.1538438
Received: 02 December 2024; Accepted: 24 February 2025;
Published: 12 March 2025.
Edited by:
Lara Carminati, University of Twente, NetherlandsReviewed by:
Patrik Pluchino, University of Padua, ItalyCopyright © 2025 Morandini, Fraboni, Hall, Quintana-Amate and Pietrantoni. This is an open-access article distributed under the terms of the Creative Commons Attribution License (CC BY). The use, distribution or reproduction in other forums is permitted, provided the original author(s) and the copyright owner(s) are credited and that the original publication in this journal is cited, in accordance with accepted academic practice. No use, distribution or reproduction is permitted which does not comply with these terms.
*Correspondence: Luca Pietrantoni, bHVjYS5waWV0cmFudG9uaUB1bmliby5pdA==
Disclaimer: All claims expressed in this article are solely those of the authors and do not necessarily represent those of their affiliated organizations, or those of the publisher, the editors and the reviewers. Any product that may be evaluated in this article or claim that may be made by its manufacturer is not guaranteed or endorsed by the publisher.
Research integrity at Frontiers
Learn more about the work of our research integrity team to safeguard the quality of each article we publish.